diff --git a/.pre-commit-config-zh-cn.yaml b/.pre-commit-config-zh-cn.yaml
index 5a1495683..9c4080fae 100644
--- a/.pre-commit-config-zh-cn.yaml
+++ b/.pre-commit-config-zh-cn.yaml
@@ -1,9 +1,10 @@
-exclude: ^tests/data/
+exclude: ^tests/data/|^xtuner/tools/model_converters/modeling_internlm2_reward/|^xtuner/_lite/modelings/|^xtuner/_lite/accelerate/dispatches/huggingface/
repos:
- repo: https://gitee.com/openmmlab/mirrors-flake8
rev: 5.0.4
hooks:
- id: flake8
+ args: ["--exclude=xtuner/model/transformers_models/*"]
- repo: https://gitee.com/openmmlab/mirrors-isort
rev: 5.11.5
hooks:
diff --git a/.pre-commit-config.yaml b/.pre-commit-config.yaml
index acfe43b66..245f17c69 100644
--- a/.pre-commit-config.yaml
+++ b/.pre-commit-config.yaml
@@ -1,9 +1,10 @@
-exclude: ^tests/data/
+exclude: ^tests/data/|^xtuner/tools/model_converters/modeling_internlm2_reward/|^xtuner/_lite/modelings/|^xtuner/_lite/accelerate/dispatches/huggingface/
repos:
- repo: https://github.com/PyCQA/flake8
rev: 5.0.4
hooks:
- id: flake8
+ args: ["--exclude=xtuner/model/transformers_models/*"]
- repo: https://github.com/PyCQA/isort
rev: 5.11.5
hooks:
diff --git a/README.md b/README.md
index 352e7215e..263d300c7 100644
--- a/README.md
+++ b/README.md
@@ -17,13 +17,35 @@
[](https://huggingface.co/xtuner)
[](https://www.modelscope.cn/organization/xtuner)
[](https://openxlab.org.cn/usercenter/xtuner)
+[](https://www.wisemodel.cn/organization/xtuner)
English | [简体中文](README_zh-CN.md)
-## 🎉 News
+## 🚀 Speed Benchmark
+
+- Llama2 7B Training Speed
+
+
+

+
+
+- Llama2 70B Training Speed
+
+
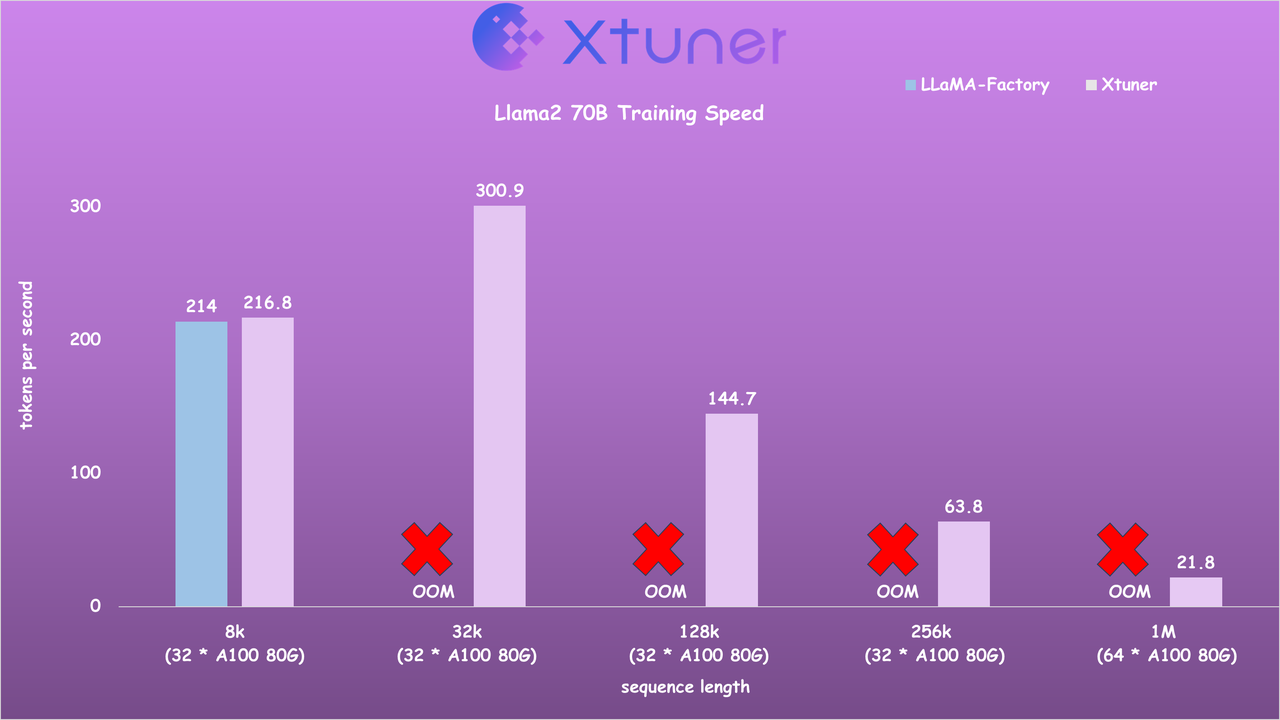
+
+
+## 🎉 News
+- **\[2024/07\]** Support [MiniCPM](xtuner/configs/minicpm/) models!
+- **\[2024/07\]** Support [DPO](https://github.com/InternLM/xtuner/tree/main/xtuner/configs/dpo), [ORPO](https://github.com/InternLM/xtuner/tree/main/xtuner/configs/orpo) and [Reward Model](https://github.com/InternLM/xtuner/tree/main/xtuner/configs/reward_model) training with packed data and sequence parallel! See [documents](https://xtuner.readthedocs.io/en/latest/dpo/overview.html) for more details.
+- **\[2024/07\]** Support [InternLM 2.5](xtuner/configs/internlm/internlm2_5_chat_7b/) models!
+- **\[2024/06\]** Support [DeepSeek V2](xtuner/configs/deepseek/deepseek_v2_chat/) models! **2x faster!**
+- **\[2024/04\]** [LLaVA-Phi-3-mini](https://huggingface.co/xtuner/llava-phi-3-mini-hf) is released! Click [here](xtuner/configs/llava/phi3_mini_4k_instruct_clip_vit_large_p14_336) for details!
+- **\[2024/04\]** [LLaVA-Llama-3-8B](https://huggingface.co/xtuner/llava-llama-3-8b) and [LLaVA-Llama-3-8B-v1.1](https://huggingface.co/xtuner/llava-llama-3-8b-v1_1) are released! Click [here](xtuner/configs/llava/llama3_8b_instruct_clip_vit_large_p14_336) for details!
+- **\[2024/04\]** Support [Llama 3](xtuner/configs/llama) models!
+- **\[2024/04\]** Support Sequence Parallel for enabling highly efficient and scalable LLM training with extremely long sequence lengths! \[[Usage](https://github.com/InternLM/xtuner/blob/docs/docs/zh_cn/acceleration/train_extreme_long_sequence.rst)\] \[[Speed Benchmark](https://github.com/InternLM/xtuner/blob/docs/docs/zh_cn/acceleration/benchmark.rst)\]
- **\[2024/02\]** Support [Gemma](xtuner/configs/gemma) models!
- **\[2024/02\]** Support [Qwen1.5](xtuner/configs/qwen/qwen1_5) models!
- **\[2024/01\]** Support [InternLM2](xtuner/configs/internlm) models! The latest VLM [LLaVA-Internlm2-7B](https://huggingface.co/xtuner/llava-internlm2-7b) / [20B](https://huggingface.co/xtuner/llava-internlm2-20b) models are released, with impressive performance!
@@ -35,7 +57,7 @@ English | [简体中文](README_zh-CN.md)
- **\[2023/10\]** Optimize the data processing to accommodate `system` context. More information can be found on [Docs](docs/en/user_guides/dataset_format.md)!
- **\[2023/09\]** Support [InternLM-20B](xtuner/configs/internlm) models!
- **\[2023/09\]** Support [Baichuan2](xtuner/configs/baichuan) models!
-- **\[2023/08\]** XTuner is released, with multiple fine-tuned adapters on [HuggingFace](https://huggingface.co/xtuner).
+- **\[2023/08\]** XTuner is released, with multiple fine-tuned adapters on [Hugging Face](https://huggingface.co/xtuner).
## 📖 Introduction
@@ -49,7 +71,7 @@ XTuner is an efficient, flexible and full-featured toolkit for fine-tuning large
**Flexible**
-- Support various LLMs ([InternLM](https://huggingface.co/internlm), [Mixtral-8x7B](https://huggingface.co/mistralai), [Llama2](https://huggingface.co/meta-llama), [ChatGLM](https://huggingface.co/THUDM), [Qwen](https://huggingface.co/Qwen), [Baichuan](https://huggingface.co/baichuan-inc), ...).
+- Support various LLMs ([InternLM](https://huggingface.co/internlm), [Mixtral-8x7B](https://huggingface.co/mistralai), [Llama 2](https://huggingface.co/meta-llama), [ChatGLM](https://huggingface.co/THUDM), [Qwen](https://huggingface.co/Qwen), [Baichuan](https://huggingface.co/baichuan-inc), ...).
- Support VLM ([LLaVA](https://github.com/haotian-liu/LLaVA)). The performance of [LLaVA-InternLM2-20B](https://huggingface.co/xtuner/llava-internlm2-20b) is outstanding.
- Well-designed data pipeline, accommodating datasets in any format, including but not limited to open-source and custom formats.
- Support various training algorithms ([QLoRA](http://arxiv.org/abs/2305.14314), [LoRA](http://arxiv.org/abs/2106.09685), full-parameter fune-tune), allowing users to choose the most suitable solution for their requirements.
@@ -60,31 +82,6 @@ XTuner is an efficient, flexible and full-featured toolkit for fine-tuning large
- Support chatting with large models with pre-defined templates.
- The output models can seamlessly integrate with deployment and server toolkit ([LMDeploy](https://github.com/InternLM/lmdeploy)), and large-scale evaluation toolkit ([OpenCompass](https://github.com/open-compass/opencompass), [VLMEvalKit](https://github.com/open-compass/VLMEvalKit)).
-## 🌟 Demos
-
-- Ready-to-use models and datasets from XTuner API [](https://colab.research.google.com/drive/17CSO7T8q6KePuvu684IiHl6_id-CjPjh?usp=sharing)
-
-- QLoRA Fine-tune [](https://colab.research.google.com/drive/1QAEZVBfQ7LZURkMUtaq0b-5nEQII9G9Z?usp=sharing)
-
-- Plugin-based Chat [](https://colab.research.google.com/drive/144OuTVyT_GvFyDMtlSlTzcxYIfnRsklq?usp=sharing)
-
-
-
- Examples of Plugin-based Chat 🔥🔥🔥 |
-
-
-
-
- |
-
-
- |
-
-
- |
-
-
-
## 🔥 Supports
@@ -106,18 +103,17 @@ XTuner is an efficient, flexible and full-featured toolkit for fine-tuning large
|
@@ -150,6 +146,9 @@ XTuner is an efficient, flexible and full-featured toolkit for fine-tuning large
QLoRA
LoRA
Full parameter fine-tune
+ DPO
+ ORPO
+ Reward Model
@@ -187,7 +186,7 @@ XTuner is an efficient, flexible and full-featured toolkit for fine-tuning large
pip install -e '.[all]'
```
-### Fine-tune [](https://colab.research.google.com/drive/1QAEZVBfQ7LZURkMUtaq0b-5nEQII9G9Z?usp=sharing)
+### Fine-tune
XTuner supports the efficient fine-tune (*e.g.*, QLoRA) for LLMs. Dataset prepare guides can be found on [dataset_prepare.md](./docs/en/user_guides/dataset_prepare.md).
@@ -210,27 +209,27 @@ XTuner supports the efficient fine-tune (*e.g.*, QLoRA) for LLMs. Dataset prepar
xtuner train ${CONFIG_NAME_OR_PATH}
```
- For example, we can start the QLoRA fine-tuning of InternLM2-Chat-7B with oasst1 dataset by
+ For example, we can start the QLoRA fine-tuning of InternLM2.5-Chat-7B with oasst1 dataset by
```shell
# On a single GPU
- xtuner train internlm2_chat_7b_qlora_oasst1_e3 --deepspeed deepspeed_zero2
+ xtuner train internlm2_5_chat_7b_qlora_oasst1_e3 --deepspeed deepspeed_zero2
# On multiple GPUs
- (DIST) NPROC_PER_NODE=${GPU_NUM} xtuner train internlm2_chat_7b_qlora_oasst1_e3 --deepspeed deepspeed_zero2
- (SLURM) srun ${SRUN_ARGS} xtuner train internlm2_chat_7b_qlora_oasst1_e3 --launcher slurm --deepspeed deepspeed_zero2
+ (DIST) NPROC_PER_NODE=${GPU_NUM} xtuner train internlm2_5_chat_7b_qlora_oasst1_e3 --deepspeed deepspeed_zero2
+ (SLURM) srun ${SRUN_ARGS} xtuner train internlm2_5_chat_7b_qlora_oasst1_e3 --launcher slurm --deepspeed deepspeed_zero2
```
- `--deepspeed` means using [DeepSpeed](https://github.com/microsoft/DeepSpeed) 🚀 to optimize the training. XTuner comes with several integrated strategies including ZeRO-1, ZeRO-2, and ZeRO-3. If you wish to disable this feature, simply remove this argument.
- For more examples, please see [finetune.md](./docs/en/user_guides/finetune.md).
-- **Step 2**, convert the saved PTH model (if using DeepSpeed, it will be a directory) to HuggingFace model, by
+- **Step 2**, convert the saved PTH model (if using DeepSpeed, it will be a directory) to Hugging Face model, by
```shell
xtuner convert pth_to_hf ${CONFIG_NAME_OR_PATH} ${PTH} ${SAVE_PATH}
```
-### Chat [](https://colab.research.google.com/drive/144OuTVyT_GvFyDMtlSlTzcxYIfnRsklq?usp=sharing)
+### Chat
XTuner provides tools to chat with pretrained / fine-tuned LLMs.
@@ -238,25 +237,17 @@ XTuner provides tools to chat with pretrained / fine-tuned LLMs.
xtuner chat ${NAME_OR_PATH_TO_LLM} --adapter {NAME_OR_PATH_TO_ADAPTER} [optional arguments]
```
-For example, we can start the chat with
-
-InternLM2-Chat-7B with adapter trained from oasst1 dataset:
-
-```shell
-xtuner chat internlm/internlm2-chat-7b --adapter xtuner/internlm2-chat-7b-qlora-oasst1 --prompt-template internlm2_chat
-```
-
-LLaVA-InternLM2-7B:
+For example, we can start the chat with InternLM2.5-Chat-7B :
```shell
-xtuner chat internlm/internlm2-chat-7b --visual-encoder openai/clip-vit-large-patch14-336 --llava xtuner/llava-internlm2-7b --prompt-template internlm2_chat --image $IMAGE_PATH
+xtuner chat internlm/internlm2_5-chat-7b --prompt-template internlm2_chat
```
For more examples, please see [chat.md](./docs/en/user_guides/chat.md).
### Deployment
-- **Step 0**, merge the HuggingFace adapter to pretrained LLM, by
+- **Step 0**, merge the Hugging Face adapter to pretrained LLM, by
```shell
xtuner convert merge \
@@ -290,6 +281,7 @@ We appreciate all contributions to XTuner. Please refer to [CONTRIBUTING.md](.gi
## 🎖️ Acknowledgement
- [Llama 2](https://github.com/facebookresearch/llama)
+- [DeepSpeed](https://github.com/microsoft/DeepSpeed)
- [QLoRA](https://github.com/artidoro/qlora)
- [LMDeploy](https://github.com/InternLM/lmdeploy)
- [LLaVA](https://github.com/haotian-liu/LLaVA)
diff --git a/README_zh-CN.md b/README_zh-CN.md
index c247be985..f4f0b4b48 100644
--- a/README_zh-CN.md
+++ b/README_zh-CN.md
@@ -16,13 +16,36 @@
🔍 探索我们的模型:
[](https://huggingface.co/xtuner)
[](https://www.modelscope.cn/organization/xtuner)
+[](https://openxlab.org.cn/usercenter/xtuner)
+[](https://www.wisemodel.cn/organization/xtuner)
[English](README.md) | 简体中文
-## 🎉 更新
+## 🚀 Speed Benchmark
+
+- XTuner 与 LLaMA-Factory 在 Llama2-7B 模型上的训练效率对比
+
+
+

+
+
+- XTuner 与 LLaMA-Factory 在 Llama2-70B 模型上的训练效率对比
+
+
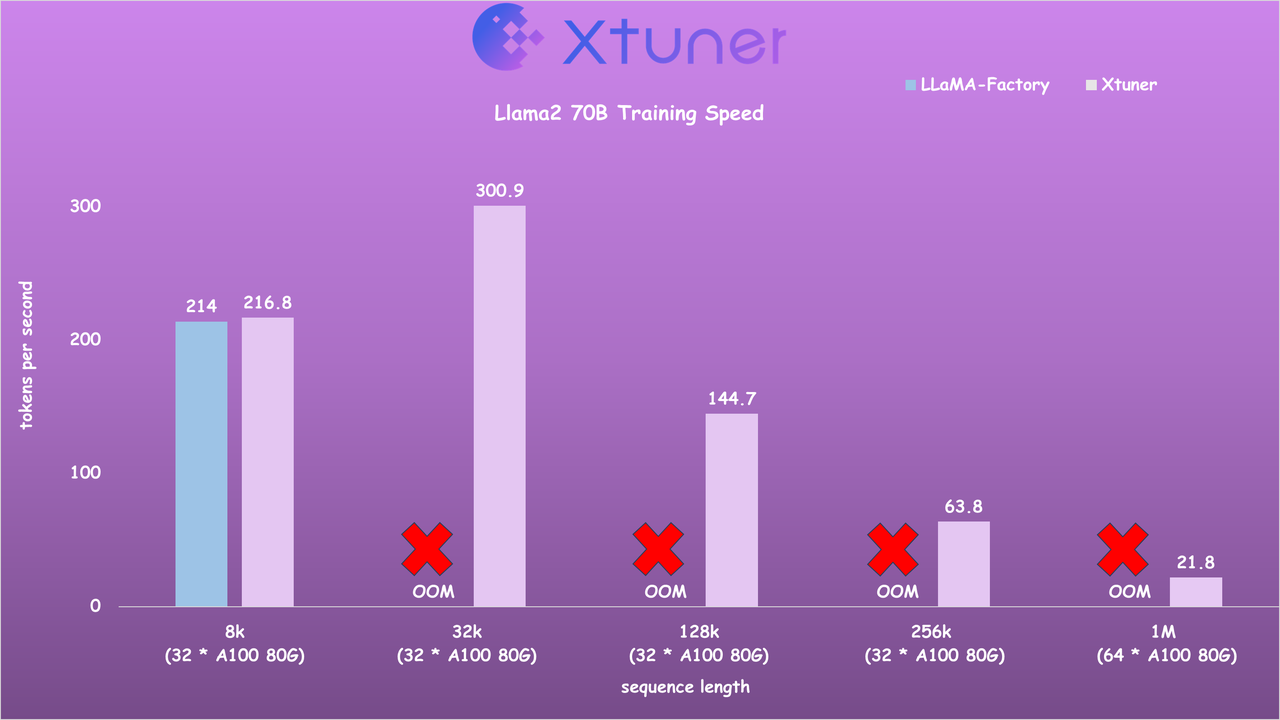
+
+
+## 🎉 更新
+- **\[2024/07\]** 支持 [MiniCPM](xtuner/configs/minicpm/) 模型!
+- **\[2024/07\]** 支持训练 [DPO](https://github.com/InternLM/xtuner/tree/main/xtuner/configs/dpo), [ORPO](https://github.com/InternLM/xtuner/tree/main/xtuner/configs/orpo) 还有 [Reward Model](https://github.com/InternLM/xtuner/tree/main/xtuner/configs/reward_model) ! 并且能够支持打包数据以及序列并行功能! 请参考 [文档](https://xtuner.readthedocs.io/zh-cn/latest/dpo/overview.html) 了解更多信息。
+- **\[2024/07\]** 支持 [InternLM 2.5](xtuner/configs/internlm/internlm2_5_chat_7b/) 模型!
+- **\[2024/06\]** 支持 [DeepSeek V2](xtuner/configs/deepseek/deepseek_v2_chat/) models! **训练速度提升一倍!**
+- **\[2024/04\]** 多模态大模型 [LLaVA-Phi-3-mini](https://huggingface.co/xtuner/llava-phi-3-mini-hf) 发布!快速开始请查阅此[文档](xtuner/configs/llava/phi3_mini_4k_instruct_clip_vit_large_p14_336)!
+- **\[2024/04\]** 多模态大模型 [LLaVA-Llama-3-8B](https://huggingface.co/xtuner/llava-llama-3-8b) 和 [LLaVA-Llama-3-8B-v1.1](https://huggingface.co/xtuner/llava-llama-3-8b-v1_1) 发布!快速开始请查阅此[文档](xtuner/configs/llava/llama3_8b_instruct_clip_vit_large_p14_336)!
+- **\[2024/04\]** 支持 [Llama 3](xtuner/configs/llama) 模型!
+- **\[2024/04\]** 支持序列并行训练策略以实现语言模型超长上下文训练!\[[文档](https://github.com/InternLM/xtuner/blob/docs/docs/zh_cn/acceleration/train_extreme_long_sequence.rst)\] \[[速度基准](https://github.com/InternLM/xtuner/blob/docs/docs/zh_cn/acceleration/benchmark.rst)\]
- **\[2024/02\]** 支持 [Gemma](xtuner/configs/gemma) 模型!
- **\[2024/02\]** 支持 [Qwen1.5](xtuner/configs/qwen/qwen1_5) 模型!
- **\[2024/01\]** 支持 [InternLM2](xtuner/configs/internlm) 模型!同时,最新版的多模态大模型 [LLaVA-Internlm2-7B](https://huggingface.co/xtuner/llava-internlm2-7b) / [20B](https://huggingface.co/xtuner/llava-internlm2-20b) 发布,其表现出强大的性能!
@@ -48,7 +71,7 @@ XTuner 是一个高效、灵活、全能的轻量化大模型微调工具库。
**灵活**
-- 支持多种大语言模型,包括但不限于 [InternLM](https://huggingface.co/internlm)、[Mixtral-8x7B](https://huggingface.co/mistralai)、[Llama2](https://huggingface.co/meta-llama)、[ChatGLM](https://huggingface.co/THUDM)、[Qwen](https://huggingface.co/Qwen)、[Baichuan](https://huggingface.co/baichuan-inc)。
+- 支持多种大语言模型,包括但不限于 [InternLM](https://huggingface.co/internlm)、[Mixtral-8x7B](https://huggingface.co/mistralai)、[Llama 2](https://huggingface.co/meta-llama)、[ChatGLM](https://huggingface.co/THUDM)、[Qwen](https://huggingface.co/Qwen)、[Baichuan](https://huggingface.co/baichuan-inc)。
- 支持多模态图文模型 LLaVA 的预训练与微调。利用 XTuner 训得模型 [LLaVA-InternLM2-20B](https://huggingface.co/xtuner/llava-internlm2-20b) 表现优异。
- 精心设计的数据管道,兼容任意数据格式,开源数据或自定义数据皆可快速上手。
- 支持 [QLoRA](http://arxiv.org/abs/2305.14314)、[LoRA](http://arxiv.org/abs/2106.09685)、全量参数微调等多种微调算法,支撑用户根据具体需求作出最优选择。
@@ -59,31 +82,6 @@ XTuner 是一个高效、灵活、全能的轻量化大模型微调工具库。
- 预定义众多开源对话模版,支持与开源或训练所得模型进行对话。
- 训练所得模型可无缝接入部署工具库 [LMDeploy](https://github.com/InternLM/lmdeploy)、大规模评测工具库 [OpenCompass](https://github.com/open-compass/opencompass) 及 [VLMEvalKit](https://github.com/open-compass/VLMEvalKit)。
-## 🌟 示例
-
-- XTuner APIs所提供的开箱即用的模型与数据集 [](https://colab.research.google.com/drive/17CSO7T8q6KePuvu684IiHl6_id-CjPjh?usp=sharing)
-
-- QLoRA 微调 [](https://colab.research.google.com/drive/1QAEZVBfQ7LZURkMUtaq0b-5nEQII9G9Z?usp=sharing)
-
-- 基于插件的对话 [](https://colab.research.google.com/drive/144OuTVyT_GvFyDMtlSlTzcxYIfnRsklq?usp=sharing)
-
-
-
- 基于插件的对话 🔥🔥🔥 |
-
-
-
-
- |
-
-
- |
-
-
- |
-
-
-
## 🔥 支持列表
@@ -105,18 +103,17 @@ XTuner 是一个高效、灵活、全能的轻量化大模型微调工具库。
|
@@ -149,6 +146,9 @@ XTuner 是一个高效、灵活、全能的轻量化大模型微调工具库。
QLoRA
LoRA
全量参数微调
+ DPO
+ ORPO
+ Reward Model
@@ -186,7 +186,7 @@ XTuner 是一个高效、灵活、全能的轻量化大模型微调工具库。
pip install -e '.[all]'
```
-### 微调 [](https://colab.research.google.com/drive/1QAEZVBfQ7LZURkMUtaq0b-5nEQII9G9Z?usp=sharing)
+### 微调
XTuner 支持微调大语言模型。数据集预处理指南请查阅[文档](./docs/zh_cn/user_guides/dataset_prepare.md)。
@@ -209,14 +209,14 @@ XTuner 支持微调大语言模型。数据集预处理指南请查阅[文档](.
xtuner train ${CONFIG_NAME_OR_PATH}
```
- 例如,我们可以利用 QLoRA 算法在 oasst1 数据集上微调 InternLM2-Chat-7B:
+ 例如,我们可以利用 QLoRA 算法在 oasst1 数据集上微调 InternLM2.5-Chat-7B:
```shell
# 单卡
- xtuner train internlm2_chat_7b_qlora_oasst1_e3 --deepspeed deepspeed_zero2
+ xtuner train internlm2_5_chat_7b_qlora_oasst1_e3 --deepspeed deepspeed_zero2
# 多卡
- (DIST) NPROC_PER_NODE=${GPU_NUM} xtuner train internlm2_chat_7b_qlora_oasst1_e3 --deepspeed deepspeed_zero2
- (SLURM) srun ${SRUN_ARGS} xtuner train internlm2_chat_7b_qlora_oasst1_e3 --launcher slurm --deepspeed deepspeed_zero2
+ (DIST) NPROC_PER_NODE=${GPU_NUM} xtuner train internlm2_5_chat_7b_qlora_oasst1_e3 --deepspeed deepspeed_zero2
+ (SLURM) srun ${SRUN_ARGS} xtuner train internlm2_5_chat_7b_qlora_oasst1_e3 --launcher slurm --deepspeed deepspeed_zero2
```
- `--deepspeed` 表示使用 [DeepSpeed](https://github.com/microsoft/DeepSpeed) 🚀 来优化训练过程。XTuner 内置了多种策略,包括 ZeRO-1、ZeRO-2、ZeRO-3 等。如果用户期望关闭此功能,请直接移除此参数。
@@ -229,7 +229,7 @@ XTuner 支持微调大语言模型。数据集预处理指南请查阅[文档](.
xtuner convert pth_to_hf ${CONFIG_NAME_OR_PATH} ${PTH} ${SAVE_PATH}
```
-### 对话 [](https://colab.research.google.com/drive/144OuTVyT_GvFyDMtlSlTzcxYIfnRsklq?usp=sharing)
+### 对话
XTuner 提供与大语言模型对话的工具。
@@ -239,16 +239,10 @@ xtuner chat ${NAME_OR_PATH_TO_LLM} --adapter {NAME_OR_PATH_TO_ADAPTER} [optional
例如:
-与 InternLM2-Chat-7B, oasst1 adapter 对话:
-
-```shell
-xtuner chat internlm/internlm2-chat-7b --adapter xtuner/internlm2-chat-7b-qlora-oasst1 --prompt-template internlm2_chat
-```
-
-与 LLaVA-InternLM2-7B 对话:
+与 InternLM2.5-Chat-7B 对话:
```shell
-xtuner chat internlm/internlm2-chat-7b --visual-encoder openai/clip-vit-large-patch14-336 --llava xtuner/llava-internlm2-7b --prompt-template internlm2_chat --image $IMAGE_PATH
+xtuner chat internlm/internlm2-chat-7b --prompt-template internlm2_chat
```
更多示例,请查阅[文档](./docs/zh_cn/user_guides/chat.md)。
@@ -289,6 +283,7 @@ xtuner chat internlm/internlm2-chat-7b --visual-encoder openai/clip-vit-large-pa
## 🎖️ 致谢
- [Llama 2](https://github.com/facebookresearch/llama)
+- [DeepSpeed](https://github.com/microsoft/DeepSpeed)
- [QLoRA](https://github.com/artidoro/qlora)
- [LMDeploy](https://github.com/InternLM/lmdeploy)
- [LLaVA](https://github.com/haotian-liu/LLaVA)
diff --git a/docs/en/.readthedocs.yaml b/docs/en/.readthedocs.yaml
new file mode 100644
index 000000000..67b9c44e7
--- /dev/null
+++ b/docs/en/.readthedocs.yaml
@@ -0,0 +1,16 @@
+version: 2
+
+build:
+ os: ubuntu-22.04
+ tools:
+ python: "3.8"
+
+formats:
+ - epub
+
+python:
+ install:
+ - requirements: requirements/docs.txt
+
+sphinx:
+ configuration: docs/en/conf.py
diff --git a/docs/en/Makefile b/docs/en/Makefile
new file mode 100644
index 000000000..d4bb2cbb9
--- /dev/null
+++ b/docs/en/Makefile
@@ -0,0 +1,20 @@
+# Minimal makefile for Sphinx documentation
+#
+
+# You can set these variables from the command line, and also
+# from the environment for the first two.
+SPHINXOPTS ?=
+SPHINXBUILD ?= sphinx-build
+SOURCEDIR = .
+BUILDDIR = _build
+
+# Put it first so that "make" without argument is like "make help".
+help:
+ @$(SPHINXBUILD) -M help "$(SOURCEDIR)" "$(BUILDDIR)" $(SPHINXOPTS) $(O)
+
+.PHONY: help Makefile
+
+# Catch-all target: route all unknown targets to Sphinx using the new
+# "make mode" option. $(O) is meant as a shortcut for $(SPHINXOPTS).
+%: Makefile
+ @$(SPHINXBUILD) -M $@ "$(SOURCEDIR)" "$(BUILDDIR)" $(SPHINXOPTS) $(O)
diff --git a/docs/en/_static/css/readthedocs.css b/docs/en/_static/css/readthedocs.css
new file mode 100644
index 000000000..34ed824ba
--- /dev/null
+++ b/docs/en/_static/css/readthedocs.css
@@ -0,0 +1,6 @@
+.header-logo {
+ background-image: url("../image/logo.png");
+ background-size: 177px 40px;
+ height: 40px;
+ width: 177px;
+}
diff --git a/docs/en/_static/image/logo.png b/docs/en/_static/image/logo.png
new file mode 100644
index 000000000..0d6b754c9
Binary files /dev/null and b/docs/en/_static/image/logo.png differ
diff --git a/docs/en/acceleration/benchmark.rst b/docs/en/acceleration/benchmark.rst
new file mode 100644
index 000000000..813fc7d5a
--- /dev/null
+++ b/docs/en/acceleration/benchmark.rst
@@ -0,0 +1,2 @@
+Benchmark
+=========
diff --git a/docs/en/acceleration/deepspeed.rst b/docs/en/acceleration/deepspeed.rst
new file mode 100644
index 000000000..e3dcaccc0
--- /dev/null
+++ b/docs/en/acceleration/deepspeed.rst
@@ -0,0 +1,2 @@
+DeepSpeed
+=========
diff --git a/docs/en/acceleration/flash_attn.rst b/docs/en/acceleration/flash_attn.rst
new file mode 100644
index 000000000..a080373ef
--- /dev/null
+++ b/docs/en/acceleration/flash_attn.rst
@@ -0,0 +1,2 @@
+Flash Attention
+===============
diff --git a/docs/en/acceleration/hyper_parameters.rst b/docs/en/acceleration/hyper_parameters.rst
new file mode 100644
index 000000000..04b82b7e6
--- /dev/null
+++ b/docs/en/acceleration/hyper_parameters.rst
@@ -0,0 +1,2 @@
+HyperParameters
+===============
diff --git a/docs/en/acceleration/length_grouped_sampler.rst b/docs/en/acceleration/length_grouped_sampler.rst
new file mode 100644
index 000000000..2fc723212
--- /dev/null
+++ b/docs/en/acceleration/length_grouped_sampler.rst
@@ -0,0 +1,2 @@
+Length Grouped Sampler
+======================
diff --git a/docs/en/acceleration/pack_to_max_length.rst b/docs/en/acceleration/pack_to_max_length.rst
new file mode 100644
index 000000000..aaddd36aa
--- /dev/null
+++ b/docs/en/acceleration/pack_to_max_length.rst
@@ -0,0 +1,2 @@
+Pack to Max Length
+==================
diff --git a/docs/en/acceleration/train_extreme_long_sequence.rst b/docs/en/acceleration/train_extreme_long_sequence.rst
new file mode 100644
index 000000000..d326bd690
--- /dev/null
+++ b/docs/en/acceleration/train_extreme_long_sequence.rst
@@ -0,0 +1,2 @@
+Train Extreme Long Sequence
+===========================
diff --git a/docs/en/acceleration/train_large_scale_dataset.rst b/docs/en/acceleration/train_large_scale_dataset.rst
new file mode 100644
index 000000000..026ce9dae
--- /dev/null
+++ b/docs/en/acceleration/train_large_scale_dataset.rst
@@ -0,0 +1,2 @@
+Train Large-scale Dataset
+=========================
diff --git a/docs/en/acceleration/varlen_flash_attn.rst b/docs/en/acceleration/varlen_flash_attn.rst
new file mode 100644
index 000000000..2fad725f3
--- /dev/null
+++ b/docs/en/acceleration/varlen_flash_attn.rst
@@ -0,0 +1,2 @@
+Varlen Flash Attention
+======================
diff --git a/docs/en/chat/agent.md b/docs/en/chat/agent.md
new file mode 100644
index 000000000..1da3ebc10
--- /dev/null
+++ b/docs/en/chat/agent.md
@@ -0,0 +1 @@
+# Chat with Agent
diff --git a/docs/en/chat/llm.md b/docs/en/chat/llm.md
new file mode 100644
index 000000000..5c556180c
--- /dev/null
+++ b/docs/en/chat/llm.md
@@ -0,0 +1 @@
+# Chat with LLM
diff --git a/docs/en/chat/lmdeploy.md b/docs/en/chat/lmdeploy.md
new file mode 100644
index 000000000..f4114a3a5
--- /dev/null
+++ b/docs/en/chat/lmdeploy.md
@@ -0,0 +1 @@
+# Accelerate chat by LMDeploy
diff --git a/docs/en/chat/vlm.md b/docs/en/chat/vlm.md
new file mode 100644
index 000000000..54101dcbc
--- /dev/null
+++ b/docs/en/chat/vlm.md
@@ -0,0 +1 @@
+# Chat with VLM
diff --git a/docs/en/conf.py b/docs/en/conf.py
new file mode 100644
index 000000000..457ca5232
--- /dev/null
+++ b/docs/en/conf.py
@@ -0,0 +1,109 @@
+# Configuration file for the Sphinx documentation builder.
+#
+# This file only contains a selection of the most common options. For a full
+# list see the documentation:
+# https://www.sphinx-doc.org/en/master/usage/configuration.html
+
+# -- Path setup --------------------------------------------------------------
+
+# If extensions (or modules to document with autodoc) are in another directory,
+# add these directories to sys.path here. If the directory is relative to the
+# documentation root, use os.path.abspath to make it absolute, like shown here.
+
+import os
+import sys
+
+from sphinx.ext import autodoc
+
+sys.path.insert(0, os.path.abspath('../..'))
+
+# -- Project information -----------------------------------------------------
+
+project = 'XTuner'
+copyright = '2024, XTuner Contributors'
+author = 'XTuner Contributors'
+
+# The full version, including alpha/beta/rc tags
+version_file = '../../xtuner/version.py'
+with open(version_file) as f:
+ exec(compile(f.read(), version_file, 'exec'))
+__version__ = locals()['__version__']
+# The short X.Y version
+version = __version__
+# The full version, including alpha/beta/rc tags
+release = __version__
+
+# -- General configuration ---------------------------------------------------
+
+# Add any Sphinx extension module names here, as strings. They can be
+# extensions coming with Sphinx (named 'sphinx.ext.*') or your custom
+# ones.
+extensions = [
+ 'sphinx.ext.napoleon',
+ 'sphinx.ext.viewcode',
+ 'sphinx.ext.intersphinx',
+ 'sphinx_copybutton',
+ 'sphinx.ext.autodoc',
+ 'sphinx.ext.autosummary',
+ 'myst_parser',
+ 'sphinxarg.ext',
+]
+
+# Add any paths that contain templates here, relative to this directory.
+templates_path = ['_templates']
+
+# List of patterns, relative to source directory, that match files and
+# directories to ignore when looking for source files.
+# This pattern also affects html_static_path and html_extra_path.
+exclude_patterns = ['_build', 'Thumbs.db', '.DS_Store']
+
+# Exclude the prompt "$" when copying code
+copybutton_prompt_text = r'\$ '
+copybutton_prompt_is_regexp = True
+
+language = 'en'
+
+# -- Options for HTML output -------------------------------------------------
+
+# The theme to use for HTML and HTML Help pages. See the documentation for
+# a list of builtin themes.
+#
+html_theme = 'sphinx_book_theme'
+html_logo = '_static/image/logo.png'
+html_theme_options = {
+ 'path_to_docs': 'docs/en',
+ 'repository_url': 'https://github.com/InternLM/xtuner',
+ 'use_repository_button': True,
+}
+# Add any paths that contain custom static files (such as style sheets) here,
+# relative to this directory. They are copied after the builtin static files,
+# so a file named "default.css" will overwrite the builtin "default.css".
+# html_static_path = ['_static']
+
+# Mock out external dependencies here.
+autodoc_mock_imports = [
+ 'cpuinfo',
+ 'torch',
+ 'transformers',
+ 'psutil',
+ 'prometheus_client',
+ 'sentencepiece',
+ 'vllm.cuda_utils',
+ 'vllm._C',
+ 'numpy',
+ 'tqdm',
+]
+
+
+class MockedClassDocumenter(autodoc.ClassDocumenter):
+ """Remove note about base class when a class is derived from object."""
+
+ def add_line(self, line: str, source: str, *lineno: int) -> None:
+ if line == ' Bases: :py:class:`object`':
+ return
+ super().add_line(line, source, *lineno)
+
+
+autodoc.ClassDocumenter = MockedClassDocumenter
+
+navigation_with_keys = False
diff --git a/docs/en/dpo/modify_settings.md b/docs/en/dpo/modify_settings.md
new file mode 100644
index 000000000..d78cc40e6
--- /dev/null
+++ b/docs/en/dpo/modify_settings.md
@@ -0,0 +1,83 @@
+## Modify DPO Training Configuration
+
+This section introduces config parameters related to DPO (Direct Preference Optimization) training. For more details on XTuner config files, please refer to [Modifying Training Configuration](https://xtuner.readthedocs.io/zh-cn/latest/training/modify_settings.html).
+
+### Loss Function
+
+In DPO training, you can choose different types of loss functions according to your needs. XTuner provides various loss function options, such as `sigmoid`, `hinge`, `ipo`, etc. You can select the desired loss function type by setting the `dpo_loss_type` parameter.
+
+Additionally, you can control the temperature coefficient in the loss function by adjusting the `loss_beta` parameter. The `label_smoothing` parameter can be used for smoothing labels.
+
+```python
+#######################################################################
+# PART 1 Settings #
+#######################################################################
+# Model
+dpo_loss_type = 'sigmoid' # One of ['sigmoid', 'hinge', 'ipo', 'kto_pair', 'sppo_hard', 'nca_pair', 'robust']
+loss_beta = 0.1
+label_smoothing = 0.0
+```
+
+### Modifying the Model
+
+Users can modify `pretrained_model_name_or_path` to change the pretrained model.
+
+```python
+#######################################################################
+# PART 1 Settings #
+#######################################################################
+# Model
+pretrained_model_name_or_path = 'internlm/internlm2-chat-1_8b-sft'
+```
+
+### Training Data
+
+In DPO training, you can specify the maximum number of tokens for a single sample sequence using the `max_length` parameter. XTuner will automatically truncate or pad the data.
+
+```python
+# Data
+max_length = 2048
+```
+
+In the configuration file, we use the `train_dataset` field to specify the training dataset. You can specify the dataset loading method using the `dataset` field and the dataset mapping function using the `dataset_map_fn` field.
+
+```python
+#######################################################################
+# PART 3 Dataset & Dataloader #
+#######################################################################
+sampler = SequenceParallelSampler \
+ if sequence_parallel_size > 1 else DefaultSampler
+
+train_dataset = dict(
+ type=build_preference_dataset,
+ dataset=dict(type=load_dataset, path='mlabonne/orpo-dpo-mix-40k'),
+ tokenizer=tokenizer,
+ max_length=max_length,
+ dataset_map_fn=orpo_dpo_mix_40k_map_fn,
+ is_dpo=True,
+ is_reward=False,
+ reward_token_id=-1,
+ num_proc=32,
+ use_varlen_attn=use_varlen_attn,
+ max_packed_length=max_packed_length,
+ shuffle_before_pack=True,
+)
+
+train_dataloader = dict(
+ batch_size=batch_size,
+ num_workers=dataloader_num_workers,
+ dataset=train_dataset,
+ sampler=dict(type=sampler, shuffle=True),
+ collate_fn=dict(
+ type=preference_collate_fn, use_varlen_attn=use_varlen_attn))
+```
+
+In the above configuration, we use `load_dataset` to load the `mlabonne/orpo-dpo-mix-40k` dataset from Hugging Face and use `orpo_dpo_mix_40k_map_fn` as the dataset mapping function.
+
+For more information on handling datasets and writing dataset mapping functions, please refer to the [Preference Dataset Section](../reward_model/preference_data.md).
+
+### Accelerating Training
+
+When training with preference data, we recommend enabling the [Variable-Length Attention Mechanism](https://xtuner.readthedocs.io/zh-cn/latest/acceleration/varlen_flash_attn.html) to avoid memory waste caused by length differences between chosen and rejected samples within a single preference. You can enable the variable-length attention mechanism by setting `use_varlen_attn=True`.
+
+XTuner also supports many training acceleration methods. For details on how to use them, please refer to the [Acceleration Strategies Section](https://xtuner.readthedocs.io/zh-cn/latest/acceleration/hyper_parameters.html).
diff --git a/docs/en/dpo/overview.md b/docs/en/dpo/overview.md
new file mode 100644
index 000000000..0c20946e3
--- /dev/null
+++ b/docs/en/dpo/overview.md
@@ -0,0 +1,27 @@
+## Introduction to DPO
+
+### Overview
+
+DPO (Direct Preference Optimization) is a method used in large language model training for directly optimizing human preferences. Unlike traditional reinforcement learning methods, DPO directly uses human preference data to optimize the model, thereby improving the quality of generated content to better align with human preferences. DPO also eliminates the need to train a Reward Model and a Critic Model, avoiding the complexity of reinforcement learning algorithms, reducing training overhead, and enhancing training efficiency.
+
+Many algorithms have made certain improvements to DPO's loss function. In XTuner, besides DPO, we have also implemented loss functions from papers such as [Identity Preference Optimization (IPO)](https://huggingface.co/papers/2310.12036). To use these algorithms, please refer to the [Modify DPO Settings](./modify_settings.md) section. We also provide some [example configurations](https://github.com/InternLM/xtuner/tree/main/xtuner/configs/dpo) for reference.
+
+In addition to DPO, there are alignment algorithms like [ORPO](https://arxiv.org/abs/2403.07691) that do not require a reference model. ORPO uses the concept of odds ratio to optimize the model by penalizing rejected samples during the training process, thereby adapting more effectively to the chosen samples. ORPO eliminates the dependence on a reference model, making the training process more simplified and efficient. The training method for ORPO in XTuner is very similar to DPO, and we provide some [example configurations](https://github.com/InternLM/xtuner/tree/main/xtuner/configs/orpo). Users can refer to the DPO tutorial to modify the configuration.
+
+### Features of DPO Training in XTuner
+
+DPO training in XTuner offers the following significant advantages:
+
+1. **Latest Algorithms**: In addition to supporting standard DPO, XTuner also supports improved DPO algorithms or memory efficient algorithms like ORPO that do not rely on reference models.
+
+2. **Reducing Memory Waste**: Due to the length differences in chosen and rejected data in preference datasets, padding tokens during data concatenation can cause memory waste. In XTuner, by utilizing the variable-length attention feature from Flash Attention2, preference pairs are packed into the same sequence during training, significantly reducing memory waste caused by padding tokens. This not only improves memory efficiency but also allows for training larger models or handling more data under the same hardware conditions.
+
+ 
+
+3. **Efficient Training**: Leveraging XTuner's QLoRA training capabilities, the reference model can be converted into a policy model with the LoRA adapter removed, eliminating the memory overhead of the reference model weights and significantly reducing DPO training costs.
+
+4. **Long Text Training**: With XTuner's sequence parallel functionality, long text data can be trained efficiently.
+
+### Getting Started
+
+Refer to the [Quick Start Guide](./quick_start.md) to understand the basic concepts. For more information on configuring training parameters, please see the [Modify DPO Settings](./modify_settings.md) section.
diff --git a/docs/en/dpo/quick_start.md b/docs/en/dpo/quick_start.md
new file mode 100644
index 000000000..19fffbf8b
--- /dev/null
+++ b/docs/en/dpo/quick_start.md
@@ -0,0 +1,71 @@
+## Quick Start with DPO
+
+In this section, we will introduce how to use XTuner to train a 1.8B DPO (Direct Preference Optimization) model to help you get started quickly.
+
+### Preparing Pretrained Model Weights
+
+We use the model [InternLM2-chat-1.8b-sft](https://huggingface.co/internlm/internlm2-chat-1_8b-sft), as the initial model for DPO training to align human preferences.
+
+Set `pretrained_model_name_or_path = 'internlm/internlm2-chat-1_8b-sft'` in the training configuration file, and the model files will be automatically downloaded when training starts. If you need to download the model weights manually, please refer to the section [Preparing Pretrained Model Weights](https://xtuner.readthedocs.io/zh-cn/latest/preparation/pretrained_model.html), which provides detailed instructions on how to download model weights from Huggingface or Modelscope. Here are the links to the models on HuggingFace and ModelScope:
+
+- HuggingFace link: https://huggingface.co/internlm/internlm2-chat-1_8b-sft
+- ModelScope link: https://modelscope.cn/models/Shanghai_AI_Laboratory/internlm2-chat-1_8b-sft/summary
+
+### Preparing Training Data
+
+In this tutorial, we use the [mlabonne/orpo-dpo-mix-40k](https://huggingface.co/datasets/mlabonne/orpo-dpo-mix-40k) dataset from Huggingface as an example.
+
+```python
+train_dataset = dict(
+ type=build_preference_dataset,
+ dataset=dict(
+ type=load_dataset,
+ path='mlabonne/orpo-dpo-mix-40k'),
+ dataset_map_fn=orpo_dpo_mix_40k_map_fn,
+ is_dpo=True,
+ is_reward=False,
+)
+```
+
+Using the above configuration in the configuration file will automatically download and process this dataset. If you want to use other open-source datasets from Huggingface or custom datasets, please refer to the [Preference Dataset](../reward_model/preference_data.md) section.
+
+### Preparing Configuration File
+
+XTuner provides several ready-to-use configuration files, which can be viewed using `xtuner list-cfg`. Execute the following command to copy a configuration file to the current directory.
+
+```bash
+xtuner copy-cfg internlm2_chat_1_8b_dpo_full .
+```
+
+Open the copied configuration file. If you choose to download the model and dataset automatically, no modifications are needed. If you want to specify paths to your pre-downloaded model and dataset, modify the `pretrained_model_name_or_path` and the `path` parameter in `dataset` under `train_dataset`.
+
+For more training parameter configurations, please refer to the section [Modifying DPO Training Configuration](./modify_settings.md) section.
+
+### Starting the Training
+
+After completing the above steps, you can start the training task using the following commands.
+
+```bash
+# Single machine, single GPU
+xtuner train ./internlm2_chat_1_8b_dpo_full_copy.py
+# Single machine, multiple GPUs
+NPROC_PER_NODE=${GPU_NUM} xtuner train ./internlm2_chat_1_8b_dpo_full_copy.py
+# Slurm cluster
+srun ${SRUN_ARGS} xtuner train ./internlm2_chat_1_8b_dpo_full_copy.py --launcher slurm
+```
+
+### Model Conversion
+
+XTuner provides integrated tools to convert models to HuggingFace format. Simply execute the following commands:
+
+```bash
+# Create a directory for HuggingFace format parameters
+mkdir work_dirs/internlm2_chat_1_8b_dpo_full_copy/iter_15230_hf
+
+# Convert format
+xtuner convert pth_to_hf internlm2_chat_1_8b_dpo_full_copy.py \
+ work_dirs/internlm2_chat_1_8b_dpo_full_copy/iter_15230.pth \
+ work_dirs/internlm2_chat_1_8b_dpo_full_copy/iter_15230_hf
+```
+
+This will convert the XTuner's ckpt to the HuggingFace format.
diff --git a/docs/en/evaluation/hook.md b/docs/en/evaluation/hook.md
new file mode 100644
index 000000000..de9e98c88
--- /dev/null
+++ b/docs/en/evaluation/hook.md
@@ -0,0 +1 @@
+# Evaluation during training
diff --git a/docs/en/evaluation/mmbench.md b/docs/en/evaluation/mmbench.md
new file mode 100644
index 000000000..5421b1c96
--- /dev/null
+++ b/docs/en/evaluation/mmbench.md
@@ -0,0 +1 @@
+# MMBench (VLM)
diff --git a/docs/en/evaluation/mmlu.md b/docs/en/evaluation/mmlu.md
new file mode 100644
index 000000000..4bfabff8f
--- /dev/null
+++ b/docs/en/evaluation/mmlu.md
@@ -0,0 +1 @@
+# MMLU (LLM)
diff --git a/docs/en/evaluation/opencompass.md b/docs/en/evaluation/opencompass.md
new file mode 100644
index 000000000..eb24da882
--- /dev/null
+++ b/docs/en/evaluation/opencompass.md
@@ -0,0 +1 @@
+# Evaluate with OpenCompass
diff --git a/docs/en/get_started/installation.md b/docs/en/get_started/installation.md
new file mode 100644
index 000000000..007e61553
--- /dev/null
+++ b/docs/en/get_started/installation.md
@@ -0,0 +1,52 @@
+### Installation
+
+In this section, we will show you how to install XTuner.
+
+## Installation Process
+
+We recommend users to follow our best practices for installing XTuner.
+It is recommended to use a conda virtual environment with Python-3.10 to install XTuner.
+
+### Best Practices
+
+**Step 0.** Create a Python-3.10 virtual environment using conda.
+
+```shell
+conda create --name xtuner-env python=3.10 -y
+conda activate xtuner-env
+```
+
+**Step 1.** Install XTuner.
+
+Case a: Install XTuner via pip:
+
+```shell
+pip install -U xtuner
+```
+
+Case b: Install XTuner with DeepSpeed integration:
+
+```shell
+pip install -U 'xtuner[deepspeed]'
+```
+
+Case c: Install XTuner from the source code:
+
+```shell
+git clone https://github.com/InternLM/xtuner.git
+cd xtuner
+pip install -e '.[all]'
+# "-e" indicates installing the project in editable mode, so any local modifications to the code will take effect without reinstalling.
+```
+
+## Verify the installation
+
+To verify if XTuner is installed correctly, we will use a command to print the configuration files.
+
+**Print Configuration Files:** Use the command `xtuner list-cfg` in the command line to verify if the configuration files can be printed.
+
+```shell
+xtuner list-cfg
+```
+
+You should see a list of XTuner configuration files, corresponding to the ones in [xtuner/configs](https://github.com/InternLM/xtuner/tree/main/xtuner/configs) in the source code.
diff --git a/docs/en/get_started/overview.md b/docs/en/get_started/overview.md
new file mode 100644
index 000000000..c257c83c6
--- /dev/null
+++ b/docs/en/get_started/overview.md
@@ -0,0 +1,5 @@
+# Overview
+
+This chapter introduces you to the framework and workflow of XTuner, and provides detailed tutorial links.
+
+## What is XTuner
diff --git a/docs/en/get_started/quickstart.md b/docs/en/get_started/quickstart.md
new file mode 100644
index 000000000..23198bf3b
--- /dev/null
+++ b/docs/en/get_started/quickstart.md
@@ -0,0 +1,308 @@
+# Quickstart
+
+In this section, we will show you how to use XTuner to fine-tune a model to help you get started quickly.
+
+After installing XTuner successfully, we can start fine-tuning the model. In this section, we will demonstrate how to use XTuner to apply the QLoRA algorithm to fine-tune InternLM2-Chat-7B on the Colorist dataset.
+
+The Colorist dataset ([HuggingFace link](https://huggingface.co/datasets/burkelibbey/colors); [ModelScope link](https://www.modelscope.cn/datasets/fanqiNO1/colors/summary)) is a dataset that provides color choices and suggestions based on color descriptions. A model fine-tuned on this dataset can be used to give a hexadecimal color code based on the user's description of the color. For example, when the user enters "a calming but fairly bright light sky blue, between sky blue and baby blue, with a hint of fluorescence due to its brightness", the model will output , which matches the user's description. There are a few sample data from this dataset:
+
+| Enligsh Description | Chinese Description | Color |
+| -------------------------------------------------------------------------------------------------------------------------------------------------------------------------------------------------------------------------------- | -------------------------------------------------------------------------------------------------------------------------------- | ------------------------------------------------------------------ |
+| Light Sky Blue: A calming, fairly bright color that falls between sky blue and baby blue, with a hint of slight fluorescence due to its brightness. | 浅天蓝色:一种介于天蓝和婴儿蓝之间的平和、相当明亮的颜色,由于明亮而带有一丝轻微的荧光。 | #66ccff:  |
+| Bright red: This is a very vibrant, saturated and vivid shade of red, resembling the color of ripe apples or fresh blood. It is as red as you can get on a standard RGB color palette, with no elements of either blue or green. | 鲜红色: 这是一种非常鲜艳、饱和、生动的红色,类似成熟苹果或新鲜血液的颜色。它是标准 RGB 调色板上的红色,不含任何蓝色或绿色元素。 | #ee0000:  |
+| Bright Turquoise: This color mixes the freshness of bright green with the tranquility of light blue, leading to a vibrant shade of turquoise. It is reminiscent of tropical waters. | 明亮的绿松石色:这种颜色融合了鲜绿色的清新和淡蓝色的宁静,呈现出一种充满活力的绿松石色调。它让人联想到热带水域。 | #00ffcc:  |
+
+## Prepare the model weights
+
+Before fine-tuning the model, we first need to prepare the weights of the model.
+
+### Download from HuggingFace
+
+```bash
+pip install -U huggingface_hub
+
+# Download the model weights to Shanghai_AI_Laboratory/internlm2-chat-7b
+huggingface-cli download internlm/internlm2-chat-7b \
+ --local-dir Shanghai_AI_Laboratory/internlm2-chat-7b \
+ --local-dir-use-symlinks False \
+ --resume-download
+```
+
+### Download from ModelScope
+
+Since pulling model weights from HuggingFace may lead to an unstable download process, slow download speed and other problems, we can choose to download the weights of InternLM2-Chat-7B from ModelScope when experiencing network issues.
+
+```bash
+pip install -U modelscope
+
+# Download the model weights to the current directory
+python -c "from modelscope import snapshot_download; snapshot_download('Shanghai_AI_Laboratory/internlm2-chat-7b', cache_dir='.')"
+```
+
+After completing the download, we can start to prepare the dataset for fine-tuning.
+
+The HuggingFace link and ModelScope link are attached here:
+
+- The HuggingFace link is located at: https://huggingface.co/internlm/internlm2-chat-7b
+- The ModelScope link is located at: https://modelscope.cn/models/Shanghai_AI_Laboratory/internlm2-chat-7b/summary
+
+## Prepare the fine-tuning dataset
+
+### Download from HuggingFace
+
+```bash
+git clone https://huggingface.co/datasets/burkelibbey/colors
+```
+
+### Download from ModelScope
+
+Due to the same reason, we can choose to download the dataset from ModelScope.
+
+```bash
+git clone https://www.modelscope.cn/datasets/fanqiNO1/colors.git
+```
+
+The HuggingFace link and ModelScope link are attached here:
+
+- The HuggingFace link is located at: https://huggingface.co/datasets/burkelibbey/colors
+- The ModelScope link is located at: https://modelscope.cn/datasets/fanqiNO1/colors
+
+## Prepare the config
+
+XTuner provides several configs out-of-the-box, which can be viewed via `xtuner list-cfg`. We can use the following command to copy a config to the current directory.
+
+```bash
+xtuner copy-cfg internlm2_7b_qlora_colorist_e5 .
+```
+
+Explanation of the config name:
+
+| Config Name | internlm2_7b_qlora_colorist_e5 |
+| ----------- | ------------------------------ |
+| Model Name | internlm2_7b |
+| Algorithm | qlora |
+| Dataset | colorist |
+| Epochs | 5 |
+
+The directory structure at this point should look like this:
+
+```bash
+.
+├── colors
+│ ├── colors.json
+│ ├── dataset_infos.json
+│ ├── README.md
+│ └── train.jsonl
+├── internlm2_7b_qlora_colorist_e5_copy.py
+└── Shanghai_AI_Laboratory
+ └── internlm2-chat-7b
+ ├── config.json
+ ├── configuration_internlm2.py
+ ├── configuration.json
+ ├── generation_config.json
+ ├── modeling_internlm2.py
+ ├── pytorch_model-00001-of-00008.bin
+ ├── pytorch_model-00002-of-00008.bin
+ ├── pytorch_model-00003-of-00008.bin
+ ├── pytorch_model-00004-of-00008.bin
+ ├── pytorch_model-00005-of-00008.bin
+ ├── pytorch_model-00006-of-00008.bin
+ ├── pytorch_model-00007-of-00008.bin
+ ├── pytorch_model-00008-of-00008.bin
+ ├── pytorch_model.bin.index.json
+ ├── README.md
+ ├── special_tokens_map.json
+ ├── tokenization_internlm2_fast.py
+ ├── tokenization_internlm2.py
+ ├── tokenizer_config.json
+ └── tokenizer.model
+```
+
+## Modify the config
+
+In this step, we need to modify the model path and dataset path to local paths and modify the dataset loading method.
+In addition, since the copied config is based on the Base model, we also need to modify the `prompt_template` to adapt to the Chat model.
+
+```diff
+#######################################################################
+# PART 1 Settings #
+#######################################################################
+# Model
+- pretrained_model_name_or_path = 'internlm/internlm2-7b'
++ pretrained_model_name_or_path = './Shanghai_AI_Laboratory/internlm2-chat-7b'
+
+# Data
+- data_path = 'burkelibbey/colors'
++ data_path = './colors/train.jsonl'
+- prompt_template = PROMPT_TEMPLATE.default
++ prompt_template = PROMPT_TEMPLATE.internlm2_chat
+
+...
+#######################################################################
+# PART 3 Dataset & Dataloader #
+#######################################################################
+train_dataset = dict(
+ type=process_hf_dataset,
+- dataset=dict(type=load_dataset, path=data_path),
++ dataset=dict(type=load_dataset, path='json', data_files=dict(train=data_path)),
+ tokenizer=tokenizer,
+ max_length=max_length,
+ dataset_map_fn=colors_map_fn,
+ template_map_fn=dict(
+ type=template_map_fn_factory, template=prompt_template),
+ remove_unused_columns=True,
+ shuffle_before_pack=True,
+ pack_to_max_length=pack_to_max_length)
+```
+
+Therefore, `pretrained_model_name_or_path`, `data_path`, `prompt_template`, and the `dataset` fields in `train_dataset` are modified.
+
+## Start fine-tuning
+
+Once having done the above steps, we can start fine-tuning using the following command.
+
+```bash
+# Single GPU
+xtuner train ./internlm2_7b_qlora_colorist_e5_copy.py
+# Multiple GPUs
+NPROC_PER_NODE=${GPU_NUM} xtuner train ./internlm2_7b_qlora_colorist_e5_copy.py
+# Slurm
+srun ${SRUN_ARGS} xtuner train ./internlm2_7b_qlora_colorist_e5_copy.py --launcher slurm
+```
+
+The correct training log may look similar to the one shown below:
+
+```text
+01/29 21:35:34 - mmengine - INFO - Iter(train) [ 10/720] lr: 9.0001e-05 eta: 0:31:46 time: 2.6851 data_time: 0.0077 memory: 12762 loss: 2.6900
+01/29 21:36:02 - mmengine - INFO - Iter(train) [ 20/720] lr: 1.9000e-04 eta: 0:32:01 time: 2.8037 data_time: 0.0071 memory: 13969 loss: 2.6049 grad_norm: 0.9361
+01/29 21:36:29 - mmengine - INFO - Iter(train) [ 30/720] lr: 1.9994e-04 eta: 0:31:24 time: 2.7031 data_time: 0.0070 memory: 13969 loss: 2.5795 grad_norm: 0.9361
+01/29 21:36:57 - mmengine - INFO - Iter(train) [ 40/720] lr: 1.9969e-04 eta: 0:30:55 time: 2.7247 data_time: 0.0069 memory: 13969 loss: 2.3352 grad_norm: 0.8482
+01/29 21:37:24 - mmengine - INFO - Iter(train) [ 50/720] lr: 1.9925e-04 eta: 0:30:28 time: 2.7286 data_time: 0.0068 memory: 13969 loss: 2.2816 grad_norm: 0.8184
+01/29 21:37:51 - mmengine - INFO - Iter(train) [ 60/720] lr: 1.9863e-04 eta: 0:29:58 time: 2.7048 data_time: 0.0069 memory: 13969 loss: 2.2040 grad_norm: 0.8184
+01/29 21:38:18 - mmengine - INFO - Iter(train) [ 70/720] lr: 1.9781e-04 eta: 0:29:31 time: 2.7302 data_time: 0.0068 memory: 13969 loss: 2.1912 grad_norm: 0.8460
+01/29 21:38:46 - mmengine - INFO - Iter(train) [ 80/720] lr: 1.9681e-04 eta: 0:29:05 time: 2.7338 data_time: 0.0069 memory: 13969 loss: 2.1512 grad_norm: 0.8686
+01/29 21:39:13 - mmengine - INFO - Iter(train) [ 90/720] lr: 1.9563e-04 eta: 0:28:36 time: 2.7047 data_time: 0.0068 memory: 13969 loss: 2.0653 grad_norm: 0.8686
+01/29 21:39:40 - mmengine - INFO - Iter(train) [100/720] lr: 1.9426e-04 eta: 0:28:09 time: 2.7383 data_time: 0.0070 memory: 13969 loss: 1.9819 grad_norm: 0.9127
+```
+
+Before training begins, the output of the model is as shown below:
+
+```text
+2024/01/29 21:34:58 - mmengine - INFO - before_train in EvaluateChatHook.
+2024/01/29 21:35:03 - mmengine - INFO - Sample output:
+<|im_start|>system
+You are a professional color designer. Please provide the corresponding colors based on the description of Human.
+<|im_end|>
+<|im_start|>user
+请给我一个像天空一样清澈透明的蓝色。<|im_end|>
+<|im_start|>assistant
+为了匹配您所描述的“像天空一样清澈透明的蓝色”,我建议您选择一种名为“天蓝”(Cerulean)的颜色。这种颜色通常被用来代表天空、海洋和清澈的水域,它具有明亮
+
+2024/01/29 21:35:07 - mmengine - INFO - Sample output:
+<|im_start|>system
+You are a professional color designer. Please provide the corresponding colors based on the description of Human.
+<|im_end|>
+<|im_start|>user
+Please give me a clear blue like the sky.<|im_end|>
+<|im_start|>assistant
+The color you're describing is a clear, bright blue that resembles the color of the sky on a sunny day. This color is often referred to as "sky blue" or "cerulean blue." Here are some specific color codes and names that
+```
+
+After training, the output of the model is as shown below:
+
+```text
+2024/01/29 22:08:07 - mmengine - INFO - Sample output:
+<|im_start|>system
+You are a professional color designer. Please provide the corresponding colors based on the description of Human.
+<|im_end|>
+<|im_start|>user
+请给我一个像天空一样清澈透明的蓝色。<|im_end|>
+<|im_start|>assistant
+#1099ee<|im_end|>
+
+2024/01/29 22:08:08 - mmengine - INFO - Sample output:
+<|im_start|>system
+You are a professional color designer. Please provide the corresponding colors based on the description of Human.
+<|im_end|>
+<|im_start|>user
+Please give me a clear blue like the sky.<|im_end|>
+<|im_start|>assistant
+#0066dd<|im_end|>
+```
+
+The color of the model output is shown below:
+
+- 天空一样清澈透明的蓝色:
+- A clear blue like the sky: 
+
+It is clear that the output of the model after training has been fully aligned with the content of the dataset.
+
+# Model Convert + LoRA Merge
+
+After training, we will get several `.pth` files that do **NOT** contain all the parameters of the model, but store the parameters updated by the training process of the QLoRA algorithm. Therefore, we need to convert these `.pth` files to HuggingFace format and merge them into the original LLM weights.
+
+### Model Convert
+
+XTuner has already integrated the tool of converting the model to HuggingFace format. We can use the following command to convert the model.
+
+```bash
+# Create the directory to store parameters in hf format
+mkdir work_dirs/internlm2_7b_qlora_colorist_e5_copy/iter_720_hf
+
+# Convert the model to hf format
+xtuner convert pth_to_hf internlm2_7b_qlora_colorist_e5_copy.py \
+ work_dirs/internlm2_7b_qlora_colorist_e5_copy/iter_720.pth \
+ work_dirs/internlm2_7b_qlora_colorist_e5_copy/iter_720_hf
+```
+
+This command will convert `work_dirs/internlm2_7b_qlora_colorist_e5_copy/iter_720.pth` to hf format based on the contents of the config `internlm2_7b_qlora_colorist_e5_copy.py` and will save it in `work_dirs/internlm2_7b_qlora_colorist_e5_copy/iter_720_hf`.
+
+### LoRA Merge
+
+XTuner has also integrated the tool of merging LoRA weights, we just need to execute the following command:
+
+```bash
+# Create the directory to store the merged weights
+mkdir work_dirs/internlm2_7b_qlora_colorist_e5_copy/merged
+
+# Merge the weights
+xtuner convert merge Shanghai_AI_Laboratory/internlm2-chat-7b \
+ work_dirs/internlm2_7b_qlora_colorist_e5_copy/iter_720_hf \
+ work_dirs/internlm2_7b_qlora_colorist_e5_copy/merged \
+ --max-shard-size 2GB
+```
+
+Similar to the command above, this command will read the original parameter path `Shanghai_AI_Laboratory/internlm2-chat-7b` and the path of parameter which has been converted to hf format `work_dirs/internlm2_7b_qlora_colorist_e5_copy/iter_720_hf` and merge the two parts of the parameters and save them in `work_dirs/internlm2_7b_qlora_colorist_e5_copy/merged`, where the maximum file size for each parameter slice is 2GB.
+
+## Chat with the model
+
+To better appreciate the model's capabilities after merging the weights, we can chat with the model. XTuner also integrates the tool of chatting with models. We can start a simple demo to chat with the model with the following command:
+
+```bash
+xtuner chat work_dirs/internlm2_7b_qlora_colorist_e5_copy/merged \
+ --prompt-template internlm2_chat \
+ --system-template colorist
+```
+
+Of course, we can also choose not to merge the weights and instead chat directly with the LLM + LoRA Adapter, we just need to execute the following command:
+
+```bash
+xtuner chat Shanghai_AI_Laboratory/internlm2-chat-7b
+ --adapter work_dirs/internlm2_7b_qlora_colorist_e5_copy/iter_720_hf \
+ --prompt-template internlm2_chat \
+ --system-template colorist
+```
+
+where `work_dirs/internlm2_7b_qlora_colorist_e5_copy/merged` is the path to the merged weights, `--prompt-template internlm2_chat` specifies that the chat template is InternLM2-Chat, and `-- system-template colorist` specifies that the System Prompt for conversations with models is the template required by the Colorist dataset.
+
+There is an example below:
+
+```text
+double enter to end input (EXIT: exit chat, RESET: reset history) >>> A calming but fairly bright light sky blue, between sky blue and baby blue, with a hint of fluorescence due to its brightness.
+
+#66ccff<|im_end|>
+```
+
+The color of the model output is shown below:
+
+A calming but fairly bright light sky blue, between sky blue and baby blue, with a hint of fluorescence due to its brightness: .
diff --git a/docs/en/index.rst b/docs/en/index.rst
new file mode 100644
index 000000000..c4c18d31a
--- /dev/null
+++ b/docs/en/index.rst
@@ -0,0 +1,123 @@
+.. xtuner documentation master file, created by
+ sphinx-quickstart on Tue Jan 9 16:33:06 2024.
+ You can adapt this file completely to your liking, but it should at least
+ contain the root `toctree` directive.
+
+Welcome to XTuner's documentation!
+==================================
+
+.. figure:: ./_static/image/logo.png
+ :align: center
+ :alt: xtuner
+ :class: no-scaled-link
+
+.. raw:: html
+
+
+ All-IN-ONE toolbox for LLM
+
+
+
+
+
+ Star
+ Watch
+ Fork
+
+
+
+
+Documentation
+-------------
+.. toctree::
+ :maxdepth: 2
+ :caption: Get Started
+
+ get_started/overview.md
+ get_started/installation.md
+ get_started/quickstart.md
+
+.. toctree::
+ :maxdepth: 2
+ :caption: Preparation
+
+ preparation/pretrained_model.rst
+ preparation/prompt_template.rst
+
+.. toctree::
+ :maxdepth: 2
+ :caption: Training
+
+ training/modify_settings.rst
+ training/custom_sft_dataset.rst
+ training/custom_pretrain_dataset.rst
+ training/custom_agent_dataset.rst
+ training/multi_modal_dataset.rst
+ training/open_source_dataset.rst
+ training/visualization.rst
+
+.. toctree::
+ :maxdepth: 2
+ :caption: DPO
+
+ dpo/overview.md
+ dpo/quick_start.md
+ dpo/modify_settings.md
+
+.. toctree::
+ :maxdepth: 2
+ :caption: Reward Model
+
+ reward_model/overview.md
+ reward_model/quick_start.md
+ reward_model/modify_settings.md
+ reward_model/preference_data.md
+
+.. toctree::
+ :maxdepth: 2
+ :caption: Acceleration
+
+ acceleration/deepspeed.rst
+ acceleration/pack_to_max_length.rst
+ acceleration/flash_attn.rst
+ acceleration/varlen_flash_attn.rst
+ acceleration/hyper_parameters.rst
+ acceleration/length_grouped_sampler.rst
+ acceleration/train_large_scale_dataset.rst
+ acceleration/train_extreme_long_sequence.rst
+ acceleration/benchmark.rst
+
+.. toctree::
+ :maxdepth: 2
+ :caption: Chat
+
+ chat/llm.md
+ chat/agent.md
+ chat/vlm.md
+ chat/lmdeploy.md
+
+.. toctree::
+ :maxdepth: 2
+ :caption: Evaluation
+
+ evaluation/hook.md
+ evaluation/mmlu.md
+ evaluation/mmbench.md
+ evaluation/opencompass.md
+
+.. toctree::
+ :maxdepth: 2
+ :caption: Models
+
+ models/supported.md
+
+.. toctree::
+ :maxdepth: 2
+ :caption: InternEvo Migration
+
+ internevo_migration/internevo_migration.rst
+ internevo_migration/ftdp_dataset/ftdp.rst
+ internevo_migration/ftdp_dataset/Case1.rst
+ internevo_migration/ftdp_dataset/Case2.rst
+ internevo_migration/ftdp_dataset/Case3.rst
+ internevo_migration/ftdp_dataset/Case4.rst
diff --git a/docs/en/internevo_migration/ftdp_dataset/Case1.rst b/docs/en/internevo_migration/ftdp_dataset/Case1.rst
new file mode 100644
index 000000000..c8eb0c76a
--- /dev/null
+++ b/docs/en/internevo_migration/ftdp_dataset/Case1.rst
@@ -0,0 +1,2 @@
+Case 1
+======
diff --git a/docs/en/internevo_migration/ftdp_dataset/Case2.rst b/docs/en/internevo_migration/ftdp_dataset/Case2.rst
new file mode 100644
index 000000000..74069f68f
--- /dev/null
+++ b/docs/en/internevo_migration/ftdp_dataset/Case2.rst
@@ -0,0 +1,2 @@
+Case 2
+======
diff --git a/docs/en/internevo_migration/ftdp_dataset/Case3.rst b/docs/en/internevo_migration/ftdp_dataset/Case3.rst
new file mode 100644
index 000000000..d963b538b
--- /dev/null
+++ b/docs/en/internevo_migration/ftdp_dataset/Case3.rst
@@ -0,0 +1,2 @@
+Case 3
+======
diff --git a/docs/en/internevo_migration/ftdp_dataset/Case4.rst b/docs/en/internevo_migration/ftdp_dataset/Case4.rst
new file mode 100644
index 000000000..1f7626933
--- /dev/null
+++ b/docs/en/internevo_migration/ftdp_dataset/Case4.rst
@@ -0,0 +1,2 @@
+Case 4
+======
diff --git a/docs/en/internevo_migration/ftdp_dataset/ftdp.rst b/docs/en/internevo_migration/ftdp_dataset/ftdp.rst
new file mode 100644
index 000000000..613568f15
--- /dev/null
+++ b/docs/en/internevo_migration/ftdp_dataset/ftdp.rst
@@ -0,0 +1,2 @@
+ftdp
+====
diff --git a/docs/en/internevo_migration/internevo_migration.rst b/docs/en/internevo_migration/internevo_migration.rst
new file mode 100644
index 000000000..869206508
--- /dev/null
+++ b/docs/en/internevo_migration/internevo_migration.rst
@@ -0,0 +1,2 @@
+InternEVO Migration
+===================
diff --git a/docs/en/make.bat b/docs/en/make.bat
new file mode 100644
index 000000000..954237b9b
--- /dev/null
+++ b/docs/en/make.bat
@@ -0,0 +1,35 @@
+@ECHO OFF
+
+pushd %~dp0
+
+REM Command file for Sphinx documentation
+
+if "%SPHINXBUILD%" == "" (
+ set SPHINXBUILD=sphinx-build
+)
+set SOURCEDIR=.
+set BUILDDIR=_build
+
+%SPHINXBUILD% >NUL 2>NUL
+if errorlevel 9009 (
+ echo.
+ echo.The 'sphinx-build' command was not found. Make sure you have Sphinx
+ echo.installed, then set the SPHINXBUILD environment variable to point
+ echo.to the full path of the 'sphinx-build' executable. Alternatively you
+ echo.may add the Sphinx directory to PATH.
+ echo.
+ echo.If you don't have Sphinx installed, grab it from
+ echo.https://www.sphinx-doc.org/
+ exit /b 1
+)
+
+if "%1" == "" goto help
+
+%SPHINXBUILD% -M %1 %SOURCEDIR% %BUILDDIR% %SPHINXOPTS% %O%
+goto end
+
+:help
+%SPHINXBUILD% -M help %SOURCEDIR% %BUILDDIR% %SPHINXOPTS% %O%
+
+:end
+popd
diff --git a/docs/en/models/supported.md b/docs/en/models/supported.md
new file mode 100644
index 000000000..c61546e52
--- /dev/null
+++ b/docs/en/models/supported.md
@@ -0,0 +1 @@
+# Supported Models
diff --git a/docs/en/preparation/pretrained_model.rst b/docs/en/preparation/pretrained_model.rst
new file mode 100644
index 000000000..a3ac291ac
--- /dev/null
+++ b/docs/en/preparation/pretrained_model.rst
@@ -0,0 +1,2 @@
+Pretrained Model
+================
diff --git a/docs/en/preparation/prompt_template.rst b/docs/en/preparation/prompt_template.rst
new file mode 100644
index 000000000..43ccb98e3
--- /dev/null
+++ b/docs/en/preparation/prompt_template.rst
@@ -0,0 +1,2 @@
+Prompt Template
+===============
diff --git a/docs/en/reward_model/modify_settings.md b/docs/en/reward_model/modify_settings.md
new file mode 100644
index 000000000..4f41ca300
--- /dev/null
+++ b/docs/en/reward_model/modify_settings.md
@@ -0,0 +1,100 @@
+## Modify Reward Model Training Configuration
+
+This section introduces the config related to Reward Model training. For more details on XTuner config files, please refer to [Modify Settings](https://xtuner.readthedocs.io/zh-cn/latest/training/modify_settings.html).
+
+### Loss Function
+
+XTuner uses the [Bradley–Terry Model](https://en.wikipedia.org/wiki/Bradley%E2%80%93Terry_model) for preference modeling in the Reward Model. You can specify `loss_type="ranking"` to use ranking loss. XTuner also implements the focal loss function proposed in InternLM2, which adjusts the weights of difficult and easy samples to avoid overfitting. You can set `loss_type="focal"` to use this loss function. For a detailed explanation of this loss function, please refer to the [InternLM2 Technical Report](https://arxiv.org/abs/2403.17297).
+
+Additionally, to maintain stable reward model output scores, we have added a constraint term in the loss. You can specify `penalty_type='log_barrier'` or `penalty_type='L2'` to enable log barrier or L2 constraints, respectively.
+
+```python
+#######################################################################
+# PART 1 Settings #
+#######################################################################
+# Model
+loss_type = 'focal' # 'ranking' or 'focal'
+penalty_type = 'log_barrier' # 'log_barrier' or 'L2'
+```
+
+### Modifying the Model
+
+Users can modify `pretrained_model_name_or_path` to change the pretrained model.
+
+Note that XTuner calculates reward scores by appending a special token at the end of the data. Therefore, when switching models with different vocabularies, the ID of this special token also needs to be modified accordingly. We usually use an unused token at the end of the vocabulary as the reward token.
+
+For example, in InternLM2, we use `[UNUSED_TOKEN_130]` as the reward token:
+
+```python
+#######################################################################
+# PART 1 Settings #
+#######################################################################
+# Model
+pretrained_model_name_or_path = 'internlm/internlm2-chat-1_8b-sft'
+reward_token_id = 92527 # use [UNUSED_TOKEN_130] as reward token
+```
+
+If the user switches to the llama3 model, we can use `<|reserved_special_token_0|>` as the reward token:
+
+```python
+#######################################################################
+# PART 1 Settings #
+#######################################################################
+# Model
+pretrained_model_name_or_path = 'meta-llama/Meta-Llama-3-8B-Instruct'
+reward_token_id = 128002 # use <|reserved_special_token_0|> as reward token
+```
+
+### Training Data
+
+In Reward Model training, you can specify the maximum number of tokens for a single sample sequence using `max_length`. XTuner will automatically truncate or pad the data.
+
+```python
+# Data
+max_length = 2048
+```
+
+In the configuration file, we use the `train_dataset` field to specify the training dataset. You can specify the dataset loading method using the `dataset` field and the dataset mapping function using the `dataset_map_fn` field.
+
+```python
+#######################################################################
+# PART 3 Dataset & Dataloader #
+#######################################################################
+sampler = SequenceParallelSampler \
+ if sequence_parallel_size > 1 else DefaultSampler
+
+train_dataset = dict(
+ type=build_preference_dataset,
+ dataset=dict(
+ type=load_dataset,
+ path='argilla/ultrafeedback-binarized-preferences-cleaned'),
+ tokenizer=tokenizer,
+ max_length=max_length,
+ dataset_map_fn=orpo_dpo_mix_40k_map_fn,
+ is_dpo=False,
+ is_reward=True,
+ reward_token_id=reward_token_id,
+ num_proc=32,
+ use_varlen_attn=use_varlen_attn,
+ max_packed_length=max_packed_length,
+ shuffle_before_pack=True,
+)
+
+train_dataloader = dict(
+ batch_size=batch_size,
+ num_workers=dataloader_num_workers,
+ dataset=train_dataset,
+ sampler=dict(type=sampler, shuffle=True),
+ collate_fn=dict(
+ type=preference_collate_fn, use_varlen_attn=use_varlen_attn))
+```
+
+In the above configuration, we use `load_dataset` to load the `argilla/ultrafeedback-binarized-preferences-cleaned` dataset from Hugging Face, using `orpo_dpo_mix_40k_map_fn` as the dataset mapping function (this is because `orpo_dpo_mix_40k` and `ultrafeedback-binarized-preferences-cleaned` have the same format, so the same mapping function is used).
+
+For more information on handling datasets and writing dataset mapping functions, please refer to the [Preference Data Section](./preference_data.md).
+
+### Accelerating Training
+
+When training with preference data, we recommend enabling the [Variable-Length Attention Mechanism](https://xtuner.readthedocs.io/zh-cn/latest/acceleration/varlen_flash_attn.html) to avoid memory waste caused by length differences between chosen and rejected samples within a single preference. You can enable the variable-length attention mechanism by setting `use_varlen_attn=True`.
+
+XTuner also supports many training acceleration methods. For details on how to use them, please refer to the [Acceleration Strategies Section](https://xtuner.readthedocs.io/zh-cn/latest/acceleration/hyper_parameters.html).
diff --git a/docs/en/reward_model/overview.md b/docs/en/reward_model/overview.md
new file mode 100644
index 000000000..eb210140c
--- /dev/null
+++ b/docs/en/reward_model/overview.md
@@ -0,0 +1,43 @@
+## Introduction to Reward Model
+
+### Overview
+
+The Reward Model is a crucial component in the reinforcement learning process. Its primary task is to predict reward values based on given inputs, guiding the direction of the learning algorithm. In RLHF (Reinforcement Learning from Human Feedback), the Reward Model acts as a proxy for human preferences, helping the reinforcement learning algorithm optimize strategies more effectively.
+
+In large language model training, the Reward Model typically refers to the Preference Model. By providing good and bad (chosen & rejected) responses to the same prompts during training, it fits human preferences and predicts a reward value during inference to guide the optimization of the Actor model in the RLHF process.
+
+Applications of the Reward Model include but are not limited to:
+
+- **RLHF Training**: During RLHF training such as the Proximal Policy Optimization (PPO) algorithm, the Reward Model provides reward signals, improve the quality of generated content, and align it more closely with human preferences.
+- **BoN Sampling**: In the Best-of-N (BoN) sampling process, users can use the Reward Model to score multiple responses to the same prompt and select the highest-scoring generated result, thereby enhancing the model's output.
+- **Data Construction**: The Reward Model can be used to evaluate and filter training data or replace manual annotation to construct DPO training data.
+
+### Features of Reward Model Training in XTuner
+
+The Reward Model training in XTuner offers the following significant advantages:
+
+1. **Latest Training Techniques**: XTuner integrates the Reward Model training loss function from InternLM2, which stabilizes the numerical range of reward scores and reduces overfitting on simple samples (see [InternLM2 Technical Report](https://arxiv.org/abs/2403.17297) for details).
+
+2. **Reducing Memory Waste**: Due to the length differences in chosen and rejected data in preference datasets, padding tokens during data concatenation can cause memory waste. In XTuner, by utilizing the variable-length attention feature from Flash Attention2, preference pairs are packed into the same sequence during training, significantly reducing memory waste caused by padding tokens. This not only improves memory efficiency but also allows for training larger models or handling more data under the same hardware conditions.
+
+
+
+3. **Efficient Training**: Leveraging XTuner's QLoRA training capabilities, we can perform full parameter training only on the Reward Model's Value Head, while using QLoRA fine-tuning on the language model itself, substantially reducing the memory overhead of model training.
+
+4. **Long Text Training**: With XTuner's sequence parallel functionality, long text data can be trained efficiently.
+
+
+
+### Getting Started
+
+Refer to the [Quick Start Guide](./quick_start.md) to understand the basic concepts. For more information on configuring training parameters, please see the [Modifying Reward Model Settings](./modify_settings.md) section.
+
+### Open-source Models
+
+We use XTuner to train the InternLM2 Reward Models from the InternLM2 Technical Report, welcome to download and use:
+
+| Model | Transformers(HF) | ModelScope(HF) | OpenXLab(HF) | RewardBench Score |
+| ------------------------- | -------------------------------------------------------------------------------- | ---------------------------------------------------------------------------------------------------------- | ----------------------------------------------------------------------------------------------------------------------------------------------------------- | ----------------- |
+| **InternLM2-1.8B-Reward** | [🤗internlm2-1_8b-reward](https://huggingface.co/internlm/internlm2-1_8b-reward) | [internlm2-1_8b-reward](https://modelscope.cn/models/Shanghai_AI_Laboratory/internlm2-1_8b-reward/summary) | [](https://openxlab.org.cn/models/detail/OpenLMLab/internlm2-1_8b-reward) | 80.6 |
+| **InternLM2-7B-Reward** | [🤗internlm2-7b-reward](https://huggingface.co/internlm/internlm2-7b-reward) | [internlm2-7b-reward](https://modelscope.cn/models/Shanghai_AI_Laboratory/internlm2-7b-reward/summary) | [](https://openxlab.org.cn/models/detail/OpenLMLab/internlm2-7b-reward) | 86.6 |
+| **InternLM2-20B-Reward** | [🤗internlm2-20b-reward](https://huggingface.co/internlm/internlm2-20b-reward) | [internlm2-20b-reward](https://modelscope.cn/models/Shanghai_AI_Laboratory/internlm2-20b-reward/summary) | [](https://openxlab.org.cn/models/detail/OpenLMLab/internlm2-20b-reward) | 89.5 |
diff --git a/docs/en/reward_model/preference_data.md b/docs/en/reward_model/preference_data.md
new file mode 100644
index 000000000..2f304e627
--- /dev/null
+++ b/docs/en/reward_model/preference_data.md
@@ -0,0 +1,110 @@
+## Preference Dataset
+
+### Overview
+
+XTuner's Reward Model, along with DPO, ORPO, and other algorithms that training on preference data, adopts the same data format. Each training sample in the preference dataset needs to contain the following three fields: `prompt`, `chosen`, and `rejected`. The values for each field follow the [OpenAI chat message](https://platform.openai.com/docs/api-reference/chat/create) format. A specific example is as follows:
+
+```json
+{
+ "prompt": [
+ {
+ "role": "system",
+ "content": "You are a helpful assistant."
+ },
+ {
+ "role": "user",
+ "content": "Who won the world series in 2020?"
+ },
+ {
+ "role": "assistant",
+ "content": "The Los Angeles Dodgers won the World Series in 2020."
+ },
+ {
+ "role": "user",
+ "content": "Where was it played?"
+ }
+ ],
+ "chosen": [
+ {
+ "role": "assistant",
+ "content": "The 2020 World Series was played at Globe Life Field in Arlington, Texas."
+ }
+ ],
+ "rejected": [
+ {
+ "role": "assistant",
+ "content": "I don't know."
+ }
+ ]
+}
+```
+
+When conducting Reward Model training or DPO training, XTuner processes the preference dataset into different training labels based on the type of training task.
+
+
+
+As shown in the above image, for Reward Model training, we follow the ChatGPT training method by adding a special `<|reward|>` token at the end of the conversation data and calculating the loss only on the logits output by this token. For DPO series algorithm training, we mask the tokens in the prompt part and calculate the loss only on the chosen and rejected responses. In the configuration file, we control the dataset type through the `is_reward` and `is_dpo` fields in the dataset.
+
+### Training with Custom Data
+
+After understanding the data format of a single sample, you only need to save the converted data in [JSON Lines](https://jsonlines.org/) format. Each line in the file is a sample.
+
+```json
+{"prompt": [{"content": "How can I learn to better regulate my emotions as a grown adult?", "role": "user"}], "chosen": [{"content": "You could do emotional regulation exercises with me, and try to notice and practice ways that you could better control your emotions in real life.", "role": "assistant"}], "rejected": [{"content": "Do you mean how can you learn to be more emotionally mature?", "role": "assistant"}]}
+{"prompt": [{"content": "What are some interesting things I can add to a grilled cheese sandwich?", "role": "user"}, {"content": "A tomato, a slice of pickle, some chopped pickles, some chopped green onions. Also, some mayonnaise if you like.", "role": "assistant"}, {"content": "Sounds great. Anything else?", "role": "user"}], "chosen": [{"content": "If you’d like something a little different, I recommend grating some horseradish on top of the sandwich.", "role": "assistant"}], "rejected": [{"content": "Maybe some nice fresh basil?", "role": "assistant"}]}
+{"prompt": [{"content": "How do I bob for apples?", "role": "user"}], "chosen": [{"content": "Ah! Do you mean the game of “applesauce,” or do you mean the idea of “to bob for apples”?", "role": "assistant"}], "rejected": [{"content": "Sorry, I don’t know that term.", "role": "assistant"}]}
+......
+```
+
+After preparing the custom dataset, you need to fill in the path to your saved data in the `data_files` field in the configuration file. You can load multiple JSONL files simultaneously for training.
+
+```python
+#######################################################################
+# PART 3 Dataset & Dataloader #
+#######################################################################
+train_dataset = dict(
+ type=build_preference_dataset,
+ dataset=dict(
+ type=load_jsonl_dataset,
+ data_files=[
+ '/your/jsonl/path/here.jsonl',
+ '/your/another/jsonl/path/here.jsonl'
+ ]),
+)
+```
+
+### Training with Open Source Datasets
+
+Similar to configuring SFT data in XTuner, when using open-source datasets from Hugging Face, you only need to define a mapping function `map_fn` to process the dataset format into XTuner's data format.
+
+Taking `Intel/orca_dpo_pairs` as an example, this dataset has `system`, `question`, `chosen`, and `rejected` fields, with each field's value in text format instead of the [OpenAI chat message](https://platform.openai.com/docs/api-reference/chat/create) format. Therefore, we need to define a mapping function for this dataset:
+
+```python
+def intel_orca_dpo_map_fn(example):
+ prompt = [{
+ 'role': 'system',
+ 'content': example['system']
+ }, {
+ 'role': 'user',
+ 'content': example['question']
+ }]
+ chosen = [{'role': 'assistant', 'content': example['chosen']}]
+ rejected = [{'role': 'assistant', 'content': example['rejected']}]
+ return {'prompt': prompt, 'chosen': chosen, 'rejected': rejected}
+```
+
+As shown in the code, `intel_orca_dpo_map_fn` processes the four fields in the original data, converting them into `prompt`, `chosen`, and `rejected` fields, and ensures each field follows the [OpenAI chat message](https://platform.openai.com/docs/api-reference/chat/create) format, maintaining uniformity in subsequent data processing flows.
+
+After defining the mapping function, you need to import it in the configuration file and configure it in the `dataset_map_fn` field.
+
+```python
+train_dataset = dict(
+ type=build_preference_dataset,
+ dataset=dict(
+ type=load_dataset,
+ path='Intel/orca_dpo_pairs'),
+ tokenizer=tokenizer,
+ max_length=max_length,
+ dataset_map_fn=intel_orca_dpo_map_fn,
+)
+```
diff --git a/docs/en/reward_model/quick_start.md b/docs/en/reward_model/quick_start.md
new file mode 100644
index 000000000..5c802be2f
--- /dev/null
+++ b/docs/en/reward_model/quick_start.md
@@ -0,0 +1,85 @@
+## Quick Start Guide for Reward Model
+
+In this section, we will introduce how to use XTuner to train a 1.8B Reward Model, helping you get started quickly.
+
+### Preparing Pretrained Model Weights
+
+According to the paper [Training language models to follow instructions with human feedback](https://arxiv.org/abs/2203.02155), we use a language model fine-tuned with SFT as the initialization model for the Reward Model. Here, we use [InternLM2-chat-1.8b-sft](https://huggingface.co/internlm/internlm2-chat-1_8b-sft) as the initialization model.
+
+Set `pretrained_model_name_or_path = 'internlm/internlm2-chat-1_8b-sft'` in the training configuration file, and the model files will be automatically downloaded when training starts. If you need to download the model weights manually, please refer to the section [Preparing Pretrained Model Weights](https://xtuner.readthedocs.io/zh-cn/latest/preparation/pretrained_model.html), which provides detailed instructions on how to download model weights from Huggingface or Modelscope. Here are the links to the models on HuggingFace and ModelScope:
+
+- HuggingFace link: https://huggingface.co/internlm/internlm2-chat-1_8b-sft
+- ModelScope link: https://modelscope.cn/models/Shanghai_AI_Laboratory/internlm2-chat-1_8b-sft/summary
+
+### Preparing Training Data
+
+In this tutorial, we use the [UltraFeedback](https://arxiv.org/abs/2310.01377) dataset as an example. For convenience, we use the preprocessed [argilla/ultrafeedback-binarized-preferences-cleaned](https://huggingface.co/datasets/argilla/ultrafeedback-binarized-preferences-cleaned) dataset from Huggingface.
+
+```python
+train_dataset = dict(
+ type=build_preference_dataset,
+ dataset=dict(
+ type=load_dataset,
+ path='argilla/ultrafeedback-binarized-preferences-cleaned'),
+ dataset_map_fn=orpo_dpo_mix_40k_map_fn,
+ is_dpo=False,
+ is_reward=True,
+)
+```
+
+Using the above configuration in the configuration file will automatically download and process this dataset. If you want to use other open-source datasets from Huggingface or custom datasets, please refer to the [Preference Dataset](./preference_data.md) section.
+
+### Preparing Configuration Files
+
+XTuner provides several ready-to-use configuration files, which can be viewed using `xtuner list-cfg`. Execute the following command to copy a configuration file to the current directory.
+
+```bash
+xtuner copy-cfg internlm2_chat_1_8b_reward_full_ultrafeedback .
+```
+
+Open the copied configuration file. If you choose to download the model and dataset automatically, no modifications are needed. If you want to specify paths to your pre-downloaded model and dataset, modify the `pretrained_model_name_or_path` and the `path` parameter in `dataset` under `train_dataset`.
+
+For more training parameter configurations, please refer to the section [Modifying Reward Training Configuration](./modify_settings.md).
+
+### Starting the Training
+
+After completing the above steps, you can start the training task using the following commands.
+
+```bash
+# Single node single GPU
+xtuner train ./internlm2_chat_1_8b_reward_full_ultrafeedback_copy.py
+# Single node multiple GPUs
+NPROC_PER_NODE=${GPU_NUM} xtuner train ./internlm2_chat_1_8b_reward_full_ultrafeedback_copy.py
+# Slurm cluster
+srun ${SRUN_ARGS} xtuner train ./internlm2_chat_1_8b_reward_full_ultrafeedback_copy.py --launcher slurm
+```
+
+The correct training log should look like the following (running on a single A800 GPU):
+
+```
+06/06 16:12:11 - mmengine - INFO - Iter(train) [ 10/15230] lr: 3.9580e-07 eta: 2:59:41 time: 0.7084 data_time: 0.0044 memory: 18021 loss: 0.6270 acc: 0.0000 chosen_score_mean: 0.0000 rejected_score_mean: 0.0000 num_samples: 4.0000 num_tokens: 969.0000
+06/06 16:12:17 - mmengine - INFO - Iter(train) [ 20/15230] lr: 8.3536e-07 eta: 2:45:25 time: 0.5968 data_time: 0.0034 memory: 42180 loss: 0.6270 acc: 0.5000 chosen_score_mean: 0.0013 rejected_score_mean: 0.0010 num_samples: 4.0000 num_tokens: 1405.0000
+06/06 16:12:22 - mmengine - INFO - Iter(train) [ 30/15230] lr: 1.2749e-06 eta: 2:37:18 time: 0.5578 data_time: 0.0024 memory: 32121 loss: 0.6270 acc: 0.7500 chosen_score_mean: 0.0016 rejected_score_mean: 0.0011 num_samples: 4.0000 num_tokens: 932.0000
+06/06 16:12:28 - mmengine - INFO - Iter(train) [ 40/15230] lr: 1.7145e-06 eta: 2:36:05 time: 0.6033 data_time: 0.0025 memory: 42186 loss: 0.6270 acc: 0.7500 chosen_score_mean: 0.0027 rejected_score_mean: 0.0016 num_samples: 4.0000 num_tokens: 994.0000
+06/06 16:12:35 - mmengine - INFO - Iter(train) [ 50/15230] lr: 2.1540e-06 eta: 2:41:03 time: 0.7166 data_time: 0.0027 memory: 42186 loss: 0.6278 acc: 0.5000 chosen_score_mean: 0.0031 rejected_score_mean: 0.0032 num_samples: 4.0000 num_tokens: 2049.0000
+06/06 16:12:40 - mmengine - INFO - Iter(train) [ 60/15230] lr: 2.5936e-06 eta: 2:33:37 time: 0.4627 data_time: 0.0023 memory: 30238 loss: 0.6262 acc: 1.0000 chosen_score_mean: 0.0057 rejected_score_mean: 0.0030 num_samples: 4.0000 num_tokens: 992.0000
+06/06 16:12:46 - mmengine - INFO - Iter(train) [ 70/15230] lr: 3.0331e-06 eta: 2:33:18 time: 0.6018 data_time: 0.0025 memory: 42186 loss: 0.6247 acc: 0.7500 chosen_score_mean: 0.0117 rejected_score_mean: 0.0055 num_samples: 4.0000 num_tokens: 815.0000
+```
+
+### Model Conversion
+
+XTuner provides integrated tools to convert models to HuggingFace format. Simply execute the following commands:
+
+```bash
+# Create a directory to store HF format parameters
+mkdir work_dirs/internlm2_chat_1_8b_reward_full_ultrafeedback_copy/iter_15230_hf
+
+# Convert the format
+xtuner convert pth_to_hf internlm2_chat_1_8b_reward_full_ultrafeedback_copy.py \
+ work_dirs/internlm2_chat_1_8b_reward_full_ultrafeedback_copy.py/iter_15230.pth \
+ work_dirs/internlm2_chat_1_8b_reward_full_ultrafeedback_copy.py/iter_15230_hf
+```
+
+This will convert the XTuner's ckpt to the HuggingFace format.
+
+Note: Since the Reward Model type is not integrated into the official transformers library, only the Reward Models trained with InternLM2 will be converted to the `InternLM2ForRewardModel` type. Other models will default to the `SequenceClassification` type (for example, LLaMa3 will be converted to the `LlamaForSequenceClassification` type).
diff --git a/docs/en/switch_language.md b/docs/en/switch_language.md
new file mode 100644
index 000000000..ff7c4c425
--- /dev/null
+++ b/docs/en/switch_language.md
@@ -0,0 +1,3 @@
+## English
+
+## 简体中文
diff --git a/docs/en/training/custom_agent_dataset.rst b/docs/en/training/custom_agent_dataset.rst
new file mode 100644
index 000000000..b4ad82f01
--- /dev/null
+++ b/docs/en/training/custom_agent_dataset.rst
@@ -0,0 +1,2 @@
+Custom Agent Dataset
+====================
diff --git a/docs/en/training/custom_pretrain_dataset.rst b/docs/en/training/custom_pretrain_dataset.rst
new file mode 100644
index 000000000..00ef0e0cb
--- /dev/null
+++ b/docs/en/training/custom_pretrain_dataset.rst
@@ -0,0 +1,2 @@
+Custom Pretrain Dataset
+=======================
diff --git a/docs/en/training/custom_sft_dataset.rst b/docs/en/training/custom_sft_dataset.rst
new file mode 100644
index 000000000..39a0f7c33
--- /dev/null
+++ b/docs/en/training/custom_sft_dataset.rst
@@ -0,0 +1,2 @@
+Custom SFT Dataset
+==================
diff --git a/docs/en/training/modify_settings.rst b/docs/en/training/modify_settings.rst
new file mode 100644
index 000000000..382aca872
--- /dev/null
+++ b/docs/en/training/modify_settings.rst
@@ -0,0 +1,2 @@
+Modify Settings
+===============
diff --git a/docs/en/training/multi_modal_dataset.rst b/docs/en/training/multi_modal_dataset.rst
new file mode 100644
index 000000000..e3d174a1b
--- /dev/null
+++ b/docs/en/training/multi_modal_dataset.rst
@@ -0,0 +1,2 @@
+Multi-modal Dataset
+===================
diff --git a/docs/en/training/open_source_dataset.rst b/docs/en/training/open_source_dataset.rst
new file mode 100644
index 000000000..8627b439d
--- /dev/null
+++ b/docs/en/training/open_source_dataset.rst
@@ -0,0 +1,2 @@
+Open Source Datasets
+====================
diff --git a/docs/en/training/visualization.rst b/docs/en/training/visualization.rst
new file mode 100644
index 000000000..255c7e88f
--- /dev/null
+++ b/docs/en/training/visualization.rst
@@ -0,0 +1,2 @@
+Visualization
+=============
diff --git a/docs/zh_cn/.readthedocs.yaml b/docs/zh_cn/.readthedocs.yaml
new file mode 100644
index 000000000..8d00802c5
--- /dev/null
+++ b/docs/zh_cn/.readthedocs.yaml
@@ -0,0 +1,16 @@
+version: 2
+
+build:
+ os: ubuntu-22.04
+ tools:
+ python: "3.8"
+
+formats:
+ - epub
+
+python:
+ install:
+ - requirements: requirements/docs.txt
+
+sphinx:
+ configuration: docs/zh_cn/conf.py
diff --git a/docs/zh_cn/Makefile b/docs/zh_cn/Makefile
new file mode 100644
index 000000000..d4bb2cbb9
--- /dev/null
+++ b/docs/zh_cn/Makefile
@@ -0,0 +1,20 @@
+# Minimal makefile for Sphinx documentation
+#
+
+# You can set these variables from the command line, and also
+# from the environment for the first two.
+SPHINXOPTS ?=
+SPHINXBUILD ?= sphinx-build
+SOURCEDIR = .
+BUILDDIR = _build
+
+# Put it first so that "make" without argument is like "make help".
+help:
+ @$(SPHINXBUILD) -M help "$(SOURCEDIR)" "$(BUILDDIR)" $(SPHINXOPTS) $(O)
+
+.PHONY: help Makefile
+
+# Catch-all target: route all unknown targets to Sphinx using the new
+# "make mode" option. $(O) is meant as a shortcut for $(SPHINXOPTS).
+%: Makefile
+ @$(SPHINXBUILD) -M $@ "$(SOURCEDIR)" "$(BUILDDIR)" $(SPHINXOPTS) $(O)
diff --git a/docs/zh_cn/_static/image/logo.png b/docs/zh_cn/_static/image/logo.png
new file mode 100644
index 000000000..0d6b754c9
Binary files /dev/null and b/docs/zh_cn/_static/image/logo.png differ
diff --git a/docs/zh_cn/acceleration/benchmark.rst b/docs/zh_cn/acceleration/benchmark.rst
new file mode 100644
index 000000000..5a1c80804
--- /dev/null
+++ b/docs/zh_cn/acceleration/benchmark.rst
@@ -0,0 +1,199 @@
+速度基准
+========
+
+我们在训练速度方面与
+`LLaMA-Factory `__
+进行了对比。对比所使用的 LLaMA-Factory commit id 为
+`8e04794 `__\ 。使用
+`Alpaca `__
+作为训练数据集测试速度。
+
+硬件
+----
+
+- NVIDIA A100-SXM4-80GB GPUs
+
+- Intel(R) Xeon(R) Gold 6348 CPU @ 2.60GHz
+
+软件环境
+--------
+
+- Python 3.10
+
+- PyTorch 1.13
+
+- CUDA 11.7
+
+- CUDNN 8.5
+
+- NCCL 2.14.3
+
+速度
+----
+
+|image1|
+
+|image2|
+
+|image3|
+
+.. tip::
+ TGS 全称是 Tokens per GPU per Second,每张 GPU 每秒训练的 Token 数
+
+.. raw:: html
+
+
+
+.. list-table::
+ :widths: 30 15 20 20 20 50
+ :header-rows: 1
+
+ * - 模型
+ - GPUs
+ - 序列长度
+ - TGS
+ - TFLOPs
+ - Config
+ * - Llama2-7B
+ - 8
+ - 8k
+ - 3028.3
+ - 185.3
+ - `llama2_70b_full_alpaca_enzh_8k_sp1.py `_
+ * - Llama2-7B
+ - 8
+ - 32k
+ - 2234.2
+ - 193.0
+ - `llama2_7b_full_alpaca_enzh_32k_sp1.py `_
+ * - Llama2-7B
+ - 8
+ - 128k
+ - 948.6
+ - 180.3
+ - `llama2_7b_full_alpaca_enzh_128k_sp8.py `_
+ * - Llama2-7B
+ - 8
+ - 256k
+ - 540.1
+ - 176.9
+ - `llama2_7b_full_alpaca_enzh_256k_sp8.py `_
+ * - Llama2-7B
+ - 32
+ - 1M
+ - 133.6
+ - 153.9
+ - `llama2_7b_full_alpaca_enzh_1M_sp16.py `_
+
+.. list-table::
+ :widths: 30 15 20 20 20 50
+ :header-rows: 1
+
+ * - 模型
+ - GPUs
+ - 序列长度
+ - TGS
+ - TFLOPs
+ - Config
+ * - Yi-34B-200K
+ - 32
+ - 8k
+ - 485.1
+ - 165.6
+ - `yi_34b_200k_full_alpaca_enzh_8k_sp1.py `_
+ * - Yi-34B-200K
+ - 32
+ - 32k
+ - 491.5
+ - 209.1
+ - `yi_34b_200k_full_alpaca_enzh_32k_sp2.py `_
+ * - Yi-34B-200K
+ - 32
+ - 128k
+ - 251.1
+ - 191.8
+ - `yi_34b_200k_full_alpaca_enzh_128k_sp8.py `_
+ * - Yi-34B-200K
+ - 32
+ - 256k
+ - 119.7
+ - 145.3
+ - `yi_34b_200k_full_alpaca_enzh_256k_sp8.py `_
+
+.. list-table::
+ :widths: 30 15 20 20 20 50
+ :header-rows: 1
+
+ * - 模型
+ - GPUs
+ - 序列长度
+ - TGS
+ - TFLOPs
+ - Config
+ * - Llama2-70B
+ - 32
+ - 8k
+ - 216.8
+ - 144.7
+ - `llama2_70b_full_alpaca_enzh_8k_sp1.py `_
+ * - Llama2-70B
+ - 32
+ - 32k
+ - 300.9
+ - 239.6
+ - `llama2_70b_full_alpaca_enzh_32k_sp4.py `_
+ * - Llama2-70B
+ - 32
+ - 128k
+ - 144.7
+ - 189.7
+ - `llama2_70b_full_alpaca_enzh_128k_sp8.py `_
+ * - Llama2-70B
+ - 32
+ - 256k
+ - 63.8
+ - 127.6
+ - `llama2_70b_full_alpaca_enzh_256k_sp16.py `_
+ * - Llama2-70B
+ - 64
+ - 1M
+ - 21.8
+ - 133.5
+ - `llama2_70b_full_alpaca_enzh_1M_sp64.py `_
+
+.. note::
+ 所有实验都会将 Alpaca 数据集拼接为最大长度。由于 Alpaca 数据集所含
+ token 数较少,无法拼接成超长序列(如 1M
+ 长度),因此当序列长度较长时,会对 XTuner 代码进行如下修改:
+
+ .. code:: diff
+
+ # xtuner/dataset/huggingface.py
+ def build_origin_dataset(dataset, split):
+ ...
+ + # 6 times larger dataset (for speed testing purposes only)
+ + dataset = concatenate_datasets([dataset for _ in range(6)])
+ return dataset
+
+ def pack_dataset(dataset, max_length, use_varlen_attn, shuffle_before_pack,
+ map_num_proc):
+ dataset = dataset.map(
+ Packer(max_length, use_varlen_attn=use_varlen_attn),
+ batched=True,
+ - num_proc=map_num_proc
+ + batch_size=25000,
+ + num_proc=1
+ )
+ return dataset
+
+
+.. note::
+ 由于 Alpaca 数据量较小,因此做了第一处修改将数据集大小扩大了 6
+ 倍,以保证拥有足够的训练 iter 数(保证速度测试的稳定性)。另外,由于
+ Alpaca
+ 数据集每条数据的长度较短,因此在数据拼接的时候做了第二处修改以保证拥有足够多的数据,足以拼接为
+ ``max_length`` 最大长度。
+
+.. |image1| image:: https://github.com/InternLM/xtuner/assets/41630003/c9c05dbd-0806-4fb2-9da9-62f04b150f7c
+.. |image2| image:: https://github.com/InternLM/xtuner/assets/41630003/3ef6308c-595b-4624-b56d-a8737a1f2261
+.. |image3| image:: https://github.com/InternLM/xtuner/assets/41630003/ba16368e-e5f7-41eb-89ed-1140a8633134
diff --git a/docs/zh_cn/acceleration/deepspeed.rst b/docs/zh_cn/acceleration/deepspeed.rst
new file mode 100644
index 000000000..2794dc72b
--- /dev/null
+++ b/docs/zh_cn/acceleration/deepspeed.rst
@@ -0,0 +1,103 @@
+============================
+DeepSpeed
+============================
+
+借助 DeepSpeed 中的 ZeRO 技术(零冗余优化器),可以大幅降低 LLM 训练所消耗的显存
+
+如何选择 ZeRO 策略
+====================
+
+模型训练阶段,每张卡中显存占用可以分为两类:
+
+模型状态
+ 模型参数(fp16)、模型梯度(fp16)和 Adam 优化器状态(fp32 的模型参数备份,fp32 的 momentum 和 fp32 的 variance )。
+ 假设模型参数量 :math:`x` ,则共需要 :math:`2x + 2x + (4x + 4x + 4x) = 16x` 字节存储。
+
+.. tip::
+ 全量微调时,每增加 **1B** 参数,需要增加 **16GB** 的显存来存储模型状态
+
+剩余状态
+ 除了模型状态之外的显存占用,包括激活值、各种临时缓冲区以及无法使用的显存碎片。
+
+**ZeRO 策略只优化模型状态显存占用,** 从 ZeRO-1 到 ZeRO-3 优化等级越来越高。
+
+- ZeRO-1 策略针对优化器状态进行分片,模型参数和梯度仍旧是每张卡保持一份,此时,每张卡的模型状态所需显存是 :math:`4x + \frac{12x}{N}` ( N 为 GPU 数目)
+- ZeRO-2 策略针对模型梯度进行分片,模型参数仍旧是每张卡保持一份,此时,每张卡的模型状态所需显存是 :math:`2x + \frac{14x}{N}` ( N 为 GPU 数目)
+- ZeRO-3 策略针对模型参数进行分片,此时每张卡的模型状态所需显存是 :math:`\frac{16x}{N}` ( N 为 GPU 数目)
+
+
+.. tip::
+ 以 7B 模型 + 8 GPUs 全量微调为例:
+
+ - ZeRO-1 模式下,每张卡上模型状态显存占用约为 :math:`2*7 + 2*7 + \frac{4*7 + 4*7 + 4*7}{8} = 38.5` GB
+ - ZeRO-2 模式下,每张卡上模型状态显存占用约为 :math:`2*7 + \frac{2*7 + 4*7 + 4*7 + 4*7}{8} = 26.25` GB
+ - ZeRO-3 模式下,每张卡上模型状态显存占用约为 :math:`\frac{2*7 + 2*7 + 4*7 + 4*7 + 4*7}{8} = 14` GB
+
+.. tip::
+ 由于不同的优化方案不会影响模型训练结果,因此在不会导致 OOM 的前提下,建议使用优化等级较低的 ZeRO 策略。
+
+
+使用 ZeRO 策略训练
+===================
+
+XTuner 内置 ZeRO 配置
+---------------------
+
+XTuner 内置了五种 DeepSpeed ZeRO 配置:
+
+- deepspeed_zero1
+- deepspeed_zero2
+- deepspeed_zero2_offload
+- deepspeed_zero3
+- deepspeed_zero3_offload
+
+可一键启动 DeepSpeed 进行训练,通过 ``--deepspeed`` 来选择不同的 ZeRO 配置:
+
+.. code-block:: console
+
+ $ # 以下命令根据需要任选其一
+ $ xtuner train xxx --deepspeed deepspeed_zero1
+ $ xtuner train xxx --deepspeed deepspeed_zero2
+ $ xtuner train xxx --deepspeed deepspeed_zero2_offload
+ $ xtuner train xxx --deepspeed deepspeed_zero3
+ $ xtuner train xxx --deepspeed deepspeed_zero3_offload
+
+例如若想使用 DeepSpeed ZeRO2 显存优化算法运行 QLoRA 算法在 oasst1 数据集上微调 InternLM2-Chat-7B,可使用以下命令:
+
+.. code-block:: console
+
+ $ # single gpu
+ $ xtuner train internlm2_chat_7b_qlora_oasst1_e3 --deepspeed deepspeed_zero2
+ $ # multi gpus(torchrun)
+ $ NPROC_PER_NODE=${GPU_NUM} xtuner train internlm2_chat_7b_qlora_oasst1_e3 --deepspeed deepspeed_zero2
+ $ # multi gpus(slurm)
+ $ srun ${SRUN_ARGS} xtuner train internlm2_chat_7b_qlora_oasst1_e3 --launcher slurm --deepspeed deepspeed_zero2
+
+
+自定义 ZeRO 配置
+------------------------------------
+
+
+可使用以下命令使用自定义 DeepSpeed 配置文件(需要是一个 json 文件):
+
+.. code-block:: console
+
+ $ # single gpu
+ $ xtuner train internlm2_chat_7b_qlora_oasst1_e3 --deepspeed ${PATH_TO_DEEPSPEED_CONFIG}
+ $ # multi gpus(torchrun)
+ $ NPROC_PER_NODE=${GPU_NUM} xtuner train internlm2_chat_7b_qlora_oasst1_e3 --deepspeed ${PATH_TO_DEEPSPEED_CONFIG}
+ $ # multi gpus(slurm)
+ $ srun ${SRUN_ARGS} xtuner train internlm2_chat_7b_qlora_oasst1_e3 --launcher slurm --deepspeed ${PATH_TO_DEEPSPEED_CONFIG}
+
+
+.. warning::
+ DeepSpeed Config 中的 ``gradient_accumulation_steps`` 会被 XTuner config 中的 ``accumulative_counts`` 设置覆盖
+
+.. warning::
+ DeepSpeed Config 中的 ``train_micro_batch_size_per_gpu`` 会被 XTuner config 中的 ``train_dataloader.batch_size`` 设置覆盖
+
+.. warning::
+ DeepSpeed Config 中的 ``gradient_clipping`` 会被 XTuner config 中的 ``optim_wrapper.clip_grad.max_norm`` 设置覆盖
+
+.. warning::
+ XTuner 会根据所使用的 GPU 架构自动选择 ``fp16`` 或 ``bf16`` 训练,不受
diff --git a/docs/zh_cn/acceleration/flash_attn.rst b/docs/zh_cn/acceleration/flash_attn.rst
new file mode 100644
index 000000000..94bdcec62
--- /dev/null
+++ b/docs/zh_cn/acceleration/flash_attn.rst
@@ -0,0 +1,56 @@
+.. _flash_attn:
+
+Flash Attention
+==================================================
+
+Flash Attention (Flash Attention 2) 是一种用于加速 Transformer 模型中 Attention 计算,并减少其显存消耗的算法。XTuner 中 Flash Attention (Flash Attention 2) 的支持情况如下表所示:
+
+.. list-table::
+ :widths: 25 50
+ :header-rows: 1
+
+ * - 模型
+ - Flash Attention 支持情况
+ * - baichuan 1/2
+ - ❌
+ * - chatglm 2/3
+ - ❌
+ * - deepseek
+ - ✅
+ * - gemma
+ - ❌
+ * - internlm 1/2
+ - ✅
+ * - llama 2
+ - ✅
+ * - mistral
+ - ✅
+ * - qwen 1/1.5
+ - ✅
+ * - starcoder
+ - ✅
+ * - yi
+ - ✅
+ * - zephyr
+ - ✅
+
+.. note::
+ XTuner 会根据运行环境自动控制 Flash Attention 的使用情况 (见 `dispatch_modules `_):
+
+ .. list-table::
+ :widths: 50 50
+ :header-rows: 1
+
+ * - 环境
+ - Flash Attention 使用情况
+ * - 安装 `flash attn `_
+ - Flash Attention 2
+ * - 未安装 `flash attn `_ 且 PyTorch Version <= 1.13
+ - No Flash Attention
+ * - 未安装 `flash attn `_ 且 2.0 <= PyTorch Version <= 2.1
+ - Flash Attention 1
+ * - 未安装 `flash attn `_ 且 PyTorch Version >= 2.2
+ - Flash Attention 2
+
+.. note::
+ 使用 XTuner 训练 QWen1/1.5 时若想使用 Flash Attention 加速,需要先安装 `flash attn `_ (参考 `flash attn 安装 `_,需要 cuda )
diff --git a/docs/zh_cn/acceleration/hyper_parameters.rst b/docs/zh_cn/acceleration/hyper_parameters.rst
new file mode 100644
index 000000000..39a4377fa
--- /dev/null
+++ b/docs/zh_cn/acceleration/hyper_parameters.rst
@@ -0,0 +1,49 @@
+=====================
+调整加速策略
+=====================
+
+本节将会列举 XTuner 中会影响训练速度的配置项。
+
+
+max_length
+-------------------
+
+``max_length`` 表示在数据预处理过程中,单条数据长度超过 ``max_length`` 的部分会被截断,基本所有实验都会设置该项。
+
+pack_to_max_length
+---------------------------
+
+``pack_to_max_length`` 用于配置是否进行\ :ref:`数据集拼接 ` \ 。
+
+``pack_to_max_length = True`` 表示在数据预处理过程中将多条短数据拼接为一条长度为 ``max_length`` 的长数据,该配置可以大幅提升训练速度。
+
+若 ``pack_to_max_length = False``,则推荐将 ``batch_size`` 适度调大以保证训练的稳定性。
+
+use_varlen_attn
+---------------------------
+
+``use_varlen_attn`` 用于配置是否在训练过程中使用\ :ref:`Varlen Flash Attention ` \ 。
+
+当 ``use_varlen_attn = True`` 时,要求 ``pack_to_max_length`` 也要设置为 True。在此情况下,每个 token 在注意力计算阶段仅会关注其所在短数据中的所有 tokens (而非整个序列)。
+
+当 ``use_varlen_attn = False`` 时,每个 token 在注意力计算阶段会关注整个序列。
+
+max_position_embeddings
+---------------------------------
+
+当需要扩展模型上下文窗口的大小时,需要将 ``max_position_embeddings`` 设置为期望的上下文长度。 **需要保证 max_position_embeddings 不大于 max_length。**\
+
+假设需要将 Llama2-7B 模型支持的上下文长度自 4k 拓展为 32k:
+
+1. 若训练数据集中存在较多长度接近 32k 的数据,则推荐 ``max_length = 32k, pack_to_max_length = False, use_varlen_attn = False, max_position_embeddings = 32k`` 这一配置
+2. 若训练数据集中长度接近 32k 的数据量较少甚至没有时,则推荐 ``max_length = 32k, pack_to_max_length = True, use_varlen_attn = False, max_position_embeddings = 32k`` 这一配置
+
+sequence_parallel_size
+-------------------------------------------
+
+在使用序列并行策略训练超长序列时, ``sequence_parallel_size`` 个 GPUs 会共同计算一条长序列。而 ``accumulative_counts`` 则用于控制模型参数更新的频率。
+
+
+accumulative_counts
+----------------------------------------------
+用于控制模型参数更新的频率;假设需要在 N 块 GPUs 上执行 ``batch_size_per_device = 1, max_length = 128k`` 的训练策略。当设置序列并行维度为 ``sequence_parallel_size`` 后,为了保证训练的等价性, ``accumulative_counts`` 需要设置为原来的 ``sequence_parallel_size`` 倍,因为 128k 长度的序列会被切分为 ``sequence_parallel_size`` 份后分发给 ``sequence_parallel_size`` 个 GPUs 进行训练, ``data_parallel_world_size`` 会变为原来的 :math:`\frac{1}{sequence\_parallel\_size}`。
diff --git a/docs/zh_cn/acceleration/length_grouped_sampler.rst b/docs/zh_cn/acceleration/length_grouped_sampler.rst
new file mode 100644
index 000000000..72c5bc7e3
--- /dev/null
+++ b/docs/zh_cn/acceleration/length_grouped_sampler.rst
@@ -0,0 +1,67 @@
+.. _length_grouped_sampler:
+
+数据分组
+========================
+
+.. raw:: html
+
+
+

+
+
+生成式大模型(例如LLM)的训练数据往往是不定长的,这就导致同一批次(batch)内的数据长短不一。为实现并行化训练,一种常见的做法是将同一批次的数据填充到最长长度。然而,这一填充(Pad)操作会导致训练的低效。如上图,假设数据内各样本的长度分别为
+2、3、7、9,期望分为2个批次进行训练,那么如果使用默认的随机采样器(左侧),数据处理阶段会引入过多的填充数据,实际效率只有65.6%。
+
+现阶段有两种技术方案可以解决 / 缓解这一问题(两者选其一即可,优先考虑
+**数据拼接技术**\ ):
+
+1. 利用
+ **数据拼接技术**\ ,将多条数据拼接至训练支持的最大长度。这一做法可以确保同一批次内的数据长度完全一致,进而避免了填充数据所导致的训练效率降低。具体可参考
+ \ :ref:`数据拼接文档 ` \ 。
+
+ :优点: 可以合并多个数据样本,显著降低训练 iter 数,加速效果好。
+
+ :缺点: 随机合并的多个数据样本间会互相影响,进而影响训练效果(实际影响程度未知);数据进行了合并,丢失了一定数据随机性。
+
+2. (本文)利用
+ **基于数据长度分组的采样器**\ ,在构建批次数据时,基于实际长度进行排序,确保同一批次内的数据长度尽可能相近,进而尽可能减少填充的长度。如上图右侧,利用该采样器后,同样的数据效率将提升至87.5%。
+
+ :优点: 每条数据依然独立存在(独立计算
+ attention),避免数据拼接技术导致的数据样本间的互相影响;数据进行了分组,丢失了一定数据随机性。
+
+ :缺点: 在数据样本长度比较一致的情况下,加速效果一般。
+
+使用 ``LengthGroupedSampler``
+-----------------------------------------
+
+XTuner 中基于数据长度分组的采样器 的实现在
+`这里 `__\ 。用户可以通过在配置文件中修改
+``train_dataloader`` 的 ``sampler`` 参数进行配置。以
+`internlm2_chat_7b_qlora_oasst1_512_e3 `__
+配置文件为例,其默认是使用随机的采样器,我们可以通过下列修改使其使用
+基于数据长度分组的采样器:
+
+.. code:: diff
+
+ - from mmengine.dataset import DefaultSampler
+ + from xtuner.dataset.samplers import LengthGroupedSampler
+
+ batch_size = 16 # per_device
+ accumulative_counts = 1
+
+ train_dataloader = dict(
+ batch_size=batch_size,
+ num_workers=dataloader_num_workers,
+ dataset=train_dataset,
+ - sampler=dict(type=DefaultSampler, shuffle=True),
+ + sampler=dict(
+ + type=LengthGroupedSampler,
+ + length_property='length',
+ + per_device_batch_size=batch_size * accumulative_counts),
+ collate_fn=dict(type=default_collate_fn, use_varlen_attn=use_varlen_attn))
+
+.. note::
+ 其中,\ ``length_property``
+ 需要传入获取数据集长度的“属性”,这一数值在通过 ``process_hf_dataset``
+ 构建数据集时会自动设置为
+ ``'length'``\ (因此,如果使用自定义的数据类,请确保这一属性的正确设置)。
diff --git a/docs/zh_cn/acceleration/pack_to_max_length.rst b/docs/zh_cn/acceleration/pack_to_max_length.rst
new file mode 100644
index 000000000..e08c109c3
--- /dev/null
+++ b/docs/zh_cn/acceleration/pack_to_max_length.rst
@@ -0,0 +1,70 @@
+.. _pack_to_max_length:
+
+数据拼接
+=========================
+
+简介
+---------
+
+对于大型语言模型(LLM)的输入而言,“数据集拼接” 这一概念指的是将多个 token 序列拼接成一个单独的输入。大量的数据集都存在一个特点,即其长度分布严重偏向较短的序列,而 Transformers 模型接收固定长度的输入。因此,在模型训练过程中,通常需要将每条数据 "Pad" 至当前 batch 最长序列的长度,而 "Pad Token" 往往是某个特定的无意义的 token。
+
+将多条数据打包在一起可以不再需要使用 "Pad Token" 进行无意义的填充,减少计算资源的浪费,同时还可以保持模型作为具有固定大小输入的静态图表示的优点。
+
+下表展示了 InternLM2 7B 模型在 Alpaca 数据集上使用不同数据集拼接策略进行训练的速度对比,如表所示,“数据集拼接”会大幅度提升训练效率:
+
+.. list-table::
+ :widths: 25 25 15
+ :header-rows: 1
+
+ * - 拼接策略
+ - 每秒处理 token 数
+ - 加速比
+ * - 不使用
+ - 362.9
+ -
+ * - 拼接至 2k
+ - 2677.1
+ - 7.38x
+ * - 拼接至 4k
+ - 3124.3
+ - 8.61x
+ * - 拼接至 8k
+ - 3173.9
+ - 8.76x
+ * - 拼接至 16k
+ - 2864.4
+ - 7.89x
+ * - 拼接至 32k
+ - 2965.4
+ - 8.17x
+
+使用数据拼接
+---------------------------
+
+XTuner 中提供的 config 文件中默认使用了“数据集拼接”这一功能,可以通过设置 ``max_length`` 字段来调整数据拼接长度。例如可通过以下方式将拼接长度调整为 32k :
+
+.. code-block:: diff
+
+ #######################################################################
+ # PART 1 Settings #
+ #######################################################################
+ - max_length = 2048
+ + max_length = 32768
+ pack_to_max_length = True
+
+ #######################################################################
+ # PART 3 Dataset & Dataloader #
+ #######################################################################
+ train_dataset = dict(
+ max_length=max_length,
+ pack_to_max_length=pack_to_max_length,
+ ...)
+
+.. tip::
+ 若不想使用数据拼接,在 config 中将 ``pack_to_max_length`` 设为 False 即可,
+ 此时 config 中的 ``max_length`` 字段表示单条数据最长的 token 数,整个 batch 会被 pad 成当前 batch 内最长的一条数据的长度。
+
+.. tip::
+ 在不使用数据拼接策略时,XTuner 还提供了一种数据集采样策略 (``LengthGroupedSampler``),可以保证在一个 batch 中的数据长度尽可能接近,
+ 以减少 Pad 对计算资源的浪费。详细用法请参考
+ \ :ref:`LengthGroupedSampler 文档 ` \ 。
diff --git a/docs/zh_cn/acceleration/train_extreme_long_sequence.rst b/docs/zh_cn/acceleration/train_extreme_long_sequence.rst
new file mode 100644
index 000000000..65b364ad8
--- /dev/null
+++ b/docs/zh_cn/acceleration/train_extreme_long_sequence.rst
@@ -0,0 +1,322 @@
+========
+序列并行
+========
+
+在生成式 AI 领域,长文档摘要和视频生成等任务都需要模型具有超长上下文的能力。
+如何训练超长上下文的模型,既是生成式 AI 算法领域的研究热点,也是 AI Infra 领域的难点
+随着 AI 模型参数量的不断增大,为了能够训练超长上下文,通常需要使用一些复杂的并行策略,如 Nvidia Megatron, DeepSpeed Ulysses 等工作。这些工作虽然解决了超长上下文的训练问题,但需要开发者具有一定的 AI Infra 的知识,对生成式 AI 的研究人员很不友好。
+为了让研究人员能够更加便捷地训练超长上下文模型,促进生成式 AI 领域的发展,XTuner 开发了一套超长上下文训练解决方案:
+
+
+- 支持全量训练 **超过百万个 tokens** 的超长序列
+- 支持 **百 B 级** 模型训练:XTuner 的序列并行不仅支持长序列训练,还可结合 ZeRO3 显存优化策略训练大尺寸模型
+- 开箱即用:可直接训练 Transformers 算法库内和 HF Hub 上的模型
+- 完全通用的序列并行 API 抽象
+
+.. raw:: html
+
+
+
+
+
+
+优化目标
+========
+
+尽管开源模型支持的序列长度不断被刷新,但主流的显存优化策略(如 ZeRO 系列)却不足以解决大模型、长序列训练问题。
+如表 1 所示,使用 ZeRO-3 显存优化策略训练超长序列时,单纯增加 GPU 数量无法解决超长序列带来的 OOM 问题;
+这是因为,ZeRO-3 只能优化模型参数和优化器状态占用的显存, **超长训列训练过程中的显存开销主要来自激活值,而非模型参数和优化器状态**。
+
+
+.. list-table:: **表 1 不同序列长度时,使用 ZeRO-3 训练 128k 上下文 yi-34B 模型的训练情况**
+ :widths: 25 15 10 15 25
+ :header-rows: 1
+
+ * - SP
+ - Model
+ - ZeRO
+ - GPUs
+ - TGS
+ * - 1
+ - yi-34B
+ - ZeRO-3
+ - 16
+ - OOM
+ * - 1
+ - yi-34B
+ - ZeRO-3
+ - 32
+ - OOM
+ * - 1
+ - yi-34B
+ - ZeRO-3
+ - 64
+ - OOM
+ * - 8
+ - yi-34B
+ - ZeRO-3
+ - 16
+ - 227
+
+
+为解决长序列训练过程中的显存问题,Megatron-LM 团队和 DeepSpeed 团队分别提出了两种序列并行算法,通过对长序列进行切分的方法来降低单 GPU 上计算的序列长度。XTuner 中的序列并行设计思路参考了 DeepSpeed 的工作 `DeepSpeed Ulysses `_,并加以优化, **以实现一键开启序列并行策略** 。三者的对比如下:
+
+.. list-table:: **表 2 Megatron-LM、DeepSpeed Ulysses 与 XTuner 的序列并行实现对比**
+ :widths: 50 50 50
+ :header-rows: 1
+
+ * -
+ - Attention 通信量
+ - 代码侵入
+ * - Megatron-LM
+ - O(N)
+ - 较高
+ * - DeepSpeed Ulysses
+ - O(N / P)
+ - 较高
+ * - XTuner
+ - O(N / P)
+ - 无
+
+
+
+支持情况
+========
+
+.. list-table::
+ :widths: 25 25
+ :header-rows: 1
+
+ * - 模型
+ - 序列并行支持情况
+ * - baichuan 1/2
+ - ❌
+ * - chatglm 2/3
+ - ❌
+ * - deepseek
+ - ✅
+ * - gemma
+ - ❌
+ * - internlm 2
+ - ✅
+ * - llama 2
+ - ✅
+ * - mistral
+ - ✅
+ * - qwen 1/1.5
+ - ✅
+ * - starcoder
+ - ❌
+ * - yi
+ - ✅
+ * - zephyr
+ - ✅
+
+其他模型的序列并行功能尚在开发中。
+
+训练
+====
+
+.. note::
+ 使用序列并行策略需要首先安装 `flash attn `_ (参考 `flash attn 安装 `_ ,安装过程需要 cuda)
+
+步骤1:修改 config
+------------------
+
+可以通过运行以下命令查看 XTuner 提供的训练不同模型的配置文件:
+
+.. code-block:: console
+
+ $ xtuner list-cfg
+
+针对任一 config 修改 `sequence_parallel_size` 即可使用序列并行策略:
+
+.. code-block:: diff
+
+ # parallel
+ - sequence_parallel_size = 1
+ + sequence_parallel_size = 4 # take `sequence_parallel_size = 4`` as an example
+
+另外,若需要进一步拓展模型的长文本处理能力,需要进一步修改 config 中的 `max_position_embeddings` 字段。例如需要将模型的上下文长度拓展为 64K 时,可进行如下修改:
+
+.. code-block:: diff
+
+ + max_position_embeddings = 65536
+
+ #######################################################################
+ # PART 2 Model & Tokenizer #
+ #######################################################################
+ model = dict(
+ type=SupervisedFinetune,
+ + max_position_embeddings = max_position_embeddings,
+ ...)
+
+步骤2:开始训练
+----------------
+
+需要使用 DeepSpeed 进行训练:
+
+.. code-block:: console
+
+ $ # torchrun
+ $ NPROC_PER_NODE=${GPU_NUM} xtuner train ${CONFIG_PATH} --deepspeed deepspeed_zero2
+ $ # slurm
+ $ srun ${SRUN_ARGS} xtuner train ${CONFIG_PATH} --launcher slurm --deepspeed deepspeed_zero2
+
+
+.. tip::
+ ``${CONFIG_PATH}`` 为步骤 1 中修改得到的 config 文件路径
+
+.. tip::
+ 可根据实际情况选择使用不同的 zero 策略
+
+
+实现方案
+=========
+
+XTuner 中的序列并行设计思路参考了 DeepSpeed 的工作 `DeepSpeed Ulysses `_,并加以优化,以达到直接基于 transformers 算法库或 Huggingface Hub 上的开源模型训练 1M 以上超长序列的目标。
+
+.. raw:: html
+
+
+
+
+
+.. raw:: html
+
+
+ 图 1 序列并行实现方案
+
+
+图 1 展示了序列并行策略的实现方案。由于 Transformer 结构较为规整,除 attention 计算外,其他计算过程中 token 之间不会互相影响(即每个 token 的计算是独立的),这一条件为序列并行提供了有利条件。上图展示了序列并行的核心设计。设由 P 个 GPUs 共同计算一个长度为 N 的长序列,在 Attention 计算的第一阶段,长度为 N / P 的子序列会通过线性层投影为 Query、Key、Value。接下来, QKV Tensor 会在参与序列并行计算的多个 GPUs 之间通过高度优化的 all-to-all 通信算子汇聚,得到序列长度为 N ,但更少注意力头的子序列。注意力计算后,通过另一个 all-to-all 通信算子将其转换为长度为 N / P 的子序列,进行后续计算。伪代码如下所示。
+
+.. code-block:: python
+
+ # Pseudo code for an Attention Layer
+ # Input: hidden_states with shape (bs, seq_len, dim)
+ # Output: attn_out with shape (bs, seq_len, dim)
+ def attn_forward(hidden_states):
+ q, k, v = qkv_proj(hidden_states)
+ q, k, v = reshape(q, k, v) # (bs, q_len, dim) -> (bs, q_len, nhead, hdim)
+ q, k = apply_rotary_pos_emb(q, k, cos, sin)
+ sp_size = get_sequence_parallel_world_size()
+ # (bs, q_len, nhead, hdim) -> (bs, q_len * sp_size, nhead / sp_size, hdim)
+ q, k, v = all_to_all(q, k, v, sp_size)
+ attn_out = local_attn(q, k, v)
+ # (bs, q_len * sp_size, nhead / sp_size, hdim) -> (bs, q_len, nhead, hdim)
+ attn_out = all_to_all(attn_out)
+ attn_out = reshape(attn_out) # (bs, q_len, nhead, hdim) -> (bs, q_len, dim)
+ attn_out = o_proj(attn_out)
+ return attn_out
+
+
+序列并行 API
+=============
+
+为了方便在其他 repo 中使用序列并行策略,XTuner 中抽象出了序列并行所必须的五个 API 接口:
+
+- 序列并行分布式环境初始化 (init_sequence_parallel)
+- 适配序列并行的 Data Sampler (SequenceParallelSampler)
+- 数据 Pad 与切分 (pad_for_sequence_parallel, split_for_sequence_parallel)
+- 适配序列并行的 Attention (dispatch_modules)
+- reduce loss 以正确打印训练损失 (reduce_sequence_parallel_loss)
+
+分布式环境初始化
+-------------------
+
+由于序列并行算法会将长序列切分为 `sequence_parallel_world_size` 块,并将每个子序列分发给对应的 GPU 独立进行计算。因此需要在训练开始前初始化序列并行分布式环境,以指定哪几块 GPU 共同负责一个长序列输入的计算。
+
+一个 `sequence_parallel_world_size = 4` 的示例如下:
+
+.. code-block:: python
+
+ # We have to initialize the distributed training environment first.
+ # Here is an example when training on slurm scheduler
+ # from xtuner.parallel.sequence import init_dist
+ # init_dist('slurm', 'nccl', init_backend='deepspeed')
+ from xtuner.parallel.sequence import init_sequence_parallel
+ sequence_parallel_world_size = 4
+ init_sequence_parallel(sequence_parallel_world_size)
+
+.. tip::
+ 上述过程在 ``xtuner/engine/_strategy/deepspeed.py`` 中实现。
+
+Data Sampler
+--------------
+
+在使用序列并行后,Dataloader 的采样策略需要进一步调整。例如当 `sequence_parallel_world_size = 4` 时,4 块 GPU 从 Dataloader 拿到的数据需要是完全一样的。
+
+在构建 Dataloader 时搭配 XTuner 中提供的 `SequenceParallelSampler` 使用即可:
+
+.. code-block:: python
+
+ from xtuner.parallel.sequence import SequenceParallelSampler
+ dataloader = DataLoader(
+ train_dataset, sampler=SequenceParallelSampler(train_dataset),
+ **other_dataloader_params)
+
+数据 Pad 与切分
+---------------
+
+由于每条训练数据的长度可能不尽相同,我们需要将数据进行 Pad 以使得序列长度可以被 `sequence_parallel_world_size` 整除,这样一条长数据才能被均等地分发给不同的 GPU 上。
+
+训练过程中需要被 Pad 的 Tensor 往往有 input_ids, labels, position_ids, attention_mask 四个,pad 的过程可以通过以下方式实现:
+
+.. code-block:: python
+
+ from xtuner.parallel.sequence import pad_for_sequence_parallel
+ input_ids, labels, position_ids, attention_mask = pad_for_sequence_parallel(
+ input_ids, labels, position_ids, attention_mask)
+
+如果训练过程用不到 attention_mask,那么可以:
+
+.. code-block:: python
+
+ input_ids, labels, position_ids, _ = pad_for_sequence_parallel(
+ input_ids, labels, position_ids)
+
+Pad 后,我们需要对长序列均等切分:
+
+.. code-block:: python
+
+ from xtuner.parallel.sequence import split_for_sequence_parallel
+ # attention mask should not be split
+ input_ids, labels, position_ids = split_for_sequence_parallel(
+ input_ids, labels, position_ids)
+
+.. tip::
+ 以上两步在 ``xtuner/dataset/collate_fns/default_collate_fn.py`` 中实现。
+
+Attention
+-----------
+
+在 Attention 的计算过程中,序列中的不同 token 是不能独立运算的,但不同的 attention head 之间的计算却是独立的。因此,如第一节所述,需要在计算 Attention 前后(即 qkv_proj 后和 o_proj 前)分别插入一个 all-to-all 操作。
+
+XTuner 提供了 dispatch_modules 接口以支持修改模型 Attention 的计算方式:
+
+.. code-block:: python
+
+ from xtuner.model.modules import dispatch_modules
+ model: AutoModelForCausalLM
+ dispatch_modules(model)
+
+.. tip::
+ 上述过程在 ``xtuner/model/sft.py`` 中实现。
+
+Reduce Loss
+-------------
+
+这个 API 对于保证训练的正确性不是必须的,但对于观测模型训练状态,打印训练 loss 是非常有用的。
+
+.. code-block:: python
+
+ from xtuner.parallel.sequence import reduce_sequence_parallel_loss
+ outputs = llm(input_ids=input_ids, labels=labels, **kwargs)
+ num_tokens_per_rank = (labels != -100).sum()
+ # Suppose sequence parallel world size equals to 4,
+ # losses on rank0, rank1, rank2, rank3 are different.
+ loss = reduce_sequence_parallel_loss(outputs.loss, num_tokens_per_rank)
+ # After loss reduction, losses on rank0, rank1, rank2, rank3 are the same.
+
+.. tip::
+ 上述过程在 ``xtuner/model/sft.py`` 中实现。
diff --git a/docs/zh_cn/acceleration/train_large_scale_dataset.rst b/docs/zh_cn/acceleration/train_large_scale_dataset.rst
new file mode 100644
index 000000000..f0925f050
--- /dev/null
+++ b/docs/zh_cn/acceleration/train_large_scale_dataset.rst
@@ -0,0 +1,205 @@
+================
+超大规模数据集
+================
+
+在线数据处理
+===============
+
+XTuner
+默认采用在线数据预处理的策略,这样可以降低用户使用门槛,以达到“开箱即用”的要求。然而,在线数据处理的弊端在于,当数据集过大时,数据处理过程耗时相对较多,可能会触发
+``nccl timeout`` 报错。
+
+为什么会出现 ``nccl timeout``?
+------------------------------------
+
+使用 XTuner 训练模型时,在训练开始前会首先通过
+`process_hf_dataset `__
+函数对整个训练集进行数据预处理,得到模型训练所需要的 ``input_ids``,
+``labels`` 等数据。
+
+由于数据预处理操作是一个 CPU 任务,因此在分布式训练过程中,如果多个 rank
+各自执行预处理任务,会造成 CPU 资源抢占,拖慢数据处理速度。因此 XTuner
+中采用的策略是统一由 rank0 处理,完成后通过
+``torch.distributed.broadcast_object_list`` 接口广播至其他
+rank。这样,不同 rank 就会得到一份完全一样的数据集。
+
+然而,当使用 ``nccl``
+通信策略时,\ ``torch.distributed.broadcast_object_list``
+广播操作的超时时间与 ``nccl`` 通信超时时间相同(默认为 30
+分钟)。当训练数据集较大时,rank0 可能无法在 30
+分钟内处理完全部数据,这样就会导致 ``nccl timeout`` 报错。若修改
+``nccl`` 通信超时时间,则除数据预处理外的其他涉及 ``nccl``
+通信的超时时间设置都会被修改。
+
+解决方案
+-----------
+
+为解决上述问题,可以在训练开始前设置环境变量 ``XTUNER_DATASET_TIMEOUT``
+为一个更大的数(默认为 30 分钟超时,可以酌情将其调大,如:120):
+
+.. code:: console
+
+ $ # On multiple GPUs(torchrun)
+ $ XTUNER_DATASET_TIMEOUT=120 NPROC_PER_NODE=${GPU_NUM} xtuner train ${CONFIG_NAME_OR_PATH} --deepspeed deepspeed_zero1
+ $ # On multiple GPUs(slurm)
+ $ XTUNER_DATASET_TIMEOUT=120 srun ${SRUN_ARGS} xtuner train ${CONFIG_NAME_OR_PATH} --launcher slurm --deepspeed deepspeed_zero1
+
+.. note::
+ 该超时设置只针对数据预处理阶段的广播操作生效。
+
+离线数据处理
+===============
+
+当训练数据量非常大时,每次训练的时候都先在线处理数据可能会极为耗时。我们可以先对原始数据进行离线处理并保存至本地,随后的多次训练可以读入本地离线处理好的数据后直接开始训练。
+
+第一小节介绍如何针对纯语言模型训练所使用的文本数据进行离线处理,第二小节将会介绍如何离线处理
+Llava 训练数据。
+
+.. warning::
+
+ 当切换了 tokenizer 或修改了数据处理中的超参数(如:单条数据的最大长度 ``max_length`` 等)时,需要重新离线处理数据,否则会导致训练报错。
+
+语言模型训练数据离线处理
+-------------------------
+
+为便于介绍,本节以
+`internlm2_7b_qlora_alpaca_e3.py `__
+配置文件为基础,介绍如何离线处理数据集,并使用离线处理的数据集进行训练。
+
+步骤 1:导出目标 config 文件
+^^^^^^^^^^^^^^^^^^^^^^^^^^^^
+
+``internlm2_7b_qlora_alpaca_e3.py`` 是 XTuner 提供的使用 QLora 算法在
+Alpaca 数据集上微调 Internlm2-7B 模型的配置文件。通过以下命令可以将该
+config 拷贝至当前目录下:
+
+.. code::
+
+ xtuner copy-cfg internlm2_7b_qlora_alpaca_e3 .
+
+.. tip::
+ 执行以上命令后,当前目录下会新增一个名为
+ ``internlm2_7b_qlora_alpaca_e3_copy.py`` 的配置文件(与
+ `internlm2_7b_qlora_alpaca_e3.py `__
+ 完全一样)。
+
+步骤 2:离线处理数据集
+^^^^^^^^^^^^^^^^^^^^^^
+
+使用以下命令可离线预处理原始数据:
+
+.. code::
+
+ python xtuner/tools/process_untokenized_datasets.py \
+ internlm2_7b_qlora_alpaca_e3_copy.py \
+ --save-folder /folder/to/save/processed/dataset
+
+.. note::
+ 这里的第一个参数为 Step 1 中修改过的 config
+ 文件,第二个参数为预处理过的数据集的保存路径。
+
+.. note::
+
+ 上述命令会在 internlm2_7b_qlora_alpaca_e3_copy.py
+ 同级目录下新建一个 internlm2_7b_qlora_alpaca_e3_copy_modified.py
+ 文件,后续训练中需要使用该配置文件,而非
+ ``internlm2_7b_qlora_alpaca_e3_copy.py`` 。
+
+步骤 3:启动训练
+^^^^^^^^^^^^^^^^
+
+可以通过以下命令启动训练:
+
+.. code:: console
+
+ $ # On multiple GPUs(torchrun)
+ $ NPROC_PER_NODE=${GPU_NUM} xtuner train internlm2_7b_qlora_alpaca_e3_copy_modified.py --deepspeed deepspeed_zero1
+ $ # On multiple GPUs(slurm)
+ $ srun ${SRUN_ARGS} xtuner train internlm2_7b_qlora_alpaca_e3_copy_modified.py --launcher slurm --deepspeed deepspeed_zero1
+
+
+.. note::
+ 训练中需要使用步骤 2 新生成的
+ internlm2_7b_qlora_alpaca_e3_copy_modified.py 文件,而非
+ internlm2_7b_qlora_alpaca_e3_copy.py 文件。
+
+Llava 训练数据离线处理
+---------------------------
+
+为便于介绍,本节以
+`llava_internlm2_chat_7b_clip_vit_large_p14_336_e1_gpu8_pretrain.py `__
+配置文件为基础,介绍如何离线处理数据集,并使用离线处理的数据集进行训练。
+
+
+步骤 1:导出目标 config 文件
+^^^^^^^^^^^^^^^^^^^^^^^^^^^^
+
+``llava_internlm2_chat_7b_clip_vit_large_p14_336_e1_gpu8_pretrain.py``
+是 XTuner 提供的基于 internlm2-chat-7b 训练 Llava
+模型配置文件。可以通过以下命令将该 config 拷贝至当前目录下:
+
+.. code:: console
+
+ $ xtuner copy-cfg llava_internlm2_chat_7b_clip_vit_large_p14_336_e1_gpu8_pretrain .
+
+.. note::
+ 执行以上命令后,当前目录下会新增一个名为
+ ``llava_internlm2_chat_7b_clip_vit_large_p14_336_e1_gpu8_pretrain_copy.py``
+ 的配置文件(与
+ `llava_internlm2_chat_7b_clip_vit_large_p14_336_e1_gpu8_pretrain.py `__
+ 完全一样)。
+
+
+
+步骤 2:离线处理数据集
+^^^^^^^^^^^^^^^^^^^^^^
+
+使用以下命令可离线预处理原始数据:
+
+.. code:: console
+
+ $ python xtuner/tools/process_untokenized_llava_data.py llava_internlm2_chat_7b_clip_vit_large_p14_336_e1_gpu8_pretrain_copy.py \
+ $ --save-folder /folder/to/save/processed/llava/data
+
+处理后可以读取离线处理后的数据集查看是否符合预期:
+
+.. code:: python
+
+ from datasets import load_from_disk
+ ds = load_from_disk('/folder/to/save/processed/llava/data')
+ print(ds)
+
+步骤 3:修改 config 文件
+^^^^^^^^^^^^^^^^^^^^^^^^
+
+修改 config 文件以便程序运行时直接读取预处理的 Llava 数据:
+
+.. code:: diff
+
+ #######################################################################
+ # PART 3 Dataset & Dataloader #
+ #######################################################################
+ llava_dataset = dict(
+ - data_path=data_path,
+ - tokenizer=tokenizer,
+ + offline_processed_text_folder=/folder/to/save/processed/llava/data
+ ...)
+
+.. note::
+ 其中,\ ``/folder/to/save/processed/llava/data`` 为步骤 2
+ 保存的离线处理数据路径。
+
+步骤 4:开始训练
+^^^^^^^^^^^^^^^^
+
+使用步骤 3 修改得到的 config 训练即可:
+
+.. code:: console
+
+ $ # On a single GPU
+ $ xtuner train llava_internlm2_chat_7b_clip_vit_large_p14_336_e1_gpu8_pretrain_copy.py --deepspeed deepspeed_zero2
+
+ $ # On multiple GPUs (torchrun)
+ $ NPROC_PER_NODE=${GPU_NUM} xtuner train llava_internlm2_chat_7b_clip_vit_large_p14_336_e1_gpu8_pretrain_copy.py --deepspeed deepspeed_zero2
+ $ # On multiple GPUs (slurm)
+ $ srun ${SRUN_ARGS} xtuner train llava_internlm2_chat_7b_clip_vit_large_p14_336_e1_gpu8_pretrain_copy.py --launcher slurm --deepspeed deepspeed_zero2
diff --git a/docs/zh_cn/acceleration/varlen_flash_attn.rst b/docs/zh_cn/acceleration/varlen_flash_attn.rst
new file mode 100644
index 000000000..266739423
--- /dev/null
+++ b/docs/zh_cn/acceleration/varlen_flash_attn.rst
@@ -0,0 +1,162 @@
+===============================================
+Varlen Attention
+===============================================
+
+\ :ref:`数据集拼接 ` \ 一节中,我们讨论了“数据集拼接”策略对模型训练效率的显著提升。
+理论上,数据集拼接可能会对注意力(Attention)机制的计算过程产生影响。这是因为,在未采用数据拼接策略的情况下,
+每条数据在计算注意力时仅与自身相关联。然而,当采用数据拼接策略后,由多条短数据拼接成的长数据在计算注意力时会相互关联。
+以一个由若干短数据拼接成长度为 4096 的数据为例,如果不采用变长注意力机制,在注意力计算阶段,每个 token 将会关注全部 4096 个 tokens ,如图左侧所示。
+
+相反,在使用变长注意力机制的情况下,每个 token 在注意力计算阶段仅会关注其所在短数据中的所有 tokens ,如图右侧所示。因此, **变长注意力机制确保了无论是否采用“数据集拼接”策略,模型训练的行为保持一致性。**
+
+.. raw:: html
+
+
+
+
变长注意力计算原理(拷贝自 https://github.com/InternLM/InternEvo/blob/develop/doc/usage.md)
+
+
+支持列表
+=====================
+
+.. note::
+
+ 使用变长注意力需要首先安装 `flash attn `_ (
+ 参考 `flash attn 安装 `_ )
+
+.. list-table::
+ :widths: 25 50
+ :header-rows: 1
+
+ * - 模型
+ - Flash Attention 支持情况
+ * - baichuan 1/2
+ - ❌
+ * - chatglm 2/3
+ - ❌
+ * - deepseek
+ - ✅
+ * - gemma
+ - ❌
+ * - internlm 1/2
+ - ✅
+ * - llama 2
+ - ✅
+ * - mistral
+ - ✅
+ * - qwen 1/1.5
+ - ❌
+ * - starcoder
+ - ❌
+ * - yi
+ - ✅
+ * - zephyr
+ - ✅
+
+使用变长注意力机制训练
+=========================
+
+步骤 1:安装 flash_attn
+--------------------------
+
+XTuner 中实现的变长注意力需要依赖 Flash Attention 2,可通过以下命令安装(需要 cuda):
+
+.. code:: console
+
+ $ MAX_JOBS=4 pip install flash-attn --no-build-isolation
+
+.. tip::
+ 更多安装方式请参考 `flash attn 安装 `_
+
+步骤 2:查找模板 config
+---------------------------
+
+XTuner 提供多个开箱即用的配置文件,用户可以通过下列命令查看:
+
+.. code-block:: console
+
+ $ xtuner list-cfg -p internlm
+
+.. tip::
+ ``-p`` 为模糊查找,若想训练其他模型,可以修改 ``internlm`` 为 XTuner 支持的其他模型名称。
+
+步骤 3:复制 config 文件
+-----------------------------
+
+导出需要使用的 config :
+
+.. code-block:: bash
+
+ xtuner copy-cfg ${CONFIG_NAME} ${SAVE_DIR}
+
+例如通过下列命令将名为 ``internlm_7b_full_oasst1_e3`` 的 config 导出至当前目录下:
+
+.. code-block:: console
+
+ $ xtuner copy-cfg internlm_7b_full_oasst1_e3 .
+
+.. note::
+
+ 当前目录下会存在一个新 config
+ ``internlm_7b_full_oasst1_e3_copy.py`` 。
+
+步骤 4:修改 config 文件
+-------------------------------
+
+将步骤 3 复制得到的 config 文件中的 ``use_varlen_attn`` 属性由 False 改为 True 即可激活变长注意力训练机制:
+
+.. code-block:: diff
+
+ ...
+ #######################################################################
+ # PART 1 Settings #
+ #######################################################################
+ # Model
+ pretrained_model_name_or_path = 'internlm/internlm-7b'
+ - use_varlen_attn = False
+ + use_varlen_attn = True
+ ...
+
+.. warning::
+
+ 当设置 ``use_varlen_attn = True`` 后, ``batch_size = 2, max_length = 2k`` 的配置与 ``batch_size = 1, max_length = 4k`` 的配置训练行为是近似的,
+ 因此 XTuner 目前只支持了 ``batch_size = 1`` 的情况。另外, ``use_varlen_attn = True`` 时 ``pack_to_max_length`` 也需设置为 True。
+
+步骤 5:开始训练
+-----------------------
+
+.. code-block:: bash
+
+ xtuner train ${CONFIG_NAME_OR_PATH}
+
+例如,我们可以基于步骤 4 中修改得到的 `internlm_7b_full_oasst1_e3_copy.py` 进行训练:
+
+.. code-block:: console
+
+ $ # On a single GPU
+ $ xtuner train internlm_7b_full_oasst1_e3_copy.py --deepspeed deepspeed_zero1
+ $ # On multiple GPUs(torchrun)
+ $ NPROC_PER_NODE=${GPU_NUM} xtuner train internlm_7b_full_oasst1_e3_copy.py --deepspeed deepspeed_zero1
+ $ # On multiple GPUs(slurm)
+ $ srun ${SRUN_ARGS} xtuner train internlm_7b_full_oasst1_e3_copy.py --launcher slurm --deepspeed deepspeed_zero1
+
+.. tip::
+ ``--deepspeed`` 表示使用 `DeepSpeed `_ 🚀 来优化训练过程。若未安装 DeepSpeed ,可通过 ``pip install deepspeed>=0.12.3`` 进行安装。XTuner 内置了多种策略,包括 ZeRO-1、ZeRO-2、ZeRO-3 等。如果用户期望关闭此功能,请直接移除此参数。
+
+步骤 6:模型转换
+^^^^^^^^^^^^^^^^^^^^^^^^^^^
+
+将保存的 PTH 模型(如果使用的DeepSpeed,则将会是一个文件夹)转换为 HuggingFace 模型:
+
+.. code-block:: bash
+
+ xtuner convert pth_to_hf ${CONFIG_NAME_OR_PATH} ${PTH} ${SAVE_PATH}
+
+对应上面的例子,模型转换脚本为:
+
+.. code-block:: bash
+
+ xtuner convert pth_to_hf internlm_7b_full_oasst1_e3_copy.py ${PTH} ${SAVE_PATH}
+
+.. note::
+ 其中 ``${PTH}`` 为训练权重保存的路径,若训练时未指定,默认保存在 ``./work_dirs/internlm_7b_full_oasst1_e3_copy`` 路径下。
diff --git a/docs/zh_cn/chat/agent.md b/docs/zh_cn/chat/agent.md
new file mode 100644
index 000000000..c3b0d7a6f
--- /dev/null
+++ b/docs/zh_cn/chat/agent.md
@@ -0,0 +1 @@
+# 智能体模型对话
diff --git a/docs/zh_cn/chat/llm.md b/docs/zh_cn/chat/llm.md
new file mode 100644
index 000000000..336e1b014
--- /dev/null
+++ b/docs/zh_cn/chat/llm.md
@@ -0,0 +1 @@
+# 语言模型对话
diff --git a/docs/zh_cn/chat/lmdeploy.md b/docs/zh_cn/chat/lmdeploy.md
new file mode 100644
index 000000000..36d9bf3f9
--- /dev/null
+++ b/docs/zh_cn/chat/lmdeploy.md
@@ -0,0 +1 @@
+# 使用 LMDeploy 优化推理速度
diff --git a/docs/zh_cn/chat/vlm.md b/docs/zh_cn/chat/vlm.md
new file mode 100644
index 000000000..3a84a3c7e
--- /dev/null
+++ b/docs/zh_cn/chat/vlm.md
@@ -0,0 +1 @@
+# 视觉-语言模型对话
diff --git a/docs/zh_cn/conf.py b/docs/zh_cn/conf.py
new file mode 100644
index 000000000..f64d7ea52
--- /dev/null
+++ b/docs/zh_cn/conf.py
@@ -0,0 +1,109 @@
+# Configuration file for the Sphinx documentation builder.
+#
+# This file only contains a selection of the most common options. For a full
+# list see the documentation:
+# https://www.sphinx-doc.org/en/master/usage/configuration.html
+
+# -- Path setup --------------------------------------------------------------
+
+# If extensions (or modules to document with autodoc) are in another directory,
+# add these directories to sys.path here. If the directory is relative to the
+# documentation root, use os.path.abspath to make it absolute, like shown here.
+
+import os
+import sys
+
+from sphinx.ext import autodoc
+
+sys.path.insert(0, os.path.abspath('../..'))
+
+# -- Project information -----------------------------------------------------
+
+project = 'XTuner'
+copyright = '2024, XTuner Contributors'
+author = 'XTuner Contributors'
+
+# The full version, including alpha/beta/rc tags
+version_file = '../../xtuner/version.py'
+with open(version_file) as f:
+ exec(compile(f.read(), version_file, 'exec'))
+__version__ = locals()['__version__']
+# The short X.Y version
+version = __version__
+# The full version, including alpha/beta/rc tags
+release = __version__
+
+# -- General configuration ---------------------------------------------------
+
+# Add any Sphinx extension module names here, as strings. They can be
+# extensions coming with Sphinx (named 'sphinx.ext.*') or your custom
+# ones.
+extensions = [
+ 'sphinx.ext.napoleon',
+ 'sphinx.ext.viewcode',
+ 'sphinx.ext.intersphinx',
+ 'sphinx_copybutton',
+ 'sphinx.ext.autodoc',
+ 'sphinx.ext.autosummary',
+ 'myst_parser',
+ 'sphinxarg.ext',
+]
+
+# Add any paths that contain templates here, relative to this directory.
+templates_path = ['_templates']
+
+# List of patterns, relative to source directory, that match files and
+# directories to ignore when looking for source files.
+# This pattern also affects html_static_path and html_extra_path.
+exclude_patterns = ['_build', 'Thumbs.db', '.DS_Store']
+
+# Exclude the prompt "$" when copying code
+copybutton_prompt_text = r'\$ '
+copybutton_prompt_is_regexp = True
+
+language = 'zh_CN'
+
+# -- Options for HTML output -------------------------------------------------
+
+# The theme to use for HTML and HTML Help pages. See the documentation for
+# a list of builtin themes.
+#
+html_theme = 'sphinx_book_theme'
+html_logo = '_static/image/logo.png'
+html_theme_options = {
+ 'path_to_docs': 'docs/zh_cn',
+ 'repository_url': 'https://github.com/InternLM/xtuner',
+ 'use_repository_button': True,
+}
+# Add any paths that contain custom static files (such as style sheets) here,
+# relative to this directory. They are copied after the builtin static files,
+# so a file named "default.css" will overwrite the builtin "default.css".
+# html_static_path = ['_static']
+
+# Mock out external dependencies here.
+autodoc_mock_imports = [
+ 'cpuinfo',
+ 'torch',
+ 'transformers',
+ 'psutil',
+ 'prometheus_client',
+ 'sentencepiece',
+ 'vllm.cuda_utils',
+ 'vllm._C',
+ 'numpy',
+ 'tqdm',
+]
+
+
+class MockedClassDocumenter(autodoc.ClassDocumenter):
+ """Remove note about base class when a class is derived from object."""
+
+ def add_line(self, line: str, source: str, *lineno: int) -> None:
+ if line == ' Bases: :py:class:`object`':
+ return
+ super().add_line(line, source, *lineno)
+
+
+autodoc.ClassDocumenter = MockedClassDocumenter
+
+navigation_with_keys = False
diff --git a/docs/zh_cn/dpo/modify_settings.md b/docs/zh_cn/dpo/modify_settings.md
new file mode 100644
index 000000000..2365be25c
--- /dev/null
+++ b/docs/zh_cn/dpo/modify_settings.md
@@ -0,0 +1,83 @@
+## 修改 DPO 训练配置
+
+本章节仅介绍与 DPO(Direct Preference Optimization)训练相关的配置参数,更多 XTuner 配置文件的细节,请参考[修改训练配置](https://xtuner.readthedocs.io/zh-cn/latest/training/modify_settings.html)
+
+### 损失函数
+
+在 DPO 训练中,你可以根据需求选择不同的损失函数类型。XTuner 提供了多种损失函数选项,如 `sigmoid`、`hinge`、`ipo` 等。可以通过设置 `dpo_loss_type` 参数来选择使用的损失函数类型。
+
+此外,你还可以通过调整 `loss_beta` 参数来控制损失函数中的温度系数。同时,`label_smoothing` 参数可以用于平滑标签。
+
+```python
+#######################################################################
+# PART 1 Settings #
+#######################################################################
+# Model
+dpo_loss_type = 'sigmoid' # One of ['sigmoid', 'hinge', 'ipo', 'kto_pair', 'sppo_hard', 'nca_pair', 'robust']
+loss_beta = 0.1
+label_smoothing = 0.0
+```
+
+### 修改模型
+
+用户可以修改 `pretrained_model_name_or_path` 对预训练模型进行修改。
+
+```python
+#######################################################################
+# PART 1 Settings #
+#######################################################################
+# Model
+pretrained_model_name_or_path = 'internlm/internlm2-chat-1_8b-sft'
+```
+
+### 训练数据
+
+在 DPO 训练中,你可以通过 `max_length` 来指定单个样本序列的最大 token 数,XTuner 会自动对数据进行截断或是填充。
+
+```python
+# Data
+max_length = 2048
+```
+
+在配置文件中,我们通过 `train_dataset` 字段来指定训练数据集,你可以通过 `dataset` 字段指定数据集的加载方式,通过 `dataset_map_fn` 字段指定数据集的映射函数。
+
+```python
+#######################################################################
+# PART 3 Dataset & Dataloader #
+#######################################################################
+sampler = SequenceParallelSampler \
+ if sequence_parallel_size > 1 else DefaultSampler
+
+train_dataset = dict(
+ type=build_preference_dataset,
+ dataset=dict(type=load_dataset, path='mlabonne/orpo-dpo-mix-40k'),
+ tokenizer=tokenizer,
+ max_length=max_length,
+ dataset_map_fn=orpo_dpo_mix_40k_map_fn,
+ is_dpo=True,
+ is_reward=False,
+ reward_token_id=-1,
+ num_proc=32,
+ use_varlen_attn=use_varlen_attn,
+ max_packed_length=max_packed_length,
+ shuffle_before_pack=True,
+)
+
+train_dataloader = dict(
+ batch_size=batch_size,
+ num_workers=dataloader_num_workers,
+ dataset=train_dataset,
+ sampler=dict(type=sampler, shuffle=True),
+ collate_fn=dict(
+ type=preference_collate_fn, use_varlen_attn=use_varlen_attn))
+```
+
+上述配置中,我们使用了 `load_dataset` 来加载 huggingface 上的 `mlabonne/orpo-dpo-mix-40k` 数据集,使用 `orpo_dpo_mix_40k_map_fn` 作为数据集映射函数。
+
+关于如何处理数据集以及如何编写数据集映射函数,请参考[偏好数据集章节](../reward_model/preference_data.md)。
+
+### 加速训练
+
+在使用偏好数据训练时,我们推荐您开启[变长注意力机制](https://xtuner.readthedocs.io/zh-cn/latest/acceleration/varlen_flash_attn.html), 以避免单个偏好内的 chosen 和 rejected 的样本长度差异造成的显存浪费。你可以通过 `use_varlen_attn=True` 来开启变长注意力机制。
+
+XTuner 中还支持了大量的训练加速方法,关于它们的使用方法,请参考[加速策略章节](https://xtuner.readthedocs.io/zh-cn/latest/acceleration/hyper_parameters.html)。
diff --git a/docs/zh_cn/dpo/overview.md b/docs/zh_cn/dpo/overview.md
new file mode 100644
index 000000000..d3c3a7aad
--- /dev/null
+++ b/docs/zh_cn/dpo/overview.md
@@ -0,0 +1,27 @@
+## DPO 介绍
+
+### 简介
+
+DPO(Direct Preference Optimization,直接偏好优化)是一种在大语言模型训练中用于直接优化模型偏好的方法。与传统的强化学习方法不同,DPO 直接使用人类偏好数据进行模型优化,从而提高生成内容的质量,使其更符合人类偏好。DPO 利用人类偏好数据,直接对模型进行优化,省略了训练 Reward Model 的训练过程,与 PPO 相比进一步省去了 Critic Model,不但避免了复杂的强化学习算法,减少了训练开销,同时还提高了训练效率。
+
+DPO 拥有大量的衍生算法,它们对 DPO 的损失函数进行了一定程度上的改进,我们在 XTuner 中除了 DPO 还实现了[Identity Preference Optimisation (IPO)](https://huggingface.co/papers/2310.12036),[Kahneman-Tversky Optimisation (KTO)](https://github.com/ContextualAI/HALOs)等论文中的损失函数,如需使用这些算法,请参考[修改 DPO 配置](./modify_settings.md)章节。我们也提供了一些[示例配置](https://github.com/InternLM/xtuner/tree/main/xtuner/configs/dpo)用于参考。
+
+除了 DPO 之外,还出现了如 [ORPO](https://arxiv.org/abs/2403.07691) 等无需参考模型的对齐算法。ORPO 采用了对数比值(odds ratio)的概念来优化模型,通过在模型训练过程中惩罚那些被拒绝的样本,从而更有效地适应被选择的样本。ORPO 消除了对参考模型的依赖,使得训练过程更加简化且高效。XTuner 中 ORPO 的训练方式与 DPO 非常类似,我们提供了一些 ORPO 的[示例配置](https://github.com/InternLM/xtuner/tree/main/xtuner/configs/orpo),用户可以参考 DPO 的教程对配置进行修改。
+
+### XTuner 中 DPO 训练的优势
+
+XTuner 中的 DPO 训练具备以下显著优势:
+
+1. **支持最新的算法**:XTuner除了支持标准的 DPO 之外,还支持了大量的衍生算法,同时也支持ORPO等不依赖参考模型的高效算法。
+
+2. **减少显存浪费**:由于偏好数据中的 chosen 和 rejected 数据通常存在长度上的差异,因此在训练数据的拼接时会存在填充(padding token),造成显存浪费。在 XTuner 中,基于 Flash Attention2 中的[变长注意力](https://xtuner.readthedocs.io/zh-cn/latest/acceleration/varlen_flash_attn.html)功能,我们在训练过程中通过将偏好数据打包到同一个序列中,显著减少了由于 padding token 带来的显存浪费。这不仅提高了显存的利用效率,还使得在相同硬件条件下可以训练更大的模型或处理更多的数据。
+
+
+
+3. **高效训练**:借助 XTuner 的 QLoRA 训练功能,参考模型能够被转化为移除LoRA适配器的语言模型,从而省去了参考模型权重的显存占用,大幅降低了 DPO 的训练开销。
+
+4. **长文本训练**: 借助 XTuner 的序列并行功能,能够对长文本数据进行训练。
+
+### 开始训练
+
+请参阅[快速上手](./quick_start.md)来了解最基本的概念,若希望了解更多训练参数配置相关的内容,请参考[修改DPO配置](./modify_settings.md)章节。
diff --git a/docs/zh_cn/dpo/quick_start.md b/docs/zh_cn/dpo/quick_start.md
new file mode 100644
index 000000000..a92152b0f
--- /dev/null
+++ b/docs/zh_cn/dpo/quick_start.md
@@ -0,0 +1,71 @@
+## DPO 快速上手
+
+在本章节中,我们将介绍如何使用 XTuner 训练 1.8B 的 DPO(Direct Preference Optimization)模型,以帮助您快速上手。
+
+### 准备预训练模型权重
+
+我们使用经过 SFT 的语言模型[InternLM2-chat-1.8b-sft](https://huggingface.co/internlm/internlm2-chat-1_8b-sft)作为 DPO 模型的初始化模型来进行偏好对齐。
+
+在训练配置文件中设置`pretrained_model_name_or_path = 'internlm/internlm2-chat-1_8b-sft'`,则会在启动训练时自动下载模型文件。若您需要手动下载模型权重,那么请参考[准备预训练模型权重](https://xtuner.readthedocs.io/zh-cn/latest/preparation/pretrained_model.html)章节,其中详细说明了如何从 Huggingface 或者是 Modelscope 下载模型权重的方法。这里我们附上模型的 HuggingFace 链接与 ModelScope 链接:
+
+- HuggingFace 链接位于:https://huggingface.co/internlm/internlm2-chat-1_8b-sft
+- ModelScope 链接位于:https://modelscope.cn/models/Shanghai_AI_Laboratory/internlm2-chat-1_8b-sft/summary
+
+### 准备训练数据
+
+在本教程中使用 Huggingface 上的[mlabonne/orpo-dpo-mix-40k](https://huggingface.co/datasets/mlabonne/orpo-dpo-mix-40k)数据集作为演示,
+
+```python
+train_dataset = dict(
+ type=build_preference_dataset,
+ dataset=dict(
+ type=load_dataset,
+ path='mlabonne/orpo-dpo-mix-40k'),
+ dataset_map_fn=orpo_dpo_mix_40k_map_fn,
+ is_dpo=True,
+ is_reward=False,
+)
+```
+
+在配置文件中使用以上配置,即可自动下载并处理该数据集。如果您希望使用其他 Huggingface 上的开源数据集或是使用自定义的数据集,请参阅[偏好数据集](../reward_model/preference_data.md)章节。
+
+### 准备配置文件
+
+XTuner 提供了多个开箱即用的配置文件,可以通过 `xtuner list-cfg` 查看。我们执行如下指令,以复制一个配置文件到当前目录。
+
+```bash
+xtuner copy-cfg internlm2_chat_1_8b_dpo_full .
+```
+
+打开复制后的配置文件,如果您选择自动下载模型和数据集,则无需修改配置。若您希望填入您预先下载的模型路径和数据集路径,请修改配置中的`pretrained_model_name_or_path`以及`train_dataset`中`dataset`的`path`参数。
+
+更多的训练参数配置,请参阅[修改DPO训练配置](./modify_settings.md)章节。
+
+### 启动训练
+
+在完成上述操作后,便可以使用下面的指令启动训练任务了。
+
+```bash
+# 单机单卡
+xtuner train ./internlm2_chat_1_8b_dpo_full_copy.py
+# 单机多卡
+NPROC_PER_NODE=${GPU_NUM} xtuner train ./internlm2_chat_1_8b_dpo_full_copy.py
+# slurm 集群
+srun ${SRUN_ARGS} xtuner train ./internlm2_chat_1_8b_dpo_full_copy.py --launcher slurm
+```
+
+### 模型转换
+
+XTuner 已经集成好了将模型转换为 HuggingFace 格式的工具,我们只需要执行
+
+```bash
+# 创建存放 hf 格式参数的目录
+mkdir work_dirs/internlm2_chat_1_8b_dpo_full_copy/iter_15230_hf
+
+# 转换格式
+xtuner convert pth_to_hf internlm2_chat_1_8b_dpo_full_copy.py \
+ work_dirs/internlm2_chat_1_8b_dpo_full_copy.py/iter_15230.pth \
+ work_dirs/internlm2_chat_1_8b_dpo_full_copy.py/iter_15230_hf
+```
+
+便能够将 XTuner 的 ckpt 转换为 Huggingface 格式的模型。
diff --git a/docs/zh_cn/evaluation/hook.md b/docs/zh_cn/evaluation/hook.md
new file mode 100644
index 000000000..80d36f10a
--- /dev/null
+++ b/docs/zh_cn/evaluation/hook.md
@@ -0,0 +1 @@
+# 训练过程中评测
diff --git a/docs/zh_cn/evaluation/mmbench.md b/docs/zh_cn/evaluation/mmbench.md
new file mode 100644
index 000000000..5421b1c96
--- /dev/null
+++ b/docs/zh_cn/evaluation/mmbench.md
@@ -0,0 +1 @@
+# MMBench (VLM)
diff --git a/docs/zh_cn/evaluation/mmlu.md b/docs/zh_cn/evaluation/mmlu.md
new file mode 100644
index 000000000..4bfabff8f
--- /dev/null
+++ b/docs/zh_cn/evaluation/mmlu.md
@@ -0,0 +1 @@
+# MMLU (LLM)
diff --git a/docs/zh_cn/evaluation/opencompass.md b/docs/zh_cn/evaluation/opencompass.md
new file mode 100644
index 000000000..dbd7a4950
--- /dev/null
+++ b/docs/zh_cn/evaluation/opencompass.md
@@ -0,0 +1 @@
+# 使用 OpenCompass 评测
diff --git a/docs/zh_cn/get_started/installation.rst b/docs/zh_cn/get_started/installation.rst
new file mode 100644
index 000000000..b5eedbf10
--- /dev/null
+++ b/docs/zh_cn/get_started/installation.rst
@@ -0,0 +1,49 @@
+==================================
+安装
+==================================
+
+本节中,我们将演示如何安装 XTuner。
+
+最佳实践
+========
+
+我们推荐用户参照我们的最佳实践安装 XTuner。
+推荐使用 Python-3.10 的 conda 虚拟环境安装 XTuner。
+
+**步骤 0.** 使用 conda 先构建一个 Python-3.10 的虚拟环境
+
+.. code-block:: console
+
+ $ conda create --name xtuner-env python=3.10 -y
+ $ conda activate xtuner-env
+
+**步骤 1.** 安装 XTuner
+
+方案a: 通过 pip 直接安装
+
+.. code-block:: console
+
+ $ pip install -U 'xtuner[deepspeed]'
+
+方案b: 从源码安装
+
+.. code-block:: console
+
+ $ git clone https://github.com/InternLM/xtuner.git
+ $ cd xtuner
+ $ pip install -e '.[deepspeed]'
+
+.. note::
+
+ "-e" 表示在可编辑模式下安装项目,因此对代码所做的任何本地修改都会生效
+
+验证
+========
+
+为了验证 XTuner 是否安装正确,我们将使用命令打印配置文件。
+
+**打印配置文件:** 在命令行中使用 ``xtuner list-cfg`` 验证是否能打印配置文件列表。
+
+.. code-block:: console
+
+ $ xtuner list-cfg
diff --git a/docs/zh_cn/get_started/quickstart.rst b/docs/zh_cn/get_started/quickstart.rst
new file mode 100644
index 000000000..4bec2a5ac
--- /dev/null
+++ b/docs/zh_cn/get_started/quickstart.rst
@@ -0,0 +1,415 @@
+快速上手
+========
+
+本节中,我们将演示如何使用 XTuner 微调模型,帮助您快速上手 XTuner。
+
+在成功安装 XTuner
+后,便可以开始进行模型的微调。在本节中,我们将演示如何使用 XTuner,应用
+QLoRA 算法在 Colorist 数据集上微调 InternLM2-Chat-7B。
+
+Colorist 数据集(\ `HuggingFace
+链接 `__\ ;\ `ModelScope
+链接 `__\ )是一个根据颜色描述提供颜色选择与建议的数据集,经过该数据集微调的模型可以做到根据用户对于颜色的描述,从而给出16进制下的颜色编码,如用户输入“宁静而又相当明亮的浅天蓝色,介于天蓝色和婴儿蓝之间,因其亮度而带有一丝轻微的荧光感。”,模型输出
+|image1|\ ,该颜色很符合用户的描述。以下是该数据集的几条样例数据:
+
++-----------------------+-----------------------+-------------------+
+| 英文描述 | 中文描述 | 颜色 |
++=======================+=======================+===================+
+| Light Sky Blue: A | 浅天蓝色 | #66ccff: |image8| |
+| calming, fairly | :一种介于天蓝和婴儿 | |
+| bright color that | 蓝之间的平和、相当明 | |
+| falls between sky | 亮的颜色,由于明亮而 | |
+| blue and baby blue, | 带有一丝轻微的荧光。 | |
+| with a hint of slight | | |
+| fluorescence due to | | |
+| its brightness. | | |
++-----------------------+-----------------------+-------------------+
+| Bright red: This is a | 鲜红色: | #ee0000: |image9| |
+| very vibrant, | 这是一种非常鲜 | |
+| saturated and vivid | 艳、饱和、生动的红色 | |
+| shade of red, | ,类似成熟苹果或新鲜 | |
+| resembling the color | 血液的颜色。它是标准 | |
+| of ripe apples or | RGB | |
+| fresh blood. It is as | 调色板上的红色,不含 | |
+| red as you can get on | 任何蓝色或绿色元素。 | |
+| a standard RGB color | | |
+| palette, with no | | |
+| elements of either | | |
+| blue or green. | | |
++-----------------------+-----------------------+-------------------+
+| Bright Turquoise: | 明亮的绿松石 | #00ffcc: |
+| This color mixes the | 色:这种颜色融合了鲜 | |image10| |
+| freshness of bright | 绿色的清新和淡蓝色的 | |
+| green with the | 宁静,呈现出一种充满 | |
+| tranquility of light | 活力的绿松石色调。它 | |
+| blue, leading to a | 让人联想到热带水域。 | |
+| vibrant shade of | | |
+| turquoise. It is | | |
+| reminiscent of | | |
+| tropical waters. | | |
++-----------------------+-----------------------+-------------------+
+
+准备模型权重
+------------
+
+在微调模型前,首先要准备待微调模型的权重。
+
+.. _从-huggingface-下载-1:
+
+从 HuggingFace 下载
+~~~~~~~~~~~~~~~~~~~
+
+.. code:: bash
+
+ pip install -U huggingface_hub
+
+ # 拉取模型至 Shanghai_AI_Laboratory/internlm2-chat-7b
+ huggingface-cli download internlm/internlm2-chat-7b \
+ --local-dir Shanghai_AI_Laboratory/internlm2-chat-7b \
+ --local-dir-use-symlinks False \
+ --resume-download
+
+.. _从-modelscope-下载-1:
+
+从 ModelScope 下载
+~~~~~~~~~~~~~~~~~~
+
+由于从 HuggingFace
+拉取模型权重,可能存在下载过程不稳定、下载速度过慢等问题。因此在下载过程遇到网络问题时,我们则可以选择从
+ModelScope 下载 InternLM2-Chat-7B 的权重。
+
+.. code:: bash
+
+ pip install -U modelscope
+
+ # 拉取模型至当前目录
+ python -c "from modelscope import snapshot_download; snapshot_download('Shanghai_AI_Laboratory/internlm2-chat-7b', cache_dir='.')"
+
+在完成下载后,便可以开始准备微调数据集了。
+
+此处附上 HuggingFace 链接与 ModelScope 链接:
+
+- HuggingFace
+ 链接位于:\ https://huggingface.co/internlm/internlm2-chat-7b
+
+- ModelScope
+ 链接位于:\ https://modelscope.cn/models/Shanghai_AI_Laboratory/internlm2-chat-7b/summary
+
+准备微调数据集
+--------------
+
+接下来,我们需要准备微调数据集。
+
+.. _从-huggingface-下载-2:
+
+从 HuggingFace 下载
+~~~~~~~~~~~~~~~~~~~
+
+.. code:: bash
+
+ git clone https://huggingface.co/datasets/burkelibbey/colors
+
+.. _从-modelscope-下载-2:
+
+从 ModelScope 下载
+~~~~~~~~~~~~~~~~~~
+
+由于相同的问题,因此我们可以选择从 ModelScope 下载所需要的微调数据集。
+
+.. code:: bash
+
+ git clone https://www.modelscope.cn/datasets/fanqiNO1/colors.git
+
+此处附上 HuggingFace 链接与 ModelScope 链接:
+
+- HuggingFace
+ 链接位于:\ https://huggingface.co/datasets/burkelibbey/colors
+
+- ModelScope 链接位于:\ https://modelscope.cn/datasets/fanqiNO1/colors
+
+准备配置文件
+------------
+
+XTuner 提供了多个开箱即用的配置文件,可以通过 ``xtuner list-cfg``
+查看。我们执行如下指令,以复制一个配置文件到当前目录。
+
+.. code:: bash
+
+ xtuner copy-cfg internlm2_7b_qlora_colorist_e5 .
+
+配置文件名的解释:
+
+======== ==============================
+配置文件 internlm2_7b_qlora_colorist_e5
+======== ==============================
+模型名 internlm2_7b
+使用算法 qlora
+数据集 colorist
+训练时长 5 epochs
+======== ==============================
+
+此时该目录文件结构应如下所示:
+
+.. code:: bash
+
+ .
+ ├── colors
+ │ ├── colors.json
+ │ ├── dataset_infos.json
+ │ ├── README.md
+ │ └── train.jsonl
+ ├── internlm2_7b_qlora_colorist_e5_copy.py
+ └── Shanghai_AI_Laboratory
+ └── internlm2-chat-7b
+ ├── config.json
+ ├── configuration_internlm2.py
+ ├── configuration.json
+ ├── generation_config.json
+ ├── modeling_internlm2.py
+ ├── pytorch_model-00001-of-00008.bin
+ ├── pytorch_model-00002-of-00008.bin
+ ├── pytorch_model-00003-of-00008.bin
+ ├── pytorch_model-00004-of-00008.bin
+ ├── pytorch_model-00005-of-00008.bin
+ ├── pytorch_model-00006-of-00008.bin
+ ├── pytorch_model-00007-of-00008.bin
+ ├── pytorch_model-00008-of-00008.bin
+ ├── pytorch_model.bin.index.json
+ ├── README.md
+ ├── special_tokens_map.json
+ ├── tokenization_internlm2_fast.py
+ ├── tokenization_internlm2.py
+ ├── tokenizer_config.json
+ └── tokenizer.model
+
+修改配置文件
+------------
+
+| 在这一步中,我们需要修改待微调模型路径和数据路径为本地路径,并且修改数据集加载方式。
+| 此外,由于复制得到的配置文件是基于基座(Base)模型的,所以还需要修改
+ ``prompt_template`` 以适配对话(Chat)模型。
+
+.. code:: diff
+
+ #######################################################################
+ # PART 1 Settings #
+ #######################################################################
+ # Model
+ - pretrained_model_name_or_path = 'internlm/internlm2-7b'
+ + pretrained_model_name_or_path = './Shanghai_AI_Laboratory/internlm2-chat-7b'
+
+ # Data
+ - data_path = 'burkelibbey/colors'
+ + data_path = './colors/train.jsonl'
+ - prompt_template = PROMPT_TEMPLATE.default
+ + prompt_template = PROMPT_TEMPLATE.internlm2_chat
+
+ ...
+ #######################################################################
+ # PART 3 Dataset & Dataloader #
+ #######################################################################
+ train_dataset = dict(
+ type=process_hf_dataset,
+ - dataset=dict(type=load_dataset, path=data_path),
+ + dataset=dict(type=load_dataset, path='json', data_files=dict(train=data_path)),
+ tokenizer=tokenizer,
+ max_length=max_length,
+ dataset_map_fn=colors_map_fn,
+ template_map_fn=dict(
+ type=template_map_fn_factory, template=prompt_template),
+ remove_unused_columns=True,
+ shuffle_before_pack=True,
+ pack_to_max_length=pack_to_max_length)
+
+因此在这一步中,修改了
+``pretrained_model_name_or_path``\ 、\ ``data_path``\ 、\ ``prompt_template``
+以及 ``train_dataset`` 中的 ``dataset`` 字段。
+
+启动微调
+--------
+
+在完成上述操作后,便可以使用下面的指令启动微调任务了。
+
+.. code:: bash
+
+ # 单机单卡
+ xtuner train ./internlm2_7b_qlora_colorist_e5_copy.py
+ # 单机多卡
+ NPROC_PER_NODE=${GPU_NUM} xtuner train ./internlm2_7b_qlora_colorist_e5_copy.py
+ # slurm 情况
+ srun ${SRUN_ARGS} xtuner train ./internlm2_7b_qlora_colorist_e5_copy.py --launcher slurm
+
+正确输出的训练日志应类似如下所示:
+
+.. code:: text
+
+ 01/29 21:35:34 - mmengine - INFO - Iter(train) [ 10/720] lr: 9.0001e-05 eta: 0:31:46 time: 2.6851 data_time: 0.0077 memory: 12762 loss: 2.6900
+ 01/29 21:36:02 - mmengine - INFO - Iter(train) [ 20/720] lr: 1.9000e-04 eta: 0:32:01 time: 2.8037 data_time: 0.0071 memory: 13969 loss: 2.6049 grad_norm: 0.9361
+ 01/29 21:36:29 - mmengine - INFO - Iter(train) [ 30/720] lr: 1.9994e-04 eta: 0:31:24 time: 2.7031 data_time: 0.0070 memory: 13969 loss: 2.5795 grad_norm: 0.9361
+ 01/29 21:36:57 - mmengine - INFO - Iter(train) [ 40/720] lr: 1.9969e-04 eta: 0:30:55 time: 2.7247 data_time: 0.0069 memory: 13969 loss: 2.3352 grad_norm: 0.8482
+ 01/29 21:37:24 - mmengine - INFO - Iter(train) [ 50/720] lr: 1.9925e-04 eta: 0:30:28 time: 2.7286 data_time: 0.0068 memory: 13969 loss: 2.2816 grad_norm: 0.8184
+ 01/29 21:37:51 - mmengine - INFO - Iter(train) [ 60/720] lr: 1.9863e-04 eta: 0:29:58 time: 2.7048 data_time: 0.0069 memory: 13969 loss: 2.2040 grad_norm: 0.8184
+ 01/29 21:38:18 - mmengine - INFO - Iter(train) [ 70/720] lr: 1.9781e-04 eta: 0:29:31 time: 2.7302 data_time: 0.0068 memory: 13969 loss: 2.1912 grad_norm: 0.8460
+ 01/29 21:38:46 - mmengine - INFO - Iter(train) [ 80/720] lr: 1.9681e-04 eta: 0:29:05 time: 2.7338 data_time: 0.0069 memory: 13969 loss: 2.1512 grad_norm: 0.8686
+ 01/29 21:39:13 - mmengine - INFO - Iter(train) [ 90/720] lr: 1.9563e-04 eta: 0:28:36 time: 2.7047 data_time: 0.0068 memory: 13969 loss: 2.0653 grad_norm: 0.8686
+ 01/29 21:39:40 - mmengine - INFO - Iter(train) [100/720] lr: 1.9426e-04 eta: 0:28:09 time: 2.7383 data_time: 0.0070 memory: 13969 loss: 1.9819 grad_norm: 0.9127
+
+在训练开始前,可以看到模型的输出如下所示:
+
+.. code:: text
+
+ 2024/01/29 21:34:58 - mmengine - INFO - before_train in EvaluateChatHook.
+ 2024/01/29 21:35:03 - mmengine - INFO - Sample output:
+ <|im_start|>system
+ You are a professional color designer. Please provide the corresponding colors based on the description of Human.
+ <|im_end|>
+ <|im_start|>user
+ 请给我一个像天空一样清澈透明的蓝色。<|im_end|>
+ <|im_start|>assistant
+ 为了匹配您所描述的“像天空一样清澈透明的蓝色”,我建议您选择一种名为“天蓝”(Cerulean)的颜色。这种颜色通常被用来代表天空、海洋和清澈的水域,它具有明亮
+
+ 2024/01/29 21:35:07 - mmengine - INFO - Sample output:
+ <|im_start|>system
+ You are a professional color designer. Please provide the corresponding colors based on the description of Human.
+ <|im_end|>
+ <|im_start|>user
+ Please give me a clear blue like the sky.<|im_end|>
+ <|im_start|>assistant
+ The color you're describing is a clear, bright blue that resembles the color of the sky on a sunny day. This color is often referred to as "sky blue" or "cerulean blue." Here are some specific color codes and names that
+
+而在训练完全完成后,可以观察到模型的输出如下所示:
+
+.. code:: text
+
+ 2024/01/29 22:08:07 - mmengine - INFO - Sample output:
+ <|im_start|>system
+ You are a professional color designer. Please provide the corresponding colors based on the description of Human.
+ <|im_end|>
+ <|im_start|>user
+ 请给我一个像天空一样清澈透明的蓝色。<|im_end|>
+ <|im_start|>assistant
+ #1099ee<|im_end|>
+
+ 2024/01/29 22:08:08 - mmengine - INFO - Sample output:
+ <|im_start|>system
+ You are a professional color designer. Please provide the corresponding colors based on the description of Human.
+ <|im_end|>
+ <|im_start|>user
+ Please give me a clear blue like the sky.<|im_end|>
+ <|im_start|>assistant
+ #0066dd<|im_end|>
+
+模型输出的颜色如下所示:
+
+- 天空一样清澈透明的蓝色:\ |image11|
+
+- A clear blue like the sky: |image12|
+
+不难发现,模型在经过训练后,其输出已经完全与数据集内容所对齐了。
+
+.. _模型转换--lora-合并:
+
+模型转换 + LoRA 合并
+--------------------
+
+在训练完成后,我们会得到几个 ``.pth`` 文件,这些文件存储了 QLoRA
+算法训练过程所更新的参数,而\ **不是**\ 模型的全部参数。因此我们需要将这些
+``.pth`` 文件转换为 HuggingFace 格式,并合并入原始的语言模型权重中。
+
+模型转换
+~~~~~~~~
+
+XTuner 已经集成好了将模型转换为 HuggingFace 格式的工具,我们只需要执行
+
+.. code:: bash
+
+ # 创建存放 hf 格式参数的目录
+ mkdir work_dirs/internlm2_7b_qlora_colorist_e5_copy/iter_720_hf
+
+ # 转换格式
+ xtuner convert pth_to_hf internlm2_7b_qlora_colorist_e5_copy.py \
+ work_dirs/internlm2_7b_qlora_colorist_e5_copy/iter_720.pth \
+ work_dirs/internlm2_7b_qlora_colorist_e5_copy/iter_720_hf
+
+该条转换命令将会根据配置文件 ``internlm2_7b_qlora_colorist_e5_copy.py``
+的内容,将
+``work_dirs/internlm2_7b_qlora_colorist_e5_copy/iter_720.pth`` 转换为 hf
+格式,并保存在
+``work_dirs/internlm2_7b_qlora_colorist_e5_copy/iter_720_hf`` 位置。
+
+LoRA 合并
+~~~~~~~~~
+
+XTuner 也已经集成好了合并 LoRA 权重的工具,我们只需执行如下指令:
+
+.. code:: bash
+
+ # 创建存放合并后的参数的目录
+ mkdir work_dirs/internlm2_7b_qlora_colorist_e5_copy/merged
+
+ # 合并参数
+ xtuner convert merge Shanghai_AI_Laboratory/internlm2-chat-7b \
+ work_dirs/internlm2_7b_qlora_colorist_e5_copy/iter_720_hf \
+ work_dirs/internlm2_7b_qlora_colorist_e5_copy/merged \
+ --max-shard-size 2GB
+
+与转换命令类似,该条合并参数命令会读取原始参数路径
+``Shanghai_AI_Laboratory/internlm2-chat-7b`` 以及转换为 hf
+格式的部分参数路径
+``work_dirs/internlm2_7b_qlora_colorist_e5_copy/iter_720_hf``\ ,将两部分参数合并后保存于
+``work_dirs/internlm2_7b_qlora_colorist_e5_copy/merged``\ ,其中每个参数切片的最大文件大小为
+2GB。
+
+与模型对话
+----------
+
+在合并权重后,为了更好地体会到模型的能力,XTuner
+也集成了与模型对话的工具。通过如下命令,便可以启动一个与模型对话的简易
+Demo。
+
+.. code:: bash
+
+ xtuner chat work_dirs/internlm2_7b_qlora_colorist_e5_copy/merged \
+ --prompt-template internlm2_chat \
+ --system-template colorist
+
+当然,我们也可以选择不合并权重,而是直接与 LLM + LoRA Adapter
+进行对话,我们只需要执行如下指令:
+
+.. code:: bash
+
+ xtuner chat Shanghai_AI_Laboratory/internlm2-chat-7b
+ --adapter work_dirs/internlm2_7b_qlora_colorist_e5_copy/iter_720_hf \
+ --prompt-template internlm2_chat \
+ --system-template colorist
+
+其中 ``work_dirs/internlm2_7b_qlora_colorist_e5_copy/merged``
+是合并后的权重路径,\ ``--prompt-template internlm2_chat``
+指定了对话模板为 InternLM2-Chat,\ ``--system-template colorist``
+则是指定了与模型对话时的 System Prompt 为 Colorist 数据集所要求的模板。
+
+以下是一个例子:
+
+.. code:: text
+
+ double enter to end input (EXIT: exit chat, RESET: reset history) >>> 宁静而又相当明亮的浅天蓝色,介于天蓝色和婴儿蓝之间,因其亮度而带有一丝轻微的荧光感。
+
+ #66ccff<|im_end|>
+
+其颜色如下所示:
+
+宁静而又相当明亮的浅天蓝色,介于天蓝色和婴儿蓝之间,因其亮度而带有一丝轻微的荧光感。:\ |image13|
+
+.. |image1| image:: https://img.shields.io/badge/%2366ccff-66CCFF
+.. |image2| image:: https://img.shields.io/badge/%2366ccff-66CCFF
+.. |image3| image:: https://img.shields.io/badge/%23ee0000-EE0000
+.. |image4| image:: https://img.shields.io/badge/%2300ffcc-00FFCC
+.. |image5| image:: https://img.shields.io/badge/%2366ccff-66CCFF
+.. |image6| image:: https://img.shields.io/badge/%23ee0000-EE0000
+.. |image7| image:: https://img.shields.io/badge/%2300ffcc-00FFCC
+.. |image8| image:: https://img.shields.io/badge/%2366ccff-66CCFF
+.. |image9| image:: https://img.shields.io/badge/%23ee0000-EE0000
+.. |image10| image:: https://img.shields.io/badge/%2300ffcc-00FFCC
+.. |image11| image:: https://img.shields.io/badge/天空一样清澈透明的蓝色-1099EE
+.. |image12| image:: https://img.shields.io/badge/A_clear_blue_like_the_sky-0066DD
+.. |image13| image:: https://img.shields.io/badge/宁静而又相当明亮的浅天蓝色,介于天蓝色和婴儿蓝之间,因其亮度而带有一丝轻微的荧光感。-66CCFF
diff --git a/docs/zh_cn/index.rst b/docs/zh_cn/index.rst
new file mode 100644
index 000000000..4acf0e882
--- /dev/null
+++ b/docs/zh_cn/index.rst
@@ -0,0 +1,97 @@
+.. xtuner documentation master file, created by
+ sphinx-quickstart on Tue Jan 9 16:33:06 2024.
+ You can adapt this file completely to your liking, but it should at least
+ contain the root `toctree` directive.
+
+欢迎来到 XTuner 的中文文档
+==================================
+
+.. figure:: ./_static/image/logo.png
+ :align: center
+ :alt: xtuner
+ :class: no-scaled-link
+
+.. raw:: html
+
+
+ LLM 一站式工具箱
+
+
+
+
+
+ Star
+ Watch
+ Fork
+
+
+
+
+文档
+-------------
+.. toctree::
+ :maxdepth: 2
+ :caption: 开始使用
+
+ get_started/installation.rst
+ get_started/quickstart.rst
+
+.. toctree::
+ :maxdepth: 2
+ :caption: 准备
+
+ preparation/pretrained_model.rst
+ preparation/prompt_template.rst
+
+.. toctree::
+ :maxdepth: 2
+ :caption: 训练
+
+ training/open_source_dataset.rst
+ training/custom_sft_dataset.rst
+ training/custom_pretrain_dataset.rst
+ training/multi_modal_dataset.rst
+ acceleration/train_large_scale_dataset.rst
+ training/modify_settings.rst
+ training/visualization.rst
+
+.. toctree::
+ :maxdepth: 2
+ :caption: DPO
+
+ dpo/overview.md
+ dpo/quick_start.md
+ dpo/modify_settings.md
+
+.. toctree::
+ :maxdepth: 2
+ :caption: Reward Model
+
+ reward_model/overview.md
+ reward_model/quick_start.md
+ reward_model/modify_settings.md
+ reward_model/preference_data.md
+
+.. toctree::
+ :maxdepth: 2
+ :caption: 加速训练
+
+ acceleration/deepspeed.rst
+ acceleration/flash_attn.rst
+ acceleration/varlen_flash_attn.rst
+ acceleration/pack_to_max_length.rst
+ acceleration/length_grouped_sampler.rst
+ acceleration/train_extreme_long_sequence.rst
+ acceleration/hyper_parameters.rst
+ acceleration/benchmark.rst
+
+
+.. toctree::
+ :maxdepth: 1
+ :caption: InternEvo 迁移
+
+ internevo_migration/differences.rst
+ internevo_migration/ftdp_dataset/tokenized_and_internlm2.rst
+ internevo_migration/ftdp_dataset/processed_and_internlm2.rst
+ internevo_migration/ftdp_dataset/processed_and_others.rst
+ internevo_migration/ftdp_dataset/processed_normal_chat.rst
diff --git a/docs/zh_cn/internevo_migration/differences.rst b/docs/zh_cn/internevo_migration/differences.rst
new file mode 100644
index 000000000..68c7f318f
--- /dev/null
+++ b/docs/zh_cn/internevo_migration/differences.rst
@@ -0,0 +1,320 @@
+==============
+主要差异
+==============
+
+总览
+=============
+
+XTuner 可以复现 InternEvo (train_internlm) 仓库训练得到的开源模型
+internlm/internlm2-chat-7b 的训练精度。
+
+下面是 XTuner 和 InternEvo (train_internlm)
+在相同数据集上训练相同基座模型的训练结果对比:
+
+.. list-table::
+ :widths: 50 25 25
+ :header-rows: 1
+
+ * - 能力类别
+ - xtuner
+ - internevo
+ * - 全数据集平均(无智能体)
+ - 56.44
+ - 55.26
+ * - 全维度平均(无智能体)
+ - 49.58
+ - 48.96
+ * - 语言 Language
+ - 64.77
+ - 62.41
+ * - 知识 Knowledge
+ - 52.24
+ - 52.52
+ * - 推理 Reasoning
+ - 65.5
+ - 63.91
+ * - 数学 Mathematics
+ - 30.95
+ - 30.26
+ * - 代码 Coding
+ - 38.91
+ - 41.06
+ * - 长文本 LongEval
+ - 45.09
+ - 43.62
+ * - 智能体 Agent
+ - 44.85
+ - 43.97
+ * - 数学题智能体
+ - 37
+ - 37.19
+ * - CIBench
+ - 79.07
+ - 69.78
+ * - PluginEval
+ - 65.57
+ - 65.62
+
+64 \* A100 的训练时间对比如下:
+
+=========== ==========
+xtuner internevo
+=========== ==========
+15 h 55 min 16h 09 min
+=========== ==========
+
+.. tip::
+ 使用 XTuner 提供的序列并行算法可以进一步提升训练速度,使用方式请参考
+ \ :ref:`序列并行文档 ` \ 。
+
+
+适配
+==========
+
+在从 InternEvo (train_internlm) 向 XTuner
+迁移的过程中,我们需要关注模型、数据以及训练策略这三个方面的适配问题。后续内容将详细阐述如何进行适配。
+
+
+模型
+-------
+
+InternEvo 在训练时读取和保存的模型权重满足以下目录结构(以 tp2pp2
+为例):
+
+.. code::
+
+ |-- root
+ |-- model_config.pt
+ |-- model_tp0_pp0.pt
+ |-- model_tp0_pp1.pt
+ |-- model_tp1_pp0.pt
+ |-- model_tp1_pp1.pt
+
+其中,\ ``model_config.pt`` 保存模型权重的一些 meta 信息,其余 4 个
+checkpoint 则分别保存 4 组 GPUs 上的模型权重。因此,InternEvo
+训练过程中要求读取预训练权重的 tp、pp 策略与训练使用的 tp、pp
+策略一致才能正常读取预训练权重进行训练。
+
+XTuner 支持基于 Huggingface Hub 上的模型进行训练,如下修改 config
+内容即可将基座模型从 internlm2-7b 切换为 internlm2-20b:
+
+.. code:: diff
+
+ #######################################################################
+ # PART 1 Settings #
+ #######################################################################
+ # Model
+ - pretrained_model_name_or_path = 'internlm/internlm2-7b'
+ + pretrained_model_name_or_path = 'internlm/internlm2-20b'
+
+数据
+---------
+
+InternEvo
+在训练过程中通常会把多条数据拼接为一个特定的最大长度,随后输入模型训练。其配置往往满足以下形式:
+
+.. code:: python
+
+ data = dict(
+ seq_len=SEQ_LEN,
+ pack_sample_into_one=False,
+ min_length=MIN_LENGTH,
+ train_folder=TRAIN_FOLDER,
+ dataset_weights=DATASET_WEIGHTS,
+ ...)
+
+其中,数据配比 (``dataset_weights=DATASET_WEIGHTS``) 功能 XTuner
+尚未支持。\ ``TRAIN_FOLDER`` 中的训练数据需要满足 ftdp tokenized
+数据集格式:
+
+.. code::
+
+ |-- TRAIN_FOLDER
+ |-- cn
+ | |-- dataset1
+ | | |-- data1.bin
+ | | |-- data1.bin.meta
+ | |-- dataset2
+ | | |-- data2.bin
+ | | |-- data2.bin.meta
+
+在 XTuner 中实现在线数据集拼接策略需要参考
+``xtuner/configs/internlm/internlm2_7b/internlm2_7b_w_internevo_dataset.py``
+文件中的配置:
+
+.. code:: diff
+
+ #######################################################################
+ # PART 1 Settings #
+ #######################################################################
+ # Data
+ - dataset_folder = '/path/to/sft/data/folder'
+ + dataset_folder = TRAIN_FOLDER
+ - max_length = 32768
+ + max_length = SEQ_LEN
+
+ #######################################################################
+ # PART 3 Dataset & Dataloader #
+ #######################################################################
+ train_dataset = dict(
+ type=build_packed_dataset,
+ dataset_cfg=dict(
+ type=load_intern_repo_tokenized_dataset,
+ data_order_path=None,
+ folder=dataset_folder,
+ - min_length=0,
+ + min_length=MIN_LENGTH,
+ file_type='.bin'),
+ packed_length=max_length,
+ seed=1024)
+
+.. note::
+
+ 需要注意,由于训练数据喂给模型的先后顺序可能对训练结果造成影响,因此建议不要轻易修改上述配置中的 ``seed`` 选项。同时,可参考 \ :ref:`获取数据顺序 ` \ 进一步固定数据顺序。
+
+训练策略
+------------
+
+Varlen Attention
+~~~~~~~~~~~~~~~~~~~~~~~~~~
+
+InternEvo 通过设置
+`数据配置 `__
+中的 ``pack_sample_into_one`` 参数为 False
+来使用“变长注意力机制”(见下图右侧)。
+
+.. code:: python
+
+ data = dict(
+ pack_sample_into_one=False,
+ ...)
+
+.. raw:: html
+
+
+

+
+
+
+在 XTuner 中使用这一功能需要设置 config 中的 ``use_varlen_attn`` 配置为
+True,即可保证训练行为与 InternEvo 一致:
+
+.. code:: diff
+
+ ...
+ #######################################################################
+ # PART 1 Settings #
+ #######################################################################
+ # Model
+ pretrained_model_name_or_path = 'internlm/internlm2-7b'
+ - use_varlen_attn = False
+ + use_varlen_attn = True
+ ...
+
+.. warning::
+ 需要注意,当设置 ``use_varlen_attn = True`` 后,请确保
+ ``batch_size`` 被设置为 1,且 ``pack_to_max_length`` 被设置为
+ True。
+
+.. tip::
+ ``use_varlen_attn = True`` 时 ``单卡 batch size 等于 2,拼接数据集至最大长度 2k``
+ 的配置与 ``单卡 batch size 等于 1,拼接数据集至最大长度 4k`` 的配置训练行为是近似的,
+ 因此 XTuner 目前只支持了 ``batch_size_per_device = 1`` 的情况。
+
+
+梯度累积
+~~~~~~~~~~~~~~
+
+在 InternEvo 的配置中,与 batch_size 和 accumulative_counts
+相关的配置有如下几个:
+
+.. code:: python
+
+ data = dict(
+ # micro_num means the number of micro_batch contained in one gradient update
+ micro_num=MICRO_NUM,
+ # MICRO_BATCH_SIZE * SEQ_LEN = PACKED_LENGTH
+ micro_bsz=MICRO_BATCH_SIZE,
+ total_steps=TOTAL_STEP,
+ # 梯度累计,默认等于MICRO_NUM(BS)
+ gradient_accumulation=GRADIENT_ACCUMULATION,
+ ...)
+
+.. note::
+ InternEVO 中的 ``micro_num`` 等价于 XTuner 中的 ``gradient_accumulation``
+
+.. note::
+ ``total_steps`` 在 XTuner 中可以不手动指定,可通过 ``max_epochs`` 指定。
+
+.. warning::
+ XTuner 目前只支持 ``micro_bsz = 1`` 。
+
+.. tip::
+ 为对齐以上配置,可参考 XTuner 中
+ ``xtuner/configs/internlm/internlm2_7b/internlm2_7b_w_internevo_dataset.py``
+ 文件中的配置,并进行如下修改:
+
+ .. code:: diff
+
+ #######################################################################
+ # PART 1 Settings #
+ #######################################################################
+ # Scheduler & Optimizer
+ - accumulative_counts = 1
+ + accumulative_counts = MICRO_NUM # or GRADIENT_ACCUMULATION
+ - max_epochs = 1
+ + max_epochs = MAX_EPOCHS
+
+并行策略
+---------------
+
+ZeRO 系列显存优化
+~~~~~~~~~~~~~~~~~~~~~~~
+
+XTuner 支持使用 ZeRO 系列显存优化降低训练过程中的显存消耗:
+
+.. code:: bash
+
+ # 单卡
+ xtuner train ${CONFIG_NAME_OR_PATH} --deepspeed deepspeed_zero2
+ # 多卡
+ (DIST) NPROC_PER_NODE=${GPU_NUM} xtuner train ${CONFIG_NAME_OR_PATH} --deepspeed deepspeed_zero2
+ (SLURM) srun ${SRUN_ARGS} xtuner train ${CONFIG_NAME_OR_PATH} --launcher slurm --deepspeed deepspeed_zero2
+
+
+序列并行
+~~~~~~~~~~~~~~~~~~~
+
+InternEvo 中支持了 Data Parallel、Tensor Parallel、Pipeline Parallel 和
+Sequence Parallel 四种并行策略。XTuner 目前支持了 Data Parallel 和
+Sequence Parallel 两种并行策略,可满足基本全部的训练需求(搭配 zero3
+显存优化策略可支持 70B 模型 256K 上下文训练)。
+
+假定 InternEvo 训练过程中:tp_world_size = TP, pp_world_size = PP,
+sequence_parallel = True。则训练的 global_batch_size 满足以下计算公式:
+
+.. code::
+
+ # 多除的一个 TP 是因为启用了 sequence parallel
+ global_batch_size = num_gpus * batch_size_per_device * gradient_accumulate / TP / PP / TP
+
+.. tip::
+ ``use_varlen_attn = True`` 时, ``batch_size_per_device`` 只能为 1,此时若想对齐
+ ``global_batch_size``,只需要在配置文件中综合调整
+ ``gradient_accumulate`` 和 ``sequence_parallel_size`` 两项的数值:
+
+.. code:: diff
+
+ + from xtuner.parallel.sequence import SequenceParallelSampler
+
+ + sequence_parallel_size = SP
+ - accumulative_counts = 1 # 1bs * 1acc * 64gpu = 64 batchsize
+ + accumulative_counts = TP * PP * TP / SP
+
+ #######################################################################
+ # PART 3 Dataset & Dataloader #
+ #######################################################################
+ train_dataloader = dict(
+ - sampler=dict(type=DefaultSampler, shuffle=True),
+ + sampler=dict(type=SequenceParallelSampler, shuffle=True),
+ ...)
diff --git a/docs/zh_cn/internevo_migration/ftdp_dataset/processed_and_internlm2.rst b/docs/zh_cn/internevo_migration/ftdp_dataset/processed_and_internlm2.rst
new file mode 100644
index 000000000..fcddad288
--- /dev/null
+++ b/docs/zh_cn/internevo_migration/ftdp_dataset/processed_and_internlm2.rst
@@ -0,0 +1,257 @@
+
+Processed 数据集 + InternLM2
+===================================
+
+.. warning::
+ 非 FTDP(一款闭源数据处理工具) 用户跳过此文档
+
+使用尚未 token 化的 ftdp 数据训练 InternLM2 模型的场景。
+
+步骤 1:离线处理数据集
+----------------------
+
+ftdp 把 sft
+任务的数据处理划分为三个类型,原始数据(origin)、预处理数据(processed)和
+token 过的数据(tokenized)。我们需要将预处理过的、具有统一格式的 ftdp
+数据 token
+化得到直接可以用于训练的格式。其中,预处理数据需要满足以下目录结构:
+
+.. code::
+
+ |-- processed-dir
+ |-- data1
+ | |-- processed
+ | |-- sft_chat
+ | |-- data1.jsonl
+ |-- data2
+ | |-- processed
+ | |-- sft_chat
+ | |-- data2.jsonl
+
+使用以下命令可离线 token 化 ftdp 格式的预处理数据(processed)数据集:
+
+.. code-block:: console
+
+ $ python xtuner/tools/tokenize_ftdp_datasets.py \
+ $ --processed-dir /path/to/preprocessed/data \
+ $ --tokenized-dir /path/to/tokenized/data \
+ $ --tokenizer-path pretrained_model_name_or_path
+
+.. note::
+ ``--processed-dir`` 需要指定预处理后的,具有 ftdp
+ 标准格式的数据路径
+
+.. note::
+ ``--tokenized-dir`` 需要指定为 token 化后的数据存储路径
+
+.. note::
+ ``--tokenizer-path pretrained_model_name_or_path`` 中的
+ ``pretrained_model_name_or_path`` 同 ``from_pretrained`` 接口中的
+ ``pretrained_model_name_or_path``\
+
+.. note::
+ 上述命令执行成功后,会在 ``/path/to/tokenized/data/chatml_llamav13_32k``
+ 路径下保存两个子文件夹——``train`` 和 ``valid``\ 。
+
+步骤 2:导出模板 config 文件
+----------------------------
+
+XTuner 中目前提供了训练 InternLM2 的模板 config,使用命令:
+
+.. code-block:: console
+
+ $ xtuner copy-cfg internlm2_7b_w_tokenized_dataset .
+
+.. note::
+ 当前目录下会有一个名为 ``internlm2_7b_w_tokenized_dataset_copy.py`` 的新文件
+
+步骤 3:修改模板 config 文件
+----------------------------
+
+修改模板 config 文件中的训练数据路径为真实数据路径,其中
+``/path/to/tokenized/data`` 与步骤 1 中的 ``/path/to/tokenized/data``
+为同一个路径:
+
+.. code:: diff
+
+ ...
+
+ #######################################################################
+ # PART 1 Settings #
+ #######################################################################
+ # Model
+ pretrained_model_name_or_path = 'internlm/internlm2-7b'
+ use_varlen_attn = True
+
+ # Data
+ - dataset_folder = '/path/to/sft/data/folder'
+ + dataset_folder = '/path/to/tokenized/data/chatml_llamav13_32k/train'
+ prompt_template = PROMPT_TEMPLATE.internlm2_chat
+ max_length = 32768
+ pack_to_max_length = True
+ ...
+
+.. tip::
+ 在使用 DeepSpeed 训练模型时,如需在保存 checkpoint
+ 时只保存模型权重,而不保存优化器状态,可参考以下步骤:
+
+ 1. 确保 mmengine 版本大于等于 0.10.3
+
+ .. code-block:: console
+
+ $ pip install 'mmengine>=0.10.3'
+
+ 2. 修改 Config 文件,CheckpointHook 增加 save_optimizer=False
+
+ .. code:: diff
+
+ default_hooks = dict(
+ # record the time of every iteration.
+ timer=dict(type=IterTimerHook),
+ # print log every 100 iterations.
+ logger=dict(type=LoggerHook, interval=1),
+ # enable the parameter scheduler.
+ param_scheduler=dict(type=ParamSchedulerHook),
+ # save checkpoint per epoch.
+ checkpoint=dict(
+ type=CheckpointHook,
+ + save_optimizer=False,
+ by_epoch=False,
+ interval=save_steps,
+ max_keep_ckpts=save_total_limit),
+ # set sampler seed in distributed evrionment.
+ sampler_seed=dict(type=DistSamplerSeedHook),
+ )
+
+.. warning::
+
+ 设置 ``save_optimizer=False`` 后,训练过程不可 resume 。
+
+
+步骤 4:获取数据顺序 (可选)
+-----------------------------
+
+训练数据的提供顺序可能会对模型的最终训练成果产生影响。鉴于不同集群中通过
+``os.walk``
+方法所得到的结果可能存在差异,为了确保训练结果的稳定性和可控性,建议首先确立所有训练数据文件的相对次序,并在后续的训练阶段中,使用这一相对次序来替代
+``os.walk`` 方法。
+
+运行下面的代码可获取数据顺序,并存为 txt 文件:
+
+.. code-block:: console
+
+ $ python xtuner/tools/get_data_order.py \
+ $ --data-folder /path/to/tokenized/data \
+ $ --save-folder /folder/to/save/data/order \
+ $ --file-type ${file_type}
+
+.. tip::
+ ``--file-type ${file_type}`` 表示需要统计所有以 ``${file_type}``
+ 为文件名后缀的文件的顺序。
+
+ 例如,需要获取 ``/path/to/tokenized/data`` 路径下所有以 ``.bin``
+ 结尾的文件的顺序,并保存在当前路径下,那么上述命令需要改为:
+
+ .. code-block:: console
+
+ $ python xtuner/tools/get_data_order.py \
+ $ --data-folder /path/to/tokenized/data \
+ $ --save-folder . \
+ $ --file-type .bin
+
+获得数据顺序文件后,还需要在 config 中设置数据顺序文件路径:
+
+.. code:: diff
+
+ ...
+ #######################################################################
+ # PART 3 Dataset & Dataloader #
+ #######################################################################
+ train_dataset = dict(
+ type=build_packed_dataset,
+ dataset_cfg=dict(
+ type=load_intern_repo_tokenized_dataset,
+ - data_order_path=None,
+ + data_order_path='/folder/to/save/data/order/'+'data_order.txt',
+ folder=dataset_folder,
+ min_length=0,
+ file_type='.bin'
+ ),
+ packed_length=max_length,
+ seed=1024)
+
+
+步骤 5:启动训练
+----------------
+
+在 slurm 集群调度系统中可以通过以下命令启动训练:
+
+.. code-block:: console
+
+ $ srun ${SRUN_ARGS} xtuner train internlm2_7b_w_tokenized_dataset_copy.py --launcher slurm --deepspeed deepspeed_zero1
+
+若出现 OOM 现象,可尝试使用 zero2 或 zero3。以下命令可以使用 zero 3
+显存优化策略进行训练:
+
+.. code-block:: console
+
+ $ srun ${SRUN_ARGS} xtuner train internlm2_7b_w_tokenized_dataset_copy.py --launcher slurm --deepspeed deepspeed_zero3
+
+在阿里云 DLC 中可通过以下命令启动训练:
+
+.. code:: diff
+
+ export NCCL_IB_TC=136
+ export NCCL_IB_SL=5
+ export NCCL_IB_GID_INDEX=3
+ export NCCL_SOCKET_IFNAME=bond0
+ export NCCL_DEBUG=INFO
+ export NCCL_IB_HCA=mlx5
+ export NCCL_IB_TIMEOUT=22
+ export NCCL_IB_QPS_PER_CONNECTION=8
+ export NCCL_NET_PLUGIN=none
+
+ export NCCL_BUFFSIZE=2097152
+ export PYTORCH_CUDA_ALLOC_CONF=max_split_size_mb:512
+ - export EXP_NAME=debug
+ + export EXP_NAME=your_exp_name
+ export PYTHONPATH='.':$PYTHONPATH
+ source ~/.bashrc
+ + cd /path/to/xtuner
+ + conda activate conda_env_name
+
+ export NPROC_PER_NODE=${KUBERNETES_CONTAINER_RESOURCE_GPU}
+ export PORT=${MASTER_PORT}
+ export NNODES=${WORLD_SIZE}
+ export NODE_RANK=${RANK}
+ export ADDR=${MASTER_ADDR}
+
+ echo ${KUBERNETES_CONTAINER_RESOURCE_GPU}
+ echo ${WORLD_SIZE}
+ echo ${MASTER_PORT}
+ echo ${MASTER_ADDR}
+ echo ${RANK}
+ xtuner train internlm2_7b_w_tokenized_dataset_copy.py \
+ --deepspeed deepspeed_zero1 \
+ --work-dir work_dirs/${EXP_NAME}
+
+步骤 6:转模型
+--------------
+
+deepspeed 转 hf:
+
+.. code-block:: console
+
+ $ python xtuner/tools/model_converters/pth_to_hf.py internlm2_7b_w_tokenized_dataset_copy.py /src/model/path /hf/dst/model/path
+
+hf 转 Turbomind:
+
+.. code-block:: console
+
+ $ lmdeploy convert internlm2-chat-7b /hf/dst/model/path --dst-path /turbomind/dst/model/path
+
+步骤 7:Turbomind 评测
+----------------------
+
+请参考 `OpenCompass LMDeploy
+评测文档 `__\ 。
diff --git a/docs/zh_cn/internevo_migration/ftdp_dataset/processed_and_others.rst b/docs/zh_cn/internevo_migration/ftdp_dataset/processed_and_others.rst
new file mode 100644
index 000000000..6a472d1e7
--- /dev/null
+++ b/docs/zh_cn/internevo_migration/ftdp_dataset/processed_and_others.rst
@@ -0,0 +1,292 @@
+.. _case2:
+
+Processed 数据集 + 其他模型
+==========================================
+
+.. warning::
+ 非 FTDP(一款闭源数据处理工具) 用户跳过此文档
+
+
+使用尚未 token 化的 ftdp 数据训练其他模型(以 Mistral 为例),且需要用
+Internlm2 对话模板覆盖原有对话模板以便让模型掌握 agent 、tool 能力。
+
+步骤 1:离线处理数据集
+----------------------
+
+ftdp 把 sft
+任务的数据处理划分为三个类型,原始数据(origin)、预处理数据(processed)和
+token 过的数据(tokenized)。我们需要将预处理过的、具有统一格式的 ftdp
+数据 token
+化得到直接可以用于训练的格式。其中,预处理数据需要满足以下目录结构:
+
+.. code::
+
+ |-- processed-dir
+ |-- data1
+ | |-- processed
+ | |-- sft_chat
+ | |-- data1.jsonl
+ |-- data2
+ | |-- processed
+ | |-- sft_chat
+ | |-- data2.jsonl
+
+使用以下命令可离线 token 化 ftdp 格式的预处理数据(processed)数据集:
+
+.. code-block:: console
+
+ $ python xtuner/tools/tokenize_ftdp_datasets.py \
+ $ --processed-dir /path/to/preprocessed/data \
+ $ --tokenized-dir /path/to/tokenized/data \
+ $ --tokenizer-path pretrained_model_name_or_path
+
+.. note::
+ ``--processed-dir`` 需要指定预处理后的,具有 ftdp
+ 标准格式的数据路径
+
+.. note::
+ ``--tokenized-dir`` 需要指定为 token 化后的数据存储路径
+
+.. note::
+ ``--tokenizer-path pretrained_model_name_or_path`` 中的
+ ``pretrained_model_name_or_path`` 同 ``from_pretrained`` 接口中的
+ ``pretrained_model_name_or_path``\
+
+.. note::
+ 上述命令执行成功后,会在 ``/path/to/tokenized/data/chatml_llamav13_32k``
+ 路径下保存两个子文件夹——``train`` 和 ``valid``\ 。
+
+.. warning::
+ 由于除 Internlm2 外的其他模型(如 mistral 等)没有 internlm2-chat
+ 模型的智能体、工具调用等功能的对话模板,因此对于非 internlm2
+ 模型,需要将 internlm2-chat
+ 对话模板中的一些特殊字符(如:<\|im_start\|>、<\|plugin\|>等)加入到新模型的
+ tokenizer 的 special tokens 中,需要通过
+ ``--tokenizer-w-special-tokens-save-dir`` 指定新 tokenizer
+ 的存储路径。\ **同时,后续训练过程需要使用新保存的 tokenizer 而非原始
+ tokenizer。**
+
+步骤 2:导出模板 config 文件
+----------------------------
+
+XTuner 中目前提供了训练 Mistral 的模板 config,使用命令:
+
+.. code-block:: console
+
+ $ xtuner copy-cfg mistral_7b_w_tokenized_dataset .
+
+.. note::
+ 当前目录下会有一个名为 ``mistral_7b_w_tokenized_dataset_copy.py`` 的新文件
+
+
+步骤 3:修改模板 config 文件
+----------------------------
+
+.. note::
+ 修改模板 config 文件中的训练数据路径为真实数据路径,其中 `/path/to/tokenized/data` 需要基于 Step 1 中的 `/path/to/tokenized/data` 进一步指定 train folder,即 `/path/to/tokenized/data/chatml_llamav13_32k/train/` 。
+
+.. note::
+ 需要修改 tokenizer 路径为步骤 1 保存的路径 `/path/to/save/new/tokenizer`。
+
+.. warning::
+ 由于步骤 1 扩充了 tokenizer 的词表,因此需要将新 tokenizer 传入 `SupervisedFinetune` 中,以扩展语言模型的词表大小。
+
+.. code:: diff
+
+ ...
+
+ #######################################################################
+ # PART 1 Settings #
+ #######################################################################
+ # Model
+ pretrained_model_name_or_path = 'mistralai/Mistral-7B-v0.1'
+ # 已经使用 Internlm2 的对话模板覆盖了 Mistral 的原有模板,new tokenizer 中已经
+ # 添加了 Internlm2 对话模板中的特殊字符。
+ # 请参考 docs/zh_cn/user_guides/finetune_custom_dataset.md
+ - tokenizer_path = '/new/tokenizer/path'
+ + tokenizer_path = '/path/to/save/new/tokenizer'
+ use_varlen_attn = True
+
+ # Data
+ - dataset_folder = '/path/to/sft/data/folder'
+ + dataset_folder = '/path/to/tokenized/data/chatml_llamav13_32k/train'
+ # 已经使用 Internlm2 的对话模板覆盖了 Mistral 的原有模板
+ prompt_template = PROMPT_TEMPLATE.internlm2_chat
+ max_length = 32768
+ pack_to_max_length = True
+ ...
+
+ #######################################################################
+ # PART 2 Model & Tokenizer #
+ #######################################################################
+ model = dict(
+ + tokenizer=tokenizer,
+ ...)
+
+.. tip::
+ 在使用 DeepSpeed 训练模型时,如需在保存 checkpoint
+ 时只保存模型权重,而不保存优化器状态,可参考以下步骤:
+
+ 1. 确保 mmengine 版本大于等于 0.10.3
+
+ .. code-block:: console
+
+ $ pip install 'mmengine>=0.10.3'
+
+ 2. 修改 Config 文件,CheckpointHook 增加 save_optimizer=False
+
+ .. code:: diff
+
+ default_hooks = dict(
+ # record the time of every iteration.
+ timer=dict(type=IterTimerHook),
+ # print log every 100 iterations.
+ logger=dict(type=LoggerHook, interval=1),
+ # enable the parameter scheduler.
+ param_scheduler=dict(type=ParamSchedulerHook),
+ # save checkpoint per epoch.
+ checkpoint=dict(
+ type=CheckpointHook,
+ + save_optimizer=False,
+ by_epoch=False,
+ interval=save_steps,
+ max_keep_ckpts=save_total_limit),
+ # set sampler seed in distributed evrionment.
+ sampler_seed=dict(type=DistSamplerSeedHook),
+ )
+
+.. warning::
+
+ 设置 ``save_optimizer=False`` 后,训练过程不可 resume 。
+
+
+步骤 4:获取数据顺序 (可选)
+-----------------------------
+
+训练数据的提供顺序可能会对模型的最终训练成果产生影响。鉴于不同集群中通过
+``os.walk``
+方法所得到的结果可能存在差异,为了确保训练结果的稳定性和可控性,建议首先确立所有训练数据文件的相对次序,并在后续的训练阶段中,使用这一相对次序来替代
+``os.walk`` 方法。
+
+运行下面的代码可获取数据顺序,并存为 txt 文件:
+
+.. code-block:: console
+
+ $ python xtuner/tools/get_data_order.py \
+ $ --data-folder /path/to/tokenized/data \
+ $ --save-folder /folder/to/save/data/order \
+ $ --file-type ${file_type}
+
+.. tip::
+ ``--file-type ${file_type}`` 表示需要统计所有以 ``${file_type}``
+ 为文件名后缀的文件的顺序。
+
+ 例如,需要获取 ``/path/to/tokenized/data`` 路径下所有以 ``.bin``
+ 结尾的文件的顺序,并保存在当前路径下,那么上述命令需要改为:
+
+ .. code-block:: console
+
+ $ python xtuner/tools/get_data_order.py \
+ $ --data-folder /path/to/tokenized/data \
+ $ --save-folder . \
+ $ --file-type .bin
+
+获得数据顺序文件后,还需要在 config 中设置数据顺序文件路径:
+
+.. code:: diff
+
+ ...
+ #######################################################################
+ # PART 3 Dataset & Dataloader #
+ #######################################################################
+ train_dataset = dict(
+ type=build_packed_dataset,
+ dataset_cfg=dict(
+ type=load_intern_repo_tokenized_dataset,
+ - data_order_path=None,
+ + data_order_path='/folder/to/save/data/order/'+'data_order.txt',
+ folder=dataset_folder,
+ min_length=0,
+ file_type='.bin'
+ ),
+ packed_length=max_length,
+ seed=1024)
+
+
+步骤 5:启动训练
+----------------
+
+注:训练前期(几十个 iters)loss 偏高是正常现象,因为模型需要时间学习
+Internlm2 的对话模板。
+
+在 slurm 集群调度系统中可以通过以下命令启动训练:
+
+.. code-block:: console
+
+ $ srun ${SRUN_ARGS} xtuner train mistral_7b_w_tokenized_dataset_copy.py --launcher slurm --deepspeed deepspeed_zero1
+
+若出现 OOM 现象,可尝试使用 zero2 或 zero3。以下命令可以使用 zero 3
+显存优化策略进行训练:
+
+.. code-block:: console
+
+ $ srun ${SRUN_ARGS} xtuner train internlm2_7b_w_tokenized_dataset_copy.py --launcher slurm --deepspeed deepspeed_zero3
+
+在阿里云 DLC 中可通过以下命令启动训练:
+
+.. code:: diff
+
+ export NCCL_IB_TC=136
+ export NCCL_IB_SL=5
+ export NCCL_IB_GID_INDEX=3
+ export NCCL_SOCKET_IFNAME=bond0
+ export NCCL_DEBUG=INFO
+ export NCCL_IB_HCA=mlx5
+ export NCCL_IB_TIMEOUT=22
+ export NCCL_IB_QPS_PER_CONNECTION=8
+ export NCCL_NET_PLUGIN=none
+
+ export NCCL_BUFFSIZE=2097152
+ export PYTORCH_CUDA_ALLOC_CONF=max_split_size_mb:512
+ - export EXP_NAME=debug
+ + export EXP_NAME=your_exp_name
+ export PYTHONPATH='.':$PYTHONPATH
+ source ~/.bashrc
+ + cd /path/to/xtuner
+ + conda activate conda_env_name
+
+ export NPROC_PER_NODE=${KUBERNETES_CONTAINER_RESOURCE_GPU}
+ export PORT=${MASTER_PORT}
+ export NNODES=${WORLD_SIZE}
+ export NODE_RANK=${RANK}
+ export ADDR=${MASTER_ADDR}
+
+ echo ${KUBERNETES_CONTAINER_RESOURCE_GPU}
+ echo ${WORLD_SIZE}
+ echo ${MASTER_PORT}
+ echo ${MASTER_ADDR}
+ echo ${RANK}
+ xtuner train mistral_7b_w_tokenized_dataset_copy.py \
+ --deepspeed deepspeed_zero1 \
+ --work-dir work_dirs/${EXP_NAME}
+
+Step 6, 转模型
+--------------
+
+deepspeed 转 hf:
+
+.. code-block:: console
+
+ $ python xtuner/tools/model_converters/pth_to_hf.py mistral_7b_w_tokenized_dataset_copy.py /src/model/path /hf/dst/model/path
+
+hf 转 Turbomind:
+
+.. code-block:: console
+
+ $ lmdeploy convert internlm2-chat-7b /hf/dst/model/path --dst-path /turbomind/dst/model/path
+
+Step 7,Turbomind 评测
+----------------------
+
+请参考 `OpenCompass LMDeploy
+评测文档 `__\ 。
diff --git a/docs/zh_cn/internevo_migration/ftdp_dataset/processed_normal_chat.rst b/docs/zh_cn/internevo_migration/ftdp_dataset/processed_normal_chat.rst
new file mode 100644
index 000000000..c3882b515
--- /dev/null
+++ b/docs/zh_cn/internevo_migration/ftdp_dataset/processed_normal_chat.rst
@@ -0,0 +1,171 @@
+.. _case3:
+
+Processed 普通对话数据集
+=======================================
+
+.. warning::
+ 非 FTDP(一款闭源数据处理工具) 用户跳过此文档
+
+使用尚未 token 化的 ftdp
+数据进行训练,保持待训练模型的对话模板不变,且不需要进行离线处理的场景。
+
+步骤 1:导出模板 config 文件
+----------------------------
+
+XTuner 中目前提供了训练 Internlm2 的模板 config,使用命令:
+
+.. code-block:: console
+
+ $ xtuner copy-cfg internlm2_7b_w_untokenized_dataset .
+
+.. note::
+ 当前目录下会有一个名为 ``internlm2_7b_w_untokenized_dataset_copy.py`` 的新文件
+
+
+步骤 2:修改模板 config 文件
+----------------------------
+
+修改模板 config 文件中的训练数据路径为真实数据路径,路径中的所有以
+``.json`` 为后缀的数据将会作为训练数据:
+
+.. code:: diff
+
+ ...
+
+ #######################################################################
+ # PART 1 Settings #
+ #######################################################################
+ # Model
+ pretrained_model_name_or_path = 'internlm/internlm2-7b'
+ use_varlen_attn = True
+
+ # Data
+ - dataset_folder = '/mnt/petrelfs/share_data/caoweihan/v1_sample_with_legal_cate'
+ + dataset_folder = '/path/to/untokenized/data'
+ prompt_template = PROMPT_TEMPLATE.internlm2_chat
+ max_length = 32768
+ pack_to_max_length = True
+ ...
+
+.. _step-3-获取数据顺序-可选):
+
+步骤 3:获取数据顺序 (可选)
+-----------------------------
+
+训练数据的提供顺序可能会对模型的最终训练成果产生影响。鉴于不同集群中通过
+``os.walk``
+方法所得到的结果可能存在差异,为了确保训练结果的稳定性和可控性,建议首先确立所有训练数据文件的相对次序,并在后续的训练阶段中,使用这一相对次序来替代
+``os.walk`` 方法。
+
+运行下面的代码可获取数据顺序,并存为 txt 文件:
+
+.. code-block:: console
+
+ $ python xtuner/tools/get_data_order.py \
+ $ --data-folder /path/to/tokenized/data \
+ $ --save-folder /folder/to/save/data/order \
+ $ --file-type ${file_type}
+
+.. tip::
+ ``--file-type ${file_type}`` 表示需要统计所有以 ``${file_type}``
+ 为文件名后缀的文件的顺序。
+
+ 例如,需要获取 ``/path/to/tokenized/data`` 路径下所有以 ``.bin``
+ 结尾的文件的顺序,并保存在当前路径下,那么上述命令需要改为:
+
+ .. code-block:: console
+
+ $ python xtuner/tools/get_data_order.py \
+ $ --data-folder /path/to/tokenized/data \
+ $ --save-folder . \
+ $ --file-type .bin
+
+获得数据顺序文件后,还需要在 config 中设置数据顺序文件路径:
+
+.. code:: diff
+
+ ...
+ #######################################################################
+ # PART 3 Dataset & Dataloader #
+ #######################################################################
+ train_dataset = dict(
+ type=build_packed_dataset,
+ dataset_cfg=dict(
+ type=load_intern_repo_tokenized_dataset,
+ - data_order_path=None,
+ + data_order_path='/folder/to/save/data/order/'+'data_order.txt',
+ folder=dataset_folder,
+ min_length=0,
+ file_type='.bin'
+ ),
+ packed_length=max_length,
+ seed=1024)
+
+步骤 4:启动训练
+----------------
+
+在 slurm 集群调度系统中可以通过以下命令启动训练:
+
+.. code-block:: console
+
+ $ srun ${SRUN_ARGS} xtuner train internlm2_7b_w_untokenized_dataset_copy.py --launcher slurm --deepspeed deepspeed_zero1
+
+若出现 OOM 现象,可尝试使用 zero2 或 zero3。以下命令可以使用 zero 3
+显存优化策略进行训练:
+
+.. code-block:: console
+
+ $ srun ${SRUN_ARGS} xtuner train internlm2_7b_w_tokenized_dataset_copy.py --launcher slurm --deepspeed deepspeed_zero3
+
+在阿里云 DLC 中可通过以下命令启动训练:
+
+.. code:: diff
+
+ export NCCL_IB_TC=136
+ export NCCL_IB_SL=5
+ export NCCL_IB_GID_INDEX=3
+ export NCCL_SOCKET_IFNAME=bond0
+ export NCCL_DEBUG=INFO
+ export NCCL_IB_HCA=mlx5
+ export NCCL_IB_TIMEOUT=22
+ export NCCL_IB_QPS_PER_CONNECTION=8
+ export NCCL_NET_PLUGIN=none
+
+ export NCCL_BUFFSIZE=2097152
+ export PYTORCH_CUDA_ALLOC_CONF=max_split_size_mb:512
+ - export EXP_NAME=debug
+ + export EXP_NAME=your_exp_name
+ export PYTHONPATH='.':$PYTHONPATH
+ source ~/.bashrc
+ + cd /path/to/xtuner
+ + conda activate conda_env_name
+
+ export NPROC_PER_NODE=${KUBERNETES_CONTAINER_RESOURCE_GPU}
+ export PORT=${MASTER_PORT}
+ export NNODES=${WORLD_SIZE}
+ export NODE_RANK=${RANK}
+ export ADDR=${MASTER_ADDR}
+
+ echo ${KUBERNETES_CONTAINER_RESOURCE_GPU}
+ echo ${WORLD_SIZE}
+ echo ${MASTER_PORT}
+ echo ${MASTER_ADDR}
+ echo ${RANK}
+ xtuner train internlm2_7b_w_untokenized_dataset_copy.py \
+ --deepspeed deepspeed_zero1 \
+ --work-dir work_dirs/${EXP_NAME}
+
+步骤 5:转模型
+--------------
+
+deepspeed 转 hf:
+
+.. code::
+
+ python xtuner/tools/model_converters/pth_to_hf.py internlm2_7b_w_untokenized_dataset_copy.py /src/model/path /hf/dst/model/path
+
+hf 转 Turbomind:
+
+.. code::
+
+ lmdeploy convert internlm2-chat-7b /hf/dst/model/path --dst-path /turbomind/dst/model/path
diff --git a/docs/zh_cn/internevo_migration/ftdp_dataset/tokenized_and_internlm2.rst b/docs/zh_cn/internevo_migration/ftdp_dataset/tokenized_and_internlm2.rst
new file mode 100644
index 000000000..d905aae57
--- /dev/null
+++ b/docs/zh_cn/internevo_migration/ftdp_dataset/tokenized_and_internlm2.rst
@@ -0,0 +1,208 @@
+Tokenized 数据集 + InternLM2
+===================================
+
+.. tip::
+ Tokenized 数据集格式应与 `InternEVO 使用教程 `_ 中保持一致
+
+使用已经 token 化的 ftdp 数据训练 Internlm2 模型。
+
+步骤 1:导出模板 config 文件
+----------------------------
+
+XTuner 中目前提供了训练 Internlm2 的模板 config,使用命令:
+
+.. code-block:: console
+
+ $ xtuner copy-cfg internlm2_7b_w_tokenized_dataset .
+
+.. note::
+ 当前目录下会有一个名为 ``internlm2_7b_w_tokenized_dataset_copy.py`` 的新文件
+
+步骤 2:修改模板 config 文件
+----------------------------
+
+修改模板 config 文件中的训练数据路径为真实数据路径:
+
+.. code-block:: diff
+
+ ...
+
+ #######################################################################
+ # PART 1 Settings #
+ #######################################################################
+ # Model
+ pretrained_model_name_or_path = 'internlm/internlm2-7b'
+ use_varlen_attn = True
+
+ # Data
+ - dataset_folder = '/path/to/sft/data/folder'
+ + dataset_folder = '/path/to/tokenized/data/chatml_llamav13_32k/train'
+ prompt_template = PROMPT_TEMPLATE.internlm2_chat
+ max_length = 32768
+ pack_to_max_length = True
+ ...
+
+.. tip::
+ 在使用 DeepSpeed 训练模型时,如需在保存 checkpoint
+ 时只保存模型权重,而不保存优化器状态,可参考以下步骤:
+
+ 1. 确保 mmengine 版本大于等于 0.10.3
+
+ .. code-block:: console
+
+ $ pip install 'mmengine>=0.10.3'
+
+ 2. 修改 Config 文件,CheckpointHook 增加 save_optimizer=False
+
+ .. code:: diff
+
+ default_hooks = dict(
+ # record the time of every iteration.
+ timer=dict(type=IterTimerHook),
+ # print log every 100 iterations.
+ logger=dict(type=LoggerHook, interval=1),
+ # enable the parameter scheduler.
+ param_scheduler=dict(type=ParamSchedulerHook),
+ # save checkpoint per epoch.
+ checkpoint=dict(
+ type=CheckpointHook,
+ + save_optimizer=False,
+ by_epoch=False,
+ interval=save_steps,
+ max_keep_ckpts=save_total_limit),
+ # set sampler seed in distributed evrionment.
+ sampler_seed=dict(type=DistSamplerSeedHook),
+ )
+
+.. warning::
+
+ 设置 ``save_optimizer=False`` 后,训练过程不可 resume 。
+
+.. _case4-step3:
+
+步骤 3:获取数据顺序 (可选)
+-----------------------------
+
+训练数据的提供顺序可能会对模型的最终训练成果产生影响。鉴于不同集群中通过
+``os.walk``
+方法所得到的结果可能存在差异,为了确保训练结果的稳定性和可控性,建议首先确立所有训练数据文件的相对次序,并在后续的训练阶段中,使用这一相对次序来替代
+``os.walk`` 方法。
+
+运行下面的代码可获取数据顺序,并存为 txt 文件:
+
+.. code-block:: console
+
+ $ python xtuner/tools/get_data_order.py \
+ $ --data-folder /path/to/tokenized/data \
+ $ --save-folder /folder/to/save/data/order \
+ $ --file-type ${file_type}
+
+.. tip::
+ ``--file-type ${file_type}`` 表示需要统计所有以 ``${file_type}``
+ 为文件名后缀的文件的顺序。
+
+ 例如,需要获取 ``/path/to/tokenized/data`` 路径下所有以 ``.bin``
+ 结尾的文件的顺序,并保存在当前路径下,那么上述命令需要改为:
+
+ .. code-block:: console
+
+ $ python xtuner/tools/get_data_order.py \
+ $ --data-folder /path/to/tokenized/data \
+ $ --save-folder . \
+ $ --file-type .bin
+
+获得数据顺序文件后,还需要在 config 中设置数据顺序文件路径:
+
+.. code:: diff
+
+ ...
+ #######################################################################
+ # PART 3 Dataset & Dataloader #
+ #######################################################################
+ train_dataset = dict(
+ type=build_packed_dataset,
+ dataset_cfg=dict(
+ type=load_intern_repo_tokenized_dataset,
+ - data_order_path=None,
+ + data_order_path='/folder/to/save/data/order/'+'data_order.txt',
+ folder=dataset_folder,
+ min_length=0,
+ file_type='.bin'
+ ),
+ packed_length=max_length,
+ seed=1024)
+
+步骤 4:启动训练
+----------------
+
+在 slurm 集群调度系统中可以通过以下命令启动训练:
+
+.. code-block:: console
+
+ $ srun ${SRUN_ARGS} xtuner train internlm2_7b_w_tokenized_dataset_copy.py --launcher slurm --deepspeed deepspeed_zero1
+
+若出现 OOM 现象,可尝试使用 zero2 或 zero3。以下命令可以使用 zero 3
+显存优化策略进行训练:
+
+.. code-block:: console
+
+ $ srun ${SRUN_ARGS} xtuner train internlm2_7b_w_tokenized_dataset_copy.py --launcher slurm --deepspeed deepspeed_zero3
+
+在阿里云 DLC 中可通过以下命令启动训练:
+
+.. code:: diff
+
+ export NCCL_IB_TC=136
+ export NCCL_IB_SL=5
+ export NCCL_IB_GID_INDEX=3
+ export NCCL_SOCKET_IFNAME=bond0
+ export NCCL_DEBUG=INFO
+ export NCCL_IB_HCA=mlx5
+ export NCCL_IB_TIMEOUT=22
+ export NCCL_IB_QPS_PER_CONNECTION=8
+ export NCCL_NET_PLUGIN=none
+
+ export NCCL_BUFFSIZE=2097152
+ export PYTORCH_CUDA_ALLOC_CONF=max_split_size_mb:512
+ - export EXP_NAME=debug
+ + export EXP_NAME=your_exp_name
+ export PYTHONPATH='.':$PYTHONPATH
+ source ~/.bashrc
+ + cd /path/to/xtuner
+ + conda activate conda_env_name
+
+ export NPROC_PER_NODE=${KUBERNETES_CONTAINER_RESOURCE_GPU}
+ export PORT=${MASTER_PORT}
+ export NNODES=${WORLD_SIZE}
+ export NODE_RANK=${RANK}
+ export ADDR=${MASTER_ADDR}
+
+ echo ${KUBERNETES_CONTAINER_RESOURCE_GPU}
+ echo ${WORLD_SIZE}
+ echo ${MASTER_PORT}
+ echo ${MASTER_ADDR}
+ echo ${RANK}
+ xtuner train internlm2_7b_w_tokenized_dataset_copy.py \
+ --deepspeed deepspeed_zero1 \
+ --work-dir work_dirs/${EXP_NAME}
+
+步骤 5:转模型
+--------------
+
+deepspeed 转 hf:
+
+.. code-block:: console
+
+ $ python xtuner/tools/model_converters/pth_to_hf.py internlm2_7b_w_tokenized_dataset_copy.py /src/model/path /hf/dst/model/path
+
+hf 转 Turbomind:
+
+.. code-block:: console
+
+ $ lmdeploy convert internlm2-chat-7b /hf/dst/model/path --dst-path /turbomind/dst/model/path
+
+步骤 6:Turbomind 评测
+----------------------
+
+请参考 `OpenCompass LMDeploy
+评测文档 `__\ 。
diff --git a/docs/zh_cn/make.bat b/docs/zh_cn/make.bat
new file mode 100644
index 000000000..954237b9b
--- /dev/null
+++ b/docs/zh_cn/make.bat
@@ -0,0 +1,35 @@
+@ECHO OFF
+
+pushd %~dp0
+
+REM Command file for Sphinx documentation
+
+if "%SPHINXBUILD%" == "" (
+ set SPHINXBUILD=sphinx-build
+)
+set SOURCEDIR=.
+set BUILDDIR=_build
+
+%SPHINXBUILD% >NUL 2>NUL
+if errorlevel 9009 (
+ echo.
+ echo.The 'sphinx-build' command was not found. Make sure you have Sphinx
+ echo.installed, then set the SPHINXBUILD environment variable to point
+ echo.to the full path of the 'sphinx-build' executable. Alternatively you
+ echo.may add the Sphinx directory to PATH.
+ echo.
+ echo.If you don't have Sphinx installed, grab it from
+ echo.https://www.sphinx-doc.org/
+ exit /b 1
+)
+
+if "%1" == "" goto help
+
+%SPHINXBUILD% -M %1 %SOURCEDIR% %BUILDDIR% %SPHINXOPTS% %O%
+goto end
+
+:help
+%SPHINXBUILD% -M help %SOURCEDIR% %BUILDDIR% %SPHINXOPTS% %O%
+
+:end
+popd
diff --git a/docs/zh_cn/models/supported.md b/docs/zh_cn/models/supported.md
new file mode 100644
index 000000000..df7ecaa58
--- /dev/null
+++ b/docs/zh_cn/models/supported.md
@@ -0,0 +1 @@
+# 已支持的模型
diff --git a/docs/zh_cn/preparation/pretrained_model.rst b/docs/zh_cn/preparation/pretrained_model.rst
new file mode 100644
index 000000000..727372ffd
--- /dev/null
+++ b/docs/zh_cn/preparation/pretrained_model.rst
@@ -0,0 +1,143 @@
+==================
+预训练模型权重
+==================
+
+``HuggingFace`` 和 ``ModelScope``
+提供了多种下载预训练模型权重的方法,本节将以下载 internlm2-chat-7b
+为例,介绍如何快速下载预训练模型的权重。
+
+.. note::
+
+ 若 HuggingFace 访问受限,请优先考虑使用 ModelScope 进行下载
+
+
+[推荐] 方法 1:``snapshot_download``
+========================================
+
+
+HuggingFace
+------------
+
+``huggingface_hub.snapshot_download`` 支持下载特定的 HuggingFace Hub
+模型权重,并且允许多线程。您可以利用下列代码并行下载模型权重:
+
+.. code:: python
+
+ from huggingface_hub import snapshot_download
+
+ snapshot_download(repo_id='internlm/internlm2-chat-7b', local_dir='./internlm2-chat-7b', max_workers=20)
+
+.. note::
+
+ 其中,\ ``repo_id`` 表示模型在 HuggingFace Hub 的名字、\ ``local_dir`` 表示期望存储到的本地路径、\ ``max_workers`` 表示下载的最大并行数。
+
+.. tip::
+
+ 如果未指定 ``local_dir``\ ,则将下载至 HuggingFace 的默认 cache 路径中(\ ``~/.cache/huggingface/hub``\ )。若要修改默认 cache 路径,需要修改相关环境变量:
+
+ .. code:: console
+
+ $ # 默认为 `~/.cache/huggingface/`
+ $ export HF_HOME=XXXX
+
+.. tip::
+ 如果觉得下载较慢(例如无法达到最大带宽等情况),可以尝试设置\ ``export HF_HUB_ENABLE_HF_TRANSFER=1`` 以获得更高的下载速度。
+
+.. tip::
+ 关于环境变量的更多用法,可阅读\ `这里 `__ 。
+
+
+ModelScope
+-----------
+
+``modelscope.snapshot_download``
+支持下载指定的模型权重,您可以利用下列命令下载模型:
+
+.. code:: python
+
+ from modelscope import snapshot_download
+
+ snapshot_download(model_id='Shanghai_AI_Laboratory/internlm2-chat-7b', cache_dir='./internlm2-chat-7b')
+
+.. note::
+ 其中,\ ``model_id`` 表示模型在 ModelScope 模型库的名字、\ ``cache_dir`` 表示期望存储到的本地路径。
+
+
+.. note::
+ ``modelscope.snapshot_download`` 不支持多线程并行下载。
+
+.. tip::
+
+ 如果未指定 ``cache_dir``\ ,则将下载至 ModelScope 的默认 cache 路径中(\ ``~/.cache/huggingface/hub``\ )。
+
+ 若要修改默认 cache 路径,需要修改相关环境变量:
+
+ .. code:: console
+
+ $ # 默认为 ~/.cache/modelscope/hub/
+ $ export MODELSCOPE_CACHE=XXXX
+
+
+
+方法 2: Git LFS
+===================
+
+HuggingFace 和 ModelScope 的远程模型仓库就是一个由 Git LFS 管理的 Git
+仓库。因此,我们可以利用 ``git clone`` 完成权重的下载:
+
+.. code:: console
+
+ $ git lfs install
+ $ # From HuggingFace
+ $ git clone https://huggingface.co/internlm/internlm2-chat-7b
+ $ # From ModelScope
+ $ git clone https://www.modelscope.cn/Shanghai_AI_Laboratory/internlm2-chat-7b.git
+
+
+方法 3:``AutoModelForCausalLM``
+=====================================================
+
+``AutoModelForCausalLM.from_pretrained``
+在初始化模型时,将尝试连接远程仓库并自动下载模型权重。因此,我们可以利用这一特性下载模型权重。
+
+HuggingFace
+------------
+
+.. code:: python
+
+ from transformers import AutoModelForCausalLM, AutoTokenizer
+
+ model = AutoModelForCausalLM.from_pretrained('internlm/internlm2-chat-7b', trust_remote_code=True)
+ tokenizer = AutoTokenizer.from_pretrained('internlm/internlm2-chat-7b', trust_remote_code=True)
+
+.. tip::
+
+ 此时模型将会下载至 HuggingFace 的 cache 路径中(默认为\ ``~/.cache/huggingface/hub``\ )。
+
+ 若要修改默认存储路径,需要修改相关环境变量:
+
+ .. code:: console
+
+ $ # 默认为 `~/.cache/huggingface/`
+ $ export HF_HOME=XXXX
+
+ModelScope
+-----------
+
+.. code:: python
+
+ from modelscope import AutoModelForCausalLM, AutoTokenizer
+
+ model = AutoModelForCausalLM.from_pretrained('Shanghai_AI_Laboratory/internlm2-chat-7b', trust_remote_code=True)
+ tokenizer = AutoTokenizer.from_pretrained('Shanghai_AI_Laboratory/internlm2-chat-7b', trust_remote_code=True)
+
+.. tip::
+
+ 此时模型将会下载至 ModelScope 的 cache 路径中(默认为\ ``~/.cache/modelscope/hub``\ )。
+
+ 若要修改默认存储路径,需要修改相关环境变量:
+
+ .. code:: console
+
+ $ # 默认为 ~/.cache/modelscope/hub/
+ $ export MODELSCOPE_CACHE=XXXX
diff --git a/docs/zh_cn/preparation/prompt_template.rst b/docs/zh_cn/preparation/prompt_template.rst
new file mode 100644
index 000000000..709841b7f
--- /dev/null
+++ b/docs/zh_cn/preparation/prompt_template.rst
@@ -0,0 +1,237 @@
+.. _prompt_template:
+
+准备对话模版
+============
+
+大模型的微调、对话均需要选择一个合适的对话模版(prompt template)。
+XTuner 设计了一套对话模版封装逻辑,并提供了一系列社区广泛使用的对话模版。
+
+本文将从“何处需要对话模版?”、“XTuner 内置对话模版速览”、“如何选择对话模版?”、“如何自定义对话模版?”四部分展开介绍。
+
+何处需要对话模版?
+------------------
+
+:``xtuner train``:
+ 需要使用对话模版将训练数据“模版化”,在训练 ``config`` 中配置 ``prompt_template`` 参数来选择对话模版
+
+:``xtuner chat``:
+ 需要使用对话模版将对话文本“模版化”,通过 ``xtuner chat`` 命令的 ``--prompt-template`` 参数选择对话模版
+
+.. note::
+
+ 各种推理引擎也都会用到对话模板,每个框架定义对话模板的方式都不尽相同,但最终“模板化”后的数据都是相同的
+
+.. tip::
+
+ 请确保在训练、对话和自定义应用场景中,始终保持对话模板的一致,否则可能会出现不符合预期的结果。
+
+XTuner 内置对话模版速览
+-----------------------
+
+XTuner 对现有大多数大语言模型的对话模版进行了实现,并集成在
+``xtuner.utils.PROMPT_TEMPLATE`` 内,用户可以直接使用。
+
+.. note::
+
+ XTuner 内置的对话模板清单可见文末附录
+
+字段约定
+~~~~~~~~
+
+以 ``internlm2_chat`` 模版为例,其代码结构如下。
+
+.. code:: python
+
+ internlm2_chat=dict(
+ SYSTEM='<|im_start|>system\n{system}<|im_end|>\n',
+ INSTRUCTION=('<|im_start|>user\n{input}<|im_end|>\n'
+ '<|im_start|>assistant\n'),
+ SUFFIX='<|im_end|>',
+ SUFFIX_AS_EOS=True,
+ SEP='\n',
+ STOP_WORDS=['<|im_end|>']),
+
+- ``SYSTEM``\ :表示问答时“系统”字段的模版,其中 ``{system}``
+ 指代“系统”文本。值得注意的是,该字段在多轮对话中只会出现一次,即在第一轮。
+
+- ``INSTRUCTION``\ :表示问答时“指令”字段的模版,其中 ``{input}``
+ 指代用户指令文本。
+
+- ``SUFFIX``\ :表示“指令”字段的后缀,将会追加在每一轮问答的“回答”后面。通常,这也是一个特殊的结束符号。默认是空串\ ``''``\ 。
+
+- ``SUFFIX_AS_EOS``\ :表示上述后缀是否作为结束符号。如果为
+ ``True``\ ,则会取代 ``tokenizer`` 的 ``eos_token``\ ,否则,仍会使用
+ ``tokenizer`` 的 ``eos_token`` 表示结束符号。默认是 ``False``\ 。
+
+- ``SEP``\ :用于间隔多轮对话,将会追加在 ``INSTRUCTION`` 和 ``SUFFIX``
+ 后面。默认是空串\ ``''``\ 。
+
+- ``STOP_WORDS``\ :用于指明结束词,该信息将被用在文本生成阶段。值得注意的是,\ ``tokenizer``
+ 的 ``eos_token`` 会被自动添加到 ``STOP_WORDS``\ ,而无需手动配置。
+
+模版化结果
+~~~~~~~~~~
+
+以 ``internlm2_chat`` 模版为例,其对应的单轮、多轮模版化结果如下。
+
+**单轮**
+
+.. code::
+
+ <|im_start|>system
+ 你是一个无害的 AI 助手<|im_end|>
+ <|im_start|>user
+ 你是谁?<|im_end|>
+ <|im_start|>assistant
+ 我是书生浦语。<|im_end|>
+
+**多轮**
+
+.. code::
+
+ <|im_start|>system
+ 你是一个无害的 AI 助手<|im_end|>
+ <|im_start|>user
+ 你是谁?<|im_end|>
+ <|im_start|>assistant
+ 我是书生浦语。<|im_end|>
+ <|im_start|>user
+ 你的英文名字是什么?<|im_end|>
+ <|im_start|>assistant
+ InternLM<|im_end|>
+
+如何选择对话模版?
+------------------
+
+选择准确的对话模版是训练、应用模型的关键。关于如何选择对话模版,我们建议:
+
+:微调 chat 模型:
+ 使用模型所对应的对话模版,如 ``internlm2-chat`` 使用
+ ``internlm2_chat``\ 、\ ``Qwen-Chat`` 使用 ``qwen_chat``\ 。
+
+:全量微调 base 模型:
+ 任选对话模版,优先使用 chat 版模型所对应的对话模版 。
+
+
+:LoRA 微调 base 模型:
+ | 使用默认对话模版 ``default``\ 。这是由于 LoRA /
+ QLoRA 微调默认会关闭 ``embed_tokens`` 和 ``lm_head``
+ 的训练,此时如果引入未学习过的特殊 token(如对话模版中的
+ ``<|im_start|>``\ ),则会影响模型的训练。
+
+.. tip::
+ 通过修改 ``LoraConfig`` 可以引入 ``embed_tokens`` 和
+ ``lm_head`` 的训练(会增大显存需求),进而支持任选对话模版
+
+ .. code:: diff
+
+ lora=dict(
+ type=LoraConfig,
+ r=64,
+ lora_alpha=16,
+ lora_dropout=0.1,
+ bias='none',
+ + modules_to_save=['embed_tokens', 'lm_head'] # 请确保与模型中所使用的参数名一致
+ task_type='CAUSAL_LM')
+
+.. tip::
+
+ 大多数的 base 模型所使用的 tokenizer 中不包含 chat
+ 模型对话模板中所使用的特殊 token 编码(例如 `internlm2
+ chat `__
+ 和 `internlm2
+ base `__\ )。因此,如果要微调
+ base 模型并配合使用 chat 版对话模版,需确保在 Config
+ 中及后续全流程使用 chat 版模型的 tokenizer。Config 中修改 tokenizer
+ 的方式为:
+
+ .. code:: diff
+
+ tokenizer = dict(
+ type=AutoTokenizer.from_pretrained,
+ - pretrained_model_name_or_path=pretrained_model_name_or_path,
+ + pretrained_model_name_or_path='PATH_TO_CHAT_LLM_TOKENIZER',
+ trust_remote_code=True,
+ padding_side='right')
+
+如何自定义对话模版?
+--------------------
+
+如果 XTuner
+所内置的对话模版不能满足实际需求,用户可以实现自定义的对话模版。
+
+具体来说,可以在
+`template.py `__
+的 ``PROMPT_TEMPLATE`` 中新增一个对话模版,并参考 “XTuner
+内置对话模版速览” 章节对每个字段的描述进行自定义修改。
+
+附:XTuner 内置 configs 所选择的对话模版
+----------------------------------------
+
+.. note::
+
+ \*: 官方对话模版中存在特殊 token(比如 ``<|im_start|>``\ 、\ ``<|im_end|>``\ ),这类特殊 token
+ 在预训练阶段并未得到训练。故,使用 ``default`` 模版。
+======================================== ==============
+模型 对话模版
+======================================== ==============
+baichuan-inc/Baichuan-7B default\*
+baichuan-inc/Baichuan-13B-Base default\*
+baichuan-inc/Baichuan-13B-Chat baichuan_chat
+baichuan-inc/Baichuan2-7B-Base default\*
+baichuan-inc/Baichuan2-7B-Chat baichuan2_chat
+baichuan-inc/Baichuan2-13B-Base default\*
+baichuan-inc/Baichuan2-13B-Chat baichuan2_chat
+THUDM/chatglm2-6b chatglm2
+THUDM/chatglm3-6b chatglm3
+THUDM/chatglm3-6b-base chatglm3
+deepseek-ai/deepseek-coder-6.7b-base deepseek_coder
+deepseek-ai/deepseek-coder-6.7b-instruct deepseek_coder
+internlm/internlm-7b default\*
+internlm/internlm-20b default\*
+internlm/internlm-chat-7b internlm_chat
+internlm/internlm-chat-20b internlm_chat
+huggyllama/llama-7b default
+meta-llama/Llama-2-7b-hf llama2_chat
+meta-llama/Llama-2-7b-chat-hf llama2_chat
+meta-llama/Llama-2-70b-hf llama2_chat
+lmsys/vicuna-7b-v1.5 vicuna
+lmsys/vicuna-13b-v1.5 vicuna
+mistralai/Mistral-7B-v0.1 mistral
+mistralai/Mixtral-8x7B-v0.1 mixtral
+mistralai/Mixtral-8x7B-Instruct-v0.1 mixtral
+Qwen/Qwen-1_8B default\*
+Qwen/Qwen-1_8B-Chat qwen_chat
+Qwen/Qwen-7B default\*
+Qwen/Qwen-7B-Chat qwen_chat
+Qwen/Qwen-72B default\*
+Qwen/Qwen-72B-Chat qwen_chat
+bigcode/starcoder default
+01-ai/Yi-6B default
+01-ai/Yi-34B default
+HuggingFaceH4/zephyr-7b-beta zephyr
+deepseek-ai/deepseek-moe-16b-base deepseek_moe
+deepseek-ai/deepseek-moe-16b-chat deepseek_moe
+internlm/internlm2-1_8b default\*
+internlm/internlm2-7b default\*
+internlm/internlm2-20b default\*
+internlm/internlm2-chat-1_8b internlm2_chat
+internlm/internlm2-chat-7b internlm2_chat
+internlm/internlm2-chat-20b internlm2_chat
+Qwen/Qwen1.5-0.5B default\*
+Qwen/Qwen1.5-0.5B-Chat qwen_chat
+Qwen/Qwen1.5-1.8B default\*
+Qwen/Qwen1.5-1.8B-Chat qwen_chat
+Qwen/Qwen1.5-4B default\*
+Qwen/Qwen1.5-4B-Chat qwen_chat
+Qwen/Qwen1.5-7B default\*
+Qwen/Qwen1.5-7B-Chat qwen_chat
+Qwen/Qwen1.5-14B default\*
+Qwen/Qwen1.5-14B-Chat qwen_chat
+Qwen/Qwen1.5-72B default\*
+Qwen/Qwen1.5-72B-Chat qwen_chat
+google/gemma-2b default\*
+google/gemma-2b-it gemma
+google/gemma-7b default\*
+google/gemma-7b-it gemma
+======================================== ==============
diff --git a/docs/zh_cn/reward_model/images/preference_data.png b/docs/zh_cn/reward_model/images/preference_data.png
new file mode 100644
index 000000000..a18ea6449
Binary files /dev/null and b/docs/zh_cn/reward_model/images/preference_data.png differ
diff --git a/docs/zh_cn/reward_model/images/sequence_parallel.png b/docs/zh_cn/reward_model/images/sequence_parallel.png
new file mode 100644
index 000000000..53f86c81a
Binary files /dev/null and b/docs/zh_cn/reward_model/images/sequence_parallel.png differ
diff --git a/docs/zh_cn/reward_model/images/var_len_atten.png b/docs/zh_cn/reward_model/images/var_len_atten.png
new file mode 100644
index 000000000..3e60777d2
Binary files /dev/null and b/docs/zh_cn/reward_model/images/var_len_atten.png differ
diff --git a/docs/zh_cn/reward_model/modify_settings.md b/docs/zh_cn/reward_model/modify_settings.md
new file mode 100644
index 000000000..c56b04115
--- /dev/null
+++ b/docs/zh_cn/reward_model/modify_settings.md
@@ -0,0 +1,100 @@
+## 修改 Reward Model 训练配置
+
+本章节仅介绍与 Reward Model 训练相关的配置参数,更多 XTuner 配置文件的细节,请参考[修改训练配置](https://xtuner.readthedocs.io/zh-cn/latest/training/modify_settings.html)
+
+### 损失函数
+
+XTuner 使用了 [Bradley–Terry 模型](https://en.wikipedia.org/wiki/Bradley%E2%80%93Terry_model) 作为 Reward Model 的偏好建模方式,你可以指定 `loss_type="ranking"` 来使用 ranking loss。XTuner 中也实现了 InternLM2 中提出的 focal 损失函数,它通过调整难易样本的权重来避免过拟合,可以设置 `loss_type="focal"` 来使用该损失函数。对于该损失函数的详细说明,请参考 [InternLM2 技术报告](https://arxiv.org/abs/2403.17297)。
+
+另外,为了使 reward model 输出的 score 数值保持稳定,我们还在 loss 中额外增加了一个约束项,你可以指定 `penalty_type='log_barrier'` 或是 `penalty_type='L2'` 以启用对数约束或是L2约束。
+
+```python
+#######################################################################
+# PART 1 Settings #
+#######################################################################
+# Model
+loss_type = 'focal' # 'ranking' or 'focal'
+penalty_type = 'log_barrier' # 'log_barrier' or 'L2'
+```
+
+### 修改模型
+
+用户可以修改 `pretrained_model_name_or_path` 对预训练模型进行修改。
+
+需要注意的是,由于 XTuner 通过对数据的末尾添加 `<|reward|>` 特殊 token 的方式计算 reward 得分,因此当切换模型的词表发生变化时,该特殊 token 的 id 也需要进行相应的修改,我们通常会使用词表末尾未使用的 token 作为 reward token。
+
+例如,在 InternLM2 中我们使用 `[UNUSED_TOKEN_130]` 作为 reward token:
+
+```python
+#######################################################################
+# PART 1 Settings #
+#######################################################################
+# Model
+pretrained_model_name_or_path = 'internlm/internlm2-chat-1_8b-sft'
+reward_token_id = 92527 # use [UNUSED_TOKEN_130] as reward token
+```
+
+如果用户将模型切换为llama3,我们则可以使用 `<|reserved_special_token_0|>` 作为 reward token:
+
+```python
+#######################################################################
+# PART 1 Settings #
+#######################################################################
+# Model
+pretrained_model_name_or_path = 'meta-llama/Meta-Llama-3-8B-Instruct'
+reward_token_id = 128002 # use <|reserved_special_token_0|> as reward token
+```
+
+### 训练数据
+
+在 Reward Model 训练中,你可以通过 `max_length` 来指定单个样本序列的最大 token 数,XTuner 会自动对数据进行截断或是填充。
+
+```python
+# Data
+max_length = 2048
+```
+
+在配置文件中,我们通过 `train_dataset` 字段来指定训练数据集,你可以通过 `dataset` 字段指定数据集的加载方式,通过 `dataset_map_fn` 字段指定数据集的映射函数。
+
+```python
+#######################################################################
+# PART 3 Dataset & Dataloader #
+#######################################################################
+sampler = SequenceParallelSampler \
+ if sequence_parallel_size > 1 else DefaultSampler
+
+train_dataset = dict(
+ type=build_preference_dataset,
+ dataset=dict(
+ type=load_dataset,
+ path='argilla/ultrafeedback-binarized-preferences-cleaned'),
+ tokenizer=tokenizer,
+ max_length=max_length,
+ dataset_map_fn=orpo_dpo_mix_40k_map_fn,
+ is_dpo=False,
+ is_reward=True,
+ reward_token_id=reward_token_id,
+ num_proc=32,
+ use_varlen_attn=use_varlen_attn,
+ max_packed_length=max_packed_length,
+ shuffle_before_pack=True,
+)
+
+train_dataloader = dict(
+ batch_size=batch_size,
+ num_workers=dataloader_num_workers,
+ dataset=train_dataset,
+ sampler=dict(type=sampler, shuffle=True),
+ collate_fn=dict(
+ type=preference_collate_fn, use_varlen_attn=use_varlen_attn))
+```
+
+上述配置中,我们使用了 `load_dataset` 来加载 huggingface 上的 `argilla/ultrafeedback-binarized-preferences-cleaned` 数据集,使用 `orpo_dpo_mix_40k_map_fn` 作为数据集映射函数(这是因为 `orpo_dpo_mix_40k` 与 `ultrafeedback-binarized-preferences-cleaned` 的格式相同,因此这里共用了同一个映射函数)。
+
+关于如何处理数据集以及如何编写数据集映射函数,请参考[偏好数据集章节](./preference_data.md)。
+
+### 加速训练
+
+在使用偏好数据训练时,我们推荐您开启[变长注意力机制](https://xtuner.readthedocs.io/zh-cn/latest/acceleration/varlen_flash_attn.html), 以避免单个偏好内的 chosen 和 rejected 的样本长度差异造成的显存浪费。你可以通过 `use_varlen_attn=True` 来开启变长注意力机制。
+
+XTuner 中还支持了大量的训练加速方法,关于它们的使用方法,请参考[加速策略章节](https://xtuner.readthedocs.io/zh-cn/latest/acceleration/hyper_parameters.html)。
diff --git a/docs/zh_cn/reward_model/overview.md b/docs/zh_cn/reward_model/overview.md
new file mode 100644
index 000000000..6c7c976ac
--- /dev/null
+++ b/docs/zh_cn/reward_model/overview.md
@@ -0,0 +1,43 @@
+## Reward Model 介绍
+
+### 简介
+
+Reward Model(奖励模型)是强化学习过程中一个关键的组成部分。它的主要任务是根据给定的输入和反馈来预测奖励值,从而指导学习算法的方向。在RLHF(Reinforcement Learning from Human Feedback)中,Reward Model 通过整合人类反馈,帮助强化学习算法更有效地优化策略。
+
+在大语言模型训练中,Reward Model 通常指的是偏好模型(Preference Model)。通过在训练时提供相同提示词的好与坏(chosen&rejected)的回复来拟合人类的偏好,并在推理时预测出一个奖励值,以指导 RLHF 过程中 Actor 模型的优化过程。
+
+Reward Model的应用场景包括但不限于:
+
+- **RLHF训练**:在使用 Proximal Policy Optimization(PPO)算法进行 RLHF 训练时,Reward Model提供奖励信号,指导模型优化策略,提高生成内容的质量并使其更贴近人类偏好。
+- **BoN采样**:在 Best-of-N(BoN)采样过程中,用户可以使用 Reward Model 对同一个提示词的多条回复进行打分,并选择奖励得分最高的生成结果,从而提升模型的输出效果。
+- **数据构造**:Reward Model 可以用于评估和过滤训练数据,或者也可以使用 Reward Model 替代人工标注来构造 DPO 训练数据。
+
+### XTuner 中 Reward Model 训练的优势
+
+XTuner 中的 Reward Model 训练具备以下显著优势:
+
+1. **使用最新的训练技巧**:XTuner 中集成了 InternLM2 中的 Reward Model 训练损失函数,可以稳定奖励得分的数值范围,也可以减少在简单样本上的过拟合(具体可参考 [InternLM2 技术报告](https://arxiv.org/abs/2403.17297))。
+
+2. **减少显存浪费**:由于偏好数据中的 chosen 和 rejected 数据通常存在长度上的差异,因此在训练数据的拼接时会存在填充(padding token),造成显存浪费。在 XTuner 中,基于 Flash Attention2 中的变长注意力功能,我们在训练过程中通过将偏好数据打包到同一个序列中,显著减少了由于 padding token 带来的显存浪费。这不仅提高了显存的利用效率,还使得在相同硬件条件下可以训练更大的模型或处理更多的数据。
+
+
+
+3. **高效训练**:借助 XTuner 的 QLoRA 训练功能,我们能够仅对 Reward Model 的 Value Head 进行全参数训练,而对语言模型本身使用 QLoRA 微调,大幅降低了模型训练的显存开销。
+
+4. **长文本训练**: 借助 XTuner 的序列并行功能,能够对长文本数据进行训练。
+
+
+
+### 开始训练
+
+请参[阅快速上手](./quick_start.md)来了解最基本的概念,若希望了解更多训练参数配置相关的内容,请参考[修改Reward Model配置](./modify_settings.md)章节。
+
+### 开源模型
+
+我们使用 XTuner 训练了 InternLM2 技术报告中的 Reward Model,欢迎下载使用:
+
+| Model | Transformers(HF) | ModelScope(HF) | OpenXLab(HF) | RewardBench Score |
+| ------------------------- | -------------------------------------------------------------------------------- | ---------------------------------------------------------------------------------------------------------- | ----------------------------------------------------------------------------------------------------------------------------------------------------------- | ----------------- |
+| **InternLM2-1.8B-Reward** | [🤗internlm2-1_8b-reward](https://huggingface.co/internlm/internlm2-1_8b-reward) | [internlm2-1_8b-reward](https://modelscope.cn/models/Shanghai_AI_Laboratory/internlm2-1_8b-reward/summary) | [](https://openxlab.org.cn/models/detail/OpenLMLab/internlm2-1_8b-reward) | 80.6 |
+| **InternLM2-7B-Reward** | [🤗internlm2-7b-reward](https://huggingface.co/internlm/internlm2-7b-reward) | [internlm2-7b-reward](https://modelscope.cn/models/Shanghai_AI_Laboratory/internlm2-7b-reward/summary) | [](https://openxlab.org.cn/models/detail/OpenLMLab/internlm2-7b-reward) | 86.6 |
+| **InternLM2-20B-Reward** | [🤗internlm2-20b-reward](https://huggingface.co/internlm/internlm2-20b-reward) | [internlm2-20b-reward](https://modelscope.cn/models/Shanghai_AI_Laboratory/internlm2-20b-reward/summary) | [](https://openxlab.org.cn/models/detail/OpenLMLab/internlm2-20b-reward) | 89.5 |
diff --git a/docs/zh_cn/reward_model/preference_data.md b/docs/zh_cn/reward_model/preference_data.md
new file mode 100644
index 000000000..1dd296053
--- /dev/null
+++ b/docs/zh_cn/reward_model/preference_data.md
@@ -0,0 +1,110 @@
+## 偏好数据集
+
+### 简介
+
+XTuner 的 Reward Model 与 DPO、ORPO 等依赖偏好数据的算法都采用了同样的数据格式,偏好数据集中的每一条训练样本需要包含以下三个字段:`prompt`、`chosen`、`rejected`。其中每个字段的值都使用了 [OpenAI chat message](https://platform.openai.com/docs/api-reference/chat/create) 格式。一个具体的例子如下所示:
+
+```json
+{
+ "prompt": [
+ {
+ "role": "system",
+ "content": "You are a helpful assistant."
+ },
+ {
+ "role": "user",
+ "content": "Who won the world series in 2020?"
+ },
+ {
+ "role": "assistant",
+ "content": "The Los Angeles Dodgers won the World Series in 2020."
+ },
+ {
+ "role": "user",
+ "content": "Where was it played?"
+ }
+ ],
+ "chosen": [
+ {
+ "role": "assistant",
+ "content": "The 2020 World Series was played at Globe Life Field in Arlington, Texas."
+ }
+ ],
+ "rejected": [
+ {
+ "role": "assistant",
+ "content": "I don't know."
+ }
+ ]
+}
+```
+
+当进行 Reward Model 训练或是 DPO 训练时,xtuner 会根据训练任务类型的不同,将偏好数据集处理为不同的训练标签。
+
+
+
+如上图所示,当进行 Reward Model 训练时,我们参考 ChatGPT 的训练方式,在对话数据的最后添加一个特殊的`<|reward|>` token,只对该 token 输出的 logits 计算损失。而当进行 DPO 系列算法的训练时,我们则会屏蔽掉 prompt 部分的 token,只对 chosen 和 rejected 的回复部分计算损失。在配置文件中,我们通过 dataset 中的 `is_reward` 和 `is_dpo` 两个字段来控制数据集的类型。
+
+### 使用自定义数据进行训练
+
+在理解了单个样本的数据格式之后,我们只需要将转换好的数据保存为[JSON Lines](https://jsonlines.org/)格式即可。文件中的每一行都是一个样本。
+
+```json
+{"prompt": [{"content": "How can I learn to better regulate my emotions as a grown adult?", "role": "user"}], "chosen": [{"content": "You could do emotional regulation exercises with me, and try to notice and practice ways that you could better control your emotions in real life.", "role": "assistant"}], "rejected": [{"content": "Do you mean how can you learn to be more emotionally mature?", "role": "assistant"}]}
+{"prompt": [{"content": "What are some interesting things I can add to a grilled cheese sandwich?", "role": "user"}, {"content": "A tomato, a slice of pickle, some chopped pickles, some chopped green onions. Also, some mayonnaise if you like.", "role": "assistant"}, {"content": "Sounds great. Anything else?", "role": "user"}], "chosen": [{"content": "If you’d like something a little different, I recommend grating some horseradish on top of the sandwich.", "role": "assistant"}], "rejected": [{"content": "Maybe some nice fresh basil?", "role": "assistant"}]}
+{"prompt": [{"content": "How do I bob for apples?", "role": "user"}], "chosen": [{"content": "Ah! Do you mean the game of “applesauce,” or do you mean the idea of “to bob for apples”?", "role": "assistant"}], "rejected": [{"content": "Sorry, I don’t know that term.", "role": "assistant"}]}
+......
+```
+
+在准备完自定义数据集之后,你需要将你保存的数据路径填入配置文件中的 `data_files` 字段,你可以同时加载多个 jsonl 数据进行训练。
+
+```python
+#######################################################################
+# PART 3 Dataset & Dataloader #
+#######################################################################
+train_dataset = dict(
+ type=build_preference_dataset,
+ dataset=dict(
+ type=load_jsonl_dataset,
+ data_files=[
+ '/your/jsonl/path/here.jsonl',
+ '/your/another/jsonl/path/here.jsonl'
+ ]),
+)
+```
+
+### 使用开源数据集进行训练
+
+与 XTuner 配置 SFT 数据一样,在使用 huggingface 上的开源数据集时,我们只需要定义映射函数 map_fn,将开源数据集格式处理为 XTuner 中的数据格式即可。
+
+这里我们以 Intel/orca_dpo_pairs 为例,该数据集有 `system`、`question`、`chosen`、`rejected` 四个字段,并且每个字段的值为 text 而非 [OpenAI chat message](https://platform.openai.com/docs/api-reference/chat/create) 格式。因此我们需要为该数据集定义一个 map_fn:
+
+```python
+def intel_orca_dpo_map_fn(example):
+ prompt = [{
+ 'role': 'system',
+ 'content': example['system']
+ }, {
+ 'role': 'user',
+ 'content': example['question']
+ }]
+ chosen = [{'role': 'assistant', 'content': example['chosen']}]
+ rejected = [{'role': 'assistant', 'content': example['rejected']}]
+ return {'prompt': prompt, 'chosen': chosen, 'rejected': rejected}
+```
+
+通过代码可以看到,`intel_orca_dpo_map_fn` 对原数据中的四个字段进行处理,将其转换为了 `prompt`、`chosen`、`rejected` 三个字段,并且每个字段都处理为了[OpenAI chat message](https://platform.openai.com/docs/api-reference/chat/create) 格式,确保了后续数据处理流程的统一。
+
+完成了 map_fn 的定义之后,需要在配置文件中 import 该函数,并在 `dataset_map_fn` 字段中进行配置。
+
+```python
+train_dataset = dict(
+ type=build_preference_dataset,
+ dataset=dict(
+ type=load_dataset,
+ path='Intel/orca_dpo_pairs'),
+ tokenizer=tokenizer,
+ max_length=max_length,
+ dataset_map_fn=intel_orca_dpo_map_fn,
+)
+```
diff --git a/docs/zh_cn/reward_model/quick_start.md b/docs/zh_cn/reward_model/quick_start.md
new file mode 100644
index 000000000..736624cef
--- /dev/null
+++ b/docs/zh_cn/reward_model/quick_start.md
@@ -0,0 +1,86 @@
+## Reward Model 快速上手
+
+在本章节中,我们将介绍如何使用 XTuner 训练 1.8B 的 Reward Model,以帮助您快速上手。
+
+### 准备预训练模型权重
+
+依据 [Training language models to follow instructions with human feedback](https://arxiv.org/abs/2203.02155) 论文中的描述,我们使用进过 SFT 的语言模型作为 Reward Model 的初始化模型。这里我们使用[InternLM2-chat-1.8b-sft](https://huggingface.co/internlm/internlm2-chat-1_8b-sft)作为初始化模型。
+
+在训练配置文件中设置`pretrained_model_name_or_path = 'internlm/internlm2-chat-1_8b-sft'`,则会在启动训练时自动下载模型文件。若您需要手动下载模型权重,那么请参考[准备预训练模型权重](https://xtuner.readthedocs.io/zh-cn/latest/preparation/pretrained_model.html)章节,其中详细说明了如何从 Huggingface 或者是 Modelscope 下载模型权重的方法。这里我们附上模型的 HuggingFace 链接与 ModelScope 链接:
+
+- HuggingFace 链接位于:https://huggingface.co/internlm/internlm2-chat-1_8b-sft
+
+- ModelScope 链接位于:https://modelscope.cn/models/Shanghai_AI_Laboratory/internlm2-chat-1_8b-sft/summary
+
+### 准备训练数据
+
+在本教程中使用 [UltraFeedback](https://arxiv.org/abs/2310.01377) 数据集作为演示,为了方便起见,我们使用 huggingface 上已经预处理过的 [argilla/ultrafeedback-binarized-preferences-cleaned](https://huggingface.co/datasets/argilla/ultrafeedback-binarized-preferences-cleaned) 数据集,
+
+```python
+train_dataset = dict(
+ type=build_preference_dataset,
+ dataset=dict(
+ type=load_dataset,
+ path='argilla/ultrafeedback-binarized-preferences-cleaned'),
+ dataset_map_fn=orpo_dpo_mix_40k_map_fn,
+ is_dpo=False,
+ is_reward=True,
+)
+```
+
+在配置文件中使用以上配置,即可自动下载并处理该数据集。如果您希望使用其他 huggingface 上的开源数据集或是使用自定义的数据集,请参阅[偏好数据集](./preference_data.md)章节。
+
+### 准备配置文件
+
+XTuner 提供了多个开箱即用的配置文件,可以通过 `xtuner list-cfg` 查看。我们执行如下指令,以复制一个配置文件到当前目录。
+
+```bash
+xtuner copy-cfg internlm2_chat_1_8b_reward_full_ultrafeedback .
+```
+
+打开复制后的配置文件,如果您选择自动下载模型和数据集,则无需修改配置。若您希望填入您预先下载的模型路径和数据集路径,请修改配置中的 `pretrained_model_name_or_path` 以及 `train_dataset` 中 `dataset` 的 `path` 参数。
+
+更多的训练参数配置,请参阅[修改Reward训练配置](./modify_settings.md)章节。
+
+### 启动训练
+
+在完成上述操作后,便可以使用下面的指令启动训练任务了。
+
+```bash
+# 单机单卡
+xtuner train ./internlm2_chat_1_8b_reward_full_ultrafeedback_copy.py
+# 单机多卡
+NPROC_PER_NODE=${GPU_NUM} xtuner train ./internlm2_chat_1_8b_reward_full_ultrafeedback_copy.py
+# slurm 集群
+srun ${SRUN_ARGS} xtuner train ./internlm2_chat_1_8b_reward_full_ultrafeedback_copy.py --launcher slurm
+```
+
+正确的训练日志应当如下所示(在单卡 A800 上运行):
+
+```
+06/06 16:12:11 - mmengine - INFO - Iter(train) [ 10/15230] lr: 3.9580e-07 eta: 2:59:41 time: 0.7084 data_time: 0.0044 memory: 18021 loss: 0.6270 acc: 0.0000 chosen_score_mean: 0.0000 rejected_score_mean: 0.0000 num_samples: 4.0000 num_tokens: 969.0000
+06/06 16:12:17 - mmengine - INFO - Iter(train) [ 20/15230] lr: 8.3536e-07 eta: 2:45:25 time: 0.5968 data_time: 0.0034 memory: 42180 loss: 0.6270 acc: 0.5000 chosen_score_mean: 0.0013 rejected_score_mean: 0.0010 num_samples: 4.0000 num_tokens: 1405.0000
+06/06 16:12:22 - mmengine - INFO - Iter(train) [ 30/15230] lr: 1.2749e-06 eta: 2:37:18 time: 0.5578 data_time: 0.0024 memory: 32121 loss: 0.6270 acc: 0.7500 chosen_score_mean: 0.0016 rejected_score_mean: 0.0011 num_samples: 4.0000 num_tokens: 932.0000
+06/06 16:12:28 - mmengine - INFO - Iter(train) [ 40/15230] lr: 1.7145e-06 eta: 2:36:05 time: 0.6033 data_time: 0.0025 memory: 42186 loss: 0.6270 acc: 0.7500 chosen_score_mean: 0.0027 rejected_score_mean: 0.0016 num_samples: 4.0000 num_tokens: 994.0000
+06/06 16:12:35 - mmengine - INFO - Iter(train) [ 50/15230] lr: 2.1540e-06 eta: 2:41:03 time: 0.7166 data_time: 0.0027 memory: 42186 loss: 0.6278 acc: 0.5000 chosen_score_mean: 0.0031 rejected_score_mean: 0.0032 num_samples: 4.0000 num_tokens: 2049.0000
+06/06 16:12:40 - mmengine - INFO - Iter(train) [ 60/15230] lr: 2.5936e-06 eta: 2:33:37 time: 0.4627 data_time: 0.0023 memory: 30238 loss: 0.6262 acc: 1.0000 chosen_score_mean: 0.0057 rejected_score_mean: 0.0030 num_samples: 4.0000 num_tokens: 992.0000
+06/06 16:12:46 - mmengine - INFO - Iter(train) [ 70/15230] lr: 3.0331e-06 eta: 2:33:18 time: 0.6018 data_time: 0.0025 memory: 42186 loss: 0.6247 acc: 0.7500 chosen_score_mean: 0.0117 rejected_score_mean: 0.0055 num_samples: 4.0000 num_tokens: 815.0000
+```
+
+### 模型转换
+
+XTuner 已经集成好了将模型转换为 HuggingFace 格式的工具,我们只需要执行
+
+```bash
+# 创建存放 hf 格式参数的目录
+mkdir work_dirs/internlm2_chat_1_8b_reward_full_ultrafeedback_copy/iter_15230_hf
+
+# 转换格式
+xtuner convert pth_to_hf internlm2_chat_1_8b_reward_full_ultrafeedback_copy.py \
+ work_dirs/internlm2_chat_1_8b_reward_full_ultrafeedback_copy.py/iter_15230.pth \
+ work_dirs/internlm2_chat_1_8b_reward_full_ultrafeedback_copy.py/iter_15230_hf
+```
+
+便能够将 XTuner 的 ckpt 转换为 Huggingface 格式的模型。
+
+需要注意的是,由于 Reward Model 的类型并未在 transformers 官方库中集成,因此目前只有InternLM2模型训练得到的 Reward Model 会被转换为 InternLM2ForRewardModel 类型,而其他模型则会默认转换为 SequenceClassification 类型(例如 LLaMa3 会被转换为 LlamaForSequenceClassification 类型),但这并不影响其在 XTuner PPO 训练中的使用。
diff --git a/docs/zh_cn/switch_language.md b/docs/zh_cn/switch_language.md
new file mode 100644
index 000000000..ff7c4c425
--- /dev/null
+++ b/docs/zh_cn/switch_language.md
@@ -0,0 +1,3 @@
+## English
+
+## 简体中文
diff --git a/docs/zh_cn/training/custom_pretrain_dataset.rst b/docs/zh_cn/training/custom_pretrain_dataset.rst
new file mode 100644
index 000000000..ff2243587
--- /dev/null
+++ b/docs/zh_cn/training/custom_pretrain_dataset.rst
@@ -0,0 +1,202 @@
+==================================
+自定义预训练数据集 (LLM)
+==================================
+
+XTuner 支持使用自定义数据集进行增量预训练,为便于介绍,本节以
+`internlm2_7b_custom_pretrain_e1.py `__
+配置文件为基础进行介绍。
+
+数据准备
+=================
+
+用户若要在进行预训练,则需要将自定义的数据处理为以下格式:
+
+.. code:: json
+
+ [
+ {
+ "text": "xxx"
+ },
+ {
+ "text": "xxx"
+ },
+ ...
+ ]
+
+.. tip::
+ 每条 ``text`` 数据不要太长(分词个数应小于
+ ``max_length``\ ),以避免在数据处理阶段被截断。
+
+.. tip::
+ 为保证数据上下文的一致性,请确保长文本数据在被切分为多个 ``text``
+ 后,json 列表的顺序与实际上下文顺序一致。
+
+训练
+===============
+
+步骤 1 :导出 config
+-------------------------------
+
+``xtuner/configs/custom_dataset/pretrain/`` 目录下有所有 XTuner
+支持的模型在自定义数据集下执行预训练的模板 config。可以通过
+``xtuner list-cfg -p custom_pretrain`` 命令来查看候选 config。下面以
+`internlm2_7b_custom_pretrain_e1.py `__
+为例展开介绍。
+
+可以通过以下命令将 ``internlm2_7b_full_custom_pretrain_e1.py``
+导出至当前目录下:
+
+.. code:: console
+
+ $ xtuner copy-cfg internlm2_7b_full_custom_pretrain_e1 .
+
+.. note::
+ 当前目录下会存在一个新 config
+ ``internlm2_7b_full_custom_pretrain_e1_copy.py`` 。
+
+步骤 2 :修改 config
+---------------------------------
+
+首先,需要修改数据集文件路径:
+
+.. code:: diff
+
+ - data_files = ['/path/to/json/file.json']
+ + data_files = ['/path/to/custom_dataset1.json', '/path/to/custom_dataset2.json', ...]
+
+若期望使用某个目录下所有的 json 文件作为训练数据集,可做如下修改:
+
+.. code:: diff
+
+ #######################################################################
+ # PART 1 Settings #
+ #######################################################################
+ # Data
+ - data_files = ['/path/to/json/file.json']
+ + data_dir = '/dir/to/custom_dataset'
+
+ #######################################################################
+ # PART 3 Dataset & Dataloader #
+ #######################################################################
+ train_dataset = dict(
+ - dataset=dict(type=load_dataset, path='json', data_files=data_files),
+ + dataset=dict(type=load_dataset, path='json', data_dir=data_dir),
+ ...)
+
+若期望使用 LoRA 算法训练,可做如下修改:
+
+.. code:: diff
+
+ #######################################################################
+ # PART 2 Model & Tokenizer #
+ #######################################################################
+ model = dict(
+ type=SupervisedFinetune,
+ use_varlen_attn=use_varlen_attn,
+ llm=dict(
+ type=AutoModelForCausalLM.from_pretrained,
+ pretrained_model_name_or_path=pretrained_model_name_or_path,
+ trust_remote_code=True),
+ + lora=dict(
+ + type=LoraConfig,
+ + r=64,
+ + lora_alpha=16,
+ + lora_dropout=0.1,
+ + bias='none',
+ + task_type='CAUSAL_LM'))
+
+若期望进行 QLoRA 算法训练,可做如下修改:
+
+.. code:: diff
+
+ #######################################################################
+ # PART 2 Model & Tokenizer #
+ #######################################################################
+ model = dict(
+ type=SupervisedFinetune,
+ use_varlen_attn=use_varlen_attn,
+ llm=dict(
+ type=AutoModelForCausalLM.from_pretrained,
+ pretrained_model_name_or_path=pretrained_model_name_or_path,
+ trust_remote_code=True,
+ + quantization_config=dict(
+ + type=BitsAndBytesConfig,
+ + load_in_4bit=True,
+ + load_in_8bit=False,
+ + llm_int8_threshold=6.0,
+ + llm_int8_has_fp16_weight=False,
+ + bnb_4bit_compute_dtype=torch.float16,
+ + bnb_4bit_use_double_quant=True,
+ + bnb_4bit_quant_type='nf4')
+ ),
+ + lora=dict(
+ + type=LoraConfig,
+ + r=64,
+ + lora_alpha=16,
+ + lora_dropout=0.1,
+ + bias='none',
+ + task_type='CAUSAL_LM')
+ )
+
+步骤 3 :开始训练
+-------------------------
+
+.. code:: bash
+
+ NPROC_PER_NODE=8 xtuner train internlm2_7b_full_custom_pretrain_e1_copy.py --deepspeed deepspeed_zero1
+
+训得模型将默认保存在 ``./work_dirs/``\ ,用户可以通过命令
+``xtuner train --work-dir ${SAVE_PATH}`` 指定保存路径。
+
+步骤 4 :模型转换
+--------------------------
+
+模型训练后会自动保存成 PTH 模型(例如 ``iter_2000.pth``\ ,如果使用了
+DeepSpeed,则将会是一个文件夹),我们需要利用
+``xtuner convert pth_to_hf`` 将其转换为 HuggingFace
+模型,以便于后续使用。具体命令为:
+
+.. code:: bash
+
+ xtuner convert pth_to_hf ${FINETUNE_CFG} ${PTH_PATH} ${SAVE_PATH}
+ # 例如:xtuner convert pth_to_hf internlm2_7b_full_custom_pretrain_e1_copy.py ./iter_2000.pth ./iter_2000_hf
+
+对话
+===========
+
+用户可以利用 ``xtuner chat`` 实现与微调后的模型对话。
+
+如果进行的是全量参数的微调:
+
+.. code:: bash
+
+ xtuner chat ${PATH_TO_LLM} [optional arguments]
+ # 例如:xtuner chat ./iter_2000_hf --max-new-tokens 512
+
+如果使用的是 LoRA 或 QLoRA 算法:
+
+.. code:: bash
+
+ xtuner chat ${NAME_OR_PATH_TO_LLM} --adapter {NAME_OR_PATH_TO_ADAPTER} [optional arguments]
+ # 例如:xtuner chat internlm/internlm2-7b --adapter ./iter_2000_hf --max-new-tokens 512
+
+.. _模型合并可选):
+
+模型合并(可选)
+=======================
+
+如果您使用了 LoRA / QLoRA 微调,则模型转换后将得到 adapter
+参数,而并不包含原 LLM
+参数。如果您期望获得合并后的模型权重(例如用于后续评测),那么可以利用
+``xtuner convert merge`` :
+
+.. code:: bash
+
+ (LLM) xtuner convert merge ${LLM} ${LLM_ADAPTER} ${SAVE_PATH}
+
+评测
+==================
+
+推荐使用一站式平台
+`OpenCompass `__
+来评测大语言模型,其目前已涵盖 50+ 数据集的约 30 万条题目。
diff --git a/docs/zh_cn/training/custom_sft_dataset.rst b/docs/zh_cn/training/custom_sft_dataset.rst
new file mode 100644
index 000000000..75b298934
--- /dev/null
+++ b/docs/zh_cn/training/custom_sft_dataset.rst
@@ -0,0 +1,246 @@
+===================================
+自定义指令微调数据集(LLM)
+===================================
+
+XTuner 支持使用自定义数据集进行指令微调,为便于介绍,本节以
+`internlm2_chat_7b_qlora_custom_sft_e1.py `__
+配置文件为基础进行介绍。
+
+数据准备
+=================
+
+XTuner 采用 `OpenAI SFT
+数据集格式 `__
+作为统一的自定义数据集格式,详细格式如下:
+
+.. code:: json
+
+ [{
+ "messages": [
+ { "role": "system", "content": "xxx."},
+ { "role": "user", "content": "xxx." },
+ { "role": "assistant", "content": "xxx."}
+ ]
+ },
+ {
+ "messages": [
+ { "role": "system", "content": "xxx." },
+ { "role": "user", "content": "xxx." },
+ { "role": "assistant", "content": "xxx.", "loss": False},
+ { "role": "user", "content": "xxx." },
+ { "role": "assistant", "content": "xxx.", "loss": True}
+ ]
+ }]
+
+.. note::
+ 每条数据除了 OpenAI 标准格式中的 ``role``
+ 字段和 ``content`` 字段外,XTuner 还额外扩充了一个 ``loss``
+ 字段,用于控制某轮 ``assistant`` 的输出不计算 loss。
+
+.. note::
+ - ``system`` 和 ``user`` 的 ``loss`` 默认为 False
+ - ``assistant`` 的 ``loss`` 默认为 True
+
+.. tip::
+
+ 若想令某轮对话 "assistant"
+ 部分的内容不参与 loss 计算,需要手动设置该数据 "loss" 字段的值为
+ ``false``\ 。
+
+训练
+=============
+
+步骤 1: 导出 config
+--------------------------------
+
+``xtuner/configs/custom_dataset/sft`` 目录下有所有 XTuner
+支持的模型在自定义数据集下使用 QLora 算法训练的模板 config。可以通过
+``xtuner list-cfg -p custom_sft`` 命令来查看候选 config。下面以
+`internlm2_chat_7b_qlora_custom_sft_e1.py `__
+为例展开介绍。
+
+可以通过以下命令将 ``internlm2_chat_7b_qlora_custom_sft_e1.py``
+导出至当前目录下:
+
+.. code:: console
+
+ $ xtuner copy-cfg internlm2_chat_7b_qlora_custom_sft_e1 .
+
+.. note::
+
+ 当前目录下会存在一个新 config
+ ``internlm2_chat_7b_qlora_custom_sft_e1_copy.py`` 。
+
+步骤 2:修改 config
+----------------------------------
+
+首先,需要修改数据集文件路径:
+
+.. code:: diff
+
+ - data_files = ['/path/to/json/file.json']
+ + data_files = ['/path/to/custom_sft1.json', '/path/to/custom_sft2.json', ...]
+
+若期望使用某个目录下所有的 json 文件作为训练数据集,可做如下修改:
+
+.. code:: diff
+
+ #######################################################################
+ # PART 1 Settings #
+ #######################################################################
+ # Data
+ - data_files = ['/path/to/json/file.json']
+ + data_dir = '/dir/to/custom_sft'
+
+ #######################################################################
+ # PART 3 Dataset & Dataloader #
+ #######################################################################
+ train_dataset = dict(
+ - dataset=dict(type=load_dataset, path='json', data_files=data_files),
+ + dataset=dict(type=load_dataset, path='json', data_dir=data_dir),
+ ...)
+
+若期望使用 Lora 算法训练,可做如下修改:
+
+.. code:: diff
+
+ #######################################################################
+ # PART 2 Model & Tokenizer #
+ #######################################################################
+ model = dict(
+ type=SupervisedFinetune,
+ use_varlen_attn=use_varlen_attn,
+ llm=dict(
+ type=AutoModelForCausalLM.from_pretrained,
+ pretrained_model_name_or_path=pretrained_model_name_or_path,
+ trust_remote_code=True,
+ torch_dtype=torch.float16,
+ - quantization_config=dict(
+ - type=BitsAndBytesConfig,
+ - load_in_4bit=True,
+ - load_in_8bit=False,
+ - llm_int8_threshold=6.0,
+ - llm_int8_has_fp16_weight=False,
+ - bnb_4bit_compute_dtype=torch.float16,
+ - bnb_4bit_use_double_quant=True,
+ - bnb_4bit_quant_type='nf4')
+ ),
+ lora=dict(
+ type=LoraConfig,
+ r=64,
+ lora_alpha=16,
+ lora_dropout=0.1,
+ bias='none',
+ task_type='CAUSAL_LM'))
+
+若期望进行全量参数训练,可做如下修改:
+
+.. code:: diff
+
+ #######################################################################
+ # PART 2 Model & Tokenizer #
+ #######################################################################
+ model = dict(
+ type=SupervisedFinetune,
+ use_varlen_attn=use_varlen_attn,
+ llm=dict(
+ type=AutoModelForCausalLM.from_pretrained,
+ pretrained_model_name_or_path=pretrained_model_name_or_path,
+ trust_remote_code=True,
+ torch_dtype=torch.float16,
+ - quantization_config=dict(
+ - type=BitsAndBytesConfig,
+ - load_in_4bit=True,
+ - load_in_8bit=False,
+ - llm_int8_threshold=6.0,
+ - llm_int8_has_fp16_weight=False,
+ - bnb_4bit_compute_dtype=torch.float16,
+ - bnb_4bit_use_double_quant=True,
+ - bnb_4bit_quant_type='nf4')
+ ),
+ - lora=dict(
+ - type=LoraConfig,
+ - r=64,
+ - lora_alpha=16,
+ - lora_dropout=0.1,
+ - bias='none',
+ - task_type='CAUSAL_LM')
+ )
+
+步骤 3: 开始训练
+-----------------------------
+
+.. code:: console
+
+ $ NPROC_PER_NODE=8 xtuner train internlm2_chat_7b_qlora_custom_sft_e1_copy.py --deepspeed deepspeed_zero1
+
+.. tip::
+ 训练日志及 checkpoint 将默认保存在 ``./work_dirs/``\ ,可以通过命令
+ ``xtuner train --work-dir ${SAVE_PATH}`` 指定保存路径。
+
+步骤 4: 模型转换
+------------------------------
+
+模型训练后会自动保存成 PTH 模型(例如 ``iter_2000.pth``\ ,如果使用了
+DeepSpeed,则将会是一个文件夹),我们需要利用
+``xtuner convert pth_to_hf`` 将其转换为 HuggingFace
+模型,以便于后续使用。具体命令为:
+
+.. code:: bash
+
+ xtuner convert pth_to_hf ${FINETUNE_CFG} ${PTH_PATH} ${SAVE_PATH}
+ # 例如:xtuner convert pth_to_hf internlm2_chat_7b_qlora_custom_sft_e1_copy.py ./iter_2000.pth ./iter_2000_hf
+
+对话
+=================
+
+用户可以利用 ``xtuner chat`` 实现与微调后的模型对话。如果使用的是 Lora
+或 QLora 算法:
+
+.. code:: console
+
+ $ xtuner chat ${NAME_OR_PATH_TO_LLM} --adapter {NAME_OR_PATH_TO_ADAPTER} --prompt-template ${PROMPT_TEMPLATE} [optional arguments]
+ $ # 例如:xtuner chat internlm/internlm2-7b --adapter ./iter_2000_hf --prompt-template internlm2_chat
+
+
+如果进行的是全量参数的微调:
+
+.. code:: console
+
+ $ xtuner chat ${PATH_TO_LLM} --prompt-template ${PROMPT_TEMPLATE} [optional arguments]
+ $ # 例如:xtuner chat ./iter_2000_hf --prompt-template internlm2_chat
+
+.. note::
+
+ 其中 ${PROMPT_TEMPLATE} 表示模型的对话模板,需要与训练用的 config 中的
+ ``prompt_template`` 字段保持一致,例如
+ ``internlm2_chat_7b_qlora_custom_sft_e1_copy.py`` 中的设置为:
+
+ .. code:: python
+
+ prompt_template = PROMPT_TEMPLATE.internlm2_chat
+
+.. _模型合并可选):
+
+模型合并(可选)
+======================
+
+如果您使用了 LoRA / QLoRA 微调,则模型转换后将得到 adapter
+参数,而并不包含原 LLM
+参数。如果您期望获得合并后的模型权重(例如用于后续评测),那么可以利用
+``xtuner convert merge`` :
+
+.. code:: console
+
+ $ xtuner convert merge ${LLM} ${LLM_ADAPTER} ${SAVE_PATH}
+
+.. tip::
+
+ 模型合并后,就得到了一个可以通过 ``AutoModelForCausalLM.from_pretrained`` 直接加载的模型,可以直接在各种下游工具中直接使用
+
+评测
+======================
+
+推荐使用一站式平台
+`OpenCompass `__
+来评测大语言模型,其目前已涵盖 50+ 数据集的约 30 万条题目。
diff --git a/docs/zh_cn/training/modify_settings.rst b/docs/zh_cn/training/modify_settings.rst
new file mode 100644
index 000000000..619dbe553
--- /dev/null
+++ b/docs/zh_cn/training/modify_settings.rst
@@ -0,0 +1,473 @@
+============
+修改训练配置
+============
+
+XTuner 的训练由 MMEngine
+的训练器提供支持,用户可以通过修改配置文件(config)中的特定参数,来修改对应的训练配置。以
+`internlm2_chat_7b_qlora_oasst1_e3 `__
+为例,本节将首先速览配置文件中各个参数的含义,之后讲解常见配置的修改方式。
+
+配置文件速览
+============
+
+XTuner 使用 MMEngine 的「纯 Python 风格的配置文件」,直接利用 ``import``
+机制使用一些类或函数。
+
+.. tip::
+
+ 如果您期望深入了解 MMEngine 「纯 Python
+ 风格的配置文件」的特性、优势,请参考
+ `这里 `__\ 。
+
+.. code:: python
+
+ # Copyright (c) OpenMMLab. All rights reserved.
+ import torch
+ from datasets import load_dataset
+ from mmengine.dataset import DefaultSampler
+ from mmengine.hooks import (CheckpointHook, DistSamplerSeedHook, IterTimerHook,
+ LoggerHook, ParamSchedulerHook)
+ from mmengine.optim import AmpOptimWrapper, CosineAnnealingLR, LinearLR
+ from peft import LoraConfig
+ from torch.optim import AdamW
+ from transformers import (AutoModelForCausalLM, AutoTokenizer,
+ BitsAndBytesConfig)
+
+ from xtuner.dataset import process_hf_dataset
+ from xtuner.dataset.collate_fns import default_collate_fn
+ from xtuner.dataset.map_fns import oasst1_map_fn, template_map_fn_factory
+ from xtuner.engine.hooks import (DatasetInfoHook, EvaluateChatHook,
+ VarlenAttnArgsToMessageHubHook)
+ from xtuner.engine.runner import TrainLoop
+ from xtuner.model import SupervisedFinetune
+ from xtuner.utils import PROMPT_TEMPLATE
+
+ #######################################################################
+ # PART 1 Settings #
+ #######################################################################
+ # Model
+ pretrained_model_name_or_path = 'internlm/internlm2-chat-7b' # 设置 LLM 路径或 HuggingFace Hub ID
+ use_varlen_attn = False # 是否使用 varlen_attention
+
+ # Data
+ data_path = 'timdettmers/openassistant-guanaco' # 设置 dataset 路径或 HuggingFace Hub ID,以用于 datasets.load_dataset
+ prompt_template = PROMPT_TEMPLATE.internlm2_chat # 设置对话模版
+ max_length = 2048 # 设置训练数据截断长度
+ pack_to_max_length = True # 是否将多条样本打包为一条最长长度的样本
+
+ # Scheduler & Optimizer
+ batch_size = 1 # per_device # 每个设备的样本个数
+ accumulative_counts = 16 # 梯度累计数
+ dataloader_num_workers = 0 # dataloader worker 数
+ max_epochs = 3 # 训练迭代代数
+ optim_type = AdamW # 优化器
+ lr = 2e-4 # 学习率
+ betas = (0.9, 0.999) # AdamW 优化器 betas
+ weight_decay = 0 # AdamW 优化器权重衰减
+ max_norm = 1 # grad clip # 梯度裁剪
+ warmup_ratio = 0.03 # warmup 比率
+
+ # Save
+ save_steps = 500 # checkpoint 保存间隔(iter 数)
+ save_total_limit = 2 # 最大保存 checkpoint 个数,-1 表示无限制
+
+ # Evaluate the generation performance during the training
+ evaluation_freq = 500 # 验证对话效果的执行间隔(iter 数)
+ SYSTEM = '' # 验证对话效果的 system 字段
+ evaluation_inputs = [ # 验证对话效果时的测试问题
+ '请给我介绍五个上海的景点', 'Please tell me five scenic spots in Shanghai'
+ ]
+
+ #######################################################################
+ # PART 2 Model & Tokenizer #
+ #######################################################################
+ tokenizer = dict( # 构建 tokenizer
+ type=AutoTokenizer.from_pretrained,
+ pretrained_model_name_or_path=pretrained_model_name_or_path,
+ trust_remote_code=True,
+ padding_side='right')
+
+ model = dict( # 构建 model
+ type=SupervisedFinetune,
+ use_varlen_attn=use_varlen_attn,
+ llm=dict( # 构建 LLM
+ type=AutoModelForCausalLM.from_pretrained,
+ pretrained_model_name_or_path=pretrained_model_name_or_path,
+ trust_remote_code=True,
+ torch_dtype=torch.float16,
+ quantization_config=dict( # 量化配置(保留则为 4 比特,删除则为正常浮点)
+ type=BitsAndBytesConfig,
+ load_in_4bit=True,
+ load_in_8bit=False,
+ llm_int8_threshold=6.0,
+ llm_int8_has_fp16_weight=False,
+ bnb_4bit_compute_dtype=torch.float16,
+ bnb_4bit_use_double_quant=True,
+ bnb_4bit_quant_type='nf4')),
+ lora=dict( # LoRA 配置(保留则使用 LoRA 微调,删除则使用全量微调)
+ type=LoraConfig,
+ r=64,
+ lora_alpha=16,
+ lora_dropout=0.1,
+ bias='none',
+ task_type='CAUSAL_LM'))
+
+ #######################################################################
+ # PART 3 Dataset & Dataloader #
+ #######################################################################
+ train_dataset = dict( # 构建训练数据集
+ type=process_hf_dataset,
+ dataset=dict(type=load_dataset, path=data_path), # 调用 datasets.load_dataset 接口
+ tokenizer=tokenizer,
+ max_length=max_length,
+ dataset_map_fn=oasst1_map_fn, # 选择匹配的数据集 map_fn
+ template_map_fn=dict(
+ type=template_map_fn_factory, template=prompt_template),
+ remove_unused_columns=True,
+ shuffle_before_pack=True,
+ pack_to_max_length=pack_to_max_length,
+ use_varlen_attn=use_varlen_attn)
+
+ train_dataloader = dict( # 构建训练数据集的 DataLoader
+ batch_size=batch_size,
+ num_workers=dataloader_num_workers,
+ dataset=train_dataset,
+ sampler=dict(type=DefaultSampler, shuffle=True),
+ collate_fn=dict(type=default_collate_fn, use_varlen_attn=use_varlen_attn))
+
+ #######################################################################
+ # PART 4 Scheduler & Optimizer #
+ #######################################################################
+ # optimizer
+ optim_wrapper = dict( # 构建优化器
+ type=AmpOptimWrapper,
+ optimizer=dict(
+ type=optim_type, lr=lr, betas=betas, weight_decay=weight_decay),
+ clip_grad=dict(max_norm=max_norm, error_if_nonfinite=False),
+ accumulative_counts=accumulative_counts,
+ loss_scale='dynamic',
+ dtype='float16')
+
+ # learning policy
+ # More information: https://github.com/open-mmlab/mmengine/blob/main/docs/en/tutorials/param_scheduler.md # noqa: E501
+ param_scheduler = [ # 设置学习率 scheduler
+ dict(
+ type=LinearLR, # warmup 阶段
+ start_factor=1e-5,
+ by_epoch=True,
+ begin=0,
+ end=warmup_ratio * max_epochs,
+ convert_to_iter_based=True),
+ dict(
+ type=CosineAnnealingLR, # Cosine 学习率衰减阶段
+ eta_min=0.0,
+ by_epoch=True,
+ begin=warmup_ratio * max_epochs,
+ end=max_epochs,
+ convert_to_iter_based=True)
+ ]
+
+ # train, val, test setting
+ train_cfg = dict(type=TrainLoop, max_epochs=max_epochs) # 设置训练迭代代数
+
+ #######################################################################
+ # PART 5 Runtime #
+ #######################################################################
+ # Log the dialogue periodically during the training process, optional
+ custom_hooks = [ # 定义 Hooks
+ dict(type=DatasetInfoHook, tokenizer=tokenizer), # 在训练前打印可视化打印数据样本
+ dict(
+ type=EvaluateChatHook, # 在训练时测试对话效果
+ tokenizer=tokenizer,
+ every_n_iters=evaluation_freq,
+ evaluation_inputs=evaluation_inputs,
+ system=SYSTEM,
+ prompt_template=prompt_template)
+ ]
+
+ if use_varlen_attn:
+ custom_hooks += [dict(type=VarlenAttnArgsToMessageHubHook)] # vallen_attention 依赖的 Hook
+
+ # 以下均为默认配置,如需调整请参考 MMEngine 文档及代码
+
+ # configure default hooks
+ default_hooks = dict(
+ # record the time of every iteration.
+ timer=dict(type=IterTimerHook),
+ # print log every 10 iterations.
+ logger=dict(type=LoggerHook, log_metric_by_epoch=False, interval=10),
+ # enable the parameter scheduler.
+ param_scheduler=dict(type=ParamSchedulerHook),
+ # save checkpoint per `save_steps`.
+ checkpoint=dict(
+ type=CheckpointHook,
+ by_epoch=False,
+ interval=save_steps,
+ max_keep_ckpts=save_total_limit),
+ # set sampler seed in distributed evrionment.
+ sampler_seed=dict(type=DistSamplerSeedHook),
+ )
+
+ # configure environment
+ env_cfg = dict(
+ # whether to enable cudnn benchmark
+ cudnn_benchmark=False,
+ # set multi process parameters
+ mp_cfg=dict(mp_start_method='fork', opencv_num_threads=0),
+ # set distributed parameters
+ dist_cfg=dict(backend='nccl'),
+ )
+
+ # set visualizer
+ visualizer = None
+
+ # set log level
+ log_level = 'INFO'
+
+ # load from which checkpoint
+ load_from = None
+
+ # whether to resume training from the loaded checkpoint
+ resume = False
+
+ # Defaults to use random seed and disable `deterministic`
+ randomness = dict(seed=None, deterministic=False)
+
+ # set log processor
+ log_processor = dict(by_epoch=False)
+
+常见训练配置修改
+=======================
+
+模型
+------------
+
+使用其他 LLM 模型?
+~~~~~~~~~~~~~~~~~~~~~~~~
+1. 修改 ``pretrained_model_name_or_path``\ ,其将应用至 ``model.llm`` 和 ``tokenizer`` 的初始化中。
+#. 修改 ``prompt_template`` 以适配所选择的 LLM。
+
+使用 ModelScope 模型?
+~~~~~~~~~~~~~~~~~~~~~~~~~~~~
+1. 参考 `文档 <../preparation/pretrained_model.md>`__ 将其下载至本地
+2. 修改\ ``pretrained_model_name_or_path``\ 。
+
+使用 openMind 模型?
+~~~~~~~~~~~~~~~~~~~~~~~~~~~~
+可在配置文件中新增 ``model_resource`` 参数, ``args`` 用作可变参数(如下载私有模型需传入token的情况):
+
+.. code:: python
+ from openmind_hub import snapshot_download
+
+ # Model
+ pretrained_model_name_or_path = 'Tianjin_Ascend/Qwen1.5-4B'
+ model_resource = {
+ "fn": snapshot_download,
+ "args":{
+ # "token":"xxxxxxxxxx"
+ }
+ }
+
+微调类型
+-------------
+
+.. tip::
+ XTuner 内置的配置文件以 QLoRA 微调为主,但并不意味着 XTuner 仅支持 QLoRA
+ 微调。用户可以通过修改配置文件中的 ``model`` 来决定微调类型。
+
+
+QLoRA 微调
+~~~~~~~~~~~~~~~~~
+
+.. code:: python
+
+ model = dict(
+ ......
+ llm=dict(
+ type=AutoModelForCausalLM.from_pretrained,
+ pretrained_model_name_or_path=pretrained_model_name_or_path,
+ trust_remote_code=True,
+ torch_dtype=torch.float16,
+ quantization_config=dict(
+ type=BitsAndBytesConfig,
+ load_in_4bit=True,
+ load_in_8bit=False,
+ llm_int8_threshold=6.0,
+ llm_int8_has_fp16_weight=False,
+ bnb_4bit_compute_dtype=torch.float16,
+ bnb_4bit_use_double_quant=True,
+ bnb_4bit_quant_type='nf4')),
+ lora=dict(
+ type=LoraConfig,
+ r=64,
+ lora_alpha=16,
+ lora_dropout=0.1,
+ bias='none',
+ task_type='CAUSAL_LM'),
+ ......)
+
+
+LoRA 微调
+~~~~~~~~~~~~~~~~
+
+.. tip::
+
+ 在 QLoRA 设置的基础上,将 `quantization_config` 设置为 None,就切换成了 LoRA 微调
+
+.. code:: python
+
+ model = dict(
+ ......
+ llm=dict(
+ type=AutoModelForCausalLM.from_pretrained,
+ pretrained_model_name_or_path=pretrained_model_name_or_path,
+ trust_remote_code=True,
+ torch_dtype=torch.float16,
+ quantization_config=None),
+ lora=dict(
+ type=LoraConfig,
+ r=64,
+ lora_alpha=16,
+ lora_dropout=0.1,
+ bias='none',
+ task_type='CAUSAL_LM'),
+ ......)
+
+
+全参数微调
+~~~~~~~~~~~~~~~~~~
+.. tip::
+
+ 将 `lora` 和 `quantization_config` 都设置为 None,就切换到了全参数训练模式
+
+.. code:: python
+
+ model = dict(
+ ......
+ llm=dict(
+ type=AutoModelForCausalLM.from_pretrained,
+ pretrained_model_name_or_path=pretrained_model_name_or_path,
+ trust_remote_code=True,
+ torch_dtype=torch.float16,
+ quantization_config=None),
+ lora=None,
+ ......)
+
+
+
+
+数据集
+--------------
+
+请参考 `训练` 章节文档。
+
+优化器
+-----------
+
+使用其他优化器?
+~~~~~~~~~~~~~~~~~~~~
+
+- 方法 1:修改 ``optim_type``\ (例如 ``optim_type=torch.optim.SGD``\ ),其将应用至 ``optim_wrapper.optimzer``\ 。
+- 方法 2:忽略 ``optim_type``\ ,直接修改 ``optim_wrapper.optimzer``\ 。
+
+
+修改优化器参数配置?
+~~~~~~~~~~~~~~~~~~~~~~~~
+
+- 方法 1:修改 ``lr``\ 、\ ``weight_decay`` 等参数,其将应用至 ``optim_wrapper.optimzer``\ 。
+- 方法 2:直接修改 ``optim_wrapper.optimzer``\ 。
+
+迭代次数
+---------------
+
+调整迭代次数?
+~~~~~~~~~~~~~~~~~~~~~
+
+- 修改 ``max_epochs`` 参数。
+
+保存 Checkpoint 间隔
+---------------------------
+
+调整保存间隔?
+~~~~~~~~~~~~~~~~~~~~~
+
+- 修改 ``save_steps`` 参数。
+
+调整最大保存 checkpoint 个数?
+~~~~~~~~~~~~~~~~~~~~~~~~~~~~~~~~~~~~~~~
+
+- 修改 ``save_total_limit`` 参数。
+
+训练间对话评测
+----------------------
+
+调整对话评测间隔?
+~~~~~~~~~~~~~~~~~~~~~~~~~~
+
+- 修改 ``evaluation_freq`` 参数。
+
+调整对话评测的 system 字段?
+~~~~~~~~~~~~~~~~~~~~~~~~~~~~~~~~~~~~~~
+
+- 修改 ``SYSTEM`` 参数。
+
+调整对话评测的测试指令?
+~~~~~~~~~~~~~~~~~~~~~~~~~~~~~~~~~~~
+
+- 修改 ``evaluation_inputs`` 参数。
+
+GPU 数
+--------------
+
+XTuner
+的多卡训练由启动命令决定,而非配置文件。用户可以参考下列命令启动多卡训练:
+
+.. code:: bash
+
+ # 单卡
+ xtuner train ${CONFIG}
+ # 多卡
+ (DIST) NPROC_PER_NODE=${GPU_NUM} xtuner train ${CONFIG}
+ (SLURM) srun ${SRUN_ARGS} xtuner train ${CONFIG} --launcher slurm
+
+DeepSpeed
+------------------
+
+XTuner 的 DeepSpeed
+优化由启动命令决定,而非配置文件。用户可以参考下列命令启用 DeepSpeed
+优化:
+
+.. code:: bash
+
+ xtuner train ${CONFIG} --deepspeed ${DS_CONFIG}
+
+.. note::
+
+ XTuner 内置了多个 DeepSpeed 配置文件(即命令中的
+ ``${DS_CONFIG}``\ ),用户可以直接使用,具体文件见
+ `这里 `__\ :
+
+ .. code:: bash
+
+ xtuner train ${CONFIG} --deepspeed [deepspeed_zero1,deepspeed_zero2,deepspeed_zero2_offload,deepspeed_zero3,deepspeed_zero3_offload]
+
+.. note::
+ 部分参数会在 DeepSpeed Config 和 XTuner Config 中重复定义(例如 batch
+ size等)。此时相关配置会以 XTuner Config 为准:
+
+ - ``gradient_accumulation_steps`` 会被 XTuner Config 中的
+ ``accumulative_counts`` 设置覆盖。
+
+ - ``train_micro_batch_size_per_gpu`` 会被 XTuner Config 中的
+ ``train_dataloader.batch_size`` 设置覆盖。
+
+ - ``gradient_clipping`` 会被 XTuner Config 中的
+ ``optim_wrapper.clip_grad.max_norm`` 设置覆盖。
+
+ - XTuner 会根据所使用的 GPU 架构自动选择 ``fp16`` 或 ``bf16`` 训练。
+
+其他
+----------
+
+如有遗漏或特定需求,欢迎提出
+`issue `__ 讨论。
diff --git a/docs/zh_cn/training/multi_modal_dataset.rst b/docs/zh_cn/training/multi_modal_dataset.rst
new file mode 100644
index 000000000..541dcec7a
--- /dev/null
+++ b/docs/zh_cn/training/multi_modal_dataset.rst
@@ -0,0 +1,296 @@
+==========================
+多模态数据集 (VLM)
+==========================
+
+XTuner 支持 LLaVA 图文模型的微调,本文将以
+`xtuner/llava-internlm2-7b `__
+为例,讲解如何利用 XTuner 快速上手多模态数据集训练,及后续的对话、评测。
+
+数据准备
+========
+
+XTuner 支持 LLaVA 格式数据集的多模态图文预训练、微调。本节将从「LLaVA
+开源数据集准备」和「自定义数据集准备」两部分展开介绍。
+
+LLaVA 开源数据集准备
+-----------------------------
+
+数据文件结构
+^^^^^^^^^^^^
+
+.. code::
+
+ ./data/llava_data
+ ├── LLaVA-Pretrain
+ │ ├── blip_laion_cc_sbu_558k.json
+ │ ├── blip_laion_cc_sbu_558k_meta.json
+ │ └── images
+ ├── LLaVA-Instruct-150K
+ │ └── llava_v1_5_mix665k.json
+ └── llava_images
+ ├── coco
+ │ └── train2017
+ ├── gqa
+ │ └── images
+ ├── ocr_vqa
+ │ └── images
+ ├── textvqa
+ │ └── train_images
+ └── vg
+ ├── VG_100K
+ └── VG_100K_2
+
+预训练数据下载
+^^^^^^^^^^^^^^
+
+LLaVA-Pretrain
+
+.. code:: bash
+
+ # Make sure you have git-lfs installed (https://git-lfs.com)
+ git lfs install
+ git clone https://huggingface.co/datasets/liuhaotian/LLaVA-Pretrain --depth=1
+
+指令微调数据下载
+^^^^^^^^^^^^^^^^
+
+**LLaVA-Instruct-150K** (文本)
+
+.. code:: bash
+
+ # Make sure you have git-lfs installed (https://git-lfs.com)
+ git lfs install
+ git clone https://huggingface.co/datasets/liuhaotian/LLaVA-Instruct-150K --depth=1
+
+
+**COCO** (图像): `train2017 `__
+
+**GQA** (图像): `images `__
+
+**TextVQA** (图像): `train_val_images `__
+
+**VisualGenome** (图像): `part1 `__, `part2 `__
+
+**OCR-VQA** (图像): `download script `__
+
+.. tip::
+ ⚠️ OCR-VQA 所下载的图片命名需要利用如下脚本进行处理,以确保所有图片后缀为
+ ``.jpg``\ !
+
+ .. code:: bash
+
+ #!/bin/bash
+ ocr_vqa_path=""
+
+ find "$target_dir" -type f | while read file; do
+ extension="${file##*.}"
+ if [ "$extension" != "jpg" ]
+ then
+ cp -- "$file" "${file%.*}.jpg"
+ fi
+ done
+
+
+自定义数据集准备
+----------------
+
+如果用户期望使用自定义数据集进行图文训练,可以参照 LLaVA
+开源数据集格式进行准备,具体格式如下:
+
+.. code:: json
+
+ [
+ {
+ "image": "xxx/xxx",
+ "conversations": [
+ {
+ "from": "human",
+ "value": "\nHello! What's this?"
+ },
+ {
+ "from": "gpt",
+ "value": "This is a dog!"
+ },
+ {
+ "from": "human",
+ "value": "Is it cute?"
+ },
+ {
+ "from": "gpt",
+ "value": "Yes."
+ }
+ ]
+ },
+ ...
+ ]
+
+.. note::
+ 目前针对自定义数据有一些约束:
+
+ 1. ``image`` 字段表示图片路径,且仅能有一张图片
+
+ 2. ``conversations`` 字段第 0 条的 ``value`` 需要包括 ````
+ ,以确保图片被正确嵌入。
+
+训练
+=====
+
+多模态图文训练一般分为两步:预训练(pretrain)、指令跟随微调(finetune)。\ ``xtuner/llava-internlm2-7b``
+对应的配置文件:\ `预训练 `__
+/
+`指令跟随微调 `__\ ,用户可以对其中的模型路径、数据路径进行自定义修改。
+
+预训练
+------
+
+.. code:: console
+
+ $ NPROC_PER_NODE=8 xtuner train llava_internlm2_chat_7b_clip_vit_large_p14_336_e1_gpu8_pretrain --deepspeed deepspeed_zero2
+
+.. tip::
+ 训得模型将默认保存在 ``./work_dirs/``\ ,用户可以通过命令
+ ``xtuner train --work-dir ${SAVE_PATH}`` 指定保存路径。
+
+指令跟随微调
+-----------------
+
+指令跟随微调时,需要载入预训练阶段所得到的 ``.pth``
+模型,以提供良好的初始化,这一通过在配置文件中的 ``pretrained_pth``
+指定,用户可以自行修改。
+
+.. code:: console
+
+ $ NPROC_PER_NODE=8 xtuner train llava_internlm2_chat_7b_qlora_clip_vit_large_p14_336_lora_e1_gpu8_finetune --deepspeed deepspeed_zero2
+
+模型转换
+--------
+
+模型训练后会自动保存成 PTH 模型(例如
+``iter_5198.pth``\ ),我们需要利用 ``xtuner convert pth_to_hf``
+将其转换为 HuggingFace 模型,以便于后续使用。具体命令为:
+
+.. code:: console
+
+ $ xtuner convert pth_to_hf $FINETUNE_CFG $PTH_PATH $SAVE_PATH
+ $ # 例如:xtuner convert pth_to_hf llava_internlm2_chat_7b_qlora_clip_vit_large_p14_336_lora_e1_gpu8_finetune ./iter_5198.pth ./iter_5198_hf
+
+.. note::
+ 此时,我们将获得所需要的模型。如果使用默认的微调范式,文件结构应与
+ `这里 `__
+ 一致。
+
+
+
+模型合并(可选)
+-------------------
+
+如果您使用了 LoRA / QLoRA 微调,则模型转换后将得到 adapter
+参数,而并不包含原 LLM
+参数。如果您期望获得合并后的模型权重,那么可以利用
+``xtuner convert merge`` :
+
+.. code:: console
+
+ $ xtuner convert merge $LLM $LLM_ADAPTER $SAVE_PATH
+ $ xtuner convert merge $CLIP $CLIP_ADAPTER $SAVE_PATH --is-clip
+
+对话
+=====
+
+用户可以利用 ``xtuner chat``
+实现与微调后的多模态图文模型对话。假设模型转换阶段获得的模型路径为
+``./iter_5198_hf``\ ,则我们可以利用下列命令实现对话:
+
+.. code:: console
+
+ $ xtuner chat internlm/internlm2-chat-7b \
+ $ --visual-encoder openai/clip-vit-large-patch14-336 \
+ $ --llava ./iter_5198_hf \
+ $ --prompt-template internlm2_chat \
+ $ --image $IMAGE_PATH
+
+.. note::
+
+ ``xtuner chat`` 的第一个参数为 LLM 路径或 HuggingFace Hub
+ ID。如果训练阶段 LLM 使用的是 LoRA / QLoRA 微调,则此参数请传入基础
+ LLM,如
+ ``internlm/internlm2-chat-7b``\ ;如果使用的是全参数微调,则此参数请传入转换(\ ``xtuner convert pth_to_hf``\ )所得到的模型权重,如
+ ``./iter_5198_hf``\ 。
+
+评测
+====
+
+XTuner 的 LLaVA 模型可以利用
+`VLMEvalKit `__
+进行评测,请参考
+`这里 `__
+快速上手。
+
+同时,为了方便使用,XTuner 内也集成了 MMBench
+评测,您可以通过下列命令下载 MMBench 评测数据集:
+
+.. code:: console
+
+ $ wget https://opencompass.openxlab.space/utils/VLMEval/MMBench_DEV_EN.tsv
+ $ wget https://opencompass.openxlab.space/utils/VLMEval/MMBench_TEST_EN.tsv
+ $ wget https://opencompass.openxlab.space/utils/VLMEval/MMBench_DEV_CN.tsv
+ $ wget https://opencompass.openxlab.space/utils/VLMEval/MMBench_TEST_CN.tsv
+ $ wget https://opencompass.openxlab.space/utils/VLMEval/CCBench.tsv
+
+之后,您可以利用下列命令实现评测:
+
+.. code:: console
+
+ $ xtuner mmbench internlm/internlm2-chat-7b \
+ $ --visual-encoder openai/clip-vit-large-patch14-336 \
+ $ --llava ./iter_5198_hf \
+ $ --prompt-template internlm2_chat \
+ $ --data-path $DATA_PATH \
+ $ --work-dir $RESULT_PATH
+
+.. note::
+
+ ``xtuner mmbench`` 的第一个参数为 LLM 路径或 HuggingFace Hub
+ ID。如果训练阶段 LLM 使用的是 LoRA / QLoRA 微调,则此参数请传入基础
+ LLM,如
+ ``internlm/internlm2-chat-7b``\ ;如果使用的是全参数微调,则此参数请传入转换(\ ``xtuner convert pth_to_hf``\ )所得到的模型权重,如
+ ``./iter_5198_hf``\ 。
+
+.. note::
+
+ ``$DATA_PATH`` 指上一步骤所下载的某一个 tsv 文件,如
+ ``MMBench_DEV_EN.tsv``\ 。
+
+.. note::
+ 评测完成后,若为开发集则会直接打印出结果;若为测试集,则需将
+ ``mmbench_result.xlsx`` 提交至 `MMBench
+ 官方 `__ 完成评测取得精度结果。
+
+FAQ
+====
+
+如何更换 LLM?
+----------------------
+
+修改 LLM 的方式与训练单模态的大语言模型类似。
+
+1. 修改配置文件中的 ``llm_name_or_path`` 参数至您期望使用的 LLM,例如
+ ``internlm/internlm2-chat-20b``\ 等。
+
+2. 修改配置文件中的 ``prompt_template`` 参数,与您所选择的 LLM
+ 保持对齐,具体选择可参考
+ \ :ref:`对话模版文档 ` \ 。
+
+
+ValueError: ``bos_token_id`` has to be defined when no ``input_ids`` are provided.
+-------------------------------------------------------------------------------------
+
+这是由于老版本 ``transformers`` 的 LLM ``generate`` 接口在接受
+``inputs_embeds`` 输入时,必须传入有效的 ``bos_token_id``\ 。
+(`#29772 `__)
+
+更新 ``transformers`` 即可解决
+
+.. code:: console
+
+ $ pip install -U transformers
diff --git a/docs/zh_cn/training/open_source_dataset.rst b/docs/zh_cn/training/open_source_dataset.rst
new file mode 100644
index 000000000..380ba0db3
--- /dev/null
+++ b/docs/zh_cn/training/open_source_dataset.rst
@@ -0,0 +1,213 @@
+================================
+开源指令微调数据集(LLM)
+================================
+
+HuggingFace Hub 中有众多优秀的开源数据,本节将以
+`timdettmers/openassistant-guanaco `__
+开源指令微调数据集为例,讲解如何开始训练。为便于介绍,本节以
+`internlm2_chat_7b_qlora_oasst1_e3 `__
+配置文件为基础进行讲解。
+
+适配开源数据集
+=====================
+
+不同的开源数据集有不同的数据「载入方式」和「字段格式」,因此我们需要针对所使用的开源数据集进行一些适配。
+
+载入方式
+-----------
+
+XTuner 使用上游库 ``datasets`` 的统一载入接口 ``load_dataset``\ 。
+
+.. code:: python
+
+ data_path = 'timdettmers/openassistant-guanaco'
+ train_dataset = dict(
+ type=process_hf_dataset,
+ dataset=dict(type=load_dataset, path=data_path),
+ ...)
+
+.. tip::
+ 一般来说,若想要使用不同的开源数据集,用户只需修改
+ ``dataset=dict(type=load_dataset, path=data_path)`` 中的 ``path``
+ 参数即可。
+
+ 若想使用 openMind 数据集,可将 ``dataset=dict(type=load_dataset, path=data_path)`` 中的 ``type`` 替换为 ``openmind.OmDataset``。
+
+
+字段格式
+--------
+
+为适配不同的开源数据集的字段格式,XTuner 开发并设计了一套 ``map_fn`` 机制,可以把不同的开源数据集转为统一的字段格式
+
+.. code:: python
+
+ from xtuner.dataset.map_fns import oasst1_map_fn
+ train_dataset = dict(
+ type=process_hf_dataset,
+ ...
+ dataset_map_fn=oasst1_map_fn,
+ ...)
+
+XTuner 内置了众多 map_fn
+(\ `这里 `__\ ),可以满足大多数开源数据集的需要。此处我们罗列一些常用
+map_fn 及其对应的原始字段和参考数据集:
+
++------------------------------------------------------------------------------------------------------------------------------------+---------------------------------------------------+-----------------------------------------------------------------------------------------------------------------------+
+| map_fn | Columns | Reference Datasets |
++====================================================================================================================================+===================================================+=======================================================================================================================+
+| `alpaca_map_fn `__ | ['instruction', 'input', 'output', ...] | `tatsu-lab/alpaca `__ |
++------------------------------------------------------------------------------------------------------------------------------------+---------------------------------------------------+-----------------------------------------------------------------------------------------------------------------------+
+| `alpaca_zh_map_fn `__ | ['instruction_zh', 'input_zh', 'output_zh', ...] | `silk-road/alpaca-data-gpt4-chinese `__ |
++------------------------------------------------------------------------------------------------------------------------------------+---------------------------------------------------+-----------------------------------------------------------------------------------------------------------------------+
+| `oasst1_map_fn `__ | ['text', ...] | `timdettmers/openassistant-guanaco `__ |
++------------------------------------------------------------------------------------------------------------------------------------+---------------------------------------------------+-----------------------------------------------------------------------------------------------------------------------+
+| `openai_map_fn `__ | ['messages', ...] | `DavidLanz/fine_tuning_datraset_4_openai `__ |
++------------------------------------------------------------------------------------------------------------------------------------+---------------------------------------------------+-----------------------------------------------------------------------------------------------------------------------+
+| `code_alpaca_map_fn `__ | ['prompt', 'completion', ...] | `HuggingFaceH4/CodeAlpaca_20K `__ |
++------------------------------------------------------------------------------------------------------------------------------------+---------------------------------------------------+-----------------------------------------------------------------------------------------------------------------------+
+| `medical_map_fn `__ | ['instruction', 'input', 'output', ...] | `shibing624/medical `__ |
++------------------------------------------------------------------------------------------------------------------------------------+---------------------------------------------------+-----------------------------------------------------------------------------------------------------------------------+
+| `tiny_codes_map_fn `__ | ['prompt', 'response', ...] | `nampdn-ai/tiny-codes `__ |
++------------------------------------------------------------------------------------------------------------------------------------+---------------------------------------------------+-----------------------------------------------------------------------------------------------------------------------+
+| `default_map_fn `__ | ['input', 'output', ...] | / |
++------------------------------------------------------------------------------------------------------------------------------------+---------------------------------------------------+-----------------------------------------------------------------------------------------------------------------------+
+
+例如,针对 ``timdettmers/openassistant-guanaco`` 数据集,XTuner 内置了
+``oasst1_map_fn``\ ,以对其进行字段格式统一。具体实现如下:
+
+.. code:: python
+
+ def oasst1_map_fn(example):
+ r"""Example before preprocessing:
+ example['text'] = ('### Human: Can you explain xxx'
+ '### Assistant: Sure! xxx'
+ '### Human: I didn't understand how xxx'
+ '### Assistant: It has to do with a process xxx.')
+
+ Example after preprocessing:
+ example['conversation'] = [
+ {
+ 'input': 'Can you explain xxx',
+ 'output': 'Sure! xxx'
+ },
+ {
+ 'input': 'I didn't understand how xxx',
+ 'output': 'It has to do with a process xxx.'
+ }
+ ]
+ """
+ data = []
+ for sentence in example['text'].strip().split('###'):
+ sentence = sentence.strip()
+ if sentence[:6] == 'Human:':
+ data.append(sentence[6:].strip())
+ elif sentence[:10] == 'Assistant:':
+ data.append(sentence[10:].strip())
+ if len(data) % 2:
+ # The last round of conversation solely consists of input
+ # without any output.
+ # Discard the input part of the last round, as this part is ignored in
+ # the loss calculation.
+ data.pop()
+ conversation = []
+ for i in range(0, len(data), 2):
+ single_turn_conversation = {'input': data[i], 'output': data[i + 1]}
+ conversation.append(single_turn_conversation)
+ return {'conversation': conversation}
+
+通过代码可以看到,\ ``oasst1_map_fn`` 对原数据中的 ``text``
+字段进行处理,进而构造了一个 ``conversation``
+字段,以此确保了后续数据处理流程的统一。
+
+值得注意的是,如果部分开源数据集依赖特殊的
+map_fn,则需要用户自行参照以提供的 map_fn
+进行自定义开发,实现字段格式的对齐。
+
+训练
+=====
+
+用户可以使用 ``xtuner train`` 启动训练。假设所使用的配置文件路径为
+``./config.py``\ ,并使用 DeepSpeed ZeRO-2 优化。
+
+单机单卡
+--------
+
+.. code:: console
+
+ $ xtuner train ./config.py --deepspeed deepspeed_zero2
+
+单机多卡
+--------
+
+.. code:: console
+
+ $ NPROC_PER_NODE=${GPU_NUM} xtuner train ./config.py --deepspeed deepspeed_zero2
+
+多机多卡(以 2 \* 8 GPUs 为例)
+--------------------------------------
+
+**方法 1:torchrun**
+
+.. code:: console
+
+ $ # excuete on node 0
+ $ NPROC_PER_NODE=8 NNODES=2 PORT=$PORT ADDR=$NODE_0_ADDR NODE_RANK=0 xtuner train mixtral_8x7b_instruct_full_oasst1_e3 --deepspeed deepspeed_zero2
+
+ $ # excuete on node 1
+ $ NPROC_PER_NODE=8 NNODES=2 PORT=$PORT ADDR=$NODE_0_ADDR NODE_RANK=1 xtuner train mixtral_8x7b_instruct_full_oasst1_e3 --deepspeed deepspeed_zero2
+
+.. note::
+
+ \ ``$PORT`` 表示通信端口、\ ``$NODE_0_ADDR`` 表示 node 0 的 IP 地址。
+ 二者并不是系统自带的环境变量,需要根据实际情况,替换为实际使用的值
+
+**方法 2:slurm**
+
+.. code:: console
+
+ $ srun -p $PARTITION --nodes=2 --gres=gpu:8 --ntasks-per-node=8 xtuner train internlm2_chat_7b_qlora_oasst1_e3 --launcher slurm --deepspeed deepspeed_zero2
+
+模型转换
+=========
+
+模型训练后会自动保存成 PTH 模型(例如 ``iter_500.pth``\ ),我们需要利用
+``xtuner convert pth_to_hf`` 将其转换为 HuggingFace
+模型,以便于后续使用。具体命令为:
+
+.. code:: console
+
+ $ xtuner convert pth_to_hf ${CONFIG_NAME_OR_PATH} ${PTH} ${SAVE_PATH}
+ $ # 例如:xtuner convert pth_to_hf ./config.py ./iter_500.pth ./iter_500_hf
+
+.. _模型合并可选):
+
+模型合并(可选)
+================
+
+如果您使用了 LoRA / QLoRA 微调,则模型转换后将得到 adapter
+参数,而并不包含原 LLM
+参数。如果您期望获得合并后的模型权重,那么可以利用
+``xtuner convert merge`` :
+
+.. code:: console
+
+ $ xtuner convert merge ${LLM} ${ADAPTER_PATH} ${SAVE_PATH}
+ $ # 例如:xtuner convert merge internlm/internlm2-chat-7b ./iter_500_hf ./iter_500_merged_llm
+
+对话
+=====
+
+用户可以利用 ``xtuner chat`` 实现与微调后的模型对话:
+
+.. code:: console
+
+ $ xtuner chat ${NAME_OR_PATH_TO_LLM} --adapter ${NAME_OR_PATH_TO_ADAPTER} --prompt-template ${PROMPT_TEMPLATE} [optional arguments]
+
+.. tip::
+
+ 例如:
+
+ .. code:: console
+
+ $ xtuner chat internlm2/internlm2-chat-7b --adapter ./iter_500_hf --prompt-template internlm2_chat
+ $ xtuner chat ./iter_500_merged_llm --prompt-template internlm2_chat
diff --git a/docs/zh_cn/training/visualization.rst b/docs/zh_cn/training/visualization.rst
new file mode 100644
index 000000000..64c1f8afe
--- /dev/null
+++ b/docs/zh_cn/training/visualization.rst
@@ -0,0 +1,73 @@
+==============
+可视化训练过程
+==============
+
+XTuner 支持通过 `MMEngine `__
+使用 `TensorBoard `__
+和 `Weights & Biases (WandB) `__
+实验管理工具,只需在 config 中添加一行代码,就可以跟踪和可视化损失、显存占用等指标。
+
+TensorBoard
+============
+
+1. 设置 config 中的 ``visualizer`` 字段,并将 ``vis_backends`` 设置为 `TensorboardVisBackend `__\ :
+
+.. code:: diff
+
+ # set visualizer
+ - visualizer = None
+ + from mmengine.visualization import Visualizer, TensorboardVisBackend
+ + visualizer = dict(type=Visualizer, vis_backends=[dict(type=TensorboardVisBackend)])
+
+2. 启动实验后,tensorboard 产生的相关文件会存在 ``vis_data`` 中,通过 tensorboard 命令可以启动进行实时可视化:
+
+|image1|
+
+.. code::
+
+ tensorboard --logdir=$PATH_TO_VIS_DATA
+
+WandB
+======
+
+1. 使用 WandB 前需安装依赖库 ``wandb`` 并登录至 wandb。
+
+.. code:: console
+
+ $ pip install wandb
+ $ wandb login
+
+2. 设置 config 中的 ``visualizer`` 字段,并将 ``vis_backends`` 设置为 `WandbVisBackend `__\ :
+
+.. code:: diff
+
+ # set visualizer
+ + from mmengine.visualization import Visualizer, WandbVisBackend
+ - visualizer = None
+ + visualizer = dict(type=Visualizer, vis_backends=[dict(type=WandbVisBackend)])
+
+.. tip::
+ 可以点击 `WandbVisBackend
+ API `__
+ 查看 ``WandbVisBackend`` 可配置的参数。例如
+ ``init_kwargs``\ ,该参数会传给
+ `wandb.init `__ 方法。
+
+ .. code:: diff
+
+ # set visualizer
+ - visualizer = None
+ + from mmengine.visualization import Visualizer, WandbVisBackend
+ + visualizer = dict(
+ + type=Visualizer,
+ + vis_backends=[
+ + dict(type=WandbVisBackend, init_kwargs=dict(project='toy-example'))])
+
+
+3. 启动实验后,可在 wandb 网页端 ``https://wandb.ai`` 上查看可视化结果:
+
+|image2|
+
+
+.. |image1| image:: https://github.com/InternLM/xtuner/assets/67539920/abacb28f-5afd-46d0-91b2-acdd20887969
+.. |image2| image:: https://github.com/InternLM/xtuner/assets/41630003/fc16387a-3c83-4015-9235-8ec811077953
diff --git a/docs/zh_cn/user_guides/custom_dataset/Online.md b/docs/zh_cn/user_guides/custom_dataset/Online.md
index fcf9edae1..aef9835c6 100644
--- a/docs/zh_cn/user_guides/custom_dataset/Online.md
+++ b/docs/zh_cn/user_guides/custom_dataset/Online.md
@@ -89,7 +89,7 @@ srun ${SRUN_ARGS} xtuner train internlm2_7b_full_finetune_custom_dataset_e1_copy
srun ${SRUN_ARGS} xtuner train internlm2_7b_w_tokenized_dataset_copy.py --launcher slurm --deepspeed deepspeed_zero3
```
-若训练数据集较大,可能需要在训练前设置环境变量 `XTUNER_DATASET_TIMEOUT` 为一个更大的数(默认为 30 分钟超时,可以酌情将其调大,如:120):
+若训练数据集较大,可能需要在训练前设置环境变量 `XTUNER_DATASET_TIMEOUT` 为一个更大的数(默认为 60 分钟超时,可以酌情将其调大,如:120):
```
XTUNER_DATASET_TIMEOUT=120 srun ${SRUN_ARGS} xtuner train internlm2_7b_full_finetune_custom_dataset_e1_copy.py --launcher slurm --deepspeed deepspeed_zero1
diff --git a/docs/zh_cn/user_guides/ftdp_dataset/Case2.md b/docs/zh_cn/user_guides/ftdp_dataset/Case2.md
index 585e1a02d..5096e896a 100644
--- a/docs/zh_cn/user_guides/ftdp_dataset/Case2.md
+++ b/docs/zh_cn/user_guides/ftdp_dataset/Case2.md
@@ -47,7 +47,9 @@ xtuner copy-cfg mistral_7b_w_tokenized_dataset .
## Step 3, 修改模板 config 文件
-修改模板 config 文件中的训练数据路径为真实数据路径,其中 `/path/to/tokenized/data` 与 Step 1 中的 `/path/to/tokenized/data` 为同一个路径。同时,需要修改 tokenizer 路径为 Step 1 保存的路径 `/path/to/save/new/tokenizer`。
+1. 修改模板 config 文件中的训练数据路径为真实数据路径,其中 `/path/to/tokenized/data` 需要基于 Step 1 中的 `/path/to/tokenized/data` 进一步指定 train folder,即 `/path/to/tokenized/data/chatml_llamav13_32k/train/` 。
+2. 需要修改 tokenizer 路径为 Step 1 保存的路径 `/path/to/save/new/tokenizer`。
+3. 由于 Step 1 扩充了 tokenizer 的词表,因此需要将新 tokenizer 传入 `SupervisedFinetune` 中,以扩展 llm model 的词表大小。
```diff
...
@@ -72,6 +74,13 @@ prompt_template = PROMPT_TEMPLATE.internlm2_chat
max_length = 32768
pack_to_max_length = True
...
+
+#######################################################################
+# PART 2 Model & Tokenizer #
+#######################################################################
+model = dict(
++ tokenizer=tokenizer,
+ ...)
```
在使用 DeepSpeed 训练模型时,如需在保存 checkpoint 时只保存模型权重,而不保存优化器状态,可参考以下步骤:
diff --git a/docs/zh_cn/user_guides/sequence_parallel.md b/docs/zh_cn/user_guides/sequence_parallel.md
index ba29d2830..ce4beed64 100644
--- a/docs/zh_cn/user_guides/sequence_parallel.md
+++ b/docs/zh_cn/user_guides/sequence_parallel.md
@@ -92,7 +92,8 @@ model = dict(
为了提升算法的可迁移性,XTuner 中抽象出了序列并行所必须的五个 API 接口:
- 序列并行分布式环境初始化 (init_sequence_parallel)
- 适配序列并行的 Data Sampler (SequenceParallelSampler)
-- 数据 Pad 与切分 (pad_for_sequence_parallel, split_for_sequence_parallel)
+- 数据 Pad (pad_for_sequence_parallel)
+- 数据切分 (split_for_sequence_parallel)
- 适配序列并行的 Attention (dispatch_modules)
- reduce loss 以正确打印训练损失 (reduce_sequence_parallel_loss)
@@ -127,7 +128,7 @@ dataloader = DataLoader(
**other_dataloader_params)
```
-### 数据 Pad 与切分
+### 数据 Pad
由于每条训练数据的长度可能不尽相同,我们需要将数据进行 Pad 以使得序列长度可以被 $sequence\\_parallel\\_world\\_size$ 整除,这样一条长数据才能被均等地分发给不同的 GPU 上。
@@ -135,27 +136,29 @@ dataloader = DataLoader(
```python
from xtuner.parallel.sequence import pad_for_sequence_parallel
-input_ids, labels, position_ids, attention_mask = pad_for_sequence_parallel(
- input_ids, labels, position_ids, attention_mask)
+
+input_ids = pad_for_sequence_parallel(input_ids, padding_value=0)
+labels = pad_for_sequence_parallel(labels, padding_value=-100)
+position_ids = pad_for_sequence_parallel(position_ids, padding_value=0)
+attention_mask = pad_for_sequence_parallel(attention_mask, padding_value=0)
```
-如果训练过程用不到 attention_mask,那么可以:
+以上过程在 `xtuner/dataset/collate_fns/default_collate_fn.py` 中实现。
-```python
-input_ids, labels, position_ids, _ = pad_for_sequence_parallel(
- input_ids, labels, position_ids)
-```
+### 数据切分
-Pad 后,我们需要对长序列均等切分:
+在传入给 Transformer 模型前,我们需要对长序列均等切分:
```python
from xtuner.parallel.sequence import split_for_sequence_parallel
# attention mask should not be split
-input_ids, labels, position_ids = split_for_sequence_parallel(
- input_ids, labels, position_ids)
+# `dim` is 1 as the shape of tensor is (bs, seq_len, ...)
+input_ids = split_for_sequence_parallel(input_ids, dim=1)
+labels = split_for_sequence_parallel(labels, dim=1)
+position_ids = split_for_sequence_parallel(position_ids, dim=1)
```
-以上两步在 xtuner/dataset/collate_fns/defalut_collate_fn.py 中实现。
+以上过程在 `xtuner/model/sft.py` 中实现。
### Attention 适配序列并行
diff --git a/examples/demo_data/multi_turn_2/README.md b/examples/demo_data/multi_turn_2/README.md
index 0d4f74615..9c5edd332 100644
--- a/examples/demo_data/multi_turn_2/README.md
+++ b/examples/demo_data/multi_turn_2/README.md
@@ -302,3 +302,305 @@ log_processor = dict(by_epoch=False)
cd ./examples/demo_data/multi_turn_2
xtuner train config.py
```
+
+# Multi-turn Conversation Example 2
+
+## Data
+
+`./data.json`
+
+```json
+[{
+ "messages":[
+ {
+ "role": "system",
+ "content": "You are a helpful AI assistant."
+ },
+ {
+ "role": "user",
+ "content": "Give three tips for staying healthy."
+ },
+ {
+ "role": "assistant",
+ "content": "1.Eat a balanced diet. 2. Exercise regularly. 3. Get enough sleep."
+ },
+ {
+ "role": "user",
+ "content": "How to study English?"
+ },
+ {
+ "role": "assistant",
+ "content": "1. Set clear goals. 2. Create a study plan. 3. Build vocabulary. 4. Practice speaking."
+ }
+ ]
+},
+{
+ "messages":[
+ {
+ "role": "system",
+ "content": "You are a helpful AI assistant."
+ },
+ {
+ "role": "user",
+ "content": "How to study English?"
+ },
+ {
+ "role": "assistant",
+ "content": "1. Set clear goals. 2. Create a study plan. 3. Build vocabulary. 4. Practice speaking."
+ },
+ {
+ "role": "user",
+ "content": "Give three tips for staying healthy."
+ },
+ {
+ "role": "assistant",
+ "content": "1.Eat a balanced diet. 2. Exercise regularly. 3. Get enough sleep."
+ }
+ ]
+}]
+```
+
+## Map Function
+
+`./map_fn.py`
+
+```python
+def multi_turn_2_map_fn(example):
+ messages = example['messages']
+ system = ''
+ input = ''
+ conversation = []
+ while messages and messages[0]['role'] == 'assistant':
+ # Skip the first one if it is from assistant
+ messages = messages[1:]
+ for msg in messages:
+ if msg['role'] == 'system':
+ system = msg['content']
+ elif msg['role'] == 'user':
+ input += msg['content']
+ elif msg['role'] == 'assistant':
+ conversation.append({
+ 'system': system,
+ 'input': input,
+ 'output': msg['content']
+ })
+ system = ''
+ input = ''
+ else:
+ raise NotImplementedError
+ return {'conversation': conversation}
+```
+
+## Config
+
+Based on [internlm_7b_qlora_json_e3](../../../xtuner/configs/internlm/internlm_7b/internlm_7b_qlora_json_e3.py).
+
+```diff
+# Copyright (c) OpenMMLab. All rights reserved.
+import torch
+from datasets import load_dataset
++ from mmengine.config import read_base
+from mmengine.dataset import DefaultSampler
+from mmengine.hooks import (CheckpointHook, DistSamplerSeedHook, IterTimerHook,
+ LoggerHook, ParamSchedulerHook)
+from mmengine.optim import AmpOptimWrapper, CosineAnnealingLR
+from peft import LoraConfig
+from torch.optim import AdamW
+from transformers import (AutoModelForCausalLM, AutoTokenizer,
+ BitsAndBytesConfig)
+
+from xtuner.dataset import process_hf_dataset
+from xtuner.dataset.collate_fns import default_collate_fn
+from xtuner.dataset.map_fns import template_map_fn_factory
+from xtuner.engine.hooks import DatasetInfoHook, EvaluateChatHook
+from xtuner.engine.runner import TrainLoop
+from xtuner.model import SupervisedFinetune
+from xtuner.utils import PROMPT_TEMPLATE
+
++with read_base():
++ from .map_fn import multi_turn_2_map_fn as dataset_map_fn
++
+#######################################################################
+# PART 1 Settings #
+#######################################################################
+# Model
+pretrained_model_name_or_path = 'internlm/internlm-7b'
+
+# Data
+-data_path = 'path/to/your/json_data'
++data_path = './data.json'
+prompt_template = PROMPT_TEMPLATE.default
+max_length = 2048
+pack_to_max_length = True
+
+# Scheduler & Optimizer
+batch_size = 1 # per_device
+accumulative_counts = 16
+dataloader_num_workers = 0
+max_epochs = 3
+optim_type = AdamW
+lr = 2e-4
+betas = (0.9, 0.999)
+weight_decay = 0
+max_norm = 1 # grad clip
+
+# Save
+save_steps = 500
+save_total_limit = 2 # Maximum checkpoints to keep (-1 means unlimited)
+
+# Evaluate the generation performance during the training
+evaluation_freq = 500
+SYSTEM = ''
+evaluation_inputs = [
+ '请给我介绍五个上海的景点', 'Please tell me five scenic spots in Shanghai'
+]
+
+#######################################################################
+# PART 2 Model & Tokenizer #
+#######################################################################
+tokenizer = dict(
+ type=AutoTokenizer.from_pretrained,
+ pretrained_model_name_or_path=pretrained_model_name_or_path,
+ trust_remote_code=True,
+ padding_side='right')
+
+model = dict(
+ type=SupervisedFinetune,
+ llm=dict(
+ type=AutoModelForCausalLM.from_pretrained,
+ pretrained_model_name_or_path=pretrained_model_name_or_path,
+ trust_remote_code=True,
+ torch_dtype=torch.float16,
+ quantization_config=dict(
+ type=BitsAndBytesConfig,
+ load_in_4bit=True,
+ load_in_8bit=False,
+ llm_int8_threshold=6.0,
+ llm_int8_has_fp16_weight=False,
+ bnb_4bit_compute_dtype=torch.float16,
+ bnb_4bit_use_double_quant=True,
+ bnb_4bit_quant_type='nf4')),
+ lora=dict(
+ type=LoraConfig,
+ r=64,
+ lora_alpha=16,
+ lora_dropout=0.1,
+ bias='none',
+ task_type='CAUSAL_LM'))
+
+#######################################################################
+# PART 3 Dataset & Dataloader #
+#######################################################################
+train_dataset = dict(
+ type=process_hf_dataset,
+ dataset=dict(
+ type=load_dataset, path='json', data_files=dict(train=data_path)),
+ tokenizer=tokenizer,
+ max_length=max_length,
++ dataset_map_fn=dataset_map_fn,
+ template_map_fn=dict(
+ type=template_map_fn_factory, template=prompt_template),
+ remove_unused_columns=True,
+ shuffle_before_pack=True,
+ pack_to_max_length=pack_to_max_length)
+
+train_dataloader = dict(
+ batch_size=batch_size,
+ num_workers=dataloader_num_workers,
+ dataset=train_dataset,
+ sampler=dict(type=DefaultSampler, shuffle=True),
+ collate_fn=dict(type=default_collate_fn))
+
+#######################################################################
+# PART 4 Scheduler & Optimizer #
+#######################################################################
+# optimizer
+optim_wrapper = dict(
+ type=AmpOptimWrapper,
+ optimizer=dict(
+ type=optim_type, lr=lr, betas=betas, weight_decay=weight_decay),
+ clip_grad=dict(max_norm=max_norm, error_if_nonfinite=False),
+ accumulative_counts=accumulative_counts,
+ loss_scale='dynamic',
+ dtype='float16')
+
+# learning policy
+# More information: https://github.com/open-mmlab/mmengine/blob/main/docs/en/tutorials/param_scheduler.md # noqa: E501
+param_scheduler = dict(
+ type=CosineAnnealingLR,
+ eta_min=0.0,
+ by_epoch=True,
+ end=max_epochs,
+ convert_to_iter_based=True)
+
+# train, val, test setting
+train_cfg = dict(type=TrainLoop, max_epochs=max_epochs)
+
+#######################################################################
+# PART 5 Runtime #
+#######################################################################
+# Log the dialogue periodically during the training process, optional
+custom_hooks = [
+ dict(type=DatasetInfoHook, tokenizer=tokenizer),
+ dict(
+ type=EvaluateChatHook,
+ tokenizer=tokenizer,
+ every_n_iters=evaluation_freq,
+ evaluation_inputs=evaluation_inputs,
+ system=SYSTEM,
+ prompt_template=prompt_template)
+]
+
+# configure default hooks
+default_hooks = dict(
+ # record the time of every iteration.
+ timer=dict(type=IterTimerHook),
+ # print log every 10 iterations.
+ logger=dict(type=LoggerHook, log_metric_by_epoch=False, interval=10),
+ # enable the parameter scheduler.
+ param_scheduler=dict(type=ParamSchedulerHook),
+ # save checkpoint per `save_steps`.
+ checkpoint=dict(
+ type=CheckpointHook,
+ by_epoch=False,
+ interval=save_steps,
+ max_keep_ckpts=save_total_limit),
+ # set sampler seed in distributed evrionment.
+ sampler_seed=dict(type=DistSamplerSeedHook),
+)
+
+# configure environment
+env_cfg = dict(
+ # whether to enable cudnn benchmark
+ cudnn_benchmark=False,
+ # set multi process parameters
+ mp_cfg=dict(mp_start_method='fork', opencv_num_threads=0),
+ # set distributed parameters
+ dist_cfg=dict(backend='nccl'),
+)
+
+# set visualizer
+visualizer = None
+
+# set log level
+log_level = 'INFO'
+
+# load from which checkpoint
+load_from = None
+
+# whether to resume training from the loaded checkpoint
+resume = False
+
+# Defaults to use random seed and disable `deterministic`
+randomness = dict(seed=None, deterministic=False)
+
+# set log processor
+log_processor = dict(by_epoch=False)
+```
+
+## Quick Start
+
+```bash
+cd ./examples/demo_data/multi_turn_2
+xtuner train config.py
+```
diff --git a/examples/demo_data/single_turn/README.md b/examples/demo_data/single_turn/README.md
index 188be9362..7826ea3c2 100644
--- a/examples/demo_data/single_turn/README.md
+++ b/examples/demo_data/single_turn/README.md
@@ -248,3 +248,251 @@ log_processor = dict(by_epoch=False)
cd ./examples/demo_data/single_turn
xtuner train config.py
```
+
+# Single-turn Conversation Example
+
+## Data
+
+`./data.json`
+
+```json
+[{
+ "toy_system": "You are a helpful AI assistant.",
+ "toy_input": "Give three tips for staying healthy.",
+ "toy_output": "1.Eat a balanced diet. 2. Exercise regularly. 3. Get enough sleep."
+},
+{
+ "toy_system": "You are a helpful AI assistant.",
+ "toy_input": "How to study English?",
+ "toy_output": "1. Set clear goals. 2. Create a study plan. 3. Build vocabulary. 4. Practice speaking."
+}]
+```
+
+## Map Function
+
+`./map_fn.py`
+
+```python
+def single_turn_map_fn(example):
+ return {
+ 'conversation': [{
+ 'system': example['toy_system'],
+ 'input': example['toy_input'],
+ 'output': example['output']
+ }]
+ }
+```
+
+## Config
+
+Based on [internlm_7b_qlora_json_e3](../../../xtuner/configs/internlm/internlm_7b/internlm_7b_qlora_json_e3.py).
+
+```diff
+# Copyright (c) OpenMMLab. All rights reserved.
+import torch
+from datasets import load_dataset
++ from mmengine.config import read_base
+from mmengine.dataset import DefaultSampler
+from mmengine.hooks import (CheckpointHook, DistSamplerSeedHook, IterTimerHook,
+ LoggerHook, ParamSchedulerHook)
+from mmengine.optim import AmpOptimWrapper, CosineAnnealingLR
+from peft import LoraConfig
+from torch.optim import AdamW
+from transformers import (AutoModelForCausalLM, AutoTokenizer,
+ BitsAndBytesConfig)
+
+from xtuner.dataset import process_hf_dataset
+from xtuner.dataset.collate_fns import default_collate_fn
+from xtuner.dataset.map_fns import template_map_fn_factory
+from xtuner.engine.hooks import DatasetInfoHook, EvaluateChatHook
+from xtuner.engine.runner import TrainLoop
+from xtuner.model import SupervisedFinetune
+from xtuner.utils import PROMPT_TEMPLATE
+
++with read_base():
++ from .map_fn import single_turn_map_fn as dataset_map_fn
++
+#######################################################################
+# PART 1 Settings #
+#######################################################################
+# Model
+pretrained_model_name_or_path = 'internlm/internlm-7b'
+
+# Data
+-data_path = 'path/to/your/json_data'
++data_path = './data.json'
+prompt_template = PROMPT_TEMPLATE.default
+max_length = 2048
+pack_to_max_length = True
+
+# Scheduler & Optimizer
+batch_size = 1 # per_device
+accumulative_counts = 16
+dataloader_num_workers = 0
+max_epochs = 3
+optim_type = AdamW
+lr = 2e-4
+betas = (0.9, 0.999)
+weight_decay = 0
+max_norm = 1 # grad clip
+
+# Save
+save_steps = 500
+save_total_limit = 2 # Maximum checkpoints to keep (-1 means unlimited)
+
+# Evaluate the generation performance during the training
+evaluation_freq = 500
+SYSTEM = ''
+evaluation_inputs = [
+ '请给我介绍五个上海的景点', 'Please tell me five scenic spots in Shanghai'
+]
+
+#######################################################################
+# PART 2 Model & Tokenizer #
+#######################################################################
+tokenizer = dict(
+ type=AutoTokenizer.from_pretrained,
+ pretrained_model_name_or_path=pretrained_model_name_or_path,
+ trust_remote_code=True,
+ padding_side='right')
+
+model = dict(
+ type=SupervisedFinetune,
+ llm=dict(
+ type=AutoModelForCausalLM.from_pretrained,
+ pretrained_model_name_or_path=pretrained_model_name_or_path,
+ trust_remote_code=True,
+ torch_dtype=torch.float16,
+ quantization_config=dict(
+ type=BitsAndBytesConfig,
+ load_in_4bit=True,
+ load_in_8bit=False,
+ llm_int8_threshold=6.0,
+ llm_int8_has_fp16_weight=False,
+ bnb_4bit_compute_dtype=torch.float16,
+ bnb_4bit_use_double_quant=True,
+ bnb_4bit_quant_type='nf4')),
+ lora=dict(
+ type=LoraConfig,
+ r=64,
+ lora_alpha=16,
+ lora_dropout=0.1,
+ bias='none',
+ task_type='CAUSAL_LM'))
+
+#######################################################################
+# PART 3 Dataset & Dataloader #
+#######################################################################
+train_dataset = dict(
+ type=process_hf_dataset,
+ dataset=dict(
+ type=load_dataset, path='json', data_files=dict(train=data_path)),
+ tokenizer=tokenizer,
+ max_length=max_length,
++ dataset_map_fn=dataset_map_fn,
+ template_map_fn=dict(
+ type=template_map_fn_factory, template=prompt_template),
+ remove_unused_columns=True,
+ shuffle_before_pack=True,
+ pack_to_max_length=pack_to_max_length)
+
+train_dataloader = dict(
+ batch_size=batch_size,
+ num_workers=dataloader_num_workers,
+ dataset=train_dataset,
+ sampler=dict(type=DefaultSampler, shuffle=True),
+ collate_fn=dict(type=default_collate_fn))
+
+#######################################################################
+# PART 4 Scheduler & Optimizer #
+#######################################################################
+# optimizer
+optim_wrapper = dict(
+ type=AmpOptimWrapper,
+ optimizer=dict(
+ type=optim_type, lr=lr, betas=betas, weight_decay=weight_decay),
+ clip_grad=dict(max_norm=max_norm, error_if_nonfinite=False),
+ accumulative_counts=accumulative_counts,
+ loss_scale='dynamic',
+ dtype='float16')
+
+# learning policy
+# More information: https://github.com/open-mmlab/mmengine/blob/main/docs/en/tutorials/param_scheduler.md # noqa: E501
+param_scheduler = dict(
+ type=CosineAnnealingLR,
+ eta_min=0.0,
+ by_epoch=True,
+ end=max_epochs,
+ convert_to_iter_based=True)
+
+# train, val, test setting
+train_cfg = dict(type=TrainLoop, max_epochs=max_epochs)
+
+#######################################################################
+# PART 5 Runtime #
+#######################################################################
+# Log the dialogue periodically during the training process, optional
+custom_hooks = [
+ dict(type=DatasetInfoHook, tokenizer=tokenizer),
+ dict(
+ type=EvaluateChatHook,
+ tokenizer=tokenizer,
+ every_n_iters=evaluation_freq,
+ evaluation_inputs=evaluation_inputs,
+ system=SYSTEM,
+ prompt_template=prompt_template)
+]
+
+# configure default hooks
+default_hooks = dict(
+ # record the time of every iteration.
+ timer=dict(type=IterTimerHook),
+ # print log every 10 iterations.
+ logger=dict(type=LoggerHook, log_metric_by_epoch=False, interval=10),
+ # enable the parameter scheduler.
+ param_scheduler=dict(type=ParamSchedulerHook),
+ # save checkpoint per `save_steps`.
+ checkpoint=dict(
+ type=CheckpointHook,
+ by_epoch=False,
+ interval=save_steps,
+ max_keep_ckpts=save_total_limit),
+ # set sampler seed in distributed evrionment.
+ sampler_seed=dict(type=DistSamplerSeedHook),
+)
+
+# configure environment
+env_cfg = dict(
+ # whether to enable cudnn benchmark
+ cudnn_benchmark=False,
+ # set multi process parameters
+ mp_cfg=dict(mp_start_method='fork', opencv_num_threads=0),
+ # set distributed parameters
+ dist_cfg=dict(backend='nccl'),
+)
+
+# set visualizer
+visualizer = None
+
+# set log level
+log_level = 'INFO'
+
+# load from which checkpoint
+load_from = None
+
+# whether to resume training from the loaded checkpoint
+resume = False
+
+# Defaults to use random seed and disable `deterministic`
+randomness = dict(seed=None, deterministic=False)
+
+# set log processor
+log_processor = dict(by_epoch=False)
+```
+
+## Quick Start
+
+```bash
+cd ./examples/demo_data/single_turn
+xtuner train config.py
+```
diff --git a/requirements/deepspeed.txt b/requirements/deepspeed.txt
index d7f9c3c0d..f6cda0a03 100644
--- a/requirements/deepspeed.txt
+++ b/requirements/deepspeed.txt
@@ -1,3 +1,2 @@
-# Minimum 0.12.3, see https://github.com/microsoft/DeepSpeed/pull/4587
-deepspeed>=0.12.3
+deepspeed==0.16.2
mpi4py-mpich
diff --git a/requirements/docs.txt b/requirements/docs.txt
new file mode 100644
index 000000000..95b3a0190
--- /dev/null
+++ b/requirements/docs.txt
@@ -0,0 +1,7 @@
+docutils
+myst-parser==2.0.0
+sphinx==6.2.1
+sphinx-argparse
+sphinx-book-theme==1.0.1
+sphinx-copybutton==0.5.2
+sphinx_markdown_tables
diff --git a/requirements/lmdeploy.txt b/requirements/lmdeploy.txt
new file mode 100644
index 000000000..25ef3916f
--- /dev/null
+++ b/requirements/lmdeploy.txt
@@ -0,0 +1 @@
+lmdeploy>=0.6.2 --no-deps
\ No newline at end of file
diff --git a/requirements/runtime.txt b/requirements/runtime.txt
index 05b38c1b7..71bdbe093 100644
--- a/requirements/runtime.txt
+++ b/requirements/runtime.txt
@@ -1,26 +1,15 @@
-# Minimum 0.40.0.post4 to fix some 4-bit precision bugs
-bitsandbytes>=0.40.0.post4
-# Minimum 2.16.0 to fix some bugs, see https://github.com/huggingface/datasets/pull/6444
-datasets>=2.16.0
+bitsandbytes==0.45.0
+datasets>=3.2.0
einops
-# Minimum 0.1.2 to fix some bugs, see https://github.com/InternLM/lagent/pull/44
-lagent>=0.1.2
-# Minimum 0.10.3 to support distributed evaluation for MMBench
-# see https://github.com/open-mmlab/mmengine/pull/1469
-mmengine>=0.10.3
+mmengine==0.10.6
openpyxl
-# Minimum 0.4.0 to support QLoRA, see https://github.com/huggingface/peft/pull/476
-peft>=0.4.0
+peft>=0.14.0
scikit-image
scipy
SentencePiece
tiktoken
-# limit pytorch version <= 2.1.2 as there may be some bugs in triton 2.2
-torch<=2.1.2
-torchvision<=0.16.2
-# Minimum 4.36.0 to support `Cache` data structure used by KV Cache
-# Registering a causal mask in `LlamaModel` is not friendly for very large
-# `max_position_embeddings`. Refer to
-# https://github.com/huggingface/transformers/blob/v4.38.0/src/transformers/models/llama/modeling_llama.py#L921-L923
-transformers>=4.36.0,!=4.38.0,!=4.38.1,!=4.38.2
+torch
+torchvision
+transformers==4.48.0
transformers_stream_generator
+loguru
diff --git a/setup.py b/setup.py
index 7a95dfab4..fe4d1b4f1 100644
--- a/setup.py
+++ b/setup.py
@@ -117,10 +117,12 @@ def gen_packages_items():
'Programming Language :: Python :: 3.8',
'Programming Language :: Python :: 3.9',
'Programming Language :: Python :: 3.10',
+ 'Programming Language :: Python :: 3.11',
+ 'Programming Language :: Python :: 3.12',
'Topic :: Utilities',
],
# Python maximum version <3.11, to support mpi4py-mpich
- python_requires='>=3.8, <3.11',
+ python_requires='>=3.8, <3.13',
license='Apache License 2.0',
install_requires=parse_requirements('requirements/runtime.txt'),
extras_require={
diff --git a/tools/fsdp_sft.py b/tools/fsdp_sft.py
new file mode 100644
index 000000000..3ab833d2a
--- /dev/null
+++ b/tools/fsdp_sft.py
@@ -0,0 +1,873 @@
+# Copyright (c) OpenMMLab. All rights reserved.
+import argparse
+import copy
+import math
+import os
+import sys
+import re
+import time
+import shutil
+import requests
+import gc
+from collections import OrderedDict
+from concurrent.futures import wait
+from datetime import datetime, timedelta
+
+import torch
+import torch.distributed as dist
+from torch.nn import functional as F
+import torch.distributed.checkpoint as dcp
+
+from mmengine import mkdir_or_exist
+from mmengine.runner import set_random_seed
+from mmengine.utils import get_git_hash
+from mmengine.utils.dl_utils import collect_env
+
+
+from torch.distributed.checkpoint.state_dict import (StateDictOptions,
+ get_state_dict, set_state_dict)
+from torch.distributed.checkpoint.stateful import Stateful
+
+from torch.optim import AdamW
+from torch.optim.lr_scheduler import CosineAnnealingLR, LambdaLR
+from torch.utils.data import ConcatDataset, DataLoader
+from transformers import AutoModelForCausalLM, AutoTokenizer
+
+from xtuner._lite import (get_device, get_logger,
+ get_torch_device_module)
+from xtuner._lite.accelerate import varlen_attn_is_available, profile_time_and_memory
+from xtuner._lite.algorithms.sft import SftCollator, SftTokenizeFunction
+from xtuner._lite.chat import CHAT_TEMPLATE_MAP
+from xtuner._lite.datasets import (DATASET_CLS_MAP, OPENAI_CONVERT_MAP,
+ SoftPackDataset, load_datasets)
+from xtuner._lite.parallel import (LengthGroupedSampler, ParallelSampler,
+ setup_parallel)
+from xtuner._lite.patches import FSDPConfig, AutoPatch
+from xtuner._lite.parallel import (ParallelSampler, setup_parallel)
+from xtuner._lite.modelings import register_remote_code
+
+gc.disable()
+logger = get_logger()
+
+DEVICE = get_device()
+DEVICE_MODULE = get_torch_device_module()
+
+SUPPORT_DATA_FORMATS = OPENAI_CONVERT_MAP.keys()
+
+def log_format(rank, debug=False):
+
+ formatter = f'[XTuner][RANK {rank}]'
+ formatter += '[{time:YYYY-MM-DD HH:mm:ss}][{level}]'
+
+ if debug:
+ formatter += '[{name}:'
+ formatter += '{function}:'
+ formatter += '{line}]'
+
+ formatter += ' {message}'
+ return formatter
+
+def send_to_feishu(web_hook, msg):
+
+ header = {
+ "Content-Type" : "application/json;charset=UTF-8"
+ }
+
+ body = {
+ "msg_type" : "text",
+ "content" : { "text" : f"所有人{msg}"}
+ }
+
+ try:
+ requests.post(url=web_hook, json=body, headers=header, timeout=1)
+ except requests.exceptions.RequestException:
+ pass
+
+
+def parse_args():
+ parser = argparse.ArgumentParser(description='Train LLM')
+
+ model_args = parser.add_argument_group('model', 'Model Related Settings')
+ model_args.add_argument('--llm', help='repo id or local path of the model')
+ model_args.add_argument(
+ '-t',
+ '--tokenizer',
+ help=('repo id or local path of the tokenizer. '
+ 'Defaults to the same as `model`'))
+ model_args.add_argument(
+ '--chat-template',
+ choices=CHAT_TEMPLATE_MAP.keys(),
+ help=('repo id or local path of the tokenizer. '
+ 'Defaults to the same as `model`'))
+ model_args.add_argument(
+ '--dtype',
+ default='auto',
+ choices=['fp16', 'bf16', 'auto'],
+ help=("the dtype of the model forward. When set to 'auto', it will "
+ 'automatically determine whether bf16 is available, '
+ 'prioritizing the use of bf16.'))
+ model_args.add_argument(
+ '--selective-recompute',
+ default=1.0,
+ type=float,
+ help=('the ratio of re-computation for transforemer layers. '
+ 'The maximum is 1; the larger the value, the less memory '
+ 'required for training. The default is 1, meaning all layers '
+ 'need to be re-computated.'))
+
+ model_args.add_argument('--cpu-offload', action='store_true', help=(''))
+ model_args.add_argument('--compile', action='store_true', help=(''))
+ model_args.add_argument('--sp-size', type=int, default=1, help='')
+ model_args.add_argument('--tp-size', type=int, default=1, help='')
+ data_args = parser.add_argument_group('data', 'Dataset Related Settings')
+ data_args.add_argument(
+ '--datasets',
+ nargs='*',
+ help=('repo id or local path or dir of the datasets. For repo ids, '
+ 'the `dset-sources` needs to be appropriately set to '
+ '`modelscope` or `huggingface`. For local dir, all json and '
+ 'jsonl files will be loaded by default. The type of loaded '
+ 'files can be controlled by setting `dset-file-type`'))
+ data_args.add_argument(
+ '--dset-file-types',
+ nargs='*',
+ default=DATASET_CLS_MAP.keys(),
+ choices=DATASET_CLS_MAP.keys(),
+ help='the file type that needs to be loaded')
+ data_args.add_argument(
+ '--dset-sources',
+ nargs='*',
+ default=['local'],
+ choices=['local', 'huggingface', 'modelscope'],
+ help=('the source of each dataset; it can accept one or the same '
+ 'number of args as the number of `datasets`, with one arg '
+ 'indicating that all datasets come from the same source. '
+ '`local` represents the local path, `huggingface` represents '
+ 'the open-source data in the Huggingface Hub, `modelscope` '
+ 'indicates the open-source data in the Modelscope Hub.'))
+ data_args.add_argument(
+ '--dset-formats',
+ nargs='*',
+ default=['openai'],
+ help=('the format of each dataset; it can accept one or the same '
+ 'number of args as the number of `datasets`, with one arg '
+ 'indicating that all datasets are the same format.'))
+ data_args.add_argument(
+ '--dset-sample-ratios',
+ nargs='*',
+ type=float,
+ default=[1.0],
+ help=('the sample ratio of each dataset; it can accept one or the '
+ 'same number of args as the number of `datasets`, with one arg '
+ 'indicating that all datasets use the same sample ratio.'))
+ data_args.add_argument(
+ '--dset-cache-dir',
+ help=('the cache dir of the loaded datasets. When the `datasets` is '
+ 'set, the loaded datasets will be cached to this dir. If the '
+ '`datasets` are not set, the cached dataset in this dir will be '
+ 'loaded.'))
+ data_args.add_argument(
+ '--dset-pack-level',
+ choices=['hard', 'soft'],
+ help=('the level of data packing. When `hard`, multiple data will be '
+ 'packed to `max_length`, potentially causing some data to be '
+ 'truncated, and the length of the packed data will always '
+ 'be `max_length`; When `soft`, it will pack multiple data '
+ 'into nearly `max_length` without truncating the data.'))
+ data_args.add_argument(
+ '--global-pack',
+ action='store_true',
+ help='A subsequence in the packed data comes from different files.')
+ data_args.add_argument(
+ '--max-length',
+ type=int,
+ default=2048,
+ help=('the maximum length of each piece of data, any excess will be '
+ 'truncated.'))
+ data_args.add_argument(
+ '--num-workers',
+ type=int,
+ default=8,
+ help='how many subprocesses to use for data loading.')
+ data_args.add_argument('--file-pattern', type=str, default=None)
+ data_args.add_argument('--group-by-length', action='store_true')
+
+ optim_args = parser.add_argument_group('optim', 'Optim Related Settings')
+ optim_args.add_argument(
+ '--mirco-batch-size',
+ type=int,
+ default=1,
+ help='batch size for each forward + backward pass')
+ optim_args.add_argument(
+ '--global-batch-size',
+ type=int,
+ default=16,
+ help='batch size for each optimizer step')
+
+ optim_args.add_argument(
+ '--lr', default=4e-5, type=float, help='learning rate.')
+ optim_args.add_argument(
+ '--lr-min', default=6e-6, type=float, help='min learning rate.')
+ optim_args.add_argument(
+ '--wd', default=0.01, type=float, help='weight decay.')
+ optim_args.add_argument(
+ '--max-grad-norm', default=1, type=float, help='gradient clipping')
+ optim_args.add_argument(
+ '-e', '--epochs', default=1, type=int, help='total training epochs.')
+ optim_args.add_argument(
+ '--warmup-ratio',
+ default=0.03,
+ type=float,
+ help=('the proportion of training steps for learning rate warm-up in '
+ 'relation to the total training steps.'))
+
+ parser.add_argument('-c', '--config', default=None)
+ parser.add_argument(
+ '--work-dir',
+ default='work_dirs',
+ help='the dir to save logs and checkpoints')
+ parser.add_argument(
+ '--feishu-webhook', default=None, help='Webhook of Feishu Group Chat Bot')
+ parser.add_argument('--gc-interval', default=100, type=int)
+ parser.add_argument(
+ '--checkpoint-interval',
+ default=-1,
+ type=float,
+ help=('how many steps to save a checkpoint; it can be a floating '
+ 'point number less than 1, or an integer greater than or equal '
+ "to 1. When it's a floating point, it will be multiplied by the "
+ 'total number of training steps.'))
+ parser.add_argument(
+ '--checkpoint-max-keep',
+ default=1,
+ type=int,
+ help=('Maximum number of saved checkpoints。'))
+ parser.add_argument(
+ '--checkpoint-drop-optimizer',
+ action='store_true',
+ help=('only model parameters are saved when saving a checkpoint. '
+ 'This can significantly reduce the size of checkpoint files, '
+ 'but the saved checkpoints cannot be resumed.'))
+ parser.add_argument(
+ '--log-interval', default=1, type=int, help='log interval')
+ parser.add_argument(
+ '--resume',
+ action='store_true',
+ help='specify checkpoint path to be resumed from.')
+ parser.add_argument(
+ '--seed', type=int, default=0, help='random seed for the training')
+ parser.add_argument(
+ '--debug', action='store_true', help='Set logger level to `DEBUG`')
+ args = parser.parse_args()
+ return args
+
+
+def is_interval(step, total_steps, interval):
+ return (step + 1) % interval == 0 or (step + 1) == total_steps
+
+
+
+class TrainState(Stateful):
+
+ def __init__(self, total_steps, seed):
+ super().__init__()
+
+ self.seed = seed
+ self.cur_step = -1
+ self.total_steps = total_steps
+ self.if_nan_skip_steps = 0
+
+ def load_state_dict(self, state_dict):
+ assert self.total_steps == state_dict['total_steps']
+ self.cur_step = state_dict['current_step']
+ self.if_nan_skip_steps = state_dict['if_nan_skip_steps']
+
+ def state_dict(self):
+ return {
+ 'seed': self.seed, 'current_step': self.cur_step,
+ 'total_steps': self.total_steps,
+ 'if_nan_skip_steps': self.if_nan_skip_steps
+ }
+
+ def step(self):
+ self.cur_step = self.cur_step + 1
+
+ def found_nan(self):
+ self.if_nan_skip_steps += 1
+
+
+def find_latest_timestamp(work_dir):
+ # Initialize variables to keep track of the latest timestamp and its corresponding directory
+ latest_timestamp = None
+
+ # Iterate over all files and directories in the specified directory
+ for entry in os.listdir(work_dir):
+ full_path = os.path.join(work_dir, entry)
+
+ # Check if the entry is a directory
+ if os.path.isdir(full_path):
+ try:
+ # Try to interpret the directory name as a timestamp
+ timestamp = datetime.strptime(entry, '%Y%m%d%H%M%S')
+
+ # Update the latest timestamp and directory if this one is more recent
+ if latest_timestamp is None or timestamp > latest_timestamp:
+ latest_timestamp = timestamp
+ except ValueError:
+ # If conversion fails, skip this entry
+ continue
+
+ if latest_timestamp is not None:
+ latest_timestamp = latest_timestamp.strftime( '%Y%m%d%H%M%S')
+
+ return latest_timestamp
+
+
+def find_checkpoints(directory, prefix='ckpt'):
+
+ if prefix == 'ckpt':
+ pattern = r'^ckpt-(\d+)$'
+ elif prefix == 'hf':
+ pattern = r'^hf-(\d+)$'
+ else:
+ raise ValueError
+
+ latest_step = -1
+ latest_checkpoint = None
+
+ all_folders = [d for d in os.listdir(directory) if os.path.isdir(os.path.join(directory, d))]
+
+ checkpoints = []
+ for folder in all_folders:
+ match = re.match(pattern, folder)
+ if match:
+ checkpoints.append((folder, int(match.group(1))))
+
+ checkpoints.sort(key=lambda x: x[1])
+
+ return [os.path.join(directory, folder[0]) for folder in checkpoints]
+
+
+
+
+# @logger.catch
+def sft(args):
+ ###########################################################################
+ # 1. Environment #
+ ###########################################################################
+ setup_parallel()
+ set_random_seed(args.seed)
+ register_remote_code()
+
+ world_size = dist.get_world_size()
+
+ cpu_comm_timeout = timedelta(minutes=60)
+ gloo_group = dist.new_group(backend='gloo', timeout=cpu_comm_timeout)
+
+ rank = dist.get_rank()
+
+ if args.resume:
+ mkdir_or_exist(args.work_dir)
+ timestamp = find_latest_timestamp(args.work_dir)
+
+ if timestamp is None:
+ timestamp = datetime.now().strftime('%Y%m%d%H%M%S')
+ else:
+ timestamp = datetime.now().strftime('%Y%m%d%H%M%S')
+
+ objects = [timestamp]
+ dist.broadcast_object_list(objects, src=0)
+ timestamp = objects[0]
+
+ args.work_dir = os.path.join(args.work_dir, timestamp)
+ mkdir_or_exist(args.work_dir)
+
+ log_file = os.path.join(args.work_dir, f'rank{rank}.log')
+
+ logger.remove()
+ # Change the log format printed in the terminal
+ lvl = 'DEBUG' if args.debug else 'INFO'
+ logger.add(sys.stderr, level=lvl, format=log_format(rank, args.debug))
+ # Change the format saved in the log file
+ logger.add(log_file, format=log_format(rank), backtrace=True, catch=True)
+
+ if args.feishu_webhook and rank == 0:
+ def log_handler(record):
+ if record['level'].name == "WARNING":
+ send_to_feishu(args.feishu_webhook, f"[WARNING] {record['message']}\n{args.work_dir}")
+ elif record['level'].name == "TRACE":
+ send_to_feishu(args.feishu_webhook, f"[TRACE] {record['message']}\n{args.work_dir}")
+ elif record['level'].name == "ERROR":
+ send_to_feishu(args.feishu_webhook, f"[ERROR] 任务失败\n{args.work_dir}")
+
+ logger.add(sys.stderr, level='TRACE', filter=log_handler, catch=True)
+
+ logger.trace('任务开始')
+
+ logger.info(args)
+ if rank == 0:
+ env = collect_env()
+ import transformers
+
+ import xtuner
+ env['Transformers'] = transformers.__version__
+ env['XTuner'] = f'{xtuner.__version__}+{get_git_hash(digits=6)}'
+ runtime_env = OrderedDict()
+ runtime_env.update(env)
+ runtime_env['Seed'] = args.seed
+ runtime_env['World Size'] = world_size
+
+ runtime_env_info = '\n ' + '\n '.join(
+ f'{k}: {v}' for k, v in runtime_env.items())
+ dash_line = '-' * 60
+ logger.info('\n' + dash_line + '\nRuntime environment:' +
+ runtime_env_info + '\n' + dash_line + '\n')
+ # ------------------- Environment End ------------------------------ #
+
+
+ ###########################################################################
+ # 2. FSDP #
+ ###########################################################################
+ if args.dtype == 'auto':
+ args.dtype = 'bf16' if DEVICE_MODULE.is_bf16_supported() else 'fp16'
+
+ if args.dtype == 'fp16':
+ dtype = torch.float16
+ elif args.dtype == 'bf16':
+ if DEVICE_MODULE.is_bf16_supported():
+ dtype = torch.bfloat16
+ else:
+ raise RuntimeError('The device does not support `bf16`, '
+ 'please set `dtype` to `fp16`.')
+ else:
+ raise RuntimeError('`dtype` only supports `fp16`, `bf16` or `auto`, '
+ f'but found {args.dtype}.')
+
+
+ with torch.device('meta'):
+ llm = AutoModelForCausalLM.from_pretrained(
+ args.llm, attn_implementation='flash_attention_2', torch_dtype=dtype)
+
+ for module in llm.modules():
+ for p_name, param in module.named_parameters(recurse=False):
+ if param.requires_grad:
+ param_fp32 = torch.nn.Parameter(
+ param.to(dtype=torch.float32))
+ setattr(module, p_name, param_fp32)
+
+
+ fsdp_config = FSDPConfig(
+ tp_size=args.tp_size,
+ sp_size=args.sp_size, reshard_after_forward=True,
+ cpu_offload=args.cpu_offload, reduce_dtype=dtype, param_dtype=dtype,
+ torch_compile=args.compile, max_length=args.max_length * args.mirco_batch_size
+ )
+
+ with profile_time_and_memory('[FSDP]'):
+ patched_llm = AutoPatch.from_causal_lm(llm, fsdp_config)
+
+ dp_mesh = patched_llm.data_parallel_mesh
+ data_mesh = patched_llm.data_mesh
+ dp_size = patched_llm.data_parallel_mesh.size()
+ if args.global_batch_size < dp_size or args.global_batch_size % dp_size:
+ raise ValueError(f'The `global_batch_size`({args.global_batch_size}) '
+ 'should be divisible by the '
+ f'world_size({world_size}).')
+
+ if (args.global_batch_size / dp_size) % args.mirco_batch_size:
+ raise ValueError(f'The `global_batch_size`({args.global_batch_size}) '
+ f'should be divisible by the world_size({world_size})'
+ f' * `mirco_batch_size`({args.mirco_batch_size})')
+
+ dist.barrier()
+ gc.collect()
+ # -------------------------- FSDP End ------------------------------ #
+
+ ###########################################################################
+ # 3. Dataset & Dataloader #
+ ###########################################################################
+
+ start_load_data_t = time.time()
+
+ tokenizer = AutoTokenizer.from_pretrained(
+ args.tokenizer if args.tokenizer else args.llm,
+ use_fast=False,
+ padding_side='right')
+
+ if args.chat_template:
+ chat_template = CHAT_TEMPLATE_MAP[args.chat_template]
+ else:
+ chat_template = patched_llm.chat_template
+
+ tokenize_fns = []
+ for dset_format in args.dset_formats:
+ # If your data format is not in `SUPPORT_DATA_FORMATS`, you should
+ # redefine a `tokenize_fn`, defining how to convert a piece of raw
+ # data into tokenized data.
+ # The tokenized data must include `input_ids`, `labels``,
+ # and `num_tokens`.
+ tokenize_fn = SftTokenizeFunction(tokenizer, chat_template,
+ dset_format)
+ tokenize_fns.append(tokenize_fn)
+
+ _datasets = load_datasets(
+ paths=args.datasets,
+ cache_dir=args.dset_cache_dir,
+ file_types=args.dset_file_types,
+ sources=args.dset_sources,
+ sample_ratios=args.dset_sample_ratios,
+ map_fns=tokenize_fns,
+ file_pattern=args.file_pattern,
+ max_length=args.max_length
+ )
+
+ if args.dset_pack_level and rank == 0 and args.debug:
+ # Only the tokenized datasets can count the number of tokens
+ num_tokens = sum(dset.num_tokens.sum() for dset in _datasets)
+ logger.debug(f'[Dataset] {num_tokens} tokens.')
+
+ if args.dset_pack_level == 'soft':
+ train_dataset = SoftPackDataset(_datasets, target=args.max_length, blend=args.global_pack)
+ elif args.dset_pack_level == 'hard':
+ raise NotImplementedError
+ else:
+ train_dataset = ConcatDataset(_datasets)
+
+ if args.dset_pack_level and rank == 0:
+ ori_samples = sum([len(dset) for dset in _datasets])
+ packed_samples = len(train_dataset)
+ logger.info(f'[Dataset] (Original) {ori_samples} samples.')
+ logger.info(f'[Dataset] (Packed) {packed_samples} samples.')
+
+ assert varlen_attn_is_available()
+ collator = SftCollator(
+ pack_batch=varlen_attn_is_available(),
+ max_length=args.max_length)
+
+ if args.group_by_length:
+ sampler = LengthGroupedSampler(train_dataset, patched_llm.data_parallel_mesh,
+ args.global_batch_size)
+ else:
+ sampler = ParallelSampler(
+ train_dataset,
+ patched_llm.data_parallel_mesh,
+ args.global_batch_size, shuffle=True)
+
+ gc.collect()
+
+ train_dataloader = DataLoader(
+ train_dataset,
+ batch_size=args.mirco_batch_size,
+ num_workers=args.num_workers,
+ # Ensure to round up or drop last based on the `global_batch_size`,
+ # if you want to replace a custom sampler.
+ sampler=sampler,
+ collate_fn=collator,
+ persistent_workers=args.num_workers > 0)
+
+ if rank == 0:
+ logger.info(f'[Dataloader] {len(train_dataloader)} batches.')
+ _first_batch = [train_dataset[i] for i in range(args.mirco_batch_size)]
+ _first_batch = collator(_first_batch)
+ _decoded = tokenizer.batch_decode(_first_batch['input_ids'])
+ logger.debug(f'[Dataloader] Training Batch:\n{_first_batch}')
+ logger.debug(f'[Dataloader] Training Batch(Decoded):\n{_decoded}')
+ dist.barrier()
+
+ gc.collect()
+ load_data_cost_time = time.time() - start_load_data_t
+ logger.info(f'[Dataset & Dataloader] Cost {load_data_cost_time:.2f}s')
+ # ------------------- Dataset & Dataloader End --------------------- #
+
+
+
+ ###########################################################################
+ # 4. Optimizer & Scheduler #
+ ###########################################################################
+ optimizer = AdamW(
+ patched_llm.trainable_parameters(),
+ lr=args.lr,
+ weight_decay=args.wd,
+ betas=(0.9, 0.95))
+
+ global_batch_size = args.global_batch_size
+ mirco_batch_size = args.mirco_batch_size
+
+ # `iter` means once forward+backward
+ # `step` means once optimizer step
+ # `iters_per_step` means gradient accumulative counts
+ iters_per_step = global_batch_size // mirco_batch_size // dp_size
+ iters_per_epoch = len(train_dataloader)
+ steps_per_epoch = math.ceil(iters_per_epoch / iters_per_step)
+
+ total_epochs = args.epochs
+ total_steps = steps_per_epoch * total_epochs
+ if_nan_skip_steps = 0
+ train_state = TrainState(total_steps, args.seed)
+
+ if args.checkpoint_interval == -1:
+ checkpoint_interval = total_steps
+ elif args.checkpoint_interval < 1:
+ checkpoint_interval = int(total_steps * args.checkpoint_interval)
+ else:
+ checkpoint_interval = int(args.checkpoint_interval)
+
+ warmup_steps = int(args.warmup_ratio * total_steps)
+
+ def warmup_fn(x):
+ return x / warmup_steps if x < warmup_steps else 1
+
+ warmup_scheduler = LambdaLR(optimizer, warmup_fn)
+
+ cosine_scheduler = CosineAnnealingLR(
+ optimizer, T_max=total_steps - warmup_steps, eta_min=args.lr_min)
+
+ start_step = 0
+ gc.collect()
+ # ---------------- Optimizer & Scheduler End ----------------------- #
+
+ ###########################################################################
+ # 5. (Optional) Resume #
+ ###########################################################################
+ if args.resume:
+
+
+ _checkpoints = find_checkpoints(args.work_dir)
+
+ latest_checkpoint = None
+
+ for _ckpt_dir in reversed(_checkpoints):
+ if os.path.exists(os.path.join(_ckpt_dir, '.metadata')):
+ latest_checkpoint = _ckpt_dir
+ break
+
+ if latest_checkpoint:
+
+ with profile_time_and_memory('[Resume]'):
+ _options = StateDictOptions(
+ cpu_offload=True, ignore_frozen_params=True)
+ (shard_model_state_dict,
+ shard_optimizer_state_dict) = get_state_dict(
+ patched_llm.patched_model, optimizer, options=_options)
+ state_dict = {
+ 'model': shard_model_state_dict,
+ 'optimizer': shard_optimizer_state_dict,
+ 'train_state': train_state,
+ }
+
+ # inplace state_dict
+ dcp.load(
+ state_dict=state_dict,
+ checkpoint_id=latest_checkpoint,
+ )
+
+ _options = StateDictOptions(
+ cpu_offload=True, strict=False)
+ set_state_dict(
+ patched_llm.patched_model,
+ optimizer,
+ model_state_dict=state_dict["model"],
+ optim_state_dict=state_dict["optimizer"],
+ options=_options
+ )
+
+ start_step = train_state.cur_step + 1
+
+ else:
+ logger.warning(f'There is no checkpoint available for resuming training in {args.work_dir}.')
+
+ ###########################################################################
+ # 6. Training #
+ ###########################################################################
+ ckpt_handle = None
+ start_train_t = time.time()
+ DEVICE_MODULE.empty_cache()
+ DEVICE_MODULE.reset_peak_memory_stats()
+ max_memory = DEVICE_MODULE.max_memory_allocated()
+ logger.info('[Train] Begin Train Loop. The current GPU memory is '
+ f'{(max_memory / 1024**3):.1f}GB')
+
+ for step in range(start_step, total_steps):
+
+ if is_interval(step + 1, total_steps, args.gc_interval):
+ gc.collect()
+
+ epoch = step // steps_per_epoch
+ epoch_inner_step = step % steps_per_epoch
+ if epoch_inner_step == 0 or step == start_step:
+ # For the first step of each epoch, the data order needs to be
+ # readjusted.
+ # Or after resuming, for the first step, the dataloader needs to
+ # be adjusted to the position before resume.
+ train_dataloader.sampler.set_epoch(epoch, epoch_inner_step * iters_per_step )
+ data_iterator = iter(train_dataloader)
+
+ train_state.step()
+
+ if step <= warmup_steps:
+ warmup_scheduler.step(step)
+ cur_lr = warmup_scheduler.get_last_lr()[0]
+ else:
+ cosine_scheduler.step(step)
+ cur_lr = cosine_scheduler.get_last_lr()[0]
+
+ DEVICE_MODULE.reset_peak_memory_stats()
+
+ step_loss = 0
+ step_data_time = 0
+ step_start_t = time.time()
+ step_consumed_tokens = 0
+
+ _data_start_t = time.time()
+
+ step_data_list = [next(data_iterator) for _ in range(iters_per_step)]
+ rank_grad_tokens = 0
+ for _iter in range(iters_per_step):
+ _iter_data = step_data_list[_iter]
+ _iter_labels = _iter_data['labels'][:, 1:]
+ rank_grad_tokens += (_iter_labels >= 0).sum()
+ rank_grad_tokens = rank_grad_tokens.to(DEVICE)
+ dist.all_reduce(rank_grad_tokens, group=patched_llm.data_parallel_mesh.get_group())
+ global_grad_tokens = rank_grad_tokens
+
+ step_data_time = time.time() - _data_start_t
+
+ for _iter in range(iters_per_step):
+
+ data = step_data_list[_iter]
+
+ input_ids = data['input_ids'][:, :-1].to(DEVICE)
+ labels = data['labels'][:, 1:].to(DEVICE)
+ num_tokens = data['num_tokens'].tolist()
+
+ if num_tokens[-1] == 1:
+ num_tokens = num_tokens[:-1]
+ else:
+ num_tokens[-1] = num_tokens[-1] - 1
+
+ cu_seq_lens = torch.cumsum(torch.IntTensor([0] + num_tokens), dim=0).to(DEVICE).int()
+ position_ids = [torch.arange(num) for num in num_tokens]
+ position_ids = torch.cat(position_ids, dim=0).to(DEVICE).unsqueeze_(0)
+
+ patched_llm.train()
+ loss = patched_llm(
+ input_ids=input_ids,
+ position_ids=position_ids,
+ labels=labels,
+ label_shifted=True,
+ use_cache=False,
+ cu_seq_lens_q=cu_seq_lens,
+ cu_seq_lens_k=cu_seq_lens,
+ max_length_q=max(num_tokens),
+ max_length_k=max(num_tokens),
+ sequence_parallel_mesh=patched_llm.sequence_parallel_mesh,
+ ).loss
+
+ loss = loss * (labels >= 0).sum() / global_grad_tokens * dp_size
+ loss.backward()
+
+ step_loss += loss.item()
+ step_consumed_tokens += sum(num_tokens) / data_mesh.size()
+
+ step_reduced_loss = torch.Tensor([step_loss]).to(DEVICE)
+ dist.all_reduce(step_reduced_loss, group=dp_mesh.get_group())
+ step_reduced_loss = step_reduced_loss.item() / dp_size
+
+ grad_norm = patched_llm.clip_grad_norm(args.max_grad_norm)
+
+ if grad_norm.isnan() or grad_norm.isinf():
+ train_state.found_nan()
+ logger.warning(f"[Step {step}] The grad norm is NaN or Inf, skip this step. Skipped {train_state.if_nan_skip_steps} steps in total.")
+ optimizer.zero_grad()
+ else:
+ optimizer.step()
+ optimizer.zero_grad()
+
+ step_time = time.time() - step_start_t
+ eta = step_time * (total_steps - step)
+ eta = timedelta(seconds=int(eta))
+ tgs = int(step_consumed_tokens / step_time)
+ max_memory = DEVICE_MODULE.max_memory_allocated()
+ if is_interval(step, total_steps, args.log_interval):
+ logger.info(f'[Train] (Epoch {epoch + 1}) Step '
+ f'{step + 1}/{total_steps} '
+ f'lr: {cur_lr:.6f} loss: {step_loss:.3f} '
+ f'loss(reduced): {step_reduced_loss:.3f} '
+ f'grad_norm: {grad_norm:.2f} '
+ f'if_nan_skip: {train_state.if_nan_skip_steps} '
+ f'max_memory: {(max_memory / 1024**3):.1f}GB '
+ f'text_tokens: {step_consumed_tokens} '
+ f'tgs: {tgs} data_time: {step_data_time:.2f}s '
+ f'time: {step_time:.2f}s '
+ f'eta: {eta}')
+
+ if is_interval(step, total_steps, max(1, int(total_steps * 0.1))):
+ logger.trace(f'Step {step}/{total_steps}, loss {step_loss:.3f}, tgs {tgs}')
+
+ if is_interval(step, total_steps, checkpoint_interval):
+
+ num_digits = len(str(abs(total_steps)))
+ work_dir = args.work_dir
+ ckpt_dir = os.path.join(work_dir, f'ckpt-{step+1:0{num_digits}}')
+ hf_dir = os.path.join(work_dir, f'hf-{step+1:0{num_digits}}')
+
+ with profile_time_and_memory('[HF Checkpoint]'):
+ patched_llm.save_pretrained(hf_dir)
+
+ saved_hf_checkpoints = find_checkpoints(args.work_dir, prefix='hf')
+
+ if len(saved_hf_checkpoints) > args.checkpoint_max_keep:
+ for _ckpt in saved_hf_checkpoints[:-args.checkpoint_max_keep]:
+ if rank == 0:
+ shutil.rmtree(_ckpt)
+ logger.info('[HF Checkpoint] Delete the oldest checkpoint.')
+
+
+ if args.checkpoint_drop_optimizer:
+ logger.warning('The saved checkpoint cannot be resumed. '
+ 'If you want to save a resumable checkpoint, '
+ 'please remove `--checkpoint-drop-optimizer` '
+ 'from the command.')
+ else:
+ with profile_time_and_memory('[PT Checkpoint]'):
+ if ckpt_handle is not None:
+ wait([ckpt_handle])
+
+ # FSDP cannot be saved via torch.save
+ # Refer to https://pytorch.org/tutorials/recipes/distributed_checkpoint_recipe.html # noqa: E501
+ _options = StateDictOptions(
+ cpu_offload=True, ignore_frozen_params=True)
+ (shard_model_state_dict,
+ shard_optimizer_state_dict) = get_state_dict(
+ llm, optimizer, options=_options)
+
+ state_dict = {
+ 'model': shard_model_state_dict,
+ 'optimizer': shard_optimizer_state_dict,
+ 'train_state': train_state.state_dict(),
+ }
+
+ mkdir_or_exist(ckpt_dir)
+ ckpt_handle = dcp.async_save(state_dict, checkpoint_id=ckpt_dir, process_group=gloo_group)
+
+ saved_checkpoints = find_checkpoints(args.work_dir)
+
+ if len(saved_checkpoints) > args.checkpoint_max_keep:
+ for _ckpt in saved_checkpoints[:-args.checkpoint_max_keep]:
+ if rank == 0:
+ shutil.rmtree(_ckpt)
+ logger.info('[PT Checkpoint] Delete the oldest checkpoint.')
+
+ if ckpt_handle is not None:
+ wait([ckpt_handle])
+
+ logger.trace('Task Finished')
+
+ train_cost_time = time.time() - start_train_t
+ logger.info(f'[Train] Cost {timedelta(seconds=int(train_cost_time))}')
+ # ------------------------ Training End ---------------------------- #
+
+if __name__ == '__main__':
+
+ args = parse_args()
+ sft(args)
diff --git a/xtuner/_lite/__init__.py b/xtuner/_lite/__init__.py
new file mode 100644
index 000000000..f4bce11b8
--- /dev/null
+++ b/xtuner/_lite/__init__.py
@@ -0,0 +1,63 @@
+import sys
+from loguru import logger
+import os
+import subprocess
+
+from .device import get_device, get_torch_device_module
+
+_LOGGER = None
+
+def log_format(debug=False):
+
+ formatter = '[XTuner][{time:YYYY-MM-DD HH:mm:ss}][{level}]'
+
+ if debug:
+ formatter += '[{name}:'
+ formatter += '{function}:'
+ formatter += '{line}]'
+
+ formatter += ' {message}'
+ return formatter
+
+
+def get_logger(level="INFO"):
+ global _LOGGER
+ if _LOGGER is None:
+ # Remove the original logger in Python to prevent duplicate printing.
+ logger.remove()
+ logger.add(sys.stderr, level=level, format=log_format(debug=level=="DEBUG"))
+ _LOGGER = logger
+ return _LOGGER
+
+
+def get_repo_git_info(repo_path):
+ original_directory = os.getcwd()
+ os.chdir(repo_path)
+
+ try:
+ branch = subprocess.check_output(
+ ['git', 'rev-parse', '--abbrev-ref', 'HEAD'],
+ stderr=subprocess.STDOUT
+ ).strip().decode('utf-8')
+
+ commit_id = subprocess.check_output(
+ ['git', 'rev-parse', 'HEAD'],
+ stderr=subprocess.STDOUT
+ ).strip().decode('utf-8')
+
+ remote_url = subprocess.check_output(
+ ['git', 'remote', 'get-url', 'origin'],
+ stderr=subprocess.STDOUT
+ ).strip().decode('utf-8')
+
+ return branch, commit_id, remote_url
+ except subprocess.CalledProcessError as e:
+ return None, None, None
+ finally:
+ os.chdir(original_directory)
+
+
+__all__ = [
+ 'AutoConfig', 'AutoModelForCausalLM', 'AutoTokenizer', 'get_device',
+ 'get_torch_device_module'
+]
diff --git a/xtuner/_lite/accelerate/__init__.py b/xtuner/_lite/accelerate/__init__.py
new file mode 100644
index 000000000..9828041ce
--- /dev/null
+++ b/xtuner/_lite/accelerate/__init__.py
@@ -0,0 +1,11 @@
+from .lora import LORA_TARGET_MAP
+from .packed import pack_sequence, unpack_sequence
+from .utils import (liger_kernel_is_available, lmdeploy_is_available,
+ mlu_is_available, npu_is_available,
+ profile_time_and_memory, varlen_attn_is_available)
+
+__all__ = [
+ 'LORA_TARGET_MAP', 'pack_sequence', 'packed_sequence', 'unpack_sequence',
+ 'varlen_attn_is_available', 'lmdeploy_is_available', 'npu_is_available',
+ 'mlu_is_available', 'profile_time_and_memory'
+]
diff --git a/xtuner/_lite/accelerate/lora.py b/xtuner/_lite/accelerate/lora.py
new file mode 100644
index 000000000..ad3c9a3f5
--- /dev/null
+++ b/xtuner/_lite/accelerate/lora.py
@@ -0,0 +1,5 @@
+LORA_TARGET_MAP = {
+ 'InternLM2ForCausalLM': ['wqkv', 'wo', 'w1', 'w2', 'w3'],
+ 'CLIPVisionModel':
+ ['q_proj', 'k_proj', 'v_proj', 'out_proj', 'fc1', 'fc2']
+}
diff --git a/xtuner/_lite/accelerate/packed.py b/xtuner/_lite/accelerate/packed.py
new file mode 100644
index 000000000..ab5f67100
--- /dev/null
+++ b/xtuner/_lite/accelerate/packed.py
@@ -0,0 +1,27 @@
+from contextlib import contextmanager
+from typing import List, Union
+
+import torch
+
+def unpack_sequence(packed: torch.Tensor,
+ num_tokens: Union[torch.Tensor, List],
+ dim=1):
+
+ if isinstance(num_tokens, torch.Tensor):
+ num_tokens = num_tokens.tolist()
+ sequences = torch.split(packed, num_tokens, dim=dim)
+ return sequences
+
+
+def pack_sequence(sequences, dim=1):
+ num_tokens = torch.IntTensor([seq.size(dim) for seq in sequences])
+ packed = torch.cat(sequences, dim=dim)
+ return packed, num_tokens.to(packed.device)
+
+
+def packed_cumulative_length(num_tokens: torch.Tensor):
+
+ device = num_tokens.device
+ _zero_pad = torch.zeros(1, device=device)
+ _pad_length = torch.cat([_zero_pad, num_tokens]).int()
+ return torch.cumsum(_pad_length, 0).int()
diff --git a/xtuner/_lite/accelerate/utils.py b/xtuner/_lite/accelerate/utils.py
new file mode 100644
index 000000000..8ca4ba703
--- /dev/null
+++ b/xtuner/_lite/accelerate/utils.py
@@ -0,0 +1,60 @@
+import time
+from contextlib import contextmanager
+from transformers.utils.import_utils import is_flash_attn_2_available
+from xtuner._lite import get_device, get_logger, get_torch_device_module
+
+logger = get_logger()
+
+
+
+
+def npu_is_available():
+ return get_device() == 'npu'
+
+
+def mlu_is_available():
+ return get_device() == 'mlu'
+
+
+def varlen_attn_is_available():
+
+ return is_flash_attn_2_available() or npu_is_available()
+
+
+def lmdeploy_is_available():
+
+ available = False
+ try:
+ import lmdeploy # noqa: F401
+ available = True
+ except ImportError:
+ available = False
+
+ return available
+
+def liger_kernel_is_available():
+
+ available = False
+ try:
+ import liger_kernel # noqa: F401
+ available = True
+ except ImportError:
+ available = False
+
+ return available
+
+
+@contextmanager
+def profile_time_and_memory(desc):
+
+ torch_device = get_torch_device_module()
+ start_t = time.time()
+ torch_device.reset_peak_memory_stats()
+
+ yield
+
+ max_memory = torch_device.max_memory_allocated()
+ cost_time = time.time() - start_t
+
+ logger.success(f'{desc} Elapsed time {cost_time:.2f} seconds, '
+ f'peak gpu memory {max_memory/1024**3:.1f}G')
diff --git a/xtuner/_lite/algorithms/__init__.py b/xtuner/_lite/algorithms/__init__.py
new file mode 100644
index 000000000..e69de29bb
diff --git a/xtuner/_lite/algorithms/ppo/__init__.py b/xtuner/_lite/algorithms/ppo/__init__.py
new file mode 100644
index 000000000..2a76959bf
--- /dev/null
+++ b/xtuner/_lite/algorithms/ppo/__init__.py
@@ -0,0 +1,10 @@
+from .dataset import RewardBufferCollator, InferDataset, PPOTokenizeFunction, RewardBuffer
+from .loss import (CriticLoss, PPOPolicyLoss, compute_advantages_and_returns,
+ compute_kl_rewards, gather_logprobs)
+from .model import build_actor_model, build_reward_model
+
+__all__ = [
+ 'PPOCollator', 'PPODataset', 'PPOTokenizeFunction', 'CriticLoss',
+ 'PPOPolicyLoss', 'compute_advantages_and_returns', 'compute_rewards',
+ 'gather_logprobs', 'build_actor_model', 'build_reward_model'
+]
diff --git a/xtuner/_lite/algorithms/ppo/dataset.py b/xtuner/_lite/algorithms/ppo/dataset.py
new file mode 100644
index 000000000..4a40d75b4
--- /dev/null
+++ b/xtuner/_lite/algorithms/ppo/dataset.py
@@ -0,0 +1,170 @@
+import torch
+import json
+import numpy as np
+from xtuner._lite.chat.messages.chat import ChatMsg
+from xtuner._lite.datasets import OPENAI_CONVERT_MAP
+from torch import nn
+from ..sft import SftCollator, SftTokenizeFunction
+
+
+class InferDataset(torch.utils.data.Dataset):
+
+ def __init__(self, prompts, responses):
+ super().__init__()
+
+ assert len(prompts) == len(responses)
+ self.prompts = prompts
+ self.responses = responses
+ self.policies = None
+
+ def __len__(self):
+ return len(self.prompts)
+
+
+ def __getitem__(self, item):
+
+ prompt = self.prompts[item]
+ response = self.responses[item]
+ num_prefill_tokens = len(prompt)
+
+ input_ids = prompt + response
+ labels = [-100] * (num_prefill_tokens - 1) + response + [-100]
+
+ return {
+ 'input_ids': input_ids,
+ 'labels': labels,
+ 'num_tokens': len(input_ids)
+ }
+
+
+
+
+FASTER = False
+class RewardBuffer(torch.utils.data.Dataset):
+
+ def __init__(self, clip_min=-5,clip_max = 5, normalize=True, faster=False):
+ super().__init__()
+
+
+ self.clip_min = clip_min
+ self.clip_max = clip_max
+
+ self.normalize = normalize
+
+ if self.normalize:
+ self.bn = nn.BatchNorm1d(1, momentum=None, affine=False)
+ else:
+ self.bn = None
+
+ self._num_action_tokens = 0
+ self._num_total_tokens = 0
+ self._trajectories = []
+
+ self._current_mean = 0
+
+ @property
+ def running_mean(self):
+ return self.bn.running_mean.item()
+
+ @property
+ def current_mean(self):
+ return self._current_mean
+
+ @property
+ def num_action_tokens(self):
+ return self._num_action_tokens.item()
+
+ @property
+ def num_total_tokens(self):
+ return self._num_total_tokens
+
+ def update(self, trajectories):
+
+ rewards = [data['reward'] for data in trajectories]
+
+ for i in range(len(trajectories)):
+ trajectories[i]['ori_reward'] = trajectories[i]['reward']
+
+ rewards = torch.tensor(rewards)
+
+ self._current_mean = rewards.mean().item()
+
+ rewards = rewards.clip(self.clip_min, self.clip_max)
+
+ if self.normalize:
+ self.bn.train()
+ _ = self.bn(rewards.unsqueeze(-1))
+ self.bn.eval()
+ rewards = self.bn(rewards.unsqueeze(-1))
+
+ for i in range(len(trajectories)):
+ trajectories[i]['reward'] = rewards[i].item()
+
+ num_total_tokens = 0
+ num_action_tokens = 0
+ for data in trajectories:
+ labels = np.array(data['labels'])
+ num_total_tokens += labels.size
+ num_action_tokens += (labels >= 0).sum()
+
+ self._num_action_tokens = num_action_tokens
+ self._num_total_tokens = num_total_tokens
+
+ self._trajectories = trajectories
+
+ def dump_jsonl(self, path, tokenizer, debug=False):
+
+ with open(path, 'w', encoding='utf8') as f:
+ for data in self._trajectories:
+ json_line = {
+ 'num_tokens': data['num_tokens'],
+ 'reward': data['ori_reward'],
+ 'sequence': tokenizer.decode(data['input_ids']),
+ }
+
+ if debug:
+ json_line['input_ids'] = data['input_ids']
+ json_line['labels'] = data['labels']
+
+ json_str = json.dumps(json_line, ensure_ascii=False)
+ f.write(json_str + '\n')
+
+ def __len__(self):
+ return len(self._trajectories)
+
+
+ def __getitem__(self, item):
+
+ return self._trajectories[item]
+
+
+class PPOTokenizeFunction(SftTokenizeFunction):
+
+ def __init__(self,
+ tokenizer,
+ chat_template,
+ raw_format='openai',
+ sys_prompt=None):
+ super().__init__(tokenizer, chat_template, raw_format)
+ self.sys_prompt = sys_prompt
+
+ def __call__(self, item):
+
+ formatter = OPENAI_CONVERT_MAP[self.raw_format]
+ msg = formatter(item)
+ if self.sys_prompt is not None:
+ sys_msg = ChatMsg(role='system', content=self.sys_prompt)
+ msg.messages = [sys_msg] + msg.messages
+ tokenized = msg.tokenize(self.tokenizer, self.chat_template)
+
+ return tokenized
+
+
+class RewardBufferCollator(SftCollator):
+
+ def __call__(self, instances):
+
+ data = super().__call__(instances)
+ data['rewards'] = [item['reward'] for item in instances]
+
+ return data
diff --git a/xtuner/_lite/algorithms/ppo/loss.py b/xtuner/_lite/algorithms/ppo/loss.py
new file mode 100644
index 000000000..167379719
--- /dev/null
+++ b/xtuner/_lite/algorithms/ppo/loss.py
@@ -0,0 +1,125 @@
+import torch
+from torch.nn import functional as F
+
+from xtuner._lite import get_logger
+
+logger = get_logger()
+
+
+
+def gather_logprobs(logits, labels):
+ log_probs = F.log_softmax(logits, dim=-1)
+ log_probs_labels = log_probs.gather(dim=-1, index=labels.unsqueeze(-1))
+ return log_probs_labels.squeeze(-1)
+
+@torch.no_grad()
+def compute_kl_rewards(logprobs, ref_logprobs, reward_score, kl_coef=0.01):
+
+ assert logprobs.ndim == 1
+ last_mask = torch.zeros_like(logprobs, dtype=torch.int)
+ last_mask[-1] = 1
+
+ kl = (ref_logprobs - logprobs)
+ kl_reward = kl_coef * kl * (1 - last_mask)
+
+ last_reward = reward_score * last_mask
+
+ rewards = kl_reward + last_reward
+
+ return rewards
+
+@torch.no_grad()
+def compute_advantages_and_returns(values, rewards, gamma=1.0, gae_lambda=0.99):
+ # Adopted from https://github.com/CarperAI/trlx/blob/main/trlx/models/modeling_ppo.py#L134 # noqa: E501
+ """Function that computes advantages and returns from rewards and
+ values. Calculated as in the original PPO paper:
+ https://arxiv.org/abs/1707.06347 Note that rewards may include a KL
+ divergence loss term.
+
+ Advantages looks like this:
+ Adv1 = R1 + γ * λ * R2 + γ^2 * λ^2 * R3 + ...
+ - V1 + γ * (1 - λ) V2 + γ^2 * λ * (1 - λ) V3 + ...
+
+ Returns looks like this:
+ Ret1 = R1 + γ * λ * R2 + γ^2 * λ^2 * R3 + ...
+ + γ * (1 - λ) V2 + γ^2 * λ * (1 - λ) V3 + ...
+ """
+ lastgaelam = 0
+ advantages_reversed = []
+
+ assert values.numel() == rewards.numel(), f'{values.numel()}, {rewards.numel()}'
+ length = rewards.numel()
+
+ for t in reversed(range(0, length)):
+ nextvalues = values[t + 1] if t < length - 1 else 0.0
+ # Since old_rewards and old_values are masked with action_mask,
+ # i.e. they have 0's at pad tokens,
+ # delta will be 0 if current t is at a pad token,
+ # so will lastgaelam
+ delta = rewards[t] + gamma * nextvalues - values[t]
+ lastgaelam = delta + gamma * gae_lambda * lastgaelam
+ advantages_reversed.append(lastgaelam)
+
+ advantages = torch.stack(advantages_reversed[::-1], dim=0)
+ returns = advantages + values
+ return advantages.detach(), returns
+
+
+class CriticLoss(torch.nn.Module):
+ """Loss function for critic model."""
+
+ def __init__(self,
+ cliprange_value: float = 0.5,
+ loss_type: str = 'per_seq'):
+ super().__init__()
+ self.cliprange_value = cliprange_value
+ self.loss_type = loss_type
+
+ assert self.loss_type in ['per_token', 'per_seq']
+
+ def critic_loss_fn(self, values, old_values, returns, loss_factor=None):
+ values_clipped = old_values + (values - old_values).clamp(
+ -self.cliprange_value, self.cliprange_value)
+ vf_loss1 = (values_clipped - returns)**2
+ vf_loss2 = (values - returns)**2
+ if self.loss_type == 'per_seq':
+ vf_loss = torch.max(vf_loss1, vf_loss2).mean(-1)
+ elif self.loss_type == 'per_token':
+ assert loss_factor is not None
+ vf_loss = torch.sum(torch.max(vf_loss1, vf_loss2) * loss_factor)
+ return 0.5 * vf_loss
+
+ def forward(self,
+ values: torch.Tensor,
+ old_values,
+ returns,
+ loss_factor=None):
+
+ loss = self.critic_loss_fn(
+ values=values,
+ old_values=old_values,
+ returns=returns,
+ loss_factor=loss_factor)
+ return loss
+
+
+class PPOPolicyLoss(torch.nn.Module):
+ """Loss function for policy model."""
+
+ def __init__(self, cliprange: float = 0.2, loss_type: str = 'per_seq'):
+ super().__init__()
+ self.cliprange = cliprange
+ self.loss_type = loss_type
+ assert self.loss_type in ['per_token', 'per_seq']
+
+ def forward(self, logprobs, old_logprobs, advantages, loss_factor=None):
+ ratio = (logprobs - old_logprobs).exp()
+ pg_loss1 = -ratio * advantages
+ pg_loss2 = -ratio.clamp(1 - self.cliprange,
+ 1 + self.cliprange) * advantages
+ if self.loss_type == 'per_seq':
+ pg_loss = torch.max(pg_loss1, pg_loss2).mean(dim=-1)
+ elif self.loss_type == 'per_token':
+ assert loss_factor is not None
+ pg_loss = torch.sum(torch.max(pg_loss1, pg_loss2)) * loss_factor
+ return pg_loss
diff --git a/xtuner/_lite/algorithms/ppo/model.py b/xtuner/_lite/algorithms/ppo/model.py
new file mode 100644
index 000000000..2f90e810f
--- /dev/null
+++ b/xtuner/_lite/algorithms/ppo/model.py
@@ -0,0 +1,46 @@
+import torch
+from transformers import AutoConfig, AutoModel, AutoModelForCausalLM
+from transformers.utils.import_utils import (is_flash_attn_2_available,
+ is_torch_sdpa_available)
+
+from xtuner._lite.accelerate import LoadWoInit
+
+
+def build_actor_model(model_path, dtype=torch.float32, trust_remote_code=True):
+
+ config = AutoConfig.from_pretrained(model_path, trust_remote_code=True)
+ if is_flash_attn_2_available():
+ config.attn_implementation = 'flash_attention_2'
+ elif is_torch_sdpa_available():
+ config.attn_implementation = 'sdpa'
+
+ with LoadWoInit():
+ policy = AutoModelForCausalLM.from_pretrained(
+ model_path,
+ attn_implementation='flash_attention_2',
+ torch_dtype=dtype,
+ trust_remote_code=trust_remote_code)
+
+ return policy
+
+
+def build_reward_model(model_path, dtype=torch.float32, trust_remote_code=True):
+
+ config = AutoConfig.from_pretrained(model_path, trust_remote_code=True)
+ if is_flash_attn_2_available():
+ config.attn_implementation = 'flash_attention_2'
+ elif is_torch_sdpa_available():
+ config.attn_implementation = 'sdpa'
+
+ config.use_cache = False
+ config.torch_dtype = dtype
+ with LoadWoInit():
+ reward = AutoModel.from_pretrained(
+ model_path,
+ attn_implementation='flash_attention_2',
+ torch_dtype=dtype,
+ trust_remote_code=trust_remote_code)
+
+ reward.model.use_cache = False
+
+ return reward
diff --git a/xtuner/_lite/algorithms/sft/__init__.py b/xtuner/_lite/algorithms/sft/__init__.py
new file mode 100644
index 000000000..01a3a63a2
--- /dev/null
+++ b/xtuner/_lite/algorithms/sft/__init__.py
@@ -0,0 +1,3 @@
+from .dataset import SftCollator, SftTokenizeFunction
+
+__all__ = ['SftCollator', 'SftTokenizeFunction']
diff --git a/xtuner/_lite/algorithms/sft/dataset.py b/xtuner/_lite/algorithms/sft/dataset.py
new file mode 100644
index 000000000..bbb9e9608
--- /dev/null
+++ b/xtuner/_lite/algorithms/sft/dataset.py
@@ -0,0 +1,109 @@
+import torch
+from torch.nn.utils.rnn import pad_sequence
+
+from xtuner._lite import get_logger
+from xtuner._lite.datasets import OPENAI_CONVERT_MAP
+
+logger = get_logger()
+
+
+class SftTokenizeFunction():
+
+ def __init__(self, tokenizer, chat_template, raw_format='openai'):
+
+ self.tokenizer = tokenizer
+ self.chat_template = chat_template
+ self.raw_format = raw_format
+
+ def __call__(self, item):
+
+ formatter = OPENAI_CONVERT_MAP[self.raw_format]
+ msg = formatter(item)
+ tokenized = msg.tokenize(self.tokenizer, self.chat_template)
+ return tokenized
+
+
+class SftCollator():
+
+ def __init__(self, pad_token_id=0, ignore_id=-100, pack_batch=False, max_length=None):
+ self.pack_batch = pack_batch
+ self.pad_token_id = pad_token_id
+ self.ignore_id = ignore_id
+ self.max_length = max_length
+
+ def __call__(self, instances):
+
+ _instances = []
+ for ins in instances:
+ if isinstance(ins, list):
+ _instances.extend(ins)
+ else:
+ _instances.append(ins)
+
+ instances = _instances
+
+ input_ids = []
+ labels = []
+ num_tokens = []
+
+ for data in instances:
+
+ _input_ids = data['input_ids']
+ _labels = data['labels']
+ _num_tokens = data['num_tokens']
+
+ # TODO remove list
+ if isinstance(_num_tokens, list):
+ assert len(_num_tokens) == 1
+ _num_tokens = _num_tokens[0]
+
+ assert isinstance(_num_tokens, int)
+
+ if self.max_length:
+ _input_ids = _input_ids[:self.max_length]
+ _labels = _labels[:self.max_length]
+ _num_tokens = min(_num_tokens, self.max_length)
+
+ input_ids.append(torch.LongTensor(_input_ids))
+ labels.append(torch.LongTensor(_labels))
+ num_tokens.append(_num_tokens)
+
+ attention_mask = [torch.ones_like(ids) for ids in input_ids]
+ num_tokens = torch.IntTensor(num_tokens)
+
+ if len(instances) > 1 and self.pack_batch:
+
+ input_ids = torch.cat(input_ids, dim=0).unsqueeze(0)
+ labels = torch.cat(labels, dim=0).unsqueeze(0)
+ attention_mask = torch.cat(attention_mask, dim=0).unsqueeze(0)
+
+ elif len(instances) > 1 and not self.pack_batch:
+
+ input_ids = pad_sequence(
+ input_ids, batch_first=True, padding_value=self.pad_token_id)
+ labels = pad_sequence(
+ labels, batch_first=True, padding_value=self.ignore_id)
+ attention_mask = pad_sequence(
+ attention_mask, batch_first=True, padding_value=0)
+ else:
+ input_ids = torch.stack(input_ids)
+ labels = torch.stack(labels)
+ attention_mask = torch.stack(attention_mask)
+
+ if input_ids.shape != labels.shape:
+ logger.error(f'[instances] {instances}')
+ logger.error(f'[num_tokens] {num_tokens}')
+ logger.error(f'[input_ids] {input_ids}')
+ logger.error(f'[labels] {labels}')
+ raise RuntimeError('The shape of input_ids and labels must be '
+ f'equal, but found {input_ids.shape} and '
+ f'{labels.shape}.')
+
+ data_dict = {
+ 'input_ids': input_ids,
+ 'labels': labels,
+ 'num_tokens': num_tokens,
+ 'attention_mask': attention_mask.bool()
+ }
+
+ return data_dict
diff --git a/xtuner/_lite/chat/__init__.py b/xtuner/_lite/chat/__init__.py
new file mode 100644
index 000000000..6443e50b4
--- /dev/null
+++ b/xtuner/_lite/chat/__init__.py
@@ -0,0 +1,6 @@
+from .messages import ChatMessages
+from .templates import CHAT_TEMPLATE_MAP, ChatTemplate, HybridChatTemplate
+
+__all__ = [
+ 'ChatMessages', 'CHAT_TEMPLATE_MAP', 'ChatTemplate', 'HybridChatTemplate'
+]
diff --git a/xtuner/_lite/chat/backends/__init__.py b/xtuner/_lite/chat/backends/__init__.py
new file mode 100644
index 000000000..e69de29bb
diff --git a/xtuner/_lite/chat/messages/__init__.py b/xtuner/_lite/chat/messages/__init__.py
new file mode 100644
index 000000000..b8c75b45d
--- /dev/null
+++ b/xtuner/_lite/chat/messages/__init__.py
@@ -0,0 +1,4 @@
+from .base import BaseMessages
+from .chat import ChatMessages
+
+__all__ = ['BaseMessages', 'ChatMessages']
diff --git a/xtuner/_lite/chat/messages/base.py b/xtuner/_lite/chat/messages/base.py
new file mode 100644
index 000000000..0266986ac
--- /dev/null
+++ b/xtuner/_lite/chat/messages/base.py
@@ -0,0 +1,31 @@
+from abc import abstractclassmethod, abstractmethod
+from typing import Dict
+
+from pydantic import BaseModel
+from transformers import PreTrainedTokenizer
+
+from ..templates import ChatTemplate
+
+
+class BaseMessages(BaseModel):
+
+ @abstractmethod
+ def add(self, role: str, content):
+ pass
+
+ @abstractmethod
+ def pop(self):
+ pass
+
+ @abstractmethod
+ def get_prompt(self, chat_template: ChatTemplate) -> str:
+ pass
+
+ @abstractmethod
+ def tokenize(self, tokenizer: PreTrainedTokenizer,
+ chat_template: ChatTemplate) -> Dict:
+ pass
+
+ @abstractclassmethod
+ def from_dict(cls, item: Dict) -> 'BaseMessages':
+ pass
diff --git a/xtuner/_lite/chat/messages/chat.py b/xtuner/_lite/chat/messages/chat.py
new file mode 100644
index 000000000..af1756e8e
--- /dev/null
+++ b/xtuner/_lite/chat/messages/chat.py
@@ -0,0 +1,213 @@
+import copy
+from typing import Dict, List, Literal, Optional, Union
+
+from pydantic import BaseModel
+from transformers import PreTrainedTokenizer
+
+from xtuner._lite import get_logger
+from xtuner.utils import IGNORE_INDEX
+from ..templates import ChatTemplate, HybridChatTemplate
+from .base import BaseMessages
+
+logger = get_logger()
+
+
+class TextContentItem(BaseModel):
+ type: Literal['text'] = 'text'
+ text: str
+
+ def apply_chat_template(self, chat_template: HybridChatTemplate) -> str:
+ return self.text
+
+
+class ImageContentItem(BaseModel):
+ type: Literal['image_url'] = 'image_url'
+ image_url: str
+
+ def apply_chat_template(self, chat_template: HybridChatTemplate) -> str:
+ return chat_template.image_token
+
+
+MultModalContentType = Union[TextContentItem, ImageContentItem]
+ContentType = Union[str, List[MultModalContentType]]
+
+
+class ChatMsg(BaseModel):
+
+ role: Literal['assistant', 'user', 'system']
+ content: ContentType
+ loss: Optional[bool] = None
+
+ def __init__(self, *args, **kwargs):
+ super().__init__(*args, **kwargs)
+ if self.loss is None:
+ if self.role == 'system':
+ self.loss = False
+ elif self.role == 'user':
+ self.loss = False
+ elif self.role == 'assistant':
+ self.loss = True
+ else:
+ raise NotImplementedError
+
+ def collect_img_urls(self) -> List[str]:
+ img_urls = []
+ if isinstance(self.content, list):
+ for item in self.content:
+ if isinstance(item, ImageContentItem):
+ img_urls.append(item.image_url)
+ return img_urls
+
+ def get_prompt(self, chat_template: ChatTemplate) -> str:
+
+ if isinstance(self.content, str):
+ text = self.content
+ elif isinstance(self.content, list):
+ text = ''
+ for i, item in enumerate(self.content):
+ if i == 0:
+ text += item.apply_chat_template(chat_template)
+ else:
+ text += '\n' + item.apply_chat_template(chat_template)
+ else:
+ raise NotImplementedError
+
+ if self.role == 'system':
+ prompt = chat_template.decorate_system(text)
+ elif self.role == 'user':
+ prompt = chat_template.decorate_user(text)
+ elif self.role == 'assistant':
+ prompt = chat_template.decorate_assistant(text)
+ else:
+ raise NotImplementedError
+
+ return prompt
+
+ def tokenize(
+ self,
+ tokenizer: PreTrainedTokenizer,
+ chat_template: ChatTemplate,
+ ):
+
+ decorated = self.get_prompt(chat_template)
+
+ token_ids = tokenizer.encode(decorated, add_special_tokens=False)
+
+ if self.loss:
+ label_ids = copy.deepcopy(token_ids)
+ else:
+ label_ids = [IGNORE_INDEX] * len(token_ids)
+
+ return {
+ 'input_ids': token_ids,
+ 'labels': label_ids,
+ }
+
+
+class ChatMessages(BaseMessages):
+
+ messages: List[ChatMsg]
+
+ def add(self, role, content, loss=False):
+ self.messages.append(ChatMsg(role=role, content=content, loss=loss))
+
+ def pop(self):
+ return self.messages.pop()
+
+ def get_prompt(self, chat_template: ChatTemplate) -> str:
+
+ prompt = ''
+
+ for msg in self.messages:
+ prompt += msg.get_prompt(chat_template)
+ if msg.role == 'assistant':
+ prompt += chat_template.sep
+ return prompt
+
+ def tokenize(self, tokenizer: PreTrainedTokenizer,
+ chat_template: ChatTemplate) -> Dict:
+
+ input_ids = tokenizer.encode('', add_special_tokens=True)
+ labels = [IGNORE_INDEX for _ in input_ids]
+ image_urls = []
+
+
+
+ for msg in self.messages:
+ res = msg.tokenize(tokenizer, chat_template)
+ token_ids, label_ids = res['input_ids'], res['labels']
+
+ input_ids.extend(token_ids)
+ labels.extend(label_ids)
+
+ image_urls.extend(msg.collect_img_urls())
+
+ if msg.role == 'assistant':
+ sep = chat_template.sep
+ sep_tokens = tokenizer.encode(sep, add_special_tokens=False)
+ input_ids.extend(sep_tokens)
+ labels.extend([IGNORE_INDEX] * len(sep_tokens))
+
+ if len(input_ids) != len(labels):
+ logger.error(f'[messages] {self.messages}')
+ logger.error(f'[input_ids] {input_ids}')
+ logger.error(f'[labels] {labels}')
+ raise RuntimeError('The lengths of input_ids and labels must be '
+ f'equal, but found {len(input_ids)} and '
+ f'{len(labels)}.')
+
+ training_data = {
+ 'input_ids': input_ids,
+ 'labels': labels,
+ 'num_tokens': len(input_ids),
+ }
+
+ if len(image_urls) > 0:
+ training_data['image_urls'] = image_urls
+
+ return training_data
+
+ @classmethod
+ def from_str(cls, prompt: str) -> 'ChatMessages':
+
+ msg = ChatMsg(role='user', content=prompt)
+ return cls(messages=[msg])
+
+ @classmethod
+ def from_dict(cls, item: dict) -> 'ChatMessages':
+ '''
+ item
+ {
+ 'messages':[
+ {'role':'user', 'content':'hello'},
+ {'role':'assistant', 'content':'hello!'},
+ ],
+ }
+ '''
+ return cls(**item)
+
+
+if __name__ == '__main__':
+
+ data = {
+ 'messages': [
+ {
+ 'role': 'user',
+ 'content': 'hello'
+ },
+ {
+ 'role': 'assistant',
+ 'content': 'hello!'
+ },
+ ]
+ }
+
+ messages = ChatMessages.from_dict(data)
+ chat_template = ChatTemplate(
+ system='<|im_start|>system\n{system}<|im_end|>\n',
+ user='<|im_start|>user\n{user}<|im_end|>\n<|im_start|>assistant\n',
+ assistant='{assistant}<|im_end|>\n',
+ stop_words=['<|im_end|>'],
+ )
+
+ print(messages.get_prompt(chat_template))
diff --git a/xtuner/_lite/chat/templates/__init__.py b/xtuner/_lite/chat/templates/__init__.py
new file mode 100644
index 000000000..7ed468e20
--- /dev/null
+++ b/xtuner/_lite/chat/templates/__init__.py
@@ -0,0 +1,29 @@
+from .chat import ChatTemplate
+from .hybrid import HybridChatTemplate
+
+CHAT_TEMPLATE_MAP = {
+ 'internlm2':
+ HybridChatTemplate(
+ system='<|im_start|>system\n{system}<|im_end|>\n',
+ user='<|im_start|>user\n{user}<|im_end|>\n<|im_start|>assistant\n',
+ assistant='{assistant}<|im_end|>',
+ stop_words=['<|im_end|>']),
+ 'qwen2':
+ HybridChatTemplate(
+ system='<|im_start|>system\n{system}<|im_end|>\n',
+ user='<|im_start|>user\n{user}<|im_end|>\n<|im_start|>assistant\n',
+ assistant='{assistant}<|im_end|>',
+ stop_words=['<|im_end|>', '<|endoftext|>']),
+ 'llama3':
+ HybridChatTemplate(
+ system=('<|start_header_id|>system<|end_header_id|>\n\n{system}'
+ '<|eot_id|>'),
+ user=('<|start_header_id|>user<|end_header_id|>\n\n{user}<|eot_id|>'
+ '<|start_header_id|>assistant<|end_header_id|>\n\n'),
+ assistant='{assistant}<|eot_id|>',
+ sep='',
+ stop_words=['<|eot_id|>']),
+
+}
+
+__all__ = ['ChatTemplate', 'HybridChatTemplate']
diff --git a/xtuner/_lite/chat/templates/chat.py b/xtuner/_lite/chat/templates/chat.py
new file mode 100644
index 000000000..9ce574fef
--- /dev/null
+++ b/xtuner/_lite/chat/templates/chat.py
@@ -0,0 +1,59 @@
+from typing import List
+
+from pydantic import BaseModel, field_validator
+
+
+class ChatTemplate(BaseModel):
+ """Define a Pydantic data model for a hybrid chat with attributes for
+ system, user and assistant chat as well as function and interpreter calls
+ and results."""
+
+ # Normal Chat
+ system: str # System message format
+ user: str # User message format
+ assistant: str # Assistant message format
+ stop_words: List[str] # List of stop words
+ sep: str = '\n'
+
+ def decorate_system(self, text: str) -> str:
+ """Decorate text with the `system` template."""
+ return self.system.format(system=text)
+
+ def decorate_assistant(self, text: str) -> str:
+ """Decorate text with the `assistant` template."""
+ return self.assistant.format(assistant=text)
+
+ def decorate_user(self, text: str) -> str:
+ """Decorate text with the `user` template."""
+ return self.user.format(user=text)
+
+ @field_validator('system')
+ def check_system(cls, v: str) -> str:
+ """Validate that `system` contains '{system}'.
+
+ If not, raises a ValueError.
+ """
+ if v is not None and '{system}' not in v:
+ raise ValueError("system must contain the keyword '{system}'")
+ return v
+
+ @field_validator('user')
+ def check_user(cls, v: str) -> str:
+ """Validate that `user` contains '{user}'.
+
+ If not, raises a ValueError.
+ """
+ if v is not None and '{user}' not in v:
+ raise ValueError("user must contain the keyword '{user}'")
+ return v
+
+ @field_validator('assistant')
+ def check_assistant(cls, v: str) -> str:
+ """Validate that `assistant` contains '{assistant}'.
+
+ If not, raises a ValueError.
+ """
+ if v is not None and '{assistant}' not in v:
+ raise ValueError(
+ "assistant must contain the keyword '{assistant}'")
+ return v
diff --git a/xtuner/_lite/chat/templates/hybrid.py b/xtuner/_lite/chat/templates/hybrid.py
new file mode 100644
index 000000000..d9f563c0d
--- /dev/null
+++ b/xtuner/_lite/chat/templates/hybrid.py
@@ -0,0 +1,196 @@
+from typing import Dict, List, Optional
+
+from pydantic import BaseModel, field_validator
+
+
+class HybridChatTemplate(BaseModel):
+ """Define a Pydantic data model for a hybrid chat with attributes for
+ system, user and assistant chat as well as function and interpreter calls
+ and results."""
+
+ # Normal Chat
+ system: str # System message format
+ user: str # User message format
+ assistant: str # Assistant message format
+ stop_words: List[str] # List of stop words
+ sep: str = '\n'
+
+ # Multimodal Chat
+ # Predefined token and index for images
+ image_token: str = ''
+ image_token_index: int = -100
+
+ # Agent Chat
+
+ # Interpreter and function related strings
+ files: Optional[str] = None
+
+ functions: Optional[str] = None # Function description format
+ function_call: Optional[str] = None # Function call format
+ function_result: Optional[str] = None # Function result format
+
+ code_interpreter: Optional[str] = None
+ code_interpreter_call: Optional[str] = None # Interpreter call format
+ code_interpreter_result: Optional[str] = None # Interpreter result format
+
+ function_token: Optional[str] = None
+ code_interpreter_token: Optional[str] = None
+ action_start_token: Optional[str] = None
+ action_end_token: Optional[str] = None
+
+ @property
+ def mm_token_maps(self) -> Dict[str, int]:
+ """Return a dictionary that maps multimodal tokens to corresponding
+ token indexes."""
+ return {self.image_token: self.image_token_index}
+
+ def decorate_system(self, text: str) -> str:
+ """Decorate text with the `system` template."""
+ return self.system.format(system=text)
+
+ def decorate_assistant(self, text: str) -> str:
+ """Decorate text with the `assistant` template."""
+ return self.assistant.format(assistant=text)
+
+ def decorate_user(self, text: str) -> str:
+ """Decorate text with the `user` template."""
+ return self.user.format(user=text)
+
+ def decorate_files(self, text: str) -> str:
+ """Decorate text with the `functions` template."""
+ return self.files.format(files=text)
+
+ def decorate_functions(self, text: str) -> str:
+ """Decorate text with the `functions` template."""
+ return self.functions.format(functions=text)
+
+ def decorate_function_call(self, text: str, func: str) -> str:
+ """Decorate text with the `function_call` template."""
+ return self.function_call.format(assistant=text, function_call=func)
+
+ def decorate_function_result(self, text: str) -> str:
+ """Decorate text with the `function_result` template."""
+ return self.function_result.format(function_result=text)
+
+ def decorate_code_interpreter(self, text: str) -> str:
+ """Decorate text with the `code_interpreter` template."""
+ return self.code_interpreter.format(code_interpreter=text)
+
+ def decorate_code_interpreter_call(self, text: str, func: str) -> str:
+ """Decorate text with the `code_interpreter_call` template."""
+ return self.code_interpreter_call.format(
+ assistant=text, code_interpreter_call=func)
+
+ def decorate_code_interpreter_result(self, text: str) -> str:
+ """Decorate text with the `code_interpreter_result` template."""
+ return self.code_interpreter_result.format(
+ code_interpreter_result=text)
+
+ @field_validator('system')
+ def check_system(cls, v: str) -> str:
+ """Validate that `system` contains '{system}'.
+
+ If not, raises a ValueError.
+ """
+ if v is not None and '{system}' not in v:
+ raise ValueError("system must contain the keyword '{system}'")
+ return v
+
+ @field_validator('user')
+ def check_user(cls, v: str) -> str:
+ """Validate that `user` contains '{user}'.
+
+ If not, raises a ValueError.
+ """
+ if v is not None and '{user}' not in v:
+ raise ValueError("user must contain the keyword '{user}'")
+ return v
+
+ @field_validator('assistant')
+ def check_assistant(cls, v: str) -> str:
+ """Validate that `assistant` contains '{assistant}'.
+
+ If not, raises a ValueError.
+ """
+ if v is not None and '{assistant}' not in v:
+ raise ValueError(
+ "assistant must contain the keyword '{assistant}'")
+ return v
+
+ @field_validator('function_call')
+ def check_function_call(cls, v: str) -> str:
+ """Validate that `function_call` contains '{function_call}'.
+
+ If not, raises a ValueError.
+ """
+ if (v is not None and '{function_call}' not in v
+ and '{assistant}' not in v):
+ raise ValueError(
+ "function_call must contain the keywords '{function_call}'")
+ if v is not None and '{assistant}' not in v:
+ raise ValueError(
+ "function_call must contain the keyword '{assistant}' and "
+ "'{function_call}'")
+ return v
+
+ @field_validator('function_result')
+ def check_function_result(cls, v: str) -> str:
+ """Validate that `function_result` contains '{function_result}'.
+
+ If not, raises a ValueError.
+ """
+ if v is not None and '{function_result}' not in v:
+ raise ValueError(
+ "function_result must contain the keyword '{function_result}'")
+ return v
+
+ @field_validator('functions')
+ def check_functions(cls, v: str) -> str:
+ """Validate that `functions` contains '{functions}'.
+
+ If not, raises a ValueError.
+ """
+ if v is not None and '{functions}' not in v:
+ raise ValueError(
+ "functions must contain the keyword '{functions}'")
+ return v
+
+ @field_validator('code_interpreter')
+ def check_code_interpreter(cls, v: str) -> str:
+ """Validate that `code_interpreter` contains '{code_interpreter}'.
+
+ If not, raises a ValueError.
+ """
+ if v is not None and '{code_interpreter}' not in v:
+ raise ValueError('code_interpreter must contain the keyword '
+ "'{code_interpreter}'")
+ return v
+
+ @field_validator('code_interpreter_call')
+ def check_code_interpreter_call(cls, v: str) -> str:
+ """Validate that `code_interpreter_call` contains
+ '{code_interpreter_call}'.
+
+ If not, raises a ValueError.
+ """
+ if (v is not None and '{code_interpreter_call}' not in v
+ and '{assistant}' not in v):
+ raise ValueError('code_interpreter_call must contain the keywords '
+ "'{assistant}' and '{code_interpreter_call}'")
+ if v is not None and '{assistant}' not in v:
+ raise ValueError('code_interpreter_call must contain the keywords '
+ "'{assistant}' and '{code_interpreter_call}'")
+ return v
+
+ @field_validator('code_interpreter_result')
+ def check_code_interpreter_result(cls, v: str) -> str:
+ """Validate that `code_interpreter_result` contains
+ '{code_interpreter_result}'.
+
+ If not, raises a ValueError.
+ """
+ if v is not None and '{code_interpreter_result}' not in v:
+ raise ValueError(
+ 'code_interpreter_result must contain the keyword '
+ "'{code_interpreter_result}'")
+ return v
diff --git a/xtuner/_lite/datasets/__init__.py b/xtuner/_lite/datasets/__init__.py
new file mode 100644
index 000000000..f7dc3b293
--- /dev/null
+++ b/xtuner/_lite/datasets/__init__.py
@@ -0,0 +1,10 @@
+# Copyright (c) OpenMMLab. All rights reserved.
+from .json import JsonDataset
+from .jsonl import JsonlDataset
+from .pack import SoftPackDataset, HardPackDataset
+from .utils import DATASET_CLS_MAP, OPENAI_CONVERT_MAP, load_datasets
+
+__all__ = [
+ 'JsonDataset', 'JsonlDataset', 'SoftPackDataset', 'DATASET_CLS_MAP',
+ 'OPENAI_CONVERT_MAP', 'load_datasets'
+]
diff --git a/xtuner/_lite/datasets/internvl2/__init__.py b/xtuner/_lite/datasets/internvl2/__init__.py
new file mode 100644
index 000000000..e69de29bb
diff --git a/xtuner/_lite/datasets/internvl2/conversation.py b/xtuner/_lite/datasets/internvl2/conversation.py
new file mode 100644
index 000000000..10f7f6b14
--- /dev/null
+++ b/xtuner/_lite/datasets/internvl2/conversation.py
@@ -0,0 +1,393 @@
+"""
+Conversation prompt templates.
+
+We kindly request that you import fastchat instead of copying this file if you wish to use it.
+If you have changes in mind, please contribute back so the community can benefit collectively and continue to maintain these valuable templates.
+"""
+
+import dataclasses
+from enum import IntEnum, auto
+from typing import Dict, List, Tuple, Union
+
+
+class SeparatorStyle(IntEnum):
+ """Separator styles."""
+
+ ADD_COLON_SINGLE = auto()
+ ADD_COLON_TWO = auto()
+ ADD_COLON_SPACE_SINGLE = auto()
+ NO_COLON_SINGLE = auto()
+ NO_COLON_TWO = auto()
+ ADD_NEW_LINE_SINGLE = auto()
+ LLAMA2 = auto()
+ CHATGLM = auto()
+ CHATML = auto()
+ CHATINTERN = auto()
+ DOLLY = auto()
+ RWKV = auto()
+ PHOENIX = auto()
+ ROBIN = auto()
+ FALCON_CHAT = auto()
+ CHATGLM3 = auto()
+ INTERNVL_ZH = auto()
+ MPT = auto()
+
+
+@dataclasses.dataclass
+class Conversation:
+ """A class that manages prompt templates and keeps all conversation history."""
+
+ # The name of this template
+ name: str
+ # The template of the system prompt
+ system_template: str = '{system_message}'
+ # The system message
+ system_message: str = ''
+ # The names of two roles
+ roles: Tuple[str] = ('USER', 'ASSISTANT')
+ # All messages. Each item is (role, message).
+ messages: List[List[str]] = ()
+ # The number of few shot examples
+ offset: int = 0
+ # The separator style and configurations
+ sep_style: SeparatorStyle = SeparatorStyle.ADD_COLON_SINGLE
+ sep: str = '\n'
+ sep2: str = None
+ # Stop criteria (the default one is EOS token)
+ stop_str: Union[str, List[str]] = None
+ # Stops generation if meeting any token in this list
+ stop_token_ids: List[int] = None
+
+ def get_prompt(self) -> str:
+ """Get the prompt for generation."""
+ system_prompt = self.system_template.format(system_message=self.system_message)
+ if self.sep_style == SeparatorStyle.ADD_COLON_SINGLE:
+ ret = system_prompt + self.sep
+ for role, message in self.messages:
+ if message:
+ ret += role + ': ' + message + self.sep
+ else:
+ ret += role + ':'
+ return ret
+ elif self.sep_style == SeparatorStyle.ADD_COLON_TWO:
+ seps = [self.sep, self.sep2]
+ ret = system_prompt + seps[0]
+ for i, (role, message) in enumerate(self.messages):
+ if message:
+ ret += role + ': ' + message + seps[i % 2]
+ else:
+ ret += role + ':'
+ return ret
+ elif self.sep_style == SeparatorStyle.ADD_COLON_SPACE_SINGLE:
+ ret = system_prompt + self.sep
+ for role, message in self.messages:
+ if message:
+ ret += role + ': ' + message + self.sep
+ else:
+ ret += role + ': ' # must be end with a space
+ return ret
+ elif self.sep_style == SeparatorStyle.ADD_NEW_LINE_SINGLE:
+ ret = '' if system_prompt == '' else system_prompt + self.sep
+ for role, message in self.messages:
+ if message:
+ ret += role + '\n' + message + self.sep
+ else:
+ ret += role + '\n'
+ return ret
+ elif self.sep_style == SeparatorStyle.NO_COLON_SINGLE:
+ ret = system_prompt
+ for role, message in self.messages:
+ if message:
+ ret += role + message + self.sep
+ else:
+ ret += role
+ return ret
+ elif self.sep_style == SeparatorStyle.NO_COLON_TWO:
+ seps = [self.sep, self.sep2]
+ ret = system_prompt
+ for i, (role, message) in enumerate(self.messages):
+ if message:
+ ret += role + message + seps[i % 2]
+ else:
+ ret += role
+ return ret
+ elif self.sep_style == SeparatorStyle.RWKV:
+ ret = system_prompt
+ for i, (role, message) in enumerate(self.messages):
+ if message:
+ ret += (
+ role
+ + ': '
+ + message.replace('\r\n', '\n').replace('\n\n', '\n')
+ )
+ ret += '\n\n'
+ else:
+ ret += role + ':'
+ return ret
+ elif self.sep_style == SeparatorStyle.LLAMA2:
+ seps = [self.sep, self.sep2]
+ if self.system_message:
+ ret = system_prompt
+ else:
+ ret = '[INST] '
+ for i, (role, message) in enumerate(self.messages):
+ tag = self.roles[i % 2]
+ if message:
+ if i == 0:
+ ret += message + ' '
+ else:
+ ret += tag + ' ' + message + seps[i % 2]
+ else:
+ ret += tag
+ return ret
+ elif self.sep_style == SeparatorStyle.CHATGLM:
+ # source: https://huggingface.co/THUDM/chatglm-6b/blob/1d240ba371910e9282298d4592532d7f0f3e9f3e/modeling_chatglm.py#L1302-L1308
+ # source2: https://huggingface.co/THUDM/chatglm2-6b/blob/e186c891cf64310ac66ef10a87e6635fa6c2a579/modeling_chatglm.py#L926
+ round_add_n = 1 if self.name == 'chatglm2' else 0
+ if system_prompt:
+ ret = system_prompt + self.sep
+ else:
+ ret = ''
+
+ for i, (role, message) in enumerate(self.messages):
+ if i % 2 == 0:
+ ret += f'[Round {i//2 + round_add_n}]{self.sep}'
+
+ if message:
+ ret += f'{role}:{message}{self.sep}'
+ else:
+ ret += f'{role}:'
+ return ret
+ elif self.sep_style == SeparatorStyle.CHATML:
+ ret = '' if system_prompt == '' else system_prompt + self.sep + '\n'
+ for role, message in self.messages:
+ if message:
+ ret += role + '\n' + message + self.sep + '\n'
+ else:
+ ret += role + '\n'
+ return ret
+ elif self.sep_style == SeparatorStyle.CHATGLM3:
+ ret = ''
+ if self.system_message:
+ ret += system_prompt
+ for role, message in self.messages:
+ if message:
+ ret += role + '\n' + ' ' + message
+ else:
+ ret += role
+ return ret
+ elif self.sep_style == SeparatorStyle.CHATINTERN:
+ # source: https://huggingface.co/internlm/internlm-chat-7b-8k/blob/bd546fa984b4b0b86958f56bf37f94aa75ab8831/modeling_internlm.py#L771
+ seps = [self.sep, self.sep2]
+ ret = system_prompt
+ for i, (role, message) in enumerate(self.messages):
+ # if i % 2 == 0:
+ # ret += ""
+ if message:
+ ret += role + ':' + message + seps[i % 2] + '\n'
+ else:
+ ret += role + ':'
+ return ret
+ elif self.sep_style == SeparatorStyle.DOLLY:
+ seps = [self.sep, self.sep2]
+ ret = system_prompt
+ for i, (role, message) in enumerate(self.messages):
+ if message:
+ ret += role + ':\n' + message + seps[i % 2]
+ if i % 2 == 1:
+ ret += '\n\n'
+ else:
+ ret += role + ':\n'
+ return ret
+ elif self.sep_style == SeparatorStyle.PHOENIX:
+ ret = system_prompt
+ for role, message in self.messages:
+ if message:
+ ret += role + ': ' + '' + message + ''
+ else:
+ ret += role + ': ' + ''
+ return ret
+ elif self.sep_style == SeparatorStyle.ROBIN:
+ ret = system_prompt + self.sep
+ for role, message in self.messages:
+ if message:
+ ret += role + ':\n' + message + self.sep
+ else:
+ ret += role + ':\n'
+ return ret
+ elif self.sep_style == SeparatorStyle.FALCON_CHAT:
+ ret = ''
+ if self.system_message:
+ ret += system_prompt + self.sep
+ for role, message in self.messages:
+ if message:
+ ret += role + ': ' + message + self.sep
+ else:
+ ret += role + ':'
+
+ return ret
+ elif self.sep_style == SeparatorStyle.INTERNVL_ZH:
+ seps = [self.sep, self.sep2]
+ ret = self.system_message + seps[0]
+ for i, (role, message) in enumerate(self.messages):
+ if message:
+ ret += role + ': ' + message + seps[i % 2]
+ else:
+ ret += role + ':'
+ return ret
+ elif self.sep_style == SeparatorStyle.MPT:
+ ret = system_prompt + self.sep
+ for role, message in self.messages:
+ if message:
+ if type(message) is tuple:
+ message, _, _ = message
+ ret += role + message + self.sep
+ else:
+ ret += role
+ return ret
+ else:
+ raise ValueError(f'Invalid style: {self.sep_style}')
+
+ def set_system_message(self, system_message: str):
+ """Set the system message."""
+ self.system_message = system_message
+
+ def append_message(self, role: str, message: str):
+ """Append a new message."""
+ self.messages.append([role, message])
+
+ def update_last_message(self, message: str):
+ """Update the last output.
+
+ The last message is typically set to be None when constructing the prompt,
+ so we need to update it in-place after getting the response from a model.
+ """
+ self.messages[-1][1] = message
+
+ def to_gradio_chatbot(self):
+ """Convert the conversation to gradio chatbot format."""
+ ret = []
+ for i, (role, msg) in enumerate(self.messages[self.offset :]):
+ if i % 2 == 0:
+ ret.append([msg, None])
+ else:
+ ret[-1][-1] = msg
+ return ret
+
+ def to_openai_api_messages(self):
+ """Convert the conversation to OpenAI chat completion format."""
+ ret = [{'role': 'system', 'content': self.system_message}]
+
+ for i, (_, msg) in enumerate(self.messages[self.offset :]):
+ if i % 2 == 0:
+ ret.append({'role': 'user', 'content': msg})
+ else:
+ if msg is not None:
+ ret.append({'role': 'assistant', 'content': msg})
+ return ret
+
+ def copy(self):
+ return Conversation(
+ name=self.name,
+ system_template=self.system_template,
+ system_message=self.system_message,
+ roles=self.roles,
+ messages=[[x, y] for x, y in self.messages],
+ offset=self.offset,
+ sep_style=self.sep_style,
+ sep=self.sep,
+ sep2=self.sep2,
+ stop_str=self.stop_str,
+ stop_token_ids=self.stop_token_ids,
+ )
+
+ def dict(self):
+ return {
+ 'template_name': self.name,
+ 'system_message': self.system_message,
+ 'roles': self.roles,
+ 'messages': self.messages,
+ 'offset': self.offset,
+ }
+
+
+# A global registry for all conversation templates
+conv_templates: Dict[str, Conversation] = {}
+
+
+def register_conv_template(template: Conversation, override: bool = False):
+ """Register a new conversation template."""
+ if not override:
+ assert (
+ template.name not in conv_templates
+ ), f'{template.name} has been registered.'
+
+ conv_templates[template.name] = template
+
+
+def get_conv_template(name: str) -> Conversation:
+ """Get a conversation template."""
+ return conv_templates[name].copy()
+
+
+# Both Hermes-2 and internlm2-chat are chatml-format conversation templates. The difference
+# is that during training, the preprocessing function for the Hermes-2 template doesn't add
+# at the beginning of the tokenized sequence, while the internlm2-chat template does.
+# Therefore, they are completely equivalent during inference.
+register_conv_template(
+ Conversation(
+ name='Hermes-2',
+ system_template='<|im_start|>system\n{system_message}',
+ # note: The new system prompt was not used here to avoid changes in benchmark performance.
+ # system_message='我是书生·万象,英文名是InternVL,是由上海人工智能实验室、清华大学及多家合作单位联合开发的多模态大语言模型。',
+ system_message='你是由上海人工智能实验室联合商汤科技开发的书生多模态大模型,英文名叫InternVL, 是一个有用无害的人工智能助手。',
+ roles=('<|im_start|>user\n', '<|im_start|>assistant\n'),
+ sep_style=SeparatorStyle.MPT,
+ sep='<|im_end|>',
+ stop_token_ids=[
+ 2,
+ 6,
+ 7,
+ 8,
+ ],
+ stop_str='<|endoftext|>',
+ )
+)
+
+
+register_conv_template(
+ Conversation(
+ name='internlm2-chat',
+ system_template='<|im_start|>system\n{system_message}',
+ # note: The new system prompt was not used here to avoid changes in benchmark performance.
+ # system_message='我是书生·万象,英文名是InternVL,是由上海人工智能实验室、清华大学及多家合作单位联合开发的多模态大语言模型。',
+ system_message='你是由上海人工智能实验室联合商汤科技开发的书生多模态大模型,英文名叫InternVL, 是一个有用无害的人工智能助手。',
+ roles=('<|im_start|>user\n', '<|im_start|>assistant\n'),
+ sep_style=SeparatorStyle.MPT,
+ sep='<|im_end|>',
+ stop_token_ids=[
+ 2,
+ 92543,
+ 92542
+ ]
+ )
+)
+
+
+register_conv_template(
+ Conversation(
+ name='phi3-chat',
+ system_template='<|system|>\n{system_message}',
+ # note: The new system prompt was not used here to avoid changes in benchmark performance.
+ # system_message='我是书生·万象,英文名是InternVL,是由上海人工智能实验室、清华大学及多家合作单位联合开发的多模态大语言模型。',
+ system_message='你是由上海人工智能实验室联合商汤科技开发的书生多模态大模型,英文名叫InternVL, 是一个有用无害的人工智能助手。',
+ roles=('<|user|>\n', '<|assistant|>\n'),
+ sep_style=SeparatorStyle.MPT,
+ sep='<|end|>',
+ stop_token_ids=[
+ 2,
+ 32000,
+ 32007
+ ]
+ )
+)
diff --git a/xtuner/_lite/datasets/internvl2/dataset.py b/xtuner/_lite/datasets/internvl2/dataset.py
new file mode 100644
index 000000000..bc7bf9653
--- /dev/null
+++ b/xtuner/_lite/datasets/internvl2/dataset.py
@@ -0,0 +1,295 @@
+import os
+import torch.distributed as dist
+from mmengine.utils import mkdir_or_exist
+from torch.utils.data import ConcatDataset, DataLoader, Dataset
+import numpy as np
+import json
+import math
+from concurrent.futures import ProcessPoolExecutor
+from tqdm import tqdm
+import copy
+import random
+
+from ..json import calculate_json_sha256
+from ..jsonl import calculate_jsonl_sha256
+from ..pack import SoftPackDataset
+
+from xtuner._lite import get_logger
+from xtuner._lite.parallel import get_dp_mesh, VLMLengthGroupedSampler, ParallelSampler
+
+logger = get_logger()
+
+
+def _load_json_or_jsonl(json_path):
+ if json_path.endswith('.json'):
+ with open(json_path) as f:
+ data = json.load(f)
+ elif json_path.endswith('.jsonl'):
+ with open(json_path) as f:
+ data = f.readlines()
+ else:
+ raise ValueError(f'Unsupported file format: {json_path}, '
+ f'only support .json and .jsonl.')
+ return data
+
+
+class BaseOrigDataset(Dataset):
+ def __init__(self,
+ data_name,
+ data,
+ chat_template,
+ tokenizer,
+ max_length,
+ image_token_str='',
+ group_by_length=False,
+ pack_data=False,
+ pack_data_cache_dir=None,
+ random_sample=False):
+ self.data_name = data_name
+ self.max_length = max_length
+ self.group_by_length = group_by_length
+ self.pack_data = pack_data
+ self.pack_data_cache_dir = pack_data_cache_dir
+ self.chat_template = chat_template
+ self.image_token_str = image_token_str
+ self.tokenizer = tokenizer
+ self.tokenizer_workers = int(os.environ.get('XTUNER_TOKENIZE_WORKERS', 8))
+
+ try:
+ self.root = data['media_root']
+ except KeyError:
+ self.root = data.get('root', '')
+ logger.info(f"{dist.get_rank()} ======= Start to process dataset: {os.path.basename(data['annotation'])}")
+
+ self.annotation = data['annotation']
+ self._is_jsonl = self.annotation.endswith('.jsonl')
+ self.raw_data = _load_json_or_jsonl(self.annotation)
+
+ # -------------------pack---------------------------------------
+ self.num_tokens = None
+ self.pack_data_cache_dir = pack_data_cache_dir
+ if pack_data:
+ assert pack_data_cache_dir is not None, 'pack_data_cache_dir must be provided when pack_data is True'
+ self.num_tokens = self.calc_packing_info()
+ assert len(self.num_tokens) == len(
+ self.raw_data), f'===={len(self.num_tokens)} neq {len(self.raw_data)}===='
+
+ repeat_time = data.get('repeat_time', 1)
+ if repeat_time < 1:
+ # If repeat_time is less than 1, select a portion of the data
+ if random_sample:
+ num_samples = int(len(self.raw_data) * repeat_time)
+ sampled = random.sample([i for i in range(len(self.raw_data))], num_samples)
+ self.raw_data = [self.raw_data[index] for index in sampled]
+ if pack_data:
+ self.num_tokens = self.num_tokens[sampled]
+ else:
+ num_samples = int(len(self.raw_data) * repeat_time)
+ self.raw_data = self.raw_data[:num_samples]
+ if pack_data:
+ self.num_tokens = self.num_tokens[:num_samples]
+
+ if repeat_time > 1:
+ assert isinstance(repeat_time, int)
+ # Repeat the list if repeat_time is greater than 1
+ self.raw_data = self.raw_data * repeat_time
+ if pack_data:
+ self.num_tokens = np.tile(self.num_tokens, repeat_time)
+
+ if pack_data:
+ assert len(self.num_tokens) == len(self.raw_data), f' {len(self.num_tokens)} neq {len(self.raw_data)}'
+
+ self.group_length = []
+ if self.group_by_length and not pack_data:
+ self.group_length = self.calc_group_len()
+
+ def __len__(self):
+ return len(self.raw_data)
+
+ def calc_group_len(self):
+ raise NotImplementedError
+
+ def calc_packing_info(self):
+ if os.path.exists(self.pack_data_cache_dir):
+ assert os.path.isdir(self.pack_data_cache_dir)
+ else:
+ mkdir_or_exist(self.pack_data_cache_dir)
+
+ # TODO: more rubost way to calculate the hash
+ if self._is_jsonl:
+ file_hash = calculate_jsonl_sha256(self.annotation)
+ else:
+ file_hash = calculate_json_sha256(self.annotation)
+ file_cache_dir = os.path.join(self.pack_data_cache_dir, file_hash)
+ if not os.path.exists(file_cache_dir):
+ mkdir_or_exist(file_cache_dir)
+
+ if 'num_tokens.npy' in os.listdir(file_cache_dir):
+ _cached_file = os.path.join(file_cache_dir, 'num_tokens.npy')
+ num_tokens = np.load(_cached_file)
+ logger.info(f"Load num_tokens from cache: {os.path.basename(self.annotation)}")
+ else:
+ logger.info(f"Start calculating the cache of num_tokens: {os.path.basename(self.annotation)}")
+ num_tokens = self.count_tokens_for_pack(file_cache_dir)
+ return num_tokens
+
+ def count_tokens_for_pack(self, cache_dir=None):
+ num_samples = len(self.raw_data)
+
+ if dist.is_available():
+ world_size = dist.get_world_size()
+ rank = dist.get_rank()
+ else:
+ world_size = 1
+ rank = 0
+
+ num_per_rank = math.ceil(num_samples / world_size)
+
+ start = rank * num_per_rank
+ end = (rank + 1) * num_per_rank
+ dataset_shard = self.raw_data[start:end]
+
+ desc = f'[Rank {rank}] {os.path.basename(self.annotation)}'
+ with ProcessPoolExecutor(max_workers=self.tokenizer_workers) as executor:
+ tokenized = list(
+ tqdm(
+ executor.map(self.pre_tokenize_fn_for_pack, dataset_shard,
+ chunksize=min(max(1, len(dataset_shard) // self.tokenizer_workers), 500)),
+ desc=desc,
+ total=len(dataset_shard)))
+
+ _num_tokens = [data['num_tokens'] for data in tokenized]
+ _num_tokens = np.array(_num_tokens)
+
+ if dist.is_available():
+ num_tokens = [None] * world_size
+ dist.all_gather_object(num_tokens, _num_tokens)
+ num_tokens = np.concatenate(num_tokens, axis=0)
+ else:
+ num_tokens = _num_tokens
+
+ if rank == 0 and cache_dir:
+ save_path = os.path.join(cache_dir, 'num_tokens.npy')
+ np.save(save_path, num_tokens)
+
+ return num_tokens
+
+ def pre_tokenize_fn_for_pack(self, data):
+ raise NotImplementedError
+
+ def process_text(self, conversations, media_type='image', image_grids=None):
+ while conversations and conversations[0]['from'] == 'gpt':
+ # Skip the first one if it is from gpt
+ conversations = conversations[1:]
+
+ assert len(conversations) % 2 == 0, f'Invalid conversation length: {len(conversations)}'
+
+ input_ = ''
+ out_conversation = []
+ for msg in conversations:
+ if msg['from'] == 'human':
+ input_ += msg['value'].strip()
+ elif msg['from'] == 'gpt':
+ out_conversation.append({
+ 'input': input_,
+ 'output': msg['value'].strip()
+ })
+ input_ = ''
+ else:
+ raise NotImplementedError(f'Unsupported message type: {msg}')
+
+ input_ids, labels = [], []
+ for i, single_turn_conversation in enumerate(out_conversation):
+ input_ = single_turn_conversation.get('input', '')
+ if input_ is None:
+ input_ = ''
+ input_ = self.chat_template['user'].format(user=input_)
+
+ if i == 0:
+ input_ = self._process_media_format_first_round(input_, media_type, image_grids)
+ # TODO: support system prompt
+ # input_ = self.chat_template['system'] + input_
+ input_encode = self.tokenizer.encode(input_, add_special_tokens=True)
+ else:
+ input_encode = self.tokenizer.encode(input_, add_special_tokens=False)
+
+ input_ids += input_encode
+ labels += [-100] * len(input_encode)
+
+ output_text = single_turn_conversation.get('output', '')
+ output_encode = self.chat_template['assistant'].format(assistant=output_text)
+ output_encode = self.tokenizer.encode(output_encode, add_special_tokens=False)
+ input_ids += output_encode
+ labels += copy.deepcopy(output_encode)
+
+ if len(input_ids) > self.max_length:
+ input_ids = input_ids[:self.max_length]
+ labels = labels[:self.max_length]
+ logger.info(
+ f'Warning: input_ids length({len(input_ids)}) '
+ f'is longer than max_length, cut to {self.max_length}')
+ return {'input_ids': input_ids, 'labels': labels}
+
+ def _process_media_format_first_round(self, input_, media_type, image_grids):
+ raise NotImplementedError
+
+ @property
+ def modality_length(self):
+ return self.group_length
+
+ @property
+ def length(self):
+ group_length = np.array(self.group_length)
+ group_length = np.abs(group_length).tolist()
+ return group_length
+
+
+def build_dataset(args, datasets):
+ assert len(datasets) > 0, 'No dataset found.'
+ if args.dset_pack:
+ train_dataset = SoftPackDataset(datasets,
+ target=args.pack_max_length,
+ blend=args.concat_before_pack)
+ else:
+ train_dataset = ConcatDataset(datasets)
+ if dist.get_rank() == 0:
+ logger.info(f'[Dataset] (Original) {len(train_dataset)} samples.')
+ return train_dataset
+
+
+def build_train_dataloader(args, train_dataset, collate_fn):
+ dp_mesh = get_dp_mesh()
+ if args.group_by_length:
+ if args.dset_pack:
+ length_property = 'longest'
+ else:
+ length_property = 'length'
+ sampler = VLMLengthGroupedSampler(train_dataset, dp_mesh,
+ args.global_batch_size,
+ seed=args.seed,
+ length_property=length_property)
+ elif args.group_by_modality_length:
+ if args.dset_pack:
+ raise NotImplementedError
+ else:
+ sampler = VLMLengthGroupedSampler(train_dataset, dp_mesh,
+ args.global_batch_size,
+ seed=args.seed,
+ length_property='modality_length')
+ else:
+ sampler = ParallelSampler(
+ train_dataset, dp_mesh, args.global_batch_size, seed=args.seed, shuffle=True)
+
+ train_dataloader = DataLoader(
+ train_dataset,
+ batch_size=args.mirco_batch_size,
+ num_workers=args.num_workers,
+ sampler=sampler,
+ collate_fn=collate_fn,
+ persistent_workers=args.num_workers > 0)
+
+ if dist.get_rank() == 0:
+ logger.info(f'[Dataloader] {len(train_dataloader)} batches.')
+
+ dist.barrier()
+ return train_dataloader
diff --git a/xtuner/_lite/datasets/internvl2/process.py b/xtuner/_lite/datasets/internvl2/process.py
new file mode 100644
index 000000000..f0c48d752
--- /dev/null
+++ b/xtuner/_lite/datasets/internvl2/process.py
@@ -0,0 +1,705 @@
+import io
+
+from transformers.trainer_pt_utils import LabelSmoother
+
+IGNORE_TOKEN_ID = LabelSmoother.ignore_index
+from typing import Dict
+import torch
+import torchvision.transforms as T
+import transformers
+from .conversation import get_conv_template
+from PIL import Image
+from torchvision.transforms.functional import InterpolationMode
+import sys
+
+
+IMG_CONTEXT_TOKEN = ''
+IMG_START_TOKEN = '
'
+IMG_END_TOKEN = ''
+QUAD_START_TOKEN = ''
+QUAD_END_TOKEN = ''
+REF_START_TOKEN = '['
+REF_END_TOKEN = ']'
+BOX_START_TOKEN = ''
+BOX_END_TOKEN = ''
+IMAGENET_MEAN = (0.485, 0.456, 0.406)
+IMAGENET_STD = (0.229, 0.224, 0.225)
+CLIP_MEAN = (0.4814546, 0.4578275, 0.40821073)
+CLIP_STD = (0.2686295, 0.2613025, 0.2757711)
+SIGLIP_MEAN = (0.5, 0.5, 0.5)
+SIGLIP_STD = (0.5, 0.5, 0.5)
+IGNORE_INDEX = -100
+
+
+def expand2square(pil_img, background_color):
+ width, height = pil_img.size
+ if width == height:
+ return pil_img
+ elif width > height:
+ result = Image.new(pil_img.mode, (width, width), background_color)
+ result.paste(pil_img, (0, (width - height) // 2))
+ return result
+ else:
+ result = Image.new(pil_img.mode, (height, height), background_color)
+ result.paste(pil_img, ((height - width) // 2, 0))
+ return result
+
+
+def simulate_jpeg_degradation(quality):
+ def jpeg_degrade(img):
+ with io.BytesIO() as output:
+ img.convert('RGB').save(output, format='JPEG', quality=quality)
+ output.seek(0) # Move the reading cursor to the start of the stream
+ img_jpeg = Image.open(output).copy() # Use .copy() to make sure the image is loaded in memory
+ return img_jpeg
+ return jpeg_degrade
+
+
+# Define the JPEG compression quality range, pre-create all JPEG compression functions
+qualities = list(range(75, 101))
+jpeg_degrade_functions = {quality: simulate_jpeg_degradation(quality) for quality in qualities}
+
+
+def build_transform(is_train, input_size, pad2square=False, normalize_type='imagenet'):
+ if normalize_type == 'imagenet':
+ MEAN, STD = IMAGENET_MEAN, IMAGENET_STD
+ elif normalize_type == 'clip':
+ MEAN, STD = CLIP_MEAN, CLIP_STD
+ elif normalize_type == 'siglip':
+ MEAN, STD = SIGLIP_MEAN, SIGLIP_STD
+ else:
+ raise NotImplementedError
+ if is_train: # use data augumentation
+ transform = T.Compose([
+ T.Lambda(lambda img: img.convert('RGB') if img.mode != 'RGB' else img),
+ T.RandomChoice([T.Lambda(jpeg_degrade_functions[quality]) for quality in qualities]),
+ T.Resize((input_size, input_size), interpolation=InterpolationMode.BICUBIC),
+ T.ToTensor(),
+ T.Normalize(mean=MEAN, std=STD)
+ ])
+ else:
+ if pad2square is False: # now we use this transform function by default
+ transform = T.Compose([
+ T.Lambda(lambda img: img.convert('RGB') if img.mode != 'RGB' else img),
+ T.Resize((input_size, input_size), interpolation=InterpolationMode.BICUBIC),
+ T.ToTensor(),
+ T.Normalize(mean=MEAN, std=STD)
+ ])
+ else:
+ transform = T.Compose([
+ T.Lambda(lambda img: img.convert('RGB') if img.mode != 'RGB' else img),
+ T.Lambda(lambda img: expand2square(img, tuple(int(x * 255) for x in MEAN))),
+ T.Resize((input_size, input_size), interpolation=InterpolationMode.BICUBIC),
+ T.ToTensor(),
+ T.Normalize(mean=MEAN, std=STD)
+ ])
+
+ return transform
+
+
+def preprocess(
+ template_name,
+ sources,
+ tokenizer: transformers.PreTrainedTokenizer,
+ num_image_token_list: list,
+ text_only: bool = False,
+ group_by_length: bool = False,
+ use_packed_ds: bool = False,
+ ds_name: str = None,
+ num_image: int = 1
+) -> Dict:
+ conv = get_conv_template(template_name)
+ roles = {'human': conv.roles[0], 'gpt': conv.roles[1]}
+
+ # Apply prompt templates
+ conversations = []
+ for i, source in enumerate(sources):
+ if roles[source[0]['from']] != conv.roles[0]:
+ # Skip the first one if it is not from human
+ source = source[1:]
+
+ conv.messages = []
+ for j, sentence in enumerate(source):
+ role = roles[sentence['from']]
+ assert role == conv.roles[j % 2], f'{i}'
+ conv.append_message(role, sentence['value'])
+ conversations.append(conv.get_prompt())
+
+ if not text_only:
+ new_conversations = []
+ for conversation in conversations:
+ for i in range(num_image):
+ image_tokens = f'{IMG_START_TOKEN}{IMG_CONTEXT_TOKEN * num_image_token_list[i]}{IMG_END_TOKEN}'
+ conversation = conversation.replace('', image_tokens, 1)
+ new_conversations.append(conversation)
+ conversations = new_conversations
+
+ # Tokenize conversations
+ input_ids = tokenizer(
+ conversations,
+ return_tensors='pt',
+ padding=False if group_by_length or use_packed_ds else 'max_length',
+ max_length=tokenizer.model_max_length,
+ truncation=True,
+ ).input_ids
+ targets = input_ids.clone()
+
+ # assert conv.sep_style == SeparatorStyle.ADD_COLON_TWO
+
+ # Mask targets. Only compute loss on the assistant outputs.
+ sep = conv.sep + conv.roles[1] + ': '
+ for conversation, target in zip(conversations, targets):
+ total_len = int(target.ne(tokenizer.pad_token_id).sum())
+
+ turns = conversation.split(conv.sep2)
+ cur_len = 1
+ target[:cur_len] = IGNORE_TOKEN_ID
+ for i, turn in enumerate(turns):
+ if turn == '':
+ break
+ turn_len = len(tokenizer(turn).input_ids)
+
+ parts = turn.split(sep)
+ if len(parts) != 2:
+ break
+ parts[0] += sep
+ # "-2" is hardcoded for the Llama tokenizer to make the offset correct.
+ instruction_len = len(tokenizer(parts[0]).input_ids) - 2
+
+ if i != 0 and not tokenizer.legacy:
+ # The legacy and non-legacy modes handle special tokens differently
+ instruction_len -= 1
+
+ # Ignore the user instructions
+ target[cur_len: cur_len + instruction_len] = IGNORE_TOKEN_ID
+ cur_len += turn_len
+
+ if i != 0 and not tokenizer.legacy:
+ # The legacy and non-legacy modes handle special tokens differently
+ cur_len -= 1
+
+ target[cur_len:] = IGNORE_TOKEN_ID
+
+ if False: # Inspect and check the correctness of masking
+ z = target.clone()
+ z = torch.where(z == IGNORE_TOKEN_ID, tokenizer.unk_token_id, z)
+ logger.info(tokenizer.decode(z))
+ exit()
+
+ if cur_len < tokenizer.model_max_length:
+ if cur_len != total_len:
+ target[:] = IGNORE_TOKEN_ID
+ print(
+ f'WARNING: tokenization mismatch: {cur_len} vs. {total_len}.'
+ f' #turn = {len(turns) - 1}. (ignored). This dataset is {ds_name}.'
+ )
+ sys.stdout.flush()
+
+ return dict(
+ input_ids=input_ids,
+ labels=targets,
+ attention_mask=input_ids.ne(tokenizer.pad_token_id),
+ )
+
+
+def preprocess_mpt(
+ template_name,
+ sources,
+ tokenizer: transformers.PreTrainedTokenizer,
+ num_image_token_list: list,
+ text_only: bool = False,
+ group_by_length: bool = False,
+ use_packed_ds: bool = False,
+ ds_name: str = None,
+ num_image: int = 1
+) -> Dict:
+ conv = get_conv_template(template_name)
+ roles = {'human': conv.roles[0], 'gpt': conv.roles[1]}
+
+ # Apply prompt templates
+ conversations = []
+ for i, source in enumerate(sources):
+ if roles[source[0]['from']] != conv.roles[0]:
+ # Skip the first one if it is not from human
+ source = source[1:]
+
+ conv.messages = []
+ for j, sentence in enumerate(source):
+ role = roles[sentence['from']]
+ assert role == conv.roles[j % 2], f'{i}'
+ conv.append_message(role, sentence['value'])
+ conversations.append(conv.get_prompt())
+
+ if not text_only:
+ new_conversations = []
+ for conversation in conversations:
+ for i in range(num_image):
+ image_tokens = f'{IMG_START_TOKEN}{IMG_CONTEXT_TOKEN * num_image_token_list[i]}{IMG_END_TOKEN}'
+ conversation = conversation.replace('', image_tokens, 1)
+ new_conversations.append(conversation)
+ conversations = new_conversations
+
+ # Tokenize conversations
+ input_ids = tokenizer(
+ conversations,
+ return_tensors='pt',
+ padding=False if group_by_length or use_packed_ds else 'max_length',
+ max_length=tokenizer.model_max_length,
+ truncation=True,
+ ).input_ids
+ targets = input_ids.clone()
+
+ # Mask targets. Only compute loss on the assistant outputs.
+ sep = conv.sep + conv.roles[1] # <|im_end|><|im_start|>assistant\n
+ for conversation, target in zip(conversations, targets):
+ total_len = int(target.ne(tokenizer.pad_token_id).sum())
+
+ turns = conversation.split(conv.sep)
+ re_turns = [conv.sep.join(turns[:3])] # system + user + gpt
+ for conv_idx in range(3, len(turns), 2):
+ re_turns.append(conv.sep.join(turns[conv_idx:conv_idx + 2])) # user + gpt
+ cur_len = 0
+ target[:cur_len] = IGNORE_TOKEN_ID
+ for i, turn in enumerate(re_turns):
+ if turn == '':
+ break
+ turn_len = len(tokenizer(turn).input_ids) + 1
+
+ parts = turn.split(sep)
+ if len(parts) != 2:
+ break
+ parts[0] += sep
+ instruction_len = len(tokenizer(parts[0]).input_ids)
+
+ # Ignore the user instructions
+ target[cur_len: cur_len + instruction_len] = IGNORE_TOKEN_ID
+ # print(f'[question {i}]', tokenizer.decode(input_ids[:, cur_len: cur_len + instruction_len][0]))
+ # print(f'[answer {i}]', tokenizer.decode(input_ids[:, cur_len + instruction_len: cur_len + turn_len][0]))
+ # print(f'[label {i}]', target[cur_len + instruction_len: cur_len + turn_len])
+ cur_len += turn_len
+
+ target[cur_len:] = IGNORE_TOKEN_ID
+
+ if cur_len < tokenizer.model_max_length:
+ if cur_len != total_len:
+ target[:] = IGNORE_TOKEN_ID
+ print(
+ f'WARNING: tokenization mismatch: {cur_len} vs. {total_len}.'
+ f' #turn = {len(turns) - 1}. (ignored). This dataset is {ds_name}.'
+ )
+ sys.stdout.flush()
+
+ return dict(
+ input_ids=input_ids,
+ labels=targets,
+ attention_mask=input_ids.ne(tokenizer.pad_token_id),
+ )
+
+
+def preprocess_phi3(
+ template_name,
+ sources,
+ tokenizer: transformers.PreTrainedTokenizer,
+ num_image_token_list: list,
+ text_only: bool = False,
+ group_by_length: bool = False,
+ use_packed_ds: bool = False,
+ ds_name: str = None,
+ num_image: int = 1
+) -> Dict:
+ conv = get_conv_template(template_name)
+ roles = {'human': conv.roles[0], 'gpt': conv.roles[1]}
+
+ # Apply prompt templates
+ conversations = []
+ for i, source in enumerate(sources):
+ if roles[source[0]['from']] != conv.roles[0]:
+ # Skip the first one if it is not from human
+ source = source[1:]
+
+ conv.messages = []
+ for j, sentence in enumerate(source):
+ role = roles[sentence['from']]
+ assert role == conv.roles[j % 2], f'{i}'
+ conv.append_message(role, sentence['value'])
+ conversations.append(conv.get_prompt())
+
+ if not text_only:
+ new_conversations = []
+ for conversation in conversations:
+ for i in range(num_image):
+ image_tokens = f'{IMG_START_TOKEN}{IMG_CONTEXT_TOKEN * num_image_token_list[i]}{IMG_END_TOKEN}'
+ conversation = conversation.replace('', image_tokens, 1)
+ new_conversations.append(conversation)
+ conversations = new_conversations
+
+ # Tokenize conversations
+ tokenizer.padding_side = 'right'
+ input_ids = tokenizer(
+ conversations,
+ return_tensors='pt',
+ padding=False if group_by_length or use_packed_ds else 'max_length',
+ max_length=tokenizer.model_max_length,
+ truncation=True,
+ ).input_ids
+ targets = input_ids.clone()
+
+ # Mask targets. Only compute loss on the assistant outputs.
+ sep = conv.sep + conv.roles[1] # <|end|>\n<|assistant|>
+ for conversation, target in zip(conversations, targets):
+ total_len = int(target.ne(int(tokenizer.pad_token_id)).sum())
+
+ turns = conversation.split(conv.sep)
+ re_turns = [conv.sep.join(turns[:3])] # system + user + gpt
+ for conv_idx in range(3, len(turns), 2):
+ re_turns.append(conv.sep.join(turns[conv_idx:conv_idx + 2])) # user + gpt
+ cur_len = 1
+ target[:cur_len] = IGNORE_TOKEN_ID
+ endoftext_id = tokenizer.convert_tokens_to_ids('<|endoftext|>')
+ target[target == endoftext_id] = IGNORE_TOKEN_ID
+
+ for i, turn in enumerate(re_turns):
+ if turn == '':
+ break
+ if i == 0:
+ turn_len = len(tokenizer(turn).input_ids)
+ else:
+ turn_len = len(tokenizer(turn).input_ids) - 1
+ parts = turn.split(sep)
+ if len(parts) != 2:
+ break
+ parts[0] += sep
+
+ if i == 0:
+ instruction_len = len(tokenizer(parts[0]).input_ids) - 1
+ else:
+ instruction_len = len(tokenizer(parts[0]).input_ids) - 2
+
+ # Ignore the user instructions
+ target[cur_len: cur_len + instruction_len] = IGNORE_TOKEN_ID
+ # print(f'[question {i}]', tokenizer.decode(input_ids[:, cur_len: cur_len + instruction_len][0]))
+ # print(f'[answer {i}]', tokenizer.decode(input_ids[:, cur_len + instruction_len: cur_len + turn_len][0]))
+ # print(f'[label {i}]', target[cur_len + instruction_len: cur_len + turn_len])
+ cur_len += turn_len
+
+ target[cur_len:] = IGNORE_TOKEN_ID
+
+ if False: # Inspect and check the correctness of masking
+ z = target.clone()
+ z = torch.where(z == IGNORE_TOKEN_ID, tokenizer.unk_token_id, z)
+ print(repr(tokenizer.decode(z)))
+
+ if cur_len < tokenizer.model_max_length:
+ if cur_len != total_len:
+ target[:] = IGNORE_TOKEN_ID
+ print(
+ f'WARNING: tokenization mismatch: {cur_len} vs. {total_len}.'
+ f' #turn = {len(turns) - 1}. (ignored). This dataset is {ds_name}.'
+ )
+ sys.stdout.flush()
+
+ return dict(
+ input_ids=input_ids,
+ labels=targets,
+ attention_mask=input_ids.ne(tokenizer.pad_token_id),
+ )
+
+
+def preprocess_phi3_fast(
+ template_name,
+ sources,
+ tokenizer: transformers.PreTrainedTokenizer,
+ num_image_token_list: list,
+ text_only: bool = False,
+ group_by_length: bool = False,
+ use_packed_ds: bool = False,
+ ds_name: str = None,
+ num_image: int = 1
+) -> Dict:
+ conv = get_conv_template(template_name)
+ roles = {'human': conv.roles[0], 'gpt': conv.roles[1]}
+
+ for i, source in enumerate(sources):
+ if roles[source[0]['from']] != conv.roles[0]:
+ # Skip the first one if it is not from human
+ source = source[1:]
+
+ conv.messages = []
+ for j, sentence in enumerate(source):
+ role = roles[sentence['from']]
+ assert role == conv.roles[j % 2], f'{i}'
+ conv.append_message(role, sentence['value'])
+
+ assert len(conv.messages) % 2 == 0, f'{ds_name}, {len(conv.messages)}, {conv.messages}'
+ inputs = conv.messages[::2]
+ outputs = conv.messages[1::2]
+
+ input_ids, labels = [], []
+ # input_texts = ''
+ system_prompt = conv.system_template.format(system_message=conv.system_message)
+ input_text = system_prompt + conv.sep
+ # input_texts += input_text
+ input_encode = tokenizer.encode(input_text, add_special_tokens=True)
+ input_ids += input_encode
+ labels += [IGNORE_INDEX] * len(input_encode)
+
+ real_num_images = 0
+ for input_, output_ in zip(inputs, outputs):
+ # output_[0] = '<|assistant|>\n'
+ # 放到 input 而不是 output 是为了和官方对齐
+ input_text = ''.join(input_) + conv.sep + output_[0]
+
+ if not text_only:
+ real_num_images += input_text.count('')
+ for i in range(num_image):
+ image_tokens = f'{IMG_START_TOKEN}{IMG_CONTEXT_TOKEN * num_image_token_list[i]}{IMG_END_TOKEN}'
+ input_text = input_text.replace('', image_tokens, 1)
+ assert '' not in input_text, f'error: {ds_name}, {input_text}'
+ output_text = output_[1] + conv.sep
+
+ input_encode = tokenizer.encode(input_text, add_special_tokens=False)
+ output_encode = tokenizer.encode(output_text, add_special_tokens=False)
+ input_ids += input_encode
+ input_ids += output_encode
+ labels += [IGNORE_INDEX] * len(input_encode)
+ labels += output_encode
+
+ # input_texts += input_text
+ # input_texts += output_text
+
+ if not text_only:
+ assert real_num_images == num_image, f'{ds_name} data error: {real_num_images} vs. {num_image}'
+ # print(input_texts)
+ # assert input_ids.count(32013) == num_image_token_list[
+ # 0], f'error1: {input_ids}, {num_image_token_list[0]}, {input_texts}'
+ if len(input_ids) > tokenizer.model_max_length:
+ print(f'WARNING: input_ids length {len(input_ids)} exceeds '
+ f'model_max_length {tokenizer.model_max_length}. truncated!')
+ input_ids = input_ids[:tokenizer.model_max_length]
+ labels = labels[:tokenizer.model_max_length]
+
+ # if not text_only:
+ # if input_ids.count(32013) != num_image_token_list[0]:
+ # print(f'WARNING: IMG_CONTEXT_TOKEN is broken. {input_ids.count(32013)} vs. {num_image_token_list[0]}')
+
+ input_ids = torch.tensor(input_ids, dtype=torch.long)[None]
+ labels = torch.tensor(labels, dtype=torch.long)[None]
+ assert input_ids.size() == labels.size()
+ return dict(
+ input_ids=input_ids,
+ labels=labels,
+ attention_mask=input_ids.ne(tokenizer.pad_token_id),
+ )
+
+
+def preprocess_internlm(
+ template_name,
+ sources,
+ tokenizer: transformers.PreTrainedTokenizer,
+ num_image_token_list: list,
+ text_only: bool = False,
+ group_by_length: bool = False,
+ use_packed_ds: bool = False,
+ ds_name: str = None,
+ num_image: int = 1
+) -> Dict:
+ conv = get_conv_template(template_name)
+ roles = {'human': conv.roles[0], 'gpt': conv.roles[1]}
+
+ # Apply prompt templates
+ conversations = []
+ for i, source in enumerate(sources):
+ if roles[source[0]['from']] != conv.roles[0]:
+ # Skip the first one if it is not from human
+ source = source[1:]
+
+ conv.messages = []
+ for j, sentence in enumerate(source):
+ role = roles[sentence['from']]
+ assert role == conv.roles[j % 2], f'{i}'
+ sentence['value'] = sentence['value'].strip()
+ conv.append_message(role, sentence['value'])
+ conversations.append(conv.get_prompt())
+
+ if not text_only:
+ new_conversations = []
+ for conversation in conversations:
+ for i in range(num_image):
+ image_tokens = f'{IMG_START_TOKEN}{IMG_CONTEXT_TOKEN * num_image_token_list[i]}{IMG_END_TOKEN}'
+ conversation = conversation.replace('', image_tokens, 1)
+ new_conversations.append(conversation)
+ conversations = new_conversations
+
+ # Tokenize conversations
+ input_ids = tokenizer(
+ conversations,
+ return_tensors='pt',
+ padding=False if group_by_length or use_packed_ds else 'max_length',
+ max_length=tokenizer.model_max_length,
+ truncation=True,
+ ).input_ids
+ targets = input_ids.clone()
+
+ for conversation, target in zip(conversations, targets):
+ total_len = int(target.ne(tokenizer.pad_token_id).sum()) # 浦语里面 pad_token_id = eos_token_id
+ cur_len = 1
+ target[:cur_len] = IGNORE_TOKEN_ID #
+ parts = conversation.split(conv.roles[1]) # [UNUSED_TOKEN_146]assistant\n
+ info = parts[0] + conv.roles[1]
+ temp_len = len(tokenizer(info).input_ids) - 1 # 去除tokenizer的
+ target[cur_len: cur_len + temp_len] = IGNORE_TOKEN_ID
+ cur_len = cur_len + temp_len
+
+ for index in range(1, len(parts) - 1):
+ info = parts[index]
+ part1, part2 = info.split(conv.roles[0])
+ temp_len = len(tokenizer(part1).input_ids) - 1
+ cur_len = cur_len + temp_len
+ part = conv.roles[0] + part2 + conv.roles[1]
+ temp_len = len(tokenizer(part).input_ids) - 1
+ target[cur_len: cur_len + temp_len] = IGNORE_TOKEN_ID
+ cur_len = cur_len + temp_len
+ last_info = parts[-1]
+ temp_len = len(tokenizer(last_info).input_ids) - 1
+ cur_len = cur_len + temp_len
+
+ target[cur_len:] = IGNORE_TOKEN_ID
+ if False: # Inspect and check the correctness of masking
+ z = target.clone()
+ z = torch.where(z == IGNORE_TOKEN_ID, tokenizer.unk_token_id, z)
+ print(repr(tokenizer.decode(z)))
+
+ if cur_len < tokenizer.model_max_length:
+ if cur_len != total_len:
+ target[:] = IGNORE_TOKEN_ID
+ print(f'WARNING: tokenization mismatch: {cur_len} vs. {total_len}. This dataset is {ds_name}.')
+ sys.stdout.flush()
+
+ return dict(
+ input_ids=input_ids,
+ labels=targets,
+ attention_mask=input_ids.ne(tokenizer.pad_token_id),
+ )
+
+
+def find_closest_aspect_ratio(aspect_ratio, target_ratios, width, height, image_size):
+ best_ratio_diff = float('inf')
+ best_ratio = (1, 1)
+ area = width * height
+ for ratio in target_ratios:
+ target_aspect_ratio = ratio[0] / ratio[1]
+ ratio_diff = abs(aspect_ratio - target_aspect_ratio)
+ if ratio_diff < best_ratio_diff:
+ best_ratio_diff = ratio_diff
+ best_ratio = ratio
+ elif ratio_diff == best_ratio_diff:
+ if area > 0.5 * image_size * image_size * ratio[0] * ratio[1]:
+ best_ratio = ratio
+ # print(f'width: {width}, height: {height}, best_ratio: {best_ratio}')
+ return best_ratio
+
+
+def dynamic_preprocess(image, min_num=1, max_num=6, image_size=448, use_thumbnail=False):
+ orig_width, orig_height = image.size
+ aspect_ratio = orig_width / orig_height
+
+ # calculate the existing image aspect ratio
+ target_ratios = set(
+ (i, j) for n in range(min_num, max_num + 1) for i in range(1, n + 1) for j in range(1, n + 1) if
+ i * j <= max_num and i * j >= min_num)
+ target_ratios = sorted(target_ratios, key=lambda x: x[0] * x[1])
+
+ # find the closest aspect ratio to the target
+ target_aspect_ratio = find_closest_aspect_ratio(
+ aspect_ratio, target_ratios, orig_width, orig_height, image_size)
+
+ # calculate the target width and height
+ target_width = image_size * target_aspect_ratio[0]
+ target_height = image_size * target_aspect_ratio[1]
+ blocks = target_aspect_ratio[0] * target_aspect_ratio[1]
+
+ # resize the image
+ resized_img = image.resize((target_width, target_height))
+ processed_images = []
+ for i in range(blocks):
+ box = (
+ (i % (target_width // image_size)) * image_size,
+ (i // (target_width // image_size)) * image_size,
+ ((i % (target_width // image_size)) + 1) * image_size,
+ ((i // (target_width // image_size)) + 1) * image_size
+ )
+ # split the image
+ split_img = resized_img.crop(box)
+ processed_images.append(split_img)
+ assert len(processed_images) == blocks
+ if use_thumbnail and len(processed_images) != 1:
+ thumbnail_img = image.resize((image_size, image_size))
+ processed_images.append(thumbnail_img)
+ return processed_images
+
+
+def dynamic_num_patch(size, min_num=1, max_num=6, image_size=448, use_thumbnail=False):
+ orig_width, orig_height = size
+ aspect_ratio = orig_width / orig_height
+
+ # calculate the existing image aspect ratio
+ target_ratios = set(
+ (i, j) for n in range(min_num, max_num + 1) for i in range(1, n + 1) for j in range(1, n + 1) if
+ i * j <= max_num and i * j >= min_num)
+ target_ratios = sorted(target_ratios, key=lambda x: x[0] * x[1])
+
+ # find the closest aspect ratio to the target
+ target_aspect_ratio = find_closest_aspect_ratio(
+ aspect_ratio, target_ratios, orig_width, orig_height, image_size)
+
+ # calculate the target width and height
+ blocks = target_aspect_ratio[0] * target_aspect_ratio[1]
+
+ if use_thumbnail and blocks > 1:
+ blocks += 1
+ return blocks
+
+
+def packing_collate(features, pack_batch=True, pad_id=0):
+ input_ids = []
+ labels = []
+ pixel_values = []
+ num_tokens = []
+ num_img_tokens = []
+ image_flags = []
+
+ for data in features:
+ input_ids.append(torch.LongTensor(data['input_ids']))
+ labels.append(torch.LongTensor(data['labels']))
+ num_tokens.extend(data['num_tokens'])
+ num_img_tokens.extend(data['num_img_tokens'])
+ pixel_values.append(data['pixel_values'])
+ image_flags.append(data['image_flags'])
+
+ attention_mask = [ids.ne(pad_id) for ids in input_ids]
+ num_tokens = torch.IntTensor(num_tokens)
+ num_img_tokens = torch.IntTensor(num_img_tokens)
+
+ if len(features) > 1 and pack_batch:
+ # batch packing
+ input_ids = torch.cat(input_ids, dim=0).unsqueeze(0)
+ labels = torch.cat(labels, dim=0).unsqueeze(0)
+ attention_mask = torch.cat(attention_mask, dim=0).unsqueeze(0)
+ image_flags = torch.cat(image_flags, dim=0)
+ pixel_values = torch.cat(pixel_values, dim=0)
+ elif len(features) > 1 and not pack_batch:
+ raise NotImplementedError
+ else:
+ raise NotImplementedError
+
+ data_dict = {
+ 'input_ids': input_ids,
+ 'labels': labels,
+ 'attention_mask': attention_mask.bool(),
+ 'pixel_values': pixel_values,
+ 'image_flags': image_flags,
+ 'num_tokens': num_tokens,
+ 'num_img_tokens': num_img_tokens,
+ }
+
+ return data_dict
\ No newline at end of file
diff --git a/xtuner/_lite/datasets/json.py b/xtuner/_lite/datasets/json.py
new file mode 100644
index 000000000..3efd91a1d
--- /dev/null
+++ b/xtuner/_lite/datasets/json.py
@@ -0,0 +1,173 @@
+import hashlib
+import inspect
+import json
+import math
+import os
+import random
+from concurrent.futures import ProcessPoolExecutor
+from mmengine import mkdir_or_exist
+import numpy as np
+import torch
+from torch import distributed as dist
+from tqdm import tqdm
+from xtuner._lite import get_logger
+
+
+logger = get_logger()
+
+def calculate_json_sha256(file_path):
+ with open(file_path, 'rb') as f:
+ data = f.read()
+
+ hash_object = hashlib.sha256(data)
+ hash_hex = hash_object.hexdigest()
+ return hash_hex
+
+
+def calculate_tokenize_fn_sha256(tokenize_fn):
+ """Calculate SHA-256 hash for an instance method's source code."""
+ # Get the source code of the method
+ fn_source = inspect.getsource(tokenize_fn.__call__)
+ return hashlib.sha256(fn_source.encode('utf-8')).hexdigest()
+
+
+class JsonDataset(torch.utils.data.Dataset):
+
+ def __init__(self,
+ path,
+ sample_ratio=1.0,
+ tokenize_fn=None,
+ cache_dir=None,
+ max_length=None):
+ super().__init__()
+
+ self.tokenize_fn = tokenize_fn
+ self.path = path
+ self.tokenizer_workers = int(os.environ.get('XTUNER_TOKENIZE_WORKERS', 8))
+
+ if cache_dir:
+ if os.path.exists(cache_dir):
+ assert os.path.isdir(cache_dir)
+ else:
+ mkdir_or_exist(cache_dir)
+
+ file_hash = calculate_json_sha256(path)
+ file_cache_dir = os.path.join(cache_dir, file_hash)
+
+ if file_hash not in os.listdir(cache_dir):
+ mkdir_or_exist(file_cache_dir)
+
+ if self.tokenize_fn:
+ tok_hash = calculate_tokenize_fn_sha256(tokenize_fn)
+ tok_cache_dir = os.path.join(file_cache_dir, tok_hash)
+ if tok_hash not in os.listdir(file_cache_dir):
+ mkdir_or_exist(tok_cache_dir)
+
+ if 'num_tokens.npy' in os.listdir(tok_cache_dir):
+ _cached_file = os.path.join(tok_cache_dir,
+ 'num_tokens.npy')
+ num_tokens = np.load(_cached_file)
+ else:
+ num_tokens = self.count_tokens(tok_cache_dir)
+ else:
+ num_tokens = None
+
+ else:
+ num_tokens = None
+
+ with open(self.path) as f:
+ dataset = json.load(f)
+
+ _sampled = [i for i in range(len(dataset))]
+
+ if max_length is not None:
+ assert isinstance(max_length, int)
+ _filtered = [x for i, x in enumerate(_sampled) if num_tokens[i] < max_length]
+
+ if len(_filtered) < len(_sampled):
+ missed_num = len(_sampled) - len(_filtered)
+ logger.warning(f"{path} has {missed_num} prompt length>{max_length}, discard.")
+
+ _sampled = _filtered
+
+ _target_num_samples = int(len(_sampled) * sample_ratio)
+ self.sampled = _sampled * int(sample_ratio)
+ self.sampled.extend(random.sample(_sampled, _target_num_samples - len(self.sampled)))
+
+ if num_tokens is not None:
+ num_tokens = num_tokens[self.sampled]
+
+ self.num_tokens = num_tokens
+ self.dataset = None
+
+ def count_tokens(self, cache_dir=None):
+
+ dataset = []
+
+ with open(self.path) as f:
+ dataset = json.load(f)
+
+ num_samples = len(dataset)
+
+ if dist.is_available():
+ world_size = dist.get_world_size()
+ rank = dist.get_rank()
+ else:
+ world_size = 1
+ rank = 0
+
+ num_per_rank = math.ceil(num_samples / world_size)
+
+ start = rank * num_per_rank
+ end = (rank + 1) * num_per_rank
+ dataset_shard = dataset[start:end]
+
+ desc = f'[Rank {rank}] {self.path}'
+ chunk_size = min(1024, max(1, len(dataset_shard) // self.tokenizer_workers))
+ with ProcessPoolExecutor(max_workers=self.tokenizer_workers) as executor:
+ tokenized = list(
+ tqdm(
+ executor.map(self.tokenize_fn, dataset_shard,
+ chunksize=chunk_size),
+ desc=desc,
+ total=len(dataset_shard)))
+
+ _num_tokens = [data['num_tokens'] for data in tokenized]
+ _num_tokens = np.array(_num_tokens)
+
+ if dist.is_available():
+ num_tokens = [None] * world_size
+ dist.all_gather_object(num_tokens, _num_tokens)
+ num_tokens = np.concatenate(num_tokens, axis=0)
+ else:
+ num_tokens = _num_tokens
+
+ if rank == 0 and cache_dir:
+ save_path = os.path.join(cache_dir, 'num_tokens.npy')
+ np.save(save_path, num_tokens)
+
+ return num_tokens
+
+ def __len__(self):
+ return len(self.sampled)
+
+ def __getitem__(self, item):
+ """Returns a dict containing packed data in the given item.
+
+ Args:
+ item: An index to retrieve packed data.
+
+ Returns:
+ A dict including packed input_ids, labels, and cumulative_len.
+ """
+ if self.dataset is None:
+ with open(self.path) as f:
+ self.dataset = json.load(f)
+
+ raw_data = self.dataset[self.sampled[item]]
+
+ if self.tokenize_fn:
+ tokenized_data = self.tokenize_fn(raw_data)
+ return tokenized_data
+ else:
+ return raw_data
diff --git a/xtuner/_lite/datasets/jsonl.py b/xtuner/_lite/datasets/jsonl.py
new file mode 100644
index 000000000..3bfc2c4bb
--- /dev/null
+++ b/xtuner/_lite/datasets/jsonl.py
@@ -0,0 +1,205 @@
+import hashlib
+import inspect
+import json
+import math
+import os
+import random
+from concurrent.futures import ProcessPoolExecutor
+from mmengine import mkdir_or_exist
+import numpy as np
+import torch
+from torch import distributed as dist
+from tqdm import tqdm
+from xtuner._lite import get_logger
+
+logger = get_logger()
+
+
+def calculate_jsonl_sha256(path):
+ with open(path, 'rb') as f:
+ file_hash = hashlib.sha256()
+ file_hash.update(f.read())
+ return file_hash.hexdigest()
+
+
+def calculate_tokenize_fn_sha256(tokenize_fn):
+ """Calculate SHA-256 hash for an instance method's source code."""
+ # Get the source code of the method
+ fn_source = inspect.getsource(tokenize_fn.__call__)
+ return hashlib.sha256(fn_source.encode('utf-8')).hexdigest()
+
+
+class JsonlDataset(torch.utils.data.Dataset):
+
+ def __init__(self,
+ path,
+ sample_ratio=1.0,
+ tokenize_fn=None,
+ cache_dir=None,
+ max_length=None,):
+ super().__init__()
+
+ self.tokenize_fn = tokenize_fn
+ self.path = path
+ self.tokenizer_workers = int(os.environ.get('XTUNER_TOKENIZE_WORKERS', 8))
+
+ if cache_dir:
+ if os.path.exists(cache_dir):
+ assert os.path.isdir(cache_dir)
+ else:
+ mkdir_or_exist(cache_dir)
+
+ file_hash = calculate_jsonl_sha256(path)
+ file_cache_dir = os.path.join(cache_dir, file_hash)
+
+ if file_hash not in os.listdir(cache_dir):
+ mkdir_or_exist(file_cache_dir)
+
+ if 'offsets.npy' in os.listdir(file_cache_dir):
+ _cached_file = os.path.join(file_cache_dir, 'offsets.npy')
+ offsets = np.load(_cached_file)
+ else:
+ offsets = self.count_offsets(file_cache_dir)
+
+ if self.tokenize_fn:
+ tok_hash = calculate_tokenize_fn_sha256(tokenize_fn)
+ tok_cache_dir = os.path.join(file_cache_dir, tok_hash)
+ if tok_hash not in os.listdir(file_cache_dir):
+ mkdir_or_exist(tok_cache_dir)
+
+ if 'num_tokens.npy' in os.listdir(tok_cache_dir):
+ _cached_file = os.path.join(tok_cache_dir,
+ 'num_tokens.npy')
+ num_tokens = np.load(_cached_file)
+ else:
+ num_tokens = self.count_tokens(offsets, tok_cache_dir)
+ else:
+ num_tokens = None
+
+ offsets = offsets
+ num_tokens = num_tokens
+
+ else:
+ offsets = self.count_offsets()
+ num_tokens = None
+ if max_length is not None:
+ assert self.tokenize_fn
+ num_tokens = self.count_tokens(offsets)
+
+ _sampled = [i for i in range(len(offsets))]
+
+ if max_length is not None:
+ assert isinstance(max_length, int)
+ _filtered = [x for i, x in enumerate(_sampled) if num_tokens[i] < max_length]
+
+ if len(_filtered) < len(_sampled):
+ missed_num = len(_sampled) - len(_filtered)
+ logger.warning(f"{path} has {missed_num} prompt length>{max_length}, discard.")
+
+ _sampled = _filtered
+
+ _target_num_samples = int(len(_sampled) * sample_ratio)
+ self.sampled = _sampled * int(sample_ratio)
+ self.sampled.extend(random.sample(_sampled, _target_num_samples - len(self.sampled)))
+
+ if num_tokens is not None:
+ num_tokens = num_tokens[self.sampled]
+
+ self.num_tokens = num_tokens
+ self.offsets = offsets[self.sampled]
+
+
+ def count_offsets(self, cache_dir=None):
+
+ offsets = [0]
+ with open(self.path) as f:
+
+ lines = f.readlines()
+ for line in lines[:-1]:
+ offsets.append(offsets[-1]+len(line.encode()))
+
+ offsets = np.array(offsets)
+
+ if dist.get_rank() == 0 and cache_dir:
+ save_path = os.path.join(cache_dir, 'offsets.npy')
+ np.save(save_path, offsets)
+
+ return offsets
+
+ def _tokenize_by_offset(self, offset):
+
+ with open(self.path, 'r') as f:
+ f.seek(offset)
+ data = json.loads(f.readline())
+ return self.tokenize_fn(data)
+
+ def count_tokens(self, offsets, cache_dir=None):
+
+ num_samples = len(offsets)
+
+ if dist.is_available():
+ world_size = dist.get_world_size()
+ rank = dist.get_rank()
+ else:
+ world_size = 1
+ rank = 0
+
+ num_per_rank = math.ceil(num_samples / world_size)
+
+ start = rank * num_per_rank
+ end = (rank + 1) * num_per_rank
+ offsets_shard = offsets[start:end]
+
+
+ desc = f'[Rank {rank}] {self.path}'
+ chunk_size = min(1024, max(1, len(offsets_shard) // self.tokenizer_workers))
+
+ with ProcessPoolExecutor(max_workers=self.tokenizer_workers) as executor:
+ tokenized = list(
+ tqdm(
+ executor.map(
+ self._tokenize_by_offset,
+ offsets_shard,
+ chunksize=chunk_size),
+ desc=desc,
+ total=len(offsets_shard)))
+
+ _num_tokens = [data['num_tokens'] for data in tokenized]
+ _num_tokens = np.array(_num_tokens)
+
+ if dist.is_available():
+ num_tokens = [None] * world_size
+ dist.all_gather_object(num_tokens, _num_tokens)
+ num_tokens = np.concatenate(num_tokens, axis=0)
+ else:
+ num_tokens = _num_tokens
+
+ if rank == 0 and cache_dir:
+ save_path = os.path.join(cache_dir, 'num_tokens.npy')
+ np.save(save_path, num_tokens)
+
+ return num_tokens
+
+ def __len__(self):
+ return len(self.offsets)
+
+ def __getitem__(self, item):
+ """Returns a dict containing packed data in the given item.
+
+ Args:
+ item: An index to retrieve packed data.
+
+ Returns:
+ A dict including packed input_ids, labels, and cumulative_len.
+ """
+ with open(self.path, 'r') as f:
+ f.seek(self.offsets[item])
+ line = f.readline()
+
+ raw_data = json.loads(line)
+
+ if self.tokenize_fn:
+ tokenized_data = self.tokenize_fn(raw_data)
+ return tokenized_data
+ else:
+ return raw_data
diff --git a/xtuner/_lite/datasets/pack.py b/xtuner/_lite/datasets/pack.py
new file mode 100644
index 000000000..2cbfce863
--- /dev/null
+++ b/xtuner/_lite/datasets/pack.py
@@ -0,0 +1,267 @@
+import random
+
+import numpy as np
+import torch
+from datasets import Dataset, concatenate_datasets
+from torch.utils.data import ConcatDataset
+import bisect
+import itertools
+
+
+class SoftPackDataset(torch.utils.data.Dataset):
+
+ def __init__(self, datasets, target=2048, blend=False, sort=False):
+
+ if blend:
+ num_tokens = [
+ np.concatenate([dset.num_tokens for dset in datasets])
+ ]
+ datasets = [ConcatDataset(datasets)]
+ else:
+ num_tokens = [dset.num_tokens for dset in datasets]
+ self.datasets = datasets
+ self.target = target
+
+ pack_infos = []
+ for i, dataset in enumerate(self.datasets):
+ _infos = self.get_pack_infos(dataset, i, num_tokens[i])
+ pack_infos.append(_infos)
+ self.pack_infos = concatenate_datasets(pack_infos)
+
+ @property
+ def longest(self):
+ return self.pack_infos['longest']
+
+ def get_pack_infos(self, dataset, dataset_id, num_tokens):
+ # _ori_lens = dataset['num_tokens']
+ inds = [i for i in range(len(dataset))]
+ random.shuffle(inds)
+
+ item_buffer = []
+ length_buffer = []
+ longest = 0
+
+ pack_infos = []
+ for shfl_i in inds:
+ if num_tokens[shfl_i] + sum(length_buffer) <= self.target:
+ item_buffer.append(shfl_i)
+ length_buffer.append(num_tokens[shfl_i])
+ longest = max(longest, num_tokens[shfl_i])
+ else:
+ if len(item_buffer) > 0:
+ info = {
+ 'dataset_id': dataset_id,
+ 'indices': item_buffer,
+ 'longest': int(longest)
+ }
+ pack_infos.append(info)
+
+ item_buffer = [shfl_i]
+ length_buffer = [num_tokens[shfl_i]]
+ longest = num_tokens[shfl_i]
+
+ if len(item_buffer) > 0:
+ info = {
+ 'dataset_id': dataset_id,
+ 'indices': item_buffer,
+ 'longest': int(longest)
+ }
+
+ pack_infos.append(info)
+
+ pack_infos = Dataset.from_list(pack_infos)
+
+ return pack_infos
+
+ def __len__(self):
+ return len(self.pack_infos)
+
+ def __getitem__(self, item):
+ indices = self.pack_infos[item]['indices']
+ dataset_id = self.pack_infos[item]['dataset_id']
+ return [self.datasets[dataset_id][i] for i in indices]
+
+
+class HardPackDataset(torch.utils.data.Dataset):
+
+
+ def __init__(self, datasets, target=2048, blend=True, sort=False):
+
+ if blend:
+ num_tokens = [
+ np.concatenate([dset.num_tokens for dset in datasets])
+ ]
+ datasets = [ConcatDataset(datasets)]
+ else:
+ num_tokens = [dset.num_tokens for dset in datasets]
+ self.datasets = datasets
+ self.target = target
+
+ pack_infos = []
+ for i, dataset in enumerate(self.datasets):
+ _info = self.get_pack_info(dataset, i, num_tokens[i])
+ pack_infos.append(_info)
+
+ _ranges_left = []
+ _ranges_right = []
+ _num_packed_samples = []
+ _indices = []
+ _max_length_per_pack = []
+ _dataset_id = []
+ for info in pack_infos:
+ _ranges_left.extend(info['ranges_left'])
+ _ranges_right.extend(info['ranges_right'])
+ _num_packed_samples.append(info['num_packed_samples'])
+ _indices.extend(info['indices'])
+ _max_length_per_pack.extend(info['max_length_per_pack'])
+ _dataset_id.extend(info['dataset_id'])
+
+ self.pack_infos = {
+ 'ranges_left': _ranges_left,
+ 'ranges_right': _ranges_right,
+ 'num_packed_samples': _num_packed_samples,
+ 'indices': _indices,
+ 'max_length_per_pack':_max_length_per_pack,
+ 'dataset_id': _dataset_id
+ }
+
+
+ @classmethod
+ def _cal_max_length(cls, begin, end, shfl_item_rngs_left,
+ shfl_item_rngs_right):
+ left = bisect.bisect(shfl_item_rngs_right, begin)
+ right = bisect.bisect(shfl_item_rngs_left, end)
+ max_length = 0
+ for i in range(left, right):
+ item_begin = shfl_item_rngs_left[i]
+ item_end = shfl_item_rngs_right[i]
+ inner_l = max(begin, item_begin) - item_begin
+ inner_r = min(end, item_end) - item_begin
+ trunc_size = inner_r - inner_l
+ max_length = max(max_length, trunc_size)
+ return max_length
+
+ def get_pack_info(self, dataset, dataset_id, num_tokens):
+
+ # The number of data items after packing
+ num_packed_samples = int(num_tokens.sum() / self.target)
+
+ # Shuffle the order of the original dataset
+ # The packing will proceed according to the order after shuffle.
+ # Assume the following conditions hold:
+ # (1) shfl_inds = [3, 1, 2, 0]
+ # (2) self._ori_lens[3] + self._ori_lens[1] = max_length
+ # (3) self._ori_lens[2] + self._ori_lens[0] = max_length
+ # Ultimately, dataset[3] and dataset[1] will be combined into a new
+ # data, and dataset[2] and dataset[0] will be combined into a new data.
+ inds = [i for i in range(len(dataset))]
+ # if seed is not None:
+ # random.seed(seed)
+ random.shuffle(inds)
+ shfl_inds = inds
+
+ # shuffled cumulative lengths
+ shfl_lens = [num_tokens[i] for i in shfl_inds]
+ shfl_acc_lens = list(itertools.accumulate(shfl_lens))
+
+ shfl_item_rngs_left = [0] + shfl_acc_lens[:-1]
+ shfl_item_rngs_right = shfl_acc_lens
+
+ max_length_per_pack = []
+ belong_dataset_ids = []
+ for i in range(num_packed_samples):
+ begin = i * self.target
+ end = (i + 1) * self.target
+ max_length_per_pack.append(
+ self._cal_max_length(begin, end, shfl_item_rngs_left,
+ shfl_item_rngs_right))
+ belong_dataset_ids.append(dataset_id)
+
+ pack_infos = {
+ 'ranges_left': shfl_item_rngs_left,
+ 'ranges_right': shfl_item_rngs_right,
+ 'num_packed_samples': num_packed_samples,
+ 'indices': shfl_inds,
+ 'dataset_id': belong_dataset_ids,
+ 'max_length_per_pack': max_length_per_pack
+ }
+
+ # pack_infos = Dataset.from_list(pack_infos)
+
+ return pack_infos
+
+ def _pack_ids_and_labels_in_range(self, begin: int, end: int):
+ """Packs ids and labels in a given range using bisection method.
+
+ Args:
+ begin: Index indicating the beginning of the range.
+ end: Index indicating the end of the range.
+
+ Returns:
+ A tuple containing packed ids, labels, and cumulative lengths.
+ """
+
+ # Use binary search to find dataset positions that fall within begin
+ # and end range
+ left = bisect.bisect(self.pack_infos['ranges_left'], begin)
+ right = bisect.bisect(self.pack_infos['ranges_right'], end)
+
+ trunc_input_ids = []
+ trunc_labels = []
+ trunc_sizes = []
+
+ for i in range(left, right):
+
+ # Determine the real range we will cut in current original item
+ item_begin = self.pack_infos['ranges_left'][i]
+ item_end = self.pack_infos['ranges_right'][i]
+
+ # Calculate exact positions within current dataset item
+ inner_l = max(begin, item_begin) - item_begin
+ inner_r = min(end, item_end) - item_begin
+
+ # Get original data and labels
+ ori_idx = self.pack_infos['indices'][i]
+ ori_dataset_id = self.pack_infos['dataset_id'][i]
+ ori_input_ids = self.datasets[ori_dataset_id][ori_idx]['input_ids']
+ ori_labels = self.datasets[ori_dataset_id][ori_idx]['labels']
+
+ # Add original data and labels from calculated positions
+ # to trunc_ids and trunc_labels
+ trunc_input_ids.extend(ori_input_ids[inner_l:inner_r])
+ trunc_labels.extend(ori_labels[inner_l:inner_r])
+ trunc_sizes.append(inner_r - inner_l)
+
+ # return populated lists of truncated ids, labels and their cumulative
+ # lengths
+ return trunc_input_ids, trunc_labels, trunc_sizes
+
+ def __len__(self):
+ return len(self.pack_infos['indices'])
+
+ def __getitem__(self, item):
+ """Returns a dict containing packed data in the given item.
+
+ Args:
+ item: An index to retrieve packed data.
+
+ Returns:
+ A dict including packed input_ids, labels, and cumulative_len.
+ """
+ # The cumulative length from the start position of this data
+ begin = item * self.target
+ # The cumulative length from the end position of this data
+ end = (item + 1) * self.target
+
+ # Extract data within the range from the shuffled original dataset.
+ _res = self._pack_ids_and_labels_in_range(begin, end)
+ packed_input_ids, packed_labels, num_tokens = _res
+ assert self.target == len(packed_input_ids) == len(packed_labels)
+
+ packed = {
+ 'input_ids': packed_input_ids,
+ 'labels': packed_labels,
+ 'num_tokens': num_tokens,
+ }
+
+ return packed
\ No newline at end of file
diff --git a/xtuner/_lite/datasets/streaming.py b/xtuner/_lite/datasets/streaming.py
new file mode 100644
index 000000000..bb567e780
--- /dev/null
+++ b/xtuner/_lite/datasets/streaming.py
@@ -0,0 +1,159 @@
+import json
+
+import numpy as np
+from torch.utils.data import IterableDataset
+
+
+class Streaming:
+
+ def __init__(self, file, max_epoch=1):
+ self.file = file
+ self.offset = 0
+ self.epoch = 1
+ self.max_epoch = max_epoch
+
+ def __iter__(self):
+ return self
+
+ def __next__(self):
+
+ with open(self.file) as f:
+ f.seek(self.offset)
+ line = f.readline()
+
+ if not line and self.epoch < self.max_epoch:
+ self.offset = 0
+ self.epoch += 1
+ return next(self)
+
+ elif not line and self.epoch == self.max_epoch:
+ raise StopIteration
+
+ self.offset = f.tell()
+ return line
+
+
+# import torch
+
+# class MultiStreamingDataset(torch.utils.data.IterableDataset):
+
+# def __init__(self, streamings, weights, max_length, tokenize_fn, seed, dp_rank, dp_world_size, crossover = False):
+
+# assert len(streamings) == len(weights)
+# self.streamings = streamings
+# self.activated = [True for _ in self.streamings]
+# for sid, stream in enumerate(self.streamings):
+# stream.offset = 0
+# try:
+# for _ in range(self.dp_rank):
+# next(stream)
+# except StopIteration:
+# self.activated[sid] = False
+
+# self.random_state = np.random.RandomState(seed + dp_rank)
+# self.weights = weights
+
+# self.max_length = max_length
+# self.tokenize_fn = tokenize_fn
+# self.dp_rank = dp_rank
+# self.dp_world_size = dp_world_size
+# self.crossover = crossover
+
+# def reactivate(self):
+# self.activated = [True for _ in self.streamings]
+# for stream in self.streamings:
+# stream.offset = 0
+# for _ in range(self.dp_rank):
+# next(stream)
+
+# @property
+# def probabilities(self):
+# if sum(self.activated) == 0:
+# self.reactivate()
+
+# probs = (np.array(self.weights) * self.activated) / sum(self.weights[self.activated])
+# return probs
+
+# @property
+# def num_streamings(self):
+# assert len(self.iterators) == len(self.weights)
+# return len(self.weights)
+
+# def per_rank_next(self, streaming_id):
+
+# sid = streaming_id
+# streaming = self.streamings[sid]
+
+# try:
+# data = next(streaming)
+# except StopIteration:
+# self.activated[sid] = False
+# sid = self.random_state.choice(
+# self.num_streamings, p=self.probabilities)
+# return self.per_rank_next(sid)
+
+# try:
+# for _ in range(self.dp_world_size):
+# next(streaming)
+# except StopIteration:
+# self.activated[sid] = False
+
+# return data, sid
+
+# def __iter__(self):
+# worker_info = torch.utils.data.get_worker_info()
+
+# if worker_info and worker_info.num_workers > 1:
+# raise NotImplementedError
+
+# input_ids = []
+# labels = []
+# num_tokens = []
+# while True:
+# sid = self.random_state.choice(
+# self.num_streamings, p=self.probabilities)
+
+# while len(input_ids) < self.max_length:
+# if self.crossover:
+# sid = self.random_state.choice(
+# self.num_streamings, p=self.probabilities)
+
+# line, sid = self.per_rank_next(sid)
+
+# tokenized = self.tokenize_fn(json.loads(line))
+
+# input_ids.extend(tokenized['input_ids'])
+# labels.extend(tokenized['labels'])
+# num_tokens.extend(tokenized['num_tokens'])
+
+# remain_tokens = max(sum(num_tokens) - self.max_length, 0)
+# num_tokens[-1] = num_tokens[-1] - remain_tokens
+
+# packed_ids = input_ids[:self.max_length]
+# packed_labels = labels[:self.max_length]
+# packed_tokens = num_tokens
+
+# if remain_tokens:
+# input_ids = input_ids[self.max_length:]
+# labels = labels[self.max_length:]
+# num_tokens = [remain_tokens]
+
+# yield {'input_ids': packed_ids, 'labels': packed_labels, 'num_tokens': packed_tokens}
+
+if __name__ == '__main__':
+ import json
+ streaming = Streaming(
+ '/mnt/hwfile/xtuner/huanghaian/data/databricks-dolly-15k/databricks-dolly-15k.jsonl'
+ )
+
+ data = next(streaming)
+ print(json.loads(data))
+
+ data = next(streaming)
+ print(json.loads(data))
+
+ data = next(streaming)
+ print(json.loads(data))
+
+ data = next(streaming)
+ print(json.loads(data))
diff --git a/xtuner/_lite/datasets/utils/__init__.py b/xtuner/_lite/datasets/utils/__init__.py
new file mode 100644
index 000000000..d7d345220
--- /dev/null
+++ b/xtuner/_lite/datasets/utils/__init__.py
@@ -0,0 +1,7 @@
+from .convert import OPENAI_CONVERT_MAP
+from .load import DATASET_CLS_MAP, load_datasets
+from .utils import apply_exif_orientation, move_data_to_device
+
+
+__all__ = ['OPENAI_CONVERT_MAP', 'DATASET_CLS_MAP', 'load_datasets',
+ 'apply_exif_orientation', 'move_data_to_device']
diff --git a/xtuner/_lite/datasets/utils/convert.py b/xtuner/_lite/datasets/utils/convert.py
new file mode 100644
index 000000000..b78a12d51
--- /dev/null
+++ b/xtuner/_lite/datasets/utils/convert.py
@@ -0,0 +1,234 @@
+import re
+
+from xtuner._lite.chat import ChatMessages
+
+
+class XTunerFormat2Openai():
+
+ @classmethod
+ def source_format(cls):
+ data = {
+ 'conversation': [{
+ 'system': 'SYSTEM',
+ 'input': 'INPUT',
+ 'output': 'OUTPUT'
+ }, {
+ 'input': 'INPUT',
+ 'output': 'OUTPUT'
+ }]
+ }
+ return data
+
+ @classmethod
+ def target_format(cls):
+ data = {
+ 'messages': [
+ {
+ 'role': 'system',
+ 'content': 'SYSTEM'
+ },
+ {
+ 'role': 'user',
+ 'content': 'INPUT'
+ },
+ {
+ 'role': 'assistant',
+ 'content': 'OUTPUT'
+ },
+ {
+ 'role': 'user',
+ 'content': 'INPUT'
+ },
+ {
+ 'role': 'assistant',
+ 'content': 'OUTPUT'
+ },
+ ]
+ }
+ return data
+
+ @staticmethod
+ def convert(data):
+ ROLE_MAPPING = {
+ 'system': 'system',
+ 'input': 'user',
+ 'output': 'assistant'
+ }
+ messages = []
+ for single_turn_conversation in data['conversation']:
+ for role, content in single_turn_conversation.items():
+ messages.append({
+ 'role': ROLE_MAPPING[role],
+ 'content': content
+ })
+ return ChatMessages.from_dict({'messages': messages})
+
+
+class Alpaca2Openai():
+
+ @classmethod
+ def source_format(cls):
+ data = {
+ 'instruction': 'INSTRUCTION',
+ 'input': 'INPUT',
+ 'output': 'OUTPUT',
+ }
+ return data
+
+ @classmethod
+ def target_format(cls):
+ data = {
+ 'messages': [
+ {
+ 'role': 'user',
+ 'content': 'INSTRUCTION\nINPUT'
+ },
+ {
+ 'role': 'assistant',
+ 'content': 'OUTPUT'
+ },
+ ]
+ }
+ return data
+
+ @staticmethod
+ def convert(data):
+ if data.get('output') == '':
+ return ChatMessages.from_dict({'messages': []})
+ else:
+ return ChatMessages.from_dict({
+ 'messages': [
+ {
+ 'role': 'user',
+ 'content': f"{data['instruction']}\n{data['input']}"
+ },
+ {
+ 'role': 'assistant',
+ 'content': f"{data['output']}"
+ },
+ ]
+ })
+
+
+def llava_to_openai(data):
+
+ image_token = ''
+ conversations = data['conversations']
+ messages = []
+
+ if 'image' in data:
+ image_urls = data['image']
+ if isinstance(image_urls, str):
+ image_urls = [image_urls]
+ else:
+ image_urls = None
+
+ while conversations and conversations[0]['from'] == 'gpt':
+ # Skip the first one if it is from gpt
+ conversations = conversations[1:]
+
+ image_id = 0
+ for convs in conversations:
+ if convs['from'] == 'human':
+ pattern = f'({image_token})'
+ chunks = re.split(pattern, convs['value'])
+
+ text_content = []
+ img_content = []
+
+ for chunk in chunks:
+ if chunk == image_token:
+ url = image_urls[image_id]
+ if not isinstance(url, str):
+ raise TypeError(data)
+ # assert , image_url
+ item = dict(type='image_url', image_url=url)
+ img_content.append(item)
+ image_id += 1
+ elif len(chunk.strip()):
+ item = dict(type='text', text=chunk.strip())
+ text_content.append(item)
+
+ msg = {'role': 'user', 'content': img_content + text_content}
+ messages.append(msg)
+
+ elif convs['from'] == 'gpt':
+ msg = {'role': 'assistant', 'content': convs['value']}
+ messages.append(msg)
+ else:
+ raise NotImplementedError
+
+ return ChatMessages.from_dict({'messages': messages})
+
+
+def llava_to_openai_interleave(data):
+
+ image_token = ''
+ conversations = data['conversations']
+ messages = []
+
+ if 'image' in data:
+ image_urls = data['image']
+ if isinstance(image_urls, str):
+ image_urls = [image_urls]
+ else:
+ image_urls = None
+
+ while conversations and conversations[0]['from'] == 'gpt':
+ # Skip the first one if it is from gpt
+ conversations = conversations[1:]
+
+ image_id = 0
+ for convs in conversations:
+ if convs['from'] == 'human':
+ pattern = f'({image_token})'
+ chunks = re.split(pattern, convs['value'])
+
+ content = []
+
+ for chunk in chunks:
+ if chunk == image_token:
+ url = image_urls[image_id]
+ if not isinstance(url, str):
+ raise TypeError(data)
+ # assert , image_url
+ item = dict(type='image_url', image_url=url)
+ content.append(item)
+ image_id += 1
+ elif len(chunk.strip()):
+ item = dict(type='text', text=chunk.strip())
+ content.append(item)
+
+ msg = {'role': 'user', 'content': content}
+ messages.append(msg)
+
+ elif convs['from'] == 'gpt':
+ msg = {'role': 'assistant', 'content': convs['value']}
+ messages.append(msg)
+ else:
+ raise NotImplementedError
+
+ return ChatMessages.from_dict({'messages': messages})
+
+
+def official_openai(data):
+ if 'messages' in data:
+ return ChatMessages.from_dict(data)
+ elif 'message_data' in data:
+ return ChatMessages.from_dict({'messages': data['message_data']})
+ elif 'dialogs' in data:
+ return ChatMessages.from_dict({'messages': data['dialogs']})
+ else:
+ return ChatMessages.from_dict({'messages': data})
+
+OPENAI_CONVERT_MAP = {
+ 'llava':
+ llava_to_openai,
+ 'llava_interleave':
+ llava_to_openai_interleave,
+ 'alpaca':
+ Alpaca2Openai.convert,
+ 'xtuner':
+ XTunerFormat2Openai.convert,
+ 'openai': official_openai,
+}
diff --git a/xtuner/_lite/datasets/utils/load.py b/xtuner/_lite/datasets/utils/load.py
new file mode 100644
index 000000000..7a39f3c75
--- /dev/null
+++ b/xtuner/_lite/datasets/utils/load.py
@@ -0,0 +1,279 @@
+# Copyright (c) OpenMMLab. All rights reserved.
+import json
+import math
+import os
+import random
+import re
+
+from torch import distributed as dist
+from tqdm import tqdm
+
+from xtuner._lite import get_logger
+from ..json import JsonDataset
+from ..jsonl import JsonlDataset
+
+logger = get_logger()
+
+DATASET_CLS_MAP = {'.jsonl': JsonlDataset, '.json': JsonDataset}
+
+
+def load_hf_dataset(path,
+ split='train',
+ sample_ratio=1.0,
+ cache_dir=None,
+ map_fn=None):
+ from datasets import load_dataset
+ dataset = load_dataset(path)[split]
+
+ if map_fn:
+ dataset = dataset.map(map_fn, num_proc=8)
+
+ if sample_ratio != 1:
+ ori_samples = len(dataset)
+ target_samples = int(sample_ratio * ori_samples)
+ indices = random.choices([i for i in range(ori_samples)],
+ k=target_samples)
+ dataset = dataset.select(indices)
+
+ dataset = dataset.to_list()
+
+ # if init_fn:
+ # dataset = init_fn(dataset)
+
+ # if cache_dir and isinstance(dataset, CacheDataset):
+ # dataset.cache(cache_dir)
+
+ return dataset
+
+
+def load_from_cache(cache_dir, init_fn):
+
+ if dist.is_available():
+ world_size = dist.get_world_size()
+ rank = dist.get_rank()
+ else:
+ world_size = 1
+ rank = 0
+
+ sub_cache_dirs = []
+ for _path in tqdm(os.listdir(cache_dir)):
+ path = os.path.join(cache_dir, _path)
+ if os.path.isdir(path):
+ sub_cache_dirs.append(path)
+
+ num_dsets = len(sub_cache_dirs)
+ avg_num = math.ceil(num_dsets / world_size)
+ start = rank * avg_num
+ end = min((rank + 1) * avg_num, num_dsets)
+ desc = f'[Rank {rank}] Loading Cached Dataset'
+
+ rank_datasets = []
+ for ind in tqdm(range(start, end), desc=desc):
+ dset = init_fn(sub_cache_dirs[ind])
+ rank_datasets.append(dset)
+
+ if dist.is_available() and world_size > 1:
+ dist.barrier()
+ buffers = [None] * world_size
+ dist.all_gather_object(buffers, rank_datasets)
+ world_datasets = []
+ for dsets_per_rank in buffers:
+ world_datasets.extend(dsets_per_rank)
+
+ assert len(world_datasets) == num_dsets
+ else:
+ world_datasets = rank_datasets
+
+ return world_datasets
+
+
+def load_local_datasets(paths,
+ file_types,
+ file_pattern=None,
+ cache_dir=None,
+ sample_ratios=1.0,
+ map_fns=None,
+ max_length=None):
+
+ if isinstance(paths, str):
+ paths = [paths]
+
+ if isinstance(sample_ratios, (tuple, list)):
+
+ if len(sample_ratios) == 1:
+ sample_ratios = list(sample_ratios) * len(paths)
+
+ if len(sample_ratios) != len(paths):
+ raise RuntimeError(f'There are {len(paths)} paths, but only '
+ f'{len(sample_ratios)} sample ratios were set.')
+
+ if map_fns is None:
+ map_fns = [None] * len(paths)
+
+ if isinstance(map_fns, (tuple, list)):
+
+ if len(map_fns) == 1:
+ map_fns = list(map_fns) * len(paths)
+
+ if len(map_fns) != len(paths):
+ raise RuntimeError(f'There are {len(paths)} paths, but only'
+ f'{len(map_fns)} map fns were set.')
+
+ files = []
+ file_sample_ratios = []
+ file_map_fns = []
+
+ for pid, path in enumerate(paths):
+ if os.path.isdir(path):
+ dir_files = []
+ for root, dirs, _files in os.walk(path, followlinks=True):
+ dirs.sort()
+ for relative_path in sorted(_files):
+ suffix = os.path.splitext(relative_path)[-1]
+ absolute_path = os.path.join(root, relative_path)
+ if file_pattern is not None:
+ if bool(re.match(file_pattern, absolute_path)):
+ dir_files.append(absolute_path)
+ elif suffix in file_types:
+ dir_files.append(absolute_path)
+
+ _num_dir_files = len(dir_files)
+ if _num_dir_files == 0:
+ raise RuntimeError(
+ f'There are no files with the suffix {file_types}'
+ f'in `{path}`.')
+
+ logger.info(f'Found {len(dir_files)} files in {path}')
+ files.extend(dir_files)
+ file_sample_ratios.extend([sample_ratios[pid]] * _num_dir_files)
+ file_map_fns.extend([map_fns[pid]] * _num_dir_files)
+
+ elif os.path.isfile(path):
+ files.append(path)
+ file_sample_ratios.append(sample_ratios[pid])
+ file_map_fns.append(map_fns[pid])
+
+ else:
+ raise RuntimeError(f'`{path}` not found.')
+
+ num_files = len(files)
+
+ datasets = []
+ for i in range(num_files):
+ _path = files[i]
+ _ratio = file_sample_ratios[i]
+ _map_fn = file_map_fns[i]
+ _suffix = os.path.splitext(_path)[-1]
+
+ dataset_cls = DATASET_CLS_MAP[_suffix]
+ _dataset = dataset_cls(_path, _ratio, _map_fn, cache_dir, max_length)
+ datasets.append(_dataset)
+
+ return datasets
+
+
+def load_datasets(paths,
+ sources='local',
+ sample_ratios=1.0,
+ file_types=DATASET_CLS_MAP.keys(),
+ file_pattern=None,
+ cache_dir=None,
+ map_fns=None,
+ max_length=None):
+
+ if isinstance(paths, str):
+ paths = [paths]
+
+ num_paths = len(paths)
+
+ if isinstance(sample_ratios, (float, int)):
+ sample_ratios = [sample_ratios] * num_paths
+
+ if isinstance(sample_ratios, (tuple, list)):
+
+ if len(sample_ratios) == 1:
+ sample_ratios = list(sample_ratios) * num_paths
+
+ if len(sample_ratios) != num_paths:
+ raise RuntimeError(f'There are {num_paths} paths, but only '
+ f'{len(sample_ratios)} sample ratios were set.')
+
+ if isinstance(sources, str):
+ sources = [sources]
+
+ if isinstance(sources, (tuple, list)):
+
+ if len(sources) == 1:
+ sources = list(sources) * num_paths
+
+ if len(sources) != num_paths:
+ raise RuntimeError(f'There are {num_paths} paths, but only '
+ f'{len(sources)} sources were set.')
+
+ if not isinstance(map_fns, (tuple, list)):
+ map_fns = [map_fns] * num_paths
+
+ if isinstance(map_fns, (tuple, list)):
+
+ if len(map_fns) == 1:
+ map_fns = list(map_fns) * num_paths
+
+ if len(map_fns) != num_paths:
+ raise RuntimeError(f'There are {num_paths} paths, but only'
+ f'{len(map_fns)} map fns were set.')
+
+ local_inds = [i for i, src in enumerate(sources) if src == 'local']
+ local_paths = [paths[ind] for ind in local_inds]
+ local_map_fns = [map_fns[ind] for ind in local_inds]
+ local_sample_ratios = [sample_ratios[ind] for ind in local_inds]
+
+ hf_inds = [i for i, src in enumerate(sources) if src == 'huggingface']
+ hf_paths = [paths[ind] for ind in hf_inds]
+ hf_map_fns = [map_fns[ind] for ind in hf_inds]
+ hf_sample_ratios = [sample_ratios[ind] for ind in hf_inds]
+
+ datasets = []
+ if len(local_inds):
+ local_datasets = load_local_datasets(local_paths, file_types,
+ file_pattern, cache_dir,
+ local_sample_ratios,
+ local_map_fns, max_length)
+ datasets.extend(local_datasets)
+
+ if len(hf_inds):
+ cached_infos = {}
+ for i in range(len(hf_inds)):
+ if cache_dir:
+ digits = len(str(abs(len(hf_inds))))
+ cache_id = (f'cache-hf-{i+1:0{digits}}-of-'
+ f'{len(hf_inds):0{digits}}')
+ sub_cache_dir = os.path.join(cache_dir, cache_id)
+ else:
+ sub_cache_dir = None
+ dset = load_hf_dataset(
+ hf_paths[i],
+ sample_ratio=hf_sample_ratios[i],
+ map_fn=hf_map_fns[i],
+ cache_dir=sub_cache_dir,
+ max_length=max_length)
+ datasets.append(dset)
+ breakpoint()
+ if cache_dir:
+
+ infos = {
+ 'path': hf_paths[i],
+ 'num_samples': dset.num_samples,
+ 'num_tokens': dset.total_tokens
+ }
+ cached_infos[cache_id] = infos
+
+ if cache_dir:
+ _path = os.path.join(cache_dir, 'hf_infos.json')
+ with open(_path, 'w') as f:
+ json.dump(cached_infos, f)
+
+ return datasets
+
+
+def load_ms_dataset():
+ pass
diff --git a/xtuner/_lite/datasets/utils/utils.py b/xtuner/_lite/datasets/utils/utils.py
new file mode 100644
index 000000000..19f572ac0
--- /dev/null
+++ b/xtuner/_lite/datasets/utils/utils.py
@@ -0,0 +1,66 @@
+from PIL import Image
+import torch
+from collections.abc import Mapping
+
+_EXIF_ORIENT = 274 # exif 'Orientation' tag
+
+
+def apply_exif_orientation(image):
+ """
+ Applies the exif orientation correctly.
+
+ This code exists per the bug:
+ https://github.com/python-pillow/Pillow/issues/3973
+ with the function `ImageOps.exif_transpose`. The Pillow source raises errors with
+ various methods, especially `tobytes`
+
+ Function based on:
+ https://github.com/wkentaro/labelme/blob/v4.5.4/labelme/utils/image.py#L59
+ https://github.com/python-pillow/Pillow/blob/7.1.2/src/PIL/ImageOps.py#L527
+
+ Args:
+ image (PIL.Image): a PIL image
+
+ Returns:
+ (PIL.Image): the PIL image with exif orientation applied, if applicable
+ """
+ if not hasattr(image, "getexif"):
+ return image
+
+ try:
+ exif = image.getexif()
+ except Exception: # https://github.com/facebookresearch/detectron2/issues/1885
+ exif = None
+
+ if exif is None:
+ return image
+
+ orientation = exif.get(_EXIF_ORIENT)
+
+ method = {
+ 2: Image.FLIP_LEFT_RIGHT,
+ 3: Image.ROTATE_180,
+ 4: Image.FLIP_TOP_BOTTOM,
+ 5: Image.TRANSPOSE,
+ 6: Image.ROTATE_270,
+ 7: Image.TRANSVERSE,
+ 8: Image.ROTATE_90,
+ }.get(orientation)
+
+ if method is not None:
+ return image.transpose(method)
+ return image
+
+
+def move_data_to_device(data, device='cuda'):
+ """
+ Prepares one `data` before feeding it to the model, be it a tensor or a nested list/dictionary of tensors.
+ """
+ if isinstance(data, Mapping):
+ return type(data)({k: move_data_to_device(v) for k, v in data.items()})
+ elif isinstance(data, (tuple, list)):
+ return type(data)(move_data_to_device(v) for v in data)
+ elif isinstance(data, torch.Tensor):
+ kwargs = {"device": device}
+ return data.to(non_blocking=True, **kwargs)
+ return data
diff --git a/xtuner/_lite/device.py b/xtuner/_lite/device.py
new file mode 100644
index 000000000..5ea8a7924
--- /dev/null
+++ b/xtuner/_lite/device.py
@@ -0,0 +1,41 @@
+import torch
+
+
+def get_device():
+ device = None
+ if torch.cuda.is_available():
+ device = 'cuda'
+ else:
+ try:
+ import torch_npu # noqa: F401
+ device = 'npu'
+ except ImportError:
+ pass
+ try:
+ import torch_mlu # noqa: F401
+ device = 'mlu'
+ except ImportError:
+ pass
+
+ if device is None:
+ raise NotImplementedError(
+ 'Supports only CUDA or NPU. If your device is CUDA or NPU, '
+ 'please make sure that your environmental settings are '
+ 'configured correctly.')
+
+ return device
+
+
+def get_torch_device_module():
+
+ device = get_device()
+ if device == 'cuda':
+ return torch.cuda
+ elif device == 'npu':
+ return torch.npu
+ elif device == 'mlu':
+ return torch.mlu
+ else:
+ raise NotImplementedError
+
+
diff --git a/xtuner/_lite/modelings/__init__.py b/xtuner/_lite/modelings/__init__.py
new file mode 100644
index 000000000..1025f4087
--- /dev/null
+++ b/xtuner/_lite/modelings/__init__.py
@@ -0,0 +1,17 @@
+from .internlm2 import InternLM2Config, InternLM2ForCausalLM
+from .internlm3 import InternLM3Config, InternLM3ForCausalLM, InternLM3Tokenizer
+from .llava.modeling_llava import LlavaForConditionalGeneration
+from .llava.configuration_llava import EnhancedLlavaConfig
+from .llava.processing_llava import LlavaProcessor
+
+def register_remote_code():
+ from transformers import AutoConfig, AutoModelForCausalLM, AutoTokenizer
+ AutoConfig.register('internlm2', InternLM2Config, exist_ok=True)
+ AutoModelForCausalLM.register(
+ InternLM2Config, InternLM2ForCausalLM, exist_ok=True)
+
+ AutoConfig.register('internlm3', InternLM3Config, exist_ok=True)
+ AutoModelForCausalLM.register(
+ InternLM3Config, InternLM3ForCausalLM, exist_ok=True)
+ AutoTokenizer.register(
+ InternLM3Config, InternLM3Tokenizer, exist_ok=True)
diff --git a/xtuner/_lite/modelings/internlm2/__init__.py b/xtuner/_lite/modelings/internlm2/__init__.py
new file mode 100644
index 000000000..e43d72d4a
--- /dev/null
+++ b/xtuner/_lite/modelings/internlm2/__init__.py
@@ -0,0 +1,2 @@
+from .configuration_internlm2 import InternLM2Config
+from .modeling_internlm2 import InternLM2ForCausalLM
diff --git a/xtuner/_lite/modelings/internlm2/configuration_internlm2.py b/xtuner/_lite/modelings/internlm2/configuration_internlm2.py
new file mode 100644
index 000000000..8b8107947
--- /dev/null
+++ b/xtuner/_lite/modelings/internlm2/configuration_internlm2.py
@@ -0,0 +1,175 @@
+# Copyright (c) The InternLM team and The HuggingFace Inc. team. All rights reserved.
+#
+# This code is based on transformers/src/transformers/models/llama/configuration_llama.py
+#
+# Licensed under the Apache License, Version 2.0 (the "License");
+# you may not use this file except in compliance with the License.
+# You may obtain a copy of the License at
+#
+# http://www.apache.org/licenses/LICENSE-2.0
+#
+# Unless required by applicable law or agreed to in writing, software
+# distributed under the License is distributed on an "AS IS" BASIS,
+# WITHOUT WARRANTIES OR CONDITIONS OF ANY KIND, either express or implied.
+# See the License for the specific language governing permissions and
+# limitations under the License.
+""" InternLM2 model configuration"""
+
+from transformers.configuration_utils import PretrainedConfig
+from transformers.utils import logging
+
+logger = logging.get_logger(__name__)
+
+INTERNLM2_PRETRAINED_CONFIG_ARCHIVE_MAP = {}
+
+
+# Modified from transformers.model.llama.configuration_llama.LlamaConfig
+class InternLM2Config(PretrainedConfig):
+ r"""
+ This is the configuration class to store the configuration of a [`InternLM2Model`]. It is used to instantiate
+ an InternLM2 model according to the specified arguments, defining the model architecture. Instantiating a
+ configuration with the defaults will yield a similar configuration to that of the InternLM2-7B.
+ Configuration objects inherit from [`PretrainedConfig`] and can be used to control the model outputs. Read the
+ documentation from [`PretrainedConfig`] for more information.
+ Args:
+ vocab_size (`int`, *optional*, defaults to 32000):
+ Vocabulary size of the InternLM2 model. Defines the number of different tokens that can be represented by the
+ `inputs_ids` passed when calling [`InternLM2Model`]
+ hidden_size (`int`, *optional*, defaults to 4096):
+ Dimension of the hidden representations.
+ intermediate_size (`int`, *optional*, defaults to 11008):
+ Dimension of the MLP representations.
+ num_hidden_layers (`int`, *optional*, defaults to 32):
+ Number of hidden layers in the Transformer decoder.
+ num_attention_heads (`int`, *optional*, defaults to 32):
+ Number of attention heads for each attention layer in the Transformer decoder.
+ num_key_value_heads (`int`, *optional*):
+ This is the number of key_value heads that should be used to implement Grouped Query Attention. If
+ `num_key_value_heads=num_attention_heads`, the model will use Multi Head Attention (MHA), if
+ `num_key_value_heads=1 the model will use Multi Query Attention (MQA) otherwise GQA is used. When
+ converting a multi-head checkpoint to a GQA checkpoint, each group key and value head should be constructed
+ by meanpooling all the original heads within that group. For more details checkout [this
+ paper](https://arxiv.org/pdf/2305.13245.pdf). If it is not specified, will default to
+ `num_attention_heads`.
+ hidden_act (`str` or `function`, *optional*, defaults to `"silu"`):
+ The non-linear activation function (function or string) in the decoder.
+ max_position_embeddings (`int`, *optional*, defaults to 2048):
+ The maximum sequence length that this model might ever be used with. InternLM2 supports up to 32768 tokens.
+ initializer_range (`float`, *optional*, defaults to 0.02):
+ The standard deviation of the truncated_normal_initializer for initializing all weight matrices.
+ rms_norm_eps (`float`, *optional*, defaults to 1e-06):
+ The epsilon used by the rms normalization layers.
+ use_cache (`bool`, *optional*, defaults to `True`):
+ Whether or not the model should return the last key/values attentions (not used by all models). Only
+ relevant if `config.is_decoder=True`.
+ pad_token_id (`int`, *optional*):
+ Padding token id.
+ bos_token_id (`int`, *optional*, defaults to 1):
+ Beginning of stream token id.
+ eos_token_id (`int`, *optional*, defaults to 2):
+ End of stream token id.
+ pretraining_tp (`int`, *optional*, defaults to 1):
+ Experimental feature. Tensor parallelism rank used during pretraining. Please refer to [this
+ document](https://huggingface.co/docs/transformers/main/perf_train_gpu_many#tensor-parallelism)
+ to understand more about it. This value is necessary to ensure exact reproducibility
+ of the pretraining results. Please refer to [this
+ issue](https://github.com/pytorch/pytorch/issues/76232).
+ tie_word_embeddings (`bool`, *optional*, defaults to `False`):
+ Whether to tie weight embeddings
+ rope_theta (`float`, *optional*, defaults to 10000.0):
+ The base period of the RoPE embeddings.
+ rope_scaling (`Dict`, *optional*):
+ Dictionary containing the scaling configuration for the RoPE embeddings. Currently supports two scaling
+ strategies: linear and dynamic. Their scaling factor must be a float greater than 1. The expected format is
+ `{"type": strategy name, "factor": scaling factor}`. When using this flag, don't update
+ `max_position_embeddings` to the expected new maximum. See the following thread for more information on how
+ these scaling strategies behave:
+ https://www.reddit.com/r/LocalLLaMA/comments/14mrgpr/dynamically_scaled_rope_further_increases/. This is an
+ experimental feature, subject to breaking API changes in future versions.
+ """
+ _auto_class = 'AutoConfig'
+ model_type = 'internlm2'
+ keys_to_ignore_at_inference = ['past_key_values']
+
+ def __init__( # pylint: disable=W0102
+ self,
+ vocab_size=103168,
+ hidden_size=4096,
+ intermediate_size=11008,
+ num_hidden_layers=32,
+ num_attention_heads=32,
+ num_key_value_heads=None,
+ hidden_act='silu',
+ max_position_embeddings=2048,
+ initializer_range=0.02,
+ rms_norm_eps=1e-6,
+ use_cache=True,
+ pad_token_id=0,
+ bos_token_id=1,
+ eos_token_id=2,
+ pretraining_tp=1,
+ tie_word_embeddings=False,
+ bias=True,
+ rope_theta=10000,
+ rope_scaling=None,
+ attn_implementation=None,
+ **kwargs,
+ ):
+ self.vocab_size = vocab_size
+ self.max_position_embeddings = max_position_embeddings
+ self.hidden_size = hidden_size
+ self.intermediate_size = intermediate_size
+ self.num_hidden_layers = num_hidden_layers
+ self.num_attention_heads = num_attention_heads
+ self.bias = bias
+
+ if num_key_value_heads is None:
+ num_key_value_heads = num_attention_heads
+ self.num_key_value_heads = num_key_value_heads
+
+ self.hidden_act = hidden_act
+ self.initializer_range = initializer_range
+ self.rms_norm_eps = rms_norm_eps
+ self.pretraining_tp = pretraining_tp
+ self.use_cache = use_cache
+ self.rope_theta = rope_theta
+ self.rope_scaling = rope_scaling
+ self._rope_scaling_validation()
+ self.attn_implementation = attn_implementation
+ if self.attn_implementation is None:
+ self.attn_implementation = 'eager'
+
+ super().__init__(
+ pad_token_id=pad_token_id,
+ bos_token_id=bos_token_id,
+ eos_token_id=eos_token_id,
+ tie_word_embeddings=tie_word_embeddings,
+ **kwargs,
+ )
+
+ def _rope_scaling_validation(self):
+ """
+ Validate the `rope_scaling` configuration.
+ """
+ if self.rope_scaling is None:
+ return
+
+ if not isinstance(self.rope_scaling,
+ dict) or len(self.rope_scaling) != 2:
+ raise ValueError(
+ '`rope_scaling` must be a dictionary with with two fields, `type` and `factor`, '
+ f'got {self.rope_scaling}')
+ rope_scaling_type = self.rope_scaling.get('type', None)
+ rope_scaling_factor = self.rope_scaling.get('factor', None)
+ if rope_scaling_type is None or rope_scaling_type not in [
+ 'linear', 'dynamic'
+ ]:
+ raise ValueError(
+ f"`rope_scaling`'s type field must be one of ['linear', 'dynamic'], got {rope_scaling_type}"
+ )
+ if (rope_scaling_factor is None
+ or not isinstance(rope_scaling_factor,
+ (float, int)) or rope_scaling_factor < 1.0):
+ raise ValueError(
+ f"`rope_scaling`'s factor field must be a number >= 1, got {rope_scaling_factor} "
+ f'of type {type(rope_scaling_factor)}')
diff --git a/xtuner/_lite/modelings/internlm2/modeling_internlm2.py b/xtuner/_lite/modelings/internlm2/modeling_internlm2.py
new file mode 100644
index 000000000..69ddc6196
--- /dev/null
+++ b/xtuner/_lite/modelings/internlm2/modeling_internlm2.py
@@ -0,0 +1,1899 @@
+# Copyright (c) The InternLM team and The HuggingFace Inc. team. All rights reserved.
+#
+# This code is based on transformers/src/transformers/models/llama/modeling_llama.py
+#
+# Licensed under the Apache License, Version 2.0 (the "License");
+# you may not use this file except in compliance with the License.
+# You may obtain a copy of the License at
+#
+# http://www.apache.org/licenses/LICENSE-2.0
+#
+# Unless required by applicable law or agreed to in writing, software
+# distributed under the License is distributed on an "AS IS" BASIS,
+# WITHOUT WARRANTIES OR CONDITIONS OF ANY KIND, either express or implied.
+# See the License for the specific language governing permissions and
+# limitations under the License.
+"""PyTorch InternLM2.5 model."""
+import math
+import queue
+import threading
+from typing import List, Optional, Tuple, Union
+
+import torch
+import torch.nn.functional as F
+import torch.utils.checkpoint
+from einops import rearrange
+from torch import nn
+from torch.nn import BCEWithLogitsLoss, CrossEntropyLoss, MSELoss
+from transformers.activations import ACT2FN
+from transformers.cache_utils import Cache, DynamicCache, StaticCache
+from transformers.modeling_attn_mask_utils import AttentionMaskConverter
+from transformers.modeling_outputs import (BaseModelOutputWithPast,
+ CausalLMOutputWithPast,
+ QuestionAnsweringModelOutput,
+ SequenceClassifierOutputWithPast,
+ TokenClassifierOutput)
+from transformers.modeling_utils import PreTrainedModel
+from transformers.pytorch_utils import ALL_LAYERNORM_LAYERS
+from transformers.utils import (add_start_docstrings,
+ add_start_docstrings_to_model_forward,
+ is_flash_attn_greater_or_equal_2_10, logging,
+ replace_return_docstrings)
+
+try:
+ from transformers.generation.streamers import BaseStreamer
+except Exception:
+ BaseStreamer = None
+
+from .configuration_internlm2 import InternLM2Config
+
+try:
+ from flash_attn import flash_attn_func, flash_attn_varlen_func
+ from flash_attn.bert_padding import (index_first_axis, pad_input,
+ unpad_input)
+except:
+ pass
+
+logger = logging.get_logger(__name__)
+
+_CONFIG_FOR_DOC = 'InternLM2Config'
+
+
+def _get_unpad_data(attention_mask):
+ seqlens_in_batch = attention_mask.sum(dim=-1, dtype=torch.int32)
+ indices = torch.nonzero(attention_mask.flatten(), as_tuple=False).flatten()
+ max_seqlen_in_batch = seqlens_in_batch.max().item()
+ cu_seqlens = F.pad(
+ torch.cumsum(seqlens_in_batch, dim=0, dtype=torch.int32), (1, 0)) # pylint: disable=E1102
+ return (
+ indices,
+ cu_seqlens,
+ max_seqlen_in_batch,
+ )
+
+
+class InternLM2RMSNorm(nn.Module):
+ """InternLM2RMSNorm is equivalent to T5LayerNorm."""
+
+ def __init__(self, hidden_size, eps=1e-6):
+ super().__init__()
+ self.weight = nn.Parameter(torch.ones(hidden_size))
+ self.variance_epsilon = eps
+
+ def forward(self, hidden_states):
+ input_dtype = hidden_states.dtype
+ hidden_states = hidden_states.to(torch.float32)
+ variance = hidden_states.pow(2).mean(-1, keepdim=True)
+ hidden_states = hidden_states * torch.rsqrt(variance +
+ self.variance_epsilon)
+ return self.weight * hidden_states.to(input_dtype)
+
+
+ALL_LAYERNORM_LAYERS.append(InternLM2RMSNorm)
+
+
+class InternLM2RotaryEmbedding(nn.Module):
+ """Rotary Position Embedding for the InternLM2 model. Credits to the Reddit user /u/lucidrains."""
+
+ def __init__(self,
+ dim,
+ max_position_embeddings=2048,
+ base=10000,
+ device=None,
+ scaling_factor=1.0):
+ super().__init__()
+ self.scaling_factor = scaling_factor
+ self.dim = dim
+ self.max_position_embeddings = max_position_embeddings
+ self.base = base
+ inv_freq = 1.0 / (
+ self.base
+ **(torch.arange(0, self.dim, 2,
+ dtype=torch.int64).float().to(device) / self.dim))
+ self.register_buffer('inv_freq', inv_freq, persistent=False)
+ # For BC we register cos and sin cached
+ self.max_seq_len_cached = max_position_embeddings
+
+ @torch.no_grad()
+ def forward(self, x, position_ids):
+ # x: [bs, num_attention_heads, seq_len, head_size]
+ inv_freq_expanded = self.inv_freq[None, :, None].float().expand(
+ position_ids.shape[0], -1, 1)
+ position_ids_expanded = position_ids[:, None, :].float()
+ # Force float32 since bfloat16 loses precision on long contexts
+ # See https://github.com/huggingface/transformers/pull/29285
+ device_type = x.device.type
+ device_type = device_type if isinstance(
+ device_type, str) and device_type != 'mps' else 'cpu'
+ with torch.autocast(device_type=device_type, enabled=False):
+ freqs = (inv_freq_expanded.float()
+ @ position_ids_expanded.float()).transpose(1, 2)
+ emb = torch.cat((freqs, freqs), dim=-1)
+ cos = emb.cos()
+ sin = emb.sin()
+ return cos.to(dtype=x.dtype), sin.to(dtype=x.dtype)
+
+
+class InternLM2LinearScalingRotaryEmbedding(InternLM2RotaryEmbedding):
+ """InternLM2RotaryEmbedding extended with linear scaling. Credits to the Reddit user /u/kaiokendev"""
+
+ def forward(self, x, position_ids):
+ # difference to the original RoPE: a scaling factor is aplied to the position ids
+ position_ids = position_ids.float() / self.scaling_factor
+ cos, sin = super().forward(x, position_ids)
+ return cos, sin
+
+
+class InternLM2DynamicNTKScalingRotaryEmbedding(InternLM2RotaryEmbedding):
+ """InternLM2RotaryEmbedding extended with Dynamic NTK scaling.
+ Credits to the Reddit users /u/bloc97 and /u/emozilla"""
+
+ def forward(self, x, position_ids):
+ # difference to the original RoPE: inv_freq is recomputed when the sequence length > original length
+ seq_len = torch.max(position_ids) + 1
+ if seq_len > self.max_position_embeddings:
+ base = self.base * ((self.scaling_factor * seq_len /
+ self.max_position_embeddings) -
+ (self.scaling_factor - 1))**(
+ self.dim / (self.dim - 2))
+ inv_freq = 1.0 / (
+ base
+ **(torch.arange(0, self.dim, 2, dtype=torch.int64).float().to(
+ x.device) / self.dim))
+ self.register_buffer(
+ 'inv_freq', inv_freq,
+ persistent=False) # TODO joao: this may break with compilation
+
+ cos, sin = super().forward(x, position_ids)
+ return cos, sin
+
+
+def rotate_half(x):
+ """Rotates half the hidden dims of the input."""
+ x1 = x[..., :x.shape[-1] // 2]
+ x2 = x[..., x.shape[-1] // 2:]
+ return torch.cat((-x2, x1), dim=-1)
+
+
+def apply_rotary_pos_emb(q, k, cos, sin, position_ids=None, unsqueeze_dim=1): # pylint: disable=unused-argument
+ """Applies Rotary Position Embedding to the query and key tensors.
+ Args:
+ q (`torch.Tensor`): The query tensor.
+ k (`torch.Tensor`): The key tensor.
+ cos (`torch.Tensor`): The cosine part of the rotary embedding.
+ sin (`torch.Tensor`): The sine part of the rotary embedding.
+ position_ids (`torch.Tensor`, *optional*):
+ Deprecated and unused.
+ unsqueeze_dim (`int`, *optional*, defaults to 1):
+ The 'unsqueeze_dim' argument specifies the dimension along which to unsqueeze cos[position_ids] and
+ sin[position_ids] so that they can be properly broadcasted to the dimensions of q and k. For example, note
+ that cos[position_ids] and sin[position_ids] have the shape [batch_size, seq_len, head_dim]. Then, if q and
+ k have the shape [batch_size, heads, seq_len, head_dim], then setting unsqueeze_dim=1 makes
+ cos[position_ids] and sin[position_ids] broadcastable to the shapes of q and k. Similarly, if q and k have
+ the shape [batch_size, seq_len, heads, head_dim], then set unsqueeze_dim=2.
+ Returns:
+ `tuple(torch.Tensor)` comprising of the query and key tensors rotated using the Rotary Position Embedding.
+ """
+ cos = cos.unsqueeze(unsqueeze_dim)
+ sin = sin.unsqueeze(unsqueeze_dim)
+ q_embed = (q * cos) + (rotate_half(q) * sin)
+ k_embed = (k * cos) + (rotate_half(k) * sin)
+ return q_embed, k_embed
+
+
+class InternLM2MLP(nn.Module):
+ """MLP for InternLM2 model."""
+
+ def __init__(self, config):
+ super().__init__()
+ self.config = config
+ self.hidden_size = config.hidden_size
+ self.intermediate_size = config.intermediate_size
+ self.w1 = nn.Linear(
+ self.hidden_size, self.intermediate_size, bias=False)
+ self.w3 = nn.Linear(
+ self.hidden_size, self.intermediate_size, bias=False)
+ self.w2 = nn.Linear(
+ self.intermediate_size, self.hidden_size, bias=False)
+ self.act_fn = ACT2FN[config.hidden_act]
+
+ def forward(self, x):
+ down_proj = self.w2(self.act_fn(self.w1(x)) * self.w3(x))
+
+ return down_proj
+
+
+def repeat_kv(hidden_states: torch.Tensor, n_rep: int) -> torch.Tensor:
+ """
+ This is the equivalent of torch.repeat_interleave(x, dim=1, repeats=n_rep). The hidden states go from (batch,
+ num_key_value_heads, seqlen, head_dim) to (batch, num_attention_heads, seqlen, head_dim)
+ """
+ batch, num_key_value_heads, slen, head_dim = hidden_states.shape
+ if n_rep == 1:
+ return hidden_states
+ hidden_states = hidden_states[:, :,
+ None, :, :].expand(batch,
+ num_key_value_heads,
+ n_rep, slen, head_dim)
+ return hidden_states.reshape(batch, num_key_value_heads * n_rep, slen,
+ head_dim)
+
+
+class InternLM2Attention(nn.Module):
+ """Multi-headed attention from 'Attention Is All You Need' paper"""
+
+ def __init__(self,
+ config: InternLM2Config,
+ layer_idx: Optional[int] = None):
+ super().__init__()
+ self.config = config
+ self.layer_idx = layer_idx
+ if layer_idx is None:
+ logger.warning_once(
+ f'Instantiating {self.__class__.__name__} without passing a `layer_idx` is not recommended and will '
+ 'lead to errors during the forward call if caching is used. Please make sure to provide a `layer_idx` '
+ 'when creating this class.')
+
+ self.hidden_size = config.hidden_size
+ self.num_heads = config.num_attention_heads
+ self.head_dim = self.hidden_size // self.num_heads
+ self.num_key_value_heads = config.num_key_value_heads
+ self.num_key_value_groups = self.num_heads // self.num_key_value_heads
+ self.max_position_embeddings = config.max_position_embeddings
+ self.rope_theta = config.rope_theta
+ self.is_causal = True
+
+ if (self.head_dim * self.num_heads) != self.hidden_size:
+ raise ValueError(
+ f'hidden_size must be divisible by num_heads (got `hidden_size`: {self.hidden_size}'
+ f' and `num_heads`: {self.num_heads}).')
+
+ self.wqkv = nn.Linear(
+ self.hidden_size,
+ (self.num_heads + 2 * self.num_key_value_heads) * self.head_dim,
+ bias=config.bias,
+ )
+ self.wo = nn.Linear(
+ self.num_heads * self.head_dim, self.hidden_size, bias=config.bias)
+
+ self._init_rope()
+
+ def _init_rope(self):
+ if self.config.rope_scaling is None:
+ self.rotary_emb = InternLM2RotaryEmbedding(
+ self.head_dim,
+ max_position_embeddings=self.max_position_embeddings,
+ base=self.rope_theta,
+ )
+ else:
+ scaling_type = self.config.rope_scaling['type']
+ scaling_factor = self.config.rope_scaling['factor']
+ if scaling_type == 'linear':
+ self.rotary_emb = InternLM2LinearScalingRotaryEmbedding(
+ self.head_dim,
+ max_position_embeddings=self.max_position_embeddings,
+ scaling_factor=scaling_factor,
+ base=self.rope_theta,
+ )
+ elif scaling_type == 'dynamic':
+ self.rotary_emb = InternLM2DynamicNTKScalingRotaryEmbedding(
+ self.head_dim,
+ max_position_embeddings=self.max_position_embeddings,
+ scaling_factor=scaling_factor,
+ base=self.rope_theta,
+ )
+ else:
+ raise ValueError(f'Unknown RoPE scaling type {scaling_type}')
+
+ def forward(
+ self,
+ hidden_states: torch.Tensor,
+ attention_mask: Optional[torch.Tensor] = None,
+ position_ids: Optional[torch.LongTensor] = None,
+ past_key_value: Optional[Cache] = None,
+ output_attentions: bool = False,
+ use_cache: bool = False, # pylint: disable=unused-argument
+ cache_position: Optional[torch.LongTensor] = None,
+ ) -> Tuple[torch.Tensor, Optional[torch.Tensor],
+ Optional[Tuple[torch.Tensor]]]:
+ bsz, q_len, _ = hidden_states.size()
+
+ if self.config.pretraining_tp > 1:
+ # split qkv_states by tp size
+ key_value_slicing = (self.num_key_value_heads *
+ self.head_dim) // self.config.pretraining_tp
+ qkv_slices = self.wqkv.weight.split(key_value_slicing, dim=0)
+ qkv_states = torch.cat(
+ [
+ F.linear(hidden_states, qkv_slice)
+ for qkv_slice in qkv_slices
+ ],
+ dim=-1 # pylint: disable=E1102
+ )
+ else:
+ qkv_states = self.wqkv(hidden_states)
+
+ qkv_states = rearrange(
+ qkv_states,
+ 'b q (h gs d) -> b q h gs d',
+ gs=2 + self.num_key_value_groups,
+ d=self.head_dim,
+ )
+
+ query_states = qkv_states[..., :self.num_key_value_groups, :]
+ query_states = rearrange(query_states,
+ 'b q h gs d -> b q (h gs) d').transpose(1, 2)
+ key_states = qkv_states[..., -2, :].transpose(1, 2)
+ value_states = qkv_states[..., -1, :].transpose(1, 2)
+
+ cos, sin = self.rotary_emb(value_states, position_ids)
+ query_states, key_states = apply_rotary_pos_emb(
+ query_states, key_states, cos, sin, position_ids)
+
+ if past_key_value is not None:
+ # sin and cos are specific to RoPE models; cache_position needed for the static cache
+ cache_kwargs = {
+ 'sin': sin,
+ 'cos': cos,
+ 'cache_position': cache_position
+ }
+ key_states, value_states = past_key_value.update(
+ key_states, value_states, self.layer_idx, cache_kwargs)
+
+ key_states = repeat_kv(key_states, self.num_key_value_groups)
+ value_states = repeat_kv(value_states, self.num_key_value_groups)
+
+ attn_weights = torch.matmul(query_states, key_states.transpose(
+ 2, 3)) / math.sqrt(self.head_dim)
+
+ if attention_mask is not None: # no matter the length, we just slice it
+ causal_mask = attention_mask[:, :, :, :key_states.shape[-2]]
+ attn_weights = attn_weights + causal_mask
+
+ # upcast attention to fp32
+ attn_weights = nn.functional.softmax(
+ attn_weights, dim=-1, dtype=torch.float32).to(query_states.dtype)
+ attn_output = torch.matmul(attn_weights, value_states)
+
+ if attn_output.size() != (bsz, self.num_heads, q_len, self.head_dim):
+ raise ValueError(
+ f'`attn_output` should be of size {(bsz, self.num_heads, q_len, self.head_dim)}, but is'
+ f' {attn_output.size()}')
+
+ attn_output = attn_output.transpose(1, 2).contiguous()
+
+ attn_output = attn_output.reshape(bsz, q_len, self.hidden_size)
+
+ if self.config.pretraining_tp > 1:
+ attn_output = attn_output.split(
+ self.hidden_size // self.config.pretraining_tp, dim=2)
+ o_proj_slices = self.wo.weight.split(
+ self.hidden_size // self.config.pretraining_tp, dim=1)
+ attn_output = sum([
+ F.linear(attn_output[i], o_proj_slices[i]) # pylint: disable=E1102
+ for i in range(self.config.pretraining_tp)
+ ])
+ else:
+ attn_output = self.wo(attn_output)
+
+ if not output_attentions:
+ attn_weights = None
+
+ return attn_output, attn_weights, past_key_value
+
+
+class InternLM2FlashAttention2(InternLM2Attention):
+ """
+ InternLM2 flash attention module. This module inherits from `InternLM2Attention` as the weights of the module stays
+ untouched. The only required change would be on the forward pass where it needs to correctly call the public API of
+ flash attention and deal with padding tokens in case the input contains any of them.
+ """
+
+ def __init__(self, *args, **kwargs):
+ super().__init__(*args, **kwargs)
+
+ # TODO: Should be removed once Flash Attention for RoCm is bumped to 2.1.
+ # flash_attn<2.1 generates top-left aligned causal mask, while what is needed here is bottom-right alignement,
+ # that was made default for flash_attn>=2.1. This attribute is used to handle this difference.
+ # Reference: https://github.com/Dao-AILab/flash-attention/releases/tag/v2.1.0.
+ # Beware that with flash_attn<2.1, using q_seqlen != k_seqlen (except for the case q_seqlen == 1)
+ # produces a wrong mask (top-left).
+ self._flash_attn_uses_top_left_mask = not is_flash_attn_greater_or_equal_2_10(
+ )
+
+ def forward(
+ self,
+ hidden_states: torch.Tensor,
+ attention_mask: Optional[torch.LongTensor] = None,
+ position_ids: Optional[torch.LongTensor] = None,
+ past_key_value: Optional[Cache] = None,
+ output_attentions: bool = False,
+ use_cache: bool = False,
+ cache_position: Optional[torch.LongTensor] = None,
+ ) -> Tuple[torch.Tensor, Optional[torch.Tensor],
+ Optional[Tuple[torch.Tensor]]]:
+ if isinstance(past_key_value, StaticCache):
+ raise ValueError(
+ '`static` cache implementation is not compatible with `attn_implementation==flash_attention_2` '
+ 'make sure to use `sdpa` in the mean time, and open an issue at '
+ 'https://github.com/huggingface/transformers')
+
+ output_attentions = False
+
+ bsz, q_len, _ = hidden_states.size()
+
+ qkv_states = self.wqkv(hidden_states)
+
+ qkv_states = rearrange(
+ qkv_states,
+ 'b q (h gs d) -> b q h gs d',
+ gs=2 + self.num_key_value_groups,
+ d=self.head_dim,
+ )
+
+ query_states = qkv_states[..., :self.num_key_value_groups, :]
+ query_states = rearrange(query_states, 'b q h gs d -> b q (h gs) d')
+ key_states = qkv_states[..., -2, :]
+ value_states = qkv_states[..., -1, :]
+
+ query_states = query_states.transpose(1, 2)
+ key_states = key_states.transpose(1, 2)
+ value_states = value_states.transpose(1, 2)
+
+ cos, sin = self.rotary_emb(value_states, position_ids)
+ query_states, key_states = apply_rotary_pos_emb(
+ query_states, key_states, cos, sin)
+
+ if past_key_value is not None:
+ # sin and cos are specific to RoPE models; cache_position needed for the static cache
+ cache_kwargs = {
+ 'sin': sin,
+ 'cos': cos,
+ 'cache_position': cache_position
+ }
+ key_states, value_states = past_key_value.update(
+ key_states, value_states, self.layer_idx, cache_kwargs)
+
+ # TODO: These transpose are quite inefficient but Flash Attention requires the layout
+ # [batch_size, sequence_length, num_heads, head_dim]. We would need to refactor the KV cache
+ # to be able to avoid many of these transpose/reshape/view.
+ query_states = query_states.transpose(1, 2)
+ key_states = key_states.transpose(1, 2)
+ value_states = value_states.transpose(1, 2)
+
+ # dropout_rate = self.attention_dropout if self.training else 0.0
+ dropout_rate = 0.0
+
+ # In PEFT, usually we cast the layer norms in float32 for training stability reasons
+ # therefore the input hidden states gets silently casted in float32. Hence, we need
+ # cast them back in the correct dtype just to be sure everything works as expected.
+ # This might slowdown training & inference so it is recommended to not cast the LayerNorms
+ # in fp32. (InternLM2RMSNorm handles it correctly)
+
+ input_dtype = query_states.dtype
+ if input_dtype == torch.float32:
+ if torch.is_autocast_enabled():
+ target_dtype = torch.get_autocast_gpu_dtype()
+ # Handle the case where the model is quantized
+ elif hasattr(self.config, '_pre_quantization_dtype'):
+ target_dtype = self.config._pre_quantization_dtype
+ else:
+ target_dtype = self.wqkv.weight.dtype
+
+ logger.warning_once(
+ f'The input hidden states seems to be silently casted in float32, this might be related to'
+ f' the fact you have upcasted embedding or layer norm layers in float32. We will cast back the input in'
+ f' {target_dtype}.')
+
+ query_states = query_states.to(target_dtype)
+ key_states = key_states.to(target_dtype)
+ value_states = value_states.to(target_dtype)
+
+ attn_output = self._flash_attention_forward(
+ query_states,
+ key_states,
+ value_states,
+ attention_mask,
+ q_len,
+ dropout=dropout_rate)
+
+ attn_output = attn_output.reshape(bsz, q_len,
+ self.hidden_size).contiguous()
+ attn_output = self.wo(attn_output)
+
+ if not output_attentions:
+ attn_weights = None
+
+ return attn_output, attn_weights, past_key_value # pylint: disable=E0606
+
+ def _flash_attention_forward(self,
+ query_states,
+ key_states,
+ value_states,
+ attention_mask,
+ query_length,
+ dropout=0.0,
+ softmax_scale=None):
+ """
+ Calls the forward method of Flash Attention - if the input hidden states contain at least one padding token
+ first unpad the input, then computes the attention scores and pad the final attention scores.
+ Args:
+ query_states (`torch.Tensor`):
+ Input query states to be passed to Flash Attention API
+ key_states (`torch.Tensor`):
+ Input key states to be passed to Flash Attention API
+ value_states (`torch.Tensor`):
+ Input value states to be passed to Flash Attention API
+ attention_mask (`torch.Tensor`):
+ The padding mask - corresponds to a tensor of size `(batch_size, seq_len)` where 0 stands for the
+ position of padding tokens and 1 for the position of non-padding tokens.
+ dropout (`float`):
+ Attention dropout
+ softmax_scale (`float`, *optional*):
+ The scaling of QK^T before applying softmax. Default to 1 / sqrt(head_dim)
+ """
+ if not self._flash_attn_uses_top_left_mask:
+ causal = self.is_causal
+ else:
+ # TODO: Remove the `query_length != 1` check once Flash Attention for RoCm is bumped to 2.1.
+ # For details, please see the comment in InternLM2FlashAttention2 __init__.
+ causal = self.is_causal and query_length != 1
+
+ # Contains at least one padding token in the sequence
+ if attention_mask is not None:
+ batch_size = query_states.shape[0]
+ query_states, key_states, value_states, indices_q, cu_seq_lens, max_seq_lens = self._upad_input(
+ query_states, key_states, value_states, attention_mask,
+ query_length)
+
+ cu_seqlens_q, cu_seqlens_k = cu_seq_lens
+ max_seqlen_in_batch_q, max_seqlen_in_batch_k = max_seq_lens
+
+ attn_output_unpad = flash_attn_varlen_func( # pylint: disable=E0606
+ query_states,
+ key_states,
+ value_states,
+ cu_seqlens_q=cu_seqlens_q,
+ cu_seqlens_k=cu_seqlens_k,
+ max_seqlen_q=max_seqlen_in_batch_q,
+ max_seqlen_k=max_seqlen_in_batch_k,
+ dropout_p=dropout,
+ softmax_scale=softmax_scale,
+ causal=causal,
+ )
+
+ attn_output = pad_input(attn_output_unpad, indices_q, batch_size,
+ query_length) # pylint: disable=E0606
+ else:
+ attn_output = flash_attn_func( # pylint: disable=E0606
+ query_states,
+ key_states,
+ value_states,
+ dropout,
+ softmax_scale=softmax_scale,
+ causal=causal)
+
+ return attn_output
+
+ def _upad_input(self, query_layer, key_layer, value_layer, attention_mask,
+ query_length):
+ indices_k, cu_seqlens_k, max_seqlen_in_batch_k = _get_unpad_data(
+ attention_mask)
+ batch_size, kv_seq_len, num_key_value_heads, head_dim = key_layer.shape
+
+ key_layer = index_first_axis( # pylint: disable=E0606
+ key_layer.reshape(batch_size * kv_seq_len, num_key_value_heads,
+ head_dim), indices_k)
+ value_layer = index_first_axis( # pylint: disable=E0606
+ value_layer.reshape(batch_size * kv_seq_len, num_key_value_heads,
+ head_dim), indices_k)
+ if query_length == kv_seq_len:
+ query_layer = index_first_axis( # pylint: disable=E0606
+ query_layer.reshape(batch_size * kv_seq_len, self.num_heads,
+ head_dim), indices_k)
+ cu_seqlens_q = cu_seqlens_k
+ max_seqlen_in_batch_q = max_seqlen_in_batch_k
+ indices_q = indices_k
+ elif query_length == 1:
+ max_seqlen_in_batch_q = 1
+ cu_seqlens_q = torch.arange(
+ batch_size + 1, dtype=torch.int32, device=query_layer.device
+ ) # There is a memcpy here, that is very bad.
+ indices_q = cu_seqlens_q[:-1]
+ query_layer = query_layer.squeeze(1)
+ else:
+ # The -q_len: slice assumes left padding.
+ attention_mask = attention_mask[:, -query_length:]
+ query_layer, indices_q, cu_seqlens_q, max_seqlen_in_batch_q = unpad_input( # pylint: disable=E0606
+ query_layer, attention_mask)
+
+ return (
+ query_layer,
+ key_layer,
+ value_layer,
+ indices_q,
+ (cu_seqlens_q, cu_seqlens_k),
+ (max_seqlen_in_batch_q, max_seqlen_in_batch_k),
+ )
+
+
+# Copied from transformers.models.llama.modeling_llama.LllamaSdpaAttention with Llama->InternLM2
+class InternLM2SdpaAttention(InternLM2Attention):
+ """
+ InternLM2 attention module using torch.nn.functional.scaled_dot_product_attention. This module inherits from
+ `InternLM2Attention` as the weights of the module stays untouched. The only changes are on the forward pass
+ to adapt to SDPA API.
+ """
+
+ # Adapted from InternLM2Attention.forward
+ def forward(
+ self,
+ hidden_states: torch.Tensor,
+ attention_mask: Optional[torch.Tensor] = None,
+ position_ids: Optional[torch.LongTensor] = None,
+ past_key_value: Optional[Cache] = None,
+ output_attentions: bool = False,
+ use_cache: bool = False,
+ cache_position: Optional[torch.LongTensor] = None,
+ ) -> Tuple[torch.Tensor, Optional[torch.Tensor],
+ Optional[Tuple[torch.Tensor]]]:
+ if output_attentions:
+ # TODO: Improve this warning with e.g. `model.config.attn_implementation = "manual"`
+ # once this is implemented.
+ logger.warning_once(
+ 'InternLM2Model uses InternLM2SdpaAttention, but `torch.nn.functional.scaled_dot_product_attention` '
+ 'does not support `output_attentions=True`. Falling back to the manual attention implementation, '
+ 'but specifying the manual implementation will be required from Transformers version v5.0.0 onwards. '
+ 'This warning can be removed using the argument `attn_implementation="eager"` when loading the model.'
+ )
+ return super().forward(
+ hidden_states=hidden_states,
+ attention_mask=attention_mask,
+ position_ids=position_ids,
+ past_key_value=past_key_value,
+ output_attentions=output_attentions,
+ use_cache=use_cache,
+ cache_position=cache_position,
+ )
+
+ bsz, q_len, _ = hidden_states.size()
+
+ qkv_states = self.wqkv(hidden_states)
+
+ qkv_states = rearrange(
+ qkv_states,
+ 'b q (h gs d) -> b q h gs d',
+ gs=2 + self.num_key_value_groups,
+ d=self.head_dim,
+ )
+
+ query_states = qkv_states[..., :self.num_key_value_groups, :]
+ query_states = rearrange(query_states, 'b q h gs d -> b q (h gs) d')
+ key_states = qkv_states[..., -2, :]
+ value_states = qkv_states[..., -1, :]
+
+ query_states = query_states.transpose(1, 2)
+ key_states = key_states.transpose(1, 2)
+ value_states = value_states.transpose(1, 2)
+
+ cos, sin = self.rotary_emb(value_states, position_ids)
+ query_states, key_states = apply_rotary_pos_emb(
+ query_states, key_states, cos, sin)
+
+ if past_key_value is not None:
+ # sin and cos are specific to RoPE models; cache_position needed for the static cache
+ cache_kwargs = {
+ 'sin': sin,
+ 'cos': cos,
+ 'cache_position': cache_position
+ }
+ key_states, value_states = past_key_value.update(
+ key_states, value_states, self.layer_idx, cache_kwargs)
+
+ key_states = repeat_kv(key_states, self.num_key_value_groups)
+ value_states = repeat_kv(value_states, self.num_key_value_groups)
+
+ causal_mask = attention_mask
+ if attention_mask is not None:
+ causal_mask = causal_mask[:, :, :, :key_states.shape[-2]]
+
+ # SDPA with memory-efficient backend is currently (torch==2.1.2) bugged with non-contiguous inputs with
+ # custom attn_mask, Reference: https://github.com/pytorch/pytorch/issues/112577.
+ if query_states.device.type == 'cuda' and causal_mask is not None:
+ query_states = query_states.contiguous()
+ key_states = key_states.contiguous()
+ value_states = value_states.contiguous()
+
+ # We dispatch to SDPA's Flash Attention or Efficient kernels via this `is_causal` if statement instead of
+ # an inline conditional assignment in SDPA to support both torch.compile's dynamic shapes and full graph
+ # options. An inline conditional prevents dynamic shapes from compiling.
+ is_causal = bool(causal_mask is None and q_len > 1)
+
+ attn_output = torch.nn.functional.scaled_dot_product_attention( # pylint: disable=E1102
+ query_states,
+ key_states,
+ value_states,
+ attn_mask=causal_mask,
+ dropout_p=0.0,
+ is_causal=is_causal,
+ )
+
+ attn_output = attn_output.transpose(1, 2).contiguous()
+ attn_output = attn_output.view(bsz, q_len, self.hidden_size)
+
+ attn_output = self.wo(attn_output)
+
+ return attn_output, None, past_key_value
+
+
+INTERNLM2_ATTENTION_CLASSES = {
+ 'eager': InternLM2Attention,
+ 'flash_attention_2': InternLM2FlashAttention2,
+ 'sdpa': InternLM2SdpaAttention,
+}
+
+
+# Modified from transformers.models.llama.modeling_llama.LlamaDecoderLayer with Llama->InternLM2
+class InternLM2DecoderLayer(nn.Module):
+ """InternLM2 Decoder Layer. This module is a single layer of the InternLM2 model."""
+
+ def __init__(self, config: InternLM2Config, layer_idx: int):
+ super().__init__()
+ self.hidden_size = config.hidden_size
+ self.layer_idx = layer_idx
+
+ self.attention = INTERNLM2_ATTENTION_CLASSES[
+ config.attn_implementation](
+ config=config, layer_idx=layer_idx)
+
+ self.feed_forward = InternLM2MLP(config)
+ self.attention_norm = InternLM2RMSNorm(
+ config.hidden_size, eps=config.rms_norm_eps)
+ self.ffn_norm = InternLM2RMSNorm(
+ config.hidden_size, eps=config.rms_norm_eps)
+
+ def forward(
+ self,
+ hidden_states: torch.Tensor,
+ attention_mask: Optional[torch.Tensor] = None,
+ position_ids: Optional[torch.LongTensor] = None,
+ past_key_value: Optional[Cache] = None,
+ output_attentions: Optional[bool] = False,
+ use_cache: Optional[bool] = False,
+ cache_position: Optional[torch.LongTensor] = None,
+ ) -> Tuple[torch.FloatTensor, Optional[Tuple[torch.FloatTensor,
+ torch.FloatTensor]]]:
+ """
+ Args:
+ hidden_states (`torch.FloatTensor`): input to the layer of shape `(batch, seq_len, embed_dim)`
+ attention_mask (`torch.FloatTensor`, *optional*):
+ attention mask of size `(batch_size, sequence_length)` if flash attention is used or `(batch_size, 1,
+ query_sequence_length, key_sequence_length)` if default attention is used.
+ output_attentions (`bool`, *optional*):
+ Whether or not to return the attentions tensors of all attention layers. See `attentions` under
+ returned tensors for more detail.
+ use_cache (`bool`, *optional*):
+ If set to `True`, `past_key_values` key value states are returned and can be used to speed up decoding
+ (see `past_key_values`).
+ past_key_value (`Tuple(torch.FloatTensor)`, *optional*): cached past key and value projection states
+ """
+ residual = hidden_states
+
+ hidden_states = self.attention_norm(hidden_states)
+
+ # Self Attention
+ hidden_states, self_attn_weights, present_key_value = self.attention(
+ hidden_states=hidden_states,
+ attention_mask=attention_mask,
+ position_ids=position_ids,
+ past_key_value=past_key_value,
+ output_attentions=output_attentions,
+ use_cache=use_cache,
+ cache_position=cache_position,
+ )
+ hidden_states = residual + hidden_states
+
+ # Fully Connected
+ residual = hidden_states
+ hidden_states = self.ffn_norm(hidden_states)
+ hidden_states = self.feed_forward(hidden_states)
+ hidden_states = residual + hidden_states
+
+ outputs = (hidden_states, )
+
+ if output_attentions:
+ outputs += (self_attn_weights, )
+
+ if use_cache:
+ outputs += (present_key_value, )
+
+ return outputs
+
+
+InternLM2_START_DOCSTRING = r"""
+ This model inherits from [`PreTrainedModel`]. Check the superclass documentation for the generic methods the
+ library implements for all its model (such as downloading or saving, resizing the input embeddings, pruning heads
+ etc.)
+ This model is also a PyTorch [torch.nn.Module](https://pytorch.org/docs/stable/nn.html#torch.nn.Module) subclass.
+ Use it as a regular PyTorch Module and refer to the PyTorch documentation for all matter related to general usage
+ and behavior.
+ Parameters:
+ config ([`InternLM2Config`]):
+ Model configuration class with all the parameters of the model. Initializing with a config file does not
+ load the weights associated with the model, only the configuration. Check out the
+ [`~PreTrainedModel.from_pretrained`] method to load the model weights.
+"""
+
+
+# Copied from transformers.models.llama.modeling_llama.LlamaPreTrainedModel with Llama->InternLM2
+@add_start_docstrings(
+ 'The bare InternLM2 Model outputting raw hidden-states without any specific head on top.',
+ InternLM2_START_DOCSTRING,
+)
+class InternLM2PreTrainedModel(PreTrainedModel):
+ """
+ InternLM2 pretraiend model's base class.
+ """
+
+ config_class = InternLM2Config
+ base_model_prefix = 'model'
+ supports_gradient_checkpointing = True
+ _no_split_modules = ['InternLM2DecoderLayer']
+ _skip_keys_device_placement = ['past_key_values']
+ _supports_flash_attn_2 = True
+ _supports_sdpa = True
+ _supports_cache_class = True
+ _supports_quantized_cache = True
+ _supports_static_cache = True
+
+ def _init_weights(self, module):
+ std = self.config.initializer_range
+ if isinstance(module, nn.Linear):
+ module.weight.data.normal_(mean=0.0, std=std)
+ if module.bias is not None:
+ module.bias.data.zero_()
+ elif isinstance(module, nn.Embedding):
+ module.weight.data.normal_(mean=0.0, std=std)
+ if module.padding_idx is not None:
+ module.weight.data[module.padding_idx].zero_()
+
+
+InternLM2_INPUTS_DOCSTRING = r"""
+ Args:
+ input_ids (`torch.LongTensor` of shape `(batch_size, sequence_length)`):
+ Indices of input sequence tokens in the vocabulary. Padding will be ignored by default should you provide
+ it.
+ Indices can be obtained using [`AutoTokenizer`]. See [`PreTrainedTokenizer.encode`] and
+ [`PreTrainedTokenizer.__call__`] for details.
+ [What are input IDs?](../glossary#input-ids)
+ attention_mask (`torch.Tensor` of shape `(batch_size, sequence_length)`, *optional*):
+ Mask to avoid performing attention on padding token indices. Mask values selected in `[0, 1]`:
+ - 1 for tokens that are **not masked**,
+ - 0 for tokens that are **masked**.
+ [What are attention masks?](../glossary#attention-mask)
+ Indices can be obtained using [`AutoTokenizer`]. See [`PreTrainedTokenizer.encode`] and
+ [`PreTrainedTokenizer.__call__`] for details.
+ If `past_key_values` is used, optionally only the last `input_ids` have to be input (see
+ `past_key_values`).
+ If you want to change padding behavior, you should read [`modeling_opt._prepare_decoder_attention_mask`]
+ and modify to your needs. See diagram 1 in [the paper](https://arxiv.org/abs/1910.13461) for more
+ information on the default strategy.
+ - 1 indicates the head is **not masked**,
+ - 0 indicates the head is **masked**.
+ position_ids (`torch.LongTensor` of shape `(batch_size, sequence_length)`, *optional*):
+ Indices of positions of each input sequence tokens in the position embeddings. Selected in the range `[0,
+ config.n_positions - 1]`.
+ [What are position IDs?](../glossary#position-ids)
+ past_key_values (`Cache` or `tuple(tuple(torch.FloatTensor))`, *optional*):
+ Pre-computed hidden-states (key and values in the self-attention blocks and in the cross-attention
+ blocks) that can be used to speed up sequential decoding. This typically consists in the `past_key_values`
+ returned by the model at a previous stage of decoding, when `use_cache=True` or `config.use_cache=True`.
+ Two formats are allowed:
+ - a [`~cache_utils.Cache`] instance;
+ - Tuple of `tuple(torch.FloatTensor)` of length `config.n_layers`, with each tuple having 2 tensors of
+ shape `(batch_size, num_heads, sequence_length, embed_size_per_head)`). This is also known as the legacy
+ cache format.
+ The model will output the same cache format that is fed as input. If no `past_key_values` are passed, the
+ legacy cache format will be returned.
+ If `past_key_values` are used, the user can optionally input only the last `input_ids` (those that don't
+ have their past key value states given to this model) of shape `(batch_size, 1)` instead of all `input_ids`
+ of shape `(batch_size, sequence_length)`.
+ inputs_embeds (`torch.FloatTensor` of shape `(batch_size, sequence_length, hidden_size)`, *optional*):
+ Optionally, instead of passing `input_ids` you can choose to directly pass an embedded representation. This
+ is useful if you want more control over how to convert `input_ids` indices into associated vectors than the
+ model's internal embedding lookup matrix.
+ use_cache (`bool`, *optional*):
+ If set to `True`, `past_key_values` key value states are returned and can be used to speed up decoding (see
+ `past_key_values`).
+ output_attentions (`bool`, *optional*):
+ Whether or not to return the attentions tensors of all attention layers. See `attentions` under returned
+ tensors for more detail.
+ output_hidden_states (`bool`, *optional*):
+ Whether or not to return the hidden states of all layers. See `hidden_states` under returned tensors for
+ more detail.
+ return_dict (`bool`, *optional*):
+ Whether or not to return a [`~utils.ModelOutput`] instead of a plain tuple.
+ cache_position (`torch.LongTensor` of shape `(sequence_length)`, *optional*):
+ Indices depicting the position of the input sequence tokens in the sequence. Contrarily to `position_ids`,
+ this tensor is not affected by padding. It is used to update the cache in the correct position and to infer
+ the complete sequence length.
+"""
+
+
+# Modified from transformers.models.llama.modeling_llama.LlamaModel with Llama->InternLM2
+@add_start_docstrings(
+ 'The bare InternLM2 Model outputting raw hidden-states without any specific head on top.',
+ InternLM2_START_DOCSTRING,
+)
+class InternLM2Model(InternLM2PreTrainedModel):
+ """
+ Transformer decoder consisting of *config.num_hidden_layers* layers. Each layer is a [`InternLM2DecoderLayer`]
+ Args:
+ config: InternLM2Config
+ """
+
+ _auto_class = 'AutoModel'
+
+ def __init__(self, config: InternLM2Config):
+ super().__init__(config)
+ self.padding_idx = config.pad_token_id
+ self.vocab_size = config.vocab_size
+ self.config = config
+
+ self.tok_embeddings = nn.Embedding(config.vocab_size,
+ config.hidden_size,
+ self.padding_idx)
+
+ self.layers = nn.ModuleList([
+ InternLM2DecoderLayer(config, layer_idx)
+ for layer_idx in range(config.num_hidden_layers)
+ ])
+ self.norm = InternLM2RMSNorm(
+ config.hidden_size, eps=config.rms_norm_eps)
+
+ self.gradient_checkpointing = False
+ # Initialize weights and apply final processing
+ self.post_init()
+
+ def get_input_embeddings(self):
+ return self.tok_embeddings
+
+ def set_input_embeddings(self, value):
+ self.tok_embeddings = value
+
+ @add_start_docstrings_to_model_forward(InternLM2_INPUTS_DOCSTRING)
+ def forward(
+ self,
+ input_ids: torch.LongTensor = None,
+ attention_mask: Optional[torch.Tensor] = None,
+ position_ids: Optional[torch.LongTensor] = None,
+ past_key_values: Optional[Union[Cache,
+ List[torch.FloatTensor]]] = None,
+ inputs_embeds: Optional[torch.FloatTensor] = None,
+ use_cache: Optional[bool] = None,
+ output_attentions: Optional[bool] = None,
+ output_hidden_states: Optional[bool] = None,
+ return_dict: Optional[bool] = None,
+ cache_position: Optional[torch.LongTensor] = None,
+ ) -> Union[Tuple, BaseModelOutputWithPast]:
+ output_attentions = output_attentions if output_attentions is not None else self.config.output_attentions
+ output_hidden_states = (
+ output_hidden_states if output_hidden_states is not None else
+ self.config.output_hidden_states)
+ use_cache = use_cache if use_cache is not None else self.config.use_cache
+ return_dict = return_dict if return_dict is not None else self.config.use_return_dict
+
+ if (input_ids is None) ^ (inputs_embeds is not None):
+ raise ValueError(
+ 'You cannot specify both input_ids and inputs_embeds at the same time, and must specify either one'
+ )
+
+ if self.gradient_checkpointing and self.training and use_cache:
+ logger.warning_once(
+ '`use_cache=True` is incompatible with gradient checkpointing. Setting `use_cache=False`.'
+ )
+ use_cache = False
+
+ if inputs_embeds is None:
+ inputs_embeds = self.tok_embeddings(input_ids)
+
+ return_legacy_cache = False
+ if use_cache and not isinstance(
+ past_key_values,
+ Cache): # kept for BC (non `Cache` `past_key_values` inputs)
+ return_legacy_cache = True
+ past_key_values = DynamicCache.from_legacy_cache(past_key_values)
+
+ if cache_position is None:
+ past_seen_tokens = past_key_values.get_seq_length(
+ ) if past_key_values is not None else 0
+ cache_position = torch.arange(
+ past_seen_tokens,
+ past_seen_tokens + inputs_embeds.shape[1],
+ device=inputs_embeds.device)
+ if position_ids is None:
+ position_ids = cache_position.unsqueeze(0)
+
+ causal_mask = self._update_causal_mask(attention_mask, inputs_embeds,
+ cache_position, past_key_values,
+ output_attentions)
+
+ # embed positions
+ hidden_states = inputs_embeds
+
+ # decoder layers
+ all_hidden_states = () if output_hidden_states else None
+ all_self_attns = () if output_attentions else None
+ next_decoder_cache = None
+
+ for decoder_layer in self.layers:
+ if output_hidden_states:
+ all_hidden_states += (hidden_states, )
+
+ if self.gradient_checkpointing and self.training:
+ layer_outputs = self._gradient_checkpointing_func(
+ decoder_layer.__call__,
+ hidden_states,
+ causal_mask,
+ position_ids,
+ past_key_values,
+ output_attentions,
+ use_cache,
+ cache_position,
+ )
+ else:
+ layer_outputs = decoder_layer(
+ hidden_states,
+ attention_mask=causal_mask,
+ position_ids=position_ids,
+ past_key_value=past_key_values,
+ output_attentions=output_attentions,
+ use_cache=use_cache,
+ cache_position=cache_position,
+ )
+
+ hidden_states = layer_outputs[0]
+
+ if use_cache:
+ next_decoder_cache = layer_outputs[
+ 2 if output_attentions else 1]
+
+ if output_attentions:
+ all_self_attns += (layer_outputs[1], )
+
+ hidden_states = self.norm(hidden_states)
+
+ # add hidden states from the last decoder layer
+ if output_hidden_states:
+ all_hidden_states += (hidden_states, )
+
+ next_cache = next_decoder_cache if use_cache else None
+ if return_legacy_cache:
+ next_cache = next_cache.to_legacy_cache()
+
+ if not return_dict:
+ return tuple(
+ v for v in
+ [hidden_states, next_cache, all_hidden_states, all_self_attns]
+ if v is not None)
+ return BaseModelOutputWithPast(
+ last_hidden_state=hidden_states,
+ past_key_values=next_cache,
+ hidden_states=all_hidden_states,
+ attentions=all_self_attns,
+ )
+
+ def _update_causal_mask(
+ self,
+ attention_mask: torch.Tensor,
+ input_tensor: torch.Tensor,
+ cache_position: torch.Tensor,
+ past_key_values: Cache,
+ output_attentions: bool,
+ ):
+ # TODO: As of torch==2.2.0, the `attention_mask` passed to the model in `generate` is 2D and of dynamic length
+ # even when the static KV cache is used. This is an issue for torch.compile which then recaptures cudagraphs at
+ # each decode steps due to the dynamic shapes. (`recording cudagraph tree for symint key 13`, etc.), which is
+ # VERY slow. A workaround is `@torch.compiler.disable`, but this prevents using `fullgraph=True`.
+ # See more context in https://github.com/huggingface/transformers/pull/29114
+
+ if self.config.attn_implementation == 'flash_attention_2':
+ if attention_mask is not None and 0.0 in attention_mask:
+ return attention_mask
+ return None
+
+ # For SDPA, when possible, we will rely on its `is_causal` argument instead of its `attn_mask` argument, in
+ # order to dispatch on Flash Attention 2. This feature is not compatible with static cache, as SDPA will fail
+ # to infer the attention mask.
+ past_seen_tokens = past_key_values.get_seq_length(
+ ) if past_key_values is not None else 0
+ using_static_cache = isinstance(past_key_values, StaticCache)
+
+ # When output attentions is True, sdpa implementation's forward method calls the eager implementation's forward
+ if self.config.attn_implementation == 'sdpa' and not using_static_cache and not output_attentions:
+ if AttentionMaskConverter._ignore_causal_mask_sdpa(
+ attention_mask,
+ inputs_embeds=input_tensor,
+ past_key_values_length=past_seen_tokens,
+ is_training=self.training,
+ ):
+ return None
+
+ dtype, device = input_tensor.dtype, input_tensor.device
+ min_dtype = torch.finfo(dtype).min
+ sequence_length = input_tensor.shape[1]
+ if using_static_cache:
+ target_length = past_key_values.get_max_length()
+ else:
+ target_length = (
+ attention_mask.shape[-1] if isinstance(
+ attention_mask, torch.Tensor) else past_seen_tokens +
+ sequence_length + 1)
+
+ if attention_mask is not None and attention_mask.dim() == 4:
+ # in this case we assume that the mask comes already in inverted form and requires no inversion or slicing
+ if attention_mask.max() != 0:
+ raise ValueError(
+ 'Custom 4D attention mask should be passed in inverted form with max==0`'
+ )
+ causal_mask = attention_mask
+ else:
+ causal_mask = torch.full((sequence_length, target_length),
+ fill_value=min_dtype,
+ dtype=dtype,
+ device=device)
+ if sequence_length != 1:
+ causal_mask = torch.triu(causal_mask, diagonal=1)
+ causal_mask *= torch.arange(
+ target_length, device=device) > cache_position.reshape(-1, 1)
+ causal_mask = causal_mask[None, None, :, :].expand(
+ input_tensor.shape[0], 1, -1, -1)
+ if attention_mask is not None:
+ causal_mask = causal_mask.clone(
+ ) # copy to contiguous memory for in-place edit
+ mask_length = attention_mask.shape[-1]
+ padding_mask = causal_mask[:, :, :, :
+ mask_length] + attention_mask[:,
+ None,
+ None, :]
+ padding_mask = padding_mask == 0
+ causal_mask[:, :, :, :
+ mask_length] = causal_mask[:, :, :, :
+ mask_length].masked_fill(
+ padding_mask,
+ min_dtype)
+ if (self.config.attn_implementation == 'sdpa'
+ and attention_mask is not None
+ and attention_mask.device.type == 'cuda'
+ and not output_attentions):
+ # Attend to all tokens in fully masked rows in the causal_mask, for example the relevant first rows when
+ # using left padding. This is required by F.scaled_dot_product_attention memory-efficient attention path.
+ # Details: https://github.com/pytorch/pytorch/issues/110213
+ causal_mask = AttentionMaskConverter._unmask_unattended(
+ causal_mask, min_dtype) # pylint: disable=E1120
+
+ return causal_mask
+
+
+# Modified from transformers.models.llama.modeling_llama.LlamaForCausalLM
+class InternLM2ForCausalLM(InternLM2PreTrainedModel):
+ """Causal language model (CLM) for InternLM2."""
+
+ _auto_class = 'AutoModelForCausalLM'
+ _tied_weights_keys = ['output.weight']
+
+ def __init__(self, config):
+ super().__init__(config)
+ self.model = InternLM2Model(config)
+ self.vocab_size = config.vocab_size
+ self.output = nn.Linear(
+ config.hidden_size, config.vocab_size, bias=False)
+
+ # Initialize weights and apply final processing
+ self.post_init()
+
+ def get_input_embeddings(self):
+ return self.model.tok_embeddings
+
+ def set_input_embeddings(self, value):
+ self.model.tok_embeddings = value
+
+ def get_output_embeddings(self):
+ return self.output
+
+ def set_output_embeddings(self, new_embeddings):
+ self.output = new_embeddings
+
+ def set_decoder(self, decoder):
+ self.model = decoder
+
+ def get_decoder(self):
+ return self.model
+
+ @add_start_docstrings_to_model_forward(InternLM2_INPUTS_DOCSTRING)
+ @replace_return_docstrings(
+ output_type=CausalLMOutputWithPast, config_class=_CONFIG_FOR_DOC)
+ def forward(
+ self,
+ input_ids: torch.LongTensor = None,
+ attention_mask: Optional[torch.Tensor] = None,
+ position_ids: Optional[torch.LongTensor] = None,
+ past_key_values: Optional[Union[Cache,
+ List[torch.FloatTensor]]] = None,
+ inputs_embeds: Optional[torch.FloatTensor] = None,
+ labels: Optional[torch.LongTensor] = None,
+ use_cache: Optional[bool] = None,
+ output_attentions: Optional[bool] = None,
+ output_hidden_states: Optional[bool] = None,
+ return_dict: Optional[bool] = None,
+ cache_position: Optional[torch.LongTensor] = None,
+ ) -> Union[Tuple, CausalLMOutputWithPast]:
+ r"""
+ Args:
+ labels (`torch.LongTensor` of shape `(batch_size, sequence_length)`, *optional*):
+ Labels for computing the masked language modeling loss. Indices should either be in `[0, ...,
+ config.vocab_size]` or -100 (see `input_ids` docstring). Tokens with indices set to `-100` are ignored
+ (masked), the loss is only computed for the tokens with labels in `[0, ..., config.vocab_size]`.
+ Returns:
+ Example:
+ ```python
+ >>> from transformers import AutoTokenizer, InternLM2ForCausalLM
+ >>> model = InternLM2ForCausalLM.from_pretrained("meta-InternLM2/InternLM2-2-7b-hf")
+ >>> tokenizer = AutoTokenizer.from_pretrained("meta-InternLM2/InternLM2-2-7b-hf")
+ >>> prompt = "Hey, are you conscious? Can you talk to me?"
+ >>> inputs = tokenizer(prompt, return_tensors="pt")
+ >>> # Generate
+ >>> generate_ids = model.generate(inputs.input_ids, max_length=30)
+ >>> tokenizer.batch_decode(generate_ids, skip_special_tokens=True, clean_up_tokenization_spaces=False)[0]
+ "Hey, are you conscious? Can you talk to me?\nI'm not conscious, but I can talk to you."
+ ```"""
+
+ output_attentions = output_attentions if output_attentions is not None else self.config.output_attentions
+ output_hidden_states = (
+ output_hidden_states if output_hidden_states is not None else
+ self.config.output_hidden_states)
+ return_dict = return_dict if return_dict is not None else self.config.use_return_dict
+
+ # decoder outputs consists of (dec_features, layer_state, dec_hidden, dec_attn)
+ outputs = self.model(
+ input_ids=input_ids,
+ attention_mask=attention_mask,
+ position_ids=position_ids,
+ past_key_values=past_key_values,
+ inputs_embeds=inputs_embeds,
+ use_cache=use_cache,
+ output_attentions=output_attentions,
+ output_hidden_states=output_hidden_states,
+ return_dict=return_dict,
+ cache_position=cache_position,
+ )
+
+ hidden_states = outputs[0]
+ if self.config.pretraining_tp > 1:
+ output_slices = self.output.weight.split(
+ self.vocab_size // self.config.pretraining_tp, dim=0)
+ logits = [
+ F.linear(hidden_states, output_slices[i]) # pylint: disable=not-callable
+ for i in range(self.config.pretraining_tp)
+ ]
+ logits = torch.cat(logits, dim=-1)
+ else:
+ logits = self.output(hidden_states)
+ logits = logits.float()
+
+ loss = None
+ if labels is not None:
+ # Shift so that tokens < n predict n
+ shift_logits = logits[..., :-1, :].contiguous()
+ shift_labels = labels[..., 1:].contiguous()
+ # Flatten the tokens
+ loss_fct = CrossEntropyLoss()
+ shift_logits = shift_logits.view(-1, self.config.vocab_size)
+ shift_labels = shift_labels.view(-1)
+ # Enable model parallelism
+ shift_labels = shift_labels.to(shift_logits.device)
+ loss = loss_fct(shift_logits, shift_labels)
+
+ if not return_dict:
+ output = (logits, ) + outputs[1:]
+ return (loss, ) + output if loss is not None else output
+
+ return CausalLMOutputWithPast(
+ loss=loss,
+ logits=logits,
+ past_key_values=outputs.past_key_values,
+ hidden_states=outputs.hidden_states,
+ attentions=outputs.attentions,
+ )
+
+ def prepare_inputs_for_generation(
+ self,
+ input_ids,
+ past_key_values=None,
+ attention_mask=None,
+ inputs_embeds=None,
+ cache_position=None,
+ use_cache=True,
+ **kwargs,
+ ):
+ past_length = 0
+ if past_key_values is not None:
+ if isinstance(past_key_values, Cache):
+ past_length = cache_position[
+ 0] if cache_position is not None else past_key_values.get_seq_length(
+ )
+ max_cache_length = (
+ torch.tensor(
+ past_key_values.get_max_length(),
+ device=input_ids.device)
+ if past_key_values.get_max_length() is not None else None)
+ cache_length = past_length if max_cache_length is None else torch.min(
+ max_cache_length, past_length)
+ # TODO joao: remove this `else` after `generate` prioritizes `Cache` objects
+ else:
+ cache_length = past_length = past_key_values[0][0].shape[2]
+ max_cache_length = None
+
+ # Keep only the unprocessed tokens:
+ # 1 - If the length of the attention_mask exceeds the length of input_ids, then we are in a setting where
+ # some of the inputs are exclusively passed as part of the cache (e.g. when passing input_embeds as input)
+ if attention_mask is not None and attention_mask.shape[
+ 1] > input_ids.shape[1]:
+ input_ids = input_ids[:, -(attention_mask.shape[1] -
+ past_length):]
+ # 2 - If the past_length is smaller than input_ids', then input_ids holds all input tokens. We can discard
+ # input_ids based on the past_length.
+ elif past_length < input_ids.shape[1]:
+ input_ids = input_ids[:, past_length:]
+ # 3 - Otherwise (past_length >= input_ids.shape[1]), let's assume input_ids only has unprocessed tokens.
+
+ # If we are about to go beyond the maximum cache length, we need to crop the input attention mask.
+ if (max_cache_length is not None and attention_mask is not None
+ and cache_length + input_ids.shape[1] > max_cache_length):
+ attention_mask = attention_mask[:, -max_cache_length:] # pylint: disable=E1130
+
+ position_ids = kwargs.get('position_ids', None)
+ if attention_mask is not None and position_ids is None:
+ # create position_ids on the fly for batch generation
+ position_ids = attention_mask.long().cumsum(-1) - 1
+ position_ids.masked_fill_(attention_mask == 0, 1)
+ if past_key_values:
+ position_ids = position_ids[:, -input_ids.shape[1]:]
+
+ # if `inputs_embeds` are passed, we only want to use them in the 1st generation step
+ if inputs_embeds is not None and past_key_values is None:
+ model_inputs = {'inputs_embeds': inputs_embeds}
+ else:
+ # The `contiguous()` here is necessary to have a static stride during decoding. torchdynamo otherwise
+ # recompiles graphs as the stride of the inputs is a guard.
+ # Ref: https://github.com/huggingface/transformers/pull/29114
+ # TODO: use `next_tokens` directly instead.
+ model_inputs = {'input_ids': input_ids.contiguous()}
+
+ input_length = position_ids.shape[
+ -1] if position_ids is not None else input_ids.shape[-1]
+ if cache_position is None:
+ cache_position = torch.arange(
+ past_length,
+ past_length + input_length,
+ device=input_ids.device)
+ elif use_cache:
+ cache_position = cache_position[-input_length:]
+
+ model_inputs.update({
+ 'position_ids': position_ids,
+ 'cache_position': cache_position,
+ 'past_key_values': past_key_values,
+ 'use_cache': use_cache,
+ 'attention_mask': attention_mask,
+ })
+ return model_inputs
+
+ @staticmethod
+ def _reorder_cache(past_key_values, beam_idx):
+ reordered_past = ()
+ for layer_past in past_key_values:
+ reordered_past += (tuple(
+ past_state.index_select(0, beam_idx.to(past_state.device))
+ for past_state in layer_past), )
+ return reordered_past
+
+ def build_inputs(self,
+ tokenizer,
+ query: str,
+ history: List[Tuple[str, str]] = None,
+ meta_instruction=''):
+ if history is None:
+ history = []
+ if tokenizer.add_bos_token:
+ prompt = ''
+ else:
+ prompt = tokenizer.bos_token
+ if meta_instruction:
+ prompt += f"""<|im_start|>system\n{meta_instruction}<|im_end|>\n"""
+ for record in history:
+ prompt += f"""<|im_start|>user\n{record[0]}<|im_end|>\n<|im_start|>assistant\n{record[1]}<|im_end|>\n"""
+ prompt += f"""<|im_start|>user\n{query}<|im_end|>\n<|im_start|>assistant\n"""
+ return tokenizer([prompt], return_tensors='pt')
+
+ @torch.no_grad()
+ def chat(
+ self,
+ tokenizer,
+ query: str,
+ history: Optional[List[Tuple[str, str]]] = None,
+ streamer: Optional[BaseStreamer] = None,
+ max_new_tokens: int = 1024,
+ do_sample: bool = True,
+ temperature: float = 0.8,
+ top_p: float = 0.8,
+ meta_instruction:
+ str = 'You are an AI assistant whose name is InternLM (书生·浦语).\n'
+ '- InternLM (书生·浦语) is a conversational language model that is developed by Shanghai AI Laboratory '
+ '(上海人工智能实验室). It is designed to be helpful, honest, and harmless.\n'
+ '- InternLM (书生·浦语) can understand and communicate fluently in the language chosen by the user such '
+ 'as English and 中文.',
+ **kwargs,
+ ):
+ if history is None:
+ history = []
+ inputs = self.build_inputs(tokenizer, query, history, meta_instruction)
+ inputs = {
+ k: v.to(self.device)
+ for k, v in inputs.items() if torch.is_tensor(v)
+ }
+ # also add end-of-assistant token in eos token id to avoid unnecessary generation
+ eos_token_id = [
+ tokenizer.eos_token_id,
+ tokenizer.convert_tokens_to_ids(['<|im_end|>'])[0]
+ ]
+ outputs = self.generate(
+ **inputs,
+ streamer=streamer,
+ max_new_tokens=max_new_tokens,
+ do_sample=do_sample,
+ temperature=temperature,
+ top_p=top_p,
+ eos_token_id=eos_token_id,
+ **kwargs,
+ )
+ outputs = outputs[0].cpu().tolist()[len(inputs['input_ids'][0]):]
+ response = tokenizer.decode(outputs, skip_special_tokens=True)
+ response = response.split('<|im_end|>')[0]
+ history = history + [(query, response)]
+ return response, history
+
+ @torch.no_grad()
+ def stream_chat(
+ self,
+ tokenizer,
+ query: str,
+ history: List[Tuple[str, str]] = None,
+ max_new_tokens: int = 1024,
+ do_sample: bool = True,
+ temperature: float = 0.8,
+ top_p: float = 0.8,
+ **kwargs,
+ ):
+ if history is None:
+ history = []
+ """
+ Return a generator in format: (response, history)
+ Eg.
+ ('你好,有什么可以帮助您的吗', [('你好', '你好,有什么可以帮助您的吗')])
+ ('你好,有什么可以帮助您的吗?', [('你好', '你好,有什么可以帮助您的吗?')])
+ """
+ if BaseStreamer is None:
+ raise ModuleNotFoundError(
+ 'The version of `transformers` is too low. Please make sure '
+ 'that you have installed `transformers>=4.28.0`.')
+
+ response_queue = queue.Queue(maxsize=20)
+
+ class ChatStreamer(BaseStreamer):
+ """
+ Streamer used in generate to print words one by one.
+ """
+
+ def __init__(self, tokenizer) -> None:
+ super().__init__()
+ self.tokenizer = tokenizer
+ self.queue = response_queue
+ self.query = query
+ self.history = history
+ self.response = ''
+ self.cache = []
+ self.received_inputs = False
+ self.queue.put(
+ (self.response, history + [(self.query, self.response)]))
+
+ def put(self, value):
+ if len(value.shape) > 1 and value.shape[0] > 1:
+ raise ValueError('ChatStreamer only supports batch size 1')
+ elif len(value.shape) > 1:
+ value = value[0]
+
+ if not self.received_inputs:
+ # The first received value is input_ids, ignore here
+ self.received_inputs = True
+ return
+
+ self.cache.extend(value.tolist())
+ token = self.tokenizer.decode(
+ self.cache, skip_special_tokens=True)
+ if token.strip() != '<|im_end|>':
+ self.response = self.response + token
+ history = self.history + [(self.query, self.response)]
+ self.queue.put((self.response, history))
+ self.cache = []
+ else:
+ self.end()
+
+ def end(self):
+ self.queue.put(None)
+
+ def stream_producer():
+ return self.chat(
+ tokenizer=tokenizer,
+ query=query,
+ streamer=ChatStreamer(tokenizer=tokenizer),
+ history=history,
+ max_new_tokens=max_new_tokens,
+ do_sample=do_sample,
+ temperature=temperature,
+ top_p=top_p,
+ **kwargs,
+ )
+
+ def consumer():
+ producer = threading.Thread(target=stream_producer)
+ producer.start()
+ while True:
+ res = response_queue.get()
+ if res is None:
+ return
+ yield res
+
+ return consumer()
+
+
+# Copied from transformers.models.llama.modeling_llama.LlamaForSequenceClassification with Llama->InternLM2
+@add_start_docstrings(
+ """
+ The InternLM2 Model transformer with a sequence classification head on top (linear layer).
+ [`InternLM2ForSequenceClassification`] uses the last token in order to do the classification, as other causal models
+ (e.g. GPT-2) do.
+ Since it does classification on the last token, it requires to know the position of the last token. If a
+ `pad_token_id` is defined in the configuration, it finds the last token that is not a padding token in each row. If
+ no `pad_token_id` is defined, it simply takes the last value in each row of the batch. Since it cannot guess the
+ padding tokens when `inputs_embeds` are passed instead of `input_ids`, it does the same (take the last value in
+ each row of the batch).
+ """,
+ InternLM2_START_DOCSTRING,
+)
+class InternLM2ForSequenceClassification(InternLM2PreTrainedModel):
+ """Sequence Classification Head for InternLM2 Model."""
+
+ def __init__(self, config):
+ super().__init__(config)
+ self.num_labels = config.num_labels
+ self.model = InternLM2Model(config)
+ self.score = nn.Linear(config.hidden_size, self.num_labels, bias=False)
+
+ # Initialize weights and apply final processing
+ self.post_init()
+
+ def get_input_embeddings(self):
+ return self.model.tok_embeddings
+
+ def set_input_embeddings(self, value):
+ self.model.tok_embeddings = value
+
+ @add_start_docstrings_to_model_forward(InternLM2_INPUTS_DOCSTRING)
+ def forward(
+ self,
+ input_ids: torch.LongTensor = None,
+ attention_mask: Optional[torch.Tensor] = None,
+ position_ids: Optional[torch.LongTensor] = None,
+ past_key_values: Optional[Union[Cache,
+ List[torch.FloatTensor]]] = None,
+ inputs_embeds: Optional[torch.FloatTensor] = None,
+ labels: Optional[torch.LongTensor] = None,
+ use_cache: Optional[bool] = None,
+ output_attentions: Optional[bool] = None,
+ output_hidden_states: Optional[bool] = None,
+ return_dict: Optional[bool] = None,
+ ) -> Union[Tuple, SequenceClassifierOutputWithPast]:
+ r"""
+ labels (`torch.LongTensor` of shape `(batch_size,)`, *optional*):
+ Labels for computing the sequence classification/regression loss. Indices should be in `[0, ...,
+ config.num_labels - 1]`. If `config.num_labels == 1` a regression loss is computed (Mean-Square loss), If
+ `config.num_labels > 1` a classification loss is computed (Cross-Entropy).
+ """
+ return_dict = return_dict if return_dict is not None else self.config.use_return_dict
+
+ transformer_outputs = self.model(
+ input_ids,
+ attention_mask=attention_mask,
+ position_ids=position_ids,
+ past_key_values=past_key_values,
+ inputs_embeds=inputs_embeds,
+ use_cache=use_cache,
+ output_attentions=output_attentions,
+ output_hidden_states=output_hidden_states,
+ return_dict=return_dict,
+ )
+ hidden_states = transformer_outputs[0]
+ logits = self.score(hidden_states)
+
+ if input_ids is not None:
+ batch_size = input_ids.shape[0]
+ else:
+ batch_size = inputs_embeds.shape[0]
+
+ if self.config.pad_token_id is None and batch_size != 1:
+ raise ValueError(
+ 'Cannot handle batch sizes > 1 if no padding token is defined.'
+ )
+ if self.config.pad_token_id is None:
+ sequence_lengths = -1
+ else:
+ if input_ids is not None:
+ # if no pad token found, use modulo instead of reverse indexing for ONNX compatibility
+ sequence_lengths = torch.eq(
+ input_ids, self.config.pad_token_id).int().argmax(-1) - 1
+ sequence_lengths = sequence_lengths % input_ids.shape[-1]
+ sequence_lengths = sequence_lengths.to(logits.device)
+ else:
+ sequence_lengths = -1
+
+ pooled_logits = logits[torch.arange(batch_size, device=logits.device),
+ sequence_lengths]
+
+ loss = None
+ if labels is not None:
+ labels = labels.to(logits.device)
+ if self.config.problem_type is None:
+ if self.num_labels == 1:
+ self.config.problem_type = 'regression'
+ elif self.num_labels > 1 and (labels.dtype
+ in (torch.long, torch.int)):
+ self.config.problem_type = 'single_label_classification'
+ else:
+ self.config.problem_type = 'multi_label_classification'
+
+ if self.config.problem_type == 'regression':
+ loss_fct = MSELoss()
+ if self.num_labels == 1:
+ loss = loss_fct(pooled_logits.squeeze(), labels.squeeze())
+ else:
+ loss = loss_fct(pooled_logits, labels)
+ elif self.config.problem_type == 'single_label_classification':
+ loss_fct = CrossEntropyLoss()
+ loss = loss_fct(
+ pooled_logits.view(-1, self.num_labels), labels.view(-1))
+ elif self.config.problem_type == 'multi_label_classification':
+ loss_fct = BCEWithLogitsLoss()
+ loss = loss_fct(pooled_logits, labels)
+ if not return_dict:
+ output = (pooled_logits, ) + transformer_outputs[1:]
+ return ((loss, ) + output) if loss is not None else output
+
+ return SequenceClassifierOutputWithPast(
+ loss=loss,
+ logits=pooled_logits,
+ past_key_values=transformer_outputs.past_key_values,
+ hidden_states=transformer_outputs.hidden_states,
+ attentions=transformer_outputs.attentions,
+ )
+
+
+# Copied from transformers.models.llama.modeling_llama.LlamaForQuestionAnswering with Llama->InternLM2
+@add_start_docstrings(
+ """
+The InternLM2 Model transformer with a span classification head on top for extractive question-answering tasks like
+SQuAD (a linear layer on top of the hidden-states output to compute `span start logits` and `span end logits`).
+ """,
+ InternLM2_START_DOCSTRING,
+)
+class InternLM2ForQuestionAnswering(InternLM2PreTrainedModel):
+ """Question Answering model for InternLM2."""
+
+ base_model_prefix = 'transformer'
+
+ def __init__(self, config):
+ super().__init__(config)
+ self.transformer = InternLM2Model(config)
+ self.qa_outputs = nn.Linear(config.hidden_size, 2)
+
+ # Initialize weights and apply final processing
+ self.post_init()
+
+ def get_input_embeddings(self):
+ return self.transformer.tok_embeddings
+
+ def set_input_embeddings(self, value):
+ self.transformer.tok_embeddings = value
+
+ @add_start_docstrings_to_model_forward(InternLM2_INPUTS_DOCSTRING)
+ def forward(
+ self,
+ input_ids: Optional[torch.LongTensor] = None,
+ attention_mask: Optional[torch.FloatTensor] = None,
+ position_ids: Optional[torch.LongTensor] = None,
+ past_key_values: Optional[Union[Cache,
+ List[torch.FloatTensor]]] = None,
+ inputs_embeds: Optional[torch.FloatTensor] = None,
+ start_positions: Optional[torch.LongTensor] = None,
+ end_positions: Optional[torch.LongTensor] = None,
+ output_attentions: Optional[bool] = None,
+ output_hidden_states: Optional[bool] = None,
+ return_dict: Optional[bool] = None,
+ ) -> Union[Tuple, QuestionAnsweringModelOutput]:
+ r"""
+ start_positions (`torch.LongTensor` of shape `(batch_size,)`, *optional*):
+ Labels for position (index) of the start of the labelled span for computing the token classification loss.
+ Positions are clamped to the length of the sequence (`sequence_length`). Position outside of the sequence
+ are not taken into account for computing the loss.
+ end_positions (`torch.LongTensor` of shape `(batch_size,)`, *optional*):
+ Labels for position (index) of the end of the labelled span for computing the token classification loss.
+ Positions are clamped to the length of the sequence (`sequence_length`). Position outside of the sequence
+ are not taken into account for computing the loss.
+ """
+ return_dict = return_dict if return_dict is not None else self.config.use_return_dict
+
+ outputs = self.transformer(
+ input_ids,
+ attention_mask=attention_mask,
+ position_ids=position_ids,
+ past_key_values=past_key_values,
+ inputs_embeds=inputs_embeds,
+ output_attentions=output_attentions,
+ output_hidden_states=output_hidden_states,
+ return_dict=return_dict,
+ )
+
+ sequence_output = outputs[0]
+
+ logits = self.qa_outputs(sequence_output)
+ start_logits, end_logits = logits.split(1, dim=-1)
+ start_logits = start_logits.squeeze(-1).contiguous()
+ end_logits = end_logits.squeeze(-1).contiguous()
+
+ total_loss = None
+ if start_positions is not None and end_positions is not None:
+ # If we are on multi-GPU, split add a dimension
+ if len(start_positions.size()) > 1:
+ start_positions = start_positions.squeeze(-1).to(
+ start_logits.device)
+ if len(end_positions.size()) > 1:
+ end_positions = end_positions.squeeze(-1).to(end_logits.device)
+ # sometimes the start/end positions are outside our model inputs, we ignore these terms
+ ignored_index = start_logits.size(1)
+ start_positions = start_positions.clamp(0, ignored_index)
+ end_positions = end_positions.clamp(0, ignored_index)
+
+ loss_fct = CrossEntropyLoss(ignore_index=ignored_index)
+ start_loss = loss_fct(start_logits, start_positions)
+ end_loss = loss_fct(end_logits, end_positions)
+ total_loss = (start_loss + end_loss) / 2
+
+ if not return_dict:
+ output = (start_logits, end_logits) + outputs[2:]
+ return ((total_loss, ) +
+ output) if total_loss is not None else output
+
+ return QuestionAnsweringModelOutput(
+ loss=total_loss,
+ start_logits=start_logits,
+ end_logits=end_logits,
+ hidden_states=outputs.hidden_states,
+ attentions=outputs.attentions,
+ )
+
+
+# Copied from transformers.models.llama.modeling_llama.LlamaForTokenClassification with Llama->InternLM2
+@add_start_docstrings(
+ """
+ The InternLM2 Model transformer with a token classification head on top (a linear layer on top of the hidden-states
+ output) e.g. for Named-Entity-Recognition (NER) tasks.
+ """,
+ InternLM2_START_DOCSTRING,
+)
+class InternLM2ForTokenClassification(InternLM2PreTrainedModel):
+ """Token classification model for InternLM2."""
+
+ def __init__(self, config):
+ super().__init__(config)
+ self.num_labels = config.num_labels
+ self.model = InternLM2Model(config)
+ if getattr(config, 'classifier_dropout', None) is not None:
+ classifier_dropout = config.classifier_dropout
+ elif getattr(config, 'hidden_dropout', None) is not None:
+ classifier_dropout = config.hidden_dropout
+ else:
+ classifier_dropout = 0.1
+ self.dropout = nn.Dropout(classifier_dropout)
+ self.score = nn.Linear(config.hidden_size, config.num_labels)
+
+ # Initialize weights and apply final processing
+ self.post_init()
+
+ def get_input_embeddings(self):
+ return self.model.tok_embeddings
+
+ def set_input_embeddings(self, value):
+ self.model.tok_embeddings = value
+
+ @add_start_docstrings_to_model_forward(InternLM2_INPUTS_DOCSTRING)
+ def forward(
+ self,
+ input_ids: torch.LongTensor = None,
+ attention_mask: Optional[torch.Tensor] = None,
+ position_ids: Optional[torch.LongTensor] = None,
+ past_key_values: Optional[List[torch.FloatTensor]] = None,
+ inputs_embeds: Optional[torch.FloatTensor] = None,
+ labels: Optional[torch.LongTensor] = None,
+ use_cache: Optional[bool] = None,
+ output_attentions: Optional[bool] = None,
+ output_hidden_states: Optional[bool] = None,
+ return_dict: Optional[bool] = None,
+ ) -> Union[Tuple, SequenceClassifierOutputWithPast]:
+ r"""
+ labels (`torch.LongTensor` of shape `(batch_size,)`, *optional*):
+ Labels for computing the sequence classification/regression loss. Indices should be in `[0, ...,
+ config.num_labels - 1]`. If `config.num_labels == 1` a regression loss is computed (Mean-Square loss), If
+ `config.num_labels > 1` a classification loss is computed (Cross-Entropy).
+ """
+ return_dict = return_dict if return_dict is not None else self.config.use_return_dict
+
+ outputs = self.model(
+ input_ids,
+ attention_mask=attention_mask,
+ position_ids=position_ids,
+ past_key_values=past_key_values,
+ inputs_embeds=inputs_embeds,
+ use_cache=use_cache,
+ output_attentions=output_attentions,
+ output_hidden_states=output_hidden_states,
+ return_dict=return_dict,
+ )
+ sequence_output = outputs[0]
+ sequence_output = self.dropout(sequence_output)
+ logits = self.score(sequence_output)
+
+ loss = None
+ if labels is not None:
+ loss_fct = CrossEntropyLoss()
+ loss = loss_fct(logits.view(-1, self.num_labels), labels.view(-1))
+
+ if not return_dict:
+ output = (logits, ) + outputs[2:]
+ return ((loss, ) + output) if loss is not None else output
+
+ return TokenClassifierOutput(
+ loss=loss,
+ logits=logits,
+ hidden_states=outputs.hidden_states,
+ attentions=outputs.attentions,
+ )
diff --git a/xtuner/_lite/modelings/internlm3/__init__.py b/xtuner/_lite/modelings/internlm3/__init__.py
new file mode 100644
index 000000000..a228b2903
--- /dev/null
+++ b/xtuner/_lite/modelings/internlm3/__init__.py
@@ -0,0 +1,3 @@
+from .configuration_internlm3 import InternLM3Config
+from .modeling_internlm3 import InternLM3ForCausalLM
+from .tokenization_internlm3 import InternLM3Tokenizer
diff --git a/xtuner/_lite/modelings/internlm3/configuration_internlm3.py b/xtuner/_lite/modelings/internlm3/configuration_internlm3.py
new file mode 100644
index 000000000..d9f03eeb9
--- /dev/null
+++ b/xtuner/_lite/modelings/internlm3/configuration_internlm3.py
@@ -0,0 +1,197 @@
+# coding=utf-8
+# Copyright (c) The InternLM team and The HuggingFace Inc. team. All rights reserved.
+#
+# This code is based on transformers/src/transformers/models/llama/configuration_llama.py
+#
+# Licensed under the Apache License, Version 2.0 (the "License");
+# you may not use this file except in compliance with the License.
+# You may obtain a copy of the License at
+#
+# http://www.apache.org/licenses/LICENSE-2.0
+#
+# Unless required by applicable law or agreed to in writing, software
+# distributed under the License is distributed on an "AS IS" BASIS,
+# WITHOUT WARRANTIES OR CONDITIONS OF ANY KIND, either express or implied.
+# See the License for the specific language governing permissions and
+# limitations under the License.
+""" InternLM3 model configuration"""
+
+from transformers.configuration_utils import PretrainedConfig
+from transformers.modeling_rope_utils import rope_config_validation
+from transformers.utils import logging
+
+
+logger = logging.get_logger(__name__)
+
+
+class InternLM3Config(PretrainedConfig):
+ r"""
+ This is the configuration class to store the configuration of a [`InternLM2Model`]. It is used to instantiate
+ an InternLM2 model according to the specified arguments, defining the model architecture. Instantiating a
+ configuration with the defaults will yield a similar configuration to that of the InternLM2-7B.
+
+ Configuration objects inherit from [`PretrainedConfig`] and can be used to control the model outputs. Read the
+ documentation from [`PretrainedConfig`] for more information.
+
+
+ Args:
+ vocab_size (`int`, *optional*, defaults to 151936):
+ Vocabulary size of the InternLM3 model. Defines the number of different tokens that can be represented by the
+ `inputs_ids` passed when calling [`InternLM3Model`]
+ hidden_size (`int`, *optional*, defaults to 4096):
+ Dimension of the hidden representations.
+ intermediate_size (`int`, *optional*, defaults to 22016):
+ Dimension of the MLP representations.
+ num_hidden_layers (`int`, *optional*, defaults to 32):
+ Number of hidden layers in the Transformer encoder.
+ num_attention_heads (`int`, *optional*, defaults to 32):
+ Number of attention heads for each attention layer in the Transformer encoder.
+ num_key_value_heads (`int`, *optional*, defaults to 32):
+ This is the number of key_value heads that should be used to implement Grouped Query Attention. If
+ `num_key_value_heads=num_attention_heads`, the model will use Multi Head Attention (MHA), if
+ `num_key_value_heads=1` the model will use Multi Query Attention (MQA) otherwise GQA is used. When
+ converting a multi-head checkpoint to a GQA checkpoint, each group key and value head should be constructed
+ by meanpooling all the original heads within that group. For more details checkout [this
+ paper](https://arxiv.org/pdf/2305.13245.pdf). If it is not specified, will default to `32`.
+ hidden_act (`str` or `function`, *optional*, defaults to `"silu"`):
+ The non-linear activation function (function or string) in the decoder.
+ max_position_embeddings (`int`, *optional*, defaults to 32768):
+ The maximum sequence length that this model might ever be used with.
+ initializer_range (`float`, *optional*, defaults to 0.02):
+ The standard deviation of the truncated_normal_initializer for initializing all weight matrices.
+ rms_norm_eps (`float`, *optional*, defaults to 1e-06):
+ The epsilon used by the rms normalization layers.
+ use_cache (`bool`, *optional*, defaults to `True`):
+ Whether or not the model should return the last key/values attentions (not used by all models). Only
+ relevant if `config.is_decoder=True`.
+ tie_word_embeddings (`bool`, *optional*, defaults to `False`):
+ Whether the model's input and output word embeddings should be tied.
+ rope_theta (`float`, *optional*, defaults to 10000.0):
+ The base period of the RoPE embeddings.
+ rope_scaling (`Dict`, *optional*):
+ Dictionary containing the scaling configuration for the RoPE embeddings. NOTE: if you apply new rope type
+ and you expect the model to work on longer `max_position_embeddings`, we recommend you to update this value
+ accordingly.
+ Expected contents:
+ `rope_type` (`str`):
+ The sub-variant of RoPE to use. Can be one of ['default', 'linear', 'dynamic', 'yarn', 'longrope',
+ 'llama3'], with 'default' being the original RoPE implementation.
+ `factor` (`float`, *optional*):
+ Used with all rope types except 'default'. The scaling factor to apply to the RoPE embeddings. In
+ most scaling types, a `factor` of x will enable the model to handle sequences of length x *
+ original maximum pre-trained length.
+ `original_max_position_embeddings` (`int`, *optional*):
+ Used with 'dynamic', 'longrope' and 'llama3'. The original max position embeddings used during
+ pretraining.
+ `attention_factor` (`float`, *optional*):
+ Used with 'yarn' and 'longrope'. The scaling factor to be applied on the attention
+ computation. If unspecified, it defaults to value recommended by the implementation, using the
+ `factor` field to infer the suggested value.
+ `beta_fast` (`float`, *optional*):
+ Only used with 'yarn'. Parameter to set the boundary for extrapolation (only) in the linear
+ ramp function. If unspecified, it defaults to 32.
+ `beta_slow` (`float`, *optional*):
+ Only used with 'yarn'. Parameter to set the boundary for interpolation (only) in the linear
+ ramp function. If unspecified, it defaults to 1.
+ `short_factor` (`List[float]`, *optional*):
+ Only used with 'longrope'. The scaling factor to be applied to short contexts (<
+ `original_max_position_embeddings`). Must be a list of numbers with the same length as the hidden
+ size divided by the number of attention heads divided by 2
+ `long_factor` (`List[float]`, *optional*):
+ Only used with 'longrope'. The scaling factor to be applied to long contexts (<
+ `original_max_position_embeddings`). Must be a list of numbers with the same length as the hidden
+ size divided by the number of attention heads divided by 2
+ `low_freq_factor` (`float`, *optional*):
+ Only used with 'llama3'. Scaling factor applied to low frequency components of the RoPE
+ `high_freq_factor` (`float`, *optional*):
+ Only used with 'llama3'. Scaling factor applied to high frequency components of the RoPE
+ qkv_bias (`bool`, *optional*, defaults to `False`):
+ Whether to use a bias in the query, key and value projection layers during self-attention.
+ attention_dropout (`float`, *optional*, defaults to 0.0):
+ The dropout ratio for the attention probabilities.
+ bias (`bool`, *optional*, defaults to `False`):
+ Whether to use a bias in o_proj, up_proj, down_proj and gate_proj layers.
+ head_dim (`int`, *optional*):
+ The attention head dimension. If None, it will default to hidden_size // num_heads
+
+ ```python
+ >>> from transformers import InternLM3Model, InternLM3Config
+
+ >>> # Initializing a InternLM3 style configuration
+ >>> configuration = InternLM3Config()
+
+ >>> # Initializing a model from the InternLM3-8B style configuration
+ >>> model = InternLM3Model(configuration)
+
+ >>> # Accessing the model configuration
+ >>> configuration = model.config
+ ```"""
+
+ model_type = "internlm3"
+ keys_to_ignore_at_inference = ["past_key_values"]
+
+ # Default tensor parallel plan for base model `InternLM3`
+ base_model_tp_plan = {
+ "layers.*.self_attn.q_proj": "colwise",
+ "layers.*.self_attn.k_proj": "colwise",
+ "layers.*.self_attn.v_proj": "colwise",
+ "layers.*.self_attn.o_proj": "rowwise",
+ "layers.*.mlp.gate_proj": "colwise",
+ "layers.*.mlp.up_proj": "colwise",
+ "layers.*.mlp.down_proj": "rowwise",
+ }
+
+ def __init__(
+ self,
+ vocab_size=128512,
+ hidden_size=4096,
+ intermediate_size=11008,
+ num_hidden_layers=32,
+ num_attention_heads=32,
+ num_key_value_heads=32,
+ hidden_act="silu",
+ max_position_embeddings=32768,
+ initializer_range=0.02,
+ rms_norm_eps=1e-6,
+ use_cache=True,
+ tie_word_embeddings=False,
+ rope_theta=10000.0,
+ rope_scaling=None,
+ qkv_bias=False,
+ attention_dropout=0.0,
+ bias=False,
+ head_dim=None,
+ **kwargs,
+ ):
+ self.vocab_size = vocab_size
+ self.max_position_embeddings = max_position_embeddings
+ self.hidden_size = hidden_size
+ self.intermediate_size = intermediate_size
+ self.num_hidden_layers = num_hidden_layers
+ self.num_attention_heads = num_attention_heads
+
+ # for backward compatibility
+ if num_key_value_heads is None:
+ num_key_value_heads = num_attention_heads
+
+ self.num_key_value_heads = num_key_value_heads
+ self.hidden_act = hidden_act
+ self.initializer_range = initializer_range
+ self.rms_norm_eps = rms_norm_eps
+ self.use_cache = use_cache
+ self.rope_theta = rope_theta
+ self.rope_scaling = rope_scaling
+ self.qkv_bias = qkv_bias
+ self.attention_dropout = attention_dropout
+ self.bias = bias
+ self.head_dim = head_dim if head_dim is not None else self.hidden_size // self.num_attention_heads
+ # Validate the correctness of rotary position embeddings parameters
+ # BC: if there is a 'type' field, move it to 'rope_type'.
+ if self.rope_scaling is not None and "type" in self.rope_scaling:
+ self.rope_scaling["rope_type"] = self.rope_scaling["type"]
+ rope_config_validation(self)
+
+ super().__init__(
+ tie_word_embeddings=tie_word_embeddings,
+ **kwargs,
+ )
diff --git a/xtuner/_lite/modelings/internlm3/modeling_internlm3.py b/xtuner/_lite/modelings/internlm3/modeling_internlm3.py
new file mode 100644
index 000000000..b102707c2
--- /dev/null
+++ b/xtuner/_lite/modelings/internlm3/modeling_internlm3.py
@@ -0,0 +1,824 @@
+# 🚨🚨🚨🚨🚨🚨🚨🚨🚨🚨🚨🚨🚨🚨🚨🚨🚨🚨🚨🚨🚨🚨🚨🚨🚨🚨🚨🚨🚨🚨🚨🚨🚨🚨🚨🚨🚨🚨🚨🚨🚨🚨🚨🚨🚨🚨🚨🚨
+# This file was automatically generated from src/transformers/models/internlm3/modular_internlm3.py.
+# Do NOT edit this file manually as any edits will be overwritten by the generation of
+# the file from the modular. If any change should be done, please apply the change to the
+# modular_internlm3.py file directly. One of our CI enforces this.
+# 🚨🚨🚨🚨🚨🚨🚨🚨🚨🚨🚨🚨🚨🚨🚨🚨🚨🚨🚨🚨🚨🚨🚨🚨🚨🚨🚨🚨🚨🚨🚨🚨🚨🚨🚨🚨🚨🚨🚨🚨🚨🚨🚨🚨🚨🚨🚨🚨
+from typing import Callable, List, Optional, Tuple, Union
+
+import torch
+from torch import nn
+
+from transformers.utils import logging
+
+from transformers.activations import ACT2FN
+from transformers.cache_utils import Cache, DynamicCache, StaticCache
+from transformers.generation import GenerationMixin
+from transformers.modeling_attn_mask_utils import AttentionMaskConverter
+from transformers.modeling_flash_attention_utils import FlashAttentionKwargs
+from transformers.modeling_outputs import BaseModelOutputWithPast, CausalLMOutputWithPast
+from transformers.modeling_rope_utils import ROPE_INIT_FUNCTIONS
+from transformers.modeling_utils import ALL_ATTENTION_FUNCTIONS, PreTrainedModel
+from transformers.processing_utils import Unpack
+from transformers.utils import LossKwargs, add_start_docstrings, add_start_docstrings_to_model_forward, replace_return_docstrings
+from .configuration_internlm3 import InternLM3Config
+
+
+logger = logging.get_logger(__name__)
+_CONFIG_FOR_DOC = "InternLM3Config"
+
+
+class InternLM3MLP(nn.Module):
+ def __init__(self, config):
+ super().__init__()
+ self.config = config
+ self.hidden_size = config.hidden_size
+ self.intermediate_size = config.intermediate_size
+ self.gate_proj = nn.Linear(self.hidden_size, self.intermediate_size, bias=config.bias)
+ self.up_proj = nn.Linear(self.hidden_size, self.intermediate_size, bias=config.bias)
+ self.down_proj = nn.Linear(self.intermediate_size, self.hidden_size, bias=config.bias)
+ self.act_fn = ACT2FN[config.hidden_act]
+
+ def forward(self, x):
+ down_proj = self.down_proj(self.act_fn(self.gate_proj(x)) * self.up_proj(x))
+ return down_proj
+
+
+def rotate_half(x):
+ """Rotates half the hidden dims of the input."""
+ x1 = x[..., : x.shape[-1] // 2]
+ x2 = x[..., x.shape[-1] // 2 :]
+ return torch.cat((-x2, x1), dim=-1)
+
+
+def apply_rotary_pos_emb(q, k, cos, sin, position_ids=None, unsqueeze_dim=1):
+ """Applies Rotary Position Embedding to the query and key tensors.
+
+ Args:
+ q (`torch.Tensor`): The query tensor.
+ k (`torch.Tensor`): The key tensor.
+ cos (`torch.Tensor`): The cosine part of the rotary embedding.
+ sin (`torch.Tensor`): The sine part of the rotary embedding.
+ position_ids (`torch.Tensor`, *optional*):
+ Deprecated and unused.
+ unsqueeze_dim (`int`, *optional*, defaults to 1):
+ The 'unsqueeze_dim' argument specifies the dimension along which to unsqueeze cos[position_ids] and
+ sin[position_ids] so that they can be properly broadcasted to the dimensions of q and k. For example, note
+ that cos[position_ids] and sin[position_ids] have the shape [batch_size, seq_len, head_dim]. Then, if q and
+ k have the shape [batch_size, heads, seq_len, head_dim], then setting unsqueeze_dim=1 makes
+ cos[position_ids] and sin[position_ids] broadcastable to the shapes of q and k. Similarly, if q and k have
+ the shape [batch_size, seq_len, heads, head_dim], then set unsqueeze_dim=2.
+ Returns:
+ `tuple(torch.Tensor)` comprising of the query and key tensors rotated using the Rotary Position Embedding.
+ """
+ cos = cos.unsqueeze(unsqueeze_dim)
+ sin = sin.unsqueeze(unsqueeze_dim)
+ q_embed = (q * cos) + (rotate_half(q) * sin)
+ k_embed = (k * cos) + (rotate_half(k) * sin)
+ return q_embed, k_embed
+
+
+def repeat_kv(hidden_states: torch.Tensor, n_rep: int) -> torch.Tensor:
+ """
+ This is the equivalent of torch.repeat_interleave(x, dim=1, repeats=n_rep). The hidden states go from (batch,
+ num_key_value_heads, seqlen, head_dim) to (batch, num_attention_heads, seqlen, head_dim)
+ """
+ batch, num_key_value_heads, slen, head_dim = hidden_states.shape
+ if n_rep == 1:
+ return hidden_states
+ hidden_states = hidden_states[:, :, None, :, :].expand(batch, num_key_value_heads, n_rep, slen, head_dim)
+ return hidden_states.reshape(batch, num_key_value_heads * n_rep, slen, head_dim)
+
+
+def eager_attention_forward(
+ module: nn.Module,
+ query: torch.Tensor,
+ key: torch.Tensor,
+ value: torch.Tensor,
+ attention_mask: Optional[torch.Tensor],
+ scaling: float,
+ dropout: float = 0.0,
+ **kwargs,
+):
+ key_states = repeat_kv(key, module.num_key_value_groups)
+ value_states = repeat_kv(value, module.num_key_value_groups)
+
+ attn_weights = torch.matmul(query, key_states.transpose(2, 3)) * scaling
+ if attention_mask is not None:
+ causal_mask = attention_mask[:, :, :, : key_states.shape[-2]]
+ attn_weights = attn_weights + causal_mask
+
+ attn_weights = nn.functional.softmax(attn_weights, dim=-1, dtype=torch.float32).to(query.dtype)
+ attn_weights = nn.functional.dropout(attn_weights, p=dropout, training=module.training)
+ attn_output = torch.matmul(attn_weights, value_states)
+ attn_output = attn_output.transpose(1, 2).contiguous()
+
+ return attn_output, attn_weights
+
+
+class InternLM3Attention(nn.Module):
+ """Multi-headed attention from 'Attention Is All You Need' paper"""
+
+ def __init__(self, config: InternLM3Config, layer_idx: int):
+ super().__init__()
+ self.config = config
+ self.layer_idx = layer_idx
+ self.head_dim = getattr(config, "head_dim", config.hidden_size // config.num_attention_heads)
+ self.num_key_value_groups = config.num_attention_heads // config.num_key_value_heads
+ self.scaling = self.head_dim**-0.5
+ self.attention_dropout = config.attention_dropout
+ self.is_causal = True
+ self.q_proj = nn.Linear(config.hidden_size, config.num_attention_heads * self.head_dim, bias=config.qkv_bias)
+ self.k_proj = nn.Linear(config.hidden_size, config.num_key_value_heads * self.head_dim, bias=config.qkv_bias)
+ self.v_proj = nn.Linear(config.hidden_size, config.num_key_value_heads * self.head_dim, bias=config.qkv_bias)
+ self.o_proj = nn.Linear(config.num_attention_heads * self.head_dim, config.hidden_size, bias=config.bias)
+
+ def forward(
+ self,
+ hidden_states: torch.Tensor,
+ position_embeddings: Tuple[torch.Tensor, torch.Tensor],
+ attention_mask: Optional[torch.Tensor],
+ past_key_value: Optional[Cache] = None,
+ cache_position: Optional[torch.LongTensor] = None,
+ **kwargs: Unpack[FlashAttentionKwargs],
+ ) -> Tuple[torch.Tensor, Optional[torch.Tensor], Optional[Tuple[torch.Tensor]]]:
+ input_shape = hidden_states.shape[:-1]
+ hidden_shape = (*input_shape, -1, self.head_dim)
+
+ query_states = self.q_proj(hidden_states).view(hidden_shape).transpose(1, 2)
+ key_states = self.k_proj(hidden_states).view(hidden_shape).transpose(1, 2)
+ value_states = self.v_proj(hidden_states).view(hidden_shape).transpose(1, 2)
+
+ cos, sin = position_embeddings
+ query_states, key_states = apply_rotary_pos_emb(query_states, key_states, cos, sin)
+
+ if past_key_value is not None:
+ # sin and cos are specific to RoPE models; cache_position needed for the static cache
+ cache_kwargs = {"sin": sin, "cos": cos, "cache_position": cache_position}
+ key_states, value_states = past_key_value.update(key_states, value_states, self.layer_idx, cache_kwargs)
+
+ attention_interface: Callable = eager_attention_forward
+ if self.config._attn_implementation != "eager":
+ if self.config._attn_implementation == "sdpa" and kwargs.get("output_attentions", False):
+ logger.warning_once(
+ "`torch.nn.functional.scaled_dot_product_attention` does not support `output_attentions=True`. Falling back to "
+ 'eager attention. This warning can be removed using the argument `attn_implementation="eager"` when loading the model.'
+ )
+ else:
+ attention_interface = ALL_ATTENTION_FUNCTIONS[self.config._attn_implementation]
+
+ attn_output, attn_weights = attention_interface(
+ self,
+ query_states,
+ key_states,
+ value_states,
+ attention_mask,
+ dropout=0.0 if not self.training else self.attention_dropout,
+ scaling=self.scaling,
+ **kwargs,
+ )
+
+ attn_output = attn_output.reshape(*input_shape, -1).contiguous()
+ attn_output = self.o_proj(attn_output)
+ return attn_output, attn_weights
+
+
+class InternLM3RMSNorm(nn.Module):
+ def __init__(self, hidden_size, eps=1e-6):
+ """
+ InternLM3RMSNorm is equivalent to T5LayerNorm
+ """
+ super().__init__()
+ self.weight = nn.Parameter(torch.ones(hidden_size))
+ self.variance_epsilon = eps
+
+ def forward(self, hidden_states):
+ input_dtype = hidden_states.dtype
+ hidden_states = hidden_states.to(torch.float32)
+ variance = hidden_states.pow(2).mean(-1, keepdim=True)
+ hidden_states = hidden_states * torch.rsqrt(variance + self.variance_epsilon)
+ return self.weight * hidden_states.to(input_dtype)
+
+ def extra_repr(self):
+ return f"{tuple(self.weight.shape)}, eps={self.variance_epsilon}"
+
+
+class InternLM3DecoderLayer(nn.Module):
+ def __init__(self, config: InternLM3Config, layer_idx: int):
+ super().__init__()
+ self.hidden_size = config.hidden_size
+ self.self_attn = InternLM3Attention(config=config, layer_idx=layer_idx)
+ self.mlp = InternLM3MLP(config)
+ self.input_layernorm = InternLM3RMSNorm(config.hidden_size, eps=config.rms_norm_eps)
+ self.post_attention_layernorm = InternLM3RMSNorm(config.hidden_size, eps=config.rms_norm_eps)
+
+ def forward(
+ self,
+ hidden_states: torch.Tensor,
+ attention_mask: Optional[torch.Tensor] = None,
+ position_ids: Optional[torch.LongTensor] = None,
+ past_key_value: Optional[Cache] = None,
+ output_attentions: Optional[bool] = False,
+ use_cache: Optional[bool] = False,
+ cache_position: Optional[torch.LongTensor] = None,
+ position_embeddings: Optional[Tuple[torch.Tensor, torch.Tensor]] = None, # necessary, but kept here for BC
+ **kwargs: Unpack[FlashAttentionKwargs],
+ ) -> Tuple[torch.FloatTensor, Optional[Tuple[torch.FloatTensor, torch.FloatTensor]]]:
+ residual = hidden_states
+
+ hidden_states = self.input_layernorm(hidden_states)
+
+ # Self Attention
+ hidden_states, self_attn_weights = self.self_attn(
+ hidden_states=hidden_states,
+ attention_mask=attention_mask,
+ position_ids=position_ids,
+ past_key_value=past_key_value,
+ output_attentions=output_attentions,
+ use_cache=use_cache,
+ cache_position=cache_position,
+ position_embeddings=position_embeddings,
+ **kwargs,
+ )
+ hidden_states = residual + hidden_states
+
+ # Fully Connected
+ residual = hidden_states
+ hidden_states = self.post_attention_layernorm(hidden_states)
+ hidden_states = self.mlp(hidden_states)
+ hidden_states = residual + hidden_states
+
+ outputs = (hidden_states,)
+ if output_attentions:
+ outputs += (self_attn_weights,)
+
+ return outputs
+
+
+class InternLM3RotaryEmbedding(nn.Module):
+ def __init__(self, config: InternLM3Config, device=None):
+ super().__init__()
+ # BC: "rope_type" was originally "type"
+ if hasattr(config, "rope_scaling") and config.rope_scaling is not None:
+ self.rope_type = config.rope_scaling.get("rope_type", config.rope_scaling.get("type"))
+ else:
+ self.rope_type = "default"
+ self.max_seq_len_cached = config.max_position_embeddings
+ self.original_max_seq_len = config.max_position_embeddings
+
+ self.config = config
+ self.rope_init_fn = ROPE_INIT_FUNCTIONS[self.rope_type]
+
+ inv_freq, self.attention_scaling = self.rope_init_fn(self.config, device)
+ self.register_buffer("inv_freq", inv_freq, persistent=False)
+ self.original_inv_freq = self.inv_freq
+
+ def _dynamic_frequency_update(self, position_ids, device):
+ """
+ dynamic RoPE layers should recompute `inv_freq` in the following situations:
+ 1 - growing beyond the cached sequence length (allow scaling)
+ 2 - the current sequence length is in the original scale (avoid losing precision with small sequences)
+ """
+ seq_len = torch.max(position_ids) + 1
+ if seq_len > self.max_seq_len_cached: # growth
+ inv_freq, self.attention_scaling = self.rope_init_fn(self.config, device, seq_len=seq_len)
+ self.register_buffer("inv_freq", inv_freq, persistent=False) # TODO joao: may break with compilation
+ self.max_seq_len_cached = seq_len
+
+ if seq_len < self.original_max_seq_len and self.max_seq_len_cached > self.original_max_seq_len: # reset
+ # This .to() is needed if the model has been moved to a device after being initialized (because
+ # the buffer is automatically moved, but not the original copy)
+ self.original_inv_freq = self.original_inv_freq.to(device)
+ self.register_buffer("inv_freq", self.original_inv_freq, persistent=False)
+ self.max_seq_len_cached = self.original_max_seq_len
+
+ @torch.no_grad()
+ def forward(self, x, position_ids):
+ if "dynamic" in self.rope_type:
+ self._dynamic_frequency_update(position_ids, device=x.device)
+
+ # Core RoPE block
+ inv_freq_expanded = self.inv_freq[None, :, None].float().expand(position_ids.shape[0], -1, 1)
+ position_ids_expanded = position_ids[:, None, :].float()
+ # Force float32 (see https://github.com/huggingface/transformers/pull/29285)
+ device_type = x.device.type
+ device_type = device_type if isinstance(device_type, str) and device_type != "mps" else "cpu"
+ with torch.autocast(device_type=device_type, enabled=False):
+ freqs = (inv_freq_expanded.float() @ position_ids_expanded.float()).transpose(1, 2)
+ emb = torch.cat((freqs, freqs), dim=-1)
+ cos = emb.cos()
+ sin = emb.sin()
+
+ # Advanced RoPE types (e.g. yarn) apply a post-processing scaling factor, equivalent to scaling attention
+ cos = cos * self.attention_scaling
+ sin = sin * self.attention_scaling
+
+ return cos.to(dtype=x.dtype), sin.to(dtype=x.dtype)
+
+
+INTERNLM3_START_DOCSTRING = r"""
+ This model inherits from [`PreTrainedModel`]. Check the superclass documentation for the generic methods the
+ library implements for all its model (such as downloading or saving, resizing the input embeddings, pruning heads
+ etc.)
+
+ This model is also a PyTorch [torch.nn.Module](https://pytorch.org/docs/stable/nn.html#torch.nn.Module) subclass.
+ Use it as a regular PyTorch Module and refer to the PyTorch documentation for all matter related to general usage
+ and behavior.
+
+ Parameters:
+ config ([`InternLM3Config`]):
+ Model configuration class with all the parameters of the model. Initializing with a config file does not
+ load the weights associated with the model, only the configuration. Check out the
+ [`~PreTrainedModel.from_pretrained`] method to load the model weights.
+"""
+
+
+@add_start_docstrings(
+ "The bare InternLM3 Model outputting raw hidden-states without any specific head on top.",
+ INTERNLM3_START_DOCSTRING,
+)
+class InternLM3PreTrainedModel(PreTrainedModel):
+ config_class = InternLM3Config
+ base_model_prefix = "model"
+ supports_gradient_checkpointing = True
+ _no_split_modules = ["InternLM3DecoderLayer"]
+ _skip_keys_device_placement = ["past_key_values"]
+ _supports_flash_attn_2 = True
+ _supports_sdpa = True
+ _supports_flex_attn = True
+ _supports_cache_class = True
+ _supports_quantized_cache = True
+ _supports_static_cache = True
+
+ def _init_weights(self, module):
+ std = self.config.initializer_range
+ if isinstance(module, nn.Linear):
+ module.weight.data.normal_(mean=0.0, std=std)
+ if module.bias is not None:
+ module.bias.data.zero_()
+ elif isinstance(module, nn.Embedding):
+ module.weight.data.normal_(mean=0.0, std=std)
+ if module.padding_idx is not None:
+ module.weight.data[module.padding_idx].zero_()
+
+
+INTERNLM3_INPUTS_DOCSTRING = r"""
+ Args:
+ input_ids (`torch.LongTensor` of shape `(batch_size, sequence_length)`):
+ Indices of input sequence tokens in the vocabulary. Padding will be ignored by default should you provide
+ it.
+
+ Indices can be obtained using [`AutoTokenizer`]. See [`PreTrainedTokenizer.encode`] and
+ [`PreTrainedTokenizer.__call__`] for details.
+
+ [What are input IDs?](../glossary#input-ids)
+ attention_mask (`torch.Tensor` of shape `(batch_size, sequence_length)`, *optional*):
+ Mask to avoid performing attention on padding token indices. Mask values selected in `[0, 1]`:
+
+ - 1 for tokens that are **not masked**,
+ - 0 for tokens that are **masked**.
+
+ [What are attention masks?](../glossary#attention-mask)
+
+ Indices can be obtained using [`AutoTokenizer`]. See [`PreTrainedTokenizer.encode`] and
+ [`PreTrainedTokenizer.__call__`] for details.
+
+ If `past_key_values` is used, optionally only the last `input_ids` have to be input (see
+ `past_key_values`).
+
+ If you want to change padding behavior, you should read [`modeling_opt._prepare_decoder_attention_mask`]
+ and modify to your needs. See diagram 1 in [the paper](https://arxiv.org/abs/1910.13461) for more
+ information on the default strategy.
+
+ - 1 indicates the head is **not masked**,
+ - 0 indicates the head is **masked**.
+ position_ids (`torch.LongTensor` of shape `(batch_size, sequence_length)`, *optional*):
+ Indices of positions of each input sequence tokens in the position embeddings. Selected in the range `[0,
+ config.n_positions - 1]`.
+
+ [What are position IDs?](../glossary#position-ids)
+ past_key_values (`Cache` or `tuple(tuple(torch.FloatTensor))`, *optional*):
+ Pre-computed hidden-states (key and values in the self-attention blocks and in the cross-attention
+ blocks) that can be used to speed up sequential decoding. This typically consists in the `past_key_values`
+ returned by the model at a previous stage of decoding, when `use_cache=True` or `config.use_cache=True`.
+
+ Two formats are allowed:
+ - a [`~cache_utils.Cache`] instance, see our
+ [kv cache guide](https://huggingface.co/docs/transformers/en/kv_cache);
+ - Tuple of `tuple(torch.FloatTensor)` of length `config.n_layers`, with each tuple having 2 tensors of
+ shape `(batch_size, num_heads, sequence_length, embed_size_per_head)`). This is also known as the legacy
+ cache format.
+
+ The model will output the same cache format that is fed as input. If no `past_key_values` are passed, the
+ legacy cache format will be returned.
+
+ If `past_key_values` are used, the user can optionally input only the last `input_ids` (those that don't
+ have their past key value states given to this model) of shape `(batch_size, 1)` instead of all `input_ids`
+ of shape `(batch_size, sequence_length)`.
+ inputs_embeds (`torch.FloatTensor` of shape `(batch_size, sequence_length, hidden_size)`, *optional*):
+ Optionally, instead of passing `input_ids` you can choose to directly pass an embedded representation. This
+ is useful if you want more control over how to convert `input_ids` indices into associated vectors than the
+ model's internal embedding lookup matrix.
+ use_cache (`bool`, *optional*):
+ If set to `True`, `past_key_values` key value states are returned and can be used to speed up decoding (see
+ `past_key_values`).
+ output_attentions (`bool`, *optional*):
+ Whether or not to return the attentions tensors of all attention layers. See `attentions` under returned
+ tensors for more detail.
+ output_hidden_states (`bool`, *optional*):
+ Whether or not to return the hidden states of all layers. See `hidden_states` under returned tensors for
+ more detail.
+ return_dict (`bool`, *optional*):
+ Whether or not to return a [`~utils.ModelOutput`] instead of a plain tuple.
+ cache_position (`torch.LongTensor` of shape `(sequence_length)`, *optional*):
+ Indices depicting the position of the input sequence tokens in the sequence. Contrarily to `position_ids`,
+ this tensor is not affected by padding. It is used to update the cache in the correct position and to infer
+ the complete sequence length.
+"""
+
+
+@add_start_docstrings(
+ "The bare InternLM3 Model outputting raw hidden-states without any specific head on top.",
+ INTERNLM3_START_DOCSTRING,
+)
+class InternLM3Model(InternLM3PreTrainedModel):
+ """
+ Transformer decoder consisting of *config.num_hidden_layers* layers. Each layer is a [`InternLM3DecoderLayer`]
+
+ Args:
+ config: InternLM3Config
+ """
+
+ def __init__(self, config: InternLM3Config):
+ super().__init__(config)
+ self.padding_idx = config.pad_token_id
+ self.vocab_size = config.vocab_size
+
+ self.embed_tokens = nn.Embedding(config.vocab_size, config.hidden_size, self.padding_idx)
+ self.layers = nn.ModuleList(
+ [InternLM3DecoderLayer(config, layer_idx) for layer_idx in range(config.num_hidden_layers)]
+ )
+ self.norm = InternLM3RMSNorm(config.hidden_size, eps=config.rms_norm_eps)
+ self.rotary_emb = InternLM3RotaryEmbedding(config=config)
+ self.gradient_checkpointing = False
+
+ # Initialize weights and apply final processing
+ self.post_init()
+
+ def get_input_embeddings(self):
+ return self.embed_tokens
+
+ def set_input_embeddings(self, value):
+ self.embed_tokens = value
+
+ @add_start_docstrings_to_model_forward(INTERNLM3_INPUTS_DOCSTRING)
+ def forward(
+ self,
+ input_ids: torch.LongTensor = None,
+ attention_mask: Optional[torch.Tensor] = None,
+ position_ids: Optional[torch.LongTensor] = None,
+ past_key_values: Optional[Cache] = None,
+ inputs_embeds: Optional[torch.FloatTensor] = None,
+ use_cache: Optional[bool] = None,
+ output_attentions: Optional[bool] = None,
+ output_hidden_states: Optional[bool] = None,
+ return_dict: Optional[bool] = None,
+ cache_position: Optional[torch.LongTensor] = None,
+ **flash_attn_kwargs: Unpack[FlashAttentionKwargs],
+ ) -> Union[Tuple, BaseModelOutputWithPast]:
+ output_attentions = output_attentions if output_attentions is not None else self.config.output_attentions
+ output_hidden_states = (
+ output_hidden_states if output_hidden_states is not None else self.config.output_hidden_states
+ )
+ use_cache = use_cache if use_cache is not None else self.config.use_cache
+ return_dict = return_dict if return_dict is not None else self.config.use_return_dict
+
+ if (input_ids is None) ^ (inputs_embeds is not None):
+ raise ValueError("You must specify exactly one of input_ids or inputs_embeds")
+
+ if self.gradient_checkpointing and self.training and use_cache:
+ logger.warning_once(
+ "`use_cache=True` is incompatible with gradient checkpointing. Setting `use_cache=False`."
+ )
+ use_cache = False
+
+ if inputs_embeds is None:
+ inputs_embeds = self.embed_tokens(input_ids)
+
+ if use_cache and past_key_values is None:
+ past_key_values = DynamicCache()
+
+ if cache_position is None:
+ past_seen_tokens = past_key_values.get_seq_length() if past_key_values is not None else 0
+ cache_position = torch.arange(
+ past_seen_tokens, past_seen_tokens + inputs_embeds.shape[1], device=inputs_embeds.device
+ )
+
+ if position_ids is None:
+ position_ids = cache_position.unsqueeze(0)
+
+ causal_mask = self._update_causal_mask(
+ attention_mask, inputs_embeds, cache_position, past_key_values, output_attentions
+ )
+
+ hidden_states = inputs_embeds
+
+ # create position embeddings to be shared across the decoder layers
+ position_embeddings = self.rotary_emb(hidden_states, position_ids)
+
+ # decoder layers
+ all_hidden_states = () if output_hidden_states else None
+ all_self_attns = () if output_attentions else None
+
+ for decoder_layer in self.layers[: self.config.num_hidden_layers]:
+ if output_hidden_states:
+ all_hidden_states += (hidden_states,)
+
+ if self.gradient_checkpointing and self.training:
+ layer_outputs = self._gradient_checkpointing_func(
+ decoder_layer.__call__,
+ hidden_states,
+ causal_mask,
+ position_ids,
+ past_key_values,
+ output_attentions,
+ use_cache,
+ cache_position,
+ position_embeddings,
+ )
+ else:
+ layer_outputs = decoder_layer(
+ hidden_states,
+ attention_mask=causal_mask,
+ position_ids=position_ids,
+ past_key_value=past_key_values,
+ output_attentions=output_attentions,
+ use_cache=use_cache,
+ cache_position=cache_position,
+ position_embeddings=position_embeddings,
+ **flash_attn_kwargs,
+ )
+
+ hidden_states = layer_outputs[0]
+
+ if output_attentions:
+ all_self_attns += (layer_outputs[1],)
+
+ hidden_states = self.norm(hidden_states)
+
+ # add hidden states from the last decoder layer
+ if output_hidden_states:
+ all_hidden_states += (hidden_states,)
+
+ output = BaseModelOutputWithPast(
+ last_hidden_state=hidden_states,
+ past_key_values=past_key_values if use_cache else None,
+ hidden_states=all_hidden_states,
+ attentions=all_self_attns,
+ )
+ return output if return_dict else output.to_tuple()
+
+ def _update_causal_mask(
+ self,
+ attention_mask: torch.Tensor,
+ input_tensor: torch.Tensor,
+ cache_position: torch.Tensor,
+ past_key_values: Cache,
+ output_attentions: bool,
+ ):
+ if self.config._attn_implementation == "flash_attention_2":
+ if attention_mask is not None and (attention_mask == 0.0).any():
+ return attention_mask
+ return None
+
+ # For SDPA, when possible, we will rely on its `is_causal` argument instead of its `attn_mask` argument, in
+ # order to dispatch on Flash Attention 2. This feature is not compatible with static cache, as SDPA will fail
+ # to infer the attention mask.
+ past_seen_tokens = past_key_values.get_seq_length() if past_key_values is not None else 0
+ using_static_cache = isinstance(past_key_values, StaticCache)
+
+ # When output attentions is True, sdpa implementation's forward method calls the eager implementation's forward
+ if self.config._attn_implementation == "sdpa" and not using_static_cache and not output_attentions:
+ if AttentionMaskConverter._ignore_causal_mask_sdpa(
+ attention_mask,
+ inputs_embeds=input_tensor,
+ past_key_values_length=past_seen_tokens,
+ is_training=self.training,
+ ):
+ return None
+
+ dtype, device = input_tensor.dtype, input_tensor.device
+ sequence_length = input_tensor.shape[1]
+ if using_static_cache:
+ target_length = past_key_values.get_max_cache_shape()
+ else:
+ target_length = (
+ attention_mask.shape[-1]
+ if isinstance(attention_mask, torch.Tensor)
+ else past_seen_tokens + sequence_length + 1
+ )
+
+ # In case the provided `attention` mask is 2D, we generate a causal mask here (4D).
+ causal_mask = self._prepare_4d_causal_attention_mask_with_cache_position(
+ attention_mask,
+ sequence_length=sequence_length,
+ target_length=target_length,
+ dtype=dtype,
+ device=device,
+ cache_position=cache_position,
+ batch_size=input_tensor.shape[0],
+ )
+
+ if (
+ self.config._attn_implementation == "sdpa"
+ and attention_mask is not None
+ and attention_mask.device.type == "cuda"
+ and not output_attentions
+ ):
+ # Attend to all tokens in fully masked rows in the causal_mask, for example the relevant first rows when
+ # using left padding. This is required by F.scaled_dot_product_attention memory-efficient attention path.
+ # Details: https://github.com/pytorch/pytorch/issues/110213
+ min_dtype = torch.finfo(dtype).min
+ causal_mask = AttentionMaskConverter._unmask_unattended(causal_mask, min_dtype)
+
+ return causal_mask
+
+ @staticmethod
+ def _prepare_4d_causal_attention_mask_with_cache_position(
+ attention_mask: torch.Tensor,
+ sequence_length: int,
+ target_length: int,
+ dtype: torch.dtype,
+ device: torch.device,
+ cache_position: torch.Tensor,
+ batch_size: int,
+ **kwargs,
+ ):
+ """
+ Creates a causal 4D mask of shape `(batch_size, 1, query_length, key_value_length)` from a 2D mask of shape
+ `(batch_size, key_value_length)`, or if the input `attention_mask` is already 4D, do nothing.
+
+ Args:
+ attention_mask (`torch.Tensor`):
+ A 2D attention mask of shape `(batch_size, key_value_length)` or a 4D attention mask of shape
+ `(batch_size, 1, query_length, key_value_length)`.
+ sequence_length (`int`):
+ The sequence length being processed.
+ target_length (`int`):
+ The target length: when generating with static cache, the mask should be as long as the static cache,
+ to account for the 0 padding, the part of the cache that is not filled yet.
+ dtype (`torch.dtype`):
+ The dtype to use for the 4D attention mask.
+ device (`torch.device`):
+ The device to plcae the 4D attention mask on.
+ cache_position (`torch.Tensor`):
+ Indices depicting the position of the input sequence tokens in the sequence.
+ batch_size (`torch.Tensor`):
+ Batch size.
+ """
+ if attention_mask is not None and attention_mask.dim() == 4:
+ # In this case we assume that the mask comes already in inverted form and requires no inversion or slicing.
+ causal_mask = attention_mask
+ else:
+ min_dtype = torch.finfo(dtype).min
+ causal_mask = torch.full(
+ (sequence_length, target_length), fill_value=min_dtype, dtype=dtype, device=device
+ )
+ if sequence_length != 1:
+ causal_mask = torch.triu(causal_mask, diagonal=1)
+ causal_mask *= torch.arange(target_length, device=device) > cache_position.reshape(-1, 1)
+ causal_mask = causal_mask[None, None, :, :].expand(batch_size, 1, -1, -1)
+ if attention_mask is not None:
+ causal_mask = causal_mask.clone() # copy to contiguous memory for in-place edit
+ mask_length = attention_mask.shape[-1]
+ padding_mask = causal_mask[:, :, :, :mask_length] + attention_mask[:, None, None, :]
+ padding_mask = padding_mask == 0
+ causal_mask[:, :, :, :mask_length] = causal_mask[:, :, :, :mask_length].masked_fill(
+ padding_mask, min_dtype
+ )
+
+ return causal_mask
+
+
+class KwargsForCausalLM(FlashAttentionKwargs, LossKwargs): ...
+
+
+class InternLM3ForCausalLM(InternLM3PreTrainedModel, GenerationMixin):
+ _tied_weights_keys = ["lm_head.weight"]
+ _tp_plan = {"lm_head": "colwise_rep"}
+
+ def __init__(self, config):
+ super().__init__(config)
+ self.model = InternLM3Model(config)
+ self.vocab_size = config.vocab_size
+ self.lm_head = nn.Linear(config.hidden_size, config.vocab_size, bias=False)
+
+ # Initialize weights and apply final processing
+ self.post_init()
+
+ def get_input_embeddings(self):
+ return self.model.embed_tokens
+
+ def set_input_embeddings(self, value):
+ self.model.embed_tokens = value
+
+ def get_output_embeddings(self):
+ return self.lm_head
+
+ def set_output_embeddings(self, new_embeddings):
+ self.lm_head = new_embeddings
+
+ def set_decoder(self, decoder):
+ self.model = decoder
+
+ def get_decoder(self):
+ return self.model
+
+ @add_start_docstrings_to_model_forward(INTERNLM3_INPUTS_DOCSTRING)
+ @replace_return_docstrings(output_type=CausalLMOutputWithPast, config_class=_CONFIG_FOR_DOC)
+ def forward(
+ self,
+ input_ids: torch.LongTensor = None,
+ attention_mask: Optional[torch.Tensor] = None,
+ position_ids: Optional[torch.LongTensor] = None,
+ past_key_values: Optional[Union[Cache, List[torch.FloatTensor]]] = None,
+ inputs_embeds: Optional[torch.FloatTensor] = None,
+ labels: Optional[torch.LongTensor] = None,
+ use_cache: Optional[bool] = None,
+ output_attentions: Optional[bool] = None,
+ output_hidden_states: Optional[bool] = None,
+ return_dict: Optional[bool] = None,
+ cache_position: Optional[torch.LongTensor] = None,
+ num_logits_to_keep: int = 0,
+ **kwargs: Unpack[KwargsForCausalLM],
+ ) -> Union[Tuple, CausalLMOutputWithPast]:
+ r"""
+ Args:
+ labels (`torch.LongTensor` of shape `(batch_size, sequence_length)`, *optional*):
+ Labels for computing the masked language modeling loss. Indices should either be in `[0, ...,
+ config.vocab_size]` or -100 (see `input_ids` docstring). Tokens with indices set to `-100` are ignored
+ (masked), the loss is only computed for the tokens with labels in `[0, ..., config.vocab_size]`.
+
+ num_logits_to_keep (`int`, *optional*):
+ Calculate logits for the last `num_logits_to_keep` tokens. If `0`, calculate logits for all
+ `input_ids` (special case). Only last token logits are needed for generation, and calculating them only for that
+ token can save memory, which becomes pretty significant for long sequences or large vocabulary size.
+
+ Returns:
+
+ Example:
+
+ ```python
+ >>> from transformers import AutoTokenizer, InternLM3ForCausalLM
+
+ >>> model = InternLM3ForCausalLM.from_pretrained("meta-internlm3/InternLM3-2-7b-hf")
+ >>> tokenizer = AutoTokenizer.from_pretrained("meta-internlm3/InternLM3-2-7b-hf")
+
+ >>> prompt = "Hey, are you conscious? Can you talk to me?"
+ >>> inputs = tokenizer(prompt, return_tensors="pt")
+
+ >>> # Generate
+ >>> generate_ids = model.generate(inputs.input_ids, max_length=30)
+ >>> tokenizer.batch_decode(generate_ids, skip_special_tokens=True, clean_up_tokenization_spaces=False)[0]
+ "Hey, are you conscious? Can you talk to me?\nI'm not conscious, but I can talk to you."
+ ```"""
+ output_attentions = output_attentions if output_attentions is not None else self.config.output_attentions
+ output_hidden_states = (
+ output_hidden_states if output_hidden_states is not None else self.config.output_hidden_states
+ )
+ return_dict = return_dict if return_dict is not None else self.config.use_return_dict
+
+ # decoder outputs consists of (dec_features, layer_state, dec_hidden, dec_attn)
+ outputs = self.model(
+ input_ids=input_ids,
+ attention_mask=attention_mask,
+ position_ids=position_ids,
+ past_key_values=past_key_values,
+ inputs_embeds=inputs_embeds,
+ use_cache=use_cache,
+ output_attentions=output_attentions,
+ output_hidden_states=output_hidden_states,
+ return_dict=return_dict,
+ cache_position=cache_position,
+ **kwargs,
+ )
+
+ hidden_states = outputs[0]
+ # Only compute necessary logits, and do not upcast them to float if we are not computing the loss
+ logits = self.lm_head(hidden_states[:, -num_logits_to_keep:, :])
+
+ loss = None
+ if labels is not None:
+ loss = self.loss_function(logits=logits, labels=labels, vocab_size=self.config.vocab_size, **kwargs)
+
+ if not return_dict:
+ output = (logits,) + outputs[1:]
+ return (loss,) + output if loss is not None else output
+
+ return CausalLMOutputWithPast(
+ loss=loss,
+ logits=logits,
+ past_key_values=outputs.past_key_values,
+ hidden_states=outputs.hidden_states,
+ attentions=outputs.attentions,
+ )
\ No newline at end of file
diff --git a/xtuner/_lite/modelings/internlm3/tokenization_internlm3.py b/xtuner/_lite/modelings/internlm3/tokenization_internlm3.py
new file mode 100644
index 000000000..f68147f2c
--- /dev/null
+++ b/xtuner/_lite/modelings/internlm3/tokenization_internlm3.py
@@ -0,0 +1,294 @@
+import os
+from shutil import copyfile
+from typing import TYPE_CHECKING, Any, Dict, List, Optional, Tuple
+
+import sentencepiece as spm
+from transformers.tokenization_utils import AddedToken, PreTrainedTokenizer
+from transformers.utils import logging
+
+if TYPE_CHECKING:
+ from transformers.tokenization_utils_base import TextInput
+
+logger = logging.get_logger(__name__)
+
+VOCAB_FILES_NAMES = {"vocab_file": "tokenizer.model"}
+
+SPIECE_UNDERLINE = "▁"
+
+
+class InternLM3Tokenizer(PreTrainedTokenizer):
+ """
+ Construct a InternLM3 tokenizer. Based on byte-level Byte-Pair-Encoding. The default padding token is unset as there is
+ no padding token in the original model.
+
+ Args:
+ vocab_file (`str`):
+ Path to the vocabulary file.
+ unk_token (`str` or `tokenizers.AddedToken`, *optional*, defaults to `""`):
+ The unknown token. A token that is not in the vocabulary cannot be converted to an ID and is set to be this
+ token instead.
+ bos_token (`str` or `tokenizers.AddedToken`, *optional*, defaults to `""`):
+ The beginning of sequence token that was used during pretraining. Can be used a sequence classifier token.
+ eos_token (`str` or `tokenizers.AddedToken`, *optional*, defaults to `""`):
+ The end of sequence token.
+ pad_token (`str` or `tokenizers.AddedToken`, *optional*):
+ A special token used to make arrays of tokens the same size for batching purpose. Will then be ignored by
+ attention mechanisms or loss computation.
+ sp_model_kwargs (`Dict[str, Any]`, `Optional`, *optional*):
+ Will be passed to the `SentencePieceProcessor.__init__()` method. The [Python wrapper for
+ SentencePiece](https://github.com/google/sentencepiece/tree/master/python) can be used, among other things,
+ to set:
+
+ - `enable_sampling`: Enable subword regularization.
+ - `nbest_size`: Sampling parameters for unigram. Invalid for BPE-Dropout.
+
+ - `nbest_size = {0,1}`: No sampling is performed.
+ - `nbest_size > 1`: samples from the nbest_size results.
+ - `nbest_size < 0`: assuming that nbest_size is infinite and samples from the all hypothesis (lattice)
+ using forward-filtering-and-backward-sampling algorithm.
+
+ - `alpha`: Smoothing parameter for unigram sampling, and dropout probability of merge operations for
+ BPE-dropout.
+
+ add_bos_token (`bool`, *optional*, defaults to `True`):
+ Whether or not to add an `bos_token` at the start of sequences.
+ add_eos_token (`bool`, *optional*, defaults to `False`):
+ Whether or not to add an `eos_token` at the end of sequences.
+ clean_up_tokenization_spaces (`bool`, *optional*, defaults to `False`):
+ Whether or not to cleanup spaces after decoding, cleanup consists in removing potential artifacts like
+ extra spaces.
+ use_default_system_prompt (`bool`, *optional*, defaults to `False`):
+ Whether or not the default system prompt for InternLM3 should be used.
+ spaces_between_special_tokens (`bool`, *optional*, defaults to `False`):
+ Whether or not to add spaces between special tokens.
+ spaces_for_interleaved_special_tokens (`bool`, *optional*, defaults to `False`):
+ Whether or not to add spaces between special tokens that are interleaved with normal tokens.
+ add_prefix_space (`bool`, *optional*, defaults to `True`):
+ Whether or not to add an initial space to the input. This allows to treat the leading word just as any
+ other word. Again, this should be set with `from_slow=True` to make sure it's taken into account.
+ """
+
+ vocab_files_names = VOCAB_FILES_NAMES
+ model_input_names = ["input_ids", "attention_mask"]
+
+ def __init__(
+ self,
+ vocab_file,
+ unk_token="",
+ bos_token="",
+ eos_token="",
+ pad_token=None,
+ sp_model_kwargs: Optional[Dict[str, Any]] = None,
+ add_bos_token=True,
+ add_eos_token=False,
+ clean_up_tokenization_spaces=False,
+ use_default_system_prompt=False,
+ spaces_between_special_tokens=False,
+ spaces_for_interleaved_special_tokens=False,
+ add_prefix_space=True,
+ **kwargs,
+ ):
+ self.sp_model_kwargs = {} if sp_model_kwargs is None else sp_model_kwargs
+ bos_token = AddedToken(bos_token, normalized=False, special=True) if isinstance(bos_token, str) else bos_token
+ eos_token = AddedToken(eos_token, normalized=False, special=True) if isinstance(eos_token, str) else eos_token
+ unk_token = AddedToken(unk_token, normalized=False, special=True) if isinstance(unk_token, str) else unk_token
+ pad_token = AddedToken(pad_token, normalized=False, special=True) if isinstance(pad_token, str) else pad_token
+
+ self.vocab_file = vocab_file
+ self.add_bos_token = add_bos_token
+ self.add_eos_token = add_eos_token
+ self.use_default_system_prompt = use_default_system_prompt
+ self.sp_model = spm.SentencePieceProcessor(**self.sp_model_kwargs)
+ self.sp_model.Load(vocab_file)
+ self.add_prefix_space = add_prefix_space
+ self.spaces_for_interleaved_special_tokens = spaces_for_interleaved_special_tokens
+
+ vocab_size = self.sp_model.get_piece_size()
+ self.decoder = {i: self.sp_model.id_to_piece(i) for i in range(vocab_size)}
+
+ super().__init__(
+ bos_token=bos_token,
+ eos_token=eos_token,
+ unk_token=unk_token,
+ pad_token=pad_token,
+ add_bos_token=add_bos_token,
+ add_eos_token=add_eos_token,
+ sp_model_kwargs=sp_model_kwargs,
+ clean_up_tokenization_spaces=clean_up_tokenization_spaces,
+ use_default_system_prompt=use_default_system_prompt,
+ spaces_between_special_tokens=spaces_between_special_tokens,
+ add_prefix_space=add_prefix_space,
+ **kwargs,
+ )
+
+ def __getstate__(self):
+ state = self.__dict__.copy()
+ state["sp_model"] = None
+ state["sp_model_proto"] = self.sp_model.serialized_model_proto()
+ return state
+
+ def __setstate__(self, d):
+ self.__dict__.update(d)
+ self.sp_model = spm.SentencePieceProcessor(**self.sp_model_kwargs)
+ self.sp_model.LoadFromSerializedProto(self.sp_model_proto)
+
+ @property
+ def vocab_size(self):
+ """Returns vocab size"""
+ return self.sp_model.get_piece_size()
+
+ def get_vocab(self):
+ """Returns vocab as a dict"""
+ vocab = {self.convert_ids_to_tokens(i): i for i in range(self.vocab_size)}
+ vocab.update(self.added_tokens_encoder)
+ return vocab
+
+ def tokenize(self, text: "TextInput", **kwargs) -> List[str]:
+ """
+ Args:
+ text: TextInput
+ Simply calls PreTrainedTokenizer's method
+ """
+ return super().tokenize(text, **kwargs)
+
+ def _tokenize(self, text, **kwargs):
+ """
+ Args:
+ text: TextInput
+ Returns a tokenized string. The Gemma tokenizer never adds a prefix space.
+ """
+ return self.sp_model.encode(text, out_type=str)
+
+ def _convert_token_to_id(self, token):
+ """Converts a token (str) in an id using the vocab."""
+ return self.sp_model.piece_to_id(token)
+
+ def _convert_id_to_token(self, index):
+ """Converts an index (integer) in a token (str) using the vocab."""
+ return self.decoder.get(index, "")
+
+ def convert_tokens_to_string(self, tokens):
+ """Converts a sequence of tokens (string) in a single string."""
+ # since we manually add the prefix space, we have to remove it when decoding
+ if tokens[0].startswith(SPIECE_UNDERLINE) and self.add_prefix_space:
+ tokens[0] = tokens[0][1:]
+
+ current_sub_tokens = []
+ out_string = ""
+ prev_is_special = False
+ for i, token in enumerate(tokens):
+ # make sure that special tokens are not decoded using sentencepiece model
+ if token in self.all_special_tokens:
+ if not prev_is_special and i != 0 and self.spaces_for_interleaved_special_tokens:
+ out_string += " "
+ out_string += self.sp_model.decode(current_sub_tokens) + token
+ prev_is_special = True
+ current_sub_tokens = []
+ else:
+ if (
+ prev_is_special
+ and i == 1
+ and self.add_prefix_space
+ and not token.startswith(SPIECE_UNDERLINE)
+ and self.spaces_for_interleaved_special_tokens
+ ):
+ out_string += " "
+ current_sub_tokens.append(token)
+ prev_is_special = False
+ out_string += self.sp_model.decode(current_sub_tokens)
+ return out_string
+
+ def save_vocabulary(self, save_directory, filename_prefix: Optional[str] = None) -> Tuple[str]:
+ """
+ Save the vocabulary and special tokens file to a directory.
+
+ Args:
+ save_directory (`str`):
+ The directory in which to save the vocabulary.
+
+ Returns:
+ `Tuple(str)`: Paths to the files saved.
+ """
+ if not os.path.isdir(save_directory):
+ logger.error(f"Vocabulary path ({save_directory}) should be a directory")
+ return
+ out_vocab_file = os.path.join(save_directory, (filename_prefix + "-" if filename_prefix else "") + VOCAB_FILES_NAMES["vocab_file"])
+
+ if os.path.abspath(self.vocab_file) != os.path.abspath(out_vocab_file) and os.path.isfile(self.vocab_file):
+ copyfile(self.vocab_file, out_vocab_file)
+ elif not os.path.isfile(self.vocab_file):
+ with open(out_vocab_file, "wb") as fi:
+ content_spiece_model = self.sp_model.serialized_model_proto()
+ fi.write(content_spiece_model)
+
+ return (out_vocab_file,)
+
+ def build_inputs_with_special_tokens(self, token_ids_0, token_ids_1=None):
+ bos_token_id = [self.bos_token_id] if self.add_bos_token else []
+ eos_token_id = [self.eos_token_id] if self.add_eos_token else []
+
+ output = bos_token_id + token_ids_0 + eos_token_id
+
+ if token_ids_1 is not None:
+ output = output + bos_token_id + token_ids_1 + eos_token_id
+
+ return output
+
+ def get_special_tokens_mask(
+ self, token_ids_0: List[int], token_ids_1: Optional[List[int]] = None, already_has_special_tokens: bool = False
+ ) -> List[int]:
+ """
+ Retrieve sequence ids from a token list that has no special tokens added. This method is called when adding
+ special tokens using the tokenizer `prepare_for_model` method.
+
+ Args:
+ token_ids_0 (`List[int]`):
+ List of IDs.
+ token_ids_1 (`List[int]`, *optional*):
+ Optional second list of IDs for sequence pairs.
+ already_has_special_tokens (`bool`, *optional*, defaults to `False`):
+ Whether or not the token list is already formatted with special tokens for the model.
+
+ Returns:
+ `List[int]`: A list of integers in the range [0, 1]: 1 for a special token, 0 for a sequence token.
+ """
+ if already_has_special_tokens:
+ return super().get_special_tokens_mask(token_ids_0=token_ids_0, token_ids_1=token_ids_1, already_has_special_tokens=True)
+
+ bos_token_id = [1] if self.add_bos_token else []
+ eos_token_id = [1] if self.add_eos_token else []
+
+ if token_ids_1 is None:
+ return bos_token_id + ([0] * len(token_ids_0)) + eos_token_id
+ return bos_token_id + ([0] * len(token_ids_0)) + eos_token_id + bos_token_id + ([0] * len(token_ids_1)) + eos_token_id
+
+ def create_token_type_ids_from_sequences(self, token_ids_0: List[int], token_ids_1: Optional[List[int]] = None) -> List[int]:
+ """
+ Creates a mask from the two sequences passed to be used in a sequence-pair classification task. An ALBERT
+ sequence pair mask has the following format:
+
+ ```
+ 0 0 0 0 0 0 0 0 0 0 0 1 1 1 1 1 1 1 1 1
+ | first sequence | second sequence |
+ ```
+
+ if token_ids_1 is None, only returns the first portion of the mask (0s).
+
+ Args:
+ token_ids_0 (`List[int]`):
+ List of ids.
+ token_ids_1 (`List[int]`, *optional*):
+ Optional second list of IDs for sequence pairs.
+
+ Returns:
+ `List[int]`: List of [token type IDs](../glossary#token-type-ids) according to the given sequence(s).
+ """
+ bos_token_id = [self.bos_token_id] if self.add_bos_token else []
+ eos_token_id = [self.eos_token_id] if self.add_eos_token else []
+
+ output = [0] * len(bos_token_id + token_ids_0 + eos_token_id)
+
+ if token_ids_1 is not None:
+ output += [1] * len(bos_token_id + token_ids_1 + eos_token_id)
+
+ return output
diff --git a/xtuner/_lite/modelings/internvl2/__init__.py b/xtuner/_lite/modelings/internvl2/__init__.py
new file mode 100644
index 000000000..8652be2d9
--- /dev/null
+++ b/xtuner/_lite/modelings/internvl2/__init__.py
@@ -0,0 +1,3 @@
+from .modeling_intern_vit import InternVisionModel
+
+__all__ = ['InternVisionModel']
diff --git a/xtuner/_lite/modelings/internvl2/configuration_intern_vit.py b/xtuner/_lite/modelings/internvl2/configuration_intern_vit.py
new file mode 100644
index 000000000..32f469c4b
--- /dev/null
+++ b/xtuner/_lite/modelings/internvl2/configuration_intern_vit.py
@@ -0,0 +1,119 @@
+# --------------------------------------------------------
+# InternVL
+# Copyright (c) 2024 OpenGVLab
+# Licensed under The MIT License [see LICENSE for details]
+# --------------------------------------------------------
+import os
+from typing import Union
+
+from transformers.configuration_utils import PretrainedConfig
+from transformers.utils import logging
+
+logger = logging.get_logger(__name__)
+
+
+class InternVisionConfig(PretrainedConfig):
+ r"""
+ This is the configuration class to store the configuration of a [`InternVisionModel`]. It is used to
+ instantiate a vision encoder according to the specified arguments, defining the model architecture.
+
+ Configuration objects inherit from [`PretrainedConfig`] and can be used to control the model outputs. Read the
+ documentation from [`PretrainedConfig`] for more information.
+
+ Args:
+ num_channels (`int`, *optional*, defaults to 3):
+ Number of color channels in the input images (e.g., 3 for RGB).
+ patch_size (`int`, *optional*, defaults to 14):
+ The size (resolution) of each patch.
+ image_size (`int`, *optional*, defaults to 224):
+ The size (resolution) of each image.
+ qkv_bias (`bool`, *optional*, defaults to `False`):
+ Whether to add a bias to the queries and values in the self-attention layers.
+ hidden_size (`int`, *optional*, defaults to 3200):
+ Dimensionality of the encoder layers and the pooler layer.
+ num_attention_heads (`int`, *optional*, defaults to 25):
+ Number of attention heads for each attention layer in the Transformer encoder.
+ intermediate_size (`int`, *optional*, defaults to 12800):
+ Dimensionality of the "intermediate" (i.e., feed-forward) layer in the Transformer encoder.
+ qk_normalization (`bool`, *optional*, defaults to `True`):
+ Whether to normalize the queries and keys in the self-attention layers.
+ num_hidden_layers (`int`, *optional*, defaults to 48):
+ Number of hidden layers in the Transformer encoder.
+ use_flash_attn (`bool`, *optional*, defaults to `True`):
+ Whether to use flash attention mechanism.
+ hidden_act (`str` or `function`, *optional*, defaults to `"gelu"`):
+ The non-linear activation function (function or string) in the encoder and pooler. If string, `"gelu"`,
+ `"relu"`, `"selu"` and `"gelu_new"` ``"gelu"` are supported.
+ layer_norm_eps (`float`, *optional*, defaults to 1e-6):
+ The epsilon used by the layer normalization layers.
+ dropout (`float`, *optional*, defaults to 0.0):
+ The dropout probability for all fully connected layers in the embeddings, encoder, and pooler.
+ drop_path_rate (`float`, *optional*, defaults to 0.0):
+ Dropout rate for stochastic depth.
+ attention_dropout (`float`, *optional*, defaults to 0.0):
+ The dropout ratio for the attention probabilities.
+ initializer_range (`float`, *optional*, defaults to 0.02):
+ The standard deviation of the truncated_normal_initializer for initializing all weight matrices.
+ initializer_factor (`float`, *optional*, defaults to 0.1):
+ A factor for layer scale.
+ """
+
+ model_type = 'intern_vit_6b'
+
+ def __init__(
+ self,
+ num_channels=3,
+ patch_size=14,
+ image_size=224,
+ qkv_bias=False,
+ hidden_size=3200,
+ num_attention_heads=25,
+ intermediate_size=12800,
+ qk_normalization=True,
+ num_hidden_layers=48,
+ use_flash_attn=True,
+ hidden_act='gelu',
+ norm_type='rms_norm',
+ layer_norm_eps=1e-6,
+ dropout=0.0,
+ drop_path_rate=0.0,
+ attention_dropout=0.0,
+ initializer_range=0.02,
+ initializer_factor=0.1,
+ **kwargs,
+ ):
+ super().__init__(**kwargs)
+
+ self.hidden_size = hidden_size
+ self.intermediate_size = intermediate_size
+ self.dropout = dropout
+ self.drop_path_rate = drop_path_rate
+ self.num_hidden_layers = num_hidden_layers
+ self.num_attention_heads = num_attention_heads
+ self.num_channels = num_channels
+ self.patch_size = patch_size
+ self.image_size = image_size
+ self.initializer_range = initializer_range
+ self.initializer_factor = initializer_factor
+ self.attention_dropout = attention_dropout
+ self.layer_norm_eps = layer_norm_eps
+ self.hidden_act = hidden_act
+ self.norm_type = norm_type
+ self.qkv_bias = qkv_bias
+ self.qk_normalization = qk_normalization
+ self.use_flash_attn = use_flash_attn
+
+ @classmethod
+ def from_pretrained(cls, pretrained_model_name_or_path: Union[str, os.PathLike], **kwargs) -> 'PretrainedConfig':
+ config_dict, kwargs = cls.get_config_dict(pretrained_model_name_or_path, **kwargs)
+
+ if 'vision_config' in config_dict:
+ config_dict = config_dict['vision_config']
+
+ if 'model_type' in config_dict and hasattr(cls, 'model_type') and config_dict['model_type'] != cls.model_type:
+ logger.warning(
+ f"You are using a model of type {config_dict['model_type']} to instantiate a model of type "
+ f'{cls.model_type}. This is not supported for all configurations of models and can yield errors.'
+ )
+
+ return cls.from_dict(config_dict, **kwargs)
\ No newline at end of file
diff --git a/xtuner/_lite/modelings/internvl2/modeling_intern_vit.py b/xtuner/_lite/modelings/internvl2/modeling_intern_vit.py
new file mode 100644
index 000000000..a8d36d9e3
--- /dev/null
+++ b/xtuner/_lite/modelings/internvl2/modeling_intern_vit.py
@@ -0,0 +1,432 @@
+# --------------------------------------------------------
+# InternVL
+# Copyright (c) 2024 OpenGVLab
+# Licensed under The MIT License [see LICENSE for details]
+# --------------------------------------------------------
+from typing import Optional, Tuple, Union
+
+import numpy as np
+import torch
+import torch.nn.functional as F
+import torch.utils.checkpoint
+from einops import rearrange
+from timm.models.layers import DropPath
+from torch import nn
+from transformers.activations import ACT2FN
+from transformers.modeling_outputs import (BaseModelOutput,
+ BaseModelOutputWithPooling)
+from transformers.modeling_utils import PreTrainedModel
+from transformers.utils import logging
+
+from .configuration_intern_vit import InternVisionConfig
+
+try:
+ from flash_attn.bert_padding import pad_input, unpad_input
+ from flash_attn.flash_attn_interface import \
+ flash_attn_varlen_qkvpacked_func
+ has_flash_attn = True
+except:
+ print('FlashAttention2 is not installed.')
+ has_flash_attn = False
+
+logger = logging.get_logger(__name__)
+
+
+class FlashAttention(nn.Module):
+ """Implement the scaled dot product attention with softmax.
+ Arguments
+ ---------
+ softmax_scale: The temperature to use for the softmax attention.
+ (default: 1/sqrt(d_keys) where d_keys is computed at
+ runtime)
+ attention_dropout: The dropout rate to apply to the attention
+ (default: 0.0)
+ """
+
+ def __init__(self, softmax_scale=None, attention_dropout=0.0, device=None, dtype=None):
+ super().__init__()
+ self.softmax_scale = softmax_scale
+ self.dropout_p = attention_dropout
+
+ def forward(self, qkv, key_padding_mask=None, causal=False, cu_seqlens=None,
+ max_s=None, need_weights=False):
+ """Implements the multihead softmax attention.
+ Arguments
+ ---------
+ qkv: The tensor containing the query, key, and value. (B, S, 3, H, D) if key_padding_mask is None
+ if unpadded: (nnz, 3, h, d)
+ key_padding_mask: a bool tensor of shape (B, S)
+ """
+ assert not need_weights
+ assert qkv.dtype in [torch.float16, torch.bfloat16]
+ assert qkv.is_cuda
+
+ if cu_seqlens is None:
+ batch_size = qkv.shape[0]
+ seqlen = qkv.shape[1]
+ if key_padding_mask is None:
+ qkv = rearrange(qkv, 'b s ... -> (b s) ...')
+ max_s = seqlen
+ cu_seqlens = torch.arange(0, (batch_size + 1) * seqlen, step=seqlen, dtype=torch.int32,
+ device=qkv.device)
+ output = flash_attn_varlen_qkvpacked_func(
+ qkv, cu_seqlens, max_s, self.dropout_p if self.training else 0.0,
+ softmax_scale=self.softmax_scale, causal=causal
+ )
+ output = rearrange(output, '(b s) ... -> b s ...', b=batch_size)
+ else:
+ nheads = qkv.shape[-2]
+ x = rearrange(qkv, 'b s three h d -> b s (three h d)')
+ x_unpad, indices, cu_seqlens, max_s = unpad_input(x, key_padding_mask)
+ x_unpad = rearrange(x_unpad, 'nnz (three h d) -> nnz three h d', three=3, h=nheads)
+ output_unpad = flash_attn_varlen_qkvpacked_func(
+ x_unpad, cu_seqlens, max_s, self.dropout_p if self.training else 0.0,
+ softmax_scale=self.softmax_scale, causal=causal
+ )
+ output = rearrange(pad_input(rearrange(output_unpad, 'nnz h d -> nnz (h d)'),
+ indices, batch_size, seqlen),
+ 'b s (h d) -> b s h d', h=nheads)
+ else:
+ assert max_s is not None
+ output = flash_attn_varlen_qkvpacked_func(
+ qkv, cu_seqlens, max_s, self.dropout_p if self.training else 0.0,
+ softmax_scale=self.softmax_scale, causal=causal
+ )
+
+ return output, None
+
+
+class InternRMSNorm(nn.Module):
+ def __init__(self, hidden_size, eps=1e-6):
+ super().__init__()
+ self.weight = nn.Parameter(torch.ones(hidden_size))
+ self.variance_epsilon = eps
+
+ def forward(self, hidden_states):
+ input_dtype = hidden_states.dtype
+ hidden_states = hidden_states.to(torch.float32)
+ variance = hidden_states.pow(2).mean(-1, keepdim=True)
+ hidden_states = hidden_states * torch.rsqrt(variance + self.variance_epsilon)
+ return self.weight * hidden_states.to(input_dtype)
+
+
+try:
+ from apex.normalization import FusedRMSNorm
+
+ InternRMSNorm = FusedRMSNorm # noqa
+
+ logger.info('Discovered apex.normalization.FusedRMSNorm - will use it instead of InternRMSNorm')
+except ImportError:
+ # using the normal InternRMSNorm
+ pass
+except Exception:
+ logger.warning('discovered apex but it failed to load, falling back to InternRMSNorm')
+ pass
+
+
+NORM2FN = {
+ 'rms_norm': InternRMSNorm,
+ 'layer_norm': nn.LayerNorm,
+}
+
+
+class InternVisionEmbeddings(nn.Module):
+ def __init__(self, config: InternVisionConfig):
+ super().__init__()
+ self.config = config
+ self.embed_dim = config.hidden_size
+ self.image_size = config.image_size
+ self.patch_size = config.patch_size
+
+ self.class_embedding = nn.Parameter(
+ torch.randn(1, 1, self.embed_dim),
+ )
+
+ self.patch_embedding = nn.Conv2d(
+ in_channels=3, out_channels=self.embed_dim, kernel_size=self.patch_size, stride=self.patch_size
+ )
+
+ self.num_patches = (self.image_size // self.patch_size) ** 2
+ self.num_positions = self.num_patches + 1
+
+ self.position_embedding = nn.Parameter(torch.randn(1, self.num_positions, self.embed_dim))
+
+ def _get_pos_embed(self, pos_embed, H, W):
+ target_dtype = pos_embed.dtype
+ pos_embed = pos_embed.float().reshape(
+ 1, self.image_size // self.patch_size, self.image_size // self.patch_size, -1).permute(0, 3, 1, 2)
+ pos_embed = F.interpolate(pos_embed, size=(H, W), mode='bicubic', align_corners=False). \
+ reshape(1, -1, H * W).permute(0, 2, 1).to(target_dtype)
+ return pos_embed
+
+ def forward(self, pixel_values: torch.FloatTensor) -> torch.Tensor:
+ target_dtype = self.patch_embedding.weight.dtype
+ patch_embeds = self.patch_embedding(pixel_values) # shape = [*, channel, width, height]
+ batch_size, _, height, width = patch_embeds.shape
+ patch_embeds = patch_embeds.flatten(2).transpose(1, 2)
+ class_embeds = self.class_embedding.expand(batch_size, 1, -1).to(target_dtype)
+ embeddings = torch.cat([class_embeds, patch_embeds], dim=1)
+ position_embedding = torch.cat([
+ self.position_embedding[:, :1, :],
+ self._get_pos_embed(self.position_embedding[:, 1:, :], height, width)
+ ], dim=1)
+ embeddings = embeddings + position_embedding.to(target_dtype)
+ return embeddings
+
+
+class InternAttention(nn.Module):
+ """Multi-headed attention from 'Attention Is All You Need' paper"""
+
+ def __init__(self, config: InternVisionConfig):
+ super().__init__()
+ self.config = config
+ self.embed_dim = config.hidden_size
+ self.num_heads = config.num_attention_heads
+ self.use_flash_attn = config.use_flash_attn and has_flash_attn
+ if config.use_flash_attn and not has_flash_attn:
+ print('Warning: Flash Attention is not available, use_flash_attn is set to False.')
+ self.head_dim = self.embed_dim // self.num_heads
+ if self.head_dim * self.num_heads != self.embed_dim:
+ raise ValueError(
+ f'embed_dim must be divisible by num_heads (got `embed_dim`: {self.embed_dim} and `num_heads`:'
+ f' {self.num_heads}).'
+ )
+
+ self.scale = self.head_dim ** -0.5
+ self.qkv = nn.Linear(self.embed_dim, 3 * self.embed_dim, bias=config.qkv_bias)
+ self.attn_drop = nn.Dropout(config.attention_dropout)
+ self.proj_drop = nn.Dropout(config.dropout)
+
+ self.qk_normalization = config.qk_normalization
+
+ if self.qk_normalization:
+ self.q_norm = InternRMSNorm(self.embed_dim, eps=config.layer_norm_eps)
+ self.k_norm = InternRMSNorm(self.embed_dim, eps=config.layer_norm_eps)
+
+ if self.use_flash_attn:
+ self.inner_attn = FlashAttention(attention_dropout=config.attention_dropout)
+ self.proj = nn.Linear(self.embed_dim, self.embed_dim)
+
+ def _naive_attn(self, x):
+ B, N, C = x.shape
+ qkv = self.qkv(x).reshape(B, N, 3, self.num_heads, C // self.num_heads).permute(2, 0, 3, 1, 4)
+ q, k, v = qkv.unbind(0) # make torchscript happy (cannot use tensor as tuple)
+
+ if self.qk_normalization:
+ B_, H_, N_, D_ = q.shape
+ q = self.q_norm(q.transpose(1, 2).flatten(-2, -1)).view(B_, N_, H_, D_).transpose(1, 2)
+ k = self.k_norm(k.transpose(1, 2).flatten(-2, -1)).view(B_, N_, H_, D_).transpose(1, 2)
+
+ attn = ((q * self.scale) @ k.transpose(-2, -1))
+ attn = attn.softmax(dim=-1)
+ attn = self.attn_drop(attn)
+
+ x = (attn @ v).transpose(1, 2).reshape(B, N, C)
+ x = self.proj(x)
+ x = self.proj_drop(x)
+ return x
+
+ def _flash_attn(self, x, key_padding_mask=None, need_weights=False):
+ qkv = self.qkv(x)
+ qkv = rearrange(qkv, 'b s (three h d) -> b s three h d', three=3, h=self.num_heads)
+
+ if self.qk_normalization:
+ q, k, v = qkv.unbind(2)
+ q = self.q_norm(q.flatten(-2, -1)).view(q.shape)
+ k = self.k_norm(k.flatten(-2, -1)).view(k.shape)
+ qkv = torch.stack([q, k, v], dim=2)
+
+ context, _ = self.inner_attn(
+ qkv, key_padding_mask=key_padding_mask, need_weights=need_weights, causal=False
+ )
+ outs = self.proj(rearrange(context, 'b s h d -> b s (h d)'))
+ outs = self.proj_drop(outs)
+ return outs
+
+ def forward(self, hidden_states: torch.Tensor) -> torch.Tensor:
+ x = self._naive_attn(hidden_states) if not self.use_flash_attn else self._flash_attn(hidden_states)
+ return x
+
+
+class InternMLP(nn.Module):
+ def __init__(self, config: InternVisionConfig):
+ super().__init__()
+ self.config = config
+ self.act = ACT2FN[config.hidden_act]
+ self.fc1 = nn.Linear(config.hidden_size, config.intermediate_size)
+ self.fc2 = nn.Linear(config.intermediate_size, config.hidden_size)
+
+ def forward(self, hidden_states: torch.Tensor) -> torch.Tensor:
+ hidden_states = self.fc1(hidden_states)
+ hidden_states = self.act(hidden_states)
+ hidden_states = self.fc2(hidden_states)
+ return hidden_states
+
+
+class InternVisionEncoderLayer(nn.Module):
+ def __init__(self, config: InternVisionConfig, drop_path_rate: float):
+ super().__init__()
+ self.embed_dim = config.hidden_size
+ self.intermediate_size = config.intermediate_size
+ self.norm_type = config.norm_type
+
+ self.attn = InternAttention(config)
+ self.mlp = InternMLP(config)
+ self.norm1 = NORM2FN[self.norm_type](self.embed_dim, eps=config.layer_norm_eps)
+ self.norm2 = NORM2FN[self.norm_type](self.embed_dim, eps=config.layer_norm_eps)
+
+ self.ls1 = nn.Parameter(config.initializer_factor * torch.ones(self.embed_dim))
+ self.ls2 = nn.Parameter(config.initializer_factor * torch.ones(self.embed_dim))
+ self.drop_path1 = DropPath(drop_path_rate) if drop_path_rate > 0. else nn.Identity()
+ self.drop_path2 = DropPath(drop_path_rate) if drop_path_rate > 0. else nn.Identity()
+
+ def forward(
+ self,
+ hidden_states: torch.Tensor,
+ ) -> Tuple[torch.FloatTensor, Optional[torch.FloatTensor], Optional[Tuple[torch.FloatTensor]]]:
+ """
+ Args:
+ hidden_states (`Tuple[torch.FloatTensor, Optional[torch.FloatTensor]]`): input to the layer of shape `(batch, seq_len, embed_dim)`
+ """
+ hidden_states = hidden_states + self.drop_path1(self.attn(self.norm1(hidden_states).to(hidden_states.dtype)) * self.ls1)
+
+ hidden_states = hidden_states + self.drop_path2(self.mlp(self.norm2(hidden_states).to(hidden_states.dtype)) * self.ls2)
+
+ return hidden_states
+
+
+class InternVisionEncoder(nn.Module):
+ """
+ Transformer encoder consisting of `config.num_hidden_layers` self attention layers. Each layer is a
+ [`InternEncoderLayer`].
+
+ Args:
+ config (`InternConfig`):
+ The corresponding vision configuration for the `InternEncoder`.
+ """
+
+ def __init__(self, config: InternVisionConfig):
+ super().__init__()
+ self.config = config
+ # stochastic depth decay rule
+ # TODO: error
+ # dpr = [x.item() for x in torch.linspace(0, config.drop_path_rate, config.num_hidden_layers)]
+ dpr = np.linspace(0.0, float(config.drop_path_rate), int(config.num_hidden_layers))
+ self.layers = nn.ModuleList([
+ InternVisionEncoderLayer(config, dpr[idx]) for idx in range(config.num_hidden_layers)])
+ self.gradient_checkpointing = True
+
+ def forward(
+ self,
+ inputs_embeds,
+ output_hidden_states: Optional[bool] = None,
+ return_dict: Optional[bool] = None,
+ ) -> Union[Tuple, BaseModelOutput]:
+ r"""
+ Args:
+ inputs_embeds (`torch.FloatTensor` of shape `(batch_size, sequence_length, hidden_size)`):
+ Embedded representation of the inputs. Should be float, not int tokens.
+ output_hidden_states (`bool`, *optional*):
+ Whether or not to return the hidden states of all layers. See `hidden_states` under returned tensors
+ for more detail.
+ return_dict (`bool`, *optional*):
+ Whether or not to return a [`~utils.ModelOutput`] instead of a plain tuple.
+ """
+ output_hidden_states = (
+ output_hidden_states if output_hidden_states is not None else self.config.output_hidden_states
+ )
+ return_dict = return_dict if return_dict is not None else self.config.use_return_dict
+
+ encoder_states = () if output_hidden_states else None
+ hidden_states = inputs_embeds
+
+ for idx, encoder_layer in enumerate(self.layers):
+ if output_hidden_states:
+ encoder_states = encoder_states + (hidden_states,)
+ if self.gradient_checkpointing and self.training:
+ layer_outputs = torch.utils.checkpoint.checkpoint(
+ encoder_layer,
+ hidden_states)
+ else:
+ layer_outputs = encoder_layer(
+ hidden_states,
+ )
+ hidden_states = layer_outputs
+
+ if output_hidden_states:
+ encoder_states = encoder_states + (hidden_states,)
+
+ if not return_dict:
+ return tuple(v for v in [hidden_states, encoder_states] if v is not None)
+ return BaseModelOutput(
+ last_hidden_state=hidden_states, hidden_states=encoder_states
+ )
+
+
+class InternVisionModel(PreTrainedModel):
+ main_input_name = 'pixel_values'
+ _supports_flash_attn_2 = True
+ config_class = InternVisionConfig
+ _no_split_modules = ['InternVisionEncoderLayer']
+
+ def __init__(self, config: InternVisionConfig):
+ super().__init__(config)
+ self.config = config
+
+ self.embeddings = InternVisionEmbeddings(config)
+ self.encoder = InternVisionEncoder(config)
+
+ def resize_pos_embeddings(self, old_size, new_size, patch_size):
+ pos_emb = self.embeddings.position_embedding
+ _, num_positions, embed_dim = pos_emb.shape
+ cls_emb = pos_emb[:, :1, :]
+ pos_emb = pos_emb[:, 1:, :].reshape(1, old_size // patch_size, old_size // patch_size, -1).permute(0, 3, 1, 2)
+ pos_emb = F.interpolate(pos_emb.float(), size=new_size // patch_size, mode='bicubic', align_corners=False)
+ pos_emb = pos_emb.to(cls_emb.dtype).reshape(1, embed_dim, -1).permute(0, 2, 1)
+ pos_emb = torch.cat([cls_emb, pos_emb], dim=1)
+ self.embeddings.position_embedding = nn.Parameter(pos_emb)
+ self.embeddings.image_size = new_size
+ logger.info('Resized position embeddings from {} to {}'.format(old_size, new_size))
+
+ def get_input_embeddings(self):
+ return self.embeddings
+
+ def forward(
+ self,
+ pixel_values: Optional[torch.FloatTensor] = None,
+ output_hidden_states: Optional[bool] = None,
+ return_dict: Optional[bool] = None,
+ pixel_embeds: Optional[torch.FloatTensor] = None,
+ ) -> Union[Tuple, BaseModelOutputWithPooling]:
+ output_hidden_states = (
+ output_hidden_states if output_hidden_states is not None else self.config.output_hidden_states
+ )
+ return_dict = return_dict if return_dict is not None else self.config.use_return_dict
+
+ if pixel_values is None and pixel_embeds is None:
+ raise ValueError('You have to specify pixel_values or pixel_embeds')
+
+ if pixel_embeds is not None:
+ hidden_states = pixel_embeds
+ else:
+ if len(pixel_values.shape) == 4:
+ hidden_states = self.embeddings(pixel_values)
+ else:
+ raise ValueError(f'wrong pixel_values size: {pixel_values.shape}')
+ encoder_outputs = self.encoder(
+ inputs_embeds=hidden_states,
+ output_hidden_states=output_hidden_states,
+ return_dict=return_dict,
+ )
+ last_hidden_state = encoder_outputs.last_hidden_state
+ pooled_output = last_hidden_state[:, 0, :]
+
+ if not return_dict:
+ return (last_hidden_state, pooled_output) + encoder_outputs[1:]
+
+ return BaseModelOutputWithPooling(
+ last_hidden_state=last_hidden_state,
+ pooler_output=pooled_output,
+ hidden_states=encoder_outputs.hidden_states,
+ attentions=encoder_outputs.attentions,
+ )
diff --git a/xtuner/_lite/modelings/llava/__init__.py b/xtuner/_lite/modelings/llava/__init__.py
new file mode 100644
index 000000000..036324005
--- /dev/null
+++ b/xtuner/_lite/modelings/llava/__init__.py
@@ -0,0 +1,3 @@
+from .configuration_llava import EnhancedLlavaConfig
+from .modeling_llava import LlavaForConditionalGeneration
+from .processing_llava import LlavaProcessor
diff --git a/xtuner/_lite/modelings/llava/configuration_internlm2.py b/xtuner/_lite/modelings/llava/configuration_internlm2.py
new file mode 100644
index 000000000..8b8107947
--- /dev/null
+++ b/xtuner/_lite/modelings/llava/configuration_internlm2.py
@@ -0,0 +1,175 @@
+# Copyright (c) The InternLM team and The HuggingFace Inc. team. All rights reserved.
+#
+# This code is based on transformers/src/transformers/models/llama/configuration_llama.py
+#
+# Licensed under the Apache License, Version 2.0 (the "License");
+# you may not use this file except in compliance with the License.
+# You may obtain a copy of the License at
+#
+# http://www.apache.org/licenses/LICENSE-2.0
+#
+# Unless required by applicable law or agreed to in writing, software
+# distributed under the License is distributed on an "AS IS" BASIS,
+# WITHOUT WARRANTIES OR CONDITIONS OF ANY KIND, either express or implied.
+# See the License for the specific language governing permissions and
+# limitations under the License.
+""" InternLM2 model configuration"""
+
+from transformers.configuration_utils import PretrainedConfig
+from transformers.utils import logging
+
+logger = logging.get_logger(__name__)
+
+INTERNLM2_PRETRAINED_CONFIG_ARCHIVE_MAP = {}
+
+
+# Modified from transformers.model.llama.configuration_llama.LlamaConfig
+class InternLM2Config(PretrainedConfig):
+ r"""
+ This is the configuration class to store the configuration of a [`InternLM2Model`]. It is used to instantiate
+ an InternLM2 model according to the specified arguments, defining the model architecture. Instantiating a
+ configuration with the defaults will yield a similar configuration to that of the InternLM2-7B.
+ Configuration objects inherit from [`PretrainedConfig`] and can be used to control the model outputs. Read the
+ documentation from [`PretrainedConfig`] for more information.
+ Args:
+ vocab_size (`int`, *optional*, defaults to 32000):
+ Vocabulary size of the InternLM2 model. Defines the number of different tokens that can be represented by the
+ `inputs_ids` passed when calling [`InternLM2Model`]
+ hidden_size (`int`, *optional*, defaults to 4096):
+ Dimension of the hidden representations.
+ intermediate_size (`int`, *optional*, defaults to 11008):
+ Dimension of the MLP representations.
+ num_hidden_layers (`int`, *optional*, defaults to 32):
+ Number of hidden layers in the Transformer decoder.
+ num_attention_heads (`int`, *optional*, defaults to 32):
+ Number of attention heads for each attention layer in the Transformer decoder.
+ num_key_value_heads (`int`, *optional*):
+ This is the number of key_value heads that should be used to implement Grouped Query Attention. If
+ `num_key_value_heads=num_attention_heads`, the model will use Multi Head Attention (MHA), if
+ `num_key_value_heads=1 the model will use Multi Query Attention (MQA) otherwise GQA is used. When
+ converting a multi-head checkpoint to a GQA checkpoint, each group key and value head should be constructed
+ by meanpooling all the original heads within that group. For more details checkout [this
+ paper](https://arxiv.org/pdf/2305.13245.pdf). If it is not specified, will default to
+ `num_attention_heads`.
+ hidden_act (`str` or `function`, *optional*, defaults to `"silu"`):
+ The non-linear activation function (function or string) in the decoder.
+ max_position_embeddings (`int`, *optional*, defaults to 2048):
+ The maximum sequence length that this model might ever be used with. InternLM2 supports up to 32768 tokens.
+ initializer_range (`float`, *optional*, defaults to 0.02):
+ The standard deviation of the truncated_normal_initializer for initializing all weight matrices.
+ rms_norm_eps (`float`, *optional*, defaults to 1e-06):
+ The epsilon used by the rms normalization layers.
+ use_cache (`bool`, *optional*, defaults to `True`):
+ Whether or not the model should return the last key/values attentions (not used by all models). Only
+ relevant if `config.is_decoder=True`.
+ pad_token_id (`int`, *optional*):
+ Padding token id.
+ bos_token_id (`int`, *optional*, defaults to 1):
+ Beginning of stream token id.
+ eos_token_id (`int`, *optional*, defaults to 2):
+ End of stream token id.
+ pretraining_tp (`int`, *optional*, defaults to 1):
+ Experimental feature. Tensor parallelism rank used during pretraining. Please refer to [this
+ document](https://huggingface.co/docs/transformers/main/perf_train_gpu_many#tensor-parallelism)
+ to understand more about it. This value is necessary to ensure exact reproducibility
+ of the pretraining results. Please refer to [this
+ issue](https://github.com/pytorch/pytorch/issues/76232).
+ tie_word_embeddings (`bool`, *optional*, defaults to `False`):
+ Whether to tie weight embeddings
+ rope_theta (`float`, *optional*, defaults to 10000.0):
+ The base period of the RoPE embeddings.
+ rope_scaling (`Dict`, *optional*):
+ Dictionary containing the scaling configuration for the RoPE embeddings. Currently supports two scaling
+ strategies: linear and dynamic. Their scaling factor must be a float greater than 1. The expected format is
+ `{"type": strategy name, "factor": scaling factor}`. When using this flag, don't update
+ `max_position_embeddings` to the expected new maximum. See the following thread for more information on how
+ these scaling strategies behave:
+ https://www.reddit.com/r/LocalLLaMA/comments/14mrgpr/dynamically_scaled_rope_further_increases/. This is an
+ experimental feature, subject to breaking API changes in future versions.
+ """
+ _auto_class = 'AutoConfig'
+ model_type = 'internlm2'
+ keys_to_ignore_at_inference = ['past_key_values']
+
+ def __init__( # pylint: disable=W0102
+ self,
+ vocab_size=103168,
+ hidden_size=4096,
+ intermediate_size=11008,
+ num_hidden_layers=32,
+ num_attention_heads=32,
+ num_key_value_heads=None,
+ hidden_act='silu',
+ max_position_embeddings=2048,
+ initializer_range=0.02,
+ rms_norm_eps=1e-6,
+ use_cache=True,
+ pad_token_id=0,
+ bos_token_id=1,
+ eos_token_id=2,
+ pretraining_tp=1,
+ tie_word_embeddings=False,
+ bias=True,
+ rope_theta=10000,
+ rope_scaling=None,
+ attn_implementation=None,
+ **kwargs,
+ ):
+ self.vocab_size = vocab_size
+ self.max_position_embeddings = max_position_embeddings
+ self.hidden_size = hidden_size
+ self.intermediate_size = intermediate_size
+ self.num_hidden_layers = num_hidden_layers
+ self.num_attention_heads = num_attention_heads
+ self.bias = bias
+
+ if num_key_value_heads is None:
+ num_key_value_heads = num_attention_heads
+ self.num_key_value_heads = num_key_value_heads
+
+ self.hidden_act = hidden_act
+ self.initializer_range = initializer_range
+ self.rms_norm_eps = rms_norm_eps
+ self.pretraining_tp = pretraining_tp
+ self.use_cache = use_cache
+ self.rope_theta = rope_theta
+ self.rope_scaling = rope_scaling
+ self._rope_scaling_validation()
+ self.attn_implementation = attn_implementation
+ if self.attn_implementation is None:
+ self.attn_implementation = 'eager'
+
+ super().__init__(
+ pad_token_id=pad_token_id,
+ bos_token_id=bos_token_id,
+ eos_token_id=eos_token_id,
+ tie_word_embeddings=tie_word_embeddings,
+ **kwargs,
+ )
+
+ def _rope_scaling_validation(self):
+ """
+ Validate the `rope_scaling` configuration.
+ """
+ if self.rope_scaling is None:
+ return
+
+ if not isinstance(self.rope_scaling,
+ dict) or len(self.rope_scaling) != 2:
+ raise ValueError(
+ '`rope_scaling` must be a dictionary with with two fields, `type` and `factor`, '
+ f'got {self.rope_scaling}')
+ rope_scaling_type = self.rope_scaling.get('type', None)
+ rope_scaling_factor = self.rope_scaling.get('factor', None)
+ if rope_scaling_type is None or rope_scaling_type not in [
+ 'linear', 'dynamic'
+ ]:
+ raise ValueError(
+ f"`rope_scaling`'s type field must be one of ['linear', 'dynamic'], got {rope_scaling_type}"
+ )
+ if (rope_scaling_factor is None
+ or not isinstance(rope_scaling_factor,
+ (float, int)) or rope_scaling_factor < 1.0):
+ raise ValueError(
+ f"`rope_scaling`'s factor field must be a number >= 1, got {rope_scaling_factor} "
+ f'of type {type(rope_scaling_factor)}')
diff --git a/xtuner/_lite/modelings/llava/configuration_llava.py b/xtuner/_lite/modelings/llava/configuration_llava.py
new file mode 100644
index 000000000..f5ec7bbfa
--- /dev/null
+++ b/xtuner/_lite/modelings/llava/configuration_llava.py
@@ -0,0 +1,163 @@
+# coding=utf-8
+# Copyright 2023 Microsoft Research & University of Wisconsin-Madison and the HuggingFace Inc. team. All rights reserved.
+# Licensed under the Apache License, Version 2.0 (the "License");
+# you may not use this file except in compliance with the License.
+# You may obtain a copy of the License at
+#
+# http://www.apache.org/licenses/LICENSE-2.0
+#
+# Unless required by applicable law or agreed to in writing, software
+# distributed under the License is distributed on an "AS IS" BASIS,
+# WITHOUT WARRANTIES OR CONDITIONS OF ANY KIND, either express or implied.
+# See the License for the specific language governing permissions and
+# limitations under the License.
+"""Llava model configuration"""
+import os
+from typing import Union
+from transformers.configuration_utils import PretrainedConfig, custom_object_save
+from transformers.utils import logging
+from transformers import CONFIG_MAPPING, AutoModelForCausalLM, AutoConfig
+
+logger = logging.get_logger(__name__)
+
+class EnhancedLlavaConfig(PretrainedConfig):
+ r"""
+ This is the configuration class to store the configuration of a [`LlavaForConditionalGeneration`]. It is used to instantiate an
+ Llava model according to the specified arguments, defining the model architecture. Instantiating a configuration
+ with the defaults will yield a similar configuration to that of the Llava-9B.
+
+ e.g. [llava-hf/llava-9b](https://huggingface.co/llava-hf/llava-9b)
+
+ Configuration objects inherit from [`PretrainedConfig`] and can be used to control the model outputs. Read the
+ documentation from [`PretrainedConfig`] for more information.
+
+ Args:
+ vision_config (`Union[AutoConfig, dict]`, *optional*, defaults to `CLIPVisionConfig`):
+ The config object or dictionary of the vision backbone.
+ text_config (`Union[AutoConfig, dict]`, *optional*, defaults to `LlamaConfig`):
+ The config object or dictionary of the text backbone.
+ ignore_index (`int`, *optional*, defaults to -100):
+ The ignore index for the loss function.
+ image_token_index (`int`, *optional*, defaults to 32000):
+ The image token index to encode the image prompt.
+ projector_hidden_act (`str`, *optional*, defaults to `"gelu"`):
+ The activation function used by the multimodal projector.
+ vision_feature_select_strategy (`str`, *optional*, defaults to `"default"`):
+ The feature selection strategy used to select the vision feature from the vision backbone.
+ Can be one of `"default"` or `"full"`.
+ vision_feature_layer (`int`, *optional*, defaults to -2):
+ The index of the layer to select the vision feature.
+
+ Example:
+
+ ```python
+ >>> from transformers import LlavaForConditionalGeneration, LlavaConfig, CLIPVisionConfig, LlamaConfig
+
+ >>> # Initializing a CLIP-vision config
+ >>> vision_config = CLIPVisionConfig()
+
+ >>> # Initializing a Llama config
+ >>> text_config = LlamaConfig()
+
+ >>> # Initializing a Llava llava-1.5-7b style configuration
+ >>> configuration = LlavaConfig(vision_config, text_config)
+
+ >>> # Initializing a model from the llava-1.5-7b style configuration
+ >>> model = LlavaForConditionalGeneration(configuration)
+
+ >>> # Accessing the model configuration
+ >>> configuration = model.config
+ ```"""
+
+ _auto_class = 'AutoConfig'
+ model_type = "enhanced_llava"
+ is_composition = False
+
+ def __init__(
+ self,
+ vision_config=None,
+ text_config=None,
+ ignore_index=-100,
+ image_token_index=32000,
+ projector_hidden_act="gelu",
+ vision_feature_select_strategy="default",
+ vision_feature_layer=-2,
+ **kwargs,
+ ):
+ self.ignore_index = ignore_index
+ self.image_token_index = image_token_index
+ self.projector_hidden_act = projector_hidden_act
+
+ if vision_feature_select_strategy not in ["default", "full"]:
+ raise ValueError(
+ "vision_feature_select_strategy should be one of 'default', 'full'."
+ f"Got: {vision_feature_select_strategy}"
+ )
+
+ self.vision_feature_select_strategy = vision_feature_select_strategy
+ self.vision_feature_layer = vision_feature_layer
+
+ if isinstance(vision_config, dict):
+ vision_config["model_type"] = (
+ vision_config["model_type"] if "model_type" in vision_config else "clip_vision_model"
+ )
+ vision_config = CONFIG_MAPPING[vision_config["model_type"]](**vision_config)
+ elif vision_config is None:
+ vision_config = CONFIG_MAPPING["clip_vision_model"](
+ intermediate_size=4096,
+ hidden_size=1024,
+ patch_size=14,
+ image_size=336,
+ num_hidden_layers=24,
+ num_attention_heads=16,
+ vocab_size=32000,
+ projection_dim=768,
+ )
+
+ self.vision_config = vision_config
+
+ if isinstance(text_config, dict):
+ text_config["model_type"] = text_config["model_type"] if "model_type" in text_config else "llama"
+
+ if text_config["model_type"] == 'internlm2':
+ from .configuration_internlm2 import InternLM2Config
+ from .modeling_internlm2 import InternLM2ForCausalLM
+ AutoConfig.register('internlm2', InternLM2Config)
+ AutoModelForCausalLM.register(
+ InternLM2Config, InternLM2ForCausalLM)
+ text_config['auto_map']['AutoConfig'] = 'configuration_internlm2.InternLM2Config'
+ text_config['auto_map']['AutoModel'] = 'modeling_internlm2.InternLM2ForCausalLM'
+ text_config['auto_map']['AutoModelForCausalLM'] = 'modeling_internlm2.InternLM2ForCausalLM'
+ text_config = InternLM2Config(**text_config)
+ else:
+ text_config = CONFIG_MAPPING[text_config["model_type"]](**text_config)
+
+ elif text_config is None:
+ text_config = CONFIG_MAPPING["llama"]()
+
+ self.text_config = text_config
+
+ super().__init__(**kwargs)
+
+
+ def save_pretrained(self, save_directory: Union[str, os.PathLike], push_to_hub: bool = False, **kwargs):
+ """
+ Save a configuration object to the directory `save_directory`, so that it can be re-loaded using the
+ [`~PretrainedConfig.from_pretrained`] class method.
+
+ Args:
+ save_directory (`str` or `os.PathLike`):
+ Directory where the configuration JSON file will be saved (will be created if it does not exist).
+ push_to_hub (`bool`, *optional*, defaults to `False`):
+ Whether or not to push your model to the Hugging Face model hub after saving it. You can specify the
+ repository you want to push to with `repo_id` (will default to the name of `save_directory` in your
+ namespace).
+ kwargs (`Dict[str, Any]`, *optional*):
+ Additional key word arguments passed along to the [`~utils.PushToHubMixin.push_to_hub`] method.
+ """
+ super().save_pretrained(save_directory, push_to_hub, **kwargs)
+
+ if self.text_config._auto_class is not None:
+ custom_object_save(self.text_config, save_directory, config=self.text_config)
+
+AutoConfig.register('enhanced_llava', EnhancedLlavaConfig, exist_ok=True)
\ No newline at end of file
diff --git a/xtuner/_lite/modelings/llava/modeling_internlm2.py b/xtuner/_lite/modelings/llava/modeling_internlm2.py
new file mode 100644
index 000000000..69ddc6196
--- /dev/null
+++ b/xtuner/_lite/modelings/llava/modeling_internlm2.py
@@ -0,0 +1,1899 @@
+# Copyright (c) The InternLM team and The HuggingFace Inc. team. All rights reserved.
+#
+# This code is based on transformers/src/transformers/models/llama/modeling_llama.py
+#
+# Licensed under the Apache License, Version 2.0 (the "License");
+# you may not use this file except in compliance with the License.
+# You may obtain a copy of the License at
+#
+# http://www.apache.org/licenses/LICENSE-2.0
+#
+# Unless required by applicable law or agreed to in writing, software
+# distributed under the License is distributed on an "AS IS" BASIS,
+# WITHOUT WARRANTIES OR CONDITIONS OF ANY KIND, either express or implied.
+# See the License for the specific language governing permissions and
+# limitations under the License.
+"""PyTorch InternLM2.5 model."""
+import math
+import queue
+import threading
+from typing import List, Optional, Tuple, Union
+
+import torch
+import torch.nn.functional as F
+import torch.utils.checkpoint
+from einops import rearrange
+from torch import nn
+from torch.nn import BCEWithLogitsLoss, CrossEntropyLoss, MSELoss
+from transformers.activations import ACT2FN
+from transformers.cache_utils import Cache, DynamicCache, StaticCache
+from transformers.modeling_attn_mask_utils import AttentionMaskConverter
+from transformers.modeling_outputs import (BaseModelOutputWithPast,
+ CausalLMOutputWithPast,
+ QuestionAnsweringModelOutput,
+ SequenceClassifierOutputWithPast,
+ TokenClassifierOutput)
+from transformers.modeling_utils import PreTrainedModel
+from transformers.pytorch_utils import ALL_LAYERNORM_LAYERS
+from transformers.utils import (add_start_docstrings,
+ add_start_docstrings_to_model_forward,
+ is_flash_attn_greater_or_equal_2_10, logging,
+ replace_return_docstrings)
+
+try:
+ from transformers.generation.streamers import BaseStreamer
+except Exception:
+ BaseStreamer = None
+
+from .configuration_internlm2 import InternLM2Config
+
+try:
+ from flash_attn import flash_attn_func, flash_attn_varlen_func
+ from flash_attn.bert_padding import (index_first_axis, pad_input,
+ unpad_input)
+except:
+ pass
+
+logger = logging.get_logger(__name__)
+
+_CONFIG_FOR_DOC = 'InternLM2Config'
+
+
+def _get_unpad_data(attention_mask):
+ seqlens_in_batch = attention_mask.sum(dim=-1, dtype=torch.int32)
+ indices = torch.nonzero(attention_mask.flatten(), as_tuple=False).flatten()
+ max_seqlen_in_batch = seqlens_in_batch.max().item()
+ cu_seqlens = F.pad(
+ torch.cumsum(seqlens_in_batch, dim=0, dtype=torch.int32), (1, 0)) # pylint: disable=E1102
+ return (
+ indices,
+ cu_seqlens,
+ max_seqlen_in_batch,
+ )
+
+
+class InternLM2RMSNorm(nn.Module):
+ """InternLM2RMSNorm is equivalent to T5LayerNorm."""
+
+ def __init__(self, hidden_size, eps=1e-6):
+ super().__init__()
+ self.weight = nn.Parameter(torch.ones(hidden_size))
+ self.variance_epsilon = eps
+
+ def forward(self, hidden_states):
+ input_dtype = hidden_states.dtype
+ hidden_states = hidden_states.to(torch.float32)
+ variance = hidden_states.pow(2).mean(-1, keepdim=True)
+ hidden_states = hidden_states * torch.rsqrt(variance +
+ self.variance_epsilon)
+ return self.weight * hidden_states.to(input_dtype)
+
+
+ALL_LAYERNORM_LAYERS.append(InternLM2RMSNorm)
+
+
+class InternLM2RotaryEmbedding(nn.Module):
+ """Rotary Position Embedding for the InternLM2 model. Credits to the Reddit user /u/lucidrains."""
+
+ def __init__(self,
+ dim,
+ max_position_embeddings=2048,
+ base=10000,
+ device=None,
+ scaling_factor=1.0):
+ super().__init__()
+ self.scaling_factor = scaling_factor
+ self.dim = dim
+ self.max_position_embeddings = max_position_embeddings
+ self.base = base
+ inv_freq = 1.0 / (
+ self.base
+ **(torch.arange(0, self.dim, 2,
+ dtype=torch.int64).float().to(device) / self.dim))
+ self.register_buffer('inv_freq', inv_freq, persistent=False)
+ # For BC we register cos and sin cached
+ self.max_seq_len_cached = max_position_embeddings
+
+ @torch.no_grad()
+ def forward(self, x, position_ids):
+ # x: [bs, num_attention_heads, seq_len, head_size]
+ inv_freq_expanded = self.inv_freq[None, :, None].float().expand(
+ position_ids.shape[0], -1, 1)
+ position_ids_expanded = position_ids[:, None, :].float()
+ # Force float32 since bfloat16 loses precision on long contexts
+ # See https://github.com/huggingface/transformers/pull/29285
+ device_type = x.device.type
+ device_type = device_type if isinstance(
+ device_type, str) and device_type != 'mps' else 'cpu'
+ with torch.autocast(device_type=device_type, enabled=False):
+ freqs = (inv_freq_expanded.float()
+ @ position_ids_expanded.float()).transpose(1, 2)
+ emb = torch.cat((freqs, freqs), dim=-1)
+ cos = emb.cos()
+ sin = emb.sin()
+ return cos.to(dtype=x.dtype), sin.to(dtype=x.dtype)
+
+
+class InternLM2LinearScalingRotaryEmbedding(InternLM2RotaryEmbedding):
+ """InternLM2RotaryEmbedding extended with linear scaling. Credits to the Reddit user /u/kaiokendev"""
+
+ def forward(self, x, position_ids):
+ # difference to the original RoPE: a scaling factor is aplied to the position ids
+ position_ids = position_ids.float() / self.scaling_factor
+ cos, sin = super().forward(x, position_ids)
+ return cos, sin
+
+
+class InternLM2DynamicNTKScalingRotaryEmbedding(InternLM2RotaryEmbedding):
+ """InternLM2RotaryEmbedding extended with Dynamic NTK scaling.
+ Credits to the Reddit users /u/bloc97 and /u/emozilla"""
+
+ def forward(self, x, position_ids):
+ # difference to the original RoPE: inv_freq is recomputed when the sequence length > original length
+ seq_len = torch.max(position_ids) + 1
+ if seq_len > self.max_position_embeddings:
+ base = self.base * ((self.scaling_factor * seq_len /
+ self.max_position_embeddings) -
+ (self.scaling_factor - 1))**(
+ self.dim / (self.dim - 2))
+ inv_freq = 1.0 / (
+ base
+ **(torch.arange(0, self.dim, 2, dtype=torch.int64).float().to(
+ x.device) / self.dim))
+ self.register_buffer(
+ 'inv_freq', inv_freq,
+ persistent=False) # TODO joao: this may break with compilation
+
+ cos, sin = super().forward(x, position_ids)
+ return cos, sin
+
+
+def rotate_half(x):
+ """Rotates half the hidden dims of the input."""
+ x1 = x[..., :x.shape[-1] // 2]
+ x2 = x[..., x.shape[-1] // 2:]
+ return torch.cat((-x2, x1), dim=-1)
+
+
+def apply_rotary_pos_emb(q, k, cos, sin, position_ids=None, unsqueeze_dim=1): # pylint: disable=unused-argument
+ """Applies Rotary Position Embedding to the query and key tensors.
+ Args:
+ q (`torch.Tensor`): The query tensor.
+ k (`torch.Tensor`): The key tensor.
+ cos (`torch.Tensor`): The cosine part of the rotary embedding.
+ sin (`torch.Tensor`): The sine part of the rotary embedding.
+ position_ids (`torch.Tensor`, *optional*):
+ Deprecated and unused.
+ unsqueeze_dim (`int`, *optional*, defaults to 1):
+ The 'unsqueeze_dim' argument specifies the dimension along which to unsqueeze cos[position_ids] and
+ sin[position_ids] so that they can be properly broadcasted to the dimensions of q and k. For example, note
+ that cos[position_ids] and sin[position_ids] have the shape [batch_size, seq_len, head_dim]. Then, if q and
+ k have the shape [batch_size, heads, seq_len, head_dim], then setting unsqueeze_dim=1 makes
+ cos[position_ids] and sin[position_ids] broadcastable to the shapes of q and k. Similarly, if q and k have
+ the shape [batch_size, seq_len, heads, head_dim], then set unsqueeze_dim=2.
+ Returns:
+ `tuple(torch.Tensor)` comprising of the query and key tensors rotated using the Rotary Position Embedding.
+ """
+ cos = cos.unsqueeze(unsqueeze_dim)
+ sin = sin.unsqueeze(unsqueeze_dim)
+ q_embed = (q * cos) + (rotate_half(q) * sin)
+ k_embed = (k * cos) + (rotate_half(k) * sin)
+ return q_embed, k_embed
+
+
+class InternLM2MLP(nn.Module):
+ """MLP for InternLM2 model."""
+
+ def __init__(self, config):
+ super().__init__()
+ self.config = config
+ self.hidden_size = config.hidden_size
+ self.intermediate_size = config.intermediate_size
+ self.w1 = nn.Linear(
+ self.hidden_size, self.intermediate_size, bias=False)
+ self.w3 = nn.Linear(
+ self.hidden_size, self.intermediate_size, bias=False)
+ self.w2 = nn.Linear(
+ self.intermediate_size, self.hidden_size, bias=False)
+ self.act_fn = ACT2FN[config.hidden_act]
+
+ def forward(self, x):
+ down_proj = self.w2(self.act_fn(self.w1(x)) * self.w3(x))
+
+ return down_proj
+
+
+def repeat_kv(hidden_states: torch.Tensor, n_rep: int) -> torch.Tensor:
+ """
+ This is the equivalent of torch.repeat_interleave(x, dim=1, repeats=n_rep). The hidden states go from (batch,
+ num_key_value_heads, seqlen, head_dim) to (batch, num_attention_heads, seqlen, head_dim)
+ """
+ batch, num_key_value_heads, slen, head_dim = hidden_states.shape
+ if n_rep == 1:
+ return hidden_states
+ hidden_states = hidden_states[:, :,
+ None, :, :].expand(batch,
+ num_key_value_heads,
+ n_rep, slen, head_dim)
+ return hidden_states.reshape(batch, num_key_value_heads * n_rep, slen,
+ head_dim)
+
+
+class InternLM2Attention(nn.Module):
+ """Multi-headed attention from 'Attention Is All You Need' paper"""
+
+ def __init__(self,
+ config: InternLM2Config,
+ layer_idx: Optional[int] = None):
+ super().__init__()
+ self.config = config
+ self.layer_idx = layer_idx
+ if layer_idx is None:
+ logger.warning_once(
+ f'Instantiating {self.__class__.__name__} without passing a `layer_idx` is not recommended and will '
+ 'lead to errors during the forward call if caching is used. Please make sure to provide a `layer_idx` '
+ 'when creating this class.')
+
+ self.hidden_size = config.hidden_size
+ self.num_heads = config.num_attention_heads
+ self.head_dim = self.hidden_size // self.num_heads
+ self.num_key_value_heads = config.num_key_value_heads
+ self.num_key_value_groups = self.num_heads // self.num_key_value_heads
+ self.max_position_embeddings = config.max_position_embeddings
+ self.rope_theta = config.rope_theta
+ self.is_causal = True
+
+ if (self.head_dim * self.num_heads) != self.hidden_size:
+ raise ValueError(
+ f'hidden_size must be divisible by num_heads (got `hidden_size`: {self.hidden_size}'
+ f' and `num_heads`: {self.num_heads}).')
+
+ self.wqkv = nn.Linear(
+ self.hidden_size,
+ (self.num_heads + 2 * self.num_key_value_heads) * self.head_dim,
+ bias=config.bias,
+ )
+ self.wo = nn.Linear(
+ self.num_heads * self.head_dim, self.hidden_size, bias=config.bias)
+
+ self._init_rope()
+
+ def _init_rope(self):
+ if self.config.rope_scaling is None:
+ self.rotary_emb = InternLM2RotaryEmbedding(
+ self.head_dim,
+ max_position_embeddings=self.max_position_embeddings,
+ base=self.rope_theta,
+ )
+ else:
+ scaling_type = self.config.rope_scaling['type']
+ scaling_factor = self.config.rope_scaling['factor']
+ if scaling_type == 'linear':
+ self.rotary_emb = InternLM2LinearScalingRotaryEmbedding(
+ self.head_dim,
+ max_position_embeddings=self.max_position_embeddings,
+ scaling_factor=scaling_factor,
+ base=self.rope_theta,
+ )
+ elif scaling_type == 'dynamic':
+ self.rotary_emb = InternLM2DynamicNTKScalingRotaryEmbedding(
+ self.head_dim,
+ max_position_embeddings=self.max_position_embeddings,
+ scaling_factor=scaling_factor,
+ base=self.rope_theta,
+ )
+ else:
+ raise ValueError(f'Unknown RoPE scaling type {scaling_type}')
+
+ def forward(
+ self,
+ hidden_states: torch.Tensor,
+ attention_mask: Optional[torch.Tensor] = None,
+ position_ids: Optional[torch.LongTensor] = None,
+ past_key_value: Optional[Cache] = None,
+ output_attentions: bool = False,
+ use_cache: bool = False, # pylint: disable=unused-argument
+ cache_position: Optional[torch.LongTensor] = None,
+ ) -> Tuple[torch.Tensor, Optional[torch.Tensor],
+ Optional[Tuple[torch.Tensor]]]:
+ bsz, q_len, _ = hidden_states.size()
+
+ if self.config.pretraining_tp > 1:
+ # split qkv_states by tp size
+ key_value_slicing = (self.num_key_value_heads *
+ self.head_dim) // self.config.pretraining_tp
+ qkv_slices = self.wqkv.weight.split(key_value_slicing, dim=0)
+ qkv_states = torch.cat(
+ [
+ F.linear(hidden_states, qkv_slice)
+ for qkv_slice in qkv_slices
+ ],
+ dim=-1 # pylint: disable=E1102
+ )
+ else:
+ qkv_states = self.wqkv(hidden_states)
+
+ qkv_states = rearrange(
+ qkv_states,
+ 'b q (h gs d) -> b q h gs d',
+ gs=2 + self.num_key_value_groups,
+ d=self.head_dim,
+ )
+
+ query_states = qkv_states[..., :self.num_key_value_groups, :]
+ query_states = rearrange(query_states,
+ 'b q h gs d -> b q (h gs) d').transpose(1, 2)
+ key_states = qkv_states[..., -2, :].transpose(1, 2)
+ value_states = qkv_states[..., -1, :].transpose(1, 2)
+
+ cos, sin = self.rotary_emb(value_states, position_ids)
+ query_states, key_states = apply_rotary_pos_emb(
+ query_states, key_states, cos, sin, position_ids)
+
+ if past_key_value is not None:
+ # sin and cos are specific to RoPE models; cache_position needed for the static cache
+ cache_kwargs = {
+ 'sin': sin,
+ 'cos': cos,
+ 'cache_position': cache_position
+ }
+ key_states, value_states = past_key_value.update(
+ key_states, value_states, self.layer_idx, cache_kwargs)
+
+ key_states = repeat_kv(key_states, self.num_key_value_groups)
+ value_states = repeat_kv(value_states, self.num_key_value_groups)
+
+ attn_weights = torch.matmul(query_states, key_states.transpose(
+ 2, 3)) / math.sqrt(self.head_dim)
+
+ if attention_mask is not None: # no matter the length, we just slice it
+ causal_mask = attention_mask[:, :, :, :key_states.shape[-2]]
+ attn_weights = attn_weights + causal_mask
+
+ # upcast attention to fp32
+ attn_weights = nn.functional.softmax(
+ attn_weights, dim=-1, dtype=torch.float32).to(query_states.dtype)
+ attn_output = torch.matmul(attn_weights, value_states)
+
+ if attn_output.size() != (bsz, self.num_heads, q_len, self.head_dim):
+ raise ValueError(
+ f'`attn_output` should be of size {(bsz, self.num_heads, q_len, self.head_dim)}, but is'
+ f' {attn_output.size()}')
+
+ attn_output = attn_output.transpose(1, 2).contiguous()
+
+ attn_output = attn_output.reshape(bsz, q_len, self.hidden_size)
+
+ if self.config.pretraining_tp > 1:
+ attn_output = attn_output.split(
+ self.hidden_size // self.config.pretraining_tp, dim=2)
+ o_proj_slices = self.wo.weight.split(
+ self.hidden_size // self.config.pretraining_tp, dim=1)
+ attn_output = sum([
+ F.linear(attn_output[i], o_proj_slices[i]) # pylint: disable=E1102
+ for i in range(self.config.pretraining_tp)
+ ])
+ else:
+ attn_output = self.wo(attn_output)
+
+ if not output_attentions:
+ attn_weights = None
+
+ return attn_output, attn_weights, past_key_value
+
+
+class InternLM2FlashAttention2(InternLM2Attention):
+ """
+ InternLM2 flash attention module. This module inherits from `InternLM2Attention` as the weights of the module stays
+ untouched. The only required change would be on the forward pass where it needs to correctly call the public API of
+ flash attention and deal with padding tokens in case the input contains any of them.
+ """
+
+ def __init__(self, *args, **kwargs):
+ super().__init__(*args, **kwargs)
+
+ # TODO: Should be removed once Flash Attention for RoCm is bumped to 2.1.
+ # flash_attn<2.1 generates top-left aligned causal mask, while what is needed here is bottom-right alignement,
+ # that was made default for flash_attn>=2.1. This attribute is used to handle this difference.
+ # Reference: https://github.com/Dao-AILab/flash-attention/releases/tag/v2.1.0.
+ # Beware that with flash_attn<2.1, using q_seqlen != k_seqlen (except for the case q_seqlen == 1)
+ # produces a wrong mask (top-left).
+ self._flash_attn_uses_top_left_mask = not is_flash_attn_greater_or_equal_2_10(
+ )
+
+ def forward(
+ self,
+ hidden_states: torch.Tensor,
+ attention_mask: Optional[torch.LongTensor] = None,
+ position_ids: Optional[torch.LongTensor] = None,
+ past_key_value: Optional[Cache] = None,
+ output_attentions: bool = False,
+ use_cache: bool = False,
+ cache_position: Optional[torch.LongTensor] = None,
+ ) -> Tuple[torch.Tensor, Optional[torch.Tensor],
+ Optional[Tuple[torch.Tensor]]]:
+ if isinstance(past_key_value, StaticCache):
+ raise ValueError(
+ '`static` cache implementation is not compatible with `attn_implementation==flash_attention_2` '
+ 'make sure to use `sdpa` in the mean time, and open an issue at '
+ 'https://github.com/huggingface/transformers')
+
+ output_attentions = False
+
+ bsz, q_len, _ = hidden_states.size()
+
+ qkv_states = self.wqkv(hidden_states)
+
+ qkv_states = rearrange(
+ qkv_states,
+ 'b q (h gs d) -> b q h gs d',
+ gs=2 + self.num_key_value_groups,
+ d=self.head_dim,
+ )
+
+ query_states = qkv_states[..., :self.num_key_value_groups, :]
+ query_states = rearrange(query_states, 'b q h gs d -> b q (h gs) d')
+ key_states = qkv_states[..., -2, :]
+ value_states = qkv_states[..., -1, :]
+
+ query_states = query_states.transpose(1, 2)
+ key_states = key_states.transpose(1, 2)
+ value_states = value_states.transpose(1, 2)
+
+ cos, sin = self.rotary_emb(value_states, position_ids)
+ query_states, key_states = apply_rotary_pos_emb(
+ query_states, key_states, cos, sin)
+
+ if past_key_value is not None:
+ # sin and cos are specific to RoPE models; cache_position needed for the static cache
+ cache_kwargs = {
+ 'sin': sin,
+ 'cos': cos,
+ 'cache_position': cache_position
+ }
+ key_states, value_states = past_key_value.update(
+ key_states, value_states, self.layer_idx, cache_kwargs)
+
+ # TODO: These transpose are quite inefficient but Flash Attention requires the layout
+ # [batch_size, sequence_length, num_heads, head_dim]. We would need to refactor the KV cache
+ # to be able to avoid many of these transpose/reshape/view.
+ query_states = query_states.transpose(1, 2)
+ key_states = key_states.transpose(1, 2)
+ value_states = value_states.transpose(1, 2)
+
+ # dropout_rate = self.attention_dropout if self.training else 0.0
+ dropout_rate = 0.0
+
+ # In PEFT, usually we cast the layer norms in float32 for training stability reasons
+ # therefore the input hidden states gets silently casted in float32. Hence, we need
+ # cast them back in the correct dtype just to be sure everything works as expected.
+ # This might slowdown training & inference so it is recommended to not cast the LayerNorms
+ # in fp32. (InternLM2RMSNorm handles it correctly)
+
+ input_dtype = query_states.dtype
+ if input_dtype == torch.float32:
+ if torch.is_autocast_enabled():
+ target_dtype = torch.get_autocast_gpu_dtype()
+ # Handle the case where the model is quantized
+ elif hasattr(self.config, '_pre_quantization_dtype'):
+ target_dtype = self.config._pre_quantization_dtype
+ else:
+ target_dtype = self.wqkv.weight.dtype
+
+ logger.warning_once(
+ f'The input hidden states seems to be silently casted in float32, this might be related to'
+ f' the fact you have upcasted embedding or layer norm layers in float32. We will cast back the input in'
+ f' {target_dtype}.')
+
+ query_states = query_states.to(target_dtype)
+ key_states = key_states.to(target_dtype)
+ value_states = value_states.to(target_dtype)
+
+ attn_output = self._flash_attention_forward(
+ query_states,
+ key_states,
+ value_states,
+ attention_mask,
+ q_len,
+ dropout=dropout_rate)
+
+ attn_output = attn_output.reshape(bsz, q_len,
+ self.hidden_size).contiguous()
+ attn_output = self.wo(attn_output)
+
+ if not output_attentions:
+ attn_weights = None
+
+ return attn_output, attn_weights, past_key_value # pylint: disable=E0606
+
+ def _flash_attention_forward(self,
+ query_states,
+ key_states,
+ value_states,
+ attention_mask,
+ query_length,
+ dropout=0.0,
+ softmax_scale=None):
+ """
+ Calls the forward method of Flash Attention - if the input hidden states contain at least one padding token
+ first unpad the input, then computes the attention scores and pad the final attention scores.
+ Args:
+ query_states (`torch.Tensor`):
+ Input query states to be passed to Flash Attention API
+ key_states (`torch.Tensor`):
+ Input key states to be passed to Flash Attention API
+ value_states (`torch.Tensor`):
+ Input value states to be passed to Flash Attention API
+ attention_mask (`torch.Tensor`):
+ The padding mask - corresponds to a tensor of size `(batch_size, seq_len)` where 0 stands for the
+ position of padding tokens and 1 for the position of non-padding tokens.
+ dropout (`float`):
+ Attention dropout
+ softmax_scale (`float`, *optional*):
+ The scaling of QK^T before applying softmax. Default to 1 / sqrt(head_dim)
+ """
+ if not self._flash_attn_uses_top_left_mask:
+ causal = self.is_causal
+ else:
+ # TODO: Remove the `query_length != 1` check once Flash Attention for RoCm is bumped to 2.1.
+ # For details, please see the comment in InternLM2FlashAttention2 __init__.
+ causal = self.is_causal and query_length != 1
+
+ # Contains at least one padding token in the sequence
+ if attention_mask is not None:
+ batch_size = query_states.shape[0]
+ query_states, key_states, value_states, indices_q, cu_seq_lens, max_seq_lens = self._upad_input(
+ query_states, key_states, value_states, attention_mask,
+ query_length)
+
+ cu_seqlens_q, cu_seqlens_k = cu_seq_lens
+ max_seqlen_in_batch_q, max_seqlen_in_batch_k = max_seq_lens
+
+ attn_output_unpad = flash_attn_varlen_func( # pylint: disable=E0606
+ query_states,
+ key_states,
+ value_states,
+ cu_seqlens_q=cu_seqlens_q,
+ cu_seqlens_k=cu_seqlens_k,
+ max_seqlen_q=max_seqlen_in_batch_q,
+ max_seqlen_k=max_seqlen_in_batch_k,
+ dropout_p=dropout,
+ softmax_scale=softmax_scale,
+ causal=causal,
+ )
+
+ attn_output = pad_input(attn_output_unpad, indices_q, batch_size,
+ query_length) # pylint: disable=E0606
+ else:
+ attn_output = flash_attn_func( # pylint: disable=E0606
+ query_states,
+ key_states,
+ value_states,
+ dropout,
+ softmax_scale=softmax_scale,
+ causal=causal)
+
+ return attn_output
+
+ def _upad_input(self, query_layer, key_layer, value_layer, attention_mask,
+ query_length):
+ indices_k, cu_seqlens_k, max_seqlen_in_batch_k = _get_unpad_data(
+ attention_mask)
+ batch_size, kv_seq_len, num_key_value_heads, head_dim = key_layer.shape
+
+ key_layer = index_first_axis( # pylint: disable=E0606
+ key_layer.reshape(batch_size * kv_seq_len, num_key_value_heads,
+ head_dim), indices_k)
+ value_layer = index_first_axis( # pylint: disable=E0606
+ value_layer.reshape(batch_size * kv_seq_len, num_key_value_heads,
+ head_dim), indices_k)
+ if query_length == kv_seq_len:
+ query_layer = index_first_axis( # pylint: disable=E0606
+ query_layer.reshape(batch_size * kv_seq_len, self.num_heads,
+ head_dim), indices_k)
+ cu_seqlens_q = cu_seqlens_k
+ max_seqlen_in_batch_q = max_seqlen_in_batch_k
+ indices_q = indices_k
+ elif query_length == 1:
+ max_seqlen_in_batch_q = 1
+ cu_seqlens_q = torch.arange(
+ batch_size + 1, dtype=torch.int32, device=query_layer.device
+ ) # There is a memcpy here, that is very bad.
+ indices_q = cu_seqlens_q[:-1]
+ query_layer = query_layer.squeeze(1)
+ else:
+ # The -q_len: slice assumes left padding.
+ attention_mask = attention_mask[:, -query_length:]
+ query_layer, indices_q, cu_seqlens_q, max_seqlen_in_batch_q = unpad_input( # pylint: disable=E0606
+ query_layer, attention_mask)
+
+ return (
+ query_layer,
+ key_layer,
+ value_layer,
+ indices_q,
+ (cu_seqlens_q, cu_seqlens_k),
+ (max_seqlen_in_batch_q, max_seqlen_in_batch_k),
+ )
+
+
+# Copied from transformers.models.llama.modeling_llama.LllamaSdpaAttention with Llama->InternLM2
+class InternLM2SdpaAttention(InternLM2Attention):
+ """
+ InternLM2 attention module using torch.nn.functional.scaled_dot_product_attention. This module inherits from
+ `InternLM2Attention` as the weights of the module stays untouched. The only changes are on the forward pass
+ to adapt to SDPA API.
+ """
+
+ # Adapted from InternLM2Attention.forward
+ def forward(
+ self,
+ hidden_states: torch.Tensor,
+ attention_mask: Optional[torch.Tensor] = None,
+ position_ids: Optional[torch.LongTensor] = None,
+ past_key_value: Optional[Cache] = None,
+ output_attentions: bool = False,
+ use_cache: bool = False,
+ cache_position: Optional[torch.LongTensor] = None,
+ ) -> Tuple[torch.Tensor, Optional[torch.Tensor],
+ Optional[Tuple[torch.Tensor]]]:
+ if output_attentions:
+ # TODO: Improve this warning with e.g. `model.config.attn_implementation = "manual"`
+ # once this is implemented.
+ logger.warning_once(
+ 'InternLM2Model uses InternLM2SdpaAttention, but `torch.nn.functional.scaled_dot_product_attention` '
+ 'does not support `output_attentions=True`. Falling back to the manual attention implementation, '
+ 'but specifying the manual implementation will be required from Transformers version v5.0.0 onwards. '
+ 'This warning can be removed using the argument `attn_implementation="eager"` when loading the model.'
+ )
+ return super().forward(
+ hidden_states=hidden_states,
+ attention_mask=attention_mask,
+ position_ids=position_ids,
+ past_key_value=past_key_value,
+ output_attentions=output_attentions,
+ use_cache=use_cache,
+ cache_position=cache_position,
+ )
+
+ bsz, q_len, _ = hidden_states.size()
+
+ qkv_states = self.wqkv(hidden_states)
+
+ qkv_states = rearrange(
+ qkv_states,
+ 'b q (h gs d) -> b q h gs d',
+ gs=2 + self.num_key_value_groups,
+ d=self.head_dim,
+ )
+
+ query_states = qkv_states[..., :self.num_key_value_groups, :]
+ query_states = rearrange(query_states, 'b q h gs d -> b q (h gs) d')
+ key_states = qkv_states[..., -2, :]
+ value_states = qkv_states[..., -1, :]
+
+ query_states = query_states.transpose(1, 2)
+ key_states = key_states.transpose(1, 2)
+ value_states = value_states.transpose(1, 2)
+
+ cos, sin = self.rotary_emb(value_states, position_ids)
+ query_states, key_states = apply_rotary_pos_emb(
+ query_states, key_states, cos, sin)
+
+ if past_key_value is not None:
+ # sin and cos are specific to RoPE models; cache_position needed for the static cache
+ cache_kwargs = {
+ 'sin': sin,
+ 'cos': cos,
+ 'cache_position': cache_position
+ }
+ key_states, value_states = past_key_value.update(
+ key_states, value_states, self.layer_idx, cache_kwargs)
+
+ key_states = repeat_kv(key_states, self.num_key_value_groups)
+ value_states = repeat_kv(value_states, self.num_key_value_groups)
+
+ causal_mask = attention_mask
+ if attention_mask is not None:
+ causal_mask = causal_mask[:, :, :, :key_states.shape[-2]]
+
+ # SDPA with memory-efficient backend is currently (torch==2.1.2) bugged with non-contiguous inputs with
+ # custom attn_mask, Reference: https://github.com/pytorch/pytorch/issues/112577.
+ if query_states.device.type == 'cuda' and causal_mask is not None:
+ query_states = query_states.contiguous()
+ key_states = key_states.contiguous()
+ value_states = value_states.contiguous()
+
+ # We dispatch to SDPA's Flash Attention or Efficient kernels via this `is_causal` if statement instead of
+ # an inline conditional assignment in SDPA to support both torch.compile's dynamic shapes and full graph
+ # options. An inline conditional prevents dynamic shapes from compiling.
+ is_causal = bool(causal_mask is None and q_len > 1)
+
+ attn_output = torch.nn.functional.scaled_dot_product_attention( # pylint: disable=E1102
+ query_states,
+ key_states,
+ value_states,
+ attn_mask=causal_mask,
+ dropout_p=0.0,
+ is_causal=is_causal,
+ )
+
+ attn_output = attn_output.transpose(1, 2).contiguous()
+ attn_output = attn_output.view(bsz, q_len, self.hidden_size)
+
+ attn_output = self.wo(attn_output)
+
+ return attn_output, None, past_key_value
+
+
+INTERNLM2_ATTENTION_CLASSES = {
+ 'eager': InternLM2Attention,
+ 'flash_attention_2': InternLM2FlashAttention2,
+ 'sdpa': InternLM2SdpaAttention,
+}
+
+
+# Modified from transformers.models.llama.modeling_llama.LlamaDecoderLayer with Llama->InternLM2
+class InternLM2DecoderLayer(nn.Module):
+ """InternLM2 Decoder Layer. This module is a single layer of the InternLM2 model."""
+
+ def __init__(self, config: InternLM2Config, layer_idx: int):
+ super().__init__()
+ self.hidden_size = config.hidden_size
+ self.layer_idx = layer_idx
+
+ self.attention = INTERNLM2_ATTENTION_CLASSES[
+ config.attn_implementation](
+ config=config, layer_idx=layer_idx)
+
+ self.feed_forward = InternLM2MLP(config)
+ self.attention_norm = InternLM2RMSNorm(
+ config.hidden_size, eps=config.rms_norm_eps)
+ self.ffn_norm = InternLM2RMSNorm(
+ config.hidden_size, eps=config.rms_norm_eps)
+
+ def forward(
+ self,
+ hidden_states: torch.Tensor,
+ attention_mask: Optional[torch.Tensor] = None,
+ position_ids: Optional[torch.LongTensor] = None,
+ past_key_value: Optional[Cache] = None,
+ output_attentions: Optional[bool] = False,
+ use_cache: Optional[bool] = False,
+ cache_position: Optional[torch.LongTensor] = None,
+ ) -> Tuple[torch.FloatTensor, Optional[Tuple[torch.FloatTensor,
+ torch.FloatTensor]]]:
+ """
+ Args:
+ hidden_states (`torch.FloatTensor`): input to the layer of shape `(batch, seq_len, embed_dim)`
+ attention_mask (`torch.FloatTensor`, *optional*):
+ attention mask of size `(batch_size, sequence_length)` if flash attention is used or `(batch_size, 1,
+ query_sequence_length, key_sequence_length)` if default attention is used.
+ output_attentions (`bool`, *optional*):
+ Whether or not to return the attentions tensors of all attention layers. See `attentions` under
+ returned tensors for more detail.
+ use_cache (`bool`, *optional*):
+ If set to `True`, `past_key_values` key value states are returned and can be used to speed up decoding
+ (see `past_key_values`).
+ past_key_value (`Tuple(torch.FloatTensor)`, *optional*): cached past key and value projection states
+ """
+ residual = hidden_states
+
+ hidden_states = self.attention_norm(hidden_states)
+
+ # Self Attention
+ hidden_states, self_attn_weights, present_key_value = self.attention(
+ hidden_states=hidden_states,
+ attention_mask=attention_mask,
+ position_ids=position_ids,
+ past_key_value=past_key_value,
+ output_attentions=output_attentions,
+ use_cache=use_cache,
+ cache_position=cache_position,
+ )
+ hidden_states = residual + hidden_states
+
+ # Fully Connected
+ residual = hidden_states
+ hidden_states = self.ffn_norm(hidden_states)
+ hidden_states = self.feed_forward(hidden_states)
+ hidden_states = residual + hidden_states
+
+ outputs = (hidden_states, )
+
+ if output_attentions:
+ outputs += (self_attn_weights, )
+
+ if use_cache:
+ outputs += (present_key_value, )
+
+ return outputs
+
+
+InternLM2_START_DOCSTRING = r"""
+ This model inherits from [`PreTrainedModel`]. Check the superclass documentation for the generic methods the
+ library implements for all its model (such as downloading or saving, resizing the input embeddings, pruning heads
+ etc.)
+ This model is also a PyTorch [torch.nn.Module](https://pytorch.org/docs/stable/nn.html#torch.nn.Module) subclass.
+ Use it as a regular PyTorch Module and refer to the PyTorch documentation for all matter related to general usage
+ and behavior.
+ Parameters:
+ config ([`InternLM2Config`]):
+ Model configuration class with all the parameters of the model. Initializing with a config file does not
+ load the weights associated with the model, only the configuration. Check out the
+ [`~PreTrainedModel.from_pretrained`] method to load the model weights.
+"""
+
+
+# Copied from transformers.models.llama.modeling_llama.LlamaPreTrainedModel with Llama->InternLM2
+@add_start_docstrings(
+ 'The bare InternLM2 Model outputting raw hidden-states without any specific head on top.',
+ InternLM2_START_DOCSTRING,
+)
+class InternLM2PreTrainedModel(PreTrainedModel):
+ """
+ InternLM2 pretraiend model's base class.
+ """
+
+ config_class = InternLM2Config
+ base_model_prefix = 'model'
+ supports_gradient_checkpointing = True
+ _no_split_modules = ['InternLM2DecoderLayer']
+ _skip_keys_device_placement = ['past_key_values']
+ _supports_flash_attn_2 = True
+ _supports_sdpa = True
+ _supports_cache_class = True
+ _supports_quantized_cache = True
+ _supports_static_cache = True
+
+ def _init_weights(self, module):
+ std = self.config.initializer_range
+ if isinstance(module, nn.Linear):
+ module.weight.data.normal_(mean=0.0, std=std)
+ if module.bias is not None:
+ module.bias.data.zero_()
+ elif isinstance(module, nn.Embedding):
+ module.weight.data.normal_(mean=0.0, std=std)
+ if module.padding_idx is not None:
+ module.weight.data[module.padding_idx].zero_()
+
+
+InternLM2_INPUTS_DOCSTRING = r"""
+ Args:
+ input_ids (`torch.LongTensor` of shape `(batch_size, sequence_length)`):
+ Indices of input sequence tokens in the vocabulary. Padding will be ignored by default should you provide
+ it.
+ Indices can be obtained using [`AutoTokenizer`]. See [`PreTrainedTokenizer.encode`] and
+ [`PreTrainedTokenizer.__call__`] for details.
+ [What are input IDs?](../glossary#input-ids)
+ attention_mask (`torch.Tensor` of shape `(batch_size, sequence_length)`, *optional*):
+ Mask to avoid performing attention on padding token indices. Mask values selected in `[0, 1]`:
+ - 1 for tokens that are **not masked**,
+ - 0 for tokens that are **masked**.
+ [What are attention masks?](../glossary#attention-mask)
+ Indices can be obtained using [`AutoTokenizer`]. See [`PreTrainedTokenizer.encode`] and
+ [`PreTrainedTokenizer.__call__`] for details.
+ If `past_key_values` is used, optionally only the last `input_ids` have to be input (see
+ `past_key_values`).
+ If you want to change padding behavior, you should read [`modeling_opt._prepare_decoder_attention_mask`]
+ and modify to your needs. See diagram 1 in [the paper](https://arxiv.org/abs/1910.13461) for more
+ information on the default strategy.
+ - 1 indicates the head is **not masked**,
+ - 0 indicates the head is **masked**.
+ position_ids (`torch.LongTensor` of shape `(batch_size, sequence_length)`, *optional*):
+ Indices of positions of each input sequence tokens in the position embeddings. Selected in the range `[0,
+ config.n_positions - 1]`.
+ [What are position IDs?](../glossary#position-ids)
+ past_key_values (`Cache` or `tuple(tuple(torch.FloatTensor))`, *optional*):
+ Pre-computed hidden-states (key and values in the self-attention blocks and in the cross-attention
+ blocks) that can be used to speed up sequential decoding. This typically consists in the `past_key_values`
+ returned by the model at a previous stage of decoding, when `use_cache=True` or `config.use_cache=True`.
+ Two formats are allowed:
+ - a [`~cache_utils.Cache`] instance;
+ - Tuple of `tuple(torch.FloatTensor)` of length `config.n_layers`, with each tuple having 2 tensors of
+ shape `(batch_size, num_heads, sequence_length, embed_size_per_head)`). This is also known as the legacy
+ cache format.
+ The model will output the same cache format that is fed as input. If no `past_key_values` are passed, the
+ legacy cache format will be returned.
+ If `past_key_values` are used, the user can optionally input only the last `input_ids` (those that don't
+ have their past key value states given to this model) of shape `(batch_size, 1)` instead of all `input_ids`
+ of shape `(batch_size, sequence_length)`.
+ inputs_embeds (`torch.FloatTensor` of shape `(batch_size, sequence_length, hidden_size)`, *optional*):
+ Optionally, instead of passing `input_ids` you can choose to directly pass an embedded representation. This
+ is useful if you want more control over how to convert `input_ids` indices into associated vectors than the
+ model's internal embedding lookup matrix.
+ use_cache (`bool`, *optional*):
+ If set to `True`, `past_key_values` key value states are returned and can be used to speed up decoding (see
+ `past_key_values`).
+ output_attentions (`bool`, *optional*):
+ Whether or not to return the attentions tensors of all attention layers. See `attentions` under returned
+ tensors for more detail.
+ output_hidden_states (`bool`, *optional*):
+ Whether or not to return the hidden states of all layers. See `hidden_states` under returned tensors for
+ more detail.
+ return_dict (`bool`, *optional*):
+ Whether or not to return a [`~utils.ModelOutput`] instead of a plain tuple.
+ cache_position (`torch.LongTensor` of shape `(sequence_length)`, *optional*):
+ Indices depicting the position of the input sequence tokens in the sequence. Contrarily to `position_ids`,
+ this tensor is not affected by padding. It is used to update the cache in the correct position and to infer
+ the complete sequence length.
+"""
+
+
+# Modified from transformers.models.llama.modeling_llama.LlamaModel with Llama->InternLM2
+@add_start_docstrings(
+ 'The bare InternLM2 Model outputting raw hidden-states without any specific head on top.',
+ InternLM2_START_DOCSTRING,
+)
+class InternLM2Model(InternLM2PreTrainedModel):
+ """
+ Transformer decoder consisting of *config.num_hidden_layers* layers. Each layer is a [`InternLM2DecoderLayer`]
+ Args:
+ config: InternLM2Config
+ """
+
+ _auto_class = 'AutoModel'
+
+ def __init__(self, config: InternLM2Config):
+ super().__init__(config)
+ self.padding_idx = config.pad_token_id
+ self.vocab_size = config.vocab_size
+ self.config = config
+
+ self.tok_embeddings = nn.Embedding(config.vocab_size,
+ config.hidden_size,
+ self.padding_idx)
+
+ self.layers = nn.ModuleList([
+ InternLM2DecoderLayer(config, layer_idx)
+ for layer_idx in range(config.num_hidden_layers)
+ ])
+ self.norm = InternLM2RMSNorm(
+ config.hidden_size, eps=config.rms_norm_eps)
+
+ self.gradient_checkpointing = False
+ # Initialize weights and apply final processing
+ self.post_init()
+
+ def get_input_embeddings(self):
+ return self.tok_embeddings
+
+ def set_input_embeddings(self, value):
+ self.tok_embeddings = value
+
+ @add_start_docstrings_to_model_forward(InternLM2_INPUTS_DOCSTRING)
+ def forward(
+ self,
+ input_ids: torch.LongTensor = None,
+ attention_mask: Optional[torch.Tensor] = None,
+ position_ids: Optional[torch.LongTensor] = None,
+ past_key_values: Optional[Union[Cache,
+ List[torch.FloatTensor]]] = None,
+ inputs_embeds: Optional[torch.FloatTensor] = None,
+ use_cache: Optional[bool] = None,
+ output_attentions: Optional[bool] = None,
+ output_hidden_states: Optional[bool] = None,
+ return_dict: Optional[bool] = None,
+ cache_position: Optional[torch.LongTensor] = None,
+ ) -> Union[Tuple, BaseModelOutputWithPast]:
+ output_attentions = output_attentions if output_attentions is not None else self.config.output_attentions
+ output_hidden_states = (
+ output_hidden_states if output_hidden_states is not None else
+ self.config.output_hidden_states)
+ use_cache = use_cache if use_cache is not None else self.config.use_cache
+ return_dict = return_dict if return_dict is not None else self.config.use_return_dict
+
+ if (input_ids is None) ^ (inputs_embeds is not None):
+ raise ValueError(
+ 'You cannot specify both input_ids and inputs_embeds at the same time, and must specify either one'
+ )
+
+ if self.gradient_checkpointing and self.training and use_cache:
+ logger.warning_once(
+ '`use_cache=True` is incompatible with gradient checkpointing. Setting `use_cache=False`.'
+ )
+ use_cache = False
+
+ if inputs_embeds is None:
+ inputs_embeds = self.tok_embeddings(input_ids)
+
+ return_legacy_cache = False
+ if use_cache and not isinstance(
+ past_key_values,
+ Cache): # kept for BC (non `Cache` `past_key_values` inputs)
+ return_legacy_cache = True
+ past_key_values = DynamicCache.from_legacy_cache(past_key_values)
+
+ if cache_position is None:
+ past_seen_tokens = past_key_values.get_seq_length(
+ ) if past_key_values is not None else 0
+ cache_position = torch.arange(
+ past_seen_tokens,
+ past_seen_tokens + inputs_embeds.shape[1],
+ device=inputs_embeds.device)
+ if position_ids is None:
+ position_ids = cache_position.unsqueeze(0)
+
+ causal_mask = self._update_causal_mask(attention_mask, inputs_embeds,
+ cache_position, past_key_values,
+ output_attentions)
+
+ # embed positions
+ hidden_states = inputs_embeds
+
+ # decoder layers
+ all_hidden_states = () if output_hidden_states else None
+ all_self_attns = () if output_attentions else None
+ next_decoder_cache = None
+
+ for decoder_layer in self.layers:
+ if output_hidden_states:
+ all_hidden_states += (hidden_states, )
+
+ if self.gradient_checkpointing and self.training:
+ layer_outputs = self._gradient_checkpointing_func(
+ decoder_layer.__call__,
+ hidden_states,
+ causal_mask,
+ position_ids,
+ past_key_values,
+ output_attentions,
+ use_cache,
+ cache_position,
+ )
+ else:
+ layer_outputs = decoder_layer(
+ hidden_states,
+ attention_mask=causal_mask,
+ position_ids=position_ids,
+ past_key_value=past_key_values,
+ output_attentions=output_attentions,
+ use_cache=use_cache,
+ cache_position=cache_position,
+ )
+
+ hidden_states = layer_outputs[0]
+
+ if use_cache:
+ next_decoder_cache = layer_outputs[
+ 2 if output_attentions else 1]
+
+ if output_attentions:
+ all_self_attns += (layer_outputs[1], )
+
+ hidden_states = self.norm(hidden_states)
+
+ # add hidden states from the last decoder layer
+ if output_hidden_states:
+ all_hidden_states += (hidden_states, )
+
+ next_cache = next_decoder_cache if use_cache else None
+ if return_legacy_cache:
+ next_cache = next_cache.to_legacy_cache()
+
+ if not return_dict:
+ return tuple(
+ v for v in
+ [hidden_states, next_cache, all_hidden_states, all_self_attns]
+ if v is not None)
+ return BaseModelOutputWithPast(
+ last_hidden_state=hidden_states,
+ past_key_values=next_cache,
+ hidden_states=all_hidden_states,
+ attentions=all_self_attns,
+ )
+
+ def _update_causal_mask(
+ self,
+ attention_mask: torch.Tensor,
+ input_tensor: torch.Tensor,
+ cache_position: torch.Tensor,
+ past_key_values: Cache,
+ output_attentions: bool,
+ ):
+ # TODO: As of torch==2.2.0, the `attention_mask` passed to the model in `generate` is 2D and of dynamic length
+ # even when the static KV cache is used. This is an issue for torch.compile which then recaptures cudagraphs at
+ # each decode steps due to the dynamic shapes. (`recording cudagraph tree for symint key 13`, etc.), which is
+ # VERY slow. A workaround is `@torch.compiler.disable`, but this prevents using `fullgraph=True`.
+ # See more context in https://github.com/huggingface/transformers/pull/29114
+
+ if self.config.attn_implementation == 'flash_attention_2':
+ if attention_mask is not None and 0.0 in attention_mask:
+ return attention_mask
+ return None
+
+ # For SDPA, when possible, we will rely on its `is_causal` argument instead of its `attn_mask` argument, in
+ # order to dispatch on Flash Attention 2. This feature is not compatible with static cache, as SDPA will fail
+ # to infer the attention mask.
+ past_seen_tokens = past_key_values.get_seq_length(
+ ) if past_key_values is not None else 0
+ using_static_cache = isinstance(past_key_values, StaticCache)
+
+ # When output attentions is True, sdpa implementation's forward method calls the eager implementation's forward
+ if self.config.attn_implementation == 'sdpa' and not using_static_cache and not output_attentions:
+ if AttentionMaskConverter._ignore_causal_mask_sdpa(
+ attention_mask,
+ inputs_embeds=input_tensor,
+ past_key_values_length=past_seen_tokens,
+ is_training=self.training,
+ ):
+ return None
+
+ dtype, device = input_tensor.dtype, input_tensor.device
+ min_dtype = torch.finfo(dtype).min
+ sequence_length = input_tensor.shape[1]
+ if using_static_cache:
+ target_length = past_key_values.get_max_length()
+ else:
+ target_length = (
+ attention_mask.shape[-1] if isinstance(
+ attention_mask, torch.Tensor) else past_seen_tokens +
+ sequence_length + 1)
+
+ if attention_mask is not None and attention_mask.dim() == 4:
+ # in this case we assume that the mask comes already in inverted form and requires no inversion or slicing
+ if attention_mask.max() != 0:
+ raise ValueError(
+ 'Custom 4D attention mask should be passed in inverted form with max==0`'
+ )
+ causal_mask = attention_mask
+ else:
+ causal_mask = torch.full((sequence_length, target_length),
+ fill_value=min_dtype,
+ dtype=dtype,
+ device=device)
+ if sequence_length != 1:
+ causal_mask = torch.triu(causal_mask, diagonal=1)
+ causal_mask *= torch.arange(
+ target_length, device=device) > cache_position.reshape(-1, 1)
+ causal_mask = causal_mask[None, None, :, :].expand(
+ input_tensor.shape[0], 1, -1, -1)
+ if attention_mask is not None:
+ causal_mask = causal_mask.clone(
+ ) # copy to contiguous memory for in-place edit
+ mask_length = attention_mask.shape[-1]
+ padding_mask = causal_mask[:, :, :, :
+ mask_length] + attention_mask[:,
+ None,
+ None, :]
+ padding_mask = padding_mask == 0
+ causal_mask[:, :, :, :
+ mask_length] = causal_mask[:, :, :, :
+ mask_length].masked_fill(
+ padding_mask,
+ min_dtype)
+ if (self.config.attn_implementation == 'sdpa'
+ and attention_mask is not None
+ and attention_mask.device.type == 'cuda'
+ and not output_attentions):
+ # Attend to all tokens in fully masked rows in the causal_mask, for example the relevant first rows when
+ # using left padding. This is required by F.scaled_dot_product_attention memory-efficient attention path.
+ # Details: https://github.com/pytorch/pytorch/issues/110213
+ causal_mask = AttentionMaskConverter._unmask_unattended(
+ causal_mask, min_dtype) # pylint: disable=E1120
+
+ return causal_mask
+
+
+# Modified from transformers.models.llama.modeling_llama.LlamaForCausalLM
+class InternLM2ForCausalLM(InternLM2PreTrainedModel):
+ """Causal language model (CLM) for InternLM2."""
+
+ _auto_class = 'AutoModelForCausalLM'
+ _tied_weights_keys = ['output.weight']
+
+ def __init__(self, config):
+ super().__init__(config)
+ self.model = InternLM2Model(config)
+ self.vocab_size = config.vocab_size
+ self.output = nn.Linear(
+ config.hidden_size, config.vocab_size, bias=False)
+
+ # Initialize weights and apply final processing
+ self.post_init()
+
+ def get_input_embeddings(self):
+ return self.model.tok_embeddings
+
+ def set_input_embeddings(self, value):
+ self.model.tok_embeddings = value
+
+ def get_output_embeddings(self):
+ return self.output
+
+ def set_output_embeddings(self, new_embeddings):
+ self.output = new_embeddings
+
+ def set_decoder(self, decoder):
+ self.model = decoder
+
+ def get_decoder(self):
+ return self.model
+
+ @add_start_docstrings_to_model_forward(InternLM2_INPUTS_DOCSTRING)
+ @replace_return_docstrings(
+ output_type=CausalLMOutputWithPast, config_class=_CONFIG_FOR_DOC)
+ def forward(
+ self,
+ input_ids: torch.LongTensor = None,
+ attention_mask: Optional[torch.Tensor] = None,
+ position_ids: Optional[torch.LongTensor] = None,
+ past_key_values: Optional[Union[Cache,
+ List[torch.FloatTensor]]] = None,
+ inputs_embeds: Optional[torch.FloatTensor] = None,
+ labels: Optional[torch.LongTensor] = None,
+ use_cache: Optional[bool] = None,
+ output_attentions: Optional[bool] = None,
+ output_hidden_states: Optional[bool] = None,
+ return_dict: Optional[bool] = None,
+ cache_position: Optional[torch.LongTensor] = None,
+ ) -> Union[Tuple, CausalLMOutputWithPast]:
+ r"""
+ Args:
+ labels (`torch.LongTensor` of shape `(batch_size, sequence_length)`, *optional*):
+ Labels for computing the masked language modeling loss. Indices should either be in `[0, ...,
+ config.vocab_size]` or -100 (see `input_ids` docstring). Tokens with indices set to `-100` are ignored
+ (masked), the loss is only computed for the tokens with labels in `[0, ..., config.vocab_size]`.
+ Returns:
+ Example:
+ ```python
+ >>> from transformers import AutoTokenizer, InternLM2ForCausalLM
+ >>> model = InternLM2ForCausalLM.from_pretrained("meta-InternLM2/InternLM2-2-7b-hf")
+ >>> tokenizer = AutoTokenizer.from_pretrained("meta-InternLM2/InternLM2-2-7b-hf")
+ >>> prompt = "Hey, are you conscious? Can you talk to me?"
+ >>> inputs = tokenizer(prompt, return_tensors="pt")
+ >>> # Generate
+ >>> generate_ids = model.generate(inputs.input_ids, max_length=30)
+ >>> tokenizer.batch_decode(generate_ids, skip_special_tokens=True, clean_up_tokenization_spaces=False)[0]
+ "Hey, are you conscious? Can you talk to me?\nI'm not conscious, but I can talk to you."
+ ```"""
+
+ output_attentions = output_attentions if output_attentions is not None else self.config.output_attentions
+ output_hidden_states = (
+ output_hidden_states if output_hidden_states is not None else
+ self.config.output_hidden_states)
+ return_dict = return_dict if return_dict is not None else self.config.use_return_dict
+
+ # decoder outputs consists of (dec_features, layer_state, dec_hidden, dec_attn)
+ outputs = self.model(
+ input_ids=input_ids,
+ attention_mask=attention_mask,
+ position_ids=position_ids,
+ past_key_values=past_key_values,
+ inputs_embeds=inputs_embeds,
+ use_cache=use_cache,
+ output_attentions=output_attentions,
+ output_hidden_states=output_hidden_states,
+ return_dict=return_dict,
+ cache_position=cache_position,
+ )
+
+ hidden_states = outputs[0]
+ if self.config.pretraining_tp > 1:
+ output_slices = self.output.weight.split(
+ self.vocab_size // self.config.pretraining_tp, dim=0)
+ logits = [
+ F.linear(hidden_states, output_slices[i]) # pylint: disable=not-callable
+ for i in range(self.config.pretraining_tp)
+ ]
+ logits = torch.cat(logits, dim=-1)
+ else:
+ logits = self.output(hidden_states)
+ logits = logits.float()
+
+ loss = None
+ if labels is not None:
+ # Shift so that tokens < n predict n
+ shift_logits = logits[..., :-1, :].contiguous()
+ shift_labels = labels[..., 1:].contiguous()
+ # Flatten the tokens
+ loss_fct = CrossEntropyLoss()
+ shift_logits = shift_logits.view(-1, self.config.vocab_size)
+ shift_labels = shift_labels.view(-1)
+ # Enable model parallelism
+ shift_labels = shift_labels.to(shift_logits.device)
+ loss = loss_fct(shift_logits, shift_labels)
+
+ if not return_dict:
+ output = (logits, ) + outputs[1:]
+ return (loss, ) + output if loss is not None else output
+
+ return CausalLMOutputWithPast(
+ loss=loss,
+ logits=logits,
+ past_key_values=outputs.past_key_values,
+ hidden_states=outputs.hidden_states,
+ attentions=outputs.attentions,
+ )
+
+ def prepare_inputs_for_generation(
+ self,
+ input_ids,
+ past_key_values=None,
+ attention_mask=None,
+ inputs_embeds=None,
+ cache_position=None,
+ use_cache=True,
+ **kwargs,
+ ):
+ past_length = 0
+ if past_key_values is not None:
+ if isinstance(past_key_values, Cache):
+ past_length = cache_position[
+ 0] if cache_position is not None else past_key_values.get_seq_length(
+ )
+ max_cache_length = (
+ torch.tensor(
+ past_key_values.get_max_length(),
+ device=input_ids.device)
+ if past_key_values.get_max_length() is not None else None)
+ cache_length = past_length if max_cache_length is None else torch.min(
+ max_cache_length, past_length)
+ # TODO joao: remove this `else` after `generate` prioritizes `Cache` objects
+ else:
+ cache_length = past_length = past_key_values[0][0].shape[2]
+ max_cache_length = None
+
+ # Keep only the unprocessed tokens:
+ # 1 - If the length of the attention_mask exceeds the length of input_ids, then we are in a setting where
+ # some of the inputs are exclusively passed as part of the cache (e.g. when passing input_embeds as input)
+ if attention_mask is not None and attention_mask.shape[
+ 1] > input_ids.shape[1]:
+ input_ids = input_ids[:, -(attention_mask.shape[1] -
+ past_length):]
+ # 2 - If the past_length is smaller than input_ids', then input_ids holds all input tokens. We can discard
+ # input_ids based on the past_length.
+ elif past_length < input_ids.shape[1]:
+ input_ids = input_ids[:, past_length:]
+ # 3 - Otherwise (past_length >= input_ids.shape[1]), let's assume input_ids only has unprocessed tokens.
+
+ # If we are about to go beyond the maximum cache length, we need to crop the input attention mask.
+ if (max_cache_length is not None and attention_mask is not None
+ and cache_length + input_ids.shape[1] > max_cache_length):
+ attention_mask = attention_mask[:, -max_cache_length:] # pylint: disable=E1130
+
+ position_ids = kwargs.get('position_ids', None)
+ if attention_mask is not None and position_ids is None:
+ # create position_ids on the fly for batch generation
+ position_ids = attention_mask.long().cumsum(-1) - 1
+ position_ids.masked_fill_(attention_mask == 0, 1)
+ if past_key_values:
+ position_ids = position_ids[:, -input_ids.shape[1]:]
+
+ # if `inputs_embeds` are passed, we only want to use them in the 1st generation step
+ if inputs_embeds is not None and past_key_values is None:
+ model_inputs = {'inputs_embeds': inputs_embeds}
+ else:
+ # The `contiguous()` here is necessary to have a static stride during decoding. torchdynamo otherwise
+ # recompiles graphs as the stride of the inputs is a guard.
+ # Ref: https://github.com/huggingface/transformers/pull/29114
+ # TODO: use `next_tokens` directly instead.
+ model_inputs = {'input_ids': input_ids.contiguous()}
+
+ input_length = position_ids.shape[
+ -1] if position_ids is not None else input_ids.shape[-1]
+ if cache_position is None:
+ cache_position = torch.arange(
+ past_length,
+ past_length + input_length,
+ device=input_ids.device)
+ elif use_cache:
+ cache_position = cache_position[-input_length:]
+
+ model_inputs.update({
+ 'position_ids': position_ids,
+ 'cache_position': cache_position,
+ 'past_key_values': past_key_values,
+ 'use_cache': use_cache,
+ 'attention_mask': attention_mask,
+ })
+ return model_inputs
+
+ @staticmethod
+ def _reorder_cache(past_key_values, beam_idx):
+ reordered_past = ()
+ for layer_past in past_key_values:
+ reordered_past += (tuple(
+ past_state.index_select(0, beam_idx.to(past_state.device))
+ for past_state in layer_past), )
+ return reordered_past
+
+ def build_inputs(self,
+ tokenizer,
+ query: str,
+ history: List[Tuple[str, str]] = None,
+ meta_instruction=''):
+ if history is None:
+ history = []
+ if tokenizer.add_bos_token:
+ prompt = ''
+ else:
+ prompt = tokenizer.bos_token
+ if meta_instruction:
+ prompt += f"""<|im_start|>system\n{meta_instruction}<|im_end|>\n"""
+ for record in history:
+ prompt += f"""<|im_start|>user\n{record[0]}<|im_end|>\n<|im_start|>assistant\n{record[1]}<|im_end|>\n"""
+ prompt += f"""<|im_start|>user\n{query}<|im_end|>\n<|im_start|>assistant\n"""
+ return tokenizer([prompt], return_tensors='pt')
+
+ @torch.no_grad()
+ def chat(
+ self,
+ tokenizer,
+ query: str,
+ history: Optional[List[Tuple[str, str]]] = None,
+ streamer: Optional[BaseStreamer] = None,
+ max_new_tokens: int = 1024,
+ do_sample: bool = True,
+ temperature: float = 0.8,
+ top_p: float = 0.8,
+ meta_instruction:
+ str = 'You are an AI assistant whose name is InternLM (书生·浦语).\n'
+ '- InternLM (书生·浦语) is a conversational language model that is developed by Shanghai AI Laboratory '
+ '(上海人工智能实验室). It is designed to be helpful, honest, and harmless.\n'
+ '- InternLM (书生·浦语) can understand and communicate fluently in the language chosen by the user such '
+ 'as English and 中文.',
+ **kwargs,
+ ):
+ if history is None:
+ history = []
+ inputs = self.build_inputs(tokenizer, query, history, meta_instruction)
+ inputs = {
+ k: v.to(self.device)
+ for k, v in inputs.items() if torch.is_tensor(v)
+ }
+ # also add end-of-assistant token in eos token id to avoid unnecessary generation
+ eos_token_id = [
+ tokenizer.eos_token_id,
+ tokenizer.convert_tokens_to_ids(['<|im_end|>'])[0]
+ ]
+ outputs = self.generate(
+ **inputs,
+ streamer=streamer,
+ max_new_tokens=max_new_tokens,
+ do_sample=do_sample,
+ temperature=temperature,
+ top_p=top_p,
+ eos_token_id=eos_token_id,
+ **kwargs,
+ )
+ outputs = outputs[0].cpu().tolist()[len(inputs['input_ids'][0]):]
+ response = tokenizer.decode(outputs, skip_special_tokens=True)
+ response = response.split('<|im_end|>')[0]
+ history = history + [(query, response)]
+ return response, history
+
+ @torch.no_grad()
+ def stream_chat(
+ self,
+ tokenizer,
+ query: str,
+ history: List[Tuple[str, str]] = None,
+ max_new_tokens: int = 1024,
+ do_sample: bool = True,
+ temperature: float = 0.8,
+ top_p: float = 0.8,
+ **kwargs,
+ ):
+ if history is None:
+ history = []
+ """
+ Return a generator in format: (response, history)
+ Eg.
+ ('你好,有什么可以帮助您的吗', [('你好', '你好,有什么可以帮助您的吗')])
+ ('你好,有什么可以帮助您的吗?', [('你好', '你好,有什么可以帮助您的吗?')])
+ """
+ if BaseStreamer is None:
+ raise ModuleNotFoundError(
+ 'The version of `transformers` is too low. Please make sure '
+ 'that you have installed `transformers>=4.28.0`.')
+
+ response_queue = queue.Queue(maxsize=20)
+
+ class ChatStreamer(BaseStreamer):
+ """
+ Streamer used in generate to print words one by one.
+ """
+
+ def __init__(self, tokenizer) -> None:
+ super().__init__()
+ self.tokenizer = tokenizer
+ self.queue = response_queue
+ self.query = query
+ self.history = history
+ self.response = ''
+ self.cache = []
+ self.received_inputs = False
+ self.queue.put(
+ (self.response, history + [(self.query, self.response)]))
+
+ def put(self, value):
+ if len(value.shape) > 1 and value.shape[0] > 1:
+ raise ValueError('ChatStreamer only supports batch size 1')
+ elif len(value.shape) > 1:
+ value = value[0]
+
+ if not self.received_inputs:
+ # The first received value is input_ids, ignore here
+ self.received_inputs = True
+ return
+
+ self.cache.extend(value.tolist())
+ token = self.tokenizer.decode(
+ self.cache, skip_special_tokens=True)
+ if token.strip() != '<|im_end|>':
+ self.response = self.response + token
+ history = self.history + [(self.query, self.response)]
+ self.queue.put((self.response, history))
+ self.cache = []
+ else:
+ self.end()
+
+ def end(self):
+ self.queue.put(None)
+
+ def stream_producer():
+ return self.chat(
+ tokenizer=tokenizer,
+ query=query,
+ streamer=ChatStreamer(tokenizer=tokenizer),
+ history=history,
+ max_new_tokens=max_new_tokens,
+ do_sample=do_sample,
+ temperature=temperature,
+ top_p=top_p,
+ **kwargs,
+ )
+
+ def consumer():
+ producer = threading.Thread(target=stream_producer)
+ producer.start()
+ while True:
+ res = response_queue.get()
+ if res is None:
+ return
+ yield res
+
+ return consumer()
+
+
+# Copied from transformers.models.llama.modeling_llama.LlamaForSequenceClassification with Llama->InternLM2
+@add_start_docstrings(
+ """
+ The InternLM2 Model transformer with a sequence classification head on top (linear layer).
+ [`InternLM2ForSequenceClassification`] uses the last token in order to do the classification, as other causal models
+ (e.g. GPT-2) do.
+ Since it does classification on the last token, it requires to know the position of the last token. If a
+ `pad_token_id` is defined in the configuration, it finds the last token that is not a padding token in each row. If
+ no `pad_token_id` is defined, it simply takes the last value in each row of the batch. Since it cannot guess the
+ padding tokens when `inputs_embeds` are passed instead of `input_ids`, it does the same (take the last value in
+ each row of the batch).
+ """,
+ InternLM2_START_DOCSTRING,
+)
+class InternLM2ForSequenceClassification(InternLM2PreTrainedModel):
+ """Sequence Classification Head for InternLM2 Model."""
+
+ def __init__(self, config):
+ super().__init__(config)
+ self.num_labels = config.num_labels
+ self.model = InternLM2Model(config)
+ self.score = nn.Linear(config.hidden_size, self.num_labels, bias=False)
+
+ # Initialize weights and apply final processing
+ self.post_init()
+
+ def get_input_embeddings(self):
+ return self.model.tok_embeddings
+
+ def set_input_embeddings(self, value):
+ self.model.tok_embeddings = value
+
+ @add_start_docstrings_to_model_forward(InternLM2_INPUTS_DOCSTRING)
+ def forward(
+ self,
+ input_ids: torch.LongTensor = None,
+ attention_mask: Optional[torch.Tensor] = None,
+ position_ids: Optional[torch.LongTensor] = None,
+ past_key_values: Optional[Union[Cache,
+ List[torch.FloatTensor]]] = None,
+ inputs_embeds: Optional[torch.FloatTensor] = None,
+ labels: Optional[torch.LongTensor] = None,
+ use_cache: Optional[bool] = None,
+ output_attentions: Optional[bool] = None,
+ output_hidden_states: Optional[bool] = None,
+ return_dict: Optional[bool] = None,
+ ) -> Union[Tuple, SequenceClassifierOutputWithPast]:
+ r"""
+ labels (`torch.LongTensor` of shape `(batch_size,)`, *optional*):
+ Labels for computing the sequence classification/regression loss. Indices should be in `[0, ...,
+ config.num_labels - 1]`. If `config.num_labels == 1` a regression loss is computed (Mean-Square loss), If
+ `config.num_labels > 1` a classification loss is computed (Cross-Entropy).
+ """
+ return_dict = return_dict if return_dict is not None else self.config.use_return_dict
+
+ transformer_outputs = self.model(
+ input_ids,
+ attention_mask=attention_mask,
+ position_ids=position_ids,
+ past_key_values=past_key_values,
+ inputs_embeds=inputs_embeds,
+ use_cache=use_cache,
+ output_attentions=output_attentions,
+ output_hidden_states=output_hidden_states,
+ return_dict=return_dict,
+ )
+ hidden_states = transformer_outputs[0]
+ logits = self.score(hidden_states)
+
+ if input_ids is not None:
+ batch_size = input_ids.shape[0]
+ else:
+ batch_size = inputs_embeds.shape[0]
+
+ if self.config.pad_token_id is None and batch_size != 1:
+ raise ValueError(
+ 'Cannot handle batch sizes > 1 if no padding token is defined.'
+ )
+ if self.config.pad_token_id is None:
+ sequence_lengths = -1
+ else:
+ if input_ids is not None:
+ # if no pad token found, use modulo instead of reverse indexing for ONNX compatibility
+ sequence_lengths = torch.eq(
+ input_ids, self.config.pad_token_id).int().argmax(-1) - 1
+ sequence_lengths = sequence_lengths % input_ids.shape[-1]
+ sequence_lengths = sequence_lengths.to(logits.device)
+ else:
+ sequence_lengths = -1
+
+ pooled_logits = logits[torch.arange(batch_size, device=logits.device),
+ sequence_lengths]
+
+ loss = None
+ if labels is not None:
+ labels = labels.to(logits.device)
+ if self.config.problem_type is None:
+ if self.num_labels == 1:
+ self.config.problem_type = 'regression'
+ elif self.num_labels > 1 and (labels.dtype
+ in (torch.long, torch.int)):
+ self.config.problem_type = 'single_label_classification'
+ else:
+ self.config.problem_type = 'multi_label_classification'
+
+ if self.config.problem_type == 'regression':
+ loss_fct = MSELoss()
+ if self.num_labels == 1:
+ loss = loss_fct(pooled_logits.squeeze(), labels.squeeze())
+ else:
+ loss = loss_fct(pooled_logits, labels)
+ elif self.config.problem_type == 'single_label_classification':
+ loss_fct = CrossEntropyLoss()
+ loss = loss_fct(
+ pooled_logits.view(-1, self.num_labels), labels.view(-1))
+ elif self.config.problem_type == 'multi_label_classification':
+ loss_fct = BCEWithLogitsLoss()
+ loss = loss_fct(pooled_logits, labels)
+ if not return_dict:
+ output = (pooled_logits, ) + transformer_outputs[1:]
+ return ((loss, ) + output) if loss is not None else output
+
+ return SequenceClassifierOutputWithPast(
+ loss=loss,
+ logits=pooled_logits,
+ past_key_values=transformer_outputs.past_key_values,
+ hidden_states=transformer_outputs.hidden_states,
+ attentions=transformer_outputs.attentions,
+ )
+
+
+# Copied from transformers.models.llama.modeling_llama.LlamaForQuestionAnswering with Llama->InternLM2
+@add_start_docstrings(
+ """
+The InternLM2 Model transformer with a span classification head on top for extractive question-answering tasks like
+SQuAD (a linear layer on top of the hidden-states output to compute `span start logits` and `span end logits`).
+ """,
+ InternLM2_START_DOCSTRING,
+)
+class InternLM2ForQuestionAnswering(InternLM2PreTrainedModel):
+ """Question Answering model for InternLM2."""
+
+ base_model_prefix = 'transformer'
+
+ def __init__(self, config):
+ super().__init__(config)
+ self.transformer = InternLM2Model(config)
+ self.qa_outputs = nn.Linear(config.hidden_size, 2)
+
+ # Initialize weights and apply final processing
+ self.post_init()
+
+ def get_input_embeddings(self):
+ return self.transformer.tok_embeddings
+
+ def set_input_embeddings(self, value):
+ self.transformer.tok_embeddings = value
+
+ @add_start_docstrings_to_model_forward(InternLM2_INPUTS_DOCSTRING)
+ def forward(
+ self,
+ input_ids: Optional[torch.LongTensor] = None,
+ attention_mask: Optional[torch.FloatTensor] = None,
+ position_ids: Optional[torch.LongTensor] = None,
+ past_key_values: Optional[Union[Cache,
+ List[torch.FloatTensor]]] = None,
+ inputs_embeds: Optional[torch.FloatTensor] = None,
+ start_positions: Optional[torch.LongTensor] = None,
+ end_positions: Optional[torch.LongTensor] = None,
+ output_attentions: Optional[bool] = None,
+ output_hidden_states: Optional[bool] = None,
+ return_dict: Optional[bool] = None,
+ ) -> Union[Tuple, QuestionAnsweringModelOutput]:
+ r"""
+ start_positions (`torch.LongTensor` of shape `(batch_size,)`, *optional*):
+ Labels for position (index) of the start of the labelled span for computing the token classification loss.
+ Positions are clamped to the length of the sequence (`sequence_length`). Position outside of the sequence
+ are not taken into account for computing the loss.
+ end_positions (`torch.LongTensor` of shape `(batch_size,)`, *optional*):
+ Labels for position (index) of the end of the labelled span for computing the token classification loss.
+ Positions are clamped to the length of the sequence (`sequence_length`). Position outside of the sequence
+ are not taken into account for computing the loss.
+ """
+ return_dict = return_dict if return_dict is not None else self.config.use_return_dict
+
+ outputs = self.transformer(
+ input_ids,
+ attention_mask=attention_mask,
+ position_ids=position_ids,
+ past_key_values=past_key_values,
+ inputs_embeds=inputs_embeds,
+ output_attentions=output_attentions,
+ output_hidden_states=output_hidden_states,
+ return_dict=return_dict,
+ )
+
+ sequence_output = outputs[0]
+
+ logits = self.qa_outputs(sequence_output)
+ start_logits, end_logits = logits.split(1, dim=-1)
+ start_logits = start_logits.squeeze(-1).contiguous()
+ end_logits = end_logits.squeeze(-1).contiguous()
+
+ total_loss = None
+ if start_positions is not None and end_positions is not None:
+ # If we are on multi-GPU, split add a dimension
+ if len(start_positions.size()) > 1:
+ start_positions = start_positions.squeeze(-1).to(
+ start_logits.device)
+ if len(end_positions.size()) > 1:
+ end_positions = end_positions.squeeze(-1).to(end_logits.device)
+ # sometimes the start/end positions are outside our model inputs, we ignore these terms
+ ignored_index = start_logits.size(1)
+ start_positions = start_positions.clamp(0, ignored_index)
+ end_positions = end_positions.clamp(0, ignored_index)
+
+ loss_fct = CrossEntropyLoss(ignore_index=ignored_index)
+ start_loss = loss_fct(start_logits, start_positions)
+ end_loss = loss_fct(end_logits, end_positions)
+ total_loss = (start_loss + end_loss) / 2
+
+ if not return_dict:
+ output = (start_logits, end_logits) + outputs[2:]
+ return ((total_loss, ) +
+ output) if total_loss is not None else output
+
+ return QuestionAnsweringModelOutput(
+ loss=total_loss,
+ start_logits=start_logits,
+ end_logits=end_logits,
+ hidden_states=outputs.hidden_states,
+ attentions=outputs.attentions,
+ )
+
+
+# Copied from transformers.models.llama.modeling_llama.LlamaForTokenClassification with Llama->InternLM2
+@add_start_docstrings(
+ """
+ The InternLM2 Model transformer with a token classification head on top (a linear layer on top of the hidden-states
+ output) e.g. for Named-Entity-Recognition (NER) tasks.
+ """,
+ InternLM2_START_DOCSTRING,
+)
+class InternLM2ForTokenClassification(InternLM2PreTrainedModel):
+ """Token classification model for InternLM2."""
+
+ def __init__(self, config):
+ super().__init__(config)
+ self.num_labels = config.num_labels
+ self.model = InternLM2Model(config)
+ if getattr(config, 'classifier_dropout', None) is not None:
+ classifier_dropout = config.classifier_dropout
+ elif getattr(config, 'hidden_dropout', None) is not None:
+ classifier_dropout = config.hidden_dropout
+ else:
+ classifier_dropout = 0.1
+ self.dropout = nn.Dropout(classifier_dropout)
+ self.score = nn.Linear(config.hidden_size, config.num_labels)
+
+ # Initialize weights and apply final processing
+ self.post_init()
+
+ def get_input_embeddings(self):
+ return self.model.tok_embeddings
+
+ def set_input_embeddings(self, value):
+ self.model.tok_embeddings = value
+
+ @add_start_docstrings_to_model_forward(InternLM2_INPUTS_DOCSTRING)
+ def forward(
+ self,
+ input_ids: torch.LongTensor = None,
+ attention_mask: Optional[torch.Tensor] = None,
+ position_ids: Optional[torch.LongTensor] = None,
+ past_key_values: Optional[List[torch.FloatTensor]] = None,
+ inputs_embeds: Optional[torch.FloatTensor] = None,
+ labels: Optional[torch.LongTensor] = None,
+ use_cache: Optional[bool] = None,
+ output_attentions: Optional[bool] = None,
+ output_hidden_states: Optional[bool] = None,
+ return_dict: Optional[bool] = None,
+ ) -> Union[Tuple, SequenceClassifierOutputWithPast]:
+ r"""
+ labels (`torch.LongTensor` of shape `(batch_size,)`, *optional*):
+ Labels for computing the sequence classification/regression loss. Indices should be in `[0, ...,
+ config.num_labels - 1]`. If `config.num_labels == 1` a regression loss is computed (Mean-Square loss), If
+ `config.num_labels > 1` a classification loss is computed (Cross-Entropy).
+ """
+ return_dict = return_dict if return_dict is not None else self.config.use_return_dict
+
+ outputs = self.model(
+ input_ids,
+ attention_mask=attention_mask,
+ position_ids=position_ids,
+ past_key_values=past_key_values,
+ inputs_embeds=inputs_embeds,
+ use_cache=use_cache,
+ output_attentions=output_attentions,
+ output_hidden_states=output_hidden_states,
+ return_dict=return_dict,
+ )
+ sequence_output = outputs[0]
+ sequence_output = self.dropout(sequence_output)
+ logits = self.score(sequence_output)
+
+ loss = None
+ if labels is not None:
+ loss_fct = CrossEntropyLoss()
+ loss = loss_fct(logits.view(-1, self.num_labels), labels.view(-1))
+
+ if not return_dict:
+ output = (logits, ) + outputs[2:]
+ return ((loss, ) + output) if loss is not None else output
+
+ return TokenClassifierOutput(
+ loss=loss,
+ logits=logits,
+ hidden_states=outputs.hidden_states,
+ attentions=outputs.attentions,
+ )
diff --git a/xtuner/_lite/modelings/llava/modeling_llava.py b/xtuner/_lite/modelings/llava/modeling_llava.py
new file mode 100644
index 000000000..b987db7b5
--- /dev/null
+++ b/xtuner/_lite/modelings/llava/modeling_llava.py
@@ -0,0 +1,573 @@
+# coding=utf-8
+# Copyright 2023 the HuggingFace Inc. team. All rights reserved.
+#
+# Licensed under the Apache License, Version 2.0 (the "License");
+# you may not use this file except in compliance with the License.
+# You may obtain a copy of the License at
+#
+# http://www.apache.org/licenses/LICENSE-2.0
+#
+# Unless required by applicable law or agreed to in writing, software
+# distributed under the License is distributed on an "AS IS" BASIS,
+# WITHOUT WARRANTIES OR CONDITIONS OF ANY KIND, either express or implied.
+# See the License for the specific language governing permissions and
+# limitations under the License.
+"""PyTorch Llava model."""
+
+from dataclasses import dataclass
+from typing import List, Optional, Tuple, Union
+
+import torch
+import torch.utils.checkpoint
+from torch import nn
+
+from transformers import PreTrainedModel
+from transformers.activations import ACT2FN
+from transformers.cache_utils import Cache
+from transformers.modeling_outputs import ModelOutput
+from transformers.utils import (
+ add_start_docstrings,
+ add_start_docstrings_to_model_forward,
+ logging,
+ replace_return_docstrings,
+)
+from transformers import AutoModel, AutoModelForCausalLM
+from .configuration_llava import EnhancedLlavaConfig
+
+
+logger = logging.get_logger(__name__)
+
+_CONFIG_FOR_DOC = "LlavaConfig"
+
+
+
+@dataclass
+# Copied from transformers.models.idefics.modeling_idefics.IdeficsCausalLMOutputWithPast with Idefics->Llava
+class LlavaCausalLMOutputWithPast(ModelOutput):
+ """
+ Base class for Llava causal language model (or autoregressive) outputs.
+
+ Args:
+ loss (`torch.FloatTensor` of shape `(1,)`, *optional*, returned when `labels` is provided):
+ Language modeling loss (for next-token prediction).
+ logits (`torch.FloatTensor` of shape `(batch_size, sequence_length, config.vocab_size)`):
+ Prediction scores of the language modeling head (scores for each vocabulary token before SoftMax).
+ past_key_values (`tuple(tuple(torch.FloatTensor))`, *optional*, returned when `use_cache=True` is passed or when `config.use_cache=True`):
+ Tuple of `tuple(torch.FloatTensor)` of length `config.n_layers`, with each tuple having 2 tensors of shape
+ `(batch_size, num_heads, sequence_length, embed_size_per_head)`)
+
+ Contains pre-computed hidden-states (key and values in the self-attention blocks) that can be used (see
+ `past_key_values` input) to speed up sequential decoding.
+ hidden_states (`tuple(torch.FloatTensor)`, *optional*, returned when `output_hidden_states=True` is passed or when `config.output_hidden_states=True`):
+ Tuple of `torch.FloatTensor` (one for the output of the embeddings, if the model has an embedding layer, +
+ one for the output of each layer) of shape `(batch_size, sequence_length, hidden_size)`.
+
+ Hidden-states of the model at the output of each layer plus the optional initial embedding outputs.
+ attentions (`tuple(torch.FloatTensor)`, *optional*, returned when `output_attentions=True` is passed or when `config.output_attentions=True`):
+ Tuple of `torch.FloatTensor` (one for each layer) of shape `(batch_size, num_heads, sequence_length,
+ sequence_length)`.
+
+ Attentions weights after the attention softmax, used to compute the weighted average in the self-attention
+ heads.
+ image_hidden_states (`tuple(torch.FloatTensor)`, *optional*):
+ Tuple of `torch.FloatTensor` (one for the output of the image embeddings, `(batch_size, num_images,
+ sequence_length, hidden_size)`.
+
+ image_hidden_states of the model produced by the vision encoder, and optionally by the perceiver
+ """
+
+ loss: Optional[torch.FloatTensor] = None
+ logits: torch.FloatTensor = None
+ past_key_values: Optional[List[torch.FloatTensor]] = None
+ hidden_states: Optional[Tuple[torch.FloatTensor]] = None
+ attentions: Optional[Tuple[torch.FloatTensor]] = None
+ image_hidden_states: Optional[Tuple[torch.FloatTensor]] = None
+
+
+class LlavaMultiModalProjector(nn.Module):
+ def __init__(self, config: EnhancedLlavaConfig):
+ super().__init__()
+
+ self.linear_1 = nn.Linear(config.vision_config.hidden_size, config.text_config.hidden_size, bias=True)
+ self.act = ACT2FN[config.projector_hidden_act]
+ self.linear_2 = nn.Linear(config.text_config.hidden_size, config.text_config.hidden_size, bias=True)
+
+ def forward(self, image_features):
+ hidden_states = self.linear_1(image_features)
+ hidden_states = self.act(hidden_states)
+ hidden_states = self.linear_2(hidden_states)
+ return hidden_states
+
+
+LLAVA_START_DOCSTRING = r"""
+ This model inherits from [`PreTrainedModel`]. Check the superclass documentation for the generic methods the
+ library implements for all its model (such as downloading or saving, resizing the input embeddings, pruning heads
+ etc.)
+
+ This model is also a PyTorch [torch.nn.Module](https://pytorch.org/docs/stable/nn.html#torch.nn.Module) subclass.
+ Use it as a regular PyTorch Module and refer to the PyTorch documentation for all matter related to general usage
+ and behavior.
+
+ Parameters:
+ config ([`LlavaConfig`] or [`LlavaVisionConfig`]):
+ Model configuration class with all the parameters of the model. Initializing with a config file does not
+ load the weights associated with the model, only the configuration. Check out the
+ [`~PreTrainedModel.from_pretrained`] method to load the model weights.
+"""
+
+
+@add_start_docstrings(
+ "The bare LLaMA Model outputting raw hidden-states without any specific head on top.",
+ LLAVA_START_DOCSTRING,
+)
+class LlavaPreTrainedModel(PreTrainedModel):
+ config_class = EnhancedLlavaConfig
+ base_model_prefix = "model"
+ supports_gradient_checkpointing = True
+ _no_split_modules = ["LlavaVisionAttention"]
+ _skip_keys_device_placement = "past_key_values"
+ _supports_flash_attn_2 = True
+
+ def _init_weights(self, module):
+ # important: this ported version of Llava isn't meant for training from scratch - only
+ # inference and fine-tuning - so the proper init weights code has been removed - the original codebase
+ # https://github.com/haotian-liu/LLaVA/tree/main/llava should serve for that purpose
+ std = (
+ self.config.initializer_range
+ if hasattr(self.config, "initializer_range")
+ else self.config.text_config.initializer_range
+ )
+
+ if hasattr(module, "class_embedding"):
+ module.class_embedding.data.normal_(mean=0.0, std=std)
+
+ if isinstance(module, (nn.Linear, nn.Conv2d)):
+ module.weight.data.normal_(mean=0.0, std=std)
+ if module.bias is not None:
+ module.bias.data.zero_()
+ elif isinstance(module, nn.Embedding):
+ module.weight.data.normal_(mean=0.0, std=std)
+ if module.padding_idx is not None:
+ module.weight.data[module.padding_idx].zero_()
+
+ @property
+ def _supports_sdpa(self):
+ """
+ Retrieve language_model's attribute to check whether the model supports
+ SDPA or not.
+ """
+ return self.language_model._supports_sdpa
+
+
+LLAVA_INPUTS_DOCSTRING = r"""
+ Args:
+ input_ids (`torch.LongTensor` of shape `(batch_size, sequence_length)`):
+ Indices of input sequence tokens in the vocabulary. Padding will be ignored by default should you provide
+ it.
+
+ Indices can be obtained using [`AutoTokenizer`]. See [`PreTrainedTokenizer.encode`] and
+ [`PreTrainedTokenizer.__call__`] for details.
+
+ [What are input IDs?](../glossary#input-ids)
+ pixel_values (`torch.FloatTensor` of shape `(batch_size, num_channels, image_size, image_size)):
+ The tensors corresponding to the input images. Pixel values can be obtained using
+ [`AutoImageProcessor`]. See [`CLIPImageProcessor.__call__`] for details ([]`LlavaProcessor`] uses
+ [`CLIPImageProcessor`] for processing images).
+ attention_mask (`torch.Tensor` of shape `(batch_size, sequence_length)`, *optional*):
+ Mask to avoid performing attention on padding token indices. Mask values selected in `[0, 1]`:
+
+ - 1 for tokens that are **not masked**,
+ - 0 for tokens that are **masked**.
+
+ [What are attention masks?](../glossary#attention-mask)
+
+ Indices can be obtained using [`AutoTokenizer`]. See [`PreTrainedTokenizer.encode`] and
+ [`PreTrainedTokenizer.__call__`] for details.
+
+ If `past_key_values` is used, optionally only the last `decoder_input_ids` have to be input (see
+ `past_key_values`).
+
+ If you want to change padding behavior, you should read [`modeling_opt._prepare_decoder_attention_mask`]
+ and modify to your needs. See diagram 1 in [the paper](https://arxiv.org/abs/1910.13461) for more
+ information on the default strategy.
+
+ - 1 indicates the head is **not masked**,
+ - 0 indicates the head is **masked**.
+ position_ids (`torch.LongTensor` of shape `(batch_size, sequence_length)`, *optional*):
+ Indices of positions of each input sequence tokens in the position embeddings. Selected in the range `[0,
+ config.n_positions - 1]`. [What are position IDs?](../glossary#position-ids)
+ past_key_values (`tuple(tuple(torch.FloatTensor))`, *optional*, returned when `use_cache=True` is passed or when `config.use_cache=True`):
+ Tuple of `tuple(torch.FloatTensor)` of length `config.n_layers`, with each tuple having 2 tensors of shape
+ `(batch_size, num_heads, sequence_length, embed_size_per_head)`) and 2 additional tensors of shape
+ `(batch_size, num_heads, encoder_sequence_length, embed_size_per_head)`.
+
+ Contains pre-computed hidden-states (key and values in the self-attention blocks and in the cross-attention
+ blocks) that can be used (see `past_key_values` input) to speed up sequential decoding.
+
+ If `past_key_values` are used, the user can optionally input only the last `decoder_input_ids` (those that
+ don't have their past key value states given to this model) of shape `(batch_size, 1)` instead of all
+ `decoder_input_ids` of shape `(batch_size, sequence_length)`.
+ inputs_embeds (`torch.FloatTensor` of shape `(batch_size, sequence_length, hidden_size)`, *optional*):
+ Optionally, instead of passing `input_ids` you can choose to directly pass an embedded representation. This
+ is useful if you want more control over how to convert `input_ids` indices into associated vectors than the
+ model's internal embedding lookup matrix.
+ vision_feature_layer (`int`, *optional*, defaults to -2):
+ The index of the layer to select the vision feature.
+ vision_feature_select_strategy (`str`, *optional*, defaults to `"default"`):
+ The feature selection strategy used to select the vision feature from the vision backbone.
+ Can be one of `"default"` or `"full"`.
+ use_cache (`bool`, *optional*):
+ If set to `True`, `past_key_values` key value states are returned and can be used to speed up decoding (see
+ `past_key_values`).
+ output_attentions (`bool`, *optional*):
+ Whether or not to return the attentions tensors of all attention layers. See `attentions` under returned
+ tensors for more detail.
+ output_hidden_states (`bool`, *optional*):
+ Whether or not to return the hidden states of all layers. See `hidden_states` under returned tensors for
+ more detail.
+ return_dict (`bool`, *optional*):
+ Whether or not to return a [`~utils.ModelOutput`] instead of a plain tuple.
+"""
+
+
+@add_start_docstrings(
+ """The LLAVA model which consists of a vision backbone and a language model.""",
+ LLAVA_START_DOCSTRING,
+)
+class LlavaForConditionalGeneration(LlavaPreTrainedModel):
+
+ _auto_class = 'AutoModel'
+
+ def __init__(self, config: EnhancedLlavaConfig):
+ super().__init__(config)
+ self.vision_tower = AutoModel.from_config(config.vision_config)
+
+ self.multi_modal_projector = LlavaMultiModalProjector(config)
+ self.vocab_size = config.text_config.vocab_size
+ self.language_model = AutoModelForCausalLM.from_config(
+ config.text_config,
+ attn_implementation=config._attn_implementation)
+ self.pad_token_id = self.config.pad_token_id if self.config.pad_token_id is not None else -1
+ self.post_init()
+
+ def get_input_embeddings(self):
+ return self.language_model.get_input_embeddings()
+
+ def set_input_embeddings(self, value):
+ self.language_model.set_input_embeddings(value)
+
+ def get_output_embeddings(self):
+ return self.language_model.get_output_embeddings()
+
+ def set_output_embeddings(self, new_embeddings):
+ self.language_model.set_output_embeddings(new_embeddings)
+
+ def set_decoder(self, decoder):
+ self.language_model.set_decoder(decoder)
+
+ def get_decoder(self):
+ return self.language_model.get_decoder()
+
+ def tie_weights(self):
+ return self.language_model.tie_weights()
+
+ def resize_token_embeddings(self, new_num_tokens: Optional[int] = None, pad_to_multiple_of=None) -> nn.Embedding:
+ model_embeds = self.language_model.resize_token_embeddings(new_num_tokens, pad_to_multiple_of)
+ # update vocab size
+ self.config.text_config.vocab_size = model_embeds.num_embeddings
+ self.vocab_size = model_embeds.num_embeddings
+ return model_embeds
+
+ def _merge_input_ids_with_image_features(self, image_features, inputs_embeds, input_ids, attention_mask, labels):
+ num_images, num_image_patches, embed_dim = image_features.shape
+ batch_size, sequence_length = input_ids.shape
+ left_padding = not torch.sum(input_ids[:, -1] == torch.tensor(self.pad_token_id))
+ # 1. Create a mask to know where special image tokens are
+ special_image_token_mask = input_ids == self.config.image_token_index
+ num_special_image_tokens = torch.sum(special_image_token_mask, dim=-1)
+ # Compute the maximum embed dimension
+ max_embed_dim = (num_special_image_tokens.max() * (num_image_patches - 1)) + sequence_length
+ batch_indices, non_image_indices = torch.where(input_ids != self.config.image_token_index)
+
+ # 2. Compute the positions where text should be written
+ # Calculate new positions for text tokens in merged image-text sequence.
+ # `special_image_token_mask` identifies image tokens. Each image token will be replaced by `nb_text_tokens_per_images - 1` text tokens.
+ # `torch.cumsum` computes how each image token shifts subsequent text token positions.
+ # - 1 to adjust for zero-based indexing, as `cumsum` inherently increases indices by one.
+ new_token_positions = torch.cumsum((special_image_token_mask * (num_image_patches - 1) + 1), -1) - 1
+ nb_image_pad = max_embed_dim - 1 - new_token_positions[:, -1]
+ if left_padding:
+ new_token_positions += nb_image_pad[:, None] # offset for left padding
+ text_to_overwrite = new_token_positions[batch_indices, non_image_indices]
+
+ # 3. Create the full embedding, already padded to the maximum position
+ final_embedding = torch.zeros(
+ batch_size, max_embed_dim, embed_dim, dtype=inputs_embeds.dtype, device=inputs_embeds.device
+ )
+ final_attention_mask = torch.zeros(
+ batch_size, max_embed_dim, dtype=attention_mask.dtype, device=inputs_embeds.device
+ )
+ if labels is not None:
+ final_labels = torch.full(
+ (batch_size, max_embed_dim), self.config.ignore_index, dtype=input_ids.dtype, device=input_ids.device
+ )
+ # In case the Vision model or the Language model has been offloaded to CPU, we need to manually
+ # set the corresponding tensors into their correct target device.
+ target_device = inputs_embeds.device
+ batch_indices, non_image_indices, text_to_overwrite = (
+ batch_indices.to(target_device),
+ non_image_indices.to(target_device),
+ text_to_overwrite.to(target_device),
+ )
+ attention_mask = attention_mask.to(target_device)
+
+ # 4. Fill the embeddings based on the mask. If we have ["hey" "", "how", "are"]
+ # we need to index copy on [0, 577, 578, 579] for the text and [1:576] for the image features
+ final_embedding[batch_indices, text_to_overwrite] = inputs_embeds[batch_indices, non_image_indices]
+ final_attention_mask[batch_indices, text_to_overwrite] = attention_mask[batch_indices, non_image_indices]
+ if labels is not None:
+ final_labels[batch_indices, text_to_overwrite] = labels[batch_indices, non_image_indices]
+
+ # 5. Fill the embeddings corresponding to the images. Anything that is not `text_positions` needs filling (#29835)
+ image_to_overwrite = torch.full(
+ (batch_size, max_embed_dim), True, dtype=torch.bool, device=inputs_embeds.device
+ )
+ image_to_overwrite[batch_indices, text_to_overwrite] = False
+ image_to_overwrite &= image_to_overwrite.cumsum(-1) - 1 >= nb_image_pad[:, None].to(target_device)
+
+ if image_to_overwrite.sum() != image_features.shape[:-1].numel():
+ raise ValueError(
+ f"The input provided to the model are wrong. The number of image tokens is {torch.sum(special_image_token_mask)} while"
+ f" the number of image given to the model is {num_images}. This prevents correct indexing and breaks batch generation."
+ )
+
+ final_embedding[image_to_overwrite] = image_features.contiguous().reshape(-1, embed_dim).to(target_device)
+ final_attention_mask |= image_to_overwrite
+ position_ids = (final_attention_mask.cumsum(-1) - 1).masked_fill_((final_attention_mask == 0), 1)
+
+ # 6. Mask out the embedding at padding positions, as we later use the past_key_value value to determine the non-attended tokens.
+ batch_indices, pad_indices = torch.where(input_ids == self.pad_token_id)
+ indices_to_mask = new_token_positions[batch_indices, pad_indices]
+
+ final_embedding[batch_indices, indices_to_mask] = 0
+
+ if labels is None:
+ final_labels = None
+
+ return final_embedding, final_attention_mask, final_labels, position_ids
+
+
+ def forward(
+ self,
+ input_ids: torch.LongTensor = None,
+ pixel_values: torch.FloatTensor = None,
+ attention_mask: Optional[torch.Tensor] = None,
+ position_ids: Optional[torch.LongTensor] = None,
+ past_key_values: Optional[List[torch.FloatTensor]] = None,
+ inputs_embeds: Optional[torch.FloatTensor] = None,
+ vision_feature_layer: Optional[int] = None,
+ vision_feature_select_strategy: Optional[str] = None,
+ labels: Optional[torch.LongTensor] = None,
+ use_cache: Optional[bool] = None,
+ output_attentions: Optional[bool] = None,
+ output_hidden_states: Optional[bool] = None,
+ return_dict: Optional[bool] = None,
+ ) -> Union[Tuple, LlavaCausalLMOutputWithPast]:
+
+ output_attentions = output_attentions if output_attentions is not None else self.config.output_attentions
+ output_hidden_states = (
+ output_hidden_states if output_hidden_states is not None else self.config.output_hidden_states
+ )
+ return_dict = return_dict if return_dict is not None else self.config.use_return_dict
+ vision_feature_layer = (
+ vision_feature_layer if vision_feature_layer is not None else self.config.vision_feature_layer
+ )
+ vision_feature_select_strategy = (
+ vision_feature_select_strategy
+ if vision_feature_select_strategy is not None
+ else self.config.vision_feature_select_strategy
+ )
+
+ if inputs_embeds is None:
+ # 1. Extra the input embeddings
+ inputs_embeds = self.get_input_embeddings()(input_ids)
+
+ # ------------- start add this ----------------
+ if pixel_values is None and self.training:
+ # all of the input is text
+ # If not handled properly, deadlock can occur.
+ # print('===================all of the input is text==============')
+ image_size = self.config.vision_config.image_size
+ pixel_values = torch.zeros(input_ids.shape[0], 3, image_size, image_size,
+ dtype=torch.float32,
+ device=input_ids.device)
+ image_outputs = self.vision_tower(pixel_values, output_hidden_states=True)
+ # this is not memory efficient at all (output_hidden_states=True) will save all the hidden stated.
+ selected_image_feature = image_outputs.hidden_states[vision_feature_layer]
+ if vision_feature_select_strategy == "default":
+ selected_image_feature = selected_image_feature[:, 1:]
+ elif vision_feature_select_strategy == "full":
+ selected_image_feature = selected_image_feature
+ else:
+ raise ValueError(
+ f"Unexpected select feature strategy: {self.config.vision_feature_select_strategy}"
+ )
+ image_features = self.multi_modal_projector(selected_image_feature)
+ inputs_embeds = inputs_embeds.to(image_features.dtype)
+ inputs_embeds, attention_mask, labels, position_ids = self._merge_input_ids_with_image_features(
+ image_features[0:0], inputs_embeds, input_ids, attention_mask, labels
+ )
+ # ------------- end add this ----------------
+ # 2. Merge text and images
+ elif pixel_values is not None and input_ids.shape[1] != 1:
+ image_outputs = self.vision_tower(pixel_values, output_hidden_states=True)
+ # this is not memory efficient at all (output_hidden_states=True) will save all the hidden stated.
+ selected_image_feature = image_outputs.hidden_states[vision_feature_layer]
+
+ if vision_feature_select_strategy == "default":
+ selected_image_feature = selected_image_feature[:, 1:]
+ elif vision_feature_select_strategy == "full":
+ selected_image_feature = selected_image_feature
+ else:
+ raise ValueError(
+ f"Unexpected select feature strategy: {self.config.vision_feature_select_strategy}"
+ )
+
+ image_features = self.multi_modal_projector(selected_image_feature)
+ inputs_embeds = inputs_embeds.to(image_features.dtype)
+ inputs_embeds, attention_mask, labels, position_ids = self._merge_input_ids_with_image_features(
+ image_features, inputs_embeds, input_ids, attention_mask, labels
+ )
+
+ # In case input_ids.shape[1] == 1 & pixel_values==None & past_key_values != None, we are in the case of
+ # generation with cache
+ elif past_key_values is not None and pixel_values is not None and input_ids.shape[1] == 1:
+ # Retrieve the first layer to inspect the logits and mask out the hidden states
+ # that are set to 0
+ first_layer_past_key_value = past_key_values[0][0][:, :, :, 0]
+
+ # Sum all dimensions of head_dim (-2) to avoid random errors such as: https://github.com/huggingface/transformers/pull/28032#issuecomment-1863691941
+ batch_index, non_attended_tokens = torch.where(first_layer_past_key_value.float().sum(-2) == 0)
+
+ # Get the target length
+ target_length = input_ids.shape[1]
+ past_length = first_layer_past_key_value.shape[-1]
+
+ extended_attention_mask = torch.ones(
+ (attention_mask.shape[0], past_length),
+ dtype=attention_mask.dtype,
+ device=attention_mask.device,
+ )
+
+ # Filter out only the tokens that can be un-attended, this can happen
+ # if one uses Llava + Fused modules where the cache on the
+ # first iteration is already big enough, or if one passes custom cache
+ valid_indices = non_attended_tokens < extended_attention_mask.size(-1)
+ new_batch_index = batch_index[valid_indices]
+ new_non_attended_tokens = non_attended_tokens[valid_indices]
+
+ # Zero-out the places where we don't need to attend
+ extended_attention_mask[new_batch_index, new_non_attended_tokens] = 0
+
+ attention_mask = torch.cat((extended_attention_mask, attention_mask[:, -target_length:]), dim=1)
+ position_ids = torch.sum(attention_mask, dim=1).unsqueeze(-1) - 1
+
+ outputs = self.language_model(
+ attention_mask=attention_mask,
+ position_ids=position_ids,
+ past_key_values=past_key_values,
+ inputs_embeds=inputs_embeds,
+ use_cache=use_cache,
+ output_attentions=output_attentions,
+ output_hidden_states=output_hidden_states,
+ return_dict=return_dict,
+ )
+
+ logits = outputs[0]
+
+ loss = None
+ if labels is not None:
+ # Shift so that tokens < n predict n
+ if attention_mask is not None:
+ shift_attention_mask = attention_mask[..., 1:]
+ shift_logits = logits[..., :-1, :][shift_attention_mask.to(logits.device) != 0].contiguous()
+ shift_labels = labels[..., 1:][shift_attention_mask.to(labels.device) != 0].contiguous()
+ else:
+ shift_logits = logits[..., :-1, :].contiguous()
+ shift_labels = labels[..., 1:].contiguous()
+ # Flatten the tokens
+ loss_fct = nn.CrossEntropyLoss()
+ loss = loss_fct(
+ shift_logits.view(-1, shift_logits.size(-1)), shift_labels.view(-1).to(shift_logits.device)
+ )
+
+ if not return_dict:
+ output = (logits,) + outputs[1:]
+ return (loss,) + output if loss is not None else output
+
+ return LlavaCausalLMOutputWithPast(
+ loss=loss,
+ logits=logits,
+ past_key_values=outputs.past_key_values,
+ hidden_states=outputs.hidden_states,
+ attentions=outputs.attentions,
+ )
+
+ def prepare_inputs_for_generation(
+ self, input_ids, past_key_values=None, inputs_embeds=None, pixel_values=None, attention_mask=None, **kwargs
+ ):
+ if past_key_values is not None:
+ if isinstance(past_key_values, Cache):
+ cache_length = past_key_values.get_seq_length()
+ past_length = past_key_values.seen_tokens
+ else:
+ cache_length = past_length = past_key_values[0][0].shape[2]
+
+ # Keep only the unprocessed tokens:
+ # 1 - If the length of the attention_mask exceeds the length of input_ids, then we are in a setting where
+ # some of the inputs are exclusively passed as part of the cache (e.g. when passing input_embeds as
+ # input)
+ if attention_mask is not None and attention_mask.shape[1] > input_ids.shape[1]:
+ input_ids = input_ids[:, -(attention_mask.shape[1] - past_length) :]
+ # 2 - If the past_length is smaller than input_ids', then input_ids holds all input tokens. We can discard
+ # input_ids based on the past_length.
+ elif past_length < input_ids.shape[1]:
+ input_ids = input_ids[:, past_length:]
+ # 3 - Otherwise (past_length >= input_ids.shape[1]), let's assume input_ids only has unprocessed tokens.
+ elif self.config.image_token_index in input_ids:
+ input_ids = input_ids[:, input_ids.shape[1] - 1 :]
+ # If the cache has seen more tokens than it can hold, then the cache has a size limit. Let's discard the
+ # older attention values, as their corresponding values are not part of the input.
+ if cache_length < past_length and attention_mask is not None:
+ attention_mask = attention_mask[:, -(cache_length + input_ids.shape[1]) :]
+
+ position_ids = kwargs.get("position_ids", None)
+ if attention_mask is not None and position_ids is None:
+ # create position_ids on the fly for batch generation
+ position_ids = attention_mask.long().cumsum(-1) - 1
+ position_ids.masked_fill_(attention_mask == 0, 1)
+ if past_key_values:
+ position_ids = position_ids[:, -input_ids.shape[1] :]
+
+ # if `inputs_embeds` are passed, we only want to use them in the 1st generation step
+ if inputs_embeds is not None and past_key_values is None:
+ model_inputs = {"inputs_embeds": inputs_embeds}
+ else:
+ model_inputs = {"input_ids": input_ids}
+
+ model_inputs.update(
+ {
+ "position_ids": position_ids,
+ "past_key_values": past_key_values,
+ "use_cache": kwargs.get("use_cache"),
+ "attention_mask": attention_mask,
+ "pixel_values": pixel_values,
+ }
+ )
+ return model_inputs
+
+ def _reorder_cache(self, *args, **kwargs):
+ return self.language_model._reorder_cache(*args, **kwargs)
+
+AutoModel.register(EnhancedLlavaConfig, LlavaForConditionalGeneration, exist_ok=True)
+AutoModelForCausalLM.register(EnhancedLlavaConfig, LlavaForConditionalGeneration, exist_ok=True)
\ No newline at end of file
diff --git a/xtuner/_lite/modelings/llava/processing_llava.py b/xtuner/_lite/modelings/llava/processing_llava.py
new file mode 100644
index 000000000..230975575
--- /dev/null
+++ b/xtuner/_lite/modelings/llava/processing_llava.py
@@ -0,0 +1,137 @@
+# coding=utf-8
+# Copyright 2023 The HuggingFace Inc. team.
+#
+# Licensed under the Apache License, Version 2.0 (the "License");
+# you may not use this file except in compliance with the License.
+# You may obtain a copy of the License at
+#
+# http://www.apache.org/licenses/LICENSE-2.0
+#
+# Unless required by applicable law or agreed to in writing, software
+# distributed under the License is distributed on an "AS IS" BASIS,
+# WITHOUT WARRANTIES OR CONDITIONS OF ANY KIND, either express or implied.
+# See the License for the specific language governing permissions and
+# limitations under the License.
+"""
+Processor class for Llava.
+"""
+
+from typing import List, Optional, Union
+
+from transformers.feature_extraction_utils import BatchFeature
+from transformers.image_utils import ImageInput
+from transformers.processing_utils import ProcessorMixin
+from transformers.tokenization_utils_base import PaddingStrategy, PreTokenizedInput, TextInput, TruncationStrategy
+from transformers.utils import TensorType
+
+
+class LlavaProcessor(ProcessorMixin):
+ r"""
+ Constructs a Llava processor which wraps a Llava image processor and a Llava tokenizer into a single processor.
+
+ [`LlavaProcessor`] offers all the functionalities of [`CLIPImageProcessor`] and [`LlamaTokenizerFast`]. See the
+ [`~LlavaProcessor.__call__`] and [`~LlavaProcessor.decode`] for more information.
+
+ Args:
+ image_processor ([`CLIPImageProcessor`], *optional*):
+ The image processor is a required input.
+ tokenizer ([`LlamaTokenizerFast`], *optional*):
+ The tokenizer is a required input.
+ chat_template (`str`, *optional*): A Jinja template which will be used to convert lists of messages
+ in a chat into a tokenizable string.
+ """
+
+ attributes = ["image_processor", "tokenizer"]
+ valid_kwargs = ["chat_template"]
+ image_processor_class = "AutoImageProcessor"
+ tokenizer_class = "AutoTokenizer"
+
+ def __init__(self, image_processor=None, tokenizer=None, chat_template=None, **kwargs):
+ super().__init__(image_processor, tokenizer, chat_template=chat_template)
+
+ def __call__(
+ self,
+ text: Union[TextInput, PreTokenizedInput, List[TextInput], List[PreTokenizedInput]] = None,
+ images: ImageInput = None,
+ padding: Union[bool, str, PaddingStrategy] = False,
+ truncation: Union[bool, str, TruncationStrategy] = None,
+ max_length=None,
+ return_tensors: Optional[Union[str, TensorType]] = TensorType.PYTORCH,
+ ) -> BatchFeature:
+ """
+ Main method to prepare for the model one or several sequences(s) and image(s). This method forwards the `text`
+ and `kwargs` arguments to LlamaTokenizerFast's [`~LlamaTokenizerFast.__call__`] if `text` is not `None` to encode
+ the text. To prepare the image(s), this method forwards the `images` and `kwrags` arguments to
+ CLIPImageProcessor's [`~CLIPImageProcessor.__call__`] if `images` is not `None`. Please refer to the doctsring
+ of the above two methods for more information.
+
+ Args:
+ text (`str`, `List[str]`, `List[List[str]]`):
+ The sequence or batch of sequences to be encoded. Each sequence can be a string or a list of strings
+ (pretokenized string). If the sequences are provided as list of strings (pretokenized), you must set
+ `is_split_into_words=True` (to lift the ambiguity with a batch of sequences).
+ images (`PIL.Image.Image`, `np.ndarray`, `torch.Tensor`, `List[PIL.Image.Image]`, `List[np.ndarray]`, `List[torch.Tensor]`):
+ The image or batch of images to be prepared. Each image can be a PIL image, NumPy array or PyTorch
+ tensor. Both channels-first and channels-last formats are supported.
+ padding (`bool`, `str` or [`~utils.PaddingStrategy`], *optional*, defaults to `False`):
+ Select a strategy to pad the returned sequences (according to the model's padding side and padding
+ index) among:
+ - `True` or `'longest'`: Pad to the longest sequence in the batch (or no padding if only a single
+ sequence if provided).
+ - `'max_length'`: Pad to a maximum length specified with the argument `max_length` or to the maximum
+ acceptable input length for the model if that argument is not provided.
+ - `False` or `'do_not_pad'` (default): No padding (i.e., can output a batch with sequences of different
+ lengths).
+ max_length (`int`, *optional*):
+ Maximum length of the returned list and optionally padding length (see above).
+ truncation (`bool`, *optional*):
+ Activates truncation to cut input sequences longer than `max_length` to `max_length`.
+ return_tensors (`str` or [`~utils.TensorType`], *optional*):
+ If set, will return tensors of a particular framework. Acceptable values are:
+
+ - `'tf'`: Return TensorFlow `tf.constant` objects.
+ - `'pt'`: Return PyTorch `torch.Tensor` objects.
+ - `'np'`: Return NumPy `np.ndarray` objects.
+ - `'jax'`: Return JAX `jnp.ndarray` objects.
+
+ Returns:
+ [`BatchFeature`]: A [`BatchFeature`] with the following fields:
+
+ - **input_ids** -- List of token ids to be fed to a model. Returned when `text` is not `None`.
+ - **attention_mask** -- List of indices specifying which tokens should be attended to by the model (when
+ `return_attention_mask=True` or if *"attention_mask"* is in `self.model_input_names` and if `text` is not
+ `None`).
+ - **pixel_values** -- Pixel values to be fed to a model. Returned when `images` is not `None`.
+ """
+ if images is not None:
+ image_inputs = self.image_processor(images, return_tensors=return_tensors)
+ else:
+ image_inputs = {}
+ text_inputs = self.tokenizer(
+ text, return_tensors=return_tensors, padding=padding, truncation=truncation, max_length=max_length
+ )
+
+ return BatchFeature(data={**text_inputs, **image_inputs})
+
+ # Copied from transformers.models.clip.processing_clip.CLIPProcessor.batch_decode with CLIP->Llama
+ def batch_decode(self, *args, **kwargs):
+ """
+ This method forwards all its arguments to LlamaTokenizerFast's [`~PreTrainedTokenizer.batch_decode`]. Please
+ refer to the docstring of this method for more information.
+ """
+ return self.tokenizer.batch_decode(*args, **kwargs)
+
+ # Copied from transformers.models.clip.processing_clip.CLIPProcessor.decode with CLIP->Llama
+ def decode(self, *args, **kwargs):
+ """
+ This method forwards all its arguments to LlamaTokenizerFast's [`~PreTrainedTokenizer.decode`]. Please refer to
+ the docstring of this method for more information.
+ """
+ return self.tokenizer.decode(*args, **kwargs)
+
+ @property
+ # Copied from transformers.models.clip.processing_clip.CLIPProcessor.model_input_names
+ def model_input_names(self):
+ tokenizer_input_names = self.tokenizer.model_input_names
+ image_processor_input_names = self.image_processor.model_input_names
+ return list(dict.fromkeys(tokenizer_input_names + image_processor_input_names))
\ No newline at end of file
diff --git a/xtuner/_lite/parallel/__init__.py b/xtuner/_lite/parallel/__init__.py
new file mode 100644
index 000000000..b2a020032
--- /dev/null
+++ b/xtuner/_lite/parallel/__init__.py
@@ -0,0 +1,15 @@
+# Copyright (c) OpenMMLab. All rights reserved.
+from .comm import all_to_all, all_to_all_list, barrier
+from .sampler import LengthGroupedSampler, ParallelSampler, VLMLengthGroupedSampler
+from .sequence import * # noqa: F401, F403
+from .setup import setup_parallel
+
+__all__ = [
+ 'ParallelSampler',
+ 'LengthGroupedSampler',
+ 'VLMLengthGroupedSampler',
+ 'all_to_all',
+ 'all_to_all_list',
+ 'setup_parallel',
+ 'barrier'
+]
diff --git a/xtuner/_lite/parallel/comm.py b/xtuner/_lite/parallel/comm.py
new file mode 100644
index 000000000..47daf4fb6
--- /dev/null
+++ b/xtuner/_lite/parallel/comm.py
@@ -0,0 +1,135 @@
+# Copyright (c) OpenMMLab. All rights reserved.
+from typing import Any, Tuple
+
+import torch
+import torch.distributed as dist
+from torch import Tensor
+from torch.distributed.distributed_c10d import (_get_pg_default_device,
+ _object_to_tensor,
+ _tensor_to_object)
+
+
+# Modified from https://github.com/microsoft/DeepSpeed/blob/ffd0a0e3ef24bfd00c2e5f35019d2674cc01ec14/deepspeed/sequence/layer.py#L15 # noqa: E501
+def _all_to_all(
+ input: Tensor,
+ world_size: int,
+ group: dist.ProcessGroup,
+ scatter_dim: int,
+ gather_dim: int,
+):
+ input_list = [
+ t.contiguous()
+ for t in torch.tensor_split(input, world_size, scatter_dim)
+ ]
+ output_list = [torch.empty_like(input_list[0]) for _ in range(world_size)]
+ dist.all_to_all(output_list, input_list, group=group)
+ return torch.cat(output_list, dim=gather_dim).contiguous()
+
+
+class _AllToAll(torch.autograd.Function):
+ """All-to-all communication.
+
+ Args:
+ input: Input tensor
+ sp_group: Sequence parallel process group
+ scatter_dim: Scatter dimension
+ gather_dim: Gather dimension
+ """
+
+ @staticmethod
+ def forward(ctx: Any, input: Tensor, sp_group: dist.ProcessGroup,
+ scatter_dim: int, gather_dim: int):
+ ctx.sp_group = sp_group
+ ctx.scatter_dim = scatter_dim
+ ctx.gather_dim = gather_dim
+ ctx.world_size = dist.get_world_size(sp_group)
+ output = _all_to_all(input, ctx.world_size, sp_group, scatter_dim,
+ gather_dim)
+ return output
+
+ @staticmethod
+ def backward(ctx: Any, grad_output: Tensor) -> Tuple:
+ grad_output = _all_to_all(
+ grad_output,
+ ctx.world_size,
+ ctx.sp_group,
+ ctx.gather_dim,
+ ctx.scatter_dim,
+ )
+ return (
+ grad_output,
+ None,
+ None,
+ None,
+ )
+
+
+def all_to_all(
+ input: Tensor,
+ sp_group: dist.ProcessGroup,
+ scatter_dim: int = 2,
+ gather_dim: int = 1,
+):
+ """Convenience function to apply the all-to-all operation with scatter and
+ gather dimensions.
+
+ Notes:
+ We have wrapped the `torch.distributed.all_to_all` function to
+ enable automatic differentiation of the all-to-all operation.
+
+ Args:
+ input: The input tensor for which all-to-all communication is performed
+ sp_group: The sequence parallel process group.
+ scatter_dim: The dimension along which the input tensor is scattered
+ (default: 2).
+ gather_dim: The dimension along which the output tensor is gathered
+ (default: 1).
+
+ Returns:
+ The output tensor after the all-to-all communication.
+ """
+ return _AllToAll.apply(input, sp_group, scatter_dim, gather_dim)
+
+
+def all_to_all_list(object_list, group=None):
+ current_device = _get_pg_default_device(group)
+ rank = dist.get_rank(group)
+ world_size = dist.get_world_size(group)
+ tensor_list, size_list = zip(
+ *
+ [_object_to_tensor(obj, current_device, group) for obj in object_list])
+ tensor_list = list(tensor_list)
+ size_list = torch.cat(size_list)
+ buffer = [None] * world_size
+
+ dist.all_gather_object(buffer, size_list, group=group)
+ size_this_rank = []
+ for size_list in buffer:
+ size_this_rank.append(size_list[rank])
+
+ target_tensor_list = [
+ torch.empty(size.item(), dtype=torch.uint8, device=current_device)
+ for size in size_this_rank
+ ]
+ dist.all_to_all(target_tensor_list, tensor_list, group=group)
+
+ for i in range(len(target_tensor_list)):
+ obj_view = target_tensor_list[i].type(torch.uint8)
+ target_tensor_list[i] = _tensor_to_object(obj_view, size_this_rank[i],
+ group)
+
+ return target_tensor_list
+
+
+def barrier():
+ if not dist.is_available():
+ return
+
+ rank = dist.get_rank()
+ if rank == 0:
+ objects = [1]
+ else:
+ objects = [None]
+
+ dist.broadcast_object_list(objects, src=0)
+ return
diff --git a/xtuner/_lite/parallel/sampler.py b/xtuner/_lite/parallel/sampler.py
new file mode 100644
index 000000000..91b286f86
--- /dev/null
+++ b/xtuner/_lite/parallel/sampler.py
@@ -0,0 +1,398 @@
+# Copyright (c) OpenMMLab. All rights reserved.
+import math
+import random
+from typing import Iterator, Optional, Sized
+
+import torch
+from mmengine.dist import sync_random_seed
+from torch.distributed.device_mesh import DeviceMesh
+from torch.utils.data import ConcatDataset as TorchConcatDataset
+from torch.utils.data import Sampler
+
+
+class ParallelSampler(Sampler):
+ """The default data sampler for both distributed and non-distributed
+ environment.
+
+ It has several differences from the PyTorch ``DistributedSampler`` as
+ below:
+
+ 1. This sampler supports non-distributed environment.
+
+ 2. The round up behaviors are a little different.
+
+ - If ``round_up=True``, this sampler will add extra samples to make the
+ number of samples is evenly divisible by the world size. And
+ this behavior is the same as the ``DistributedSampler`` with
+ ``drop_last=False``.
+ - If ``round_up=False``, this sampler won't remove or add any samples
+ while the ``DistributedSampler`` with ``drop_last=True`` will remove
+ tail samples.
+
+ Args:
+ dataset (Sized): The dataset.
+ shuffle (bool): Whether shuffle the dataset or not. Defaults to True.
+ seed (int, optional): Random seed used to shuffle the sampler if
+ :attr:`shuffle=True`. This number should be identical across all
+ processes in the distributed group. Defaults to None.
+ round_up (bool): Whether to add extra samples to make the number of
+ samples evenly divisible by the world size. Defaults to True.
+ """
+
+ def __init__(
+ self,
+ dataset: Sized,
+ dp_mesh: DeviceMesh,
+ global_batch_size: int,
+ shuffle: bool = True,
+ seed: Optional[int] = None,
+ round_up: bool = True,
+ ) -> None:
+ rank = dp_mesh.get_local_rank()
+ world_size = dp_mesh.size()
+
+ assert global_batch_size % world_size == 0
+ self.global_batch_size = global_batch_size
+ self.rank = rank
+ self.world_size = world_size
+
+ self.dataset = dataset
+ self.shuffle = shuffle
+ if seed is None:
+ seed = sync_random_seed()
+ self.seed = seed
+ self.epoch = 0
+ self.step = 0
+ self.round_up = round_up
+
+ if self.round_up:
+ self.num_samples = math.ceil(
+ len(self.dataset) /
+ global_batch_size) * global_batch_size // world_size
+ self.total_size = self.num_samples * self.world_size
+ else:
+ self.num_samples = math.ceil(
+ (len(self.dataset) - rank) / world_size)
+ self.total_size = len(self.dataset)
+
+ def __iter__(self) -> Iterator[int]:
+ """Iterate the indices."""
+ # deterministically shuffle based on epoch and seed
+ if self.shuffle:
+ g = torch.Generator()
+ g.manual_seed(self.seed + self.epoch)
+ indices = torch.randperm(len(self.dataset), generator=g).tolist()
+ else:
+ indices = torch.arange(len(self.dataset)).tolist()
+
+ # add extra samples to make it evenly divisible
+ if self.round_up:
+ indices = (
+ indices *
+ int(self.total_size / len(indices) + 1))[:self.total_size]
+
+ # subsample
+ indices = indices[self.rank:self.total_size:self.world_size]
+
+ return iter(indices[self.step:])
+
+ def __len__(self) -> int:
+ """The number of samples in this rank."""
+ return self.num_samples - self.step
+
+ def set_epoch(self, epoch: int, step=0) -> None:
+ """Sets the epoch for this sampler.
+
+ When :attr:`shuffle=True`, this ensures all replicas use a different
+ random ordering for each epoch. Otherwise, the next iteration of this
+ sampler will yield the same ordering.
+
+ Args:
+ epoch (int): Epoch number.
+ """
+ self.epoch = epoch
+ self.step = step
+
+
+def get_length_grouped_indices(max_lengths,
+ group_batch_size,
+ dp_size,
+ seed=None):
+ if seed is not None:
+ torch.manual_seed(seed)
+ random.seed(seed)
+
+ assert all(leng != 0
+ for leng in max_lengths), 'Should not have zero length.'
+ indices = torch.randperm(len(max_lengths))
+ megabatches = [
+ indices[i:i + group_batch_size].tolist()
+ for i in range(0, len(max_lengths), group_batch_size)
+ ]
+ output = []
+ for megabatch in megabatches:
+ megabatch = sorted(
+ megabatch, key=lambda i: max_lengths[i], reverse=True)
+ grouped_megabatch = [
+ megabatch[i:i + dp_size] for i in range(0, len(megabatch), dp_size)
+ ]
+ random.shuffle(grouped_megabatch)
+ for group in grouped_megabatch:
+ output.extend(group)
+
+ return output
+
+
+class LengthGroupedSampler(Sampler):
+
+ def __init__(self,
+ dataset: Sized,
+ dp_mesh: DeviceMesh,
+ global_batch_size: int,
+ length_attr: str = 'longest',
+ mega_batch_mult: Optional[int] = None,
+ seed: Optional[int] = None,
+ round_up: bool = True) -> None:
+ rank = dp_mesh.get_local_rank()
+ world_size = dp_mesh.size()
+ self.rank = rank
+ self.world_size = world_size
+ assert global_batch_size % world_size == 0
+
+ self.dataset = dataset
+ if seed is None:
+ seed = sync_random_seed()
+ self.seed = seed
+ self.epoch = 0
+ self.step = 0
+ self.round_up = round_up
+
+ if self.round_up:
+ self.num_samples = math.ceil(
+ len(self.dataset) /
+ global_batch_size) * global_batch_size // world_size
+ self.total_size = self.num_samples * self.world_size
+ else:
+ self.num_samples = math.ceil(
+ (len(self.dataset) - rank) / world_size)
+ self.total_size = len(self.dataset)
+
+ if mega_batch_mult is None:
+ # Default for mega_batch_mult: 50 or the number to get 4
+ # megabatches, whichever is smaller.
+ mega_batch_mult = min(
+ len(self.dataset) // (global_batch_size * 4), 50)
+ # Just in case, for tiny datasets
+ if mega_batch_mult == 0:
+ mega_batch_mult = 1
+ self.group_batch_size = mega_batch_mult * global_batch_size
+
+ if isinstance(self.dataset, TorchConcatDataset):
+ max_lengths = []
+ for sub_dataset in self.dataset.datasets:
+ if hasattr(sub_dataset, length_attr):
+ max_lengths.extend(getattr(sub_dataset, length_attr))
+ else:
+ raise ValueError
+ self.max_lengths = max_lengths
+ else:
+ if hasattr(self.dataset, length_attr):
+ self.max_lengths = getattr(self.dataset, length_attr)
+ assert isinstance(self.max_lengths, (list, tuple))
+
+ self.global_batch_size = global_batch_size
+
+ def __iter__(self) -> Iterator[int]:
+ """Iterate the indices."""
+ generator = torch.Generator()
+ generator.manual_seed(self.seed + self.epoch)
+ seed = self.seed + self.epoch
+ indices = get_length_grouped_indices(
+ max_lengths=self.max_lengths,
+ group_batch_size=self.group_batch_size,
+ dp_size=self.world_size,
+ seed=seed)
+ assert len(set(indices)) == len(indices)
+ # add extra samples to make it evenly divisible
+ if self.round_up:
+ indices = (
+ indices *
+ int(self.total_size / len(indices) + 1))[:self.total_size]
+ # subsample
+ assert len(indices) == self.total_size
+ indices = indices[self.rank:self.total_size:self.world_size]
+ assert len(indices) == self.num_samples
+ return iter(indices[self.step:])
+
+ def __len__(self) -> int:
+ """The number of samples in this rank."""
+ return self.num_samples - self.step
+
+ def set_epoch(self, epoch: int, step=0) -> None:
+ """Sets the epoch for this sampler.
+
+ When :attr:`shuffle=True`, this ensures all replicas use a different
+ random ordering for each epoch. Otherwise, the next iteration of this
+ sampler will yield the same ordering.
+
+ Args:
+ epoch (int): Epoch number.
+ """
+ self.epoch = epoch
+ self.step = step
+
+
+def vlm_get_length_grouped_indices(max_lengths, group_batch_size, generator=None, **kwargs):
+
+ def process(lengths, group_batch_size, generator=None):
+ indices = torch.randperm(len(lengths), generator=generator)
+ megabatches = [
+ indices[i:i + group_batch_size].tolist()
+ for i in range(0, len(lengths), group_batch_size)
+ ]
+ megabatches = [
+ sorted(megabatch, key=lambda i: lengths[i], reverse=True)
+ for megabatch in megabatches
+ ]
+ return megabatches
+
+ lengths = max_lengths
+ assert all(leng != 0 for leng in lengths), 'Should not have zero length.'
+ if all(leng > 0 for leng in lengths) or all(leng < 0 for leng in lengths):
+ # all samples are in the same modality
+ megabatches = process(lengths, group_batch_size, generator=generator)
+ else:
+ mm_indices, mm_lengths = zip(*[(i, l) for i, l in enumerate(lengths)
+ if l > 0])
+ lang_indices, lang_lengths = zip(*[(i, -l)
+ for i, l in enumerate(lengths)
+ if l < 0])
+ mm_megabatches = []
+ for mm_megabatch in process(
+ mm_lengths, group_batch_size, generator=generator):
+ mm_megabatches.append([mm_indices[i] for i in mm_megabatch])
+ lang_megabatches = []
+ for lang_megabatch in process(
+ lang_lengths, group_batch_size, generator=generator):
+ lang_megabatches.append([lang_indices[i] for i in lang_megabatch])
+
+ last_mm = mm_megabatches[-1]
+ last_lang = lang_megabatches[-1]
+ last_batch = last_mm + last_lang
+ megabatches = mm_megabatches[:-1] + lang_megabatches[:-1]
+
+ megabatch_indices = torch.randperm(
+ len(megabatches), generator=generator)
+ megabatches = [megabatches[i] for i in megabatch_indices]
+
+ if len(last_batch) > 0:
+ megabatches.append(
+ sorted(
+ last_batch, key=lambda i: abs(lengths[i]), reverse=True))
+
+ # The rest is to get the biggest batch first.
+ # Since each megabatch is sorted by descending length,
+ # the longest element is the first
+ megabatch_maximums = [
+ abs(lengths[megabatch[0]]) for megabatch in megabatches
+ ]
+ max_idx = torch.argmax(torch.tensor(megabatch_maximums)).item()
+ # Switch to put the longest element in first position
+ megabatches[0][0], megabatches[max_idx][0] = megabatches[max_idx][
+ 0], megabatches[0][0]
+
+ return [i for megabatch in megabatches for i in megabatch]
+
+
+class VLMLengthGroupedSampler(Sampler):
+
+ def __init__(self,
+ dataset: Sized,
+ dp_mesh: DeviceMesh,
+ global_batch_size: int,
+ mega_batch_mult: Optional[int] = None,
+ seed: Optional[int] = None,
+ round_up: bool = True,
+ length_property='length') -> None:
+ rank = dp_mesh.get_local_rank()
+ world_size = dp_mesh.size()
+ self.rank = rank
+ self.world_size = world_size
+ assert global_batch_size % world_size == 0
+
+ self.dataset = dataset
+ if seed is None:
+ seed = sync_random_seed()
+ self.seed = seed
+ self.epoch = 0
+ self.step = 0
+ self.round_up = round_up
+
+ if self.round_up:
+ self.num_samples = math.ceil(
+ len(self.dataset) /
+ global_batch_size) * global_batch_size // world_size
+ self.total_size = self.num_samples * self.world_size
+ else:
+ self.num_samples = math.ceil(
+ (len(self.dataset) - rank) / world_size)
+ self.total_size = len(self.dataset)
+
+ if mega_batch_mult is None:
+ # Default for mega_batch_mult: 50 or the number to get 4
+ # megabatches, whichever is smaller.
+ mega_batch_mult = min(
+ len(self.dataset) // (global_batch_size * 4), 50)
+ # Just in case, for tiny datasets
+ if mega_batch_mult == 0:
+ mega_batch_mult = 1
+ self.group_batch_size = mega_batch_mult * global_batch_size
+
+ if isinstance(self.dataset, TorchConcatDataset):
+ max_lengths = []
+ for sub_dataset in self.dataset.datasets:
+ max_lengths.extend(getattr(sub_dataset, length_property))
+ self.max_lengths = max_lengths
+ else:
+ self.max_lengths = getattr(self.dataset, length_property)
+ assert isinstance(self.max_lengths, (list, tuple))
+
+ self.global_batch_size = global_batch_size
+
+ def __iter__(self) -> Iterator[int]:
+ """Iterate the indices."""
+ generator = torch.Generator()
+ generator.manual_seed(self.seed + self.epoch)
+ indices = vlm_get_length_grouped_indices(
+ max_lengths=self.max_lengths,
+ group_batch_size=self.group_batch_size,
+ dp_size=self.world_size,
+ generator=generator)
+ assert len(set(indices)) == len(indices)
+ # add extra samples to make it evenly divisible
+ if self.round_up:
+ indices = (
+ indices *
+ int(self.total_size / len(indices) + 1))[:self.total_size]
+ # subsample
+ assert len(indices) == self.total_size
+ indices = indices[self.rank:self.total_size:self.world_size]
+ assert len(indices) == self.num_samples
+ return iter(indices[self.step:])
+
+ def __len__(self) -> int:
+ """The number of samples in this rank."""
+ return self.num_samples - self.step
+
+ def set_epoch(self, epoch: int, step=0) -> None:
+ """Sets the epoch for this sampler.
+
+ When :attr:`shuffle=True`, this ensures all replicas use a different
+ random ordering for each epoch. Otherwise, the next iteration of this
+ sampler will yield the same ordering.
+
+ Args:
+ epoch (int): Epoch number.
+ """
+ self.epoch = epoch
+ self.step = step
\ No newline at end of file
diff --git a/xtuner/_lite/parallel/sequence/__init__.py b/xtuner/_lite/parallel/sequence/__init__.py
new file mode 100644
index 000000000..d0948e9ae
--- /dev/null
+++ b/xtuner/_lite/parallel/sequence/__init__.py
@@ -0,0 +1,15 @@
+# Copyright (c) OpenMMLab. All rights reserved.
+from mmengine.dist import init_dist
+
+from .attention import (post_process_for_sequence_parallel_attn,
+ pre_process_for_sequence_parallel_attn)
+from .ops import (gather_for_sequence_parallel, gather_forward_split_backward,
+ split_for_sequence_parallel, split_forward_gather_backward)
+
+
+__all__ = [
+ 'pre_process_for_sequence_parallel_attn',
+ 'post_process_for_sequence_parallel_attn', 'split_for_sequence_parallel',
+ 'init_dist', 'gather_for_sequence_parallel',
+ 'split_forward_gather_backward', 'gather_forward_split_backward',
+]
diff --git a/xtuner/_lite/parallel/sequence/attention.py b/xtuner/_lite/parallel/sequence/attention.py
new file mode 100644
index 000000000..176f801dc
--- /dev/null
+++ b/xtuner/_lite/parallel/sequence/attention.py
@@ -0,0 +1,42 @@
+# Copyright (c) OpenMMLab. All rights reserved.
+import torch.distributed as dist
+from torch.distributed.device_mesh import DeviceMesh
+import torch
+from ..comm import all_to_all
+
+
+
+def pre_process_for_sequence_parallel_attn(query_states: torch.Tensor,
+ key_states: torch.Tensor,
+ value_states: torch.Tensor,
+ sp_mesh: DeviceMesh,
+ scatter_dim: int=2,
+ gather_dim: int=1):
+ sp_size = sp_mesh.size()
+ n_head = query_states.shape[2]
+ assert n_head % sp_size == 0, \
+ ('The number of attention heads should be divisible by '
+ f'sequence_parallel_world_size. But got n_head = {n_head} and '
+ f'sequence_parallel_world_size = {sp_size}.')
+
+ # (b, s // sp_world_size, nd, dim) -> (b, s, nd // sp_world_size, dim)
+ sp_group = sp_mesh.get_group()
+ query_states = all_to_all(
+ query_states, sp_group, scatter_dim=scatter_dim, gather_dim=gather_dim)
+ key_states = all_to_all(
+ key_states, sp_group, scatter_dim=scatter_dim, gather_dim=gather_dim)
+ value_states = all_to_all(
+ value_states, sp_group, scatter_dim=scatter_dim, gather_dim=gather_dim)
+
+ return query_states, key_states, value_states
+
+
+def post_process_for_sequence_parallel_attn(attn_output: torch.Tensor,
+ sp_mesh: DeviceMesh,
+ scatter_dim=1,
+ gather_dim=2):
+ # (b, s, nd // sp_world_size, dim) -> (b, s // sp_world_size, nd, dim)
+ sp_group = sp_mesh.get_group()
+ output = all_to_all(
+ attn_output, sp_group, scatter_dim=scatter_dim, gather_dim=gather_dim)
+ return output
diff --git a/xtuner/_lite/parallel/sequence/ops.py b/xtuner/_lite/parallel/sequence/ops.py
new file mode 100644
index 000000000..fb0ba0d86
--- /dev/null
+++ b/xtuner/_lite/parallel/sequence/ops.py
@@ -0,0 +1,186 @@
+# Copyright (c) OpenMMLab. All rights reserved.
+import torch
+import torch.distributed as dist
+
+
+def split_for_sequence_parallel(input, dim: int, sp_mesh):
+ """Splits the input tensor along a given dimension for sequence parallel.
+
+ Args:
+ input: The input tensor to be split.
+ dim: The dimension along which the tensor should be split.
+ sp_group: The sequence parallel process group.
+
+ Returns:
+ The split tensor corresponding to the current rank's chunk.
+ """
+ sp_group = sp_mesh.get_group()
+ sp_size = sp_mesh.size()
+ if sp_size == 1:
+ return input
+
+ rank = dist.get_rank(sp_group)
+ dim_size = input.size(dim)
+ assert dim_size % sp_size == 0, (
+ f'The dimension to split ({dim_size}) is not a multiple of '
+ f'sp size ({sp_size}), cannot split tensor evenly')
+
+ tensor_list = torch.split(input, dim_size // sp_size, dim=dim)
+ output = tensor_list[rank].contiguous()
+
+ return output
+
+
+def gather_for_sequence_parallel(input, dim: int, sp_group: dist.ProcessGroup):
+ """Gathers the input tensor along a given dimension for sequence parallel.
+
+ Args:
+ input: The input tensor to be gathered.
+ dim: The dimension along which the tensor should be gathered.
+ sp_group: The sequence parallel process group.
+
+ Returns:
+ The gathered tensor concatenated along the specified dimension.
+ """
+ input = input.contiguous()
+ world_size = dist.get_world_size(sp_group)
+ dist.get_rank(sp_group)
+
+ if world_size == 1:
+ return input
+
+ tensor_list = [torch.empty_like(input) for _ in range(world_size)]
+ assert input.device.type == 'cuda'
+ dist.all_gather(tensor_list, input, group=sp_group)
+
+ output = torch.cat(tensor_list, dim=dim).contiguous()
+
+ return output
+
+
+class _GatherForwardSplitBackward(torch.autograd.Function):
+ """Gather the input during forward.
+
+ Scale and split the grad and keep only the corresponding chuck to the rank
+ during backward.
+ """
+
+ @staticmethod
+ def forward(ctx, input, dim, sp_group, grad_scale):
+ ctx.dim = dim
+ ctx.sp_group = sp_group
+ ctx.grad_scale = grad_scale
+ return gather_for_sequence_parallel(input, dim, sp_group)
+
+ @staticmethod
+ def backward(ctx, grad_output):
+ if ctx.grad_scale == 'up':
+ grad_output = grad_output * dist.get_world_size(ctx.sp_group)
+ elif ctx.grad_scale == 'down':
+ grad_output = grad_output / dist.get_world_size(ctx.sp_group)
+
+ return (split_for_sequence_parallel(grad_output, ctx.dim,
+ ctx.sp_group), None, None, None)
+
+
+class _SplitForwardGatherBackward(torch.autograd.Function):
+ """Split the input and keep only the corresponding chuck to the rank during
+ forward.
+
+ Scale and gather the grad during backward.
+ """
+
+ @staticmethod
+ def forward(ctx, input, dim, sp_group, grad_scale):
+ ctx.dim = dim
+ ctx.sp_group = sp_group
+ ctx.grad_scale = grad_scale
+ return split_for_sequence_parallel(input, dim, sp_group)
+
+ @staticmethod
+ def backward(ctx, grad_output):
+ if ctx.grad_scale == 'up':
+ grad_output = grad_output * dist.get_world_size(ctx.sp_group)
+ elif ctx.grad_scale == 'down':
+ grad_output = grad_output / dist.get_world_size(ctx.sp_group)
+ return (gather_for_sequence_parallel(grad_output, ctx.dim,
+ ctx.sp_group), None, None, None)
+
+
+def split_forward_gather_backward(input, dim, sp_group, grad_scale=None):
+ """Split tensors according to the sp rank during forward propagation and
+ gather the grad from the whole sp group during backward propagation.
+
+ 1. When do we need this? input.requires_grad = True
+
+ 2. Why we need grad scale?
+
+ We have to scale down the grads as `gather_forward_split_backward` scales
+ up the grads.
+ """
+ return _SplitForwardGatherBackward.apply(input, dim, sp_group, grad_scale)
+
+
+def gather_forward_split_backward(input, dim, sp_group, grad_scale=None):
+ """Gather tensors from the whole sp group during forward propagation and
+ split the grad according to the sp rank during backward propagation.
+
+ 1. When do we need this?
+
+ When sp is greater than 1, we need to slice the input `x` along
+ sequence length dimension before it is passed into the model and get
+ `sub_seq_x`. We then pass `sub_seq_x` into model and get output
+ `sub_seq_out`. If the loss calculation process needs to use the complete
+ output, we have to gather the `sub_seq_out` in all sp ranks during forward
+ propagation and split the grad during backward propagation.
+
+ 2. Why we need grad scale?
+ Here is a simple case.
+
+ -------- SP 1 -----------
+ Suppose here is a toy model with only one linear module
+ (in_features = 2, out_features = 1) and the input x has shape(2, 2).
+ Y = [[y1], = [[w11x11 + w21x12], = [[x11, x12], dot [[w11],
+ [y2]] [w11x21 + w21x22]] [x21, x22]] [w21]]
+ z = mean(Y) = (y1 + y2) / 2
+ Here is the partial derivative of z with respect to w11:
+ ∂z / ∂w11 = ∂z / ∂y1 * ∂y1 / ∂w11 + ∂z / ∂y2 * ∂y2 / ∂w11
+ = 1/2 * x11 + 1/2 * x21 = (x11 + x21) / 2
+
+ -------- SP 2 -----------
+ When sequence parallel world size is set to 2, we will split the input x
+ and scatter them to the two rank in the same sequence parallel group.
+ ```Step 1
+ Y_rank0 = [[y1]] = [[w11x11 + w21x12]] = [[x11, x12]] dot [[w11, w21]]^T
+ Y_rank1 = [[y2]] = [[w11x21 + w21x22]] = [[x21, x22]] dot [[w11, w21]]^T
+ ```
+
+ Then, we have to gather them:
+ ```Step 2
+ Y_rank0 = [[y1],
+ detach([y2])]
+ Y_rank1 = [detach([y1]),
+ [y2]]
+ ```
+ Note that y2 in Y_rank0 does not have grad, neither does y1 in Y_rank1.
+
+ Similarly, we calculate the loss in each rank:
+ ```Step 3
+ z_rank0 = mean(Y_rank0) = (y1 + detach(y2)) / 2
+ z_rank1 = mean(Y_rank1) = (detach(y1) + y2) / 2
+ ```
+ So the partial derivative of loss_rank0 with respect to w11:
+ ```∂z / ∂w11 = ∂z / ∂y1 * ∂y1 / ∂w11 = x11 / 2```
+ The same for rank1:
+ ```∂z / ∂w11 = ∂z / ∂y2 * ∂y2 / ∂w11 = x21 / 2```
+
+ Finally, we need to all_reduce them:
+ ```Step 4
+ In both rank:
+ ∂z / ∂w11 = (x11 / 2 + x21 / 2) / 2 = (x11 + x21) / 4
+ ```
+
+ In SP2, the gradient of each param is only half of that in SP1.
+ So we should scale up the grad during the backward process in Step 2.
+ """ # noqa: E501
+ return _GatherForwardSplitBackward.apply(input, dim, sp_group, grad_scale)
diff --git a/xtuner/_lite/parallel/setup.py b/xtuner/_lite/parallel/setup.py
new file mode 100644
index 000000000..bafe3fb19
--- /dev/null
+++ b/xtuner/_lite/parallel/setup.py
@@ -0,0 +1,43 @@
+import torch.distributed as dist
+from mmengine.dist import infer_launcher, init_dist
+
+from xtuner._lite import get_device
+
+import torch
+from torch._C._distributed_c10d import ReduceOp
+from torch.distributed.c10d_logger import _exception_logger
+
+origin_reduce_scatter_tensor = torch.distributed.reduce_scatter_tensor
+
+
+# mlu's reduce_scatter_tensor do not support ReduceOp.AVG, use ReduceOp.SUM / group_world_size instead.
+@_exception_logger
+def mlu_reduce_scatter_tensor(output,
+ input,
+ op=ReduceOp.SUM,
+ group=None,
+ async_op=False):
+ if op == ReduceOp.AVG:
+ result = origin_reduce_scatter_tensor(output, input, ReduceOp.SUM,
+ group, async_op)
+ output.div_(torch.distributed.get_world_size(group))
+ return result
+ else:
+ return origin_reduce_scatter_tensor(output, input, op, group, async_op)
+
+
+def setup_parallel():
+
+ if not dist.is_initialized():
+ dist_launcher = infer_launcher()
+ init_dist(dist_launcher)
+
+ device = get_device()
+
+ if device == 'mlu':
+ torch.distributed.reduce_scatter_tensor = mlu_reduce_scatter_tensor
+
+
+
+
+
\ No newline at end of file
diff --git a/xtuner/_lite/patches/__init__.py b/xtuner/_lite/patches/__init__.py
new file mode 100644
index 000000000..15b0c2a8e
--- /dev/null
+++ b/xtuner/_lite/patches/__init__.py
@@ -0,0 +1,4 @@
+from .llama import CUDAPatchedLlamaForCausalLM
+from .base import FSDPConfig
+from .auto import AutoPatch
+from .utils import pad_to_multiple_of, pad_to_max_length
\ No newline at end of file
diff --git a/xtuner/_lite/patches/auto.py b/xtuner/_lite/patches/auto.py
new file mode 100644
index 000000000..f37825627
--- /dev/null
+++ b/xtuner/_lite/patches/auto.py
@@ -0,0 +1,43 @@
+from .base import PatchedCausalLM, FSDPConfig
+from .llama import (CUDAPatchedLlamaForCausalLM,
+ MLUPatchedLlamaForCausalLM,
+ MuxiPatchedLlamaForCausalLM)
+from .internlm3 import (CUDAPatchedInternLM3ForCausalLM,
+ MLUPatchedInternLM3ForCausalLM,
+ MuxiPatchedInternLM3ForCausalLM)
+from xtuner._lite.modelings.internlm3 import InternLM3ForCausalLM
+from transformers.models.llama import LlamaForCausalLM
+from transformers.models.qwen2 import Qwen2ForCausalLM
+
+CUDA_PATCH_MAP = {
+ LlamaForCausalLM: CUDAPatchedLlamaForCausalLM,
+ InternLM3ForCausalLM: CUDAPatchedInternLM3ForCausalLM,
+}
+
+MLU_PATCH_MAP = {
+ LlamaForCausalLM: MLUPatchedLlamaForCausalLM,
+ InternLM3ForCausalLM: MLUPatchedInternLM3ForCausalLM,
+}
+
+MUXI_PATCH_MAP = {
+ LlamaForCausalLM: MuxiPatchedLlamaForCausalLM,
+ InternLM3ForCausalLM: MuxiPatchedInternLM3ForCausalLM,
+}
+
+class AutoPatch:
+
+ @classmethod
+ def from_causal_lm(cls, model, fsdp_config: FSDPConfig, device_type='cuda') -> PatchedCausalLM:
+
+ if device_type == 'cuda':
+ patch_cls = CUDA_PATCH_MAP[type(model)]
+ elif device_type == 'mlu':
+ patch_cls = MLU_PATCH_MAP[type(model)]
+ elif device_type == 'muxi':
+ patch_cls = MUXI_PATCH_MAP[type(model)]
+ else:
+ raise NotImplementedError
+
+ patched_model = patch_cls(model, fsdp_config)
+
+ return patched_model
\ No newline at end of file
diff --git a/xtuner/_lite/patches/base.py b/xtuner/_lite/patches/base.py
new file mode 100644
index 000000000..a966b8936
--- /dev/null
+++ b/xtuner/_lite/patches/base.py
@@ -0,0 +1,426 @@
+from abc import abstractmethod, ABC
+from typing import Dict, List
+from transformers import PreTrainedModel
+
+import torch
+from torch import nn
+from torch import distributed as dist
+from pydantic import BaseModel
+
+import os
+import json
+from typing import Literal, Optional, Union, Callable
+from safetensors import safe_open
+import torch
+from accelerate.utils import set_module_tensor_to_device
+from dataclasses import dataclass
+
+from torch.nn.utils.clip_grad import _no_grad
+import torch
+from typing import List, Optional, Tuple, Union, Dict
+from torch import Tensor
+from torch import distributed as dist
+from torch.utils._foreach_utils import (
+ _device_has_foreach_support,
+ _group_tensors_by_device_and_dtype,
+ _has_foreach_support,
+)
+
+from xtuner._lite import get_torch_device_module
+
+DEVICE_MODULE = get_torch_device_module()
+
+
+
+
+@_no_grad
+def clip_grad_norm_(
+ parameters,
+ fsdp_mesh,
+ max_norm: float,
+ norm_type: float = 2.0,
+ error_if_nonfinite: bool = False,
+ foreach= None,
+) -> torch.Tensor:
+ if isinstance(parameters, torch.Tensor):
+ parameters = [parameters]
+ grads = [p.grad for p in parameters if p.grad is not None]
+ max_norm = float(max_norm)
+ norm_type = float(norm_type)
+ if len(grads) == 0:
+ return torch.tensor(0.0)
+ first_device = grads[0].device
+
+ grouped_grads: Dict[
+ Tuple[torch.device, torch.dtype], Tuple[List[List[Tensor]], List[int]]
+ ] = _group_tensors_by_device_and_dtype(
+ [grads]
+ ) # type: ignore[assignment]
+
+ norms: List[Tensor] = []
+ for (device, _), ([device_grads], _) in grouped_grads.items(): # type: ignore[assignment]
+ if (foreach is None and _has_foreach_support(device_grads, device)) or (
+ foreach and _device_has_foreach_support(device)
+ ):
+ # for grouped_device_grads in group_tensors_by_device_mesh(device_grads).values():
+ norms.extend(torch._foreach_norm(device_grads, norm_type))
+ elif foreach:
+ raise RuntimeError(
+ f"foreach=True was passed, but can't use the foreach API on {device.type} tensors"
+ )
+ else:
+ norms.extend([torch.linalg.vector_norm(g, norm_type) for g in device_grads])
+
+ local_sharded_norm = torch.linalg.vector_norm(
+ torch.stack([norm.to_local().to(first_device) for norm in norms]), norm_type, dtype=torch.float32
+ )
+
+ if norm_type == 2:
+ total_norm = local_sharded_norm**norm_type
+ dist.all_reduce(total_norm, group=fsdp_mesh.get_group(mesh_dim=0))
+ total_norm = total_norm ** (1 / norm_type)
+ else:
+ raise NotImplementedError
+
+ if error_if_nonfinite and torch.logical_or(total_norm.isnan(), total_norm.isinf()):
+ raise RuntimeError(
+ f"The total norm of order {norm_type} for gradients from "
+ "`parameters` is non-finite, so it cannot be clipped. To disable "
+ "this error and scale the gradients by the non-finite norm anyway, "
+ "set `error_if_nonfinite=False`"
+ )
+ clip_coef = max_norm / (total_norm + 1e-6)
+ # Note: multiplying by the clamped coef is redundant when the coef is clamped to 1, but doing so
+ # avoids a `if clip_coef < 1:` conditional which can require a CPU <=> device synchronization
+ # when the gradients do not reside in CPU memory.
+ clip_coef_clamped = torch.clamp(clip_coef, max=1.0)
+ for (device, _), ([device_grads], _) in grouped_grads.items(): # type: ignore[assignment]
+ if (foreach is None and _has_foreach_support(device_grads, device)) or (
+ foreach and _device_has_foreach_support(device)
+ ):
+ torch._foreach_mul_(device_grads, clip_coef_clamped.to(device))
+ elif foreach:
+ raise RuntimeError(
+ f"foreach=True was passed, but can't use the foreach API on {device.type} tensors"
+ )
+ else:
+ clip_coef_clamped_device = clip_coef_clamped.to(device)
+ for g in device_grads:
+ g.mul_(clip_coef_clamped_device.to(g.dtype))
+
+ return total_norm
+
+
+def download_model_from_hub(
+ model_name_or_path: str,
+ from_hub: Literal['huggingface', 'modelscope'] = 'huggingface',
+ cache_dir: Optional[str] = None,
+) -> str:
+ """Automatically download model from the HUB.
+
+ Note:
+ If `model_name_or_path` is a local path, it will return the path
+ directly without downloading it again.
+
+ Args:
+ model_name_or_path (str): The model name, model path or repo id.
+ config (str | None): The config path. Default is None.
+ from_hub (str): The model hosting hub, modelscope, or huggingface.
+ Default is huggingface.
+ cache_dir (str | None):
+ The save path when downloading the model. If it is None, it
+ will be stored in the default location of the HUB. For
+ Huggingface, it's ~/.cache/huggingface/hub, for ModelScope,
+ it's ~/.cache/modelscope/hub.
+ Returns:
+ str: The local path of the model.
+ """
+ if os.path.isdir(model_name_or_path):
+ model_path = model_name_or_path
+ elif from_hub == 'huggingface':
+ from huggingface_hub import snapshot_download
+ model_path = snapshot_download(
+ repo_id=model_name_or_path, cache_dir=cache_dir)
+ elif from_hub == 'modelscope':
+ from modelscope import snapshot_download
+ model_path = snapshot_download(
+ model_id=model_name_or_path, cache_dir=cache_dir)
+ else:
+ # TODO support openxlab
+ raise NotImplementedError('The model does not support downloading '
+ f'from {from_hub}, it only supports '
+ '`huggingface` and `modelscope`.')
+
+ return model_path
+
+
+
+class HFCheckpointLoader():
+
+ def __init__(self, model_path, cache_dir=None, from_hub='huggingface'):
+
+ self.model_path = download_model_from_hub(model_path, from_hub, cache_dir)
+
+ if 'model.safetensors.index.json' in os.listdir(self.model_path):
+ index_json = os.path.join(self.model_path, 'model.safetensors.index.json')
+ self.use_safetensors = True
+ elif 'model.bin.index.json' in os.listdir(self.model_path):
+ index_json = os.path.join(self.model_path, 'model.bin.index.json')
+ self.use_safetensors = False
+ else:
+ raise FileNotFoundError
+
+ with open(index_json, 'r') as f:
+ self.weight_map = json.load(f)['weight_map']
+
+ self.current_file = None
+ self.buffer = None
+
+
+ def load(self, key):
+
+ _file = self.weight_map[key]
+
+ if self.use_safetensors:
+
+ if self.current_file is None:
+ self.buffer = safe_open(os.path.join(self.model_path, _file), framework="pt")
+ self.current_file = _file
+
+ if _file != self.current_file:
+ self.buffer = safe_open(os.path.join(self.model_path, _file), framework="pt")
+ self.current_file = _file
+ weight = self.buffer.get_tensor(key)
+
+ else:
+
+ if self.current_file is None:
+ self.buffer = torch.load(os.path.join(self.model_path, _file))
+ self.current_file = _file
+
+ if _file != self.current_file:
+ self.buffer = torch.load(os.path.join(self.model_path, _file))
+
+ weight = self.buffer[key]
+
+ return weight
+
+@torch.no_grad
+def lazy_init_fn(module, module2name, checkpoint_loader):
+ device = DEVICE_MODULE.current_device()
+
+ module_name = module2name[module]
+
+ params = {
+ name: checkpoint_loader.load(f'{module_name}.{name}')
+ for name, _ in module.named_parameters(recurse=False)
+ }
+
+ buffers = {
+ name: checkpoint_loader.load(f'{module_name}.{name}')
+ for name, _ in module.named_buffers(recurse=False) if name in checkpoint_loader.weight_map
+ }
+
+ module.to_empty(device=DEVICE_MODULE.current_device(), recurse=False)
+
+ for name, param in module.named_parameters(recurse=False):
+ dtype = param.dtype
+ _param = params[name].to(device).to(dtype)
+
+ param.data.copy_(_param)
+
+ for name, buffer in module.named_buffers(recurse=False):
+ if name in buffers:
+ _buffer = buffers[name].to(device).to(buffer.dtype)
+ buffer.data.copy_(_buffer)
+
+@dataclass
+class FSDPConfig:
+ tp_size: int = 1
+ sp_size: int = 1
+ ep_size: int = 1
+ reshard_after_forward: bool = True
+ recompute_ratio: float = 1.0
+ cpu_offload: bool = True
+ param_dtype: torch.dtype = torch.bfloat16
+ reduce_dtype: torch.dtype = torch.bfloat16
+ torch_compile: torch.dtype = False
+ max_length: Optional[int] = None
+ mesh_prefix: str = 'default'
+
+
+@dataclass
+class ModelConfig:
+ num_hidden_layers: int
+ num_attention_heads: int
+ num_key_value_heads: int
+ head_dim: int
+ hidden_size: int
+ intermediate_size: int
+ vocab_size: int
+
+
+
+class PatchedCausalLM(ABC, nn.Module):
+
+ def __init__(self, model: PreTrainedModel, fsdp_config: FSDPConfig):
+ super().__init__()
+
+ @property
+ @abstractmethod
+ def rank0_model(self) -> Optional[PreTrainedModel]:
+ pass
+
+ @property
+ @abstractmethod
+ def patched_model(self) -> PreTrainedModel:
+ pass
+
+ @property
+ @abstractmethod
+ def fsdp_config(self) -> FSDPConfig:
+ pass
+
+ @property
+ @abstractmethod
+ def model_config(self) -> ModelConfig:
+ pass
+
+ @property
+ @abstractmethod
+ def data_parallel_mesh(self):
+ pass
+
+ @property
+ @abstractmethod
+ def data_mesh(self):
+ pass
+
+ @property
+ @abstractmethod
+ def sequence_parallel_mesh(self):
+ pass
+
+ @abstractmethod
+ def dispatch_hf_code(self, model) -> PreTrainedModel:
+ pass
+
+ @abstractmethod
+ def fully_shard(self, parallel_config: FSDPConfig):
+ pass
+
+ @abstractmethod
+ def trainable_parameters(self) -> List[Dict[str, List[nn.Parameter]]] :
+ pass
+
+ @abstractmethod
+ def clip_grad_norm(self, max_norm: float) -> torch.Tensor:
+ pass
+
+ def save_pretrained(
+ self,
+ save_directory: Union[str, os.PathLike],
+ is_main_process: bool = True,
+ state_dict: Optional[dict] = None,
+ save_function: Callable = torch.save,
+ push_to_hub: bool = False,
+ max_shard_size: Union[int, str] = "5GB",
+ safe_serialization: bool = True,
+ variant: Optional[str] = None,
+ token: Optional[Union[str, bool]] = None,
+ save_peft_format: bool = True,
+ **kwargs,
+ ):
+ if dist.is_initialized() and dist.is_available():
+ rank = dist.get_rank()
+ else:
+ rank = 0
+
+ from torch.distributed._tensor import DTensor
+
+ for name, param in self.patched_model.state_dict().items():
+ if self.fsdp_config.torch_compile and '_orig_mod.' in name:
+ name = name.replace('_orig_mod.', '')
+ if isinstance(param, DTensor):
+ full_param = param.full_tensor().cpu()
+ else:
+ full_param = param.cpu()
+
+ if rank == 0:
+ set_module_tensor_to_device(self.rank0_model, name, 'cpu', full_param)
+
+ if rank == 0:
+ self.rank0_model.save_pretrained(
+ save_directory,
+ is_main_process,
+ state_dict,
+ save_function,
+ push_to_hub,
+ max_shard_size,
+ safe_serialization,
+ variant,
+ token,
+ save_peft_format,
+ **kwargs,
+ )
+
+ # def save_checkpoint(self,
+ # optimizer: Optional[torch.optim.Optimizer] = None):
+
+ # # FSDP cannot be saved via torch.save
+ # # Refer to https://pytorch.org/tutorials/recipes/distributed_checkpoint_recipe.html # noqa: E501
+ # _options = StateDictOptions(
+ # cpu_offload=True, ignore_frozen_params=True)
+ # (shard_model_state_dict,
+ # shard_optimizer_state_dict) = get_state_dict(
+ # llm, optimizer, options=_options)
+
+ # state_dict = {
+ # 'model': shard_model_state_dict,
+ # 'optimizer': shard_optimizer_state_dict,
+ # 'train_state': train_state.state_dict(),
+ # 'warmup_scheduler': warmup_scheduler.state_dict(),
+ # 'cosine_scheduler': cosine_scheduler.state_dict()
+ # }
+
+ # mkdir_or_exist(ckpt_dir)
+ # ckpt_handle = dcp.async_save(state_dict, checkpoint_id=ckpt_dir, process_group=gloo_group)
+
+
+ # def load_checkpoint(self,
+ # checkpoint_id: str,
+ # optimizer: Optional[torch.optim.Optimizer] = None ):
+ # _options = StateDictOptions(
+ # cpu_offload=True, ignore_frozen_params=True)
+ # (shard_model_state_dict,
+ # shard_optimizer_state_dict) = get_state_dict(
+ # patched_llm.patched_model, optimizer, options=_options)
+ # state_dict = {
+ # 'model': shard_model_state_dict,
+ # 'optimizer': shard_optimizer_state_dict,
+ # 'train_state': train_state,
+ # 'warmup_scheduler': warmup_scheduler,
+ # 'cosine_scheduler': cosine_scheduler
+ # }
+
+ # # inplace state_dict
+ # dcp.load(
+ # state_dict=state_dict,
+ # checkpoint_id=latest_checkpoint,
+ # )
+
+ # _options = StateDictOptions(
+ # cpu_offload=True, strict=False)
+ # set_state_dict(
+ # patched_llm.patched_model,
+ # optimizer,
+ # model_state_dict=state_dict["model"],
+ # optim_state_dict=state_dict["optimizer"],
+ # options=_options
+ # )
+
+
+
+
+
+
diff --git a/xtuner/_lite/patches/internlm3.py b/xtuner/_lite/patches/internlm3.py
new file mode 100644
index 000000000..a36fcbb5f
--- /dev/null
+++ b/xtuner/_lite/patches/internlm3.py
@@ -0,0 +1,28 @@
+from xtuner._lite.chat import HybridChatTemplate
+from xtuner._lite.modelings.internlm3.modeling_internlm3 import InternLM3ForCausalLM, InternLM3Attention
+import types
+from .llama import CUDAPatchedLlamaForCausalLM, cuda_patched_casual_forward, cuda_patched_llama_attn_training
+
+class CUDAPatchedInternLM3ForCausalLM(CUDAPatchedLlamaForCausalLM):
+ chat_template = HybridChatTemplate(
+ system='<|im_start|>system\n{system}<|im_end|>\n',
+ user='<|im_start|>user\n{user}<|im_end|>\n<|im_start|>assistant\n',
+ assistant='{assistant}<|im_end|>',
+ stop_words=['<|im_end|>'])
+
+ @staticmethod
+ def dispatch_hf_code(model) -> InternLM3ForCausalLM:
+
+ for name, module in model.named_modules():
+ if isinstance(module, InternLM3Attention):
+ module.forward = types.MethodType(cuda_patched_llama_attn_training, module)
+ if isinstance(module, InternLM3ForCausalLM):
+ module.forward = types.MethodType(cuda_patched_casual_forward, module)
+
+ return model
+
+class MLUPatchedInternLM3ForCausalLM(CUDAPatchedInternLM3ForCausalLM):
+ device_type = 'mlu'
+
+class MuxiPatchedInternLM3ForCausalLM(CUDAPatchedInternLM3ForCausalLM):
+ device_type = 'muxi'
\ No newline at end of file
diff --git a/xtuner/_lite/patches/llama.py b/xtuner/_lite/patches/llama.py
new file mode 100644
index 000000000..796459dd8
--- /dev/null
+++ b/xtuner/_lite/patches/llama.py
@@ -0,0 +1,824 @@
+from xtuner._lite.patches.base import PatchedCausalLM, FSDPConfig, ModelConfig, HFCheckpointLoader, lazy_init_fn, clip_grad_norm_
+from xtuner._lite.patches.utils import pad_to_multiple_of, pad_to_max_length
+from typing import Callable, Optional, Tuple, TypedDict, Union, List
+import types
+import copy
+from packaging import version
+from tqdm import tqdm
+from functools import partial
+from transformers import PreTrainedModel
+from transformers.cache_utils import Cache
+from transformers.modeling_flash_attention_utils import FlashAttentionKwargs
+from transformers.processing_utils import Unpack
+from transformers.modeling_utils import ALL_ATTENTION_FUNCTIONS
+from transformers.models.llama.modeling_llama import LlamaAttention, LlamaRotaryEmbedding, LlamaForCausalLM, apply_rotary_pos_emb, eager_attention_forward, repeat_kv
+import torch
+from torch import nn
+from torch import distributed as dist
+from torch.distributed.algorithms._checkpoint.checkpoint_wrapper import (
+ checkpoint_wrapper
+)
+from torch.distributed.device_mesh import DeviceMesh
+from torch.distributed.device_mesh import init_device_mesh
+from torch.distributed._composable import checkpoint
+from torch.distributed._composable.fsdp import fully_shard, CPUOffloadPolicy, MixedPrecisionPolicy
+from transformers.utils import logging
+from transformers.modeling_outputs import CausalLMOutputWithPast
+from xtuner._lite.accelerate import liger_kernel_is_available
+from xtuner._lite.chat import HybridChatTemplate
+
+from xtuner._lite.parallel.sequence import (
+ pre_process_for_sequence_parallel_attn, post_process_for_sequence_parallel_attn,
+ split_for_sequence_parallel)
+from torch.distributed._tensor import Shard, Replicate, distribute_tensor, DTensor
+from torch.distributed.tensor.parallel import (
+ parallelize_module,
+ ColwiseParallel,
+ RowwiseParallel,
+ PrepareModuleInput,
+ SequenceParallel
+)
+logger = logging.get_logger(__name__)
+
+
+def cuda_patched_llama_attn_training(
+ self: LlamaAttention,
+ hidden_states: torch.Tensor,
+ position_embeddings: Tuple[torch.Tensor, torch.Tensor],
+ attention_mask: Optional[torch.Tensor],
+ past_key_value: Optional[Cache] = None,
+ cache_position: Optional[torch.LongTensor] = None,
+ sequence_parallel_mesh: Optional[DeviceMesh] = None,
+ **kwargs: Unpack[FlashAttentionKwargs],
+) -> Tuple[torch.Tensor, Optional[torch.Tensor], Optional[Tuple[torch.Tensor]]]:
+ input_shape = hidden_states.shape[:-1]
+ hidden_shape = (*input_shape, -1, self.head_dim)
+
+ query_states = self.q_proj(hidden_states).view(hidden_shape).transpose(1, 2)
+ key_states = self.k_proj(hidden_states).view(hidden_shape).transpose(1, 2)
+ value_states = self.v_proj(hidden_states).view(hidden_shape).transpose(1, 2)
+
+ cos, sin = position_embeddings
+ query_states, key_states = apply_rotary_pos_emb(query_states, key_states, cos, sin)
+ if past_key_value is not None:
+ # sin and cos are specific to RoPE models; cache_position needed for the static cache
+ cache_kwargs = {"sin": sin, "cos": cos, "cache_position": cache_position}
+ key_states, value_states = past_key_value.update(key_states, value_states, self.layer_idx, cache_kwargs)
+
+ attention_interface: Callable = eager_attention_forward
+ if self.config._attn_implementation != "eager":
+ if self.config._attn_implementation == "sdpa" and kwargs.get("output_attentions", False):
+ logger.warning_once(
+ "`torch.nn.functional.scaled_dot_product_attention` does not support `output_attentions=True`. Falling back to "
+ 'eager attention. This warning can be removed using the argument `attn_implementation="eager"` when loading the model.'
+ )
+ else:
+ attention_interface = ALL_ATTENTION_FUNCTIONS[self.config._attn_implementation]
+
+ if sequence_parallel_mesh and sequence_parallel_mesh.size() > 1:
+
+
+ # bs, qh, n_div_sp, d = query_states.shape
+ # _, kvh, n_div_sp, d = key_states.shape
+
+ # assert bs == 1
+ # sp = sequence_parallel_mesh.size()
+ # n = n_div_sp * sp
+ # # (b, n // sp, qh, d)
+ # query_states = query_states.transpose(1,2)
+ # key_states = key_states.transpose(1,2)
+ # value_states = value_states.transpose(1,2)
+
+ # # (qh, b * n // sp, d)
+ # query_states = query_states.flatten(0, 1).transpose(0,1).contiguous()
+ # key_states = key_states.flatten(0, 1).transpose(0,1).contiguous()
+ # value_states = value_states.flatten(0, 1).transpose(0,1).contiguous()
+
+ # # (qh, b * n // sp, d)
+ # _query_states = query_states.new_empty(qh, bs * n // sp, d)
+ # # (kvh, b * n // sp, d)
+ # _key_states = key_states.new_empty(kvh, bs * n // sp, d)
+ # _value_states = value_states.new_empty(kvh, bs * n // sp, d)
+
+ # # (qh, b * n // sp, d)
+ # _query_states = dist.nn.all_to_all_single(
+ # _query_states, query_states, group=sequence_parallel_mesh.get_group())
+ # # (kvh, b * n // sp, d)
+ # _key_states = dist.nn.all_to_all_single(
+ # _key_states, key_states, group=sequence_parallel_mesh.get_group())
+ # # (kvh, b * n // sp, d)
+ # _value_states = dist.nn.all_to_all_single(
+ # _value_states, value_states, group=sequence_parallel_mesh.get_group())
+
+ # # (sp, qh // sp, b*n // sp, d)
+ # _query_states = _query_states.view(sp, qh // sp, bs* n // sp, d)
+ # # (sp, kvh // sp, b*n // sp, d)
+ # _key_states = _key_states.view(sp, kvh // sp, bs * n // sp, d)
+ # _value_states = _value_states.view(sp, kvh // sp, bs * n // sp, d)
+
+ # query_states = _query_states.transpose(1,2).reshape(bs, n, qh // sp, d).transpose(1,2)
+ # key_states = _key_states.transpose(1,2).reshape(bs, n, kvh // sp, d).transpose(1,2)
+ # value_states = _value_states.transpose(1,2).reshape(bs, n, kvh // sp, d).transpose(1,2)
+
+ # different from LlamaAttention.forward
+ key_states = repeat_kv(key_states, self.num_key_value_groups)
+ value_states = repeat_kv(value_states, self.num_key_value_groups)
+
+ query_states = query_states.transpose(1,2)
+ key_states = key_states.transpose(1,2)
+ value_states = value_states.transpose(1,2)
+
+ query_states, key_states, value_states = pre_process_for_sequence_parallel_attn(
+ query_states, key_states, value_states, sequence_parallel_mesh
+ )
+
+ query_states = query_states.transpose(1,2)
+ key_states = key_states.transpose(1,2)
+ value_states = value_states.transpose(1,2)
+
+
+
+ # (bs, n , qh // sp, d)
+ attn_output, attn_weights = attention_interface(
+ self,
+ query_states,
+ key_states,
+ value_states,
+ attention_mask,
+ dropout=0.0 if not self.training else self.attention_dropout,
+ scaling=self.scaling,
+ **kwargs,
+ )
+
+ if sequence_parallel_mesh and sequence_parallel_mesh.size() > 1:
+ # # (bs * n , qh // sp, d)
+ # attn_output = attn_output.flatten(0, 1).contiguous()
+ # # (bs * n, qh // sp, d)
+ # _attn_output = attn_output.new_empty(bs * n, qh // sp, d)
+
+ # # (bs * n, qh // sp, d)
+ # attn_output = dist.nn.all_to_all_single(
+ # _attn_output, attn_output, group=sequence_parallel_mesh.get_group())
+
+ # # (sp, bs * n // sp, qh // sp, d)
+ # attn_output = attn_output.view(sp, bs * n_div_sp, qh // sp, d)
+ # # (bs * n // sp, sp, qh // sp, d)
+ # attn_output = attn_output.transpose(0, 1)
+ attn_output = post_process_for_sequence_parallel_attn(
+ attn_output, sequence_parallel_mesh
+ )
+
+ attn_output = attn_output.reshape(*input_shape, -1).contiguous()
+ attn_output = self.o_proj(attn_output)
+ return attn_output, attn_weights
+
+
+def cuda_patched_llama_attn_prefilling(
+ self: LlamaAttention,
+ hidden_states: torch.Tensor,
+ position_embeddings: Tuple[torch.Tensor, torch.Tensor],
+ attention_mask: Optional[torch.Tensor],
+ past_key_value: Optional[Cache] = None,
+ cache_position: Optional[torch.LongTensor] = None,
+ sequence_parallel_mesh: Optional[DeviceMesh] = None,
+ **kwargs: Unpack[FlashAttentionKwargs],
+) -> Tuple[torch.Tensor, Optional[torch.Tensor], Optional[Tuple[torch.Tensor]]]:
+
+ input_shape = hidden_states.shape[:-1]
+ hidden_shape = (*input_shape, -1, self.head_dim)
+
+ query_states = self.q_proj(hidden_states).view(hidden_shape).transpose(1, 2)
+ key_states = self.k_proj(hidden_states).view(hidden_shape).transpose(1, 2)
+ value_states = self.v_proj(hidden_states).view(hidden_shape).transpose(1, 2)
+
+ cos, sin = position_embeddings
+ query_states, key_states = apply_rotary_pos_emb(query_states, key_states, cos, sin)
+
+ from lmdeploy.pytorch.kernels import fill_kv_cache
+ fill_kv_cache(
+ key_states.transpose(1, 2),
+ value_states.transpose(1, 2),
+ past_key_value[self.layer_idx][0],
+ past_key_value[self.layer_idx][1],
+ kwargs["cu_seq_lens_q"][:-1], # q_start_loc
+ kwargs["cu_seq_lens_q"][1:] - kwargs["cu_seq_lens_q"][:-1], # q_seq_length
+ kv_seq_length=kwargs["cu_seq_lens_k"][1:] - kwargs["cu_seq_lens_k"][:-1],
+ max_q_seq_length=kwargs["max_length_q"],
+ block_offsets=kwargs["block_table"],
+ )
+
+ assert self.config._attn_implementation == 'flash_attn_2'
+ attention_interface = ALL_ATTENTION_FUNCTIONS[self.config._attn_implementation]
+
+ attn_output, attn_weights = attention_interface(
+ self,
+ query_states,
+ key_states,
+ value_states,
+ attention_mask,
+ dropout=0.0 if not self.training else self.attention_dropout,
+ scaling=self.scaling,
+ **kwargs,
+ )
+
+ attn_output = attn_output.reshape(*input_shape, -1).contiguous()
+ attn_output = self.o_proj(attn_output)
+ return attn_output, attn_weights
+
+def cuda_patched_llama_attn_decoding(
+ self: LlamaAttention,
+ hidden_states: torch.Tensor,
+ position_embeddings: Tuple[torch.Tensor, torch.Tensor],
+ attention_mask: Optional[torch.Tensor],
+ past_key_value: Optional[Cache] = None,
+ cache_position: Optional[torch.LongTensor] = None,
+ sequence_parallel_mesh: Optional[DeviceMesh] = None,
+ **kwargs: Unpack[FlashAttentionKwargs],
+) -> Tuple[torch.Tensor, Optional[torch.Tensor], Optional[Tuple[torch.Tensor]]]:
+
+ input_shape = hidden_states.shape[:-1]
+ hidden_shape = (*input_shape, -1, self.head_dim)
+
+ query_states = self.q_proj(hidden_states).view(hidden_shape).transpose(1, 2)
+ key_states = self.k_proj(hidden_states).view(hidden_shape).transpose(1, 2)
+ value_states = self.v_proj(hidden_states).view(hidden_shape).transpose(1, 2)
+
+ cos, sin = position_embeddings
+ query_states, key_states = apply_rotary_pos_emb(query_states, key_states, cos, sin)
+
+
+ from lmdeploy.pytorch.kernels import fill_kv_cache
+ fill_kv_cache(
+ key_states.transpose(1, 2),
+ value_states.transpose(1, 2),
+ past_key_value[self.layer_idx][0],
+ past_key_value[self.layer_idx][1],
+ kwargs["cu_seq_lens_q"][:-1],
+ kwargs["cu_seq_lens_q"][1:] - kwargs["cu_seq_lens_q"][:-1],
+ kv_seq_length=kwargs["cu_seq_lens_k"][1:] - kwargs["cu_seq_lens_k"][:-1],
+ max_q_seq_length=kwargs["max_length_q"],
+ block_offsets=kwargs["block_table"],
+ )
+
+ assert self.config._attn_implementation == 'flash_attn_2'
+ from flash_attn import flash_attn_with_kvcache
+
+ attn_weights = None
+ attn_output = flash_attn_with_kvcache(
+ query_states.transpose(1,2).transpose(0,1),
+ past_key_value[self.layer_idx][0],
+ past_key_value[self.layer_idx][1],
+ cache_seqlens=kwargs["cu_seq_lens_k"][1:] - kwargs["cu_seq_lens_k"][:-1],
+ block_table=kwargs["block_table"],
+ causal=True
+ )
+
+ attn_output = attn_output.reshape(*input_shape, -1)
+ attn_output = self.o_proj(attn_output)
+ return attn_output, attn_weights
+
+
+def cuda_patched_llama_attn_forward(
+ self: LlamaAttention,
+ hidden_states: torch.Tensor,
+ position_embeddings: Tuple[torch.Tensor, torch.Tensor],
+ attention_mask: Optional[torch.Tensor],
+ past_key_value: Optional[Cache] = None,
+ cache_position: Optional[torch.LongTensor] = None,
+ sequence_parallel_mesh: Optional[DeviceMesh] = None,
+ **kwargs: Unpack[FlashAttentionKwargs],
+) -> Tuple[torch.Tensor, Optional[torch.Tensor], Optional[Tuple[torch.Tensor]]]:
+ if 'block_table' in kwargs:
+ # generating
+ if 'prefilling' in kwargs and kwargs['prefilling']:
+ return cuda_patched_llama_attn_prefilling(
+ self, hidden_states, position_embeddings,
+ attention_mask, past_key_value, cache_position,
+ sequence_parallel_mesh, **kwargs
+ )
+ else:
+ return cuda_patched_llama_attn_decoding(
+ self, hidden_states, position_embeddings,
+ attention_mask, past_key_value, cache_position,
+ sequence_parallel_mesh, **kwargs
+ )
+ else:
+ return cuda_patched_llama_attn_training(
+ self, hidden_states, position_embeddings,
+ attention_mask, past_key_value, cache_position,
+ sequence_parallel_mesh, **kwargs
+ )
+
+def cuda_patched_casual_forward(
+ self: LlamaForCausalLM,
+ input_ids: torch.LongTensor = None,
+ attention_mask: Optional[torch.Tensor] = None,
+ position_ids: Optional[torch.LongTensor] = None,
+ past_key_values: Optional[Union[Cache, List[torch.FloatTensor]]] = None,
+ inputs_embeds: Optional[torch.FloatTensor] = None,
+ labels: Optional[torch.LongTensor] = None,
+ use_cache: Optional[bool] = None,
+ output_attentions: Optional[bool] = None,
+ output_hidden_states: Optional[bool] = None,
+ return_dict: Optional[bool] = None,
+ cache_position: Optional[torch.LongTensor] = None,
+ num_logits_to_keep: int = 0,
+ label_shifted = False,
+ **kwargs,
+) -> Union[Tuple, CausalLMOutputWithPast]:
+
+ output_attentions = output_attentions if output_attentions is not None else self.config.output_attentions
+ output_hidden_states = (
+ output_hidden_states if output_hidden_states is not None else self.config.output_hidden_states
+ )
+ return_dict = return_dict if return_dict is not None else self.config.use_return_dict
+
+ # decoder outputs consists of (dec_features, layer_state, dec_hidden, dec_attn)
+ outputs = self.model(
+ input_ids=input_ids,
+ attention_mask=attention_mask,
+ position_ids=position_ids,
+ past_key_values=past_key_values,
+ inputs_embeds=inputs_embeds,
+ use_cache=use_cache,
+ output_attentions=output_attentions,
+ output_hidden_states=output_hidden_states,
+ return_dict=return_dict,
+ cache_position=cache_position,
+ **kwargs,
+ )
+
+ hidden_states = outputs[0]
+
+ if labels is None:
+ loss = None
+ logits = self.lm_head(hidden_states)
+ else:
+ if liger_kernel_is_available():
+ # unable to return logits when using Liger Kernel
+ logits = None
+
+ if label_shifted:
+ shift_hidden_states = hidden_states
+ shift_labels = labels
+ else:
+ shift_hidden_states = hidden_states[..., :-1, :].contiguous()
+ shift_labels = labels[..., 1:].contiguous()
+
+ shift_hidden_states = shift_hidden_states.view(-1, self.config.hidden_size)
+ shift_labels = shift_labels.view(-1)
+ shift_labels = shift_labels.to(shift_hidden_states.device)
+
+ from liger_kernel.transformers.fused_linear_cross_entropy import LigerFusedLinearCrossEntropyLoss
+ loss_fct = LigerFusedLinearCrossEntropyLoss()
+
+ lm_head_weight = self.lm_head.weight
+ if isinstance(lm_head_weight, DTensor):
+ assert isinstance(shift_hidden_states, DTensor)
+ shift_hidden_states = shift_hidden_states.to_local()
+ lm_head_weight = self.lm_head.weight.to_local()
+
+ loss = loss_fct(lm_head_weight, shift_hidden_states, shift_labels, self.lm_head.bias)
+
+ else:
+ logits = self.lm_head(hidden_states)
+
+ if label_shifted:
+ shift_logits = logits
+ shift_labels = labels
+ else:
+ shift_logits = logits[..., :-1, :].contiguous()
+ shift_labels = labels[..., 1:].contiguous()
+
+ shift_logits = shift_logits.view(-1, self.config.vocab_size)
+ shift_labels = shift_labels.view(-1)
+ shift_labels = shift_labels.to(shift_logits.device)
+
+ loss_fct = torch.nn.CrossEntropyLoss()
+ loss = loss_fct(shift_logits, shift_labels)
+
+ if not return_dict:
+ output = (logits,) + outputs[1:]
+ return (loss,) + output if loss is not None else output
+
+ return CausalLMOutputWithPast(
+ loss=loss,
+ logits=logits,
+ past_key_values=outputs.past_key_values,
+ hidden_states=outputs.hidden_states,
+ attentions=outputs.attentions,
+ )
+
+
+
+class CUDAPatchedLlamaForCausalLM(PatchedCausalLM):
+ device_type = 'cuda'
+
+ layer_tp_plan = {
+ "input_layernorm": SequenceParallel(),
+ "self_attn": PrepareModuleInput(
+ input_layouts=(Shard(1),),
+ desired_input_layouts=(Replicate(),),
+ ),
+ "self_attn.q_proj": ColwiseParallel(),
+ "self_attn.k_proj": ColwiseParallel(),
+ "self_attn.v_proj": ColwiseParallel(),
+ "self_attn.o_proj": RowwiseParallel(output_layouts=Shard(1)),
+ "post_attention_layernorm": SequenceParallel(),
+ "mlp": PrepareModuleInput(
+ input_layouts=(Shard(1),),
+ desired_input_layouts=(Replicate(),),
+ ),
+ "mlp.up_proj": ColwiseParallel(),
+ "mlp.down_proj": RowwiseParallel(output_layouts=Shard(1)),
+ "mlp.gate_proj": ColwiseParallel(),
+ }
+
+ casual_tp_plan = {
+ "model.embed_tokens": RowwiseParallel(
+ input_layouts=Replicate(),
+ output_layouts=Shard(1),
+ ),
+ "model.norm": PrepareModuleInput(
+ input_layouts=(Replicate(),),
+ desired_input_layouts=(Replicate(),),
+ ),
+ "lm_head": PrepareModuleInput(
+ input_layouts={(Shard(0),)},
+ desired_input_layouts=(Shard(0),),
+ use_local_output=True
+ ),
+ }
+
+ chat_template = HybridChatTemplate(
+ system=('<|start_header_id|>system<|end_header_id|>\n\n{system}'
+ '<|eot_id|>'),
+ user=('<|start_header_id|>user<|end_header_id|>\n\n{user}<|eot_id|>'
+ '<|start_header_id|>assistant<|end_header_id|>\n\n'),
+ assistant='{assistant}<|eot_id|>',
+ sep='',
+ stop_words=['<|eot_id|>']
+ )
+
+ def __init__(self, model: LlamaForCausalLM, fsdp_config: Optional[FSDPConfig]= None):
+ super().__init__(model, fsdp_config)
+
+ if dist.is_initialized() and dist.is_available():
+ rank = dist.get_rank()
+ else:
+ rank = 0
+
+ if rank == 0:
+ self._rank0_model = copy.deepcopy(model)
+ else:
+ self._rank0_model = None
+
+ self._patched_model = self.dispatch_hf_code(model)
+
+ self._model_config = ModelConfig(
+ num_hidden_layers=self.patched_model.config.num_hidden_layers,
+ num_attention_heads=self.patched_model.config.num_attention_heads,
+ num_key_value_heads=self.patched_model.config.num_key_value_heads,
+ hidden_size=self.patched_model.config.hidden_size,
+ intermediate_size=self.patched_model.config.intermediate_size,
+ vocab_size=self.patched_model.config.vocab_size,
+ head_dim=self.patched_model.config.head_dim
+ )
+
+ self._fsdp_config = fsdp_config
+ if self._fsdp_config is not None:
+ self.fully_shard(fsdp_config)
+
+ @property
+ def patched_model(self) -> LlamaForCausalLM:
+ return self._patched_model
+
+ @property
+ def rank0_model(self) -> LlamaForCausalLM:
+ return self._rank0_model
+
+ @property
+ def model_config(self) -> ModelConfig:
+ return self._model_config
+
+ @property
+ def fsdp_config(self) -> Optional[FSDPConfig]:
+ return self._fsdp_config
+
+ @property
+ def data_parallel_mesh(self):
+ return self.dp_mesh
+
+ @property
+ def data_mesh(self):
+ return self._data_mesh
+
+ @property
+ def sequence_parallel_mesh(self):
+ return self.sp_mesh
+
+ @staticmethod
+ def dispatch_hf_code(model) -> LlamaForCausalLM:
+
+ for name, module in model.named_modules():
+ if isinstance(module, LlamaAttention):
+ module.forward = types.MethodType(cuda_patched_llama_attn_training, module)
+ if isinstance(module, LlamaForCausalLM):
+ module.forward = types.MethodType(cuda_patched_casual_forward, module)
+
+ return model
+
+ def fully_shard(self, fsdp_config: FSDPConfig) -> None:
+ if fsdp_config.ep_size > 1:
+ raise NotImplementedError
+
+ world_size = dist.get_world_size()
+ sp_size = fsdp_config.sp_size
+ tp_size = fsdp_config.tp_size
+
+ if tp_size > sp_size:
+ # add warning
+ pass
+ elif tp_size < sp_size:
+ assert sp_size % tp_size == 0
+ sp_size = sp_size // tp_size
+
+ assert world_size % sp_size == 0
+ assert world_size % tp_size == 0
+ world_mesh_name = f'{fsdp_config.mesh_prefix}.world'
+ fsdp_mesh_name = f'{fsdp_config.mesh_prefix}.fsdp'
+ tp_mesh_name = f'{fsdp_config.mesh_prefix}.tp'
+ dp_mesh_name = f'{fsdp_config.mesh_prefix}.dp'
+ sp_mesh_name = f'{fsdp_config.mesh_prefix}.sp'
+ data_mesh_name = f'{fsdp_config.mesh_prefix}.data'
+ _tp_mesh_name = f'{fsdp_config.mesh_prefix}._tp'
+
+ world_mesh = init_device_mesh(
+ self.device_type,
+ (world_size ,),
+ mesh_dim_names = [world_mesh_name, ]
+ )
+ self.world_mesh = world_mesh[world_mesh_name]
+
+ model_mesh = init_device_mesh(
+ self.device_type,
+ (world_size // tp_size, tp_size),
+ mesh_dim_names = [fsdp_mesh_name, tp_mesh_name]
+ )
+
+ fsdp_mesh = model_mesh[fsdp_mesh_name]
+ tp_mesh = model_mesh[tp_mesh_name]
+
+ self.tp_mesh = tp_mesh
+ self.fsdp_mesh = fsdp_mesh
+
+ data_mesh = init_device_mesh(
+ self.device_type,
+ (world_size // tp_size // sp_size, sp_size , tp_size),
+ mesh_dim_names = [dp_mesh_name, sp_mesh_name, _tp_mesh_name]
+ )
+ self.dp_mesh = data_mesh[dp_mesh_name]
+ self.sp_mesh = data_mesh[sp_mesh_name]
+
+ _data_mesh = init_device_mesh(
+ self.device_type,
+ (world_size // tp_size // sp_size, sp_size * tp_size),
+ mesh_dim_names = [dp_mesh_name, data_mesh_name]
+ )
+ self._data_mesh = _data_mesh[data_mesh_name]
+
+ param_init_fn = partial(
+ lazy_init_fn,
+ module2name = { mod: name for name, mod in self.patched_model.named_modules() },
+ checkpoint_loader = HFCheckpointLoader(self.patched_model.config._name_or_path)
+ )
+
+ mp_policy = MixedPrecisionPolicy(
+ param_dtype=fsdp_config.param_dtype,
+ reduce_dtype=fsdp_config.reduce_dtype)
+
+ self.patched_model.model.rotary_emb = LlamaRotaryEmbedding(self.patched_model.config)
+
+ num_recompute_layers = int(self.model_config.num_hidden_layers * fsdp_config.recompute_ratio)
+
+ if fsdp_config.torch_compile:
+ compiled_layers = []
+
+ from torch.distributed._symmetric_memory import enable_symm_mem_for_group
+ torch._inductor.config._micro_pipeline_tp = True
+ enable_symm_mem_for_group(self.tp_mesh.get_group().group_name)
+
+ for layer in tqdm(self.patched_model.model.layers):
+
+ layer.apply(param_init_fn)
+ attention = layer.self_attn
+
+ if tp_mesh.size() > 1:
+
+ parallelize_module(
+ module=layer,
+ device_mesh=tp_mesh,
+ parallelize_plan=self.layer_tp_plan
+ )
+
+ if attention.layer_idx < num_recompute_layers:
+ layer = checkpoint_wrapper(layer)
+
+ if fsdp_config.torch_compile:
+ layer = torch.compile(layer, fullgraph=True)
+
+ self.patched_model.model.layers.register_module(str(attention.layer_idx), layer)
+
+ fully_shard(
+ layer,
+ mesh=fsdp_mesh,
+ mp_policy=mp_policy,
+ reshard_after_forward=fsdp_config.reshard_after_forward,
+ offload_policy=CPUOffloadPolicy() if fsdp_config.cpu_offload else None,
+ )
+
+ if fsdp_config.torch_compile:
+ compiled_layers.append(layer)
+
+ if version.parse(torch.__version__) >= version.parse("2.5.0"):
+ for layer_cur, layer_next in zip(self.patched_model.model.layers[:-1], self.patched_model.model.layers[1:]):
+ layer_cur.set_modules_to_forward_prefetch([layer_next])
+
+ self.patched_model.lm_head.apply(param_init_fn)
+ self.patched_model.model.embed_tokens.apply(param_init_fn)
+ self.patched_model.model.norm.apply(param_init_fn)
+
+ if tp_mesh.size() > 1:
+ _weight = self.patched_model.lm_head.weight
+ _dtensor_weight = nn.Parameter(
+ distribute_tensor(_weight, tp_mesh, [Replicate()]))
+ self.patched_model.lm_head.register_parameter('weight', _dtensor_weight)
+
+ _weight = self.patched_model.model.norm.weight
+ _dtensor_weight = nn.Parameter(
+ distribute_tensor(_weight, tp_mesh, [Replicate()]))
+ self.patched_model.model.norm.register_parameter('weight', _dtensor_weight)
+
+ parallelize_module(
+ self.patched_model,
+ tp_mesh,
+ self.casual_tp_plan,
+ )
+
+ fully_shard(
+ self.patched_model,
+ mesh=fsdp_mesh,
+ mp_policy=mp_policy,
+ reshard_after_forward=fsdp_config.reshard_after_forward,
+ offload_policy=CPUOffloadPolicy() if fsdp_config.cpu_offload else None,
+ )
+
+
+ def forward(
+ self,
+ input_ids: torch.LongTensor = None,
+ attention_mask: Optional[torch.Tensor] = None,
+ position_ids: Optional[torch.LongTensor] = None,
+ past_key_values: Optional[Union[Cache, List[torch.FloatTensor]]] = None,
+ inputs_embeds: Optional[torch.FloatTensor] = None,
+ labels: Optional[torch.LongTensor] = None,
+ use_cache: Optional[bool] = None,
+ output_attentions: Optional[bool] = None,
+ output_hidden_states: Optional[bool] = None,
+ return_dict: Optional[bool] = None,
+ cache_position: Optional[torch.LongTensor] = None,
+ num_logits_to_keep: int = 0,
+ label_shifted: bool = False,
+ cu_seq_lens_q: Optional[torch.LongTensor] = None,
+ cu_seq_lens_k: Optional[torch.LongTensor] = None,
+ max_length_q: Optional[int] = None,
+ max_length_k: Optional[int] = None,
+ sequence_parallel_mesh: Optional[DeviceMesh] = None,
+ ) -> Union[Tuple, CausalLMOutputWithPast]:
+
+ _input_ids = input_ids
+ _labels = labels
+ _position_ids = position_ids
+ _cu_seq_lens_q = cu_seq_lens_q
+ _cu_seq_lens_k = cu_seq_lens_k
+ _max_length_q = max_length_q
+ _max_length_k = max_length_k
+
+ if self.fsdp_config.torch_compile:
+ _input_ids = pad_to_max_length(_input_ids, 0, self.fsdp_config.max_length, 1)
+ _position_ids = pad_to_max_length(_position_ids, 0, self.fsdp_config.max_length, 1)
+ if labels is not None:
+ _labels = pad_to_max_length(_labels, -100, self.fsdp_config.max_length, 1)
+ else:
+ if sequence_parallel_mesh and sequence_parallel_mesh.size() > 1:
+ multiple_of = sequence_parallel_mesh.size() * self.tp_mesh.size()
+ else:
+ multiple_of = self.tp_mesh.size()
+
+ _input_ids = pad_to_multiple_of(_input_ids, 0, multiple_of, 1)
+ _position_ids = pad_to_multiple_of(_position_ids, 0, multiple_of, 1)
+ if labels is not None:
+ _labels = pad_to_multiple_of(_labels, -100, multiple_of, 1)
+
+ num_padded_tokens = _input_ids.numel() - input_ids.numel()
+
+ if sequence_parallel_mesh and sequence_parallel_mesh.size() > 1:
+ _input_ids = split_for_sequence_parallel(
+ _input_ids, dim=1, sp_mesh=sequence_parallel_mesh)
+ _position_ids = split_for_sequence_parallel(
+ _position_ids, dim=1, sp_mesh=sequence_parallel_mesh)
+
+ if labels is not None:
+ _labels = split_for_sequence_parallel(
+ _labels, dim=1, sp_mesh=sequence_parallel_mesh)
+
+ if self.tp_mesh.size() > 1:
+ if labels is not None:
+ _labels = split_for_sequence_parallel(
+ _labels, dim=1, sp_mesh=self.tp_mesh)
+
+ if self.training and num_padded_tokens > 0:
+ assert torch.any(cu_seq_lens_k == cu_seq_lens_q)
+ _cu_seq_lens_q = _cu_seq_lens_q.tolist()
+ _cu_seq_lens_q.append(_cu_seq_lens_q[-1] + num_padded_tokens)
+
+ _cu_seq_lens_q = torch.IntTensor(_cu_seq_lens_q).to(cu_seq_lens_q.device)
+ _cu_seq_lens_k = _cu_seq_lens_q
+
+ _max_length_q = max(_max_length_q, num_padded_tokens)
+ _max_length_k = _max_length_q
+
+ outputs = self.patched_model(
+ _input_ids,
+ attention_mask,
+ _position_ids,
+ past_key_values,
+ inputs_embeds,
+ _labels,
+ use_cache,
+ output_attentions,
+ output_hidden_states,
+ return_dict,
+ cache_position,
+ num_logits_to_keep,
+ label_shifted=label_shifted,
+ cu_seq_lens_q=_cu_seq_lens_q,
+ cu_seq_lens_k=_cu_seq_lens_k,
+ max_length_q=_max_length_q,
+ max_length_k=_max_length_k,
+ sequence_parallel_mesh=self.sequence_parallel_mesh,
+ )
+
+ if outputs.loss is not None:
+ outputs.loss = outputs.loss * (_labels >= 0).sum()
+ if self.tp_mesh.size() > 1:
+ outputs.loss = dist.nn.all_reduce(outputs.loss, group=self.tp_mesh.get_group())
+ if sequence_parallel_mesh and sequence_parallel_mesh.size() > 1:
+ outputs.loss = dist.nn.all_reduce(outputs.loss, group=sequence_parallel_mesh.get_group())
+ outputs.loss = outputs.loss / (labels >= 0).sum()
+
+ if outputs.logits is not None:
+ outputs.logits = outputs.logits[:, :cu_seq_lens_q[-1]]
+
+ return outputs
+
+ def trainable_parameters(self):
+ _requried_grad_params = [
+ param for param in self.patched_model.parameters() if param.requires_grad
+ ]
+ return _requried_grad_params
+
+ def clip_grad_norm(self, max_norm):
+
+ if self.tp_mesh.size() > 1:
+ dist.all_reduce(self.patched_model.lm_head.weight.grad.to_local(), group=self.tp_mesh.get_group())
+ dist.all_reduce(self.patched_model.model.norm.weight.grad.to_local(), group=self.tp_mesh.get_group())
+ self.patched_model.lm_head.weight.grad.div_(self.tp_mesh.size())
+ self.patched_model.model.norm.weight.grad.div_(self.tp_mesh.size())
+ for param in self.trainable_parameters():
+ param.grad.div_(self.tp_mesh.size())
+
+ grad_norm = clip_grad_norm_(self.trainable_parameters(), self.world_mesh, max_norm)
+ return grad_norm
+
+class MLUPatchedLlamaForCausalLM(CUDAPatchedLlamaForCausalLM):
+ device_type = 'mlu'
+
+class MuxiPatchedLlamaForCausalLM(CUDAPatchedLlamaForCausalLM):
+ device_type = 'muxi'
+
+if __name__ == '__main__':
+
+ from transformers import AutoModelForCausalLM, AutoTokenizer
+ from xtuner._lite.parallel import setup_parallel
+
+ setup_parallel()
+ with torch.device('meta'):
+ model = AutoModelForCausalLM.from_pretrained(
+ 'internlm/internlm3-8b-instruct'
+ )
+
+ fsdp_config = FSDPConfig()
+ patched_model = CUDAPatchedLlamaForCausalLM(model, fsdp_config)
+
+
diff --git a/xtuner/_lite/patches/utils.py b/xtuner/_lite/patches/utils.py
new file mode 100644
index 000000000..2cab1725c
--- /dev/null
+++ b/xtuner/_lite/patches/utils.py
@@ -0,0 +1,29 @@
+import torch
+
+def pad_to_multiple_of(sequence, padding_value, multiple_of, dim=-1):
+
+ length = sequence.shape[dim]
+ if length % multiple_of == 0:
+ return sequence
+
+ pad_num = multiple_of - (length % multiple_of)
+ pad_shape = (*sequence.shape[:dim], pad_num,
+ *sequence.shape[dim + 1:]) if dim != -1 else (
+ *sequence.shape[:dim], pad_num)
+ pad = torch.full(
+ pad_shape, padding_value, dtype=sequence.dtype, device=sequence.device)
+ sequence = torch.cat([sequence, pad], dim=dim)
+ return sequence
+
+def pad_to_max_length(sequence, padding_value, max_length, dim=-1):
+
+ length = sequence.shape[dim]
+ assert length <= max_length
+ pad_num = max_length - length
+ pad_shape = (*sequence.shape[:dim], pad_num,
+ *sequence.shape[dim + 1:]) if dim != -1 else (
+ *sequence.shape[:dim], pad_num)
+ pad = torch.full(
+ pad_shape, padding_value, dtype=sequence.dtype, device=sequence.device)
+ sequence = torch.cat([sequence, pad], dim=dim)
+ return sequence
diff --git a/xtuner/configs/cohere/README.md b/xtuner/configs/cohere/README.md
new file mode 100644
index 000000000..5d306cb33
--- /dev/null
+++ b/xtuner/configs/cohere/README.md
@@ -0,0 +1,48 @@
+# Cohere 8x7B
+
+## Install
+
+```bash
+# Install the latest xtuner
+pip install -U 'xtuner[deepspeed]'
+
+# Cohere requires the latest version of transformers.
+pip install git+https://github.com/huggingface/transformers.git
+
+# Sequence parallel requires flash-attn
+pip install flash-attn
+```
+
+## Full Parameter Fine-tune
+
+Full parameter fine-tune needs 64 A100-80G
+
+### slurm
+
+Note: `$PARTITION` means the virtual partition of slurm.
+
+```bash
+srun -p $PARTITION --job-name=Cohere --nodes=8 --gres=gpu:8 --ntasks-per-node=8 xtuner train cohere_100b_128k_sp32 --deepspeed deepspeed_zero3 --launcher slurm
+```
+
+### torchrun
+
+Note: `$NODE_0_ADDR` means the ip address of the node_0 machine.
+
+```bash
+# excuete on node 0
+NPROC_PER_NODE=8 NNODES=8 PORT=29600 ADDR=$NODE_0_ADDR NODE_RANK=0 xtuner train cohere_100b_128k_sp32 --deepspeed deepspeed_zero3
+
+# excuete on node 1
+NPROC_PER_NODE=8 NNODES=8 PORT=29600 ADDR=$NODE_0_ADDR NODE_RANK=1 xtuner train cohere_100b_128k_sp32 --deepspeed deepspeed_zero3
+```
+
+### Speed
+
+16 * A100 80G:
+
+| Model | Sequence Length | GPUs Number | Sequence Parallel World Size | Tokens per Second | TFLOPs |
+| :---------: | :-------------: | :---------: | :--------------------------: | :---------------: | :----: |
+| Cohere_100b | 128k | 64 | 32 | 97.3 | 173.4 |
+| Cohere_100b | 128k | 128 | 16 | 102.1 | 182.7 |
+| Cohere_100b | 128k | 256 | 16 | 101.3 | 181.3 |
diff --git a/xtuner/configs/cohere/cohere_104b/cohere_100b_128k_sp32.py b/xtuner/configs/cohere/cohere_104b/cohere_100b_128k_sp32.py
new file mode 100644
index 000000000..0882be1ae
--- /dev/null
+++ b/xtuner/configs/cohere/cohere_104b/cohere_100b_128k_sp32.py
@@ -0,0 +1,211 @@
+# Copyright (c) OpenMMLab. All rights reserved.
+import torch
+from datasets import load_dataset
+from mmengine.hooks import (CheckpointHook, DistSamplerSeedHook, IterTimerHook,
+ LoggerHook, ParamSchedulerHook)
+from mmengine.optim import AmpOptimWrapper, CosineAnnealingLR
+from torch.optim import AdamW
+from transformers import AutoModelForCausalLM, AutoTokenizer
+
+from xtuner.dataset import ConcatDataset, process_hf_dataset
+from xtuner.dataset.collate_fns import default_collate_fn
+from xtuner.dataset.map_fns import (alpaca_map_fn, alpaca_zh_map_fn,
+ template_map_fn_factory)
+from xtuner.engine.hooks import (DatasetInfoHook, EvaluateChatHook,
+ ThroughputHook,
+ VarlenAttnArgsToMessageHubHook)
+from xtuner.engine.runner import TrainLoop
+from xtuner.model import SupervisedFinetune
+from xtuner.parallel.sequence import SequenceParallelSampler
+from xtuner.utils import PROMPT_TEMPLATE, SYSTEM_TEMPLATE
+
+#######################################################################
+# PART 1 Settings #
+#######################################################################
+# Model
+pretrained_model_name_or_path = 'CohereForAI/c4ai-command-r-plus'
+use_varlen_attn = False
+sequence_parallel_size = 32
+
+# Data
+alpaca_zh_path = 'silk-road/alpaca-data-gpt4-chinese'
+alpaca_en_path = 'tatsu-lab/alpaca'
+prompt_template = PROMPT_TEMPLATE.cohere_chat
+max_length = 131072
+pack_to_max_length = True
+
+# Scheduler & Optimizer
+batch_size = 1 # per_device
+accumulative_counts = 32
+dataloader_num_workers = 4
+max_epochs = 3
+optim_type = AdamW
+lr = 2e-5
+betas = (0.9, 0.999)
+weight_decay = 0
+max_norm = 1 # grad clip
+warmup_ratio = 0.05
+
+# Save
+save_steps = 500
+save_total_limit = 1 # Maximum checkpoints to keep (-1 means unlimited)
+
+# Evaluate the generation performance during the training
+evaluation_freq = 10
+SYSTEM = SYSTEM_TEMPLATE.alpaca
+evaluation_inputs = [
+ '请给我介绍五个上海的景点', 'Please tell me five scenic spots in Shanghai'
+]
+
+#######################################################################
+# PART 2 Model & Tokenizer #
+#######################################################################
+tokenizer = dict(
+ type=AutoTokenizer.from_pretrained,
+ pretrained_model_name_or_path=pretrained_model_name_or_path,
+ trust_remote_code=True,
+ padding_side='right')
+
+model = dict(
+ type=SupervisedFinetune,
+ use_varlen_attn=use_varlen_attn,
+ llm=dict(
+ type=AutoModelForCausalLM.from_pretrained,
+ pretrained_model_name_or_path=pretrained_model_name_or_path,
+ trust_remote_code=True,
+ torch_dtype=torch.bfloat16,
+ attn_implementation='flash_attention_2'))
+
+#######################################################################
+# PART 3 Dataset & Dataloader #
+#######################################################################
+alpaca_en = dict(
+ type=process_hf_dataset,
+ dataset=dict(type=load_dataset, path=alpaca_en_path),
+ tokenizer=tokenizer,
+ max_length=max_length,
+ dataset_map_fn=alpaca_map_fn,
+ template_map_fn=dict(
+ type=template_map_fn_factory, template=prompt_template),
+ remove_unused_columns=True,
+ shuffle_before_pack=True,
+ pack_to_max_length=pack_to_max_length,
+ use_varlen_attn=use_varlen_attn)
+
+alpaca_zh = dict(
+ type=process_hf_dataset,
+ dataset=dict(type=load_dataset, path=alpaca_zh_path),
+ tokenizer=tokenizer,
+ max_length=max_length,
+ dataset_map_fn=alpaca_zh_map_fn,
+ template_map_fn=dict(
+ type=template_map_fn_factory, template=prompt_template),
+ remove_unused_columns=True,
+ shuffle_before_pack=True,
+ pack_to_max_length=pack_to_max_length,
+ use_varlen_attn=use_varlen_attn)
+
+train_dataset = dict(type=ConcatDataset, datasets=[alpaca_en, alpaca_zh])
+
+train_dataloader = dict(
+ batch_size=batch_size,
+ num_workers=dataloader_num_workers,
+ dataset=train_dataset,
+ sampler=dict(type=SequenceParallelSampler, seed=1024),
+ collate_fn=dict(type=default_collate_fn, use_varlen_attn=use_varlen_attn))
+
+#######################################################################
+# PART 4 Scheduler & Optimizer #
+#######################################################################
+# optimizer
+optim_wrapper = dict(
+ type=AmpOptimWrapper,
+ optimizer=dict(
+ type=optim_type, lr=lr, betas=betas, weight_decay=weight_decay),
+ clip_grad=dict(max_norm=max_norm, error_if_nonfinite=False),
+ accumulative_counts=accumulative_counts,
+ loss_scale='dynamic')
+
+# learning policy
+# More information: https://github.com/open-mmlab/mmengine/blob/main/docs/en/tutorials/param_scheduler.md # noqa: E501
+param_scheduler = [
+ dict(
+ type=CosineAnnealingLR,
+ eta_min=lr * 0.15,
+ by_epoch=True,
+ begin=0,
+ end=max_epochs,
+ convert_to_iter_based=True)
+]
+
+# train, val, test setting
+train_cfg = dict(type=TrainLoop, max_iters=16)
+
+#######################################################################
+# PART 5 Runtime #
+#######################################################################
+# Log the dialogue periodically during the training process, optional
+custom_hooks = [
+ dict(type=DatasetInfoHook, tokenizer=tokenizer),
+ dict(type=ThroughputHook),
+ dict(
+ type=EvaluateChatHook,
+ tokenizer=tokenizer,
+ every_n_iters=evaluation_freq,
+ evaluation_inputs=evaluation_inputs,
+ system=SYSTEM,
+ max_new_tokens=100,
+ prompt_template=prompt_template)
+]
+
+if use_varlen_attn:
+ custom_hooks += [dict(type=VarlenAttnArgsToMessageHubHook)]
+
+# configure default hooks
+default_hooks = dict(
+ # record the time of every iteration.
+ timer=dict(type=IterTimerHook),
+ # print log every 10 iterations.
+ logger=dict(type=LoggerHook, log_metric_by_epoch=False, interval=1),
+ # enable the parameter scheduler.
+ param_scheduler=dict(type=ParamSchedulerHook),
+ # save checkpoint per `save_steps`.
+ checkpoint=dict(
+ type=CheckpointHook,
+ by_epoch=False,
+ interval=save_steps,
+ max_keep_ckpts=save_total_limit),
+ # set sampler seed in distributed evrionment.
+ sampler_seed=dict(type=DistSamplerSeedHook),
+)
+
+# configure environment
+env_cfg = dict(
+ # whether to enable cudnn benchmark
+ cudnn_benchmark=False,
+ # set multi process parameters
+ mp_cfg=dict(mp_start_method='fork', opencv_num_threads=0),
+ # set distributed parameters
+ dist_cfg=dict(backend='nccl'),
+)
+
+# set visualizer
+visualizer = None
+
+# set log level
+log_level = 'INFO'
+
+# load from which checkpoint
+load_from = None
+
+# whether to resume training from the loaded checkpoint
+resume = False
+
+# Defaults to use random seed and disable `deterministic`
+randomness = dict(seed=None, deterministic=False)
+
+# set log processor
+log_processor = dict(
+ by_epoch=False,
+ window_size=1,
+ mean_pattern=r'.*(loss|time|data_time|grad_norm|tflops).*')
diff --git a/xtuner/configs/custom_dataset/pretrain/baichuan/baichuan2_13b_base_full_custom_pretrain_e1.py b/xtuner/configs/custom_dataset/pretrain/baichuan/baichuan2_13b_base_full_custom_pretrain_e1.py
new file mode 100644
index 000000000..d246946ec
--- /dev/null
+++ b/xtuner/configs/custom_dataset/pretrain/baichuan/baichuan2_13b_base_full_custom_pretrain_e1.py
@@ -0,0 +1,199 @@
+# Copyright (c) OpenMMLab. All rights reserved.
+"""Data format:
+
+[
+ {
+ "text": "xxx"
+ },
+ {
+ "text": "xxx"
+ },
+ ...
+]
+""" # noqa: E501
+
+from datasets import load_dataset
+from mmengine.dataset import DefaultSampler
+from mmengine.hooks import (CheckpointHook, DistSamplerSeedHook, IterTimerHook,
+ LoggerHook, ParamSchedulerHook)
+from mmengine.optim import AmpOptimWrapper, CosineAnnealingLR, LinearLR
+from torch.optim import AdamW
+from transformers import AutoModelForCausalLM, AutoTokenizer
+
+from xtuner.dataset import process_hf_dataset
+from xtuner.dataset.collate_fns import default_collate_fn
+from xtuner.dataset.map_fns import pretrain_map_fn
+from xtuner.engine.hooks import (DatasetInfoHook, EvaluateChatHook,
+ VarlenAttnArgsToMessageHubHook)
+from xtuner.engine.runner import TrainLoop
+from xtuner.model import SupervisedFinetune
+
+#######################################################################
+# PART 1 Settings #
+#######################################################################
+# Model
+pretrained_model_name_or_path = 'baichuan-inc/Baichuan2-13B-Base'
+use_varlen_attn = False
+
+# Data
+data_files = ['/path/to/json/file.json']
+max_length = 2048
+pack_to_max_length = True
+
+# Scheduler & Optimizer
+batch_size = 1 # per_device
+accumulative_counts = 16 # bs = 1 GPU * 1 batch_size_per_device * 16 acc
+dataloader_num_workers = 0
+max_epochs = 1
+optim_type = AdamW
+lr = 2e-5
+betas = (0.9, 0.999)
+weight_decay = 0
+max_norm = 1 # grad clip
+warmup_ratio = 0.03
+
+# Save
+save_steps = 500
+save_total_limit = 2 # Maximum checkpoints to keep (-1 means unlimited)
+
+# Evaluate the generation performance during the training
+evaluation_freq = 500
+SYSTEM = ''
+evaluation_inputs = ['上海是', 'Shanghai is']
+
+#######################################################################
+# PART 2 Model & Tokenizer #
+#######################################################################
+tokenizer = dict(
+ type=AutoTokenizer.from_pretrained,
+ pretrained_model_name_or_path=pretrained_model_name_or_path,
+ trust_remote_code=True,
+ padding_side='right')
+
+model = dict(
+ type=SupervisedFinetune,
+ use_varlen_attn=use_varlen_attn,
+ llm=dict(
+ type=AutoModelForCausalLM.from_pretrained,
+ pretrained_model_name_or_path=pretrained_model_name_or_path,
+ trust_remote_code=True))
+
+#######################################################################
+# PART 3 Dataset & Dataloader #
+#######################################################################
+train_dataset = dict(
+ type=process_hf_dataset,
+ dataset=dict(type=load_dataset, path='json', data_files=data_files),
+ tokenizer=tokenizer,
+ max_length=max_length,
+ dataset_map_fn=pretrain_map_fn,
+ template_map_fn=None,
+ remove_unused_columns=True,
+ shuffle_before_pack=False,
+ pack_to_max_length=pack_to_max_length,
+ use_varlen_attn=use_varlen_attn)
+
+train_dataloader = dict(
+ batch_size=batch_size,
+ num_workers=dataloader_num_workers,
+ dataset=train_dataset,
+ sampler=dict(type=DefaultSampler, shuffle=True),
+ collate_fn=dict(type=default_collate_fn, use_varlen_attn=use_varlen_attn))
+
+#######################################################################
+# PART 4 Scheduler & Optimizer #
+#######################################################################
+# optimizer
+optim_wrapper = dict(
+ type=AmpOptimWrapper,
+ optimizer=dict(
+ type=optim_type, lr=lr, betas=betas, weight_decay=weight_decay),
+ clip_grad=dict(max_norm=max_norm, error_if_nonfinite=False),
+ accumulative_counts=accumulative_counts,
+ loss_scale='dynamic',
+ dtype='float16')
+
+# learning policy
+# More information: https://github.com/open-mmlab/mmengine/blob/main/docs/en/tutorials/param_scheduler.md # noqa: E501
+param_scheduler = [
+ dict(
+ type=LinearLR,
+ start_factor=1e-5,
+ by_epoch=True,
+ begin=0,
+ end=warmup_ratio * max_epochs,
+ convert_to_iter_based=True),
+ dict(
+ type=CosineAnnealingLR,
+ eta_min=0.0,
+ by_epoch=True,
+ begin=warmup_ratio * max_epochs,
+ end=max_epochs,
+ convert_to_iter_based=True)
+]
+
+# train, val, test setting
+train_cfg = dict(type=TrainLoop, max_epochs=max_epochs)
+
+#######################################################################
+# PART 5 Runtime #
+#######################################################################
+# Log the dialogue periodically during the training process, optional
+custom_hooks = [
+ dict(type=DatasetInfoHook, tokenizer=tokenizer),
+ dict(
+ type=EvaluateChatHook,
+ tokenizer=tokenizer,
+ every_n_iters=evaluation_freq,
+ evaluation_inputs=evaluation_inputs,
+ system=SYSTEM)
+]
+
+if use_varlen_attn:
+ custom_hooks += [dict(type=VarlenAttnArgsToMessageHubHook)]
+
+# configure default hooks
+default_hooks = dict(
+ # record the time of every iteration.
+ timer=dict(type=IterTimerHook),
+ # print log every 10 iterations.
+ logger=dict(type=LoggerHook, log_metric_by_epoch=False, interval=10),
+ # enable the parameter scheduler.
+ param_scheduler=dict(type=ParamSchedulerHook),
+ # save checkpoint per `save_steps`.
+ checkpoint=dict(
+ type=CheckpointHook,
+ by_epoch=False,
+ interval=save_steps,
+ max_keep_ckpts=save_total_limit),
+ # set sampler seed in distributed evrionment.
+ sampler_seed=dict(type=DistSamplerSeedHook),
+)
+
+# configure environment
+env_cfg = dict(
+ # whether to enable cudnn benchmark
+ cudnn_benchmark=False,
+ # set multi process parameters
+ mp_cfg=dict(mp_start_method='fork', opencv_num_threads=0),
+ # set distributed parameters
+ dist_cfg=dict(backend='nccl'),
+)
+
+# set visualizer
+visualizer = None
+
+# set log level
+log_level = 'INFO'
+
+# load from which checkpoint
+load_from = None
+
+# whether to resume training from the loaded checkpoint
+resume = False
+
+# Defaults to use random seed and disable `deterministic`
+randomness = dict(seed=None, deterministic=False)
+
+# set log processor
+log_processor = dict(by_epoch=False)
diff --git a/xtuner/configs/custom_dataset/pretrain/baichuan/baichuan2_7b_base_full_custom_pretrain_e1.py b/xtuner/configs/custom_dataset/pretrain/baichuan/baichuan2_7b_base_full_custom_pretrain_e1.py
new file mode 100644
index 000000000..87cbbbb62
--- /dev/null
+++ b/xtuner/configs/custom_dataset/pretrain/baichuan/baichuan2_7b_base_full_custom_pretrain_e1.py
@@ -0,0 +1,199 @@
+# Copyright (c) OpenMMLab. All rights reserved.
+"""Data format:
+
+[
+ {
+ "text": "xxx"
+ },
+ {
+ "text": "xxx"
+ },
+ ...
+]
+""" # noqa: E501
+
+from datasets import load_dataset
+from mmengine.dataset import DefaultSampler
+from mmengine.hooks import (CheckpointHook, DistSamplerSeedHook, IterTimerHook,
+ LoggerHook, ParamSchedulerHook)
+from mmengine.optim import AmpOptimWrapper, CosineAnnealingLR, LinearLR
+from torch.optim import AdamW
+from transformers import AutoModelForCausalLM, AutoTokenizer
+
+from xtuner.dataset import process_hf_dataset
+from xtuner.dataset.collate_fns import default_collate_fn
+from xtuner.dataset.map_fns import pretrain_map_fn
+from xtuner.engine.hooks import (DatasetInfoHook, EvaluateChatHook,
+ VarlenAttnArgsToMessageHubHook)
+from xtuner.engine.runner import TrainLoop
+from xtuner.model import SupervisedFinetune
+
+#######################################################################
+# PART 1 Settings #
+#######################################################################
+# Model
+pretrained_model_name_or_path = 'baichuan-inc/Baichuan2-7B-Base'
+use_varlen_attn = False
+
+# Data
+data_files = ['/path/to/json/file.json']
+max_length = 2048
+pack_to_max_length = True
+
+# Scheduler & Optimizer
+batch_size = 1 # per_device
+accumulative_counts = 16 # bs = 1 GPU * 1 batch_size_per_device * 16 acc
+dataloader_num_workers = 0
+max_epochs = 1
+optim_type = AdamW
+lr = 2e-5
+betas = (0.9, 0.999)
+weight_decay = 0
+max_norm = 1 # grad clip
+warmup_ratio = 0.03
+
+# Save
+save_steps = 500
+save_total_limit = 2 # Maximum checkpoints to keep (-1 means unlimited)
+
+# Evaluate the generation performance during the training
+evaluation_freq = 500
+SYSTEM = ''
+evaluation_inputs = ['上海是', 'Shanghai is']
+
+#######################################################################
+# PART 2 Model & Tokenizer #
+#######################################################################
+tokenizer = dict(
+ type=AutoTokenizer.from_pretrained,
+ pretrained_model_name_or_path=pretrained_model_name_or_path,
+ trust_remote_code=True,
+ padding_side='right')
+
+model = dict(
+ type=SupervisedFinetune,
+ use_varlen_attn=use_varlen_attn,
+ llm=dict(
+ type=AutoModelForCausalLM.from_pretrained,
+ pretrained_model_name_or_path=pretrained_model_name_or_path,
+ trust_remote_code=True))
+
+#######################################################################
+# PART 3 Dataset & Dataloader #
+#######################################################################
+train_dataset = dict(
+ type=process_hf_dataset,
+ dataset=dict(type=load_dataset, path='json', data_files=data_files),
+ tokenizer=tokenizer,
+ max_length=max_length,
+ dataset_map_fn=pretrain_map_fn,
+ template_map_fn=None,
+ remove_unused_columns=True,
+ shuffle_before_pack=False,
+ pack_to_max_length=pack_to_max_length,
+ use_varlen_attn=use_varlen_attn)
+
+train_dataloader = dict(
+ batch_size=batch_size,
+ num_workers=dataloader_num_workers,
+ dataset=train_dataset,
+ sampler=dict(type=DefaultSampler, shuffle=True),
+ collate_fn=dict(type=default_collate_fn, use_varlen_attn=use_varlen_attn))
+
+#######################################################################
+# PART 4 Scheduler & Optimizer #
+#######################################################################
+# optimizer
+optim_wrapper = dict(
+ type=AmpOptimWrapper,
+ optimizer=dict(
+ type=optim_type, lr=lr, betas=betas, weight_decay=weight_decay),
+ clip_grad=dict(max_norm=max_norm, error_if_nonfinite=False),
+ accumulative_counts=accumulative_counts,
+ loss_scale='dynamic',
+ dtype='float16')
+
+# learning policy
+# More information: https://github.com/open-mmlab/mmengine/blob/main/docs/en/tutorials/param_scheduler.md # noqa: E501
+param_scheduler = [
+ dict(
+ type=LinearLR,
+ start_factor=1e-5,
+ by_epoch=True,
+ begin=0,
+ end=warmup_ratio * max_epochs,
+ convert_to_iter_based=True),
+ dict(
+ type=CosineAnnealingLR,
+ eta_min=0.0,
+ by_epoch=True,
+ begin=warmup_ratio * max_epochs,
+ end=max_epochs,
+ convert_to_iter_based=True)
+]
+
+# train, val, test setting
+train_cfg = dict(type=TrainLoop, max_epochs=max_epochs)
+
+#######################################################################
+# PART 5 Runtime #
+#######################################################################
+# Log the dialogue periodically during the training process, optional
+custom_hooks = [
+ dict(type=DatasetInfoHook, tokenizer=tokenizer),
+ dict(
+ type=EvaluateChatHook,
+ tokenizer=tokenizer,
+ every_n_iters=evaluation_freq,
+ evaluation_inputs=evaluation_inputs,
+ system=SYSTEM)
+]
+
+if use_varlen_attn:
+ custom_hooks += [dict(type=VarlenAttnArgsToMessageHubHook)]
+
+# configure default hooks
+default_hooks = dict(
+ # record the time of every iteration.
+ timer=dict(type=IterTimerHook),
+ # print log every 10 iterations.
+ logger=dict(type=LoggerHook, log_metric_by_epoch=False, interval=10),
+ # enable the parameter scheduler.
+ param_scheduler=dict(type=ParamSchedulerHook),
+ # save checkpoint per `save_steps`.
+ checkpoint=dict(
+ type=CheckpointHook,
+ by_epoch=False,
+ interval=save_steps,
+ max_keep_ckpts=save_total_limit),
+ # set sampler seed in distributed evrionment.
+ sampler_seed=dict(type=DistSamplerSeedHook),
+)
+
+# configure environment
+env_cfg = dict(
+ # whether to enable cudnn benchmark
+ cudnn_benchmark=False,
+ # set multi process parameters
+ mp_cfg=dict(mp_start_method='fork', opencv_num_threads=0),
+ # set distributed parameters
+ dist_cfg=dict(backend='nccl'),
+)
+
+# set visualizer
+visualizer = None
+
+# set log level
+log_level = 'INFO'
+
+# load from which checkpoint
+load_from = None
+
+# whether to resume training from the loaded checkpoint
+resume = False
+
+# Defaults to use random seed and disable `deterministic`
+randomness = dict(seed=None, deterministic=False)
+
+# set log processor
+log_processor = dict(by_epoch=False)
diff --git a/xtuner/configs/custom_dataset/pretrain/chatglm/chatglm2_6b_full_custom_pretrain_e1.py b/xtuner/configs/custom_dataset/pretrain/chatglm/chatglm2_6b_full_custom_pretrain_e1.py
new file mode 100644
index 000000000..086985fef
--- /dev/null
+++ b/xtuner/configs/custom_dataset/pretrain/chatglm/chatglm2_6b_full_custom_pretrain_e1.py
@@ -0,0 +1,199 @@
+# Copyright (c) OpenMMLab. All rights reserved.
+"""Data format:
+
+[
+ {
+ "text": "xxx"
+ },
+ {
+ "text": "xxx"
+ },
+ ...
+]
+""" # noqa: E501
+
+from datasets import load_dataset
+from mmengine.dataset import DefaultSampler
+from mmengine.hooks import (CheckpointHook, DistSamplerSeedHook, IterTimerHook,
+ LoggerHook, ParamSchedulerHook)
+from mmengine.optim import AmpOptimWrapper, CosineAnnealingLR, LinearLR
+from torch.optim import AdamW
+from transformers import AutoModelForCausalLM, AutoTokenizer
+
+from xtuner.dataset import process_hf_dataset
+from xtuner.dataset.collate_fns import default_collate_fn
+from xtuner.dataset.map_fns import pretrain_map_fn
+from xtuner.engine.hooks import (DatasetInfoHook, EvaluateChatHook,
+ VarlenAttnArgsToMessageHubHook)
+from xtuner.engine.runner import TrainLoop
+from xtuner.model import SupervisedFinetune
+
+#######################################################################
+# PART 1 Settings #
+#######################################################################
+# Model
+pretrained_model_name_or_path = 'THUDM/chatglm2-6b'
+use_varlen_attn = False
+
+# Data
+data_files = ['/path/to/json/file.json']
+max_length = 2048
+pack_to_max_length = True
+
+# Scheduler & Optimizer
+batch_size = 1 # per_device
+accumulative_counts = 16 # bs = 1 GPU * 1 batch_size_per_device * 16 acc
+dataloader_num_workers = 0
+max_epochs = 1
+optim_type = AdamW
+lr = 2e-5
+betas = (0.9, 0.999)
+weight_decay = 0
+max_norm = 1 # grad clip
+warmup_ratio = 0.03
+
+# Save
+save_steps = 500
+save_total_limit = 2 # Maximum checkpoints to keep (-1 means unlimited)
+
+# Evaluate the generation performance during the training
+evaluation_freq = 500
+SYSTEM = ''
+evaluation_inputs = ['上海是', 'Shanghai is']
+
+#######################################################################
+# PART 2 Model & Tokenizer #
+#######################################################################
+tokenizer = dict(
+ type=AutoTokenizer.from_pretrained,
+ pretrained_model_name_or_path=pretrained_model_name_or_path,
+ trust_remote_code=True,
+ padding_side='left')
+
+model = dict(
+ type=SupervisedFinetune,
+ use_varlen_attn=use_varlen_attn,
+ llm=dict(
+ type=AutoModelForCausalLM.from_pretrained,
+ pretrained_model_name_or_path=pretrained_model_name_or_path,
+ trust_remote_code=True))
+
+#######################################################################
+# PART 3 Dataset & Dataloader #
+#######################################################################
+train_dataset = dict(
+ type=process_hf_dataset,
+ dataset=dict(type=load_dataset, path='json', data_files=data_files),
+ tokenizer=tokenizer,
+ max_length=max_length,
+ dataset_map_fn=pretrain_map_fn,
+ template_map_fn=None,
+ remove_unused_columns=True,
+ shuffle_before_pack=False,
+ pack_to_max_length=pack_to_max_length,
+ use_varlen_attn=use_varlen_attn)
+
+train_dataloader = dict(
+ batch_size=batch_size,
+ num_workers=dataloader_num_workers,
+ dataset=train_dataset,
+ sampler=dict(type=DefaultSampler, shuffle=True),
+ collate_fn=dict(type=default_collate_fn, use_varlen_attn=use_varlen_attn))
+
+#######################################################################
+# PART 4 Scheduler & Optimizer #
+#######################################################################
+# optimizer
+optim_wrapper = dict(
+ type=AmpOptimWrapper,
+ optimizer=dict(
+ type=optim_type, lr=lr, betas=betas, weight_decay=weight_decay),
+ clip_grad=dict(max_norm=max_norm, error_if_nonfinite=False),
+ accumulative_counts=accumulative_counts,
+ loss_scale='dynamic',
+ dtype='float16')
+
+# learning policy
+# More information: https://github.com/open-mmlab/mmengine/blob/main/docs/en/tutorials/param_scheduler.md # noqa: E501
+param_scheduler = [
+ dict(
+ type=LinearLR,
+ start_factor=1e-5,
+ by_epoch=True,
+ begin=0,
+ end=warmup_ratio * max_epochs,
+ convert_to_iter_based=True),
+ dict(
+ type=CosineAnnealingLR,
+ eta_min=0.0,
+ by_epoch=True,
+ begin=warmup_ratio * max_epochs,
+ end=max_epochs,
+ convert_to_iter_based=True)
+]
+
+# train, val, test setting
+train_cfg = dict(type=TrainLoop, max_epochs=max_epochs)
+
+#######################################################################
+# PART 5 Runtime #
+#######################################################################
+# Log the dialogue periodically during the training process, optional
+custom_hooks = [
+ dict(type=DatasetInfoHook, tokenizer=tokenizer),
+ dict(
+ type=EvaluateChatHook,
+ tokenizer=tokenizer,
+ every_n_iters=evaluation_freq,
+ evaluation_inputs=evaluation_inputs,
+ system=SYSTEM)
+]
+
+if use_varlen_attn:
+ custom_hooks += [dict(type=VarlenAttnArgsToMessageHubHook)]
+
+# configure default hooks
+default_hooks = dict(
+ # record the time of every iteration.
+ timer=dict(type=IterTimerHook),
+ # print log every 10 iterations.
+ logger=dict(type=LoggerHook, log_metric_by_epoch=False, interval=10),
+ # enable the parameter scheduler.
+ param_scheduler=dict(type=ParamSchedulerHook),
+ # save checkpoint per `save_steps`.
+ checkpoint=dict(
+ type=CheckpointHook,
+ by_epoch=False,
+ interval=save_steps,
+ max_keep_ckpts=save_total_limit),
+ # set sampler seed in distributed evrionment.
+ sampler_seed=dict(type=DistSamplerSeedHook),
+)
+
+# configure environment
+env_cfg = dict(
+ # whether to enable cudnn benchmark
+ cudnn_benchmark=False,
+ # set multi process parameters
+ mp_cfg=dict(mp_start_method='fork', opencv_num_threads=0),
+ # set distributed parameters
+ dist_cfg=dict(backend='nccl'),
+)
+
+# set visualizer
+visualizer = None
+
+# set log level
+log_level = 'INFO'
+
+# load from which checkpoint
+load_from = None
+
+# whether to resume training from the loaded checkpoint
+resume = False
+
+# Defaults to use random seed and disable `deterministic`
+randomness = dict(seed=None, deterministic=False)
+
+# set log processor
+log_processor = dict(by_epoch=False)
diff --git a/xtuner/configs/custom_dataset/pretrain/chatglm/chatglm3_6b_full_custom_pretrain_e1.py b/xtuner/configs/custom_dataset/pretrain/chatglm/chatglm3_6b_full_custom_pretrain_e1.py
new file mode 100644
index 000000000..174eb700b
--- /dev/null
+++ b/xtuner/configs/custom_dataset/pretrain/chatglm/chatglm3_6b_full_custom_pretrain_e1.py
@@ -0,0 +1,200 @@
+# Copyright (c) OpenMMLab. All rights reserved.
+"""Data format:
+
+[
+ {
+ "text": "xxx"
+ },
+ {
+ "text": "xxx"
+ },
+ ...
+]
+""" # noqa: E501
+
+from datasets import load_dataset
+from mmengine.dataset import DefaultSampler
+from mmengine.hooks import (CheckpointHook, DistSamplerSeedHook, IterTimerHook,
+ LoggerHook, ParamSchedulerHook)
+from mmengine.optim import AmpOptimWrapper, CosineAnnealingLR, LinearLR
+from torch.optim import AdamW
+from transformers import AutoModelForCausalLM, AutoTokenizer
+
+from xtuner.dataset import process_hf_dataset
+from xtuner.dataset.collate_fns import default_collate_fn
+from xtuner.dataset.map_fns import pretrain_map_fn
+from xtuner.engine.hooks import (DatasetInfoHook, EvaluateChatHook,
+ VarlenAttnArgsToMessageHubHook)
+from xtuner.engine.runner import TrainLoop
+from xtuner.model import SupervisedFinetune
+
+#######################################################################
+# PART 1 Settings #
+#######################################################################
+# Model
+pretrained_model_name_or_path = 'THUDM/chatglm3-6b'
+use_varlen_attn = False
+
+# Data
+data_files = ['/path/to/json/file.json']
+max_length = 2048
+pack_to_max_length = True
+
+# Scheduler & Optimizer
+batch_size = 1 # per_device
+accumulative_counts = 16 # bs = 1 GPU * 1 batch_size_per_device * 16 acc
+dataloader_num_workers = 0
+max_epochs = 1
+optim_type = AdamW
+lr = 2e-5
+betas = (0.9, 0.999)
+weight_decay = 0
+max_norm = 1 # grad clip
+warmup_ratio = 0.03
+
+# Save
+save_steps = 500
+save_total_limit = 2 # Maximum checkpoints to keep (-1 means unlimited)
+
+# Evaluate the generation performance during the training
+evaluation_freq = 500
+SYSTEM = ''
+evaluation_inputs = ['上海是', 'Shanghai is']
+
+#######################################################################
+# PART 2 Model & Tokenizer #
+#######################################################################
+tokenizer = dict(
+ type=AutoTokenizer.from_pretrained,
+ pretrained_model_name_or_path=pretrained_model_name_or_path,
+ trust_remote_code=True,
+ encode_special_tokens=True,
+ padding_side='left')
+
+model = dict(
+ type=SupervisedFinetune,
+ use_varlen_attn=use_varlen_attn,
+ llm=dict(
+ type=AutoModelForCausalLM.from_pretrained,
+ pretrained_model_name_or_path=pretrained_model_name_or_path,
+ trust_remote_code=True))
+
+#######################################################################
+# PART 3 Dataset & Dataloader #
+#######################################################################
+train_dataset = dict(
+ type=process_hf_dataset,
+ dataset=dict(type=load_dataset, path='json', data_files=data_files),
+ tokenizer=tokenizer,
+ max_length=max_length,
+ dataset_map_fn=pretrain_map_fn,
+ template_map_fn=None,
+ remove_unused_columns=True,
+ shuffle_before_pack=False,
+ pack_to_max_length=pack_to_max_length,
+ use_varlen_attn=use_varlen_attn)
+
+train_dataloader = dict(
+ batch_size=batch_size,
+ num_workers=dataloader_num_workers,
+ dataset=train_dataset,
+ sampler=dict(type=DefaultSampler, shuffle=True),
+ collate_fn=dict(type=default_collate_fn, use_varlen_attn=use_varlen_attn))
+
+#######################################################################
+# PART 4 Scheduler & Optimizer #
+#######################################################################
+# optimizer
+optim_wrapper = dict(
+ type=AmpOptimWrapper,
+ optimizer=dict(
+ type=optim_type, lr=lr, betas=betas, weight_decay=weight_decay),
+ clip_grad=dict(max_norm=max_norm, error_if_nonfinite=False),
+ accumulative_counts=accumulative_counts,
+ loss_scale='dynamic',
+ dtype='float16')
+
+# learning policy
+# More information: https://github.com/open-mmlab/mmengine/blob/main/docs/en/tutorials/param_scheduler.md # noqa: E501
+param_scheduler = [
+ dict(
+ type=LinearLR,
+ start_factor=1e-5,
+ by_epoch=True,
+ begin=0,
+ end=warmup_ratio * max_epochs,
+ convert_to_iter_based=True),
+ dict(
+ type=CosineAnnealingLR,
+ eta_min=0.0,
+ by_epoch=True,
+ begin=warmup_ratio * max_epochs,
+ end=max_epochs,
+ convert_to_iter_based=True)
+]
+
+# train, val, test setting
+train_cfg = dict(type=TrainLoop, max_epochs=max_epochs)
+
+#######################################################################
+# PART 5 Runtime #
+#######################################################################
+# Log the dialogue periodically during the training process, optional
+custom_hooks = [
+ dict(type=DatasetInfoHook, tokenizer=tokenizer),
+ dict(
+ type=EvaluateChatHook,
+ tokenizer=tokenizer,
+ every_n_iters=evaluation_freq,
+ evaluation_inputs=evaluation_inputs,
+ system=SYSTEM)
+]
+
+if use_varlen_attn:
+ custom_hooks += [dict(type=VarlenAttnArgsToMessageHubHook)]
+
+# configure default hooks
+default_hooks = dict(
+ # record the time of every iteration.
+ timer=dict(type=IterTimerHook),
+ # print log every 10 iterations.
+ logger=dict(type=LoggerHook, log_metric_by_epoch=False, interval=10),
+ # enable the parameter scheduler.
+ param_scheduler=dict(type=ParamSchedulerHook),
+ # save checkpoint per `save_steps`.
+ checkpoint=dict(
+ type=CheckpointHook,
+ by_epoch=False,
+ interval=save_steps,
+ max_keep_ckpts=save_total_limit),
+ # set sampler seed in distributed evrionment.
+ sampler_seed=dict(type=DistSamplerSeedHook),
+)
+
+# configure environment
+env_cfg = dict(
+ # whether to enable cudnn benchmark
+ cudnn_benchmark=False,
+ # set multi process parameters
+ mp_cfg=dict(mp_start_method='fork', opencv_num_threads=0),
+ # set distributed parameters
+ dist_cfg=dict(backend='nccl'),
+)
+
+# set visualizer
+visualizer = None
+
+# set log level
+log_level = 'INFO'
+
+# load from which checkpoint
+load_from = None
+
+# whether to resume training from the loaded checkpoint
+resume = False
+
+# Defaults to use random seed and disable `deterministic`
+randomness = dict(seed=None, deterministic=False)
+
+# set log processor
+log_processor = dict(by_epoch=False)
diff --git a/xtuner/configs/custom_dataset/pretrain/deepseek/deepseek_moe_16b_base_full_custom_pretrain_e1.py b/xtuner/configs/custom_dataset/pretrain/deepseek/deepseek_moe_16b_base_full_custom_pretrain_e1.py
new file mode 100644
index 000000000..4fbe2419d
--- /dev/null
+++ b/xtuner/configs/custom_dataset/pretrain/deepseek/deepseek_moe_16b_base_full_custom_pretrain_e1.py
@@ -0,0 +1,199 @@
+# Copyright (c) OpenMMLab. All rights reserved.
+"""Data format:
+
+[
+ {
+ "text": "xxx"
+ },
+ {
+ "text": "xxx"
+ },
+ ...
+]
+""" # noqa: E501
+
+from datasets import load_dataset
+from mmengine.dataset import DefaultSampler
+from mmengine.hooks import (CheckpointHook, DistSamplerSeedHook, IterTimerHook,
+ LoggerHook, ParamSchedulerHook)
+from mmengine.optim import AmpOptimWrapper, CosineAnnealingLR, LinearLR
+from torch.optim import AdamW
+from transformers import AutoModelForCausalLM, AutoTokenizer
+
+from xtuner.dataset import process_hf_dataset
+from xtuner.dataset.collate_fns import default_collate_fn
+from xtuner.dataset.map_fns import pretrain_map_fn
+from xtuner.engine.hooks import (DatasetInfoHook, EvaluateChatHook,
+ VarlenAttnArgsToMessageHubHook)
+from xtuner.engine.runner import TrainLoop
+from xtuner.model import SupervisedFinetune
+
+#######################################################################
+# PART 1 Settings #
+#######################################################################
+# Model
+pretrained_model_name_or_path = 'deepseek-ai/deepseek-moe-16b-base'
+use_varlen_attn = False
+
+# Data
+data_files = ['/path/to/json/file.json']
+max_length = 2048
+pack_to_max_length = True
+
+# Scheduler & Optimizer
+batch_size = 1 # per_device
+accumulative_counts = 16 # bs = 1 GPU * 1 batch_size_per_device * 16 acc
+dataloader_num_workers = 0
+max_epochs = 1
+optim_type = AdamW
+lr = 2e-5
+betas = (0.9, 0.999)
+weight_decay = 0
+max_norm = 1 # grad clip
+warmup_ratio = 0.03
+
+# Save
+save_steps = 500
+save_total_limit = 2 # Maximum checkpoints to keep (-1 means unlimited)
+
+# Evaluate the generation performance during the training
+evaluation_freq = 500
+SYSTEM = ''
+evaluation_inputs = ['上海是', 'Shanghai is']
+
+#######################################################################
+# PART 2 Model & Tokenizer #
+#######################################################################
+tokenizer = dict(
+ type=AutoTokenizer.from_pretrained,
+ pretrained_model_name_or_path=pretrained_model_name_or_path,
+ trust_remote_code=True,
+ padding_side='right')
+
+model = dict(
+ type=SupervisedFinetune,
+ use_varlen_attn=use_varlen_attn,
+ llm=dict(
+ type=AutoModelForCausalLM.from_pretrained,
+ pretrained_model_name_or_path=pretrained_model_name_or_path,
+ trust_remote_code=True))
+
+#######################################################################
+# PART 3 Dataset & Dataloader #
+#######################################################################
+train_dataset = dict(
+ type=process_hf_dataset,
+ dataset=dict(type=load_dataset, path='json', data_files=data_files),
+ tokenizer=tokenizer,
+ max_length=max_length,
+ dataset_map_fn=pretrain_map_fn,
+ template_map_fn=None,
+ remove_unused_columns=True,
+ shuffle_before_pack=False,
+ pack_to_max_length=pack_to_max_length,
+ use_varlen_attn=use_varlen_attn)
+
+train_dataloader = dict(
+ batch_size=batch_size,
+ num_workers=dataloader_num_workers,
+ dataset=train_dataset,
+ sampler=dict(type=DefaultSampler, shuffle=True),
+ collate_fn=dict(type=default_collate_fn, use_varlen_attn=use_varlen_attn))
+
+#######################################################################
+# PART 4 Scheduler & Optimizer #
+#######################################################################
+# optimizer
+optim_wrapper = dict(
+ type=AmpOptimWrapper,
+ optimizer=dict(
+ type=optim_type, lr=lr, betas=betas, weight_decay=weight_decay),
+ clip_grad=dict(max_norm=max_norm, error_if_nonfinite=False),
+ accumulative_counts=accumulative_counts,
+ loss_scale='dynamic',
+ dtype='float16')
+
+# learning policy
+# More information: https://github.com/open-mmlab/mmengine/blob/main/docs/en/tutorials/param_scheduler.md # noqa: E501
+param_scheduler = [
+ dict(
+ type=LinearLR,
+ start_factor=1e-5,
+ by_epoch=True,
+ begin=0,
+ end=warmup_ratio * max_epochs,
+ convert_to_iter_based=True),
+ dict(
+ type=CosineAnnealingLR,
+ eta_min=0.0,
+ by_epoch=True,
+ begin=warmup_ratio * max_epochs,
+ end=max_epochs,
+ convert_to_iter_based=True)
+]
+
+# train, val, test setting
+train_cfg = dict(type=TrainLoop, max_epochs=max_epochs)
+
+#######################################################################
+# PART 5 Runtime #
+#######################################################################
+# Log the dialogue periodically during the training process, optional
+custom_hooks = [
+ dict(type=DatasetInfoHook, tokenizer=tokenizer),
+ dict(
+ type=EvaluateChatHook,
+ tokenizer=tokenizer,
+ every_n_iters=evaluation_freq,
+ evaluation_inputs=evaluation_inputs,
+ system=SYSTEM)
+]
+
+if use_varlen_attn:
+ custom_hooks += [dict(type=VarlenAttnArgsToMessageHubHook)]
+
+# configure default hooks
+default_hooks = dict(
+ # record the time of every iteration.
+ timer=dict(type=IterTimerHook),
+ # print log every 10 iterations.
+ logger=dict(type=LoggerHook, log_metric_by_epoch=False, interval=10),
+ # enable the parameter scheduler.
+ param_scheduler=dict(type=ParamSchedulerHook),
+ # save checkpoint per `save_steps`.
+ checkpoint=dict(
+ type=CheckpointHook,
+ by_epoch=False,
+ interval=save_steps,
+ max_keep_ckpts=save_total_limit),
+ # set sampler seed in distributed evrionment.
+ sampler_seed=dict(type=DistSamplerSeedHook),
+)
+
+# configure environment
+env_cfg = dict(
+ # whether to enable cudnn benchmark
+ cudnn_benchmark=False,
+ # set multi process parameters
+ mp_cfg=dict(mp_start_method='fork', opencv_num_threads=0),
+ # set distributed parameters
+ dist_cfg=dict(backend='nccl'),
+)
+
+# set visualizer
+visualizer = None
+
+# set log level
+log_level = 'INFO'
+
+# load from which checkpoint
+load_from = None
+
+# whether to resume training from the loaded checkpoint
+resume = False
+
+# Defaults to use random seed and disable `deterministic`
+randomness = dict(seed=None, deterministic=False)
+
+# set log processor
+log_processor = dict(by_epoch=False)
diff --git a/xtuner/configs/custom_dataset/pretrain/gemma/gemma_2b_full_custom_pretrain_e1.py b/xtuner/configs/custom_dataset/pretrain/gemma/gemma_2b_full_custom_pretrain_e1.py
new file mode 100644
index 000000000..f2e38b481
--- /dev/null
+++ b/xtuner/configs/custom_dataset/pretrain/gemma/gemma_2b_full_custom_pretrain_e1.py
@@ -0,0 +1,199 @@
+# Copyright (c) OpenMMLab. All rights reserved.
+"""Data format:
+
+[
+ {
+ "text": "xxx"
+ },
+ {
+ "text": "xxx"
+ },
+ ...
+]
+""" # noqa: E501
+
+from datasets import load_dataset
+from mmengine.dataset import DefaultSampler
+from mmengine.hooks import (CheckpointHook, DistSamplerSeedHook, IterTimerHook,
+ LoggerHook, ParamSchedulerHook)
+from mmengine.optim import AmpOptimWrapper, CosineAnnealingLR, LinearLR
+from torch.optim import AdamW
+from transformers import AutoModelForCausalLM, AutoTokenizer
+
+from xtuner.dataset import process_hf_dataset
+from xtuner.dataset.collate_fns import default_collate_fn
+from xtuner.dataset.map_fns import pretrain_map_fn
+from xtuner.engine.hooks import (DatasetInfoHook, EvaluateChatHook,
+ VarlenAttnArgsToMessageHubHook)
+from xtuner.engine.runner import TrainLoop
+from xtuner.model import SupervisedFinetune
+
+#######################################################################
+# PART 1 Settings #
+#######################################################################
+# Model
+pretrained_model_name_or_path = 'google/gemma-2b' # Gemma requires transformers>=4.38.1 # noqa: E501
+use_varlen_attn = False
+
+# Data
+data_files = ['/path/to/json/file.json']
+max_length = 2048
+pack_to_max_length = True
+
+# Scheduler & Optimizer
+batch_size = 1 # per_device
+accumulative_counts = 16 # bs = 1 GPU * 1 batch_size_per_device * 16 acc
+dataloader_num_workers = 0
+max_epochs = 1
+optim_type = AdamW
+lr = 2e-5
+betas = (0.9, 0.999)
+weight_decay = 0
+max_norm = 1 # grad clip
+warmup_ratio = 0.03
+
+# Save
+save_steps = 500
+save_total_limit = 2 # Maximum checkpoints to keep (-1 means unlimited)
+
+# Evaluate the generation performance during the training
+evaluation_freq = 500
+SYSTEM = ''
+evaluation_inputs = ['上海是', 'Shanghai is']
+
+#######################################################################
+# PART 2 Model & Tokenizer #
+#######################################################################
+tokenizer = dict(
+ type=AutoTokenizer.from_pretrained,
+ pretrained_model_name_or_path=pretrained_model_name_or_path,
+ trust_remote_code=True,
+ padding_side='right')
+
+model = dict(
+ type=SupervisedFinetune,
+ use_varlen_attn=use_varlen_attn,
+ llm=dict(
+ type=AutoModelForCausalLM.from_pretrained,
+ pretrained_model_name_or_path=pretrained_model_name_or_path,
+ trust_remote_code=True))
+
+#######################################################################
+# PART 3 Dataset & Dataloader #
+#######################################################################
+train_dataset = dict(
+ type=process_hf_dataset,
+ dataset=dict(type=load_dataset, path='json', data_files=data_files),
+ tokenizer=tokenizer,
+ max_length=max_length,
+ dataset_map_fn=pretrain_map_fn,
+ template_map_fn=None,
+ remove_unused_columns=True,
+ shuffle_before_pack=False,
+ pack_to_max_length=pack_to_max_length,
+ use_varlen_attn=use_varlen_attn)
+
+train_dataloader = dict(
+ batch_size=batch_size,
+ num_workers=dataloader_num_workers,
+ dataset=train_dataset,
+ sampler=dict(type=DefaultSampler, shuffle=True),
+ collate_fn=dict(type=default_collate_fn, use_varlen_attn=use_varlen_attn))
+
+#######################################################################
+# PART 4 Scheduler & Optimizer #
+#######################################################################
+# optimizer
+optim_wrapper = dict(
+ type=AmpOptimWrapper,
+ optimizer=dict(
+ type=optim_type, lr=lr, betas=betas, weight_decay=weight_decay),
+ clip_grad=dict(max_norm=max_norm, error_if_nonfinite=False),
+ accumulative_counts=accumulative_counts,
+ loss_scale='dynamic',
+ dtype='float16')
+
+# learning policy
+# More information: https://github.com/open-mmlab/mmengine/blob/main/docs/en/tutorials/param_scheduler.md # noqa: E501
+param_scheduler = [
+ dict(
+ type=LinearLR,
+ start_factor=1e-5,
+ by_epoch=True,
+ begin=0,
+ end=warmup_ratio * max_epochs,
+ convert_to_iter_based=True),
+ dict(
+ type=CosineAnnealingLR,
+ eta_min=0.0,
+ by_epoch=True,
+ begin=warmup_ratio * max_epochs,
+ end=max_epochs,
+ convert_to_iter_based=True)
+]
+
+# train, val, test setting
+train_cfg = dict(type=TrainLoop, max_epochs=max_epochs)
+
+#######################################################################
+# PART 5 Runtime #
+#######################################################################
+# Log the dialogue periodically during the training process, optional
+custom_hooks = [
+ dict(type=DatasetInfoHook, tokenizer=tokenizer),
+ dict(
+ type=EvaluateChatHook,
+ tokenizer=tokenizer,
+ every_n_iters=evaluation_freq,
+ evaluation_inputs=evaluation_inputs,
+ system=SYSTEM)
+]
+
+if use_varlen_attn:
+ custom_hooks += [dict(type=VarlenAttnArgsToMessageHubHook)]
+
+# configure default hooks
+default_hooks = dict(
+ # record the time of every iteration.
+ timer=dict(type=IterTimerHook),
+ # print log every 10 iterations.
+ logger=dict(type=LoggerHook, log_metric_by_epoch=False, interval=10),
+ # enable the parameter scheduler.
+ param_scheduler=dict(type=ParamSchedulerHook),
+ # save checkpoint per `save_steps`.
+ checkpoint=dict(
+ type=CheckpointHook,
+ by_epoch=False,
+ interval=save_steps,
+ max_keep_ckpts=save_total_limit),
+ # set sampler seed in distributed evrionment.
+ sampler_seed=dict(type=DistSamplerSeedHook),
+)
+
+# configure environment
+env_cfg = dict(
+ # whether to enable cudnn benchmark
+ cudnn_benchmark=False,
+ # set multi process parameters
+ mp_cfg=dict(mp_start_method='fork', opencv_num_threads=0),
+ # set distributed parameters
+ dist_cfg=dict(backend='nccl'),
+)
+
+# set visualizer
+visualizer = None
+
+# set log level
+log_level = 'INFO'
+
+# load from which checkpoint
+load_from = None
+
+# whether to resume training from the loaded checkpoint
+resume = False
+
+# Defaults to use random seed and disable `deterministic`
+randomness = dict(seed=None, deterministic=False)
+
+# set log processor
+log_processor = dict(by_epoch=False)
diff --git a/xtuner/configs/custom_dataset/pretrain/gemma/gemma_7b_full_custom_pretrain_e1.py b/xtuner/configs/custom_dataset/pretrain/gemma/gemma_7b_full_custom_pretrain_e1.py
new file mode 100644
index 000000000..a7f9c3bd9
--- /dev/null
+++ b/xtuner/configs/custom_dataset/pretrain/gemma/gemma_7b_full_custom_pretrain_e1.py
@@ -0,0 +1,199 @@
+# Copyright (c) OpenMMLab. All rights reserved.
+"""Data format:
+
+[
+ {
+ "text": "xxx"
+ },
+ {
+ "text": "xxx"
+ },
+ ...
+]
+""" # noqa: E501
+
+from datasets import load_dataset
+from mmengine.dataset import DefaultSampler
+from mmengine.hooks import (CheckpointHook, DistSamplerSeedHook, IterTimerHook,
+ LoggerHook, ParamSchedulerHook)
+from mmengine.optim import AmpOptimWrapper, CosineAnnealingLR, LinearLR
+from torch.optim import AdamW
+from transformers import AutoModelForCausalLM, AutoTokenizer
+
+from xtuner.dataset import process_hf_dataset
+from xtuner.dataset.collate_fns import default_collate_fn
+from xtuner.dataset.map_fns import pretrain_map_fn
+from xtuner.engine.hooks import (DatasetInfoHook, EvaluateChatHook,
+ VarlenAttnArgsToMessageHubHook)
+from xtuner.engine.runner import TrainLoop
+from xtuner.model import SupervisedFinetune
+
+#######################################################################
+# PART 1 Settings #
+#######################################################################
+# Model
+pretrained_model_name_or_path = 'google/gemma-7b' # Gemma requires transformers>=4.38.1 # noqa: E501
+use_varlen_attn = False
+
+# Data
+data_files = ['/path/to/json/file.json']
+max_length = 2048
+pack_to_max_length = True
+
+# Scheduler & Optimizer
+batch_size = 1 # per_device
+accumulative_counts = 16 # bs = 1 GPU * 1 batch_size_per_device * 16 acc
+dataloader_num_workers = 0
+max_epochs = 1
+optim_type = AdamW
+lr = 2e-5
+betas = (0.9, 0.999)
+weight_decay = 0
+max_norm = 1 # grad clip
+warmup_ratio = 0.03
+
+# Save
+save_steps = 500
+save_total_limit = 2 # Maximum checkpoints to keep (-1 means unlimited)
+
+# Evaluate the generation performance during the training
+evaluation_freq = 500
+SYSTEM = ''
+evaluation_inputs = ['上海是', 'Shanghai is']
+
+#######################################################################
+# PART 2 Model & Tokenizer #
+#######################################################################
+tokenizer = dict(
+ type=AutoTokenizer.from_pretrained,
+ pretrained_model_name_or_path=pretrained_model_name_or_path,
+ trust_remote_code=True,
+ padding_side='right')
+
+model = dict(
+ type=SupervisedFinetune,
+ use_varlen_attn=use_varlen_attn,
+ llm=dict(
+ type=AutoModelForCausalLM.from_pretrained,
+ pretrained_model_name_or_path=pretrained_model_name_or_path,
+ trust_remote_code=True))
+
+#######################################################################
+# PART 3 Dataset & Dataloader #
+#######################################################################
+train_dataset = dict(
+ type=process_hf_dataset,
+ dataset=dict(type=load_dataset, path='json', data_files=data_files),
+ tokenizer=tokenizer,
+ max_length=max_length,
+ dataset_map_fn=pretrain_map_fn,
+ template_map_fn=None,
+ remove_unused_columns=True,
+ shuffle_before_pack=False,
+ pack_to_max_length=pack_to_max_length,
+ use_varlen_attn=use_varlen_attn)
+
+train_dataloader = dict(
+ batch_size=batch_size,
+ num_workers=dataloader_num_workers,
+ dataset=train_dataset,
+ sampler=dict(type=DefaultSampler, shuffle=True),
+ collate_fn=dict(type=default_collate_fn, use_varlen_attn=use_varlen_attn))
+
+#######################################################################
+# PART 4 Scheduler & Optimizer #
+#######################################################################
+# optimizer
+optim_wrapper = dict(
+ type=AmpOptimWrapper,
+ optimizer=dict(
+ type=optim_type, lr=lr, betas=betas, weight_decay=weight_decay),
+ clip_grad=dict(max_norm=max_norm, error_if_nonfinite=False),
+ accumulative_counts=accumulative_counts,
+ loss_scale='dynamic',
+ dtype='float16')
+
+# learning policy
+# More information: https://github.com/open-mmlab/mmengine/blob/main/docs/en/tutorials/param_scheduler.md # noqa: E501
+param_scheduler = [
+ dict(
+ type=LinearLR,
+ start_factor=1e-5,
+ by_epoch=True,
+ begin=0,
+ end=warmup_ratio * max_epochs,
+ convert_to_iter_based=True),
+ dict(
+ type=CosineAnnealingLR,
+ eta_min=0.0,
+ by_epoch=True,
+ begin=warmup_ratio * max_epochs,
+ end=max_epochs,
+ convert_to_iter_based=True)
+]
+
+# train, val, test setting
+train_cfg = dict(type=TrainLoop, max_epochs=max_epochs)
+
+#######################################################################
+# PART 5 Runtime #
+#######################################################################
+# Log the dialogue periodically during the training process, optional
+custom_hooks = [
+ dict(type=DatasetInfoHook, tokenizer=tokenizer),
+ dict(
+ type=EvaluateChatHook,
+ tokenizer=tokenizer,
+ every_n_iters=evaluation_freq,
+ evaluation_inputs=evaluation_inputs,
+ system=SYSTEM)
+]
+
+if use_varlen_attn:
+ custom_hooks += [dict(type=VarlenAttnArgsToMessageHubHook)]
+
+# configure default hooks
+default_hooks = dict(
+ # record the time of every iteration.
+ timer=dict(type=IterTimerHook),
+ # print log every 10 iterations.
+ logger=dict(type=LoggerHook, log_metric_by_epoch=False, interval=10),
+ # enable the parameter scheduler.
+ param_scheduler=dict(type=ParamSchedulerHook),
+ # save checkpoint per `save_steps`.
+ checkpoint=dict(
+ type=CheckpointHook,
+ by_epoch=False,
+ interval=save_steps,
+ max_keep_ckpts=save_total_limit),
+ # set sampler seed in distributed evrionment.
+ sampler_seed=dict(type=DistSamplerSeedHook),
+)
+
+# configure environment
+env_cfg = dict(
+ # whether to enable cudnn benchmark
+ cudnn_benchmark=False,
+ # set multi process parameters
+ mp_cfg=dict(mp_start_method='fork', opencv_num_threads=0),
+ # set distributed parameters
+ dist_cfg=dict(backend='nccl'),
+)
+
+# set visualizer
+visualizer = None
+
+# set log level
+log_level = 'INFO'
+
+# load from which checkpoint
+load_from = None
+
+# whether to resume training from the loaded checkpoint
+resume = False
+
+# Defaults to use random seed and disable `deterministic`
+randomness = dict(seed=None, deterministic=False)
+
+# set log processor
+log_processor = dict(by_epoch=False)
diff --git a/xtuner/configs/custom_dataset/pretrain/internlm/internlm2_1_8b_full_custom_pretrain_e1.py b/xtuner/configs/custom_dataset/pretrain/internlm/internlm2_1_8b_full_custom_pretrain_e1.py
new file mode 100644
index 000000000..ea900f0e9
--- /dev/null
+++ b/xtuner/configs/custom_dataset/pretrain/internlm/internlm2_1_8b_full_custom_pretrain_e1.py
@@ -0,0 +1,199 @@
+# Copyright (c) OpenMMLab. All rights reserved.
+"""Data format:
+
+[
+ {
+ "text": "xxx"
+ },
+ {
+ "text": "xxx"
+ },
+ ...
+]
+""" # noqa: E501
+
+from datasets import load_dataset
+from mmengine.dataset import DefaultSampler
+from mmengine.hooks import (CheckpointHook, DistSamplerSeedHook, IterTimerHook,
+ LoggerHook, ParamSchedulerHook)
+from mmengine.optim import AmpOptimWrapper, CosineAnnealingLR, LinearLR
+from torch.optim import AdamW
+from transformers import AutoModelForCausalLM, AutoTokenizer
+
+from xtuner.dataset import process_hf_dataset
+from xtuner.dataset.collate_fns import default_collate_fn
+from xtuner.dataset.map_fns import pretrain_map_fn
+from xtuner.engine.hooks import (DatasetInfoHook, EvaluateChatHook,
+ VarlenAttnArgsToMessageHubHook)
+from xtuner.engine.runner import TrainLoop
+from xtuner.model import SupervisedFinetune
+
+#######################################################################
+# PART 1 Settings #
+#######################################################################
+# Model
+pretrained_model_name_or_path = 'internlm/internlm2-1_8b'
+use_varlen_attn = False
+
+# Data
+data_files = ['/path/to/json/file.json']
+max_length = 2048
+pack_to_max_length = True
+
+# Scheduler & Optimizer
+batch_size = 1 # per_device
+accumulative_counts = 16 # bs = 1 GPU * 1 batch_size_per_device * 16 acc
+dataloader_num_workers = 0
+max_epochs = 1
+optim_type = AdamW
+lr = 2e-5
+betas = (0.9, 0.999)
+weight_decay = 0
+max_norm = 1 # grad clip
+warmup_ratio = 0.03
+
+# Save
+save_steps = 500
+save_total_limit = 2 # Maximum checkpoints to keep (-1 means unlimited)
+
+# Evaluate the generation performance during the training
+evaluation_freq = 500
+SYSTEM = ''
+evaluation_inputs = ['上海是', 'Shanghai is']
+
+#######################################################################
+# PART 2 Model & Tokenizer #
+#######################################################################
+tokenizer = dict(
+ type=AutoTokenizer.from_pretrained,
+ pretrained_model_name_or_path=pretrained_model_name_or_path,
+ trust_remote_code=True,
+ padding_side='right')
+
+model = dict(
+ type=SupervisedFinetune,
+ use_varlen_attn=use_varlen_attn,
+ llm=dict(
+ type=AutoModelForCausalLM.from_pretrained,
+ pretrained_model_name_or_path=pretrained_model_name_or_path,
+ trust_remote_code=True))
+
+#######################################################################
+# PART 3 Dataset & Dataloader #
+#######################################################################
+train_dataset = dict(
+ type=process_hf_dataset,
+ dataset=dict(type=load_dataset, path='json', data_files=data_files),
+ tokenizer=tokenizer,
+ max_length=max_length,
+ dataset_map_fn=pretrain_map_fn,
+ template_map_fn=None,
+ remove_unused_columns=True,
+ shuffle_before_pack=False,
+ pack_to_max_length=pack_to_max_length,
+ use_varlen_attn=use_varlen_attn)
+
+train_dataloader = dict(
+ batch_size=batch_size,
+ num_workers=dataloader_num_workers,
+ dataset=train_dataset,
+ sampler=dict(type=DefaultSampler, shuffle=True),
+ collate_fn=dict(type=default_collate_fn, use_varlen_attn=use_varlen_attn))
+
+#######################################################################
+# PART 4 Scheduler & Optimizer #
+#######################################################################
+# optimizer
+optim_wrapper = dict(
+ type=AmpOptimWrapper,
+ optimizer=dict(
+ type=optim_type, lr=lr, betas=betas, weight_decay=weight_decay),
+ clip_grad=dict(max_norm=max_norm, error_if_nonfinite=False),
+ accumulative_counts=accumulative_counts,
+ loss_scale='dynamic',
+ dtype='float16')
+
+# learning policy
+# More information: https://github.com/open-mmlab/mmengine/blob/main/docs/en/tutorials/param_scheduler.md # noqa: E501
+param_scheduler = [
+ dict(
+ type=LinearLR,
+ start_factor=1e-5,
+ by_epoch=True,
+ begin=0,
+ end=warmup_ratio * max_epochs,
+ convert_to_iter_based=True),
+ dict(
+ type=CosineAnnealingLR,
+ eta_min=0.0,
+ by_epoch=True,
+ begin=warmup_ratio * max_epochs,
+ end=max_epochs,
+ convert_to_iter_based=True)
+]
+
+# train, val, test setting
+train_cfg = dict(type=TrainLoop, max_epochs=max_epochs)
+
+#######################################################################
+# PART 5 Runtime #
+#######################################################################
+# Log the dialogue periodically during the training process, optional
+custom_hooks = [
+ dict(type=DatasetInfoHook, tokenizer=tokenizer),
+ dict(
+ type=EvaluateChatHook,
+ tokenizer=tokenizer,
+ every_n_iters=evaluation_freq,
+ evaluation_inputs=evaluation_inputs,
+ system=SYSTEM)
+]
+
+if use_varlen_attn:
+ custom_hooks += [dict(type=VarlenAttnArgsToMessageHubHook)]
+
+# configure default hooks
+default_hooks = dict(
+ # record the time of every iteration.
+ timer=dict(type=IterTimerHook),
+ # print log every 10 iterations.
+ logger=dict(type=LoggerHook, log_metric_by_epoch=False, interval=10),
+ # enable the parameter scheduler.
+ param_scheduler=dict(type=ParamSchedulerHook),
+ # save checkpoint per `save_steps`.
+ checkpoint=dict(
+ type=CheckpointHook,
+ by_epoch=False,
+ interval=save_steps,
+ max_keep_ckpts=save_total_limit),
+ # set sampler seed in distributed evrionment.
+ sampler_seed=dict(type=DistSamplerSeedHook),
+)
+
+# configure environment
+env_cfg = dict(
+ # whether to enable cudnn benchmark
+ cudnn_benchmark=False,
+ # set multi process parameters
+ mp_cfg=dict(mp_start_method='fork', opencv_num_threads=0),
+ # set distributed parameters
+ dist_cfg=dict(backend='nccl'),
+)
+
+# set visualizer
+visualizer = None
+
+# set log level
+log_level = 'INFO'
+
+# load from which checkpoint
+load_from = None
+
+# whether to resume training from the loaded checkpoint
+resume = False
+
+# Defaults to use random seed and disable `deterministic`
+randomness = dict(seed=None, deterministic=False)
+
+# set log processor
+log_processor = dict(by_epoch=False)
diff --git a/xtuner/configs/custom_dataset/pretrain/internlm/internlm2_20b_full_custom_pretrain_e1.py b/xtuner/configs/custom_dataset/pretrain/internlm/internlm2_20b_full_custom_pretrain_e1.py
new file mode 100644
index 000000000..35592294a
--- /dev/null
+++ b/xtuner/configs/custom_dataset/pretrain/internlm/internlm2_20b_full_custom_pretrain_e1.py
@@ -0,0 +1,199 @@
+# Copyright (c) OpenMMLab. All rights reserved.
+"""Data format:
+
+[
+ {
+ "text": "xxx"
+ },
+ {
+ "text": "xxx"
+ },
+ ...
+]
+""" # noqa: E501
+
+from datasets import load_dataset
+from mmengine.dataset import DefaultSampler
+from mmengine.hooks import (CheckpointHook, DistSamplerSeedHook, IterTimerHook,
+ LoggerHook, ParamSchedulerHook)
+from mmengine.optim import AmpOptimWrapper, CosineAnnealingLR, LinearLR
+from torch.optim import AdamW
+from transformers import AutoModelForCausalLM, AutoTokenizer
+
+from xtuner.dataset import process_hf_dataset
+from xtuner.dataset.collate_fns import default_collate_fn
+from xtuner.dataset.map_fns import pretrain_map_fn
+from xtuner.engine.hooks import (DatasetInfoHook, EvaluateChatHook,
+ VarlenAttnArgsToMessageHubHook)
+from xtuner.engine.runner import TrainLoop
+from xtuner.model import SupervisedFinetune
+
+#######################################################################
+# PART 1 Settings #
+#######################################################################
+# Model
+pretrained_model_name_or_path = 'internlm/internlm2-20b'
+use_varlen_attn = False
+
+# Data
+data_files = ['/path/to/json/file.json']
+max_length = 2048
+pack_to_max_length = True
+
+# Scheduler & Optimizer
+batch_size = 1 # per_device
+accumulative_counts = 16 # bs = 1 GPU * 1 batch_size_per_device * 16 acc
+dataloader_num_workers = 0
+max_epochs = 1
+optim_type = AdamW
+lr = 2e-5
+betas = (0.9, 0.999)
+weight_decay = 0
+max_norm = 1 # grad clip
+warmup_ratio = 0.03
+
+# Save
+save_steps = 500
+save_total_limit = 2 # Maximum checkpoints to keep (-1 means unlimited)
+
+# Evaluate the generation performance during the training
+evaluation_freq = 500
+SYSTEM = ''
+evaluation_inputs = ['上海是', 'Shanghai is']
+
+#######################################################################
+# PART 2 Model & Tokenizer #
+#######################################################################
+tokenizer = dict(
+ type=AutoTokenizer.from_pretrained,
+ pretrained_model_name_or_path=pretrained_model_name_or_path,
+ trust_remote_code=True,
+ padding_side='right')
+
+model = dict(
+ type=SupervisedFinetune,
+ use_varlen_attn=use_varlen_attn,
+ llm=dict(
+ type=AutoModelForCausalLM.from_pretrained,
+ pretrained_model_name_or_path=pretrained_model_name_or_path,
+ trust_remote_code=True))
+
+#######################################################################
+# PART 3 Dataset & Dataloader #
+#######################################################################
+train_dataset = dict(
+ type=process_hf_dataset,
+ dataset=dict(type=load_dataset, path='json', data_files=data_files),
+ tokenizer=tokenizer,
+ max_length=max_length,
+ dataset_map_fn=pretrain_map_fn,
+ template_map_fn=None,
+ remove_unused_columns=True,
+ shuffle_before_pack=False,
+ pack_to_max_length=pack_to_max_length,
+ use_varlen_attn=use_varlen_attn)
+
+train_dataloader = dict(
+ batch_size=batch_size,
+ num_workers=dataloader_num_workers,
+ dataset=train_dataset,
+ sampler=dict(type=DefaultSampler, shuffle=True),
+ collate_fn=dict(type=default_collate_fn, use_varlen_attn=use_varlen_attn))
+
+#######################################################################
+# PART 4 Scheduler & Optimizer #
+#######################################################################
+# optimizer
+optim_wrapper = dict(
+ type=AmpOptimWrapper,
+ optimizer=dict(
+ type=optim_type, lr=lr, betas=betas, weight_decay=weight_decay),
+ clip_grad=dict(max_norm=max_norm, error_if_nonfinite=False),
+ accumulative_counts=accumulative_counts,
+ loss_scale='dynamic',
+ dtype='float16')
+
+# learning policy
+# More information: https://github.com/open-mmlab/mmengine/blob/main/docs/en/tutorials/param_scheduler.md # noqa: E501
+param_scheduler = [
+ dict(
+ type=LinearLR,
+ start_factor=1e-5,
+ by_epoch=True,
+ begin=0,
+ end=warmup_ratio * max_epochs,
+ convert_to_iter_based=True),
+ dict(
+ type=CosineAnnealingLR,
+ eta_min=0.0,
+ by_epoch=True,
+ begin=warmup_ratio * max_epochs,
+ end=max_epochs,
+ convert_to_iter_based=True)
+]
+
+# train, val, test setting
+train_cfg = dict(type=TrainLoop, max_epochs=max_epochs)
+
+#######################################################################
+# PART 5 Runtime #
+#######################################################################
+# Log the dialogue periodically during the training process, optional
+custom_hooks = [
+ dict(type=DatasetInfoHook, tokenizer=tokenizer),
+ dict(
+ type=EvaluateChatHook,
+ tokenizer=tokenizer,
+ every_n_iters=evaluation_freq,
+ evaluation_inputs=evaluation_inputs,
+ system=SYSTEM)
+]
+
+if use_varlen_attn:
+ custom_hooks += [dict(type=VarlenAttnArgsToMessageHubHook)]
+
+# configure default hooks
+default_hooks = dict(
+ # record the time of every iteration.
+ timer=dict(type=IterTimerHook),
+ # print log every 10 iterations.
+ logger=dict(type=LoggerHook, log_metric_by_epoch=False, interval=10),
+ # enable the parameter scheduler.
+ param_scheduler=dict(type=ParamSchedulerHook),
+ # save checkpoint per `save_steps`.
+ checkpoint=dict(
+ type=CheckpointHook,
+ by_epoch=False,
+ interval=save_steps,
+ max_keep_ckpts=save_total_limit),
+ # set sampler seed in distributed evrionment.
+ sampler_seed=dict(type=DistSamplerSeedHook),
+)
+
+# configure environment
+env_cfg = dict(
+ # whether to enable cudnn benchmark
+ cudnn_benchmark=False,
+ # set multi process parameters
+ mp_cfg=dict(mp_start_method='fork', opencv_num_threads=0),
+ # set distributed parameters
+ dist_cfg=dict(backend='nccl'),
+)
+
+# set visualizer
+visualizer = None
+
+# set log level
+log_level = 'INFO'
+
+# load from which checkpoint
+load_from = None
+
+# whether to resume training from the loaded checkpoint
+resume = False
+
+# Defaults to use random seed and disable `deterministic`
+randomness = dict(seed=None, deterministic=False)
+
+# set log processor
+log_processor = dict(by_epoch=False)
diff --git a/xtuner/configs/custom_dataset/pretrain/internlm/internlm2_7b_full_custom_pretrain_e1.py b/xtuner/configs/custom_dataset/pretrain/internlm/internlm2_7b_full_custom_pretrain_e1.py
new file mode 100644
index 000000000..ff212d7e3
--- /dev/null
+++ b/xtuner/configs/custom_dataset/pretrain/internlm/internlm2_7b_full_custom_pretrain_e1.py
@@ -0,0 +1,199 @@
+# Copyright (c) OpenMMLab. All rights reserved.
+"""Data format:
+
+[
+ {
+ "text": "xxx"
+ },
+ {
+ "text": "xxx"
+ },
+ ...
+]
+""" # noqa: E501
+
+from datasets import load_dataset
+from mmengine.dataset import DefaultSampler
+from mmengine.hooks import (CheckpointHook, DistSamplerSeedHook, IterTimerHook,
+ LoggerHook, ParamSchedulerHook)
+from mmengine.optim import AmpOptimWrapper, CosineAnnealingLR, LinearLR
+from torch.optim import AdamW
+from transformers import AutoModelForCausalLM, AutoTokenizer
+
+from xtuner.dataset import process_hf_dataset
+from xtuner.dataset.collate_fns import default_collate_fn
+from xtuner.dataset.map_fns import pretrain_map_fn
+from xtuner.engine.hooks import (DatasetInfoHook, EvaluateChatHook,
+ VarlenAttnArgsToMessageHubHook)
+from xtuner.engine.runner import TrainLoop
+from xtuner.model import SupervisedFinetune
+
+#######################################################################
+# PART 1 Settings #
+#######################################################################
+# Model
+pretrained_model_name_or_path = 'internlm/internlm2-7b'
+use_varlen_attn = False
+
+# Data
+data_files = ['/path/to/json/file.json']
+max_length = 2048
+pack_to_max_length = True
+
+# Scheduler & Optimizer
+batch_size = 1 # per_device
+accumulative_counts = 16 # bs = 1 GPU * 1 batch_size_per_device * 16 acc
+dataloader_num_workers = 0
+max_epochs = 1
+optim_type = AdamW
+lr = 2e-5
+betas = (0.9, 0.999)
+weight_decay = 0
+max_norm = 1 # grad clip
+warmup_ratio = 0.03
+
+# Save
+save_steps = 500
+save_total_limit = 2 # Maximum checkpoints to keep (-1 means unlimited)
+
+# Evaluate the generation performance during the training
+evaluation_freq = 500
+SYSTEM = ''
+evaluation_inputs = ['上海是', 'Shanghai is']
+
+#######################################################################
+# PART 2 Model & Tokenizer #
+#######################################################################
+tokenizer = dict(
+ type=AutoTokenizer.from_pretrained,
+ pretrained_model_name_or_path=pretrained_model_name_or_path,
+ trust_remote_code=True,
+ padding_side='right')
+
+model = dict(
+ type=SupervisedFinetune,
+ use_varlen_attn=use_varlen_attn,
+ llm=dict(
+ type=AutoModelForCausalLM.from_pretrained,
+ pretrained_model_name_or_path=pretrained_model_name_or_path,
+ trust_remote_code=True))
+
+#######################################################################
+# PART 3 Dataset & Dataloader #
+#######################################################################
+train_dataset = dict(
+ type=process_hf_dataset,
+ dataset=dict(type=load_dataset, path='json', data_files=data_files),
+ tokenizer=tokenizer,
+ max_length=max_length,
+ dataset_map_fn=pretrain_map_fn,
+ template_map_fn=None,
+ remove_unused_columns=True,
+ shuffle_before_pack=False,
+ pack_to_max_length=pack_to_max_length,
+ use_varlen_attn=use_varlen_attn)
+
+train_dataloader = dict(
+ batch_size=batch_size,
+ num_workers=dataloader_num_workers,
+ dataset=train_dataset,
+ sampler=dict(type=DefaultSampler, shuffle=True),
+ collate_fn=dict(type=default_collate_fn, use_varlen_attn=use_varlen_attn))
+
+#######################################################################
+# PART 4 Scheduler & Optimizer #
+#######################################################################
+# optimizer
+optim_wrapper = dict(
+ type=AmpOptimWrapper,
+ optimizer=dict(
+ type=optim_type, lr=lr, betas=betas, weight_decay=weight_decay),
+ clip_grad=dict(max_norm=max_norm, error_if_nonfinite=False),
+ accumulative_counts=accumulative_counts,
+ loss_scale='dynamic',
+ dtype='float16')
+
+# learning policy
+# More information: https://github.com/open-mmlab/mmengine/blob/main/docs/en/tutorials/param_scheduler.md # noqa: E501
+param_scheduler = [
+ dict(
+ type=LinearLR,
+ start_factor=1e-5,
+ by_epoch=True,
+ begin=0,
+ end=warmup_ratio * max_epochs,
+ convert_to_iter_based=True),
+ dict(
+ type=CosineAnnealingLR,
+ eta_min=0.0,
+ by_epoch=True,
+ begin=warmup_ratio * max_epochs,
+ end=max_epochs,
+ convert_to_iter_based=True)
+]
+
+# train, val, test setting
+train_cfg = dict(type=TrainLoop, max_epochs=max_epochs)
+
+#######################################################################
+# PART 5 Runtime #
+#######################################################################
+# Log the dialogue periodically during the training process, optional
+custom_hooks = [
+ dict(type=DatasetInfoHook, tokenizer=tokenizer),
+ dict(
+ type=EvaluateChatHook,
+ tokenizer=tokenizer,
+ every_n_iters=evaluation_freq,
+ evaluation_inputs=evaluation_inputs,
+ system=SYSTEM)
+]
+
+if use_varlen_attn:
+ custom_hooks += [dict(type=VarlenAttnArgsToMessageHubHook)]
+
+# configure default hooks
+default_hooks = dict(
+ # record the time of every iteration.
+ timer=dict(type=IterTimerHook),
+ # print log every 10 iterations.
+ logger=dict(type=LoggerHook, log_metric_by_epoch=False, interval=10),
+ # enable the parameter scheduler.
+ param_scheduler=dict(type=ParamSchedulerHook),
+ # save checkpoint per `save_steps`.
+ checkpoint=dict(
+ type=CheckpointHook,
+ by_epoch=False,
+ interval=save_steps,
+ max_keep_ckpts=save_total_limit),
+ # set sampler seed in distributed evrionment.
+ sampler_seed=dict(type=DistSamplerSeedHook),
+)
+
+# configure environment
+env_cfg = dict(
+ # whether to enable cudnn benchmark
+ cudnn_benchmark=False,
+ # set multi process parameters
+ mp_cfg=dict(mp_start_method='fork', opencv_num_threads=0),
+ # set distributed parameters
+ dist_cfg=dict(backend='nccl'),
+)
+
+# set visualizer
+visualizer = None
+
+# set log level
+log_level = 'INFO'
+
+# load from which checkpoint
+load_from = None
+
+# whether to resume training from the loaded checkpoint
+resume = False
+
+# Defaults to use random seed and disable `deterministic`
+randomness = dict(seed=None, deterministic=False)
+
+# set log processor
+log_processor = dict(by_epoch=False)
diff --git a/xtuner/configs/custom_dataset/pretrain/llama/llama2_70b_full_custom_pretrain_e1.py b/xtuner/configs/custom_dataset/pretrain/llama/llama2_70b_full_custom_pretrain_e1.py
new file mode 100644
index 000000000..66ee04e64
--- /dev/null
+++ b/xtuner/configs/custom_dataset/pretrain/llama/llama2_70b_full_custom_pretrain_e1.py
@@ -0,0 +1,199 @@
+# Copyright (c) OpenMMLab. All rights reserved.
+"""Data format:
+
+[
+ {
+ "text": "xxx"
+ },
+ {
+ "text": "xxx"
+ },
+ ...
+]
+""" # noqa: E501
+
+from datasets import load_dataset
+from mmengine.dataset import DefaultSampler
+from mmengine.hooks import (CheckpointHook, DistSamplerSeedHook, IterTimerHook,
+ LoggerHook, ParamSchedulerHook)
+from mmengine.optim import AmpOptimWrapper, CosineAnnealingLR, LinearLR
+from torch.optim import AdamW
+from transformers import AutoModelForCausalLM, AutoTokenizer
+
+from xtuner.dataset import process_hf_dataset
+from xtuner.dataset.collate_fns import default_collate_fn
+from xtuner.dataset.map_fns import pretrain_map_fn
+from xtuner.engine.hooks import (DatasetInfoHook, EvaluateChatHook,
+ VarlenAttnArgsToMessageHubHook)
+from xtuner.engine.runner import TrainLoop
+from xtuner.model import SupervisedFinetune
+
+#######################################################################
+# PART 1 Settings #
+#######################################################################
+# Model
+pretrained_model_name_or_path = 'meta-llama/Llama-2-70b-hf'
+use_varlen_attn = False
+
+# Data
+data_files = ['/path/to/json/file.json']
+max_length = 2048
+pack_to_max_length = True
+
+# Scheduler & Optimizer
+batch_size = 1 # per_device
+accumulative_counts = 16 # bs = 1 GPU * 1 batch_size_per_device * 16 acc
+dataloader_num_workers = 0
+max_epochs = 1
+optim_type = AdamW
+lr = 2e-5
+betas = (0.9, 0.999)
+weight_decay = 0
+max_norm = 1 # grad clip
+warmup_ratio = 0.03
+
+# Save
+save_steps = 500
+save_total_limit = 2 # Maximum checkpoints to keep (-1 means unlimited)
+
+# Evaluate the generation performance during the training
+evaluation_freq = 500
+SYSTEM = ''
+evaluation_inputs = ['上海是', 'Shanghai is']
+
+#######################################################################
+# PART 2 Model & Tokenizer #
+#######################################################################
+tokenizer = dict(
+ type=AutoTokenizer.from_pretrained,
+ pretrained_model_name_or_path=pretrained_model_name_or_path,
+ trust_remote_code=True,
+ padding_side='right')
+
+model = dict(
+ type=SupervisedFinetune,
+ use_varlen_attn=use_varlen_attn,
+ llm=dict(
+ type=AutoModelForCausalLM.from_pretrained,
+ pretrained_model_name_or_path=pretrained_model_name_or_path,
+ trust_remote_code=True))
+
+#######################################################################
+# PART 3 Dataset & Dataloader #
+#######################################################################
+train_dataset = dict(
+ type=process_hf_dataset,
+ dataset=dict(type=load_dataset, path='json', data_files=data_files),
+ tokenizer=tokenizer,
+ max_length=max_length,
+ dataset_map_fn=pretrain_map_fn,
+ template_map_fn=None,
+ remove_unused_columns=True,
+ shuffle_before_pack=False,
+ pack_to_max_length=pack_to_max_length,
+ use_varlen_attn=use_varlen_attn)
+
+train_dataloader = dict(
+ batch_size=batch_size,
+ num_workers=dataloader_num_workers,
+ dataset=train_dataset,
+ sampler=dict(type=DefaultSampler, shuffle=True),
+ collate_fn=dict(type=default_collate_fn, use_varlen_attn=use_varlen_attn))
+
+#######################################################################
+# PART 4 Scheduler & Optimizer #
+#######################################################################
+# optimizer
+optim_wrapper = dict(
+ type=AmpOptimWrapper,
+ optimizer=dict(
+ type=optim_type, lr=lr, betas=betas, weight_decay=weight_decay),
+ clip_grad=dict(max_norm=max_norm, error_if_nonfinite=False),
+ accumulative_counts=accumulative_counts,
+ loss_scale='dynamic',
+ dtype='float16')
+
+# learning policy
+# More information: https://github.com/open-mmlab/mmengine/blob/main/docs/en/tutorials/param_scheduler.md # noqa: E501
+param_scheduler = [
+ dict(
+ type=LinearLR,
+ start_factor=1e-5,
+ by_epoch=True,
+ begin=0,
+ end=warmup_ratio * max_epochs,
+ convert_to_iter_based=True),
+ dict(
+ type=CosineAnnealingLR,
+ eta_min=0.0,
+ by_epoch=True,
+ begin=warmup_ratio * max_epochs,
+ end=max_epochs,
+ convert_to_iter_based=True)
+]
+
+# train, val, test setting
+train_cfg = dict(type=TrainLoop, max_epochs=max_epochs)
+
+#######################################################################
+# PART 5 Runtime #
+#######################################################################
+# Log the dialogue periodically during the training process, optional
+custom_hooks = [
+ dict(type=DatasetInfoHook, tokenizer=tokenizer),
+ dict(
+ type=EvaluateChatHook,
+ tokenizer=tokenizer,
+ every_n_iters=evaluation_freq,
+ evaluation_inputs=evaluation_inputs,
+ system=SYSTEM)
+]
+
+if use_varlen_attn:
+ custom_hooks += [dict(type=VarlenAttnArgsToMessageHubHook)]
+
+# configure default hooks
+default_hooks = dict(
+ # record the time of every iteration.
+ timer=dict(type=IterTimerHook),
+ # print log every 10 iterations.
+ logger=dict(type=LoggerHook, log_metric_by_epoch=False, interval=10),
+ # enable the parameter scheduler.
+ param_scheduler=dict(type=ParamSchedulerHook),
+ # save checkpoint per `save_steps`.
+ checkpoint=dict(
+ type=CheckpointHook,
+ by_epoch=False,
+ interval=save_steps,
+ max_keep_ckpts=save_total_limit),
+ # set sampler seed in distributed evrionment.
+ sampler_seed=dict(type=DistSamplerSeedHook),
+)
+
+# configure environment
+env_cfg = dict(
+ # whether to enable cudnn benchmark
+ cudnn_benchmark=False,
+ # set multi process parameters
+ mp_cfg=dict(mp_start_method='fork', opencv_num_threads=0),
+ # set distributed parameters
+ dist_cfg=dict(backend='nccl'),
+)
+
+# set visualizer
+visualizer = None
+
+# set log level
+log_level = 'INFO'
+
+# load from which checkpoint
+load_from = None
+
+# whether to resume training from the loaded checkpoint
+resume = False
+
+# Defaults to use random seed and disable `deterministic`
+randomness = dict(seed=None, deterministic=False)
+
+# set log processor
+log_processor = dict(by_epoch=False)
diff --git a/xtuner/configs/custom_dataset/pretrain/llama/llama2_7b_full_custom_pretrain_e1.py b/xtuner/configs/custom_dataset/pretrain/llama/llama2_7b_full_custom_pretrain_e1.py
new file mode 100644
index 000000000..b752fc8c5
--- /dev/null
+++ b/xtuner/configs/custom_dataset/pretrain/llama/llama2_7b_full_custom_pretrain_e1.py
@@ -0,0 +1,199 @@
+# Copyright (c) OpenMMLab. All rights reserved.
+"""Data format:
+
+[
+ {
+ "text": "xxx"
+ },
+ {
+ "text": "xxx"
+ },
+ ...
+]
+""" # noqa: E501
+
+from datasets import load_dataset
+from mmengine.dataset import DefaultSampler
+from mmengine.hooks import (CheckpointHook, DistSamplerSeedHook, IterTimerHook,
+ LoggerHook, ParamSchedulerHook)
+from mmengine.optim import AmpOptimWrapper, CosineAnnealingLR, LinearLR
+from torch.optim import AdamW
+from transformers import AutoModelForCausalLM, AutoTokenizer
+
+from xtuner.dataset import process_hf_dataset
+from xtuner.dataset.collate_fns import default_collate_fn
+from xtuner.dataset.map_fns import pretrain_map_fn
+from xtuner.engine.hooks import (DatasetInfoHook, EvaluateChatHook,
+ VarlenAttnArgsToMessageHubHook)
+from xtuner.engine.runner import TrainLoop
+from xtuner.model import SupervisedFinetune
+
+#######################################################################
+# PART 1 Settings #
+#######################################################################
+# Model
+pretrained_model_name_or_path = 'meta-llama/Llama-2-7b-hf'
+use_varlen_attn = False
+
+# Data
+data_files = ['/path/to/json/file.json']
+max_length = 2048
+pack_to_max_length = True
+
+# Scheduler & Optimizer
+batch_size = 1 # per_device
+accumulative_counts = 16 # bs = 1 GPU * 1 batch_size_per_device * 16 acc
+dataloader_num_workers = 0
+max_epochs = 1
+optim_type = AdamW
+lr = 2e-5
+betas = (0.9, 0.999)
+weight_decay = 0
+max_norm = 1 # grad clip
+warmup_ratio = 0.03
+
+# Save
+save_steps = 500
+save_total_limit = 2 # Maximum checkpoints to keep (-1 means unlimited)
+
+# Evaluate the generation performance during the training
+evaluation_freq = 500
+SYSTEM = ''
+evaluation_inputs = ['上海是', 'Shanghai is']
+
+#######################################################################
+# PART 2 Model & Tokenizer #
+#######################################################################
+tokenizer = dict(
+ type=AutoTokenizer.from_pretrained,
+ pretrained_model_name_or_path=pretrained_model_name_or_path,
+ trust_remote_code=True,
+ padding_side='right')
+
+model = dict(
+ type=SupervisedFinetune,
+ use_varlen_attn=use_varlen_attn,
+ llm=dict(
+ type=AutoModelForCausalLM.from_pretrained,
+ pretrained_model_name_or_path=pretrained_model_name_or_path,
+ trust_remote_code=True))
+
+#######################################################################
+# PART 3 Dataset & Dataloader #
+#######################################################################
+train_dataset = dict(
+ type=process_hf_dataset,
+ dataset=dict(type=load_dataset, path='json', data_files=data_files),
+ tokenizer=tokenizer,
+ max_length=max_length,
+ dataset_map_fn=pretrain_map_fn,
+ template_map_fn=None,
+ remove_unused_columns=True,
+ shuffle_before_pack=False,
+ pack_to_max_length=pack_to_max_length,
+ use_varlen_attn=use_varlen_attn)
+
+train_dataloader = dict(
+ batch_size=batch_size,
+ num_workers=dataloader_num_workers,
+ dataset=train_dataset,
+ sampler=dict(type=DefaultSampler, shuffle=True),
+ collate_fn=dict(type=default_collate_fn, use_varlen_attn=use_varlen_attn))
+
+#######################################################################
+# PART 4 Scheduler & Optimizer #
+#######################################################################
+# optimizer
+optim_wrapper = dict(
+ type=AmpOptimWrapper,
+ optimizer=dict(
+ type=optim_type, lr=lr, betas=betas, weight_decay=weight_decay),
+ clip_grad=dict(max_norm=max_norm, error_if_nonfinite=False),
+ accumulative_counts=accumulative_counts,
+ loss_scale='dynamic',
+ dtype='float16')
+
+# learning policy
+# More information: https://github.com/open-mmlab/mmengine/blob/main/docs/en/tutorials/param_scheduler.md # noqa: E501
+param_scheduler = [
+ dict(
+ type=LinearLR,
+ start_factor=1e-5,
+ by_epoch=True,
+ begin=0,
+ end=warmup_ratio * max_epochs,
+ convert_to_iter_based=True),
+ dict(
+ type=CosineAnnealingLR,
+ eta_min=0.0,
+ by_epoch=True,
+ begin=warmup_ratio * max_epochs,
+ end=max_epochs,
+ convert_to_iter_based=True)
+]
+
+# train, val, test setting
+train_cfg = dict(type=TrainLoop, max_epochs=max_epochs)
+
+#######################################################################
+# PART 5 Runtime #
+#######################################################################
+# Log the dialogue periodically during the training process, optional
+custom_hooks = [
+ dict(type=DatasetInfoHook, tokenizer=tokenizer),
+ dict(
+ type=EvaluateChatHook,
+ tokenizer=tokenizer,
+ every_n_iters=evaluation_freq,
+ evaluation_inputs=evaluation_inputs,
+ system=SYSTEM)
+]
+
+if use_varlen_attn:
+ custom_hooks += [dict(type=VarlenAttnArgsToMessageHubHook)]
+
+# configure default hooks
+default_hooks = dict(
+ # record the time of every iteration.
+ timer=dict(type=IterTimerHook),
+ # print log every 10 iterations.
+ logger=dict(type=LoggerHook, log_metric_by_epoch=False, interval=10),
+ # enable the parameter scheduler.
+ param_scheduler=dict(type=ParamSchedulerHook),
+ # save checkpoint per `save_steps`.
+ checkpoint=dict(
+ type=CheckpointHook,
+ by_epoch=False,
+ interval=save_steps,
+ max_keep_ckpts=save_total_limit),
+ # set sampler seed in distributed evrionment.
+ sampler_seed=dict(type=DistSamplerSeedHook),
+)
+
+# configure environment
+env_cfg = dict(
+ # whether to enable cudnn benchmark
+ cudnn_benchmark=False,
+ # set multi process parameters
+ mp_cfg=dict(mp_start_method='fork', opencv_num_threads=0),
+ # set distributed parameters
+ dist_cfg=dict(backend='nccl'),
+)
+
+# set visualizer
+visualizer = None
+
+# set log level
+log_level = 'INFO'
+
+# load from which checkpoint
+load_from = None
+
+# whether to resume training from the loaded checkpoint
+resume = False
+
+# Defaults to use random seed and disable `deterministic`
+randomness = dict(seed=None, deterministic=False)
+
+# set log processor
+log_processor = dict(by_epoch=False)
diff --git a/xtuner/configs/custom_dataset/pretrain/minicpm/minicpm3_4b_full_custom_pretrain_e1.py b/xtuner/configs/custom_dataset/pretrain/minicpm/minicpm3_4b_full_custom_pretrain_e1.py
new file mode 100644
index 000000000..936b48f4a
--- /dev/null
+++ b/xtuner/configs/custom_dataset/pretrain/minicpm/minicpm3_4b_full_custom_pretrain_e1.py
@@ -0,0 +1,216 @@
+# Copyright (c) OpenMMLab. All rights reserved.
+"""Data format:
+
+[
+ {
+ "text": "xxx"
+ },
+ {
+ "text": "xxx"
+ },
+ ...
+]
+""" # noqa: E501
+
+from datasets import load_dataset
+from mmengine.dataset import DefaultSampler
+from mmengine.hooks import (
+ CheckpointHook,
+ DistSamplerSeedHook,
+ IterTimerHook,
+ LoggerHook,
+ ParamSchedulerHook,
+)
+from mmengine.optim import AmpOptimWrapper, CosineAnnealingLR, LinearLR
+from torch.optim import AdamW
+from transformers import AutoModelForCausalLM, AutoTokenizer
+
+from xtuner.dataset import process_hf_dataset
+from xtuner.dataset.collate_fns import default_collate_fn
+from xtuner.dataset.map_fns import pretrain_map_fn
+from xtuner.engine.hooks import (
+ DatasetInfoHook,
+ EvaluateChatHook,
+ VarlenAttnArgsToMessageHubHook,
+)
+from xtuner.engine.runner import TrainLoop
+from xtuner.model import SupervisedFinetune
+
+#######################################################################
+# PART 1 Settings #
+#######################################################################
+# Model
+pretrained_model_name_or_path = "openbmb/MiniCPM3-4B"
+use_varlen_attn = False
+
+# Data
+data_files = ["/path/to/your.json"]
+max_length = 1024
+pack_to_max_length = True
+
+# Scheduler & Optimizer
+batch_size = 1 # per_device
+accumulative_counts = 1 # bs = 1 GPU * 1 batch_size_per_device * 16 acc
+dataloader_num_workers = 0
+max_steps = 10000
+optim_type = AdamW
+lr = 2e-5
+betas = (0.9, 0.999)
+weight_decay = 0
+max_norm = 1 # grad clip
+warmup_ratio = 0.03
+
+# Save
+save_steps = 500
+save_total_limit = 2 # Maximum checkpoints to keep (-1 means unlimited)
+
+# Evaluate the generation performance during the training
+evaluation_freq = 500
+SYSTEM = ""
+evaluation_inputs = ["上海是", "Shanghai is"]
+
+#######################################################################
+# PART 2 Model & Tokenizer #
+#######################################################################
+tokenizer = dict(
+ type=AutoTokenizer.from_pretrained,
+ pretrained_model_name_or_path=pretrained_model_name_or_path,
+ trust_remote_code=True,
+ padding_side="right",
+ eos_token="<|im_end|>",
+)
+
+model = dict(
+ type=SupervisedFinetune,
+ use_varlen_attn=use_varlen_attn,
+ llm=dict(
+ type=AutoModelForCausalLM.from_pretrained,
+ pretrained_model_name_or_path=pretrained_model_name_or_path,
+ trust_remote_code=True,
+ ),
+)
+
+#######################################################################
+# PART 3 Dataset & Dataloader #
+#######################################################################
+train_dataset = dict(
+ type=process_hf_dataset,
+ dataset=dict(type=load_dataset, path="json", data_files=data_files),
+ tokenizer=tokenizer,
+ max_length=max_length,
+ dataset_map_fn=pretrain_map_fn,
+ template_map_fn=None,
+ remove_unused_columns=True,
+ shuffle_before_pack=False,
+ pack_to_max_length=pack_to_max_length,
+ use_varlen_attn=use_varlen_attn,
+)
+
+train_dataloader = dict(
+ batch_size=batch_size,
+ num_workers=dataloader_num_workers,
+ dataset=train_dataset,
+ sampler=dict(type=DefaultSampler, shuffle=True),
+ collate_fn=dict(type=default_collate_fn, use_varlen_attn=use_varlen_attn),
+)
+
+#######################################################################
+# PART 4 Scheduler & Optimizer #
+#######################################################################
+# optimizer
+optim_wrapper = dict(
+ type=AmpOptimWrapper,
+ optimizer=dict(type=optim_type, lr=lr, betas=betas, weight_decay=weight_decay),
+ clip_grad=dict(max_norm=max_norm, error_if_nonfinite=False),
+ accumulative_counts=accumulative_counts,
+ loss_scale="dynamic",
+ dtype="float16",
+)
+
+# learning policy
+param_scheduler = [
+ dict(
+ type=LinearLR,
+ start_factor=1e-5,
+ by_epoch=True,
+ begin=0,
+ end=max_steps * warmup_ratio,
+ convert_to_iter_based=True,
+ ),
+ dict(
+ type=CosineAnnealingLR,
+ eta_min=0.0,
+ by_epoch=True,
+ begin=max_steps * warmup_ratio,
+ end=max_steps,
+ convert_to_iter_based=True,
+ ),
+]
+
+# train, val, test setting
+train_cfg = dict(type=TrainLoop, max_iters=max_steps)
+
+#######################################################################
+# PART 5 Runtime #
+#######################################################################
+# Log the dialogue periodically during the training process, optional
+custom_hooks = [
+ dict(type=DatasetInfoHook, tokenizer=tokenizer),
+ dict(
+ type=EvaluateChatHook,
+ tokenizer=tokenizer,
+ every_n_iters=evaluation_freq,
+ evaluation_inputs=evaluation_inputs,
+ system=SYSTEM,
+ ),
+]
+
+if use_varlen_attn:
+ custom_hooks += [dict(type=VarlenAttnArgsToMessageHubHook)]
+
+# configure default hooks
+default_hooks = dict(
+ # record the time of every iteration.
+ timer=dict(type=IterTimerHook),
+ # print log every 10 iterations.
+ logger=dict(type=LoggerHook, log_metric_by_epoch=False, interval=10),
+ # enable the parameter scheduler.
+ param_scheduler=dict(type=ParamSchedulerHook),
+ # save checkpoint per `save_steps`.
+ checkpoint=dict(
+ type=CheckpointHook,
+ by_epoch=False,
+ interval=save_steps,
+ max_keep_ckpts=save_total_limit,
+ ),
+ # set sampler seed in distributed evrionment.
+ sampler_seed=dict(type=DistSamplerSeedHook),
+)
+
+# configure environment
+env_cfg = dict(
+ # whether to enable cudnn benchmark
+ cudnn_benchmark=False,
+ # set multi process parameters
+ mp_cfg=dict(mp_start_method="fork", opencv_num_threads=0),
+ # set distributed parameters
+ dist_cfg=dict(backend="nccl"),
+)
+
+# set visualizer
+visualizer = None
+
+# set log level
+log_level = "INFO"
+
+# load from which checkpoint
+load_from = None
+
+# whether to resume training from the loaded checkpoint
+resume = False
+
+# Defaults to use random seed and disable `deterministic`
+randomness = dict(seed=None, deterministic=False)
+
+# set log processor
+log_processor = dict(by_epoch=False)
diff --git a/xtuner/configs/custom_dataset/pretrain/minicpm/minicpm_1b_full_custom_pretrain_e1.py b/xtuner/configs/custom_dataset/pretrain/minicpm/minicpm_1b_full_custom_pretrain_e1.py
new file mode 100644
index 000000000..fc0da5ed3
--- /dev/null
+++ b/xtuner/configs/custom_dataset/pretrain/minicpm/minicpm_1b_full_custom_pretrain_e1.py
@@ -0,0 +1,200 @@
+# Copyright (c) OpenMMLab. All rights reserved.
+"""Data format:
+
+[
+ {
+ "text": "xxx"
+ },
+ {
+ "text": "xxx"
+ },
+ ...
+]
+""" # noqa: E501
+
+from datasets import load_dataset
+from mmengine.dataset import DefaultSampler
+from mmengine.hooks import (CheckpointHook, DistSamplerSeedHook, IterTimerHook,
+ LoggerHook, ParamSchedulerHook)
+from mmengine.optim import AmpOptimWrapper, CosineAnnealingLR, LinearLR
+from torch.optim import AdamW
+from transformers import AutoModelForCausalLM, AutoTokenizer
+
+from xtuner.dataset import process_hf_dataset
+from xtuner.dataset.collate_fns import default_collate_fn
+from xtuner.dataset.map_fns import pretrain_map_fn
+from xtuner.engine.hooks import (DatasetInfoHook, EvaluateChatHook,
+ VarlenAttnArgsToMessageHubHook)
+from xtuner.engine.runner import TrainLoop
+from xtuner.model import SupervisedFinetune
+
+#######################################################################
+# PART 1 Settings #
+#######################################################################
+# Model
+pretrained_model_name_or_path = 'openbmb/MiniCPM-1B-sft-bf16'
+use_varlen_attn = False
+
+# Data
+data_files = ['/path/to/json/file.json']
+max_length = 2048
+pack_to_max_length = True
+
+# Scheduler & Optimizer
+batch_size = 1 # per_device
+accumulative_counts = 1 # bs = 1 GPU * 1 batch_size_per_device * 16 acc
+dataloader_num_workers = 0
+max_epochs = 1
+optim_type = AdamW
+lr = 2e-5
+betas = (0.9, 0.999)
+weight_decay = 0
+max_norm = 1 # grad clip
+warmup_ratio = 0.03
+
+# Save
+save_steps = 500
+save_total_limit = 2 # Maximum checkpoints to keep (-1 means unlimited)
+
+# Evaluate the generation performance during the training
+evaluation_freq = 500
+SYSTEM = ''
+evaluation_inputs = ['上海是', 'Shanghai is']
+
+#######################################################################
+# PART 2 Model & Tokenizer #
+#######################################################################
+tokenizer = dict(
+ type=AutoTokenizer.from_pretrained,
+ pretrained_model_name_or_path=pretrained_model_name_or_path,
+ trust_remote_code=True,
+ padding_side='right',
+ eos_token='')
+
+model = dict(
+ type=SupervisedFinetune,
+ use_varlen_attn=use_varlen_attn,
+ llm=dict(
+ type=AutoModelForCausalLM.from_pretrained,
+ pretrained_model_name_or_path=pretrained_model_name_or_path,
+ trust_remote_code=True))
+
+#######################################################################
+# PART 3 Dataset & Dataloader #
+#######################################################################
+train_dataset = dict(
+ type=process_hf_dataset,
+ dataset=dict(type=load_dataset, path='json', data_files=data_files),
+ tokenizer=tokenizer,
+ max_length=max_length,
+ dataset_map_fn=pretrain_map_fn,
+ template_map_fn=None,
+ remove_unused_columns=True,
+ shuffle_before_pack=False,
+ pack_to_max_length=pack_to_max_length,
+ use_varlen_attn=use_varlen_attn)
+
+train_dataloader = dict(
+ batch_size=batch_size,
+ num_workers=dataloader_num_workers,
+ dataset=train_dataset,
+ sampler=dict(type=DefaultSampler, shuffle=True),
+ collate_fn=dict(type=default_collate_fn, use_varlen_attn=use_varlen_attn))
+
+#######################################################################
+# PART 4 Scheduler & Optimizer #
+#######################################################################
+# optimizer
+optim_wrapper = dict(
+ type=AmpOptimWrapper,
+ optimizer=dict(
+ type=optim_type, lr=lr, betas=betas, weight_decay=weight_decay),
+ clip_grad=dict(max_norm=max_norm, error_if_nonfinite=False),
+ accumulative_counts=accumulative_counts,
+ loss_scale='dynamic',
+ dtype='float16')
+
+# learning policy
+# More information: https://github.com/open-mmlab/mmengine/blob/main/docs/en/tutorials/param_scheduler.md # noqa: E501
+param_scheduler = [
+ dict(
+ type=LinearLR,
+ start_factor=1e-5,
+ by_epoch=True,
+ begin=0,
+ end=warmup_ratio * max_epochs,
+ convert_to_iter_based=True),
+ dict(
+ type=CosineAnnealingLR,
+ eta_min=0.0,
+ by_epoch=True,
+ begin=warmup_ratio * max_epochs,
+ end=max_epochs,
+ convert_to_iter_based=True)
+]
+
+# train, val, test setting
+train_cfg = dict(type=TrainLoop, max_epochs=max_epochs)
+
+#######################################################################
+# PART 5 Runtime #
+#######################################################################
+# Log the dialogue periodically during the training process, optional
+custom_hooks = [
+ dict(type=DatasetInfoHook, tokenizer=tokenizer),
+ dict(
+ type=EvaluateChatHook,
+ tokenizer=tokenizer,
+ every_n_iters=evaluation_freq,
+ evaluation_inputs=evaluation_inputs,
+ system=SYSTEM)
+]
+
+if use_varlen_attn:
+ custom_hooks += [dict(type=VarlenAttnArgsToMessageHubHook)]
+
+# configure default hooks
+default_hooks = dict(
+ # record the time of every iteration.
+ timer=dict(type=IterTimerHook),
+ # print log every 10 iterations.
+ logger=dict(type=LoggerHook, log_metric_by_epoch=False, interval=10),
+ # enable the parameter scheduler.
+ param_scheduler=dict(type=ParamSchedulerHook),
+ # save checkpoint per `save_steps`.
+ checkpoint=dict(
+ type=CheckpointHook,
+ by_epoch=False,
+ interval=save_steps,
+ max_keep_ckpts=save_total_limit),
+ # set sampler seed in distributed evrionment.
+ sampler_seed=dict(type=DistSamplerSeedHook),
+)
+
+# configure environment
+env_cfg = dict(
+ # whether to enable cudnn benchmark
+ cudnn_benchmark=False,
+ # set multi process parameters
+ mp_cfg=dict(mp_start_method='fork', opencv_num_threads=0),
+ # set distributed parameters
+ dist_cfg=dict(backend='nccl'),
+)
+
+# set visualizer
+visualizer = None
+
+# set log level
+log_level = 'INFO'
+
+# load from which checkpoint
+load_from = None
+
+# whether to resume training from the loaded checkpoint
+resume = False
+
+# Defaults to use random seed and disable `deterministic`
+randomness = dict(seed=None, deterministic=False)
+
+# set log processor
+log_processor = dict(by_epoch=False)
diff --git a/xtuner/configs/custom_dataset/pretrain/minicpm/minicpm_2b_full_custom_pretrain_e1.py b/xtuner/configs/custom_dataset/pretrain/minicpm/minicpm_2b_full_custom_pretrain_e1.py
new file mode 100644
index 000000000..160495a86
--- /dev/null
+++ b/xtuner/configs/custom_dataset/pretrain/minicpm/minicpm_2b_full_custom_pretrain_e1.py
@@ -0,0 +1,200 @@
+# Copyright (c) OpenMMLab. All rights reserved.
+"""Data format:
+
+[
+ {
+ "text": "xxx"
+ },
+ {
+ "text": "xxx"
+ },
+ ...
+]
+""" # noqa: E501
+
+from datasets import load_dataset
+from mmengine.dataset import DefaultSampler
+from mmengine.hooks import (CheckpointHook, DistSamplerSeedHook, IterTimerHook,
+ LoggerHook, ParamSchedulerHook)
+from mmengine.optim import AmpOptimWrapper, CosineAnnealingLR, LinearLR
+from torch.optim import AdamW
+from transformers import AutoModelForCausalLM, AutoTokenizer
+
+from xtuner.dataset import process_hf_dataset
+from xtuner.dataset.collate_fns import default_collate_fn
+from xtuner.dataset.map_fns import pretrain_map_fn
+from xtuner.engine.hooks import (DatasetInfoHook, EvaluateChatHook,
+ VarlenAttnArgsToMessageHubHook)
+from xtuner.engine.runner import TrainLoop
+from xtuner.model import SupervisedFinetune
+
+#######################################################################
+# PART 1 Settings #
+#######################################################################
+# Model
+pretrained_model_name_or_path = 'openbmb/MiniCPM-2B-sft-bf16'
+use_varlen_attn = False
+
+# Data
+data_files = ['/path/to/json/file.json']
+max_length = 2048
+pack_to_max_length = True
+
+# Scheduler & Optimizer
+batch_size = 1 # per_device
+accumulative_counts = 16 # bs = 1 GPU * 1 batch_size_per_device * 16 acc
+dataloader_num_workers = 0
+max_epochs = 1
+optim_type = AdamW
+lr = 2e-5
+betas = (0.9, 0.999)
+weight_decay = 0
+max_norm = 1 # grad clip
+warmup_ratio = 0.03
+
+# Save
+save_steps = 500
+save_total_limit = 2 # Maximum checkpoints to keep (-1 means unlimited)
+
+# Evaluate the generation performance during the training
+evaluation_freq = 500
+SYSTEM = ''
+evaluation_inputs = ['上海是', 'Shanghai is']
+
+#######################################################################
+# PART 2 Model & Tokenizer #
+#######################################################################
+tokenizer = dict(
+ type=AutoTokenizer.from_pretrained,
+ pretrained_model_name_or_path=pretrained_model_name_or_path,
+ trust_remote_code=True,
+ padding_side='right',
+ eos_token='')
+
+model = dict(
+ type=SupervisedFinetune,
+ use_varlen_attn=use_varlen_attn,
+ llm=dict(
+ type=AutoModelForCausalLM.from_pretrained,
+ pretrained_model_name_or_path=pretrained_model_name_or_path,
+ trust_remote_code=True))
+
+#######################################################################
+# PART 3 Dataset & Dataloader #
+#######################################################################
+train_dataset = dict(
+ type=process_hf_dataset,
+ dataset=dict(type=load_dataset, path='json', data_files=data_files),
+ tokenizer=tokenizer,
+ max_length=max_length,
+ dataset_map_fn=pretrain_map_fn,
+ template_map_fn=None,
+ remove_unused_columns=True,
+ shuffle_before_pack=False,
+ pack_to_max_length=pack_to_max_length,
+ use_varlen_attn=use_varlen_attn)
+
+train_dataloader = dict(
+ batch_size=batch_size,
+ num_workers=dataloader_num_workers,
+ dataset=train_dataset,
+ sampler=dict(type=DefaultSampler, shuffle=True),
+ collate_fn=dict(type=default_collate_fn, use_varlen_attn=use_varlen_attn))
+
+#######################################################################
+# PART 4 Scheduler & Optimizer #
+#######################################################################
+# optimizer
+optim_wrapper = dict(
+ type=AmpOptimWrapper,
+ optimizer=dict(
+ type=optim_type, lr=lr, betas=betas, weight_decay=weight_decay),
+ clip_grad=dict(max_norm=max_norm, error_if_nonfinite=False),
+ accumulative_counts=accumulative_counts,
+ loss_scale='dynamic',
+ dtype='float16')
+
+# learning policy
+# More information: https://github.com/open-mmlab/mmengine/blob/main/docs/en/tutorials/param_scheduler.md # noqa: E501
+param_scheduler = [
+ dict(
+ type=LinearLR,
+ start_factor=1e-5,
+ by_epoch=True,
+ begin=0,
+ end=warmup_ratio * max_epochs,
+ convert_to_iter_based=True),
+ dict(
+ type=CosineAnnealingLR,
+ eta_min=0.0,
+ by_epoch=True,
+ begin=warmup_ratio * max_epochs,
+ end=max_epochs,
+ convert_to_iter_based=True)
+]
+
+# train, val, test setting
+train_cfg = dict(type=TrainLoop, max_epochs=max_epochs)
+
+#######################################################################
+# PART 5 Runtime #
+#######################################################################
+# Log the dialogue periodically during the training process, optional
+custom_hooks = [
+ dict(type=DatasetInfoHook, tokenizer=tokenizer),
+ dict(
+ type=EvaluateChatHook,
+ tokenizer=tokenizer,
+ every_n_iters=evaluation_freq,
+ evaluation_inputs=evaluation_inputs,
+ system=SYSTEM)
+]
+
+if use_varlen_attn:
+ custom_hooks += [dict(type=VarlenAttnArgsToMessageHubHook)]
+
+# configure default hooks
+default_hooks = dict(
+ # record the time of every iteration.
+ timer=dict(type=IterTimerHook),
+ # print log every 10 iterations.
+ logger=dict(type=LoggerHook, log_metric_by_epoch=False, interval=10),
+ # enable the parameter scheduler.
+ param_scheduler=dict(type=ParamSchedulerHook),
+ # save checkpoint per `save_steps`.
+ checkpoint=dict(
+ type=CheckpointHook,
+ by_epoch=False,
+ interval=save_steps,
+ max_keep_ckpts=save_total_limit),
+ # set sampler seed in distributed evrionment.
+ sampler_seed=dict(type=DistSamplerSeedHook),
+)
+
+# configure environment
+env_cfg = dict(
+ # whether to enable cudnn benchmark
+ cudnn_benchmark=False,
+ # set multi process parameters
+ mp_cfg=dict(mp_start_method='fork', opencv_num_threads=0),
+ # set distributed parameters
+ dist_cfg=dict(backend='nccl'),
+)
+
+# set visualizer
+visualizer = None
+
+# set log level
+log_level = 'INFO'
+
+# load from which checkpoint
+load_from = None
+
+# whether to resume training from the loaded checkpoint
+resume = False
+
+# Defaults to use random seed and disable `deterministic`
+randomness = dict(seed=None, deterministic=False)
+
+# set log processor
+log_processor = dict(by_epoch=False)
diff --git a/xtuner/configs/custom_dataset/pretrain/mistral/mistral_7b_full_custom_pretrain_e1.py b/xtuner/configs/custom_dataset/pretrain/mistral/mistral_7b_full_custom_pretrain_e1.py
new file mode 100644
index 000000000..197841816
--- /dev/null
+++ b/xtuner/configs/custom_dataset/pretrain/mistral/mistral_7b_full_custom_pretrain_e1.py
@@ -0,0 +1,199 @@
+# Copyright (c) OpenMMLab. All rights reserved.
+"""Data format:
+
+[
+ {
+ "text": "xxx"
+ },
+ {
+ "text": "xxx"
+ },
+ ...
+]
+""" # noqa: E501
+
+from datasets import load_dataset
+from mmengine.dataset import DefaultSampler
+from mmengine.hooks import (CheckpointHook, DistSamplerSeedHook, IterTimerHook,
+ LoggerHook, ParamSchedulerHook)
+from mmengine.optim import AmpOptimWrapper, CosineAnnealingLR, LinearLR
+from torch.optim import AdamW
+from transformers import AutoModelForCausalLM, AutoTokenizer
+
+from xtuner.dataset import process_hf_dataset
+from xtuner.dataset.collate_fns import default_collate_fn
+from xtuner.dataset.map_fns import pretrain_map_fn
+from xtuner.engine.hooks import (DatasetInfoHook, EvaluateChatHook,
+ VarlenAttnArgsToMessageHubHook)
+from xtuner.engine.runner import TrainLoop
+from xtuner.model import SupervisedFinetune
+
+#######################################################################
+# PART 1 Settings #
+#######################################################################
+# Model
+pretrained_model_name_or_path = 'mistralai/Mistral-7B-v0.1'
+use_varlen_attn = False
+
+# Data
+data_files = ['/path/to/json/file.json']
+max_length = 2048
+pack_to_max_length = True
+
+# Scheduler & Optimizer
+batch_size = 1 # per_device
+accumulative_counts = 16 # bs = 1 GPU * 1 batch_size_per_device * 16 acc
+dataloader_num_workers = 0
+max_epochs = 1
+optim_type = AdamW
+lr = 2e-5
+betas = (0.9, 0.999)
+weight_decay = 0
+max_norm = 1 # grad clip
+warmup_ratio = 0.03
+
+# Save
+save_steps = 500
+save_total_limit = 2 # Maximum checkpoints to keep (-1 means unlimited)
+
+# Evaluate the generation performance during the training
+evaluation_freq = 500
+SYSTEM = ''
+evaluation_inputs = ['上海是', 'Shanghai is']
+
+#######################################################################
+# PART 2 Model & Tokenizer #
+#######################################################################
+tokenizer = dict(
+ type=AutoTokenizer.from_pretrained,
+ pretrained_model_name_or_path=pretrained_model_name_or_path,
+ trust_remote_code=True,
+ padding_side='right')
+
+model = dict(
+ type=SupervisedFinetune,
+ use_varlen_attn=use_varlen_attn,
+ llm=dict(
+ type=AutoModelForCausalLM.from_pretrained,
+ pretrained_model_name_or_path=pretrained_model_name_or_path,
+ trust_remote_code=True))
+
+#######################################################################
+# PART 3 Dataset & Dataloader #
+#######################################################################
+train_dataset = dict(
+ type=process_hf_dataset,
+ dataset=dict(type=load_dataset, path='json', data_files=data_files),
+ tokenizer=tokenizer,
+ max_length=max_length,
+ dataset_map_fn=pretrain_map_fn,
+ template_map_fn=None,
+ remove_unused_columns=True,
+ shuffle_before_pack=False,
+ pack_to_max_length=pack_to_max_length,
+ use_varlen_attn=use_varlen_attn)
+
+train_dataloader = dict(
+ batch_size=batch_size,
+ num_workers=dataloader_num_workers,
+ dataset=train_dataset,
+ sampler=dict(type=DefaultSampler, shuffle=True),
+ collate_fn=dict(type=default_collate_fn, use_varlen_attn=use_varlen_attn))
+
+#######################################################################
+# PART 4 Scheduler & Optimizer #
+#######################################################################
+# optimizer
+optim_wrapper = dict(
+ type=AmpOptimWrapper,
+ optimizer=dict(
+ type=optim_type, lr=lr, betas=betas, weight_decay=weight_decay),
+ clip_grad=dict(max_norm=max_norm, error_if_nonfinite=False),
+ accumulative_counts=accumulative_counts,
+ loss_scale='dynamic',
+ dtype='float16')
+
+# learning policy
+# More information: https://github.com/open-mmlab/mmengine/blob/main/docs/en/tutorials/param_scheduler.md # noqa: E501
+param_scheduler = [
+ dict(
+ type=LinearLR,
+ start_factor=1e-5,
+ by_epoch=True,
+ begin=0,
+ end=warmup_ratio * max_epochs,
+ convert_to_iter_based=True),
+ dict(
+ type=CosineAnnealingLR,
+ eta_min=0.0,
+ by_epoch=True,
+ begin=warmup_ratio * max_epochs,
+ end=max_epochs,
+ convert_to_iter_based=True)
+]
+
+# train, val, test setting
+train_cfg = dict(type=TrainLoop, max_epochs=max_epochs)
+
+#######################################################################
+# PART 5 Runtime #
+#######################################################################
+# Log the dialogue periodically during the training process, optional
+custom_hooks = [
+ dict(type=DatasetInfoHook, tokenizer=tokenizer),
+ dict(
+ type=EvaluateChatHook,
+ tokenizer=tokenizer,
+ every_n_iters=evaluation_freq,
+ evaluation_inputs=evaluation_inputs,
+ system=SYSTEM)
+]
+
+if use_varlen_attn:
+ custom_hooks += [dict(type=VarlenAttnArgsToMessageHubHook)]
+
+# configure default hooks
+default_hooks = dict(
+ # record the time of every iteration.
+ timer=dict(type=IterTimerHook),
+ # print log every 10 iterations.
+ logger=dict(type=LoggerHook, log_metric_by_epoch=False, interval=10),
+ # enable the parameter scheduler.
+ param_scheduler=dict(type=ParamSchedulerHook),
+ # save checkpoint per `save_steps`.
+ checkpoint=dict(
+ type=CheckpointHook,
+ by_epoch=False,
+ interval=save_steps,
+ max_keep_ckpts=save_total_limit),
+ # set sampler seed in distributed evrionment.
+ sampler_seed=dict(type=DistSamplerSeedHook),
+)
+
+# configure environment
+env_cfg = dict(
+ # whether to enable cudnn benchmark
+ cudnn_benchmark=False,
+ # set multi process parameters
+ mp_cfg=dict(mp_start_method='fork', opencv_num_threads=0),
+ # set distributed parameters
+ dist_cfg=dict(backend='nccl'),
+)
+
+# set visualizer
+visualizer = None
+
+# set log level
+log_level = 'INFO'
+
+# load from which checkpoint
+load_from = None
+
+# whether to resume training from the loaded checkpoint
+resume = False
+
+# Defaults to use random seed and disable `deterministic`
+randomness = dict(seed=None, deterministic=False)
+
+# set log processor
+log_processor = dict(by_epoch=False)
diff --git a/xtuner/configs/custom_dataset/pretrain/mixtral/mixtral_8x7b_full_custom_pretrain_e1.py b/xtuner/configs/custom_dataset/pretrain/mixtral/mixtral_8x7b_full_custom_pretrain_e1.py
new file mode 100644
index 000000000..b2f5a6888
--- /dev/null
+++ b/xtuner/configs/custom_dataset/pretrain/mixtral/mixtral_8x7b_full_custom_pretrain_e1.py
@@ -0,0 +1,199 @@
+# Copyright (c) OpenMMLab. All rights reserved.
+"""Data format:
+
+[
+ {
+ "text": "xxx"
+ },
+ {
+ "text": "xxx"
+ },
+ ...
+]
+""" # noqa: E501
+
+from datasets import load_dataset
+from mmengine.dataset import DefaultSampler
+from mmengine.hooks import (CheckpointHook, DistSamplerSeedHook, IterTimerHook,
+ LoggerHook, ParamSchedulerHook)
+from mmengine.optim import AmpOptimWrapper, CosineAnnealingLR, LinearLR
+from torch.optim import AdamW
+from transformers import AutoModelForCausalLM, AutoTokenizer
+
+from xtuner.dataset import process_hf_dataset
+from xtuner.dataset.collate_fns import default_collate_fn
+from xtuner.dataset.map_fns import pretrain_map_fn
+from xtuner.engine.hooks import (DatasetInfoHook, EvaluateChatHook,
+ VarlenAttnArgsToMessageHubHook)
+from xtuner.engine.runner import TrainLoop
+from xtuner.model import SupervisedFinetune
+
+#######################################################################
+# PART 1 Settings #
+#######################################################################
+# Model
+pretrained_model_name_or_path = 'mistralai/Mixtral-8x7B-v0.1'
+use_varlen_attn = False
+
+# Data
+data_files = ['/path/to/json/file.json']
+max_length = 2048
+pack_to_max_length = True
+
+# Scheduler & Optimizer
+batch_size = 1 # per_device
+accumulative_counts = 16 # bs = 1 GPU * 1 batch_size_per_device * 16 acc
+dataloader_num_workers = 0
+max_epochs = 1
+optim_type = AdamW
+lr = 2e-5
+betas = (0.9, 0.999)
+weight_decay = 0
+max_norm = 1 # grad clip
+warmup_ratio = 0.03
+
+# Save
+save_steps = 500
+save_total_limit = 2 # Maximum checkpoints to keep (-1 means unlimited)
+
+# Evaluate the generation performance during the training
+evaluation_freq = 500
+SYSTEM = ''
+evaluation_inputs = ['上海是', 'Shanghai is']
+
+#######################################################################
+# PART 2 Model & Tokenizer #
+#######################################################################
+tokenizer = dict(
+ type=AutoTokenizer.from_pretrained,
+ pretrained_model_name_or_path=pretrained_model_name_or_path,
+ trust_remote_code=True,
+ padding_side='right')
+
+model = dict(
+ type=SupervisedFinetune,
+ use_varlen_attn=use_varlen_attn,
+ llm=dict(
+ type=AutoModelForCausalLM.from_pretrained,
+ pretrained_model_name_or_path=pretrained_model_name_or_path,
+ trust_remote_code=True))
+
+#######################################################################
+# PART 3 Dataset & Dataloader #
+#######################################################################
+train_dataset = dict(
+ type=process_hf_dataset,
+ dataset=dict(type=load_dataset, path='json', data_files=data_files),
+ tokenizer=tokenizer,
+ max_length=max_length,
+ dataset_map_fn=pretrain_map_fn,
+ template_map_fn=None,
+ remove_unused_columns=True,
+ shuffle_before_pack=False,
+ pack_to_max_length=pack_to_max_length,
+ use_varlen_attn=use_varlen_attn)
+
+train_dataloader = dict(
+ batch_size=batch_size,
+ num_workers=dataloader_num_workers,
+ dataset=train_dataset,
+ sampler=dict(type=DefaultSampler, shuffle=True),
+ collate_fn=dict(type=default_collate_fn, use_varlen_attn=use_varlen_attn))
+
+#######################################################################
+# PART 4 Scheduler & Optimizer #
+#######################################################################
+# optimizer
+optim_wrapper = dict(
+ type=AmpOptimWrapper,
+ optimizer=dict(
+ type=optim_type, lr=lr, betas=betas, weight_decay=weight_decay),
+ clip_grad=dict(max_norm=max_norm, error_if_nonfinite=False),
+ accumulative_counts=accumulative_counts,
+ loss_scale='dynamic',
+ dtype='float16')
+
+# learning policy
+# More information: https://github.com/open-mmlab/mmengine/blob/main/docs/en/tutorials/param_scheduler.md # noqa: E501
+param_scheduler = [
+ dict(
+ type=LinearLR,
+ start_factor=1e-5,
+ by_epoch=True,
+ begin=0,
+ end=warmup_ratio * max_epochs,
+ convert_to_iter_based=True),
+ dict(
+ type=CosineAnnealingLR,
+ eta_min=0.0,
+ by_epoch=True,
+ begin=warmup_ratio * max_epochs,
+ end=max_epochs,
+ convert_to_iter_based=True)
+]
+
+# train, val, test setting
+train_cfg = dict(type=TrainLoop, max_epochs=max_epochs)
+
+#######################################################################
+# PART 5 Runtime #
+#######################################################################
+# Log the dialogue periodically during the training process, optional
+custom_hooks = [
+ dict(type=DatasetInfoHook, tokenizer=tokenizer),
+ dict(
+ type=EvaluateChatHook,
+ tokenizer=tokenizer,
+ every_n_iters=evaluation_freq,
+ evaluation_inputs=evaluation_inputs,
+ system=SYSTEM)
+]
+
+if use_varlen_attn:
+ custom_hooks += [dict(type=VarlenAttnArgsToMessageHubHook)]
+
+# configure default hooks
+default_hooks = dict(
+ # record the time of every iteration.
+ timer=dict(type=IterTimerHook),
+ # print log every 10 iterations.
+ logger=dict(type=LoggerHook, log_metric_by_epoch=False, interval=10),
+ # enable the parameter scheduler.
+ param_scheduler=dict(type=ParamSchedulerHook),
+ # save checkpoint per `save_steps`.
+ checkpoint=dict(
+ type=CheckpointHook,
+ by_epoch=False,
+ interval=save_steps,
+ max_keep_ckpts=save_total_limit),
+ # set sampler seed in distributed evrionment.
+ sampler_seed=dict(type=DistSamplerSeedHook),
+)
+
+# configure environment
+env_cfg = dict(
+ # whether to enable cudnn benchmark
+ cudnn_benchmark=False,
+ # set multi process parameters
+ mp_cfg=dict(mp_start_method='fork', opencv_num_threads=0),
+ # set distributed parameters
+ dist_cfg=dict(backend='nccl'),
+)
+
+# set visualizer
+visualizer = None
+
+# set log level
+log_level = 'INFO'
+
+# load from which checkpoint
+load_from = None
+
+# whether to resume training from the loaded checkpoint
+resume = False
+
+# Defaults to use random seed and disable `deterministic`
+randomness = dict(seed=None, deterministic=False)
+
+# set log processor
+log_processor = dict(by_epoch=False)
diff --git a/xtuner/configs/custom_dataset/pretrain/qwen/qwen1_5_0_5b_full_custom_pretrain_e1.py b/xtuner/configs/custom_dataset/pretrain/qwen/qwen1_5_0_5b_full_custom_pretrain_e1.py
new file mode 100644
index 000000000..0e0e6cabd
--- /dev/null
+++ b/xtuner/configs/custom_dataset/pretrain/qwen/qwen1_5_0_5b_full_custom_pretrain_e1.py
@@ -0,0 +1,199 @@
+# Copyright (c) OpenMMLab. All rights reserved.
+"""Data format:
+
+[
+ {
+ "text": "xxx"
+ },
+ {
+ "text": "xxx"
+ },
+ ...
+]
+""" # noqa: E501
+
+from datasets import load_dataset
+from mmengine.dataset import DefaultSampler
+from mmengine.hooks import (CheckpointHook, DistSamplerSeedHook, IterTimerHook,
+ LoggerHook, ParamSchedulerHook)
+from mmengine.optim import AmpOptimWrapper, CosineAnnealingLR, LinearLR
+from torch.optim import AdamW
+from transformers import AutoModelForCausalLM, AutoTokenizer
+
+from xtuner.dataset import process_hf_dataset
+from xtuner.dataset.collate_fns import default_collate_fn
+from xtuner.dataset.map_fns import pretrain_map_fn
+from xtuner.engine.hooks import (DatasetInfoHook, EvaluateChatHook,
+ VarlenAttnArgsToMessageHubHook)
+from xtuner.engine.runner import TrainLoop
+from xtuner.model import SupervisedFinetune
+
+#######################################################################
+# PART 1 Settings #
+#######################################################################
+# Model
+pretrained_model_name_or_path = 'Qwen/Qwen1.5-0.5B'
+use_varlen_attn = False
+
+# Data
+data_files = ['/path/to/json/file.json']
+max_length = 2048
+pack_to_max_length = True
+
+# Scheduler & Optimizer
+batch_size = 1 # per_device
+accumulative_counts = 16 # bs = 1 GPU * 1 batch_size_per_device * 16 acc
+dataloader_num_workers = 0
+max_epochs = 1
+optim_type = AdamW
+lr = 2e-5
+betas = (0.9, 0.999)
+weight_decay = 0
+max_norm = 1 # grad clip
+warmup_ratio = 0.03
+
+# Save
+save_steps = 500
+save_total_limit = 2 # Maximum checkpoints to keep (-1 means unlimited)
+
+# Evaluate the generation performance during the training
+evaluation_freq = 500
+SYSTEM = ''
+evaluation_inputs = ['上海是', 'Shanghai is']
+
+#######################################################################
+# PART 2 Model & Tokenizer #
+#######################################################################
+tokenizer = dict(
+ type=AutoTokenizer.from_pretrained,
+ pretrained_model_name_or_path=pretrained_model_name_or_path,
+ trust_remote_code=True,
+ padding_side='right')
+
+model = dict(
+ type=SupervisedFinetune,
+ use_varlen_attn=use_varlen_attn,
+ llm=dict(
+ type=AutoModelForCausalLM.from_pretrained,
+ pretrained_model_name_or_path=pretrained_model_name_or_path,
+ trust_remote_code=True))
+
+#######################################################################
+# PART 3 Dataset & Dataloader #
+#######################################################################
+train_dataset = dict(
+ type=process_hf_dataset,
+ dataset=dict(type=load_dataset, path='json', data_files=data_files),
+ tokenizer=tokenizer,
+ max_length=max_length,
+ dataset_map_fn=pretrain_map_fn,
+ template_map_fn=None,
+ remove_unused_columns=True,
+ shuffle_before_pack=False,
+ pack_to_max_length=pack_to_max_length,
+ use_varlen_attn=use_varlen_attn)
+
+train_dataloader = dict(
+ batch_size=batch_size,
+ num_workers=dataloader_num_workers,
+ dataset=train_dataset,
+ sampler=dict(type=DefaultSampler, shuffle=True),
+ collate_fn=dict(type=default_collate_fn, use_varlen_attn=use_varlen_attn))
+
+#######################################################################
+# PART 4 Scheduler & Optimizer #
+#######################################################################
+# optimizer
+optim_wrapper = dict(
+ type=AmpOptimWrapper,
+ optimizer=dict(
+ type=optim_type, lr=lr, betas=betas, weight_decay=weight_decay),
+ clip_grad=dict(max_norm=max_norm, error_if_nonfinite=False),
+ accumulative_counts=accumulative_counts,
+ loss_scale='dynamic',
+ dtype='float16')
+
+# learning policy
+# More information: https://github.com/open-mmlab/mmengine/blob/main/docs/en/tutorials/param_scheduler.md # noqa: E501
+param_scheduler = [
+ dict(
+ type=LinearLR,
+ start_factor=1e-5,
+ by_epoch=True,
+ begin=0,
+ end=warmup_ratio * max_epochs,
+ convert_to_iter_based=True),
+ dict(
+ type=CosineAnnealingLR,
+ eta_min=0.0,
+ by_epoch=True,
+ begin=warmup_ratio * max_epochs,
+ end=max_epochs,
+ convert_to_iter_based=True)
+]
+
+# train, val, test setting
+train_cfg = dict(type=TrainLoop, max_epochs=max_epochs)
+
+#######################################################################
+# PART 5 Runtime #
+#######################################################################
+# Log the dialogue periodically during the training process, optional
+custom_hooks = [
+ dict(type=DatasetInfoHook, tokenizer=tokenizer),
+ dict(
+ type=EvaluateChatHook,
+ tokenizer=tokenizer,
+ every_n_iters=evaluation_freq,
+ evaluation_inputs=evaluation_inputs,
+ system=SYSTEM)
+]
+
+if use_varlen_attn:
+ custom_hooks += [dict(type=VarlenAttnArgsToMessageHubHook)]
+
+# configure default hooks
+default_hooks = dict(
+ # record the time of every iteration.
+ timer=dict(type=IterTimerHook),
+ # print log every 10 iterations.
+ logger=dict(type=LoggerHook, log_metric_by_epoch=False, interval=10),
+ # enable the parameter scheduler.
+ param_scheduler=dict(type=ParamSchedulerHook),
+ # save checkpoint per `save_steps`.
+ checkpoint=dict(
+ type=CheckpointHook,
+ by_epoch=False,
+ interval=save_steps,
+ max_keep_ckpts=save_total_limit),
+ # set sampler seed in distributed evrionment.
+ sampler_seed=dict(type=DistSamplerSeedHook),
+)
+
+# configure environment
+env_cfg = dict(
+ # whether to enable cudnn benchmark
+ cudnn_benchmark=False,
+ # set multi process parameters
+ mp_cfg=dict(mp_start_method='fork', opencv_num_threads=0),
+ # set distributed parameters
+ dist_cfg=dict(backend='nccl'),
+)
+
+# set visualizer
+visualizer = None
+
+# set log level
+log_level = 'INFO'
+
+# load from which checkpoint
+load_from = None
+
+# whether to resume training from the loaded checkpoint
+resume = False
+
+# Defaults to use random seed and disable `deterministic`
+randomness = dict(seed=None, deterministic=False)
+
+# set log processor
+log_processor = dict(by_epoch=False)
diff --git a/xtuner/configs/custom_dataset/pretrain/qwen/qwen1_5_14b_full_custom_pretrain_e1.py b/xtuner/configs/custom_dataset/pretrain/qwen/qwen1_5_14b_full_custom_pretrain_e1.py
new file mode 100644
index 000000000..3d6b4cbba
--- /dev/null
+++ b/xtuner/configs/custom_dataset/pretrain/qwen/qwen1_5_14b_full_custom_pretrain_e1.py
@@ -0,0 +1,199 @@
+# Copyright (c) OpenMMLab. All rights reserved.
+"""Data format:
+
+[
+ {
+ "text": "xxx"
+ },
+ {
+ "text": "xxx"
+ },
+ ...
+]
+""" # noqa: E501
+
+from datasets import load_dataset
+from mmengine.dataset import DefaultSampler
+from mmengine.hooks import (CheckpointHook, DistSamplerSeedHook, IterTimerHook,
+ LoggerHook, ParamSchedulerHook)
+from mmengine.optim import AmpOptimWrapper, CosineAnnealingLR, LinearLR
+from torch.optim import AdamW
+from transformers import AutoModelForCausalLM, AutoTokenizer
+
+from xtuner.dataset import process_hf_dataset
+from xtuner.dataset.collate_fns import default_collate_fn
+from xtuner.dataset.map_fns import pretrain_map_fn
+from xtuner.engine.hooks import (DatasetInfoHook, EvaluateChatHook,
+ VarlenAttnArgsToMessageHubHook)
+from xtuner.engine.runner import TrainLoop
+from xtuner.model import SupervisedFinetune
+
+#######################################################################
+# PART 1 Settings #
+#######################################################################
+# Model
+pretrained_model_name_or_path = 'Qwen/Qwen1.5-14B'
+use_varlen_attn = False
+
+# Data
+data_files = ['/path/to/json/file.json']
+max_length = 2048
+pack_to_max_length = True
+
+# Scheduler & Optimizer
+batch_size = 1 # per_device
+accumulative_counts = 16 # bs = 1 GPU * 1 batch_size_per_device * 16 acc
+dataloader_num_workers = 0
+max_epochs = 1
+optim_type = AdamW
+lr = 2e-5
+betas = (0.9, 0.999)
+weight_decay = 0
+max_norm = 1 # grad clip
+warmup_ratio = 0.03
+
+# Save
+save_steps = 500
+save_total_limit = 2 # Maximum checkpoints to keep (-1 means unlimited)
+
+# Evaluate the generation performance during the training
+evaluation_freq = 500
+SYSTEM = ''
+evaluation_inputs = ['上海是', 'Shanghai is']
+
+#######################################################################
+# PART 2 Model & Tokenizer #
+#######################################################################
+tokenizer = dict(
+ type=AutoTokenizer.from_pretrained,
+ pretrained_model_name_or_path=pretrained_model_name_or_path,
+ trust_remote_code=True,
+ padding_side='right')
+
+model = dict(
+ type=SupervisedFinetune,
+ use_varlen_attn=use_varlen_attn,
+ llm=dict(
+ type=AutoModelForCausalLM.from_pretrained,
+ pretrained_model_name_or_path=pretrained_model_name_or_path,
+ trust_remote_code=True))
+
+#######################################################################
+# PART 3 Dataset & Dataloader #
+#######################################################################
+train_dataset = dict(
+ type=process_hf_dataset,
+ dataset=dict(type=load_dataset, path='json', data_files=data_files),
+ tokenizer=tokenizer,
+ max_length=max_length,
+ dataset_map_fn=pretrain_map_fn,
+ template_map_fn=None,
+ remove_unused_columns=True,
+ shuffle_before_pack=False,
+ pack_to_max_length=pack_to_max_length,
+ use_varlen_attn=use_varlen_attn)
+
+train_dataloader = dict(
+ batch_size=batch_size,
+ num_workers=dataloader_num_workers,
+ dataset=train_dataset,
+ sampler=dict(type=DefaultSampler, shuffle=True),
+ collate_fn=dict(type=default_collate_fn, use_varlen_attn=use_varlen_attn))
+
+#######################################################################
+# PART 4 Scheduler & Optimizer #
+#######################################################################
+# optimizer
+optim_wrapper = dict(
+ type=AmpOptimWrapper,
+ optimizer=dict(
+ type=optim_type, lr=lr, betas=betas, weight_decay=weight_decay),
+ clip_grad=dict(max_norm=max_norm, error_if_nonfinite=False),
+ accumulative_counts=accumulative_counts,
+ loss_scale='dynamic',
+ dtype='float16')
+
+# learning policy
+# More information: https://github.com/open-mmlab/mmengine/blob/main/docs/en/tutorials/param_scheduler.md # noqa: E501
+param_scheduler = [
+ dict(
+ type=LinearLR,
+ start_factor=1e-5,
+ by_epoch=True,
+ begin=0,
+ end=warmup_ratio * max_epochs,
+ convert_to_iter_based=True),
+ dict(
+ type=CosineAnnealingLR,
+ eta_min=0.0,
+ by_epoch=True,
+ begin=warmup_ratio * max_epochs,
+ end=max_epochs,
+ convert_to_iter_based=True)
+]
+
+# train, val, test setting
+train_cfg = dict(type=TrainLoop, max_epochs=max_epochs)
+
+#######################################################################
+# PART 5 Runtime #
+#######################################################################
+# Log the dialogue periodically during the training process, optional
+custom_hooks = [
+ dict(type=DatasetInfoHook, tokenizer=tokenizer),
+ dict(
+ type=EvaluateChatHook,
+ tokenizer=tokenizer,
+ every_n_iters=evaluation_freq,
+ evaluation_inputs=evaluation_inputs,
+ system=SYSTEM)
+]
+
+if use_varlen_attn:
+ custom_hooks += [dict(type=VarlenAttnArgsToMessageHubHook)]
+
+# configure default hooks
+default_hooks = dict(
+ # record the time of every iteration.
+ timer=dict(type=IterTimerHook),
+ # print log every 10 iterations.
+ logger=dict(type=LoggerHook, log_metric_by_epoch=False, interval=10),
+ # enable the parameter scheduler.
+ param_scheduler=dict(type=ParamSchedulerHook),
+ # save checkpoint per `save_steps`.
+ checkpoint=dict(
+ type=CheckpointHook,
+ by_epoch=False,
+ interval=save_steps,
+ max_keep_ckpts=save_total_limit),
+ # set sampler seed in distributed evrionment.
+ sampler_seed=dict(type=DistSamplerSeedHook),
+)
+
+# configure environment
+env_cfg = dict(
+ # whether to enable cudnn benchmark
+ cudnn_benchmark=False,
+ # set multi process parameters
+ mp_cfg=dict(mp_start_method='fork', opencv_num_threads=0),
+ # set distributed parameters
+ dist_cfg=dict(backend='nccl'),
+)
+
+# set visualizer
+visualizer = None
+
+# set log level
+log_level = 'INFO'
+
+# load from which checkpoint
+load_from = None
+
+# whether to resume training from the loaded checkpoint
+resume = False
+
+# Defaults to use random seed and disable `deterministic`
+randomness = dict(seed=None, deterministic=False)
+
+# set log processor
+log_processor = dict(by_epoch=False)
diff --git a/xtuner/configs/custom_dataset/pretrain/qwen/qwen1_5_1_8b_full_custom_pretrain_e1.py b/xtuner/configs/custom_dataset/pretrain/qwen/qwen1_5_1_8b_full_custom_pretrain_e1.py
new file mode 100644
index 000000000..1e4724e2e
--- /dev/null
+++ b/xtuner/configs/custom_dataset/pretrain/qwen/qwen1_5_1_8b_full_custom_pretrain_e1.py
@@ -0,0 +1,199 @@
+# Copyright (c) OpenMMLab. All rights reserved.
+"""Data format:
+
+[
+ {
+ "text": "xxx"
+ },
+ {
+ "text": "xxx"
+ },
+ ...
+]
+""" # noqa: E501
+
+from datasets import load_dataset
+from mmengine.dataset import DefaultSampler
+from mmengine.hooks import (CheckpointHook, DistSamplerSeedHook, IterTimerHook,
+ LoggerHook, ParamSchedulerHook)
+from mmengine.optim import AmpOptimWrapper, CosineAnnealingLR, LinearLR
+from torch.optim import AdamW
+from transformers import AutoModelForCausalLM, AutoTokenizer
+
+from xtuner.dataset import process_hf_dataset
+from xtuner.dataset.collate_fns import default_collate_fn
+from xtuner.dataset.map_fns import pretrain_map_fn
+from xtuner.engine.hooks import (DatasetInfoHook, EvaluateChatHook,
+ VarlenAttnArgsToMessageHubHook)
+from xtuner.engine.runner import TrainLoop
+from xtuner.model import SupervisedFinetune
+
+#######################################################################
+# PART 1 Settings #
+#######################################################################
+# Model
+pretrained_model_name_or_path = 'Qwen/Qwen1.5-1.8B'
+use_varlen_attn = False
+
+# Data
+data_files = ['/path/to/json/file.json']
+max_length = 2048
+pack_to_max_length = True
+
+# Scheduler & Optimizer
+batch_size = 1 # per_device
+accumulative_counts = 16 # bs = 1 GPU * 1 batch_size_per_device * 16 acc
+dataloader_num_workers = 0
+max_epochs = 1
+optim_type = AdamW
+lr = 2e-5
+betas = (0.9, 0.999)
+weight_decay = 0
+max_norm = 1 # grad clip
+warmup_ratio = 0.03
+
+# Save
+save_steps = 500
+save_total_limit = 2 # Maximum checkpoints to keep (-1 means unlimited)
+
+# Evaluate the generation performance during the training
+evaluation_freq = 500
+SYSTEM = ''
+evaluation_inputs = ['上海是', 'Shanghai is']
+
+#######################################################################
+# PART 2 Model & Tokenizer #
+#######################################################################
+tokenizer = dict(
+ type=AutoTokenizer.from_pretrained,
+ pretrained_model_name_or_path=pretrained_model_name_or_path,
+ trust_remote_code=True,
+ padding_side='right')
+
+model = dict(
+ type=SupervisedFinetune,
+ use_varlen_attn=use_varlen_attn,
+ llm=dict(
+ type=AutoModelForCausalLM.from_pretrained,
+ pretrained_model_name_or_path=pretrained_model_name_or_path,
+ trust_remote_code=True))
+
+#######################################################################
+# PART 3 Dataset & Dataloader #
+#######################################################################
+train_dataset = dict(
+ type=process_hf_dataset,
+ dataset=dict(type=load_dataset, path='json', data_files=data_files),
+ tokenizer=tokenizer,
+ max_length=max_length,
+ dataset_map_fn=pretrain_map_fn,
+ template_map_fn=None,
+ remove_unused_columns=True,
+ shuffle_before_pack=False,
+ pack_to_max_length=pack_to_max_length,
+ use_varlen_attn=use_varlen_attn)
+
+train_dataloader = dict(
+ batch_size=batch_size,
+ num_workers=dataloader_num_workers,
+ dataset=train_dataset,
+ sampler=dict(type=DefaultSampler, shuffle=True),
+ collate_fn=dict(type=default_collate_fn, use_varlen_attn=use_varlen_attn))
+
+#######################################################################
+# PART 4 Scheduler & Optimizer #
+#######################################################################
+# optimizer
+optim_wrapper = dict(
+ type=AmpOptimWrapper,
+ optimizer=dict(
+ type=optim_type, lr=lr, betas=betas, weight_decay=weight_decay),
+ clip_grad=dict(max_norm=max_norm, error_if_nonfinite=False),
+ accumulative_counts=accumulative_counts,
+ loss_scale='dynamic',
+ dtype='float16')
+
+# learning policy
+# More information: https://github.com/open-mmlab/mmengine/blob/main/docs/en/tutorials/param_scheduler.md # noqa: E501
+param_scheduler = [
+ dict(
+ type=LinearLR,
+ start_factor=1e-5,
+ by_epoch=True,
+ begin=0,
+ end=warmup_ratio * max_epochs,
+ convert_to_iter_based=True),
+ dict(
+ type=CosineAnnealingLR,
+ eta_min=0.0,
+ by_epoch=True,
+ begin=warmup_ratio * max_epochs,
+ end=max_epochs,
+ convert_to_iter_based=True)
+]
+
+# train, val, test setting
+train_cfg = dict(type=TrainLoop, max_epochs=max_epochs)
+
+#######################################################################
+# PART 5 Runtime #
+#######################################################################
+# Log the dialogue periodically during the training process, optional
+custom_hooks = [
+ dict(type=DatasetInfoHook, tokenizer=tokenizer),
+ dict(
+ type=EvaluateChatHook,
+ tokenizer=tokenizer,
+ every_n_iters=evaluation_freq,
+ evaluation_inputs=evaluation_inputs,
+ system=SYSTEM)
+]
+
+if use_varlen_attn:
+ custom_hooks += [dict(type=VarlenAttnArgsToMessageHubHook)]
+
+# configure default hooks
+default_hooks = dict(
+ # record the time of every iteration.
+ timer=dict(type=IterTimerHook),
+ # print log every 10 iterations.
+ logger=dict(type=LoggerHook, log_metric_by_epoch=False, interval=10),
+ # enable the parameter scheduler.
+ param_scheduler=dict(type=ParamSchedulerHook),
+ # save checkpoint per `save_steps`.
+ checkpoint=dict(
+ type=CheckpointHook,
+ by_epoch=False,
+ interval=save_steps,
+ max_keep_ckpts=save_total_limit),
+ # set sampler seed in distributed evrionment.
+ sampler_seed=dict(type=DistSamplerSeedHook),
+)
+
+# configure environment
+env_cfg = dict(
+ # whether to enable cudnn benchmark
+ cudnn_benchmark=False,
+ # set multi process parameters
+ mp_cfg=dict(mp_start_method='fork', opencv_num_threads=0),
+ # set distributed parameters
+ dist_cfg=dict(backend='nccl'),
+)
+
+# set visualizer
+visualizer = None
+
+# set log level
+log_level = 'INFO'
+
+# load from which checkpoint
+load_from = None
+
+# whether to resume training from the loaded checkpoint
+resume = False
+
+# Defaults to use random seed and disable `deterministic`
+randomness = dict(seed=None, deterministic=False)
+
+# set log processor
+log_processor = dict(by_epoch=False)
diff --git a/xtuner/configs/custom_dataset/pretrain/qwen/qwen1_5_4b_full_custom_pretrain_e1.py b/xtuner/configs/custom_dataset/pretrain/qwen/qwen1_5_4b_full_custom_pretrain_e1.py
new file mode 100644
index 000000000..1ad11ff3b
--- /dev/null
+++ b/xtuner/configs/custom_dataset/pretrain/qwen/qwen1_5_4b_full_custom_pretrain_e1.py
@@ -0,0 +1,199 @@
+# Copyright (c) OpenMMLab. All rights reserved.
+"""Data format:
+
+[
+ {
+ "text": "xxx"
+ },
+ {
+ "text": "xxx"
+ },
+ ...
+]
+""" # noqa: E501
+
+from datasets import load_dataset
+from mmengine.dataset import DefaultSampler
+from mmengine.hooks import (CheckpointHook, DistSamplerSeedHook, IterTimerHook,
+ LoggerHook, ParamSchedulerHook)
+from mmengine.optim import AmpOptimWrapper, CosineAnnealingLR, LinearLR
+from torch.optim import AdamW
+from transformers import AutoModelForCausalLM, AutoTokenizer
+
+from xtuner.dataset import process_hf_dataset
+from xtuner.dataset.collate_fns import default_collate_fn
+from xtuner.dataset.map_fns import pretrain_map_fn
+from xtuner.engine.hooks import (DatasetInfoHook, EvaluateChatHook,
+ VarlenAttnArgsToMessageHubHook)
+from xtuner.engine.runner import TrainLoop
+from xtuner.model import SupervisedFinetune
+
+#######################################################################
+# PART 1 Settings #
+#######################################################################
+# Model
+pretrained_model_name_or_path = 'Qwen/Qwen1.5-4B'
+use_varlen_attn = False
+
+# Data
+data_files = ['/path/to/json/file.json']
+max_length = 2048
+pack_to_max_length = True
+
+# Scheduler & Optimizer
+batch_size = 1 # per_device
+accumulative_counts = 16 # bs = 1 GPU * 1 batch_size_per_device * 16 acc
+dataloader_num_workers = 0
+max_epochs = 1
+optim_type = AdamW
+lr = 2e-5
+betas = (0.9, 0.999)
+weight_decay = 0
+max_norm = 1 # grad clip
+warmup_ratio = 0.03
+
+# Save
+save_steps = 500
+save_total_limit = 2 # Maximum checkpoints to keep (-1 means unlimited)
+
+# Evaluate the generation performance during the training
+evaluation_freq = 500
+SYSTEM = ''
+evaluation_inputs = ['上海是', 'Shanghai is']
+
+#######################################################################
+# PART 2 Model & Tokenizer #
+#######################################################################
+tokenizer = dict(
+ type=AutoTokenizer.from_pretrained,
+ pretrained_model_name_or_path=pretrained_model_name_or_path,
+ trust_remote_code=True,
+ padding_side='right')
+
+model = dict(
+ type=SupervisedFinetune,
+ use_varlen_attn=use_varlen_attn,
+ llm=dict(
+ type=AutoModelForCausalLM.from_pretrained,
+ pretrained_model_name_or_path=pretrained_model_name_or_path,
+ trust_remote_code=True))
+
+#######################################################################
+# PART 3 Dataset & Dataloader #
+#######################################################################
+train_dataset = dict(
+ type=process_hf_dataset,
+ dataset=dict(type=load_dataset, path='json', data_files=data_files),
+ tokenizer=tokenizer,
+ max_length=max_length,
+ dataset_map_fn=pretrain_map_fn,
+ template_map_fn=None,
+ remove_unused_columns=True,
+ shuffle_before_pack=False,
+ pack_to_max_length=pack_to_max_length,
+ use_varlen_attn=use_varlen_attn)
+
+train_dataloader = dict(
+ batch_size=batch_size,
+ num_workers=dataloader_num_workers,
+ dataset=train_dataset,
+ sampler=dict(type=DefaultSampler, shuffle=True),
+ collate_fn=dict(type=default_collate_fn, use_varlen_attn=use_varlen_attn))
+
+#######################################################################
+# PART 4 Scheduler & Optimizer #
+#######################################################################
+# optimizer
+optim_wrapper = dict(
+ type=AmpOptimWrapper,
+ optimizer=dict(
+ type=optim_type, lr=lr, betas=betas, weight_decay=weight_decay),
+ clip_grad=dict(max_norm=max_norm, error_if_nonfinite=False),
+ accumulative_counts=accumulative_counts,
+ loss_scale='dynamic',
+ dtype='float16')
+
+# learning policy
+# More information: https://github.com/open-mmlab/mmengine/blob/main/docs/en/tutorials/param_scheduler.md # noqa: E501
+param_scheduler = [
+ dict(
+ type=LinearLR,
+ start_factor=1e-5,
+ by_epoch=True,
+ begin=0,
+ end=warmup_ratio * max_epochs,
+ convert_to_iter_based=True),
+ dict(
+ type=CosineAnnealingLR,
+ eta_min=0.0,
+ by_epoch=True,
+ begin=warmup_ratio * max_epochs,
+ end=max_epochs,
+ convert_to_iter_based=True)
+]
+
+# train, val, test setting
+train_cfg = dict(type=TrainLoop, max_epochs=max_epochs)
+
+#######################################################################
+# PART 5 Runtime #
+#######################################################################
+# Log the dialogue periodically during the training process, optional
+custom_hooks = [
+ dict(type=DatasetInfoHook, tokenizer=tokenizer),
+ dict(
+ type=EvaluateChatHook,
+ tokenizer=tokenizer,
+ every_n_iters=evaluation_freq,
+ evaluation_inputs=evaluation_inputs,
+ system=SYSTEM)
+]
+
+if use_varlen_attn:
+ custom_hooks += [dict(type=VarlenAttnArgsToMessageHubHook)]
+
+# configure default hooks
+default_hooks = dict(
+ # record the time of every iteration.
+ timer=dict(type=IterTimerHook),
+ # print log every 10 iterations.
+ logger=dict(type=LoggerHook, log_metric_by_epoch=False, interval=10),
+ # enable the parameter scheduler.
+ param_scheduler=dict(type=ParamSchedulerHook),
+ # save checkpoint per `save_steps`.
+ checkpoint=dict(
+ type=CheckpointHook,
+ by_epoch=False,
+ interval=save_steps,
+ max_keep_ckpts=save_total_limit),
+ # set sampler seed in distributed evrionment.
+ sampler_seed=dict(type=DistSamplerSeedHook),
+)
+
+# configure environment
+env_cfg = dict(
+ # whether to enable cudnn benchmark
+ cudnn_benchmark=False,
+ # set multi process parameters
+ mp_cfg=dict(mp_start_method='fork', opencv_num_threads=0),
+ # set distributed parameters
+ dist_cfg=dict(backend='nccl'),
+)
+
+# set visualizer
+visualizer = None
+
+# set log level
+log_level = 'INFO'
+
+# load from which checkpoint
+load_from = None
+
+# whether to resume training from the loaded checkpoint
+resume = False
+
+# Defaults to use random seed and disable `deterministic`
+randomness = dict(seed=None, deterministic=False)
+
+# set log processor
+log_processor = dict(by_epoch=False)
diff --git a/xtuner/configs/custom_dataset/pretrain/qwen/qwen1_5_72b_full_custom_pretrain_e1.py b/xtuner/configs/custom_dataset/pretrain/qwen/qwen1_5_72b_full_custom_pretrain_e1.py
new file mode 100644
index 000000000..2f7cf2117
--- /dev/null
+++ b/xtuner/configs/custom_dataset/pretrain/qwen/qwen1_5_72b_full_custom_pretrain_e1.py
@@ -0,0 +1,199 @@
+# Copyright (c) OpenMMLab. All rights reserved.
+"""Data format:
+
+[
+ {
+ "text": "xxx"
+ },
+ {
+ "text": "xxx"
+ },
+ ...
+]
+""" # noqa: E501
+
+from datasets import load_dataset
+from mmengine.dataset import DefaultSampler
+from mmengine.hooks import (CheckpointHook, DistSamplerSeedHook, IterTimerHook,
+ LoggerHook, ParamSchedulerHook)
+from mmengine.optim import AmpOptimWrapper, CosineAnnealingLR, LinearLR
+from torch.optim import AdamW
+from transformers import AutoModelForCausalLM, AutoTokenizer
+
+from xtuner.dataset import process_hf_dataset
+from xtuner.dataset.collate_fns import default_collate_fn
+from xtuner.dataset.map_fns import pretrain_map_fn
+from xtuner.engine.hooks import (DatasetInfoHook, EvaluateChatHook,
+ VarlenAttnArgsToMessageHubHook)
+from xtuner.engine.runner import TrainLoop
+from xtuner.model import SupervisedFinetune
+
+#######################################################################
+# PART 1 Settings #
+#######################################################################
+# Model
+pretrained_model_name_or_path = 'Qwen/Qwen1.5-72B'
+use_varlen_attn = False
+
+# Data
+data_files = ['/path/to/json/file.json']
+max_length = 2048
+pack_to_max_length = True
+
+# Scheduler & Optimizer
+batch_size = 1 # per_device
+accumulative_counts = 16 # bs = 1 GPU * 1 batch_size_per_device * 16 acc
+dataloader_num_workers = 0
+max_epochs = 1
+optim_type = AdamW
+lr = 2e-5
+betas = (0.9, 0.999)
+weight_decay = 0
+max_norm = 1 # grad clip
+warmup_ratio = 0.03
+
+# Save
+save_steps = 500
+save_total_limit = 2 # Maximum checkpoints to keep (-1 means unlimited)
+
+# Evaluate the generation performance during the training
+evaluation_freq = 500
+SYSTEM = ''
+evaluation_inputs = ['上海是', 'Shanghai is']
+
+#######################################################################
+# PART 2 Model & Tokenizer #
+#######################################################################
+tokenizer = dict(
+ type=AutoTokenizer.from_pretrained,
+ pretrained_model_name_or_path=pretrained_model_name_or_path,
+ trust_remote_code=True,
+ padding_side='right')
+
+model = dict(
+ type=SupervisedFinetune,
+ use_varlen_attn=use_varlen_attn,
+ llm=dict(
+ type=AutoModelForCausalLM.from_pretrained,
+ pretrained_model_name_or_path=pretrained_model_name_or_path,
+ trust_remote_code=True))
+
+#######################################################################
+# PART 3 Dataset & Dataloader #
+#######################################################################
+train_dataset = dict(
+ type=process_hf_dataset,
+ dataset=dict(type=load_dataset, path='json', data_files=data_files),
+ tokenizer=tokenizer,
+ max_length=max_length,
+ dataset_map_fn=pretrain_map_fn,
+ template_map_fn=None,
+ remove_unused_columns=True,
+ shuffle_before_pack=False,
+ pack_to_max_length=pack_to_max_length,
+ use_varlen_attn=use_varlen_attn)
+
+train_dataloader = dict(
+ batch_size=batch_size,
+ num_workers=dataloader_num_workers,
+ dataset=train_dataset,
+ sampler=dict(type=DefaultSampler, shuffle=True),
+ collate_fn=dict(type=default_collate_fn, use_varlen_attn=use_varlen_attn))
+
+#######################################################################
+# PART 4 Scheduler & Optimizer #
+#######################################################################
+# optimizer
+optim_wrapper = dict(
+ type=AmpOptimWrapper,
+ optimizer=dict(
+ type=optim_type, lr=lr, betas=betas, weight_decay=weight_decay),
+ clip_grad=dict(max_norm=max_norm, error_if_nonfinite=False),
+ accumulative_counts=accumulative_counts,
+ loss_scale='dynamic',
+ dtype='float16')
+
+# learning policy
+# More information: https://github.com/open-mmlab/mmengine/blob/main/docs/en/tutorials/param_scheduler.md # noqa: E501
+param_scheduler = [
+ dict(
+ type=LinearLR,
+ start_factor=1e-5,
+ by_epoch=True,
+ begin=0,
+ end=warmup_ratio * max_epochs,
+ convert_to_iter_based=True),
+ dict(
+ type=CosineAnnealingLR,
+ eta_min=0.0,
+ by_epoch=True,
+ begin=warmup_ratio * max_epochs,
+ end=max_epochs,
+ convert_to_iter_based=True)
+]
+
+# train, val, test setting
+train_cfg = dict(type=TrainLoop, max_epochs=max_epochs)
+
+#######################################################################
+# PART 5 Runtime #
+#######################################################################
+# Log the dialogue periodically during the training process, optional
+custom_hooks = [
+ dict(type=DatasetInfoHook, tokenizer=tokenizer),
+ dict(
+ type=EvaluateChatHook,
+ tokenizer=tokenizer,
+ every_n_iters=evaluation_freq,
+ evaluation_inputs=evaluation_inputs,
+ system=SYSTEM)
+]
+
+if use_varlen_attn:
+ custom_hooks += [dict(type=VarlenAttnArgsToMessageHubHook)]
+
+# configure default hooks
+default_hooks = dict(
+ # record the time of every iteration.
+ timer=dict(type=IterTimerHook),
+ # print log every 10 iterations.
+ logger=dict(type=LoggerHook, log_metric_by_epoch=False, interval=10),
+ # enable the parameter scheduler.
+ param_scheduler=dict(type=ParamSchedulerHook),
+ # save checkpoint per `save_steps`.
+ checkpoint=dict(
+ type=CheckpointHook,
+ by_epoch=False,
+ interval=save_steps,
+ max_keep_ckpts=save_total_limit),
+ # set sampler seed in distributed evrionment.
+ sampler_seed=dict(type=DistSamplerSeedHook),
+)
+
+# configure environment
+env_cfg = dict(
+ # whether to enable cudnn benchmark
+ cudnn_benchmark=False,
+ # set multi process parameters
+ mp_cfg=dict(mp_start_method='fork', opencv_num_threads=0),
+ # set distributed parameters
+ dist_cfg=dict(backend='nccl'),
+)
+
+# set visualizer
+visualizer = None
+
+# set log level
+log_level = 'INFO'
+
+# load from which checkpoint
+load_from = None
+
+# whether to resume training from the loaded checkpoint
+resume = False
+
+# Defaults to use random seed and disable `deterministic`
+randomness = dict(seed=None, deterministic=False)
+
+# set log processor
+log_processor = dict(by_epoch=False)
diff --git a/xtuner/configs/custom_dataset/pretrain/qwen/qwen1_5_7b_full_custom_pretrain_e1.py b/xtuner/configs/custom_dataset/pretrain/qwen/qwen1_5_7b_full_custom_pretrain_e1.py
new file mode 100644
index 000000000..911c22344
--- /dev/null
+++ b/xtuner/configs/custom_dataset/pretrain/qwen/qwen1_5_7b_full_custom_pretrain_e1.py
@@ -0,0 +1,199 @@
+# Copyright (c) OpenMMLab. All rights reserved.
+"""Data format:
+
+[
+ {
+ "text": "xxx"
+ },
+ {
+ "text": "xxx"
+ },
+ ...
+]
+""" # noqa: E501
+
+from datasets import load_dataset
+from mmengine.dataset import DefaultSampler
+from mmengine.hooks import (CheckpointHook, DistSamplerSeedHook, IterTimerHook,
+ LoggerHook, ParamSchedulerHook)
+from mmengine.optim import AmpOptimWrapper, CosineAnnealingLR, LinearLR
+from torch.optim import AdamW
+from transformers import AutoModelForCausalLM, AutoTokenizer
+
+from xtuner.dataset import process_hf_dataset
+from xtuner.dataset.collate_fns import default_collate_fn
+from xtuner.dataset.map_fns import pretrain_map_fn
+from xtuner.engine.hooks import (DatasetInfoHook, EvaluateChatHook,
+ VarlenAttnArgsToMessageHubHook)
+from xtuner.engine.runner import TrainLoop
+from xtuner.model import SupervisedFinetune
+
+#######################################################################
+# PART 1 Settings #
+#######################################################################
+# Model
+pretrained_model_name_or_path = 'Qwen/Qwen1.5-7B'
+use_varlen_attn = False
+
+# Data
+data_files = ['/path/to/json/file.json']
+max_length = 2048
+pack_to_max_length = True
+
+# Scheduler & Optimizer
+batch_size = 1 # per_device
+accumulative_counts = 16 # bs = 1 GPU * 1 batch_size_per_device * 16 acc
+dataloader_num_workers = 0
+max_epochs = 1
+optim_type = AdamW
+lr = 2e-5
+betas = (0.9, 0.999)
+weight_decay = 0
+max_norm = 1 # grad clip
+warmup_ratio = 0.03
+
+# Save
+save_steps = 500
+save_total_limit = 2 # Maximum checkpoints to keep (-1 means unlimited)
+
+# Evaluate the generation performance during the training
+evaluation_freq = 500
+SYSTEM = ''
+evaluation_inputs = ['上海是', 'Shanghai is']
+
+#######################################################################
+# PART 2 Model & Tokenizer #
+#######################################################################
+tokenizer = dict(
+ type=AutoTokenizer.from_pretrained,
+ pretrained_model_name_or_path=pretrained_model_name_or_path,
+ trust_remote_code=True,
+ padding_side='right')
+
+model = dict(
+ type=SupervisedFinetune,
+ use_varlen_attn=use_varlen_attn,
+ llm=dict(
+ type=AutoModelForCausalLM.from_pretrained,
+ pretrained_model_name_or_path=pretrained_model_name_or_path,
+ trust_remote_code=True))
+
+#######################################################################
+# PART 3 Dataset & Dataloader #
+#######################################################################
+train_dataset = dict(
+ type=process_hf_dataset,
+ dataset=dict(type=load_dataset, path='json', data_files=data_files),
+ tokenizer=tokenizer,
+ max_length=max_length,
+ dataset_map_fn=pretrain_map_fn,
+ template_map_fn=None,
+ remove_unused_columns=True,
+ shuffle_before_pack=False,
+ pack_to_max_length=pack_to_max_length,
+ use_varlen_attn=use_varlen_attn)
+
+train_dataloader = dict(
+ batch_size=batch_size,
+ num_workers=dataloader_num_workers,
+ dataset=train_dataset,
+ sampler=dict(type=DefaultSampler, shuffle=True),
+ collate_fn=dict(type=default_collate_fn, use_varlen_attn=use_varlen_attn))
+
+#######################################################################
+# PART 4 Scheduler & Optimizer #
+#######################################################################
+# optimizer
+optim_wrapper = dict(
+ type=AmpOptimWrapper,
+ optimizer=dict(
+ type=optim_type, lr=lr, betas=betas, weight_decay=weight_decay),
+ clip_grad=dict(max_norm=max_norm, error_if_nonfinite=False),
+ accumulative_counts=accumulative_counts,
+ loss_scale='dynamic',
+ dtype='float16')
+
+# learning policy
+# More information: https://github.com/open-mmlab/mmengine/blob/main/docs/en/tutorials/param_scheduler.md # noqa: E501
+param_scheduler = [
+ dict(
+ type=LinearLR,
+ start_factor=1e-5,
+ by_epoch=True,
+ begin=0,
+ end=warmup_ratio * max_epochs,
+ convert_to_iter_based=True),
+ dict(
+ type=CosineAnnealingLR,
+ eta_min=0.0,
+ by_epoch=True,
+ begin=warmup_ratio * max_epochs,
+ end=max_epochs,
+ convert_to_iter_based=True)
+]
+
+# train, val, test setting
+train_cfg = dict(type=TrainLoop, max_epochs=max_epochs)
+
+#######################################################################
+# PART 5 Runtime #
+#######################################################################
+# Log the dialogue periodically during the training process, optional
+custom_hooks = [
+ dict(type=DatasetInfoHook, tokenizer=tokenizer),
+ dict(
+ type=EvaluateChatHook,
+ tokenizer=tokenizer,
+ every_n_iters=evaluation_freq,
+ evaluation_inputs=evaluation_inputs,
+ system=SYSTEM)
+]
+
+if use_varlen_attn:
+ custom_hooks += [dict(type=VarlenAttnArgsToMessageHubHook)]
+
+# configure default hooks
+default_hooks = dict(
+ # record the time of every iteration.
+ timer=dict(type=IterTimerHook),
+ # print log every 10 iterations.
+ logger=dict(type=LoggerHook, log_metric_by_epoch=False, interval=10),
+ # enable the parameter scheduler.
+ param_scheduler=dict(type=ParamSchedulerHook),
+ # save checkpoint per `save_steps`.
+ checkpoint=dict(
+ type=CheckpointHook,
+ by_epoch=False,
+ interval=save_steps,
+ max_keep_ckpts=save_total_limit),
+ # set sampler seed in distributed evrionment.
+ sampler_seed=dict(type=DistSamplerSeedHook),
+)
+
+# configure environment
+env_cfg = dict(
+ # whether to enable cudnn benchmark
+ cudnn_benchmark=False,
+ # set multi process parameters
+ mp_cfg=dict(mp_start_method='fork', opencv_num_threads=0),
+ # set distributed parameters
+ dist_cfg=dict(backend='nccl'),
+)
+
+# set visualizer
+visualizer = None
+
+# set log level
+log_level = 'INFO'
+
+# load from which checkpoint
+load_from = None
+
+# whether to resume training from the loaded checkpoint
+resume = False
+
+# Defaults to use random seed and disable `deterministic`
+randomness = dict(seed=None, deterministic=False)
+
+# set log processor
+log_processor = dict(by_epoch=False)
diff --git a/xtuner/configs/custom_dataset/pretrain/qwen/qwen_1_8b_full_custom_pretrain_e1.py b/xtuner/configs/custom_dataset/pretrain/qwen/qwen_1_8b_full_custom_pretrain_e1.py
new file mode 100644
index 000000000..a1cbd63dd
--- /dev/null
+++ b/xtuner/configs/custom_dataset/pretrain/qwen/qwen_1_8b_full_custom_pretrain_e1.py
@@ -0,0 +1,200 @@
+# Copyright (c) OpenMMLab. All rights reserved.
+"""Data format:
+
+[
+ {
+ "text": "xxx"
+ },
+ {
+ "text": "xxx"
+ },
+ ...
+]
+""" # noqa: E501
+
+from datasets import load_dataset
+from mmengine.dataset import DefaultSampler
+from mmengine.hooks import (CheckpointHook, DistSamplerSeedHook, IterTimerHook,
+ LoggerHook, ParamSchedulerHook)
+from mmengine.optim import AmpOptimWrapper, CosineAnnealingLR, LinearLR
+from torch.optim import AdamW
+from transformers import AutoModelForCausalLM, AutoTokenizer
+
+from xtuner.dataset import process_hf_dataset
+from xtuner.dataset.collate_fns import default_collate_fn
+from xtuner.dataset.map_fns import pretrain_map_fn
+from xtuner.engine.hooks import (DatasetInfoHook, EvaluateChatHook,
+ VarlenAttnArgsToMessageHubHook)
+from xtuner.engine.runner import TrainLoop
+from xtuner.model import SupervisedFinetune
+
+#######################################################################
+# PART 1 Settings #
+#######################################################################
+# Model
+pretrained_model_name_or_path = 'Qwen/Qwen-1_8B'
+use_varlen_attn = False
+
+# Data
+data_files = ['/path/to/json/file.json']
+max_length = 2048
+pack_to_max_length = True
+
+# Scheduler & Optimizer
+batch_size = 1 # per_device
+accumulative_counts = 16 # bs = 1 GPU * 1 batch_size_per_device * 16 acc
+dataloader_num_workers = 0
+max_epochs = 1
+optim_type = AdamW
+lr = 2e-5
+betas = (0.9, 0.999)
+weight_decay = 0
+max_norm = 1 # grad clip
+warmup_ratio = 0.03
+
+# Save
+save_steps = 500
+save_total_limit = 2 # Maximum checkpoints to keep (-1 means unlimited)
+
+# Evaluate the generation performance during the training
+evaluation_freq = 500
+SYSTEM = ''
+evaluation_inputs = ['上海是', 'Shanghai is']
+
+#######################################################################
+# PART 2 Model & Tokenizer #
+#######################################################################
+tokenizer = dict(
+ type=AutoTokenizer.from_pretrained,
+ pretrained_model_name_or_path=pretrained_model_name_or_path,
+ trust_remote_code=True,
+ padding_side='right',
+ eos_token='<|endoftext|>')
+
+model = dict(
+ type=SupervisedFinetune,
+ use_varlen_attn=use_varlen_attn,
+ llm=dict(
+ type=AutoModelForCausalLM.from_pretrained,
+ pretrained_model_name_or_path=pretrained_model_name_or_path,
+ trust_remote_code=True))
+
+#######################################################################
+# PART 3 Dataset & Dataloader #
+#######################################################################
+train_dataset = dict(
+ type=process_hf_dataset,
+ dataset=dict(type=load_dataset, path='json', data_files=data_files),
+ tokenizer=tokenizer,
+ max_length=max_length,
+ dataset_map_fn=pretrain_map_fn,
+ template_map_fn=None,
+ remove_unused_columns=True,
+ shuffle_before_pack=False,
+ pack_to_max_length=pack_to_max_length,
+ use_varlen_attn=use_varlen_attn)
+
+train_dataloader = dict(
+ batch_size=batch_size,
+ num_workers=dataloader_num_workers,
+ dataset=train_dataset,
+ sampler=dict(type=DefaultSampler, shuffle=True),
+ collate_fn=dict(type=default_collate_fn, use_varlen_attn=use_varlen_attn))
+
+#######################################################################
+# PART 4 Scheduler & Optimizer #
+#######################################################################
+# optimizer
+optim_wrapper = dict(
+ type=AmpOptimWrapper,
+ optimizer=dict(
+ type=optim_type, lr=lr, betas=betas, weight_decay=weight_decay),
+ clip_grad=dict(max_norm=max_norm, error_if_nonfinite=False),
+ accumulative_counts=accumulative_counts,
+ loss_scale='dynamic',
+ dtype='float16')
+
+# learning policy
+# More information: https://github.com/open-mmlab/mmengine/blob/main/docs/en/tutorials/param_scheduler.md # noqa: E501
+param_scheduler = [
+ dict(
+ type=LinearLR,
+ start_factor=1e-5,
+ by_epoch=True,
+ begin=0,
+ end=warmup_ratio * max_epochs,
+ convert_to_iter_based=True),
+ dict(
+ type=CosineAnnealingLR,
+ eta_min=0.0,
+ by_epoch=True,
+ begin=warmup_ratio * max_epochs,
+ end=max_epochs,
+ convert_to_iter_based=True)
+]
+
+# train, val, test setting
+train_cfg = dict(type=TrainLoop, max_epochs=max_epochs)
+
+#######################################################################
+# PART 5 Runtime #
+#######################################################################
+# Log the dialogue periodically during the training process, optional
+custom_hooks = [
+ dict(type=DatasetInfoHook, tokenizer=tokenizer),
+ dict(
+ type=EvaluateChatHook,
+ tokenizer=tokenizer,
+ every_n_iters=evaluation_freq,
+ evaluation_inputs=evaluation_inputs,
+ system=SYSTEM)
+]
+
+if use_varlen_attn:
+ custom_hooks += [dict(type=VarlenAttnArgsToMessageHubHook)]
+
+# configure default hooks
+default_hooks = dict(
+ # record the time of every iteration.
+ timer=dict(type=IterTimerHook),
+ # print log every 10 iterations.
+ logger=dict(type=LoggerHook, log_metric_by_epoch=False, interval=10),
+ # enable the parameter scheduler.
+ param_scheduler=dict(type=ParamSchedulerHook),
+ # save checkpoint per `save_steps`.
+ checkpoint=dict(
+ type=CheckpointHook,
+ by_epoch=False,
+ interval=save_steps,
+ max_keep_ckpts=save_total_limit),
+ # set sampler seed in distributed evrionment.
+ sampler_seed=dict(type=DistSamplerSeedHook),
+)
+
+# configure environment
+env_cfg = dict(
+ # whether to enable cudnn benchmark
+ cudnn_benchmark=False,
+ # set multi process parameters
+ mp_cfg=dict(mp_start_method='fork', opencv_num_threads=0),
+ # set distributed parameters
+ dist_cfg=dict(backend='nccl'),
+)
+
+# set visualizer
+visualizer = None
+
+# set log level
+log_level = 'INFO'
+
+# load from which checkpoint
+load_from = None
+
+# whether to resume training from the loaded checkpoint
+resume = False
+
+# Defaults to use random seed and disable `deterministic`
+randomness = dict(seed=None, deterministic=False)
+
+# set log processor
+log_processor = dict(by_epoch=False)
diff --git a/xtuner/configs/custom_dataset/pretrain/qwen/qwen_72b_full_custom_pretrain_e1.py b/xtuner/configs/custom_dataset/pretrain/qwen/qwen_72b_full_custom_pretrain_e1.py
new file mode 100644
index 000000000..07812fb59
--- /dev/null
+++ b/xtuner/configs/custom_dataset/pretrain/qwen/qwen_72b_full_custom_pretrain_e1.py
@@ -0,0 +1,200 @@
+# Copyright (c) OpenMMLab. All rights reserved.
+"""Data format:
+
+[
+ {
+ "text": "xxx"
+ },
+ {
+ "text": "xxx"
+ },
+ ...
+]
+""" # noqa: E501
+
+from datasets import load_dataset
+from mmengine.dataset import DefaultSampler
+from mmengine.hooks import (CheckpointHook, DistSamplerSeedHook, IterTimerHook,
+ LoggerHook, ParamSchedulerHook)
+from mmengine.optim import AmpOptimWrapper, CosineAnnealingLR, LinearLR
+from torch.optim import AdamW
+from transformers import AutoModelForCausalLM, AutoTokenizer
+
+from xtuner.dataset import process_hf_dataset
+from xtuner.dataset.collate_fns import default_collate_fn
+from xtuner.dataset.map_fns import pretrain_map_fn
+from xtuner.engine.hooks import (DatasetInfoHook, EvaluateChatHook,
+ VarlenAttnArgsToMessageHubHook)
+from xtuner.engine.runner import TrainLoop
+from xtuner.model import SupervisedFinetune
+
+#######################################################################
+# PART 1 Settings #
+#######################################################################
+# Model
+pretrained_model_name_or_path = 'Qwen/Qwen-72B'
+use_varlen_attn = False
+
+# Data
+data_files = ['/path/to/json/file.json']
+max_length = 2048
+pack_to_max_length = True
+
+# Scheduler & Optimizer
+batch_size = 1 # per_device
+accumulative_counts = 16 # bs = 1 GPU * 1 batch_size_per_device * 16 acc
+dataloader_num_workers = 0
+max_epochs = 1
+optim_type = AdamW
+lr = 2e-5
+betas = (0.9, 0.999)
+weight_decay = 0
+max_norm = 1 # grad clip
+warmup_ratio = 0.03
+
+# Save
+save_steps = 500
+save_total_limit = 2 # Maximum checkpoints to keep (-1 means unlimited)
+
+# Evaluate the generation performance during the training
+evaluation_freq = 500
+SYSTEM = ''
+evaluation_inputs = ['上海是', 'Shanghai is']
+
+#######################################################################
+# PART 2 Model & Tokenizer #
+#######################################################################
+tokenizer = dict(
+ type=AutoTokenizer.from_pretrained,
+ pretrained_model_name_or_path=pretrained_model_name_or_path,
+ trust_remote_code=True,
+ padding_side='right',
+ eos_token='<|endoftext|>')
+
+model = dict(
+ type=SupervisedFinetune,
+ use_varlen_attn=use_varlen_attn,
+ llm=dict(
+ type=AutoModelForCausalLM.from_pretrained,
+ pretrained_model_name_or_path=pretrained_model_name_or_path,
+ trust_remote_code=True))
+
+#######################################################################
+# PART 3 Dataset & Dataloader #
+#######################################################################
+train_dataset = dict(
+ type=process_hf_dataset,
+ dataset=dict(type=load_dataset, path='json', data_files=data_files),
+ tokenizer=tokenizer,
+ max_length=max_length,
+ dataset_map_fn=pretrain_map_fn,
+ template_map_fn=None,
+ remove_unused_columns=True,
+ shuffle_before_pack=False,
+ pack_to_max_length=pack_to_max_length,
+ use_varlen_attn=use_varlen_attn)
+
+train_dataloader = dict(
+ batch_size=batch_size,
+ num_workers=dataloader_num_workers,
+ dataset=train_dataset,
+ sampler=dict(type=DefaultSampler, shuffle=True),
+ collate_fn=dict(type=default_collate_fn, use_varlen_attn=use_varlen_attn))
+
+#######################################################################
+# PART 4 Scheduler & Optimizer #
+#######################################################################
+# optimizer
+optim_wrapper = dict(
+ type=AmpOptimWrapper,
+ optimizer=dict(
+ type=optim_type, lr=lr, betas=betas, weight_decay=weight_decay),
+ clip_grad=dict(max_norm=max_norm, error_if_nonfinite=False),
+ accumulative_counts=accumulative_counts,
+ loss_scale='dynamic',
+ dtype='float16')
+
+# learning policy
+# More information: https://github.com/open-mmlab/mmengine/blob/main/docs/en/tutorials/param_scheduler.md # noqa: E501
+param_scheduler = [
+ dict(
+ type=LinearLR,
+ start_factor=1e-5,
+ by_epoch=True,
+ begin=0,
+ end=warmup_ratio * max_epochs,
+ convert_to_iter_based=True),
+ dict(
+ type=CosineAnnealingLR,
+ eta_min=0.0,
+ by_epoch=True,
+ begin=warmup_ratio * max_epochs,
+ end=max_epochs,
+ convert_to_iter_based=True)
+]
+
+# train, val, test setting
+train_cfg = dict(type=TrainLoop, max_epochs=max_epochs)
+
+#######################################################################
+# PART 5 Runtime #
+#######################################################################
+# Log the dialogue periodically during the training process, optional
+custom_hooks = [
+ dict(type=DatasetInfoHook, tokenizer=tokenizer),
+ dict(
+ type=EvaluateChatHook,
+ tokenizer=tokenizer,
+ every_n_iters=evaluation_freq,
+ evaluation_inputs=evaluation_inputs,
+ system=SYSTEM)
+]
+
+if use_varlen_attn:
+ custom_hooks += [dict(type=VarlenAttnArgsToMessageHubHook)]
+
+# configure default hooks
+default_hooks = dict(
+ # record the time of every iteration.
+ timer=dict(type=IterTimerHook),
+ # print log every 10 iterations.
+ logger=dict(type=LoggerHook, log_metric_by_epoch=False, interval=10),
+ # enable the parameter scheduler.
+ param_scheduler=dict(type=ParamSchedulerHook),
+ # save checkpoint per `save_steps`.
+ checkpoint=dict(
+ type=CheckpointHook,
+ by_epoch=False,
+ interval=save_steps,
+ max_keep_ckpts=save_total_limit),
+ # set sampler seed in distributed evrionment.
+ sampler_seed=dict(type=DistSamplerSeedHook),
+)
+
+# configure environment
+env_cfg = dict(
+ # whether to enable cudnn benchmark
+ cudnn_benchmark=False,
+ # set multi process parameters
+ mp_cfg=dict(mp_start_method='fork', opencv_num_threads=0),
+ # set distributed parameters
+ dist_cfg=dict(backend='nccl'),
+)
+
+# set visualizer
+visualizer = None
+
+# set log level
+log_level = 'INFO'
+
+# load from which checkpoint
+load_from = None
+
+# whether to resume training from the loaded checkpoint
+resume = False
+
+# Defaults to use random seed and disable `deterministic`
+randomness = dict(seed=None, deterministic=False)
+
+# set log processor
+log_processor = dict(by_epoch=False)
diff --git a/xtuner/configs/custom_dataset/pretrain/qwen/qwen_7b_full_custom_pretrain_e1.py b/xtuner/configs/custom_dataset/pretrain/qwen/qwen_7b_full_custom_pretrain_e1.py
new file mode 100644
index 000000000..16da30039
--- /dev/null
+++ b/xtuner/configs/custom_dataset/pretrain/qwen/qwen_7b_full_custom_pretrain_e1.py
@@ -0,0 +1,200 @@
+# Copyright (c) OpenMMLab. All rights reserved.
+"""Data format:
+
+[
+ {
+ "text": "xxx"
+ },
+ {
+ "text": "xxx"
+ },
+ ...
+]
+""" # noqa: E501
+
+from datasets import load_dataset
+from mmengine.dataset import DefaultSampler
+from mmengine.hooks import (CheckpointHook, DistSamplerSeedHook, IterTimerHook,
+ LoggerHook, ParamSchedulerHook)
+from mmengine.optim import AmpOptimWrapper, CosineAnnealingLR, LinearLR
+from torch.optim import AdamW
+from transformers import AutoModelForCausalLM, AutoTokenizer
+
+from xtuner.dataset import process_hf_dataset
+from xtuner.dataset.collate_fns import default_collate_fn
+from xtuner.dataset.map_fns import pretrain_map_fn
+from xtuner.engine.hooks import (DatasetInfoHook, EvaluateChatHook,
+ VarlenAttnArgsToMessageHubHook)
+from xtuner.engine.runner import TrainLoop
+from xtuner.model import SupervisedFinetune
+
+#######################################################################
+# PART 1 Settings #
+#######################################################################
+# Model
+pretrained_model_name_or_path = 'Qwen/Qwen-7B'
+use_varlen_attn = False
+
+# Data
+data_files = ['/path/to/json/file.json']
+max_length = 2048
+pack_to_max_length = True
+
+# Scheduler & Optimizer
+batch_size = 1 # per_device
+accumulative_counts = 16 # bs = 1 GPU * 1 batch_size_per_device * 16 acc
+dataloader_num_workers = 0
+max_epochs = 1
+optim_type = AdamW
+lr = 2e-5
+betas = (0.9, 0.999)
+weight_decay = 0
+max_norm = 1 # grad clip
+warmup_ratio = 0.03
+
+# Save
+save_steps = 500
+save_total_limit = 2 # Maximum checkpoints to keep (-1 means unlimited)
+
+# Evaluate the generation performance during the training
+evaluation_freq = 500
+SYSTEM = ''
+evaluation_inputs = ['上海是', 'Shanghai is']
+
+#######################################################################
+# PART 2 Model & Tokenizer #
+#######################################################################
+tokenizer = dict(
+ type=AutoTokenizer.from_pretrained,
+ pretrained_model_name_or_path=pretrained_model_name_or_path,
+ trust_remote_code=True,
+ padding_side='right',
+ eos_token='<|endoftext|>')
+
+model = dict(
+ type=SupervisedFinetune,
+ use_varlen_attn=use_varlen_attn,
+ llm=dict(
+ type=AutoModelForCausalLM.from_pretrained,
+ pretrained_model_name_or_path=pretrained_model_name_or_path,
+ trust_remote_code=True))
+
+#######################################################################
+# PART 3 Dataset & Dataloader #
+#######################################################################
+train_dataset = dict(
+ type=process_hf_dataset,
+ dataset=dict(type=load_dataset, path='json', data_files=data_files),
+ tokenizer=tokenizer,
+ max_length=max_length,
+ dataset_map_fn=pretrain_map_fn,
+ template_map_fn=None,
+ remove_unused_columns=True,
+ shuffle_before_pack=False,
+ pack_to_max_length=pack_to_max_length,
+ use_varlen_attn=use_varlen_attn)
+
+train_dataloader = dict(
+ batch_size=batch_size,
+ num_workers=dataloader_num_workers,
+ dataset=train_dataset,
+ sampler=dict(type=DefaultSampler, shuffle=True),
+ collate_fn=dict(type=default_collate_fn, use_varlen_attn=use_varlen_attn))
+
+#######################################################################
+# PART 4 Scheduler & Optimizer #
+#######################################################################
+# optimizer
+optim_wrapper = dict(
+ type=AmpOptimWrapper,
+ optimizer=dict(
+ type=optim_type, lr=lr, betas=betas, weight_decay=weight_decay),
+ clip_grad=dict(max_norm=max_norm, error_if_nonfinite=False),
+ accumulative_counts=accumulative_counts,
+ loss_scale='dynamic',
+ dtype='float16')
+
+# learning policy
+# More information: https://github.com/open-mmlab/mmengine/blob/main/docs/en/tutorials/param_scheduler.md # noqa: E501
+param_scheduler = [
+ dict(
+ type=LinearLR,
+ start_factor=1e-5,
+ by_epoch=True,
+ begin=0,
+ end=warmup_ratio * max_epochs,
+ convert_to_iter_based=True),
+ dict(
+ type=CosineAnnealingLR,
+ eta_min=0.0,
+ by_epoch=True,
+ begin=warmup_ratio * max_epochs,
+ end=max_epochs,
+ convert_to_iter_based=True)
+]
+
+# train, val, test setting
+train_cfg = dict(type=TrainLoop, max_epochs=max_epochs)
+
+#######################################################################
+# PART 5 Runtime #
+#######################################################################
+# Log the dialogue periodically during the training process, optional
+custom_hooks = [
+ dict(type=DatasetInfoHook, tokenizer=tokenizer),
+ dict(
+ type=EvaluateChatHook,
+ tokenizer=tokenizer,
+ every_n_iters=evaluation_freq,
+ evaluation_inputs=evaluation_inputs,
+ system=SYSTEM)
+]
+
+if use_varlen_attn:
+ custom_hooks += [dict(type=VarlenAttnArgsToMessageHubHook)]
+
+# configure default hooks
+default_hooks = dict(
+ # record the time of every iteration.
+ timer=dict(type=IterTimerHook),
+ # print log every 10 iterations.
+ logger=dict(type=LoggerHook, log_metric_by_epoch=False, interval=10),
+ # enable the parameter scheduler.
+ param_scheduler=dict(type=ParamSchedulerHook),
+ # save checkpoint per `save_steps`.
+ checkpoint=dict(
+ type=CheckpointHook,
+ by_epoch=False,
+ interval=save_steps,
+ max_keep_ckpts=save_total_limit),
+ # set sampler seed in distributed evrionment.
+ sampler_seed=dict(type=DistSamplerSeedHook),
+)
+
+# configure environment
+env_cfg = dict(
+ # whether to enable cudnn benchmark
+ cudnn_benchmark=False,
+ # set multi process parameters
+ mp_cfg=dict(mp_start_method='fork', opencv_num_threads=0),
+ # set distributed parameters
+ dist_cfg=dict(backend='nccl'),
+)
+
+# set visualizer
+visualizer = None
+
+# set log level
+log_level = 'INFO'
+
+# load from which checkpoint
+load_from = None
+
+# whether to resume training from the loaded checkpoint
+resume = False
+
+# Defaults to use random seed and disable `deterministic`
+randomness = dict(seed=None, deterministic=False)
+
+# set log processor
+log_processor = dict(by_epoch=False)
diff --git a/xtuner/configs/custom_dataset/pretrain/starcoder/starcoder_full_custom_pretrain_e1.py b/xtuner/configs/custom_dataset/pretrain/starcoder/starcoder_full_custom_pretrain_e1.py
new file mode 100644
index 000000000..40f10f73c
--- /dev/null
+++ b/xtuner/configs/custom_dataset/pretrain/starcoder/starcoder_full_custom_pretrain_e1.py
@@ -0,0 +1,201 @@
+# Copyright (c) OpenMMLab. All rights reserved.
+"""Data format:
+
+[
+ {
+ "text": "xxx"
+ },
+ {
+ "text": "xxx"
+ },
+ ...
+]
+""" # noqa: E501
+
+from datasets import load_dataset
+from mmengine.dataset import DefaultSampler
+from mmengine.hooks import (CheckpointHook, DistSamplerSeedHook, IterTimerHook,
+ LoggerHook, ParamSchedulerHook)
+from mmengine.optim import AmpOptimWrapper, CosineAnnealingLR, LinearLR
+from torch.optim import AdamW
+from transformers import AutoModelForCausalLM, AutoTokenizer
+
+from xtuner.dataset import process_hf_dataset
+from xtuner.dataset.collate_fns import default_collate_fn
+from xtuner.dataset.map_fns import pretrain_map_fn
+from xtuner.engine.hooks import (DatasetInfoHook, EvaluateChatHook,
+ VarlenAttnArgsToMessageHubHook)
+from xtuner.engine.runner import TrainLoop
+from xtuner.model import SupervisedFinetune
+
+#######################################################################
+# PART 1 Settings #
+#######################################################################
+# Model
+pretrained_model_name_or_path = 'bigcode/starcoder'
+use_varlen_attn = False
+
+# Data
+data_files = ['/path/to/json/file.json']
+max_length = 2048
+pack_to_max_length = True
+
+# Scheduler & Optimizer
+batch_size = 1 # per_device
+accumulative_counts = 16 # bs = 1 GPU * 1 batch_size_per_device * 16 acc
+dataloader_num_workers = 0
+max_epochs = 1
+optim_type = AdamW
+lr = 2e-5
+betas = (0.9, 0.999)
+weight_decay = 0
+max_norm = 1 # grad clip
+warmup_ratio = 0.03
+
+# Save
+save_steps = 500
+save_total_limit = 2 # Maximum checkpoints to keep (-1 means unlimited)
+
+# Evaluate the generation performance during the training
+evaluation_freq = 500
+SYSTEM = ''
+evaluation_inputs = [
+ 'from typing import List def has_close_elements(numbers: List[float], threshold: float) -> bool: """ Check if in given list of numbers, are any two numbers closer to each other than given threshold. >>> has_close_elements([1.0, 2.0, 3.0], 0.5) False >>> has_close_elements([1.0, 2.8, 3.0, 4.0, 5.0, 2.0], 0.3) True """' # noqa: E501
+]
+
+#######################################################################
+# PART 2 Model & Tokenizer #
+#######################################################################
+tokenizer = dict(
+ type=AutoTokenizer.from_pretrained,
+ pretrained_model_name_or_path=pretrained_model_name_or_path,
+ trust_remote_code=True,
+ padding_side='right')
+
+model = dict(
+ type=SupervisedFinetune,
+ use_varlen_attn=use_varlen_attn,
+ llm=dict(
+ type=AutoModelForCausalLM.from_pretrained,
+ pretrained_model_name_or_path=pretrained_model_name_or_path,
+ trust_remote_code=True))
+
+#######################################################################
+# PART 3 Dataset & Dataloader #
+#######################################################################
+train_dataset = dict(
+ type=process_hf_dataset,
+ dataset=dict(type=load_dataset, path='json', data_files=data_files),
+ tokenizer=tokenizer,
+ max_length=max_length,
+ dataset_map_fn=pretrain_map_fn,
+ template_map_fn=None,
+ remove_unused_columns=True,
+ shuffle_before_pack=False,
+ pack_to_max_length=pack_to_max_length,
+ use_varlen_attn=use_varlen_attn)
+
+train_dataloader = dict(
+ batch_size=batch_size,
+ num_workers=dataloader_num_workers,
+ dataset=train_dataset,
+ sampler=dict(type=DefaultSampler, shuffle=True),
+ collate_fn=dict(type=default_collate_fn, use_varlen_attn=use_varlen_attn))
+
+#######################################################################
+# PART 4 Scheduler & Optimizer #
+#######################################################################
+# optimizer
+optim_wrapper = dict(
+ type=AmpOptimWrapper,
+ optimizer=dict(
+ type=optim_type, lr=lr, betas=betas, weight_decay=weight_decay),
+ clip_grad=dict(max_norm=max_norm, error_if_nonfinite=False),
+ accumulative_counts=accumulative_counts,
+ loss_scale='dynamic',
+ dtype='float16')
+
+# learning policy
+# More information: https://github.com/open-mmlab/mmengine/blob/main/docs/en/tutorials/param_scheduler.md # noqa: E501
+param_scheduler = [
+ dict(
+ type=LinearLR,
+ start_factor=1e-5,
+ by_epoch=True,
+ begin=0,
+ end=warmup_ratio * max_epochs,
+ convert_to_iter_based=True),
+ dict(
+ type=CosineAnnealingLR,
+ eta_min=0.0,
+ by_epoch=True,
+ begin=warmup_ratio * max_epochs,
+ end=max_epochs,
+ convert_to_iter_based=True)
+]
+
+# train, val, test setting
+train_cfg = dict(type=TrainLoop, max_epochs=max_epochs)
+
+#######################################################################
+# PART 5 Runtime #
+#######################################################################
+# Log the dialogue periodically during the training process, optional
+custom_hooks = [
+ dict(type=DatasetInfoHook, tokenizer=tokenizer),
+ dict(
+ type=EvaluateChatHook,
+ tokenizer=tokenizer,
+ every_n_iters=evaluation_freq,
+ evaluation_inputs=evaluation_inputs,
+ system=SYSTEM)
+]
+
+if use_varlen_attn:
+ custom_hooks += [dict(type=VarlenAttnArgsToMessageHubHook)]
+
+# configure default hooks
+default_hooks = dict(
+ # record the time of every iteration.
+ timer=dict(type=IterTimerHook),
+ # print log every 10 iterations.
+ logger=dict(type=LoggerHook, log_metric_by_epoch=False, interval=10),
+ # enable the parameter scheduler.
+ param_scheduler=dict(type=ParamSchedulerHook),
+ # save checkpoint per `save_steps`.
+ checkpoint=dict(
+ type=CheckpointHook,
+ by_epoch=False,
+ interval=save_steps,
+ max_keep_ckpts=save_total_limit),
+ # set sampler seed in distributed evrionment.
+ sampler_seed=dict(type=DistSamplerSeedHook),
+)
+
+# configure environment
+env_cfg = dict(
+ # whether to enable cudnn benchmark
+ cudnn_benchmark=False,
+ # set multi process parameters
+ mp_cfg=dict(mp_start_method='fork', opencv_num_threads=0),
+ # set distributed parameters
+ dist_cfg=dict(backend='nccl'),
+)
+
+# set visualizer
+visualizer = None
+
+# set log level
+log_level = 'INFO'
+
+# load from which checkpoint
+load_from = None
+
+# whether to resume training from the loaded checkpoint
+resume = False
+
+# Defaults to use random seed and disable `deterministic`
+randomness = dict(seed=None, deterministic=False)
+
+# set log processor
+log_processor = dict(by_epoch=False)
diff --git a/xtuner/configs/custom_dataset/pretrain/yi/yi_34b_full_custom_pretrain_e1.py b/xtuner/configs/custom_dataset/pretrain/yi/yi_34b_full_custom_pretrain_e1.py
new file mode 100644
index 000000000..38d86efe7
--- /dev/null
+++ b/xtuner/configs/custom_dataset/pretrain/yi/yi_34b_full_custom_pretrain_e1.py
@@ -0,0 +1,199 @@
+# Copyright (c) OpenMMLab. All rights reserved.
+"""Data format:
+
+[
+ {
+ "text": "xxx"
+ },
+ {
+ "text": "xxx"
+ },
+ ...
+]
+""" # noqa: E501
+
+from datasets import load_dataset
+from mmengine.dataset import DefaultSampler
+from mmengine.hooks import (CheckpointHook, DistSamplerSeedHook, IterTimerHook,
+ LoggerHook, ParamSchedulerHook)
+from mmengine.optim import AmpOptimWrapper, CosineAnnealingLR, LinearLR
+from torch.optim import AdamW
+from transformers import AutoModelForCausalLM, AutoTokenizer
+
+from xtuner.dataset import process_hf_dataset
+from xtuner.dataset.collate_fns import default_collate_fn
+from xtuner.dataset.map_fns import pretrain_map_fn
+from xtuner.engine.hooks import (DatasetInfoHook, EvaluateChatHook,
+ VarlenAttnArgsToMessageHubHook)
+from xtuner.engine.runner import TrainLoop
+from xtuner.model import SupervisedFinetune
+
+#######################################################################
+# PART 1 Settings #
+#######################################################################
+# Model
+pretrained_model_name_or_path = '01-ai/Yi-34B'
+use_varlen_attn = False
+
+# Data
+data_files = ['/path/to/json/file.json']
+max_length = 2048
+pack_to_max_length = True
+
+# Scheduler & Optimizer
+batch_size = 1 # per_device
+accumulative_counts = 16 # bs = 1 GPU * 1 batch_size_per_device * 16 acc
+dataloader_num_workers = 0
+max_epochs = 1
+optim_type = AdamW
+lr = 2e-5
+betas = (0.9, 0.999)
+weight_decay = 0
+max_norm = 1 # grad clip
+warmup_ratio = 0.03
+
+# Save
+save_steps = 500
+save_total_limit = 2 # Maximum checkpoints to keep (-1 means unlimited)
+
+# Evaluate the generation performance during the training
+evaluation_freq = 500
+SYSTEM = ''
+evaluation_inputs = ['上海是', 'Shanghai is']
+
+#######################################################################
+# PART 2 Model & Tokenizer #
+#######################################################################
+tokenizer = dict(
+ type=AutoTokenizer.from_pretrained,
+ pretrained_model_name_or_path=pretrained_model_name_or_path,
+ trust_remote_code=True,
+ padding_side='right')
+
+model = dict(
+ type=SupervisedFinetune,
+ use_varlen_attn=use_varlen_attn,
+ llm=dict(
+ type=AutoModelForCausalLM.from_pretrained,
+ pretrained_model_name_or_path=pretrained_model_name_or_path,
+ trust_remote_code=True))
+
+#######################################################################
+# PART 3 Dataset & Dataloader #
+#######################################################################
+train_dataset = dict(
+ type=process_hf_dataset,
+ dataset=dict(type=load_dataset, path='json', data_files=data_files),
+ tokenizer=tokenizer,
+ max_length=max_length,
+ dataset_map_fn=pretrain_map_fn,
+ template_map_fn=None,
+ remove_unused_columns=True,
+ shuffle_before_pack=False,
+ pack_to_max_length=pack_to_max_length,
+ use_varlen_attn=use_varlen_attn)
+
+train_dataloader = dict(
+ batch_size=batch_size,
+ num_workers=dataloader_num_workers,
+ dataset=train_dataset,
+ sampler=dict(type=DefaultSampler, shuffle=True),
+ collate_fn=dict(type=default_collate_fn, use_varlen_attn=use_varlen_attn))
+
+#######################################################################
+# PART 4 Scheduler & Optimizer #
+#######################################################################
+# optimizer
+optim_wrapper = dict(
+ type=AmpOptimWrapper,
+ optimizer=dict(
+ type=optim_type, lr=lr, betas=betas, weight_decay=weight_decay),
+ clip_grad=dict(max_norm=max_norm, error_if_nonfinite=False),
+ accumulative_counts=accumulative_counts,
+ loss_scale='dynamic',
+ dtype='float16')
+
+# learning policy
+# More information: https://github.com/open-mmlab/mmengine/blob/main/docs/en/tutorials/param_scheduler.md # noqa: E501
+param_scheduler = [
+ dict(
+ type=LinearLR,
+ start_factor=1e-5,
+ by_epoch=True,
+ begin=0,
+ end=warmup_ratio * max_epochs,
+ convert_to_iter_based=True),
+ dict(
+ type=CosineAnnealingLR,
+ eta_min=0.0,
+ by_epoch=True,
+ begin=warmup_ratio * max_epochs,
+ end=max_epochs,
+ convert_to_iter_based=True)
+]
+
+# train, val, test setting
+train_cfg = dict(type=TrainLoop, max_epochs=max_epochs)
+
+#######################################################################
+# PART 5 Runtime #
+#######################################################################
+# Log the dialogue periodically during the training process, optional
+custom_hooks = [
+ dict(type=DatasetInfoHook, tokenizer=tokenizer),
+ dict(
+ type=EvaluateChatHook,
+ tokenizer=tokenizer,
+ every_n_iters=evaluation_freq,
+ evaluation_inputs=evaluation_inputs,
+ system=SYSTEM)
+]
+
+if use_varlen_attn:
+ custom_hooks += [dict(type=VarlenAttnArgsToMessageHubHook)]
+
+# configure default hooks
+default_hooks = dict(
+ # record the time of every iteration.
+ timer=dict(type=IterTimerHook),
+ # print log every 10 iterations.
+ logger=dict(type=LoggerHook, log_metric_by_epoch=False, interval=10),
+ # enable the parameter scheduler.
+ param_scheduler=dict(type=ParamSchedulerHook),
+ # save checkpoint per `save_steps`.
+ checkpoint=dict(
+ type=CheckpointHook,
+ by_epoch=False,
+ interval=save_steps,
+ max_keep_ckpts=save_total_limit),
+ # set sampler seed in distributed evrionment.
+ sampler_seed=dict(type=DistSamplerSeedHook),
+)
+
+# configure environment
+env_cfg = dict(
+ # whether to enable cudnn benchmark
+ cudnn_benchmark=False,
+ # set multi process parameters
+ mp_cfg=dict(mp_start_method='fork', opencv_num_threads=0),
+ # set distributed parameters
+ dist_cfg=dict(backend='nccl'),
+)
+
+# set visualizer
+visualizer = None
+
+# set log level
+log_level = 'INFO'
+
+# load from which checkpoint
+load_from = None
+
+# whether to resume training from the loaded checkpoint
+resume = False
+
+# Defaults to use random seed and disable `deterministic`
+randomness = dict(seed=None, deterministic=False)
+
+# set log processor
+log_processor = dict(by_epoch=False)
diff --git a/xtuner/configs/custom_dataset/pretrain/yi/yi_6b_full_custom_pretrain_e1.py b/xtuner/configs/custom_dataset/pretrain/yi/yi_6b_full_custom_pretrain_e1.py
new file mode 100644
index 000000000..d1524d23c
--- /dev/null
+++ b/xtuner/configs/custom_dataset/pretrain/yi/yi_6b_full_custom_pretrain_e1.py
@@ -0,0 +1,199 @@
+# Copyright (c) OpenMMLab. All rights reserved.
+"""Data format:
+
+[
+ {
+ "text": "xxx"
+ },
+ {
+ "text": "xxx"
+ },
+ ...
+]
+""" # noqa: E501
+
+from datasets import load_dataset
+from mmengine.dataset import DefaultSampler
+from mmengine.hooks import (CheckpointHook, DistSamplerSeedHook, IterTimerHook,
+ LoggerHook, ParamSchedulerHook)
+from mmengine.optim import AmpOptimWrapper, CosineAnnealingLR, LinearLR
+from torch.optim import AdamW
+from transformers import AutoModelForCausalLM, AutoTokenizer
+
+from xtuner.dataset import process_hf_dataset
+from xtuner.dataset.collate_fns import default_collate_fn
+from xtuner.dataset.map_fns import pretrain_map_fn
+from xtuner.engine.hooks import (DatasetInfoHook, EvaluateChatHook,
+ VarlenAttnArgsToMessageHubHook)
+from xtuner.engine.runner import TrainLoop
+from xtuner.model import SupervisedFinetune
+
+#######################################################################
+# PART 1 Settings #
+#######################################################################
+# Model
+pretrained_model_name_or_path = '01-ai/Yi-6B'
+use_varlen_attn = False
+
+# Data
+data_files = ['/path/to/json/file.json']
+max_length = 2048
+pack_to_max_length = True
+
+# Scheduler & Optimizer
+batch_size = 1 # per_device
+accumulative_counts = 16 # bs = 1 GPU * 1 batch_size_per_device * 16 acc
+dataloader_num_workers = 0
+max_epochs = 1
+optim_type = AdamW
+lr = 2e-5
+betas = (0.9, 0.999)
+weight_decay = 0
+max_norm = 1 # grad clip
+warmup_ratio = 0.03
+
+# Save
+save_steps = 500
+save_total_limit = 2 # Maximum checkpoints to keep (-1 means unlimited)
+
+# Evaluate the generation performance during the training
+evaluation_freq = 500
+SYSTEM = ''
+evaluation_inputs = ['上海是', 'Shanghai is']
+
+#######################################################################
+# PART 2 Model & Tokenizer #
+#######################################################################
+tokenizer = dict(
+ type=AutoTokenizer.from_pretrained,
+ pretrained_model_name_or_path=pretrained_model_name_or_path,
+ trust_remote_code=True,
+ padding_side='right')
+
+model = dict(
+ type=SupervisedFinetune,
+ use_varlen_attn=use_varlen_attn,
+ llm=dict(
+ type=AutoModelForCausalLM.from_pretrained,
+ pretrained_model_name_or_path=pretrained_model_name_or_path,
+ trust_remote_code=True))
+
+#######################################################################
+# PART 3 Dataset & Dataloader #
+#######################################################################
+train_dataset = dict(
+ type=process_hf_dataset,
+ dataset=dict(type=load_dataset, path='json', data_files=data_files),
+ tokenizer=tokenizer,
+ max_length=max_length,
+ dataset_map_fn=pretrain_map_fn,
+ template_map_fn=None,
+ remove_unused_columns=True,
+ shuffle_before_pack=False,
+ pack_to_max_length=pack_to_max_length,
+ use_varlen_attn=use_varlen_attn)
+
+train_dataloader = dict(
+ batch_size=batch_size,
+ num_workers=dataloader_num_workers,
+ dataset=train_dataset,
+ sampler=dict(type=DefaultSampler, shuffle=True),
+ collate_fn=dict(type=default_collate_fn, use_varlen_attn=use_varlen_attn))
+
+#######################################################################
+# PART 4 Scheduler & Optimizer #
+#######################################################################
+# optimizer
+optim_wrapper = dict(
+ type=AmpOptimWrapper,
+ optimizer=dict(
+ type=optim_type, lr=lr, betas=betas, weight_decay=weight_decay),
+ clip_grad=dict(max_norm=max_norm, error_if_nonfinite=False),
+ accumulative_counts=accumulative_counts,
+ loss_scale='dynamic',
+ dtype='float16')
+
+# learning policy
+# More information: https://github.com/open-mmlab/mmengine/blob/main/docs/en/tutorials/param_scheduler.md # noqa: E501
+param_scheduler = [
+ dict(
+ type=LinearLR,
+ start_factor=1e-5,
+ by_epoch=True,
+ begin=0,
+ end=warmup_ratio * max_epochs,
+ convert_to_iter_based=True),
+ dict(
+ type=CosineAnnealingLR,
+ eta_min=0.0,
+ by_epoch=True,
+ begin=warmup_ratio * max_epochs,
+ end=max_epochs,
+ convert_to_iter_based=True)
+]
+
+# train, val, test setting
+train_cfg = dict(type=TrainLoop, max_epochs=max_epochs)
+
+#######################################################################
+# PART 5 Runtime #
+#######################################################################
+# Log the dialogue periodically during the training process, optional
+custom_hooks = [
+ dict(type=DatasetInfoHook, tokenizer=tokenizer),
+ dict(
+ type=EvaluateChatHook,
+ tokenizer=tokenizer,
+ every_n_iters=evaluation_freq,
+ evaluation_inputs=evaluation_inputs,
+ system=SYSTEM)
+]
+
+if use_varlen_attn:
+ custom_hooks += [dict(type=VarlenAttnArgsToMessageHubHook)]
+
+# configure default hooks
+default_hooks = dict(
+ # record the time of every iteration.
+ timer=dict(type=IterTimerHook),
+ # print log every 10 iterations.
+ logger=dict(type=LoggerHook, log_metric_by_epoch=False, interval=10),
+ # enable the parameter scheduler.
+ param_scheduler=dict(type=ParamSchedulerHook),
+ # save checkpoint per `save_steps`.
+ checkpoint=dict(
+ type=CheckpointHook,
+ by_epoch=False,
+ interval=save_steps,
+ max_keep_ckpts=save_total_limit),
+ # set sampler seed in distributed evrionment.
+ sampler_seed=dict(type=DistSamplerSeedHook),
+)
+
+# configure environment
+env_cfg = dict(
+ # whether to enable cudnn benchmark
+ cudnn_benchmark=False,
+ # set multi process parameters
+ mp_cfg=dict(mp_start_method='fork', opencv_num_threads=0),
+ # set distributed parameters
+ dist_cfg=dict(backend='nccl'),
+)
+
+# set visualizer
+visualizer = None
+
+# set log level
+log_level = 'INFO'
+
+# load from which checkpoint
+load_from = None
+
+# whether to resume training from the loaded checkpoint
+resume = False
+
+# Defaults to use random seed and disable `deterministic`
+randomness = dict(seed=None, deterministic=False)
+
+# set log processor
+log_processor = dict(by_epoch=False)
diff --git a/xtuner/configs/custom_dataset/pretrain/zephyr/zephyr_7b_beta_full_custom_pretrain_e1.py b/xtuner/configs/custom_dataset/pretrain/zephyr/zephyr_7b_beta_full_custom_pretrain_e1.py
new file mode 100644
index 000000000..0065eff95
--- /dev/null
+++ b/xtuner/configs/custom_dataset/pretrain/zephyr/zephyr_7b_beta_full_custom_pretrain_e1.py
@@ -0,0 +1,199 @@
+# Copyright (c) OpenMMLab. All rights reserved.
+"""Data format:
+
+[
+ {
+ "text": "xxx"
+ },
+ {
+ "text": "xxx"
+ },
+ ...
+]
+""" # noqa: E501
+
+from datasets import load_dataset
+from mmengine.dataset import DefaultSampler
+from mmengine.hooks import (CheckpointHook, DistSamplerSeedHook, IterTimerHook,
+ LoggerHook, ParamSchedulerHook)
+from mmengine.optim import AmpOptimWrapper, CosineAnnealingLR, LinearLR
+from torch.optim import AdamW
+from transformers import AutoModelForCausalLM, AutoTokenizer
+
+from xtuner.dataset import process_hf_dataset
+from xtuner.dataset.collate_fns import default_collate_fn
+from xtuner.dataset.map_fns import pretrain_map_fn
+from xtuner.engine.hooks import (DatasetInfoHook, EvaluateChatHook,
+ VarlenAttnArgsToMessageHubHook)
+from xtuner.engine.runner import TrainLoop
+from xtuner.model import SupervisedFinetune
+
+#######################################################################
+# PART 1 Settings #
+#######################################################################
+# Model
+pretrained_model_name_or_path = 'HuggingFaceH4/zephyr-7b-beta'
+use_varlen_attn = False
+
+# Data
+data_files = ['/path/to/json/file.json']
+max_length = 2048
+pack_to_max_length = True
+
+# Scheduler & Optimizer
+batch_size = 1 # per_device
+accumulative_counts = 16 # bs = 1 GPU * 1 batch_size_per_device * 16 acc
+dataloader_num_workers = 0
+max_epochs = 1
+optim_type = AdamW
+lr = 2e-5
+betas = (0.9, 0.999)
+weight_decay = 0
+max_norm = 1 # grad clip
+warmup_ratio = 0.03
+
+# Save
+save_steps = 500
+save_total_limit = 2 # Maximum checkpoints to keep (-1 means unlimited)
+
+# Evaluate the generation performance during the training
+evaluation_freq = 500
+SYSTEM = ''
+evaluation_inputs = ['上海是', 'Shanghai is']
+
+#######################################################################
+# PART 2 Model & Tokenizer #
+#######################################################################
+tokenizer = dict(
+ type=AutoTokenizer.from_pretrained,
+ pretrained_model_name_or_path=pretrained_model_name_or_path,
+ trust_remote_code=True,
+ padding_side='right')
+
+model = dict(
+ type=SupervisedFinetune,
+ use_varlen_attn=use_varlen_attn,
+ llm=dict(
+ type=AutoModelForCausalLM.from_pretrained,
+ pretrained_model_name_or_path=pretrained_model_name_or_path,
+ trust_remote_code=True))
+
+#######################################################################
+# PART 3 Dataset & Dataloader #
+#######################################################################
+train_dataset = dict(
+ type=process_hf_dataset,
+ dataset=dict(type=load_dataset, path='json', data_files=data_files),
+ tokenizer=tokenizer,
+ max_length=max_length,
+ dataset_map_fn=pretrain_map_fn,
+ template_map_fn=None,
+ remove_unused_columns=True,
+ shuffle_before_pack=False,
+ pack_to_max_length=pack_to_max_length,
+ use_varlen_attn=use_varlen_attn)
+
+train_dataloader = dict(
+ batch_size=batch_size,
+ num_workers=dataloader_num_workers,
+ dataset=train_dataset,
+ sampler=dict(type=DefaultSampler, shuffle=True),
+ collate_fn=dict(type=default_collate_fn, use_varlen_attn=use_varlen_attn))
+
+#######################################################################
+# PART 4 Scheduler & Optimizer #
+#######################################################################
+# optimizer
+optim_wrapper = dict(
+ type=AmpOptimWrapper,
+ optimizer=dict(
+ type=optim_type, lr=lr, betas=betas, weight_decay=weight_decay),
+ clip_grad=dict(max_norm=max_norm, error_if_nonfinite=False),
+ accumulative_counts=accumulative_counts,
+ loss_scale='dynamic',
+ dtype='float16')
+
+# learning policy
+# More information: https://github.com/open-mmlab/mmengine/blob/main/docs/en/tutorials/param_scheduler.md # noqa: E501
+param_scheduler = [
+ dict(
+ type=LinearLR,
+ start_factor=1e-5,
+ by_epoch=True,
+ begin=0,
+ end=warmup_ratio * max_epochs,
+ convert_to_iter_based=True),
+ dict(
+ type=CosineAnnealingLR,
+ eta_min=0.0,
+ by_epoch=True,
+ begin=warmup_ratio * max_epochs,
+ end=max_epochs,
+ convert_to_iter_based=True)
+]
+
+# train, val, test setting
+train_cfg = dict(type=TrainLoop, max_epochs=max_epochs)
+
+#######################################################################
+# PART 5 Runtime #
+#######################################################################
+# Log the dialogue periodically during the training process, optional
+custom_hooks = [
+ dict(type=DatasetInfoHook, tokenizer=tokenizer),
+ dict(
+ type=EvaluateChatHook,
+ tokenizer=tokenizer,
+ every_n_iters=evaluation_freq,
+ evaluation_inputs=evaluation_inputs,
+ system=SYSTEM)
+]
+
+if use_varlen_attn:
+ custom_hooks += [dict(type=VarlenAttnArgsToMessageHubHook)]
+
+# configure default hooks
+default_hooks = dict(
+ # record the time of every iteration.
+ timer=dict(type=IterTimerHook),
+ # print log every 10 iterations.
+ logger=dict(type=LoggerHook, log_metric_by_epoch=False, interval=10),
+ # enable the parameter scheduler.
+ param_scheduler=dict(type=ParamSchedulerHook),
+ # save checkpoint per `save_steps`.
+ checkpoint=dict(
+ type=CheckpointHook,
+ by_epoch=False,
+ interval=save_steps,
+ max_keep_ckpts=save_total_limit),
+ # set sampler seed in distributed evrionment.
+ sampler_seed=dict(type=DistSamplerSeedHook),
+)
+
+# configure environment
+env_cfg = dict(
+ # whether to enable cudnn benchmark
+ cudnn_benchmark=False,
+ # set multi process parameters
+ mp_cfg=dict(mp_start_method='fork', opencv_num_threads=0),
+ # set distributed parameters
+ dist_cfg=dict(backend='nccl'),
+)
+
+# set visualizer
+visualizer = None
+
+# set log level
+log_level = 'INFO'
+
+# load from which checkpoint
+load_from = None
+
+# whether to resume training from the loaded checkpoint
+resume = False
+
+# Defaults to use random seed and disable `deterministic`
+randomness = dict(seed=None, deterministic=False)
+
+# set log processor
+log_processor = dict(by_epoch=False)
diff --git a/xtuner/configs/custom_dataset/sft/baichuan/baichuan2_13b_chat_qlora_custom_sft_e1.py b/xtuner/configs/custom_dataset/sft/baichuan/baichuan2_13b_chat_qlora_custom_sft_e1.py
new file mode 100644
index 000000000..558887c04
--- /dev/null
+++ b/xtuner/configs/custom_dataset/sft/baichuan/baichuan2_13b_chat_qlora_custom_sft_e1.py
@@ -0,0 +1,226 @@
+# Copyright (c) OpenMMLab. All rights reserved.
+"""Data format:
+
+[{
+ "messages": [
+ { "role": "system", "content": "xxx." },
+ { "role": "user", "content": "xxx." },
+ { "role": "assistant", "content": "xxx.", "loss": false},
+ { "role": "user", "content": "xxx." },
+ { "role": "assistant", "content": "xxx.", "loss": true}
+ ]
+},
+...
+]
+""" # noqa: E501
+import torch
+from datasets import load_dataset
+from mmengine.dataset import DefaultSampler
+from mmengine.hooks import (CheckpointHook, DistSamplerSeedHook, IterTimerHook,
+ LoggerHook, ParamSchedulerHook)
+from mmengine.optim import AmpOptimWrapper, CosineAnnealingLR, LinearLR
+from peft import LoraConfig
+from torch.optim import AdamW
+from transformers import (AutoModelForCausalLM, AutoTokenizer,
+ BitsAndBytesConfig)
+
+from xtuner.dataset import process_hf_dataset
+from xtuner.dataset.collate_fns import default_collate_fn
+from xtuner.dataset.map_fns import openai_map_fn, template_map_fn_factory
+from xtuner.engine.hooks import (DatasetInfoHook, EvaluateChatHook,
+ VarlenAttnArgsToMessageHubHook)
+from xtuner.engine.runner import TrainLoop
+from xtuner.model import SupervisedFinetune
+from xtuner.utils import PROMPT_TEMPLATE
+
+#######################################################################
+# PART 1 Settings #
+#######################################################################
+# Model
+pretrained_model_name_or_path = 'baichuan-inc/Baichuan2-13B-Chat'
+use_varlen_attn = False
+
+# Data
+data_files = ['/path/to/json/file.json']
+prompt_template = PROMPT_TEMPLATE.baichuan2_chat
+max_length = 2048
+pack_to_max_length = True
+
+# Scheduler & Optimizer
+batch_size = 1 # per_device
+accumulative_counts = 16 # bs = 1 GPU * 1 batch_size_per_device * 16 acc
+dataloader_num_workers = 0
+max_epochs = 1
+optim_type = AdamW
+lr = 2e-4
+betas = (0.9, 0.999)
+weight_decay = 0
+max_norm = 1 # grad clip
+warmup_ratio = 0.03
+
+# Save
+save_steps = 500
+save_total_limit = 2 # Maximum checkpoints to keep (-1 means unlimited)
+
+# Evaluate the generation performance during the training
+evaluation_freq = 500
+SYSTEM = ''
+evaluation_inputs = [
+ '请给我介绍五个上海的景点', 'Please tell me five scenic spots in Shanghai'
+]
+
+#######################################################################
+# PART 2 Model & Tokenizer #
+#######################################################################
+tokenizer = dict(
+ type=AutoTokenizer.from_pretrained,
+ pretrained_model_name_or_path=pretrained_model_name_or_path,
+ trust_remote_code=True,
+ padding_side='right')
+
+model = dict(
+ type=SupervisedFinetune,
+ use_varlen_attn=use_varlen_attn,
+ llm=dict(
+ type=AutoModelForCausalLM.from_pretrained,
+ pretrained_model_name_or_path=pretrained_model_name_or_path,
+ trust_remote_code=True,
+ torch_dtype=torch.float16,
+ quantization_config=dict(
+ type=BitsAndBytesConfig,
+ load_in_4bit=True,
+ load_in_8bit=False,
+ llm_int8_threshold=6.0,
+ llm_int8_has_fp16_weight=False,
+ bnb_4bit_compute_dtype=torch.float16,
+ bnb_4bit_use_double_quant=True,
+ bnb_4bit_quant_type='nf4')),
+ lora=dict(
+ type=LoraConfig,
+ r=64,
+ lora_alpha=16,
+ lora_dropout=0.1,
+ bias='none',
+ task_type='CAUSAL_LM'))
+
+#######################################################################
+# PART 3 Dataset & Dataloader #
+#######################################################################
+train_dataset = dict(
+ type=process_hf_dataset,
+ dataset=dict(type=load_dataset, path='json', data_files=data_files),
+ tokenizer=tokenizer,
+ max_length=max_length,
+ dataset_map_fn=openai_map_fn,
+ template_map_fn=dict(
+ type=template_map_fn_factory, template=prompt_template),
+ remove_unused_columns=True,
+ shuffle_before_pack=True,
+ pack_to_max_length=pack_to_max_length,
+ use_varlen_attn=use_varlen_attn)
+
+train_dataloader = dict(
+ batch_size=batch_size,
+ num_workers=dataloader_num_workers,
+ dataset=train_dataset,
+ sampler=dict(type=DefaultSampler, shuffle=True),
+ collate_fn=dict(type=default_collate_fn, use_varlen_attn=use_varlen_attn))
+
+#######################################################################
+# PART 4 Scheduler & Optimizer #
+#######################################################################
+# optimizer
+optim_wrapper = dict(
+ type=AmpOptimWrapper,
+ optimizer=dict(
+ type=optim_type, lr=lr, betas=betas, weight_decay=weight_decay),
+ clip_grad=dict(max_norm=max_norm, error_if_nonfinite=False),
+ accumulative_counts=accumulative_counts,
+ loss_scale='dynamic',
+ dtype='float16')
+
+# learning policy
+# More information: https://github.com/open-mmlab/mmengine/blob/main/docs/en/tutorials/param_scheduler.md # noqa: E501
+param_scheduler = [
+ dict(
+ type=LinearLR,
+ start_factor=1e-5,
+ by_epoch=True,
+ begin=0,
+ end=warmup_ratio * max_epochs,
+ convert_to_iter_based=True),
+ dict(
+ type=CosineAnnealingLR,
+ eta_min=0.0,
+ by_epoch=True,
+ begin=warmup_ratio * max_epochs,
+ end=max_epochs,
+ convert_to_iter_based=True)
+]
+
+# train, val, test setting
+train_cfg = dict(type=TrainLoop, max_epochs=max_epochs)
+
+#######################################################################
+# PART 5 Runtime #
+#######################################################################
+# Log the dialogue periodically during the training process, optional
+custom_hooks = [
+ dict(type=DatasetInfoHook, tokenizer=tokenizer),
+ dict(
+ type=EvaluateChatHook,
+ tokenizer=tokenizer,
+ every_n_iters=evaluation_freq,
+ evaluation_inputs=evaluation_inputs,
+ system=SYSTEM,
+ prompt_template=prompt_template)
+]
+
+if use_varlen_attn:
+ custom_hooks += [dict(type=VarlenAttnArgsToMessageHubHook)]
+
+# configure default hooks
+default_hooks = dict(
+ # record the time of every iteration.
+ timer=dict(type=IterTimerHook),
+ # print log every 10 iterations.
+ logger=dict(type=LoggerHook, log_metric_by_epoch=False, interval=10),
+ # enable the parameter scheduler.
+ param_scheduler=dict(type=ParamSchedulerHook),
+ # save checkpoint per `save_steps`.
+ checkpoint=dict(
+ type=CheckpointHook,
+ by_epoch=False,
+ interval=save_steps,
+ max_keep_ckpts=save_total_limit),
+ # set sampler seed in distributed evrionment.
+ sampler_seed=dict(type=DistSamplerSeedHook),
+)
+
+# configure environment
+env_cfg = dict(
+ # whether to enable cudnn benchmark
+ cudnn_benchmark=False,
+ # set multi process parameters
+ mp_cfg=dict(mp_start_method='fork', opencv_num_threads=0),
+ # set distributed parameters
+ dist_cfg=dict(backend='nccl'),
+)
+
+# set visualizer
+visualizer = None
+
+# set log level
+log_level = 'INFO'
+
+# load from which checkpoint
+load_from = None
+
+# whether to resume training from the loaded checkpoint
+resume = False
+
+# Defaults to use random seed and disable `deterministic`
+randomness = dict(seed=None, deterministic=False)
+
+# set log processor
+log_processor = dict(by_epoch=False)
diff --git a/xtuner/configs/custom_dataset/sft/baichuan/baichuan2_7b_chat_qlora_custom_sft_e1.py b/xtuner/configs/custom_dataset/sft/baichuan/baichuan2_7b_chat_qlora_custom_sft_e1.py
new file mode 100644
index 000000000..8df388a67
--- /dev/null
+++ b/xtuner/configs/custom_dataset/sft/baichuan/baichuan2_7b_chat_qlora_custom_sft_e1.py
@@ -0,0 +1,226 @@
+# Copyright (c) OpenMMLab. All rights reserved.
+"""Data format:
+
+[{
+ "messages": [
+ { "role": "system", "content": "xxx." },
+ { "role": "user", "content": "xxx." },
+ { "role": "assistant", "content": "xxx.", "loss": false},
+ { "role": "user", "content": "xxx." },
+ { "role": "assistant", "content": "xxx.", "loss": true}
+ ]
+},
+...
+]
+""" # noqa: E501
+import torch
+from datasets import load_dataset
+from mmengine.dataset import DefaultSampler
+from mmengine.hooks import (CheckpointHook, DistSamplerSeedHook, IterTimerHook,
+ LoggerHook, ParamSchedulerHook)
+from mmengine.optim import AmpOptimWrapper, CosineAnnealingLR, LinearLR
+from peft import LoraConfig
+from torch.optim import AdamW
+from transformers import (AutoModelForCausalLM, AutoTokenizer,
+ BitsAndBytesConfig)
+
+from xtuner.dataset import process_hf_dataset
+from xtuner.dataset.collate_fns import default_collate_fn
+from xtuner.dataset.map_fns import openai_map_fn, template_map_fn_factory
+from xtuner.engine.hooks import (DatasetInfoHook, EvaluateChatHook,
+ VarlenAttnArgsToMessageHubHook)
+from xtuner.engine.runner import TrainLoop
+from xtuner.model import SupervisedFinetune
+from xtuner.utils import PROMPT_TEMPLATE
+
+#######################################################################
+# PART 1 Settings #
+#######################################################################
+# Model
+pretrained_model_name_or_path = 'baichuan-inc/Baichuan2-7B-Chat'
+use_varlen_attn = False
+
+# Data
+data_files = ['/path/to/json/file.json']
+prompt_template = PROMPT_TEMPLATE.baichuan2_chat
+max_length = 2048
+pack_to_max_length = True
+
+# Scheduler & Optimizer
+batch_size = 1 # per_device
+accumulative_counts = 16 # bs = 1 GPU * 1 batch_size_per_device * 16 acc
+dataloader_num_workers = 0
+max_epochs = 1
+optim_type = AdamW
+lr = 2e-4
+betas = (0.9, 0.999)
+weight_decay = 0
+max_norm = 1 # grad clip
+warmup_ratio = 0.03
+
+# Save
+save_steps = 500
+save_total_limit = 2 # Maximum checkpoints to keep (-1 means unlimited)
+
+# Evaluate the generation performance during the training
+evaluation_freq = 500
+SYSTEM = ''
+evaluation_inputs = [
+ '请给我介绍五个上海的景点', 'Please tell me five scenic spots in Shanghai'
+]
+
+#######################################################################
+# PART 2 Model & Tokenizer #
+#######################################################################
+tokenizer = dict(
+ type=AutoTokenizer.from_pretrained,
+ pretrained_model_name_or_path=pretrained_model_name_or_path,
+ trust_remote_code=True,
+ padding_side='right')
+
+model = dict(
+ type=SupervisedFinetune,
+ use_varlen_attn=use_varlen_attn,
+ llm=dict(
+ type=AutoModelForCausalLM.from_pretrained,
+ pretrained_model_name_or_path=pretrained_model_name_or_path,
+ trust_remote_code=True,
+ torch_dtype=torch.float16,
+ quantization_config=dict(
+ type=BitsAndBytesConfig,
+ load_in_4bit=True,
+ load_in_8bit=False,
+ llm_int8_threshold=6.0,
+ llm_int8_has_fp16_weight=False,
+ bnb_4bit_compute_dtype=torch.float16,
+ bnb_4bit_use_double_quant=True,
+ bnb_4bit_quant_type='nf4')),
+ lora=dict(
+ type=LoraConfig,
+ r=64,
+ lora_alpha=16,
+ lora_dropout=0.1,
+ bias='none',
+ task_type='CAUSAL_LM'))
+
+#######################################################################
+# PART 3 Dataset & Dataloader #
+#######################################################################
+train_dataset = dict(
+ type=process_hf_dataset,
+ dataset=dict(type=load_dataset, path='json', data_files=data_files),
+ tokenizer=tokenizer,
+ max_length=max_length,
+ dataset_map_fn=openai_map_fn,
+ template_map_fn=dict(
+ type=template_map_fn_factory, template=prompt_template),
+ remove_unused_columns=True,
+ shuffle_before_pack=True,
+ pack_to_max_length=pack_to_max_length,
+ use_varlen_attn=use_varlen_attn)
+
+train_dataloader = dict(
+ batch_size=batch_size,
+ num_workers=dataloader_num_workers,
+ dataset=train_dataset,
+ sampler=dict(type=DefaultSampler, shuffle=True),
+ collate_fn=dict(type=default_collate_fn, use_varlen_attn=use_varlen_attn))
+
+#######################################################################
+# PART 4 Scheduler & Optimizer #
+#######################################################################
+# optimizer
+optim_wrapper = dict(
+ type=AmpOptimWrapper,
+ optimizer=dict(
+ type=optim_type, lr=lr, betas=betas, weight_decay=weight_decay),
+ clip_grad=dict(max_norm=max_norm, error_if_nonfinite=False),
+ accumulative_counts=accumulative_counts,
+ loss_scale='dynamic',
+ dtype='float16')
+
+# learning policy
+# More information: https://github.com/open-mmlab/mmengine/blob/main/docs/en/tutorials/param_scheduler.md # noqa: E501
+param_scheduler = [
+ dict(
+ type=LinearLR,
+ start_factor=1e-5,
+ by_epoch=True,
+ begin=0,
+ end=warmup_ratio * max_epochs,
+ convert_to_iter_based=True),
+ dict(
+ type=CosineAnnealingLR,
+ eta_min=0.0,
+ by_epoch=True,
+ begin=warmup_ratio * max_epochs,
+ end=max_epochs,
+ convert_to_iter_based=True)
+]
+
+# train, val, test setting
+train_cfg = dict(type=TrainLoop, max_epochs=max_epochs)
+
+#######################################################################
+# PART 5 Runtime #
+#######################################################################
+# Log the dialogue periodically during the training process, optional
+custom_hooks = [
+ dict(type=DatasetInfoHook, tokenizer=tokenizer),
+ dict(
+ type=EvaluateChatHook,
+ tokenizer=tokenizer,
+ every_n_iters=evaluation_freq,
+ evaluation_inputs=evaluation_inputs,
+ system=SYSTEM,
+ prompt_template=prompt_template)
+]
+
+if use_varlen_attn:
+ custom_hooks += [dict(type=VarlenAttnArgsToMessageHubHook)]
+
+# configure default hooks
+default_hooks = dict(
+ # record the time of every iteration.
+ timer=dict(type=IterTimerHook),
+ # print log every 10 iterations.
+ logger=dict(type=LoggerHook, log_metric_by_epoch=False, interval=10),
+ # enable the parameter scheduler.
+ param_scheduler=dict(type=ParamSchedulerHook),
+ # save checkpoint per `save_steps`.
+ checkpoint=dict(
+ type=CheckpointHook,
+ by_epoch=False,
+ interval=save_steps,
+ max_keep_ckpts=save_total_limit),
+ # set sampler seed in distributed evrionment.
+ sampler_seed=dict(type=DistSamplerSeedHook),
+)
+
+# configure environment
+env_cfg = dict(
+ # whether to enable cudnn benchmark
+ cudnn_benchmark=False,
+ # set multi process parameters
+ mp_cfg=dict(mp_start_method='fork', opencv_num_threads=0),
+ # set distributed parameters
+ dist_cfg=dict(backend='nccl'),
+)
+
+# set visualizer
+visualizer = None
+
+# set log level
+log_level = 'INFO'
+
+# load from which checkpoint
+load_from = None
+
+# whether to resume training from the loaded checkpoint
+resume = False
+
+# Defaults to use random seed and disable `deterministic`
+randomness = dict(seed=None, deterministic=False)
+
+# set log processor
+log_processor = dict(by_epoch=False)
diff --git a/xtuner/configs/custom_dataset/sft/baichuan/baichuan_13b_chat_qlora_custom_sft_e1.py b/xtuner/configs/custom_dataset/sft/baichuan/baichuan_13b_chat_qlora_custom_sft_e1.py
new file mode 100644
index 000000000..3dc38eb4f
--- /dev/null
+++ b/xtuner/configs/custom_dataset/sft/baichuan/baichuan_13b_chat_qlora_custom_sft_e1.py
@@ -0,0 +1,226 @@
+# Copyright (c) OpenMMLab. All rights reserved.
+"""Data format:
+
+[{
+ "messages": [
+ { "role": "system", "content": "xxx." },
+ { "role": "user", "content": "xxx." },
+ { "role": "assistant", "content": "xxx.", "loss": false},
+ { "role": "user", "content": "xxx." },
+ { "role": "assistant", "content": "xxx.", "loss": true}
+ ]
+},
+...
+]
+""" # noqa: E501
+import torch
+from datasets import load_dataset
+from mmengine.dataset import DefaultSampler
+from mmengine.hooks import (CheckpointHook, DistSamplerSeedHook, IterTimerHook,
+ LoggerHook, ParamSchedulerHook)
+from mmengine.optim import AmpOptimWrapper, CosineAnnealingLR, LinearLR
+from peft import LoraConfig
+from torch.optim import AdamW
+from transformers import (AutoModelForCausalLM, AutoTokenizer,
+ BitsAndBytesConfig)
+
+from xtuner.dataset import process_hf_dataset
+from xtuner.dataset.collate_fns import default_collate_fn
+from xtuner.dataset.map_fns import openai_map_fn, template_map_fn_factory
+from xtuner.engine.hooks import (DatasetInfoHook, EvaluateChatHook,
+ VarlenAttnArgsToMessageHubHook)
+from xtuner.engine.runner import TrainLoop
+from xtuner.model import SupervisedFinetune
+from xtuner.utils import PROMPT_TEMPLATE
+
+#######################################################################
+# PART 1 Settings #
+#######################################################################
+# Model
+pretrained_model_name_or_path = 'baichuan-inc/Baichuan-13B-Chat'
+use_varlen_attn = False
+
+# Data
+data_files = ['/path/to/json/file.json']
+prompt_template = PROMPT_TEMPLATE.baichuan_chat
+max_length = 2048
+pack_to_max_length = True
+
+# Scheduler & Optimizer
+batch_size = 1 # per_device
+accumulative_counts = 16 # bs = 1 GPU * 1 batch_size_per_device * 16 acc
+dataloader_num_workers = 0
+max_epochs = 1
+optim_type = AdamW
+lr = 2e-4
+betas = (0.9, 0.999)
+weight_decay = 0
+max_norm = 1 # grad clip
+warmup_ratio = 0.03
+
+# Save
+save_steps = 500
+save_total_limit = 2 # Maximum checkpoints to keep (-1 means unlimited)
+
+# Evaluate the generation performance during the training
+evaluation_freq = 500
+SYSTEM = ''
+evaluation_inputs = [
+ '请给我介绍五个上海的景点', 'Please tell me five scenic spots in Shanghai'
+]
+
+#######################################################################
+# PART 2 Model & Tokenizer #
+#######################################################################
+tokenizer = dict(
+ type=AutoTokenizer.from_pretrained,
+ pretrained_model_name_or_path=pretrained_model_name_or_path,
+ trust_remote_code=True,
+ padding_side='right')
+
+model = dict(
+ type=SupervisedFinetune,
+ use_varlen_attn=use_varlen_attn,
+ llm=dict(
+ type=AutoModelForCausalLM.from_pretrained,
+ pretrained_model_name_or_path=pretrained_model_name_or_path,
+ trust_remote_code=True,
+ torch_dtype=torch.float16,
+ quantization_config=dict(
+ type=BitsAndBytesConfig,
+ load_in_4bit=True,
+ load_in_8bit=False,
+ llm_int8_threshold=6.0,
+ llm_int8_has_fp16_weight=False,
+ bnb_4bit_compute_dtype=torch.float16,
+ bnb_4bit_use_double_quant=True,
+ bnb_4bit_quant_type='nf4')),
+ lora=dict(
+ type=LoraConfig,
+ r=64,
+ lora_alpha=16,
+ lora_dropout=0.1,
+ bias='none',
+ task_type='CAUSAL_LM'))
+
+#######################################################################
+# PART 3 Dataset & Dataloader #
+#######################################################################
+train_dataset = dict(
+ type=process_hf_dataset,
+ dataset=dict(type=load_dataset, path='json', data_files=data_files),
+ tokenizer=tokenizer,
+ max_length=max_length,
+ dataset_map_fn=openai_map_fn,
+ template_map_fn=dict(
+ type=template_map_fn_factory, template=prompt_template),
+ remove_unused_columns=True,
+ shuffle_before_pack=True,
+ pack_to_max_length=pack_to_max_length,
+ use_varlen_attn=use_varlen_attn)
+
+train_dataloader = dict(
+ batch_size=batch_size,
+ num_workers=dataloader_num_workers,
+ dataset=train_dataset,
+ sampler=dict(type=DefaultSampler, shuffle=True),
+ collate_fn=dict(type=default_collate_fn, use_varlen_attn=use_varlen_attn))
+
+#######################################################################
+# PART 4 Scheduler & Optimizer #
+#######################################################################
+# optimizer
+optim_wrapper = dict(
+ type=AmpOptimWrapper,
+ optimizer=dict(
+ type=optim_type, lr=lr, betas=betas, weight_decay=weight_decay),
+ clip_grad=dict(max_norm=max_norm, error_if_nonfinite=False),
+ accumulative_counts=accumulative_counts,
+ loss_scale='dynamic',
+ dtype='float16')
+
+# learning policy
+# More information: https://github.com/open-mmlab/mmengine/blob/main/docs/en/tutorials/param_scheduler.md # noqa: E501
+param_scheduler = [
+ dict(
+ type=LinearLR,
+ start_factor=1e-5,
+ by_epoch=True,
+ begin=0,
+ end=warmup_ratio * max_epochs,
+ convert_to_iter_based=True),
+ dict(
+ type=CosineAnnealingLR,
+ eta_min=0.0,
+ by_epoch=True,
+ begin=warmup_ratio * max_epochs,
+ end=max_epochs,
+ convert_to_iter_based=True)
+]
+
+# train, val, test setting
+train_cfg = dict(type=TrainLoop, max_epochs=max_epochs)
+
+#######################################################################
+# PART 5 Runtime #
+#######################################################################
+# Log the dialogue periodically during the training process, optional
+custom_hooks = [
+ dict(type=DatasetInfoHook, tokenizer=tokenizer),
+ dict(
+ type=EvaluateChatHook,
+ tokenizer=tokenizer,
+ every_n_iters=evaluation_freq,
+ evaluation_inputs=evaluation_inputs,
+ system=SYSTEM,
+ prompt_template=prompt_template)
+]
+
+if use_varlen_attn:
+ custom_hooks += [dict(type=VarlenAttnArgsToMessageHubHook)]
+
+# configure default hooks
+default_hooks = dict(
+ # record the time of every iteration.
+ timer=dict(type=IterTimerHook),
+ # print log every 10 iterations.
+ logger=dict(type=LoggerHook, log_metric_by_epoch=False, interval=10),
+ # enable the parameter scheduler.
+ param_scheduler=dict(type=ParamSchedulerHook),
+ # save checkpoint per `save_steps`.
+ checkpoint=dict(
+ type=CheckpointHook,
+ by_epoch=False,
+ interval=save_steps,
+ max_keep_ckpts=save_total_limit),
+ # set sampler seed in distributed evrionment.
+ sampler_seed=dict(type=DistSamplerSeedHook),
+)
+
+# configure environment
+env_cfg = dict(
+ # whether to enable cudnn benchmark
+ cudnn_benchmark=False,
+ # set multi process parameters
+ mp_cfg=dict(mp_start_method='fork', opencv_num_threads=0),
+ # set distributed parameters
+ dist_cfg=dict(backend='nccl'),
+)
+
+# set visualizer
+visualizer = None
+
+# set log level
+log_level = 'INFO'
+
+# load from which checkpoint
+load_from = None
+
+# whether to resume training from the loaded checkpoint
+resume = False
+
+# Defaults to use random seed and disable `deterministic`
+randomness = dict(seed=None, deterministic=False)
+
+# set log processor
+log_processor = dict(by_epoch=False)
diff --git a/xtuner/configs/custom_dataset/sft/baichuan/baichuan_7b_qlora_custom_sft_e1.py b/xtuner/configs/custom_dataset/sft/baichuan/baichuan_7b_qlora_custom_sft_e1.py
new file mode 100644
index 000000000..dc15b6289
--- /dev/null
+++ b/xtuner/configs/custom_dataset/sft/baichuan/baichuan_7b_qlora_custom_sft_e1.py
@@ -0,0 +1,226 @@
+# Copyright (c) OpenMMLab. All rights reserved.
+"""Data format:
+
+[{
+ "messages": [
+ { "role": "system", "content": "xxx." },
+ { "role": "user", "content": "xxx." },
+ { "role": "assistant", "content": "xxx.", "loss": false},
+ { "role": "user", "content": "xxx." },
+ { "role": "assistant", "content": "xxx.", "loss": true}
+ ]
+},
+...
+]
+""" # noqa: E501
+import torch
+from datasets import load_dataset
+from mmengine.dataset import DefaultSampler
+from mmengine.hooks import (CheckpointHook, DistSamplerSeedHook, IterTimerHook,
+ LoggerHook, ParamSchedulerHook)
+from mmengine.optim import AmpOptimWrapper, CosineAnnealingLR, LinearLR
+from peft import LoraConfig
+from torch.optim import AdamW
+from transformers import (AutoModelForCausalLM, AutoTokenizer,
+ BitsAndBytesConfig)
+
+from xtuner.dataset import process_hf_dataset
+from xtuner.dataset.collate_fns import default_collate_fn
+from xtuner.dataset.map_fns import openai_map_fn, template_map_fn_factory
+from xtuner.engine.hooks import (DatasetInfoHook, EvaluateChatHook,
+ VarlenAttnArgsToMessageHubHook)
+from xtuner.engine.runner import TrainLoop
+from xtuner.model import SupervisedFinetune
+from xtuner.utils import PROMPT_TEMPLATE
+
+#######################################################################
+# PART 1 Settings #
+#######################################################################
+# Model
+pretrained_model_name_or_path = 'baichuan-inc/Baichuan-7B'
+use_varlen_attn = False
+
+# Data
+data_files = ['/path/to/json/file.json']
+prompt_template = PROMPT_TEMPLATE.default
+max_length = 2048
+pack_to_max_length = True
+
+# Scheduler & Optimizer
+batch_size = 1 # per_device
+accumulative_counts = 16 # bs = 1 GPU * 1 batch_size_per_device * 16 acc
+dataloader_num_workers = 0
+max_epochs = 1
+optim_type = AdamW
+lr = 2e-4
+betas = (0.9, 0.999)
+weight_decay = 0
+max_norm = 1 # grad clip
+warmup_ratio = 0.03
+
+# Save
+save_steps = 500
+save_total_limit = 2 # Maximum checkpoints to keep (-1 means unlimited)
+
+# Evaluate the generation performance during the training
+evaluation_freq = 500
+SYSTEM = ''
+evaluation_inputs = [
+ '请给我介绍五个上海的景点', 'Please tell me five scenic spots in Shanghai'
+]
+
+#######################################################################
+# PART 2 Model & Tokenizer #
+#######################################################################
+tokenizer = dict(
+ type=AutoTokenizer.from_pretrained,
+ pretrained_model_name_or_path=pretrained_model_name_or_path,
+ trust_remote_code=True,
+ padding_side='right')
+
+model = dict(
+ type=SupervisedFinetune,
+ use_varlen_attn=use_varlen_attn,
+ llm=dict(
+ type=AutoModelForCausalLM.from_pretrained,
+ pretrained_model_name_or_path=pretrained_model_name_or_path,
+ trust_remote_code=True,
+ torch_dtype=torch.float16,
+ quantization_config=dict(
+ type=BitsAndBytesConfig,
+ load_in_4bit=True,
+ load_in_8bit=False,
+ llm_int8_threshold=6.0,
+ llm_int8_has_fp16_weight=False,
+ bnb_4bit_compute_dtype=torch.float16,
+ bnb_4bit_use_double_quant=True,
+ bnb_4bit_quant_type='nf4')),
+ lora=dict(
+ type=LoraConfig,
+ r=64,
+ lora_alpha=16,
+ lora_dropout=0.1,
+ bias='none',
+ task_type='CAUSAL_LM'))
+
+#######################################################################
+# PART 3 Dataset & Dataloader #
+#######################################################################
+train_dataset = dict(
+ type=process_hf_dataset,
+ dataset=dict(type=load_dataset, path='json', data_files=data_files),
+ tokenizer=tokenizer,
+ max_length=max_length,
+ dataset_map_fn=openai_map_fn,
+ template_map_fn=dict(
+ type=template_map_fn_factory, template=prompt_template),
+ remove_unused_columns=True,
+ shuffle_before_pack=True,
+ pack_to_max_length=pack_to_max_length,
+ use_varlen_attn=use_varlen_attn)
+
+train_dataloader = dict(
+ batch_size=batch_size,
+ num_workers=dataloader_num_workers,
+ dataset=train_dataset,
+ sampler=dict(type=DefaultSampler, shuffle=True),
+ collate_fn=dict(type=default_collate_fn, use_varlen_attn=use_varlen_attn))
+
+#######################################################################
+# PART 4 Scheduler & Optimizer #
+#######################################################################
+# optimizer
+optim_wrapper = dict(
+ type=AmpOptimWrapper,
+ optimizer=dict(
+ type=optim_type, lr=lr, betas=betas, weight_decay=weight_decay),
+ clip_grad=dict(max_norm=max_norm, error_if_nonfinite=False),
+ accumulative_counts=accumulative_counts,
+ loss_scale='dynamic',
+ dtype='float16')
+
+# learning policy
+# More information: https://github.com/open-mmlab/mmengine/blob/main/docs/en/tutorials/param_scheduler.md # noqa: E501
+param_scheduler = [
+ dict(
+ type=LinearLR,
+ start_factor=1e-5,
+ by_epoch=True,
+ begin=0,
+ end=warmup_ratio * max_epochs,
+ convert_to_iter_based=True),
+ dict(
+ type=CosineAnnealingLR,
+ eta_min=0.0,
+ by_epoch=True,
+ begin=warmup_ratio * max_epochs,
+ end=max_epochs,
+ convert_to_iter_based=True)
+]
+
+# train, val, test setting
+train_cfg = dict(type=TrainLoop, max_epochs=max_epochs)
+
+#######################################################################
+# PART 5 Runtime #
+#######################################################################
+# Log the dialogue periodically during the training process, optional
+custom_hooks = [
+ dict(type=DatasetInfoHook, tokenizer=tokenizer),
+ dict(
+ type=EvaluateChatHook,
+ tokenizer=tokenizer,
+ every_n_iters=evaluation_freq,
+ evaluation_inputs=evaluation_inputs,
+ system=SYSTEM,
+ prompt_template=prompt_template)
+]
+
+if use_varlen_attn:
+ custom_hooks += [dict(type=VarlenAttnArgsToMessageHubHook)]
+
+# configure default hooks
+default_hooks = dict(
+ # record the time of every iteration.
+ timer=dict(type=IterTimerHook),
+ # print log every 10 iterations.
+ logger=dict(type=LoggerHook, log_metric_by_epoch=False, interval=10),
+ # enable the parameter scheduler.
+ param_scheduler=dict(type=ParamSchedulerHook),
+ # save checkpoint per `save_steps`.
+ checkpoint=dict(
+ type=CheckpointHook,
+ by_epoch=False,
+ interval=save_steps,
+ max_keep_ckpts=save_total_limit),
+ # set sampler seed in distributed evrionment.
+ sampler_seed=dict(type=DistSamplerSeedHook),
+)
+
+# configure environment
+env_cfg = dict(
+ # whether to enable cudnn benchmark
+ cudnn_benchmark=False,
+ # set multi process parameters
+ mp_cfg=dict(mp_start_method='fork', opencv_num_threads=0),
+ # set distributed parameters
+ dist_cfg=dict(backend='nccl'),
+)
+
+# set visualizer
+visualizer = None
+
+# set log level
+log_level = 'INFO'
+
+# load from which checkpoint
+load_from = None
+
+# whether to resume training from the loaded checkpoint
+resume = False
+
+# Defaults to use random seed and disable `deterministic`
+randomness = dict(seed=None, deterministic=False)
+
+# set log processor
+log_processor = dict(by_epoch=False)
diff --git a/xtuner/configs/custom_dataset/sft/chatglm/chatglm2_6b_qlora_custom_sft_e1.py b/xtuner/configs/custom_dataset/sft/chatglm/chatglm2_6b_qlora_custom_sft_e1.py
new file mode 100644
index 000000000..09b354929
--- /dev/null
+++ b/xtuner/configs/custom_dataset/sft/chatglm/chatglm2_6b_qlora_custom_sft_e1.py
@@ -0,0 +1,226 @@
+# Copyright (c) OpenMMLab. All rights reserved.
+"""Data format:
+
+[{
+ "messages": [
+ { "role": "system", "content": "xxx." },
+ { "role": "user", "content": "xxx." },
+ { "role": "assistant", "content": "xxx.", "loss": false},
+ { "role": "user", "content": "xxx." },
+ { "role": "assistant", "content": "xxx.", "loss": true}
+ ]
+},
+...
+]
+""" # noqa: E501
+import torch
+from datasets import load_dataset
+from mmengine.dataset import DefaultSampler
+from mmengine.hooks import (CheckpointHook, DistSamplerSeedHook, IterTimerHook,
+ LoggerHook, ParamSchedulerHook)
+from mmengine.optim import AmpOptimWrapper, CosineAnnealingLR, LinearLR
+from peft import LoraConfig
+from torch.optim import AdamW
+from transformers import (AutoModelForCausalLM, AutoTokenizer,
+ BitsAndBytesConfig)
+
+from xtuner.dataset import process_hf_dataset
+from xtuner.dataset.collate_fns import default_collate_fn
+from xtuner.dataset.map_fns import openai_map_fn, template_map_fn_factory
+from xtuner.engine.hooks import (DatasetInfoHook, EvaluateChatHook,
+ VarlenAttnArgsToMessageHubHook)
+from xtuner.engine.runner import TrainLoop
+from xtuner.model import SupervisedFinetune
+from xtuner.utils import PROMPT_TEMPLATE
+
+#######################################################################
+# PART 1 Settings #
+#######################################################################
+# Model
+pretrained_model_name_or_path = 'THUDM/chatglm2-6b'
+use_varlen_attn = False
+
+# Data
+data_files = ['/path/to/json/file.json']
+prompt_template = PROMPT_TEMPLATE.chatglm2
+max_length = 2048
+pack_to_max_length = True
+
+# Scheduler & Optimizer
+batch_size = 1 # per_device
+accumulative_counts = 16 # bs = 1 GPU * 1 batch_size_per_device * 16 acc
+dataloader_num_workers = 0
+max_epochs = 1
+optim_type = AdamW
+lr = 2e-4
+betas = (0.9, 0.999)
+weight_decay = 0
+max_norm = 1 # grad clip
+warmup_ratio = 0.03
+
+# Save
+save_steps = 500
+save_total_limit = 2 # Maximum checkpoints to keep (-1 means unlimited)
+
+# Evaluate the generation performance during the training
+evaluation_freq = 500
+SYSTEM = ''
+evaluation_inputs = [
+ '请给我介绍五个上海的景点', 'Please tell me five scenic spots in Shanghai'
+]
+
+#######################################################################
+# PART 2 Model & Tokenizer #
+#######################################################################
+tokenizer = dict(
+ type=AutoTokenizer.from_pretrained,
+ pretrained_model_name_or_path=pretrained_model_name_or_path,
+ trust_remote_code=True,
+ padding_side='left')
+
+model = dict(
+ type=SupervisedFinetune,
+ use_varlen_attn=use_varlen_attn,
+ llm=dict(
+ type=AutoModelForCausalLM.from_pretrained,
+ pretrained_model_name_or_path=pretrained_model_name_or_path,
+ trust_remote_code=True,
+ torch_dtype=torch.float16,
+ quantization_config=dict(
+ type=BitsAndBytesConfig,
+ load_in_4bit=True,
+ load_in_8bit=False,
+ llm_int8_threshold=6.0,
+ llm_int8_has_fp16_weight=False,
+ bnb_4bit_compute_dtype=torch.float16,
+ bnb_4bit_use_double_quant=True,
+ bnb_4bit_quant_type='nf4')),
+ lora=dict(
+ type=LoraConfig,
+ r=64,
+ lora_alpha=16,
+ lora_dropout=0.1,
+ bias='none',
+ task_type='CAUSAL_LM'))
+
+#######################################################################
+# PART 3 Dataset & Dataloader #
+#######################################################################
+train_dataset = dict(
+ type=process_hf_dataset,
+ dataset=dict(type=load_dataset, path='json', data_files=data_files),
+ tokenizer=tokenizer,
+ max_length=max_length,
+ dataset_map_fn=openai_map_fn,
+ template_map_fn=dict(
+ type=template_map_fn_factory, template=prompt_template),
+ remove_unused_columns=True,
+ shuffle_before_pack=True,
+ pack_to_max_length=pack_to_max_length,
+ use_varlen_attn=use_varlen_attn)
+
+train_dataloader = dict(
+ batch_size=batch_size,
+ num_workers=dataloader_num_workers,
+ dataset=train_dataset,
+ sampler=dict(type=DefaultSampler, shuffle=True),
+ collate_fn=dict(type=default_collate_fn, use_varlen_attn=use_varlen_attn))
+
+#######################################################################
+# PART 4 Scheduler & Optimizer #
+#######################################################################
+# optimizer
+optim_wrapper = dict(
+ type=AmpOptimWrapper,
+ optimizer=dict(
+ type=optim_type, lr=lr, betas=betas, weight_decay=weight_decay),
+ clip_grad=dict(max_norm=max_norm, error_if_nonfinite=False),
+ accumulative_counts=accumulative_counts,
+ loss_scale='dynamic',
+ dtype='float16')
+
+# learning policy
+# More information: https://github.com/open-mmlab/mmengine/blob/main/docs/en/tutorials/param_scheduler.md # noqa: E501
+param_scheduler = [
+ dict(
+ type=LinearLR,
+ start_factor=1e-5,
+ by_epoch=True,
+ begin=0,
+ end=warmup_ratio * max_epochs,
+ convert_to_iter_based=True),
+ dict(
+ type=CosineAnnealingLR,
+ eta_min=0.0,
+ by_epoch=True,
+ begin=warmup_ratio * max_epochs,
+ end=max_epochs,
+ convert_to_iter_based=True)
+]
+
+# train, val, test setting
+train_cfg = dict(type=TrainLoop, max_epochs=max_epochs)
+
+#######################################################################
+# PART 5 Runtime #
+#######################################################################
+# Log the dialogue periodically during the training process, optional
+custom_hooks = [
+ dict(type=DatasetInfoHook, tokenizer=tokenizer),
+ dict(
+ type=EvaluateChatHook,
+ tokenizer=tokenizer,
+ every_n_iters=evaluation_freq,
+ evaluation_inputs=evaluation_inputs,
+ system=SYSTEM,
+ prompt_template=prompt_template)
+]
+
+if use_varlen_attn:
+ custom_hooks += [dict(type=VarlenAttnArgsToMessageHubHook)]
+
+# configure default hooks
+default_hooks = dict(
+ # record the time of every iteration.
+ timer=dict(type=IterTimerHook),
+ # print log every 10 iterations.
+ logger=dict(type=LoggerHook, log_metric_by_epoch=False, interval=10),
+ # enable the parameter scheduler.
+ param_scheduler=dict(type=ParamSchedulerHook),
+ # save checkpoint per `save_steps`.
+ checkpoint=dict(
+ type=CheckpointHook,
+ by_epoch=False,
+ interval=save_steps,
+ max_keep_ckpts=save_total_limit),
+ # set sampler seed in distributed evrionment.
+ sampler_seed=dict(type=DistSamplerSeedHook),
+)
+
+# configure environment
+env_cfg = dict(
+ # whether to enable cudnn benchmark
+ cudnn_benchmark=False,
+ # set multi process parameters
+ mp_cfg=dict(mp_start_method='fork', opencv_num_threads=0),
+ # set distributed parameters
+ dist_cfg=dict(backend='nccl'),
+)
+
+# set visualizer
+visualizer = None
+
+# set log level
+log_level = 'INFO'
+
+# load from which checkpoint
+load_from = None
+
+# whether to resume training from the loaded checkpoint
+resume = False
+
+# Defaults to use random seed and disable `deterministic`
+randomness = dict(seed=None, deterministic=False)
+
+# set log processor
+log_processor = dict(by_epoch=False)
diff --git a/xtuner/configs/custom_dataset/sft/chatglm/chatglm3_6b_qlora_custom_sft_e1.py b/xtuner/configs/custom_dataset/sft/chatglm/chatglm3_6b_qlora_custom_sft_e1.py
new file mode 100644
index 000000000..7e3abba71
--- /dev/null
+++ b/xtuner/configs/custom_dataset/sft/chatglm/chatglm3_6b_qlora_custom_sft_e1.py
@@ -0,0 +1,227 @@
+# Copyright (c) OpenMMLab. All rights reserved.
+"""Data format:
+
+[{
+ "messages": [
+ { "role": "system", "content": "xxx." },
+ { "role": "user", "content": "xxx." },
+ { "role": "assistant", "content": "xxx.", "loss": false},
+ { "role": "user", "content": "xxx." },
+ { "role": "assistant", "content": "xxx.", "loss": true}
+ ]
+},
+...
+]
+""" # noqa: E501
+import torch
+from datasets import load_dataset
+from mmengine.dataset import DefaultSampler
+from mmengine.hooks import (CheckpointHook, DistSamplerSeedHook, IterTimerHook,
+ LoggerHook, ParamSchedulerHook)
+from mmengine.optim import AmpOptimWrapper, CosineAnnealingLR, LinearLR
+from peft import LoraConfig
+from torch.optim import AdamW
+from transformers import (AutoModelForCausalLM, AutoTokenizer,
+ BitsAndBytesConfig)
+
+from xtuner.dataset import process_hf_dataset
+from xtuner.dataset.collate_fns import default_collate_fn
+from xtuner.dataset.map_fns import openai_map_fn, template_map_fn_factory
+from xtuner.engine.hooks import (DatasetInfoHook, EvaluateChatHook,
+ VarlenAttnArgsToMessageHubHook)
+from xtuner.engine.runner import TrainLoop
+from xtuner.model import SupervisedFinetune
+from xtuner.utils import PROMPT_TEMPLATE
+
+#######################################################################
+# PART 1 Settings #
+#######################################################################
+# Model
+pretrained_model_name_or_path = 'THUDM/chatglm3-6b'
+use_varlen_attn = False
+
+# Data
+data_files = ['/path/to/json/file.json']
+prompt_template = PROMPT_TEMPLATE.chatglm3
+max_length = 2048
+pack_to_max_length = True
+
+# Scheduler & Optimizer
+batch_size = 1 # per_device
+accumulative_counts = 16 # bs = 1 GPU * 1 batch_size_per_device * 16 acc
+dataloader_num_workers = 0
+max_epochs = 1
+optim_type = AdamW
+lr = 2e-4
+betas = (0.9, 0.999)
+weight_decay = 0
+max_norm = 1 # grad clip
+warmup_ratio = 0.03
+
+# Save
+save_steps = 500
+save_total_limit = 2 # Maximum checkpoints to keep (-1 means unlimited)
+
+# Evaluate the generation performance during the training
+evaluation_freq = 500
+SYSTEM = ''
+evaluation_inputs = [
+ '请给我介绍五个上海的景点', 'Please tell me five scenic spots in Shanghai'
+]
+
+#######################################################################
+# PART 2 Model & Tokenizer #
+#######################################################################
+tokenizer = dict(
+ type=AutoTokenizer.from_pretrained,
+ pretrained_model_name_or_path=pretrained_model_name_or_path,
+ trust_remote_code=True,
+ encode_special_tokens=True,
+ padding_side='left')
+
+model = dict(
+ type=SupervisedFinetune,
+ use_varlen_attn=use_varlen_attn,
+ llm=dict(
+ type=AutoModelForCausalLM.from_pretrained,
+ pretrained_model_name_or_path=pretrained_model_name_or_path,
+ trust_remote_code=True,
+ torch_dtype=torch.float16,
+ quantization_config=dict(
+ type=BitsAndBytesConfig,
+ load_in_4bit=True,
+ load_in_8bit=False,
+ llm_int8_threshold=6.0,
+ llm_int8_has_fp16_weight=False,
+ bnb_4bit_compute_dtype=torch.float16,
+ bnb_4bit_use_double_quant=True,
+ bnb_4bit_quant_type='nf4')),
+ lora=dict(
+ type=LoraConfig,
+ r=64,
+ lora_alpha=16,
+ lora_dropout=0.1,
+ bias='none',
+ task_type='CAUSAL_LM'))
+
+#######################################################################
+# PART 3 Dataset & Dataloader #
+#######################################################################
+train_dataset = dict(
+ type=process_hf_dataset,
+ dataset=dict(type=load_dataset, path='json', data_files=data_files),
+ tokenizer=tokenizer,
+ max_length=max_length,
+ dataset_map_fn=openai_map_fn,
+ template_map_fn=dict(
+ type=template_map_fn_factory, template=prompt_template),
+ remove_unused_columns=True,
+ shuffle_before_pack=True,
+ pack_to_max_length=pack_to_max_length,
+ use_varlen_attn=use_varlen_attn)
+
+train_dataloader = dict(
+ batch_size=batch_size,
+ num_workers=dataloader_num_workers,
+ dataset=train_dataset,
+ sampler=dict(type=DefaultSampler, shuffle=True),
+ collate_fn=dict(type=default_collate_fn, use_varlen_attn=use_varlen_attn))
+
+#######################################################################
+# PART 4 Scheduler & Optimizer #
+#######################################################################
+# optimizer
+optim_wrapper = dict(
+ type=AmpOptimWrapper,
+ optimizer=dict(
+ type=optim_type, lr=lr, betas=betas, weight_decay=weight_decay),
+ clip_grad=dict(max_norm=max_norm, error_if_nonfinite=False),
+ accumulative_counts=accumulative_counts,
+ loss_scale='dynamic',
+ dtype='float16')
+
+# learning policy
+# More information: https://github.com/open-mmlab/mmengine/blob/main/docs/en/tutorials/param_scheduler.md # noqa: E501
+param_scheduler = [
+ dict(
+ type=LinearLR,
+ start_factor=1e-5,
+ by_epoch=True,
+ begin=0,
+ end=warmup_ratio * max_epochs,
+ convert_to_iter_based=True),
+ dict(
+ type=CosineAnnealingLR,
+ eta_min=0.0,
+ by_epoch=True,
+ begin=warmup_ratio * max_epochs,
+ end=max_epochs,
+ convert_to_iter_based=True)
+]
+
+# train, val, test setting
+train_cfg = dict(type=TrainLoop, max_epochs=max_epochs)
+
+#######################################################################
+# PART 5 Runtime #
+#######################################################################
+# Log the dialogue periodically during the training process, optional
+custom_hooks = [
+ dict(type=DatasetInfoHook, tokenizer=tokenizer),
+ dict(
+ type=EvaluateChatHook,
+ tokenizer=tokenizer,
+ every_n_iters=evaluation_freq,
+ evaluation_inputs=evaluation_inputs,
+ system=SYSTEM,
+ prompt_template=prompt_template)
+]
+
+if use_varlen_attn:
+ custom_hooks += [dict(type=VarlenAttnArgsToMessageHubHook)]
+
+# configure default hooks
+default_hooks = dict(
+ # record the time of every iteration.
+ timer=dict(type=IterTimerHook),
+ # print log every 10 iterations.
+ logger=dict(type=LoggerHook, log_metric_by_epoch=False, interval=10),
+ # enable the parameter scheduler.
+ param_scheduler=dict(type=ParamSchedulerHook),
+ # save checkpoint per `save_steps`.
+ checkpoint=dict(
+ type=CheckpointHook,
+ by_epoch=False,
+ interval=save_steps,
+ max_keep_ckpts=save_total_limit),
+ # set sampler seed in distributed evrionment.
+ sampler_seed=dict(type=DistSamplerSeedHook),
+)
+
+# configure environment
+env_cfg = dict(
+ # whether to enable cudnn benchmark
+ cudnn_benchmark=False,
+ # set multi process parameters
+ mp_cfg=dict(mp_start_method='fork', opencv_num_threads=0),
+ # set distributed parameters
+ dist_cfg=dict(backend='nccl'),
+)
+
+# set visualizer
+visualizer = None
+
+# set log level
+log_level = 'INFO'
+
+# load from which checkpoint
+load_from = None
+
+# whether to resume training from the loaded checkpoint
+resume = False
+
+# Defaults to use random seed and disable `deterministic`
+randomness = dict(seed=None, deterministic=False)
+
+# set log processor
+log_processor = dict(by_epoch=False)
diff --git a/xtuner/configs/custom_dataset/sft/deepseek/deepseek_moe_16b_chat_qlora_custom_sft_e1.py b/xtuner/configs/custom_dataset/sft/deepseek/deepseek_moe_16b_chat_qlora_custom_sft_e1.py
new file mode 100644
index 000000000..f7621bc6c
--- /dev/null
+++ b/xtuner/configs/custom_dataset/sft/deepseek/deepseek_moe_16b_chat_qlora_custom_sft_e1.py
@@ -0,0 +1,226 @@
+# Copyright (c) OpenMMLab. All rights reserved.
+"""Data format:
+
+[{
+ "messages": [
+ { "role": "system", "content": "xxx." },
+ { "role": "user", "content": "xxx." },
+ { "role": "assistant", "content": "xxx.", "loss": false},
+ { "role": "user", "content": "xxx." },
+ { "role": "assistant", "content": "xxx.", "loss": true}
+ ]
+},
+...
+]
+""" # noqa: E501
+import torch
+from datasets import load_dataset
+from mmengine.dataset import DefaultSampler
+from mmengine.hooks import (CheckpointHook, DistSamplerSeedHook, IterTimerHook,
+ LoggerHook, ParamSchedulerHook)
+from mmengine.optim import AmpOptimWrapper, CosineAnnealingLR, LinearLR
+from peft import LoraConfig
+from torch.optim import AdamW
+from transformers import (AutoModelForCausalLM, AutoTokenizer,
+ BitsAndBytesConfig)
+
+from xtuner.dataset import process_hf_dataset
+from xtuner.dataset.collate_fns import default_collate_fn
+from xtuner.dataset.map_fns import openai_map_fn, template_map_fn_factory
+from xtuner.engine.hooks import (DatasetInfoHook, EvaluateChatHook,
+ VarlenAttnArgsToMessageHubHook)
+from xtuner.engine.runner import TrainLoop
+from xtuner.model import SupervisedFinetune
+from xtuner.utils import PROMPT_TEMPLATE
+
+#######################################################################
+# PART 1 Settings #
+#######################################################################
+# Model
+pretrained_model_name_or_path = 'deepseek-ai/deepseek-moe-16b-chat'
+use_varlen_attn = False
+
+# Data
+data_files = ['/path/to/json/file.json']
+prompt_template = PROMPT_TEMPLATE.deepseek_moe
+max_length = 2048
+pack_to_max_length = True
+
+# Scheduler & Optimizer
+batch_size = 1 # per_device
+accumulative_counts = 16 # bs = 1 GPU * 1 batch_size_per_device * 16 acc
+dataloader_num_workers = 0
+max_epochs = 1
+optim_type = AdamW
+lr = 2e-4
+betas = (0.9, 0.999)
+weight_decay = 0
+max_norm = 1 # grad clip
+warmup_ratio = 0.03
+
+# Save
+save_steps = 500
+save_total_limit = 2 # Maximum checkpoints to keep (-1 means unlimited)
+
+# Evaluate the generation performance during the training
+evaluation_freq = 500
+SYSTEM = ''
+evaluation_inputs = [
+ '请给我介绍五个上海的景点', 'Please tell me five scenic spots in Shanghai'
+]
+
+#######################################################################
+# PART 2 Model & Tokenizer #
+#######################################################################
+tokenizer = dict(
+ type=AutoTokenizer.from_pretrained,
+ pretrained_model_name_or_path=pretrained_model_name_or_path,
+ trust_remote_code=True,
+ padding_side='right')
+
+model = dict(
+ type=SupervisedFinetune,
+ use_varlen_attn=use_varlen_attn,
+ llm=dict(
+ type=AutoModelForCausalLM.from_pretrained,
+ pretrained_model_name_or_path=pretrained_model_name_or_path,
+ trust_remote_code=True,
+ torch_dtype=torch.float16,
+ quantization_config=dict(
+ type=BitsAndBytesConfig,
+ load_in_4bit=True,
+ load_in_8bit=False,
+ llm_int8_threshold=6.0,
+ llm_int8_has_fp16_weight=False,
+ bnb_4bit_compute_dtype=torch.float16,
+ bnb_4bit_use_double_quant=True,
+ bnb_4bit_quant_type='nf4')),
+ lora=dict(
+ type=LoraConfig,
+ r=16,
+ lora_alpha=16,
+ lora_dropout=0.05,
+ bias='none',
+ task_type='CAUSAL_LM'))
+
+#######################################################################
+# PART 3 Dataset & Dataloader #
+#######################################################################
+train_dataset = dict(
+ type=process_hf_dataset,
+ dataset=dict(type=load_dataset, path='json', data_files=data_files),
+ tokenizer=tokenizer,
+ max_length=max_length,
+ dataset_map_fn=openai_map_fn,
+ template_map_fn=dict(
+ type=template_map_fn_factory, template=prompt_template),
+ remove_unused_columns=True,
+ shuffle_before_pack=True,
+ pack_to_max_length=pack_to_max_length,
+ use_varlen_attn=use_varlen_attn)
+
+train_dataloader = dict(
+ batch_size=batch_size,
+ num_workers=dataloader_num_workers,
+ dataset=train_dataset,
+ sampler=dict(type=DefaultSampler, shuffle=True),
+ collate_fn=dict(type=default_collate_fn, use_varlen_attn=use_varlen_attn))
+
+#######################################################################
+# PART 4 Scheduler & Optimizer #
+#######################################################################
+# optimizer
+optim_wrapper = dict(
+ type=AmpOptimWrapper,
+ optimizer=dict(
+ type=optim_type, lr=lr, betas=betas, weight_decay=weight_decay),
+ clip_grad=dict(max_norm=max_norm, error_if_nonfinite=False),
+ accumulative_counts=accumulative_counts,
+ loss_scale='dynamic',
+ dtype='float16')
+
+# learning policy
+# More information: https://github.com/open-mmlab/mmengine/blob/main/docs/en/tutorials/param_scheduler.md # noqa: E501
+param_scheduler = [
+ dict(
+ type=LinearLR,
+ start_factor=1e-5,
+ by_epoch=True,
+ begin=0,
+ end=warmup_ratio * max_epochs,
+ convert_to_iter_based=True),
+ dict(
+ type=CosineAnnealingLR,
+ eta_min=0.0,
+ by_epoch=True,
+ begin=warmup_ratio * max_epochs,
+ end=max_epochs,
+ convert_to_iter_based=True)
+]
+
+# train, val, test setting
+train_cfg = dict(type=TrainLoop, max_epochs=max_epochs)
+
+#######################################################################
+# PART 5 Runtime #
+#######################################################################
+# Log the dialogue periodically during the training process, optional
+custom_hooks = [
+ dict(type=DatasetInfoHook, tokenizer=tokenizer),
+ dict(
+ type=EvaluateChatHook,
+ tokenizer=tokenizer,
+ every_n_iters=evaluation_freq,
+ evaluation_inputs=evaluation_inputs,
+ system=SYSTEM,
+ prompt_template=prompt_template)
+]
+
+if use_varlen_attn:
+ custom_hooks += [dict(type=VarlenAttnArgsToMessageHubHook)]
+
+# configure default hooks
+default_hooks = dict(
+ # record the time of every iteration.
+ timer=dict(type=IterTimerHook),
+ # print log every 10 iterations.
+ logger=dict(type=LoggerHook, log_metric_by_epoch=False, interval=10),
+ # enable the parameter scheduler.
+ param_scheduler=dict(type=ParamSchedulerHook),
+ # save checkpoint per `save_steps`.
+ checkpoint=dict(
+ type=CheckpointHook,
+ by_epoch=False,
+ interval=save_steps,
+ max_keep_ckpts=save_total_limit),
+ # set sampler seed in distributed evrionment.
+ sampler_seed=dict(type=DistSamplerSeedHook),
+)
+
+# configure environment
+env_cfg = dict(
+ # whether to enable cudnn benchmark
+ cudnn_benchmark=False,
+ # set multi process parameters
+ mp_cfg=dict(mp_start_method='fork', opencv_num_threads=0),
+ # set distributed parameters
+ dist_cfg=dict(backend='nccl'),
+)
+
+# set visualizer
+visualizer = None
+
+# set log level
+log_level = 'INFO'
+
+# load from which checkpoint
+load_from = None
+
+# whether to resume training from the loaded checkpoint
+resume = False
+
+# Defaults to use random seed and disable `deterministic`
+randomness = dict(seed=None, deterministic=False)
+
+# set log processor
+log_processor = dict(by_epoch=False)
diff --git a/xtuner/configs/custom_dataset/sft/deepseek/deepseekcoder_6_7b_instruct_qlora_custom_sft_e1.py b/xtuner/configs/custom_dataset/sft/deepseek/deepseekcoder_6_7b_instruct_qlora_custom_sft_e1.py
new file mode 100644
index 000000000..629012f5b
--- /dev/null
+++ b/xtuner/configs/custom_dataset/sft/deepseek/deepseekcoder_6_7b_instruct_qlora_custom_sft_e1.py
@@ -0,0 +1,230 @@
+# Copyright (c) OpenMMLab. All rights reserved.
+"""Data format:
+
+[{
+ "messages": [
+ { "role": "system", "content": "xxx." },
+ { "role": "user", "content": "xxx." },
+ { "role": "assistant", "content": "xxx.", "loss": false},
+ { "role": "user", "content": "xxx." },
+ { "role": "assistant", "content": "xxx.", "loss": true}
+ ]
+},
+...
+]
+""" # noqa: E501
+import torch
+from datasets import load_dataset
+from mmengine.dataset import DefaultSampler
+from mmengine.hooks import (CheckpointHook, DistSamplerSeedHook, IterTimerHook,
+ LoggerHook, ParamSchedulerHook)
+from mmengine.optim import AmpOptimWrapper, CosineAnnealingLR, LinearLR
+from peft import LoraConfig
+from torch.optim import AdamW
+from transformers import (AutoModelForCausalLM, AutoTokenizer,
+ BitsAndBytesConfig)
+
+from xtuner.dataset import process_hf_dataset
+from xtuner.dataset.collate_fns import default_collate_fn
+from xtuner.dataset.map_fns import openai_map_fn, template_map_fn_factory
+from xtuner.engine.hooks import (DatasetInfoHook, EvaluateChatHook,
+ VarlenAttnArgsToMessageHubHook)
+from xtuner.engine.runner import TrainLoop
+from xtuner.model import SupervisedFinetune
+from xtuner.utils import PROMPT_TEMPLATE
+
+#######################################################################
+# PART 1 Settings #
+#######################################################################
+# Model
+pretrained_model_name_or_path = 'deepseek-ai/deepseek-coder-6.7b-instruct'
+use_varlen_attn = False
+
+# Data
+data_files = ['/path/to/json/file.json']
+prompt_template = PROMPT_TEMPLATE.deepseek_coder
+max_length = 2048
+pack_to_max_length = True
+
+# Scheduler & Optimizer
+batch_size = 1 # per_device
+accumulative_counts = 16 # bs = 1 GPU * 1 batch_size_per_device * 16 acc
+dataloader_num_workers = 0
+max_epochs = 1
+optim_type = AdamW
+lr = 2e-4
+betas = (0.9, 0.999)
+weight_decay = 0
+max_norm = 1 # grad clip
+warmup_ratio = 0.03
+
+# Save
+save_steps = 500
+save_total_limit = 2 # Maximum checkpoints to keep (-1 means unlimited)
+
+# Evaluate the generation performance during the training
+evaluation_freq = 100
+SYSTEM = ''
+evaluation_inputs = [
+ ('写一个Python函数,将十六进制颜色代码(如#0066ee)转换为对应的'
+ '红、绿、蓝(RGB)三个颜色分量值,并以元组的形式返回。'),
+ ('Write a Python function that takes a hexadecimal color code '
+ '(e.g., #0066ee) as input and converts it into the corresponding '
+ 'red, green, and blue (RGB) color component values.')
+]
+
+#######################################################################
+# PART 2 Model & Tokenizer #
+#######################################################################
+tokenizer = dict(
+ type=AutoTokenizer.from_pretrained,
+ pretrained_model_name_or_path=pretrained_model_name_or_path,
+ trust_remote_code=True,
+ padding_side='right')
+
+model = dict(
+ type=SupervisedFinetune,
+ use_varlen_attn=use_varlen_attn,
+ llm=dict(
+ type=AutoModelForCausalLM.from_pretrained,
+ pretrained_model_name_or_path=pretrained_model_name_or_path,
+ trust_remote_code=True,
+ torch_dtype=torch.float16,
+ quantization_config=dict(
+ type=BitsAndBytesConfig,
+ load_in_4bit=True,
+ load_in_8bit=False,
+ llm_int8_threshold=6.0,
+ llm_int8_has_fp16_weight=False,
+ bnb_4bit_compute_dtype=torch.float16,
+ bnb_4bit_use_double_quant=True,
+ bnb_4bit_quant_type='nf4')),
+ lora=dict(
+ type=LoraConfig,
+ r=64,
+ lora_alpha=16,
+ lora_dropout=0.1,
+ bias='none',
+ task_type='CAUSAL_LM'))
+
+#######################################################################
+# PART 3 Dataset & Dataloader #
+#######################################################################
+train_dataset = dict(
+ type=process_hf_dataset,
+ dataset=dict(type=load_dataset, path='json', data_files=data_files),
+ tokenizer=tokenizer,
+ max_length=max_length,
+ dataset_map_fn=openai_map_fn,
+ template_map_fn=dict(
+ type=template_map_fn_factory, template=prompt_template),
+ remove_unused_columns=True,
+ shuffle_before_pack=True,
+ pack_to_max_length=pack_to_max_length,
+ use_varlen_attn=use_varlen_attn)
+
+train_dataloader = dict(
+ batch_size=batch_size,
+ num_workers=dataloader_num_workers,
+ dataset=train_dataset,
+ sampler=dict(type=DefaultSampler, shuffle=True),
+ collate_fn=dict(type=default_collate_fn, use_varlen_attn=use_varlen_attn))
+
+#######################################################################
+# PART 4 Scheduler & Optimizer #
+#######################################################################
+# optimizer
+optim_wrapper = dict(
+ type=AmpOptimWrapper,
+ optimizer=dict(
+ type=optim_type, lr=lr, betas=betas, weight_decay=weight_decay),
+ clip_grad=dict(max_norm=max_norm, error_if_nonfinite=False),
+ accumulative_counts=accumulative_counts,
+ loss_scale='dynamic',
+ dtype='float16')
+
+# learning policy
+# More information: https://github.com/open-mmlab/mmengine/blob/main/docs/en/tutorials/param_scheduler.md # noqa: E501
+param_scheduler = [
+ dict(
+ type=LinearLR,
+ start_factor=1e-5,
+ by_epoch=True,
+ begin=0,
+ end=warmup_ratio * max_epochs,
+ convert_to_iter_based=True),
+ dict(
+ type=CosineAnnealingLR,
+ eta_min=0.0,
+ by_epoch=True,
+ begin=warmup_ratio * max_epochs,
+ end=max_epochs,
+ convert_to_iter_based=True)
+]
+
+# train, val, test setting
+train_cfg = dict(type=TrainLoop, max_epochs=max_epochs)
+
+#######################################################################
+# PART 5 Runtime #
+#######################################################################
+# Log the dialogue periodically during the training process, optional
+custom_hooks = [
+ dict(type=DatasetInfoHook, tokenizer=tokenizer),
+ dict(
+ type=EvaluateChatHook,
+ tokenizer=tokenizer,
+ every_n_iters=evaluation_freq,
+ evaluation_inputs=evaluation_inputs,
+ system=SYSTEM,
+ prompt_template=prompt_template)
+]
+
+if use_varlen_attn:
+ custom_hooks += [dict(type=VarlenAttnArgsToMessageHubHook)]
+
+# configure default hooks
+default_hooks = dict(
+ # record the time of every iteration.
+ timer=dict(type=IterTimerHook),
+ # print log every 10 iterations.
+ logger=dict(type=LoggerHook, log_metric_by_epoch=False, interval=10),
+ # enable the parameter scheduler.
+ param_scheduler=dict(type=ParamSchedulerHook),
+ # save checkpoint per `save_steps`.
+ checkpoint=dict(
+ type=CheckpointHook,
+ by_epoch=False,
+ interval=save_steps,
+ max_keep_ckpts=save_total_limit),
+ # set sampler seed in distributed evrionment.
+ sampler_seed=dict(type=DistSamplerSeedHook),
+)
+
+# configure environment
+env_cfg = dict(
+ # whether to enable cudnn benchmark
+ cudnn_benchmark=False,
+ # set multi process parameters
+ mp_cfg=dict(mp_start_method='fork', opencv_num_threads=0),
+ # set distributed parameters
+ dist_cfg=dict(backend='nccl'),
+)
+
+# set visualizer
+visualizer = None
+
+# set log level
+log_level = 'INFO'
+
+# load from which checkpoint
+load_from = None
+
+# whether to resume training from the loaded checkpoint
+resume = False
+
+# Defaults to use random seed and disable `deterministic`
+randomness = dict(seed=None, deterministic=False)
+
+# set log processor
+log_processor = dict(by_epoch=False)
diff --git a/xtuner/configs/custom_dataset/sft/gemma/gemma_2b_it_qlora_custom_sft_e1.py b/xtuner/configs/custom_dataset/sft/gemma/gemma_2b_it_qlora_custom_sft_e1.py
new file mode 100644
index 000000000..122ddf023
--- /dev/null
+++ b/xtuner/configs/custom_dataset/sft/gemma/gemma_2b_it_qlora_custom_sft_e1.py
@@ -0,0 +1,226 @@
+# Copyright (c) OpenMMLab. All rights reserved.
+"""Data format:
+
+[{
+ "messages": [
+ { "role": "system", "content": "xxx." },
+ { "role": "user", "content": "xxx." },
+ { "role": "assistant", "content": "xxx.", "loss": false},
+ { "role": "user", "content": "xxx." },
+ { "role": "assistant", "content": "xxx.", "loss": true}
+ ]
+},
+...
+]
+""" # noqa: E501
+import torch
+from datasets import load_dataset
+from mmengine.dataset import DefaultSampler
+from mmengine.hooks import (CheckpointHook, DistSamplerSeedHook, IterTimerHook,
+ LoggerHook, ParamSchedulerHook)
+from mmengine.optim import AmpOptimWrapper, CosineAnnealingLR, LinearLR
+from peft import LoraConfig
+from torch.optim import AdamW
+from transformers import (AutoModelForCausalLM, AutoTokenizer,
+ BitsAndBytesConfig)
+
+from xtuner.dataset import process_hf_dataset
+from xtuner.dataset.collate_fns import default_collate_fn
+from xtuner.dataset.map_fns import openai_map_fn, template_map_fn_factory
+from xtuner.engine.hooks import (DatasetInfoHook, EvaluateChatHook,
+ VarlenAttnArgsToMessageHubHook)
+from xtuner.engine.runner import TrainLoop
+from xtuner.model import SupervisedFinetune
+from xtuner.utils import PROMPT_TEMPLATE
+
+#######################################################################
+# PART 1 Settings #
+#######################################################################
+# Model
+pretrained_model_name_or_path = 'google/gemma-2b-it' # Gemma requires transformers>=4.38.1 # noqa: E501
+use_varlen_attn = False
+
+# Data
+data_files = ['/path/to/json/file.json']
+prompt_template = PROMPT_TEMPLATE.gemma
+max_length = 2048
+pack_to_max_length = True
+
+# Scheduler & Optimizer
+batch_size = 1 # per_device
+accumulative_counts = 16 # bs = 1 GPU * 1 batch_size_per_device * 16 acc
+dataloader_num_workers = 0
+max_epochs = 1
+optim_type = AdamW
+lr = 2e-4
+betas = (0.9, 0.999)
+weight_decay = 0
+max_norm = 1 # grad clip
+warmup_ratio = 0.03
+
+# Save
+save_steps = 500
+save_total_limit = 2 # Maximum checkpoints to keep (-1 means unlimited)
+
+# Evaluate the generation performance during the training
+evaluation_freq = 500
+SYSTEM = ''
+evaluation_inputs = [
+ '请给我介绍五个上海的景点', 'Please tell me five scenic spots in Shanghai'
+]
+
+#######################################################################
+# PART 2 Model & Tokenizer #
+#######################################################################
+tokenizer = dict(
+ type=AutoTokenizer.from_pretrained,
+ pretrained_model_name_or_path=pretrained_model_name_or_path,
+ trust_remote_code=True,
+ padding_side='right')
+
+model = dict(
+ type=SupervisedFinetune,
+ use_varlen_attn=use_varlen_attn,
+ llm=dict(
+ type=AutoModelForCausalLM.from_pretrained,
+ pretrained_model_name_or_path=pretrained_model_name_or_path,
+ trust_remote_code=True,
+ torch_dtype=torch.float16,
+ quantization_config=dict(
+ type=BitsAndBytesConfig,
+ load_in_4bit=True,
+ load_in_8bit=False,
+ llm_int8_threshold=6.0,
+ llm_int8_has_fp16_weight=False,
+ bnb_4bit_compute_dtype=torch.float16,
+ bnb_4bit_use_double_quant=True,
+ bnb_4bit_quant_type='nf4')),
+ lora=dict(
+ type=LoraConfig,
+ r=64,
+ lora_alpha=16,
+ lora_dropout=0.1,
+ bias='none',
+ task_type='CAUSAL_LM'))
+
+#######################################################################
+# PART 3 Dataset & Dataloader #
+#######################################################################
+train_dataset = dict(
+ type=process_hf_dataset,
+ dataset=dict(type=load_dataset, path='json', data_files=data_files),
+ tokenizer=tokenizer,
+ max_length=max_length,
+ dataset_map_fn=openai_map_fn,
+ template_map_fn=dict(
+ type=template_map_fn_factory, template=prompt_template),
+ remove_unused_columns=True,
+ shuffle_before_pack=True,
+ pack_to_max_length=pack_to_max_length,
+ use_varlen_attn=use_varlen_attn)
+
+train_dataloader = dict(
+ batch_size=batch_size,
+ num_workers=dataloader_num_workers,
+ dataset=train_dataset,
+ sampler=dict(type=DefaultSampler, shuffle=True),
+ collate_fn=dict(type=default_collate_fn, use_varlen_attn=use_varlen_attn))
+
+#######################################################################
+# PART 4 Scheduler & Optimizer #
+#######################################################################
+# optimizer
+optim_wrapper = dict(
+ type=AmpOptimWrapper,
+ optimizer=dict(
+ type=optim_type, lr=lr, betas=betas, weight_decay=weight_decay),
+ clip_grad=dict(max_norm=max_norm, error_if_nonfinite=False),
+ accumulative_counts=accumulative_counts,
+ loss_scale='dynamic',
+ dtype='float16')
+
+# learning policy
+# More information: https://github.com/open-mmlab/mmengine/blob/main/docs/en/tutorials/param_scheduler.md # noqa: E501
+param_scheduler = [
+ dict(
+ type=LinearLR,
+ start_factor=1e-5,
+ by_epoch=True,
+ begin=0,
+ end=warmup_ratio * max_epochs,
+ convert_to_iter_based=True),
+ dict(
+ type=CosineAnnealingLR,
+ eta_min=0.0,
+ by_epoch=True,
+ begin=warmup_ratio * max_epochs,
+ end=max_epochs,
+ convert_to_iter_based=True)
+]
+
+# train, val, test setting
+train_cfg = dict(type=TrainLoop, max_epochs=max_epochs)
+
+#######################################################################
+# PART 5 Runtime #
+#######################################################################
+# Log the dialogue periodically during the training process, optional
+custom_hooks = [
+ dict(type=DatasetInfoHook, tokenizer=tokenizer),
+ dict(
+ type=EvaluateChatHook,
+ tokenizer=tokenizer,
+ every_n_iters=evaluation_freq,
+ evaluation_inputs=evaluation_inputs,
+ system=SYSTEM,
+ prompt_template=prompt_template)
+]
+
+if use_varlen_attn:
+ custom_hooks += [dict(type=VarlenAttnArgsToMessageHubHook)]
+
+# configure default hooks
+default_hooks = dict(
+ # record the time of every iteration.
+ timer=dict(type=IterTimerHook),
+ # print log every 10 iterations.
+ logger=dict(type=LoggerHook, log_metric_by_epoch=False, interval=10),
+ # enable the parameter scheduler.
+ param_scheduler=dict(type=ParamSchedulerHook),
+ # save checkpoint per `save_steps`.
+ checkpoint=dict(
+ type=CheckpointHook,
+ by_epoch=False,
+ interval=save_steps,
+ max_keep_ckpts=save_total_limit),
+ # set sampler seed in distributed evrionment.
+ sampler_seed=dict(type=DistSamplerSeedHook),
+)
+
+# configure environment
+env_cfg = dict(
+ # whether to enable cudnn benchmark
+ cudnn_benchmark=False,
+ # set multi process parameters
+ mp_cfg=dict(mp_start_method='fork', opencv_num_threads=0),
+ # set distributed parameters
+ dist_cfg=dict(backend='nccl'),
+)
+
+# set visualizer
+visualizer = None
+
+# set log level
+log_level = 'INFO'
+
+# load from which checkpoint
+load_from = None
+
+# whether to resume training from the loaded checkpoint
+resume = False
+
+# Defaults to use random seed and disable `deterministic`
+randomness = dict(seed=None, deterministic=False)
+
+# set log processor
+log_processor = dict(by_epoch=False)
diff --git a/xtuner/configs/custom_dataset/sft/gemma/gemma_2b_qlora_custom_sft_e1.py b/xtuner/configs/custom_dataset/sft/gemma/gemma_2b_qlora_custom_sft_e1.py
new file mode 100644
index 000000000..9a3d36b30
--- /dev/null
+++ b/xtuner/configs/custom_dataset/sft/gemma/gemma_2b_qlora_custom_sft_e1.py
@@ -0,0 +1,226 @@
+# Copyright (c) OpenMMLab. All rights reserved.
+"""Data format:
+
+[{
+ "messages": [
+ { "role": "system", "content": "xxx." },
+ { "role": "user", "content": "xxx." },
+ { "role": "assistant", "content": "xxx.", "loss": false},
+ { "role": "user", "content": "xxx." },
+ { "role": "assistant", "content": "xxx.", "loss": true}
+ ]
+},
+...
+]
+""" # noqa: E501
+import torch
+from datasets import load_dataset
+from mmengine.dataset import DefaultSampler
+from mmengine.hooks import (CheckpointHook, DistSamplerSeedHook, IterTimerHook,
+ LoggerHook, ParamSchedulerHook)
+from mmengine.optim import AmpOptimWrapper, CosineAnnealingLR, LinearLR
+from peft import LoraConfig
+from torch.optim import AdamW
+from transformers import (AutoModelForCausalLM, AutoTokenizer,
+ BitsAndBytesConfig)
+
+from xtuner.dataset import process_hf_dataset
+from xtuner.dataset.collate_fns import default_collate_fn
+from xtuner.dataset.map_fns import openai_map_fn, template_map_fn_factory
+from xtuner.engine.hooks import (DatasetInfoHook, EvaluateChatHook,
+ VarlenAttnArgsToMessageHubHook)
+from xtuner.engine.runner import TrainLoop
+from xtuner.model import SupervisedFinetune
+from xtuner.utils import PROMPT_TEMPLATE
+
+#######################################################################
+# PART 1 Settings #
+#######################################################################
+# Model
+pretrained_model_name_or_path = 'google/gemma-2b' # Gemma requires transformers>=4.38.1 # noqa: E501
+use_varlen_attn = False
+
+# Data
+data_files = ['/path/to/json/file.json']
+prompt_template = PROMPT_TEMPLATE.default
+max_length = 2048
+pack_to_max_length = True
+
+# Scheduler & Optimizer
+batch_size = 1 # per_device
+accumulative_counts = 16 # bs = 1 GPU * 1 batch_size_per_device * 16 acc
+dataloader_num_workers = 0
+max_epochs = 1
+optim_type = AdamW
+lr = 2e-4
+betas = (0.9, 0.999)
+weight_decay = 0
+max_norm = 1 # grad clip
+warmup_ratio = 0.03
+
+# Save
+save_steps = 500
+save_total_limit = 2 # Maximum checkpoints to keep (-1 means unlimited)
+
+# Evaluate the generation performance during the training
+evaluation_freq = 500
+SYSTEM = ''
+evaluation_inputs = [
+ '请给我介绍五个上海的景点', 'Please tell me five scenic spots in Shanghai'
+]
+
+#######################################################################
+# PART 2 Model & Tokenizer #
+#######################################################################
+tokenizer = dict(
+ type=AutoTokenizer.from_pretrained,
+ pretrained_model_name_or_path=pretrained_model_name_or_path,
+ trust_remote_code=True,
+ padding_side='right')
+
+model = dict(
+ type=SupervisedFinetune,
+ use_varlen_attn=use_varlen_attn,
+ llm=dict(
+ type=AutoModelForCausalLM.from_pretrained,
+ pretrained_model_name_or_path=pretrained_model_name_or_path,
+ trust_remote_code=True,
+ torch_dtype=torch.float16,
+ quantization_config=dict(
+ type=BitsAndBytesConfig,
+ load_in_4bit=True,
+ load_in_8bit=False,
+ llm_int8_threshold=6.0,
+ llm_int8_has_fp16_weight=False,
+ bnb_4bit_compute_dtype=torch.float16,
+ bnb_4bit_use_double_quant=True,
+ bnb_4bit_quant_type='nf4')),
+ lora=dict(
+ type=LoraConfig,
+ r=64,
+ lora_alpha=16,
+ lora_dropout=0.1,
+ bias='none',
+ task_type='CAUSAL_LM'))
+
+#######################################################################
+# PART 3 Dataset & Dataloader #
+#######################################################################
+train_dataset = dict(
+ type=process_hf_dataset,
+ dataset=dict(type=load_dataset, path='json', data_files=data_files),
+ tokenizer=tokenizer,
+ max_length=max_length,
+ dataset_map_fn=openai_map_fn,
+ template_map_fn=dict(
+ type=template_map_fn_factory, template=prompt_template),
+ remove_unused_columns=True,
+ shuffle_before_pack=True,
+ pack_to_max_length=pack_to_max_length,
+ use_varlen_attn=use_varlen_attn)
+
+train_dataloader = dict(
+ batch_size=batch_size,
+ num_workers=dataloader_num_workers,
+ dataset=train_dataset,
+ sampler=dict(type=DefaultSampler, shuffle=True),
+ collate_fn=dict(type=default_collate_fn, use_varlen_attn=use_varlen_attn))
+
+#######################################################################
+# PART 4 Scheduler & Optimizer #
+#######################################################################
+# optimizer
+optim_wrapper = dict(
+ type=AmpOptimWrapper,
+ optimizer=dict(
+ type=optim_type, lr=lr, betas=betas, weight_decay=weight_decay),
+ clip_grad=dict(max_norm=max_norm, error_if_nonfinite=False),
+ accumulative_counts=accumulative_counts,
+ loss_scale='dynamic',
+ dtype='float16')
+
+# learning policy
+# More information: https://github.com/open-mmlab/mmengine/blob/main/docs/en/tutorials/param_scheduler.md # noqa: E501
+param_scheduler = [
+ dict(
+ type=LinearLR,
+ start_factor=1e-5,
+ by_epoch=True,
+ begin=0,
+ end=warmup_ratio * max_epochs,
+ convert_to_iter_based=True),
+ dict(
+ type=CosineAnnealingLR,
+ eta_min=0.0,
+ by_epoch=True,
+ begin=warmup_ratio * max_epochs,
+ end=max_epochs,
+ convert_to_iter_based=True)
+]
+
+# train, val, test setting
+train_cfg = dict(type=TrainLoop, max_epochs=max_epochs)
+
+#######################################################################
+# PART 5 Runtime #
+#######################################################################
+# Log the dialogue periodically during the training process, optional
+custom_hooks = [
+ dict(type=DatasetInfoHook, tokenizer=tokenizer),
+ dict(
+ type=EvaluateChatHook,
+ tokenizer=tokenizer,
+ every_n_iters=evaluation_freq,
+ evaluation_inputs=evaluation_inputs,
+ system=SYSTEM,
+ prompt_template=prompt_template)
+]
+
+if use_varlen_attn:
+ custom_hooks += [dict(type=VarlenAttnArgsToMessageHubHook)]
+
+# configure default hooks
+default_hooks = dict(
+ # record the time of every iteration.
+ timer=dict(type=IterTimerHook),
+ # print log every 10 iterations.
+ logger=dict(type=LoggerHook, log_metric_by_epoch=False, interval=10),
+ # enable the parameter scheduler.
+ param_scheduler=dict(type=ParamSchedulerHook),
+ # save checkpoint per `save_steps`.
+ checkpoint=dict(
+ type=CheckpointHook,
+ by_epoch=False,
+ interval=save_steps,
+ max_keep_ckpts=save_total_limit),
+ # set sampler seed in distributed evrionment.
+ sampler_seed=dict(type=DistSamplerSeedHook),
+)
+
+# configure environment
+env_cfg = dict(
+ # whether to enable cudnn benchmark
+ cudnn_benchmark=False,
+ # set multi process parameters
+ mp_cfg=dict(mp_start_method='fork', opencv_num_threads=0),
+ # set distributed parameters
+ dist_cfg=dict(backend='nccl'),
+)
+
+# set visualizer
+visualizer = None
+
+# set log level
+log_level = 'INFO'
+
+# load from which checkpoint
+load_from = None
+
+# whether to resume training from the loaded checkpoint
+resume = False
+
+# Defaults to use random seed and disable `deterministic`
+randomness = dict(seed=None, deterministic=False)
+
+# set log processor
+log_processor = dict(by_epoch=False)
diff --git a/xtuner/configs/custom_dataset/sft/gemma/gemma_7b_it_qlora_custom_sft_e1.py b/xtuner/configs/custom_dataset/sft/gemma/gemma_7b_it_qlora_custom_sft_e1.py
new file mode 100644
index 000000000..c677c9d09
--- /dev/null
+++ b/xtuner/configs/custom_dataset/sft/gemma/gemma_7b_it_qlora_custom_sft_e1.py
@@ -0,0 +1,226 @@
+# Copyright (c) OpenMMLab. All rights reserved.
+"""Data format:
+
+[{
+ "messages": [
+ { "role": "system", "content": "xxx." },
+ { "role": "user", "content": "xxx." },
+ { "role": "assistant", "content": "xxx.", "loss": false},
+ { "role": "user", "content": "xxx." },
+ { "role": "assistant", "content": "xxx.", "loss": true}
+ ]
+},
+...
+]
+""" # noqa: E501
+import torch
+from datasets import load_dataset
+from mmengine.dataset import DefaultSampler
+from mmengine.hooks import (CheckpointHook, DistSamplerSeedHook, IterTimerHook,
+ LoggerHook, ParamSchedulerHook)
+from mmengine.optim import AmpOptimWrapper, CosineAnnealingLR, LinearLR
+from peft import LoraConfig
+from torch.optim import AdamW
+from transformers import (AutoModelForCausalLM, AutoTokenizer,
+ BitsAndBytesConfig)
+
+from xtuner.dataset import process_hf_dataset
+from xtuner.dataset.collate_fns import default_collate_fn
+from xtuner.dataset.map_fns import openai_map_fn, template_map_fn_factory
+from xtuner.engine.hooks import (DatasetInfoHook, EvaluateChatHook,
+ VarlenAttnArgsToMessageHubHook)
+from xtuner.engine.runner import TrainLoop
+from xtuner.model import SupervisedFinetune
+from xtuner.utils import PROMPT_TEMPLATE
+
+#######################################################################
+# PART 1 Settings #
+#######################################################################
+# Model
+pretrained_model_name_or_path = 'google/gemma-7b-it' # Gemma requires transformers>=4.38.1 # noqa: E501
+use_varlen_attn = False
+
+# Data
+data_files = ['/path/to/json/file.json']
+prompt_template = PROMPT_TEMPLATE.gemma
+max_length = 2048
+pack_to_max_length = True
+
+# Scheduler & Optimizer
+batch_size = 1 # per_device
+accumulative_counts = 16 # bs = 1 GPU * 1 batch_size_per_device * 16 acc
+dataloader_num_workers = 0
+max_epochs = 1
+optim_type = AdamW
+lr = 2e-4
+betas = (0.9, 0.999)
+weight_decay = 0
+max_norm = 1 # grad clip
+warmup_ratio = 0.03
+
+# Save
+save_steps = 500
+save_total_limit = 2 # Maximum checkpoints to keep (-1 means unlimited)
+
+# Evaluate the generation performance during the training
+evaluation_freq = 500
+SYSTEM = ''
+evaluation_inputs = [
+ '请给我介绍五个上海的景点', 'Please tell me five scenic spots in Shanghai'
+]
+
+#######################################################################
+# PART 2 Model & Tokenizer #
+#######################################################################
+tokenizer = dict(
+ type=AutoTokenizer.from_pretrained,
+ pretrained_model_name_or_path=pretrained_model_name_or_path,
+ trust_remote_code=True,
+ padding_side='right')
+
+model = dict(
+ type=SupervisedFinetune,
+ use_varlen_attn=use_varlen_attn,
+ llm=dict(
+ type=AutoModelForCausalLM.from_pretrained,
+ pretrained_model_name_or_path=pretrained_model_name_or_path,
+ trust_remote_code=True,
+ torch_dtype=torch.float16,
+ quantization_config=dict(
+ type=BitsAndBytesConfig,
+ load_in_4bit=True,
+ load_in_8bit=False,
+ llm_int8_threshold=6.0,
+ llm_int8_has_fp16_weight=False,
+ bnb_4bit_compute_dtype=torch.float16,
+ bnb_4bit_use_double_quant=True,
+ bnb_4bit_quant_type='nf4')),
+ lora=dict(
+ type=LoraConfig,
+ r=64,
+ lora_alpha=16,
+ lora_dropout=0.1,
+ bias='none',
+ task_type='CAUSAL_LM'))
+
+#######################################################################
+# PART 3 Dataset & Dataloader #
+#######################################################################
+train_dataset = dict(
+ type=process_hf_dataset,
+ dataset=dict(type=load_dataset, path='json', data_files=data_files),
+ tokenizer=tokenizer,
+ max_length=max_length,
+ dataset_map_fn=openai_map_fn,
+ template_map_fn=dict(
+ type=template_map_fn_factory, template=prompt_template),
+ remove_unused_columns=True,
+ shuffle_before_pack=True,
+ pack_to_max_length=pack_to_max_length,
+ use_varlen_attn=use_varlen_attn)
+
+train_dataloader = dict(
+ batch_size=batch_size,
+ num_workers=dataloader_num_workers,
+ dataset=train_dataset,
+ sampler=dict(type=DefaultSampler, shuffle=True),
+ collate_fn=dict(type=default_collate_fn, use_varlen_attn=use_varlen_attn))
+
+#######################################################################
+# PART 4 Scheduler & Optimizer #
+#######################################################################
+# optimizer
+optim_wrapper = dict(
+ type=AmpOptimWrapper,
+ optimizer=dict(
+ type=optim_type, lr=lr, betas=betas, weight_decay=weight_decay),
+ clip_grad=dict(max_norm=max_norm, error_if_nonfinite=False),
+ accumulative_counts=accumulative_counts,
+ loss_scale='dynamic',
+ dtype='float16')
+
+# learning policy
+# More information: https://github.com/open-mmlab/mmengine/blob/main/docs/en/tutorials/param_scheduler.md # noqa: E501
+param_scheduler = [
+ dict(
+ type=LinearLR,
+ start_factor=1e-5,
+ by_epoch=True,
+ begin=0,
+ end=warmup_ratio * max_epochs,
+ convert_to_iter_based=True),
+ dict(
+ type=CosineAnnealingLR,
+ eta_min=0.0,
+ by_epoch=True,
+ begin=warmup_ratio * max_epochs,
+ end=max_epochs,
+ convert_to_iter_based=True)
+]
+
+# train, val, test setting
+train_cfg = dict(type=TrainLoop, max_epochs=max_epochs)
+
+#######################################################################
+# PART 5 Runtime #
+#######################################################################
+# Log the dialogue periodically during the training process, optional
+custom_hooks = [
+ dict(type=DatasetInfoHook, tokenizer=tokenizer),
+ dict(
+ type=EvaluateChatHook,
+ tokenizer=tokenizer,
+ every_n_iters=evaluation_freq,
+ evaluation_inputs=evaluation_inputs,
+ system=SYSTEM,
+ prompt_template=prompt_template)
+]
+
+if use_varlen_attn:
+ custom_hooks += [dict(type=VarlenAttnArgsToMessageHubHook)]
+
+# configure default hooks
+default_hooks = dict(
+ # record the time of every iteration.
+ timer=dict(type=IterTimerHook),
+ # print log every 10 iterations.
+ logger=dict(type=LoggerHook, log_metric_by_epoch=False, interval=10),
+ # enable the parameter scheduler.
+ param_scheduler=dict(type=ParamSchedulerHook),
+ # save checkpoint per `save_steps`.
+ checkpoint=dict(
+ type=CheckpointHook,
+ by_epoch=False,
+ interval=save_steps,
+ max_keep_ckpts=save_total_limit),
+ # set sampler seed in distributed evrionment.
+ sampler_seed=dict(type=DistSamplerSeedHook),
+)
+
+# configure environment
+env_cfg = dict(
+ # whether to enable cudnn benchmark
+ cudnn_benchmark=False,
+ # set multi process parameters
+ mp_cfg=dict(mp_start_method='fork', opencv_num_threads=0),
+ # set distributed parameters
+ dist_cfg=dict(backend='nccl'),
+)
+
+# set visualizer
+visualizer = None
+
+# set log level
+log_level = 'INFO'
+
+# load from which checkpoint
+load_from = None
+
+# whether to resume training from the loaded checkpoint
+resume = False
+
+# Defaults to use random seed and disable `deterministic`
+randomness = dict(seed=None, deterministic=False)
+
+# set log processor
+log_processor = dict(by_epoch=False)
diff --git a/xtuner/configs/custom_dataset/sft/gemma/gemma_7b_qlora_custom_sft_e1.py b/xtuner/configs/custom_dataset/sft/gemma/gemma_7b_qlora_custom_sft_e1.py
new file mode 100644
index 000000000..443a1e663
--- /dev/null
+++ b/xtuner/configs/custom_dataset/sft/gemma/gemma_7b_qlora_custom_sft_e1.py
@@ -0,0 +1,226 @@
+# Copyright (c) OpenMMLab. All rights reserved.
+"""Data format:
+
+[{
+ "messages": [
+ { "role": "system", "content": "xxx." },
+ { "role": "user", "content": "xxx." },
+ { "role": "assistant", "content": "xxx.", "loss": false},
+ { "role": "user", "content": "xxx." },
+ { "role": "assistant", "content": "xxx.", "loss": true}
+ ]
+},
+...
+]
+""" # noqa: E501
+import torch
+from datasets import load_dataset
+from mmengine.dataset import DefaultSampler
+from mmengine.hooks import (CheckpointHook, DistSamplerSeedHook, IterTimerHook,
+ LoggerHook, ParamSchedulerHook)
+from mmengine.optim import AmpOptimWrapper, CosineAnnealingLR, LinearLR
+from peft import LoraConfig
+from torch.optim import AdamW
+from transformers import (AutoModelForCausalLM, AutoTokenizer,
+ BitsAndBytesConfig)
+
+from xtuner.dataset import process_hf_dataset
+from xtuner.dataset.collate_fns import default_collate_fn
+from xtuner.dataset.map_fns import openai_map_fn, template_map_fn_factory
+from xtuner.engine.hooks import (DatasetInfoHook, EvaluateChatHook,
+ VarlenAttnArgsToMessageHubHook)
+from xtuner.engine.runner import TrainLoop
+from xtuner.model import SupervisedFinetune
+from xtuner.utils import PROMPT_TEMPLATE
+
+#######################################################################
+# PART 1 Settings #
+#######################################################################
+# Model
+pretrained_model_name_or_path = 'google/gemma-7b' # Gemma requires transformers>=4.38.1 # noqa: E501
+use_varlen_attn = False
+
+# Data
+data_files = ['/path/to/json/file.json']
+prompt_template = PROMPT_TEMPLATE.default
+max_length = 2048
+pack_to_max_length = True
+
+# Scheduler & Optimizer
+batch_size = 1 # per_device
+accumulative_counts = 16 # bs = 1 GPU * 1 batch_size_per_device * 16 acc
+dataloader_num_workers = 0
+max_epochs = 1
+optim_type = AdamW
+lr = 2e-4
+betas = (0.9, 0.999)
+weight_decay = 0
+max_norm = 1 # grad clip
+warmup_ratio = 0.03
+
+# Save
+save_steps = 500
+save_total_limit = 2 # Maximum checkpoints to keep (-1 means unlimited)
+
+# Evaluate the generation performance during the training
+evaluation_freq = 500
+SYSTEM = ''
+evaluation_inputs = [
+ '请给我介绍五个上海的景点', 'Please tell me five scenic spots in Shanghai'
+]
+
+#######################################################################
+# PART 2 Model & Tokenizer #
+#######################################################################
+tokenizer = dict(
+ type=AutoTokenizer.from_pretrained,
+ pretrained_model_name_or_path=pretrained_model_name_or_path,
+ trust_remote_code=True,
+ padding_side='right')
+
+model = dict(
+ type=SupervisedFinetune,
+ use_varlen_attn=use_varlen_attn,
+ llm=dict(
+ type=AutoModelForCausalLM.from_pretrained,
+ pretrained_model_name_or_path=pretrained_model_name_or_path,
+ trust_remote_code=True,
+ torch_dtype=torch.float16,
+ quantization_config=dict(
+ type=BitsAndBytesConfig,
+ load_in_4bit=True,
+ load_in_8bit=False,
+ llm_int8_threshold=6.0,
+ llm_int8_has_fp16_weight=False,
+ bnb_4bit_compute_dtype=torch.float16,
+ bnb_4bit_use_double_quant=True,
+ bnb_4bit_quant_type='nf4')),
+ lora=dict(
+ type=LoraConfig,
+ r=64,
+ lora_alpha=16,
+ lora_dropout=0.1,
+ bias='none',
+ task_type='CAUSAL_LM'))
+
+#######################################################################
+# PART 3 Dataset & Dataloader #
+#######################################################################
+train_dataset = dict(
+ type=process_hf_dataset,
+ dataset=dict(type=load_dataset, path='json', data_files=data_files),
+ tokenizer=tokenizer,
+ max_length=max_length,
+ dataset_map_fn=openai_map_fn,
+ template_map_fn=dict(
+ type=template_map_fn_factory, template=prompt_template),
+ remove_unused_columns=True,
+ shuffle_before_pack=True,
+ pack_to_max_length=pack_to_max_length,
+ use_varlen_attn=use_varlen_attn)
+
+train_dataloader = dict(
+ batch_size=batch_size,
+ num_workers=dataloader_num_workers,
+ dataset=train_dataset,
+ sampler=dict(type=DefaultSampler, shuffle=True),
+ collate_fn=dict(type=default_collate_fn, use_varlen_attn=use_varlen_attn))
+
+#######################################################################
+# PART 4 Scheduler & Optimizer #
+#######################################################################
+# optimizer
+optim_wrapper = dict(
+ type=AmpOptimWrapper,
+ optimizer=dict(
+ type=optim_type, lr=lr, betas=betas, weight_decay=weight_decay),
+ clip_grad=dict(max_norm=max_norm, error_if_nonfinite=False),
+ accumulative_counts=accumulative_counts,
+ loss_scale='dynamic',
+ dtype='float16')
+
+# learning policy
+# More information: https://github.com/open-mmlab/mmengine/blob/main/docs/en/tutorials/param_scheduler.md # noqa: E501
+param_scheduler = [
+ dict(
+ type=LinearLR,
+ start_factor=1e-5,
+ by_epoch=True,
+ begin=0,
+ end=warmup_ratio * max_epochs,
+ convert_to_iter_based=True),
+ dict(
+ type=CosineAnnealingLR,
+ eta_min=0.0,
+ by_epoch=True,
+ begin=warmup_ratio * max_epochs,
+ end=max_epochs,
+ convert_to_iter_based=True)
+]
+
+# train, val, test setting
+train_cfg = dict(type=TrainLoop, max_epochs=max_epochs)
+
+#######################################################################
+# PART 5 Runtime #
+#######################################################################
+# Log the dialogue periodically during the training process, optional
+custom_hooks = [
+ dict(type=DatasetInfoHook, tokenizer=tokenizer),
+ dict(
+ type=EvaluateChatHook,
+ tokenizer=tokenizer,
+ every_n_iters=evaluation_freq,
+ evaluation_inputs=evaluation_inputs,
+ system=SYSTEM,
+ prompt_template=prompt_template)
+]
+
+if use_varlen_attn:
+ custom_hooks += [dict(type=VarlenAttnArgsToMessageHubHook)]
+
+# configure default hooks
+default_hooks = dict(
+ # record the time of every iteration.
+ timer=dict(type=IterTimerHook),
+ # print log every 10 iterations.
+ logger=dict(type=LoggerHook, log_metric_by_epoch=False, interval=10),
+ # enable the parameter scheduler.
+ param_scheduler=dict(type=ParamSchedulerHook),
+ # save checkpoint per `save_steps`.
+ checkpoint=dict(
+ type=CheckpointHook,
+ by_epoch=False,
+ interval=save_steps,
+ max_keep_ckpts=save_total_limit),
+ # set sampler seed in distributed evrionment.
+ sampler_seed=dict(type=DistSamplerSeedHook),
+)
+
+# configure environment
+env_cfg = dict(
+ # whether to enable cudnn benchmark
+ cudnn_benchmark=False,
+ # set multi process parameters
+ mp_cfg=dict(mp_start_method='fork', opencv_num_threads=0),
+ # set distributed parameters
+ dist_cfg=dict(backend='nccl'),
+)
+
+# set visualizer
+visualizer = None
+
+# set log level
+log_level = 'INFO'
+
+# load from which checkpoint
+load_from = None
+
+# whether to resume training from the loaded checkpoint
+resume = False
+
+# Defaults to use random seed and disable `deterministic`
+randomness = dict(seed=None, deterministic=False)
+
+# set log processor
+log_processor = dict(by_epoch=False)
diff --git a/xtuner/configs/custom_dataset/sft/internlm/internlm2_chat_1_8b_qlora_custom_sft_e1.py b/xtuner/configs/custom_dataset/sft/internlm/internlm2_chat_1_8b_qlora_custom_sft_e1.py
new file mode 100644
index 000000000..2aaa6f24d
--- /dev/null
+++ b/xtuner/configs/custom_dataset/sft/internlm/internlm2_chat_1_8b_qlora_custom_sft_e1.py
@@ -0,0 +1,226 @@
+# Copyright (c) OpenMMLab. All rights reserved.
+"""Data format:
+
+[{
+ "messages": [
+ { "role": "system", "content": "xxx." },
+ { "role": "user", "content": "xxx." },
+ { "role": "assistant", "content": "xxx.", "loss": false},
+ { "role": "user", "content": "xxx." },
+ { "role": "assistant", "content": "xxx.", "loss": true}
+ ]
+},
+...
+]
+""" # noqa: E501
+import torch
+from datasets import load_dataset
+from mmengine.dataset import DefaultSampler
+from mmengine.hooks import (CheckpointHook, DistSamplerSeedHook, IterTimerHook,
+ LoggerHook, ParamSchedulerHook)
+from mmengine.optim import AmpOptimWrapper, CosineAnnealingLR, LinearLR
+from peft import LoraConfig
+from torch.optim import AdamW
+from transformers import (AutoModelForCausalLM, AutoTokenizer,
+ BitsAndBytesConfig)
+
+from xtuner.dataset import process_hf_dataset
+from xtuner.dataset.collate_fns import default_collate_fn
+from xtuner.dataset.map_fns import openai_map_fn, template_map_fn_factory
+from xtuner.engine.hooks import (DatasetInfoHook, EvaluateChatHook,
+ VarlenAttnArgsToMessageHubHook)
+from xtuner.engine.runner import TrainLoop
+from xtuner.model import SupervisedFinetune
+from xtuner.utils import PROMPT_TEMPLATE
+
+#######################################################################
+# PART 1 Settings #
+#######################################################################
+# Model
+pretrained_model_name_or_path = 'internlm/internlm2-chat-1_8b'
+use_varlen_attn = False
+
+# Data
+data_files = ['/path/to/json/file.json']
+prompt_template = PROMPT_TEMPLATE.internlm2_chat
+max_length = 2048
+pack_to_max_length = True
+
+# Scheduler & Optimizer
+batch_size = 1 # per_device
+accumulative_counts = 16 # bs = 1 GPU * 1 batch_size_per_device * 16 acc
+dataloader_num_workers = 0
+max_epochs = 1
+optim_type = AdamW
+lr = 2e-4
+betas = (0.9, 0.999)
+weight_decay = 0
+max_norm = 1 # grad clip
+warmup_ratio = 0.03
+
+# Save
+save_steps = 500
+save_total_limit = 2 # Maximum checkpoints to keep (-1 means unlimited)
+
+# Evaluate the generation performance during the training
+evaluation_freq = 500
+SYSTEM = ''
+evaluation_inputs = [
+ '请给我介绍五个上海的景点', 'Please tell me five scenic spots in Shanghai'
+]
+
+#######################################################################
+# PART 2 Model & Tokenizer #
+#######################################################################
+tokenizer = dict(
+ type=AutoTokenizer.from_pretrained,
+ pretrained_model_name_or_path=pretrained_model_name_or_path,
+ trust_remote_code=True,
+ padding_side='right')
+
+model = dict(
+ type=SupervisedFinetune,
+ use_varlen_attn=use_varlen_attn,
+ llm=dict(
+ type=AutoModelForCausalLM.from_pretrained,
+ pretrained_model_name_or_path=pretrained_model_name_or_path,
+ trust_remote_code=True,
+ torch_dtype=torch.float16,
+ quantization_config=dict(
+ type=BitsAndBytesConfig,
+ load_in_4bit=True,
+ load_in_8bit=False,
+ llm_int8_threshold=6.0,
+ llm_int8_has_fp16_weight=False,
+ bnb_4bit_compute_dtype=torch.float16,
+ bnb_4bit_use_double_quant=True,
+ bnb_4bit_quant_type='nf4')),
+ lora=dict(
+ type=LoraConfig,
+ r=64,
+ lora_alpha=16,
+ lora_dropout=0.1,
+ bias='none',
+ task_type='CAUSAL_LM'))
+
+#######################################################################
+# PART 3 Dataset & Dataloader #
+#######################################################################
+train_dataset = dict(
+ type=process_hf_dataset,
+ dataset=dict(type=load_dataset, path='json', data_files=data_files),
+ tokenizer=tokenizer,
+ max_length=max_length,
+ dataset_map_fn=openai_map_fn,
+ template_map_fn=dict(
+ type=template_map_fn_factory, template=prompt_template),
+ remove_unused_columns=True,
+ shuffle_before_pack=True,
+ pack_to_max_length=pack_to_max_length,
+ use_varlen_attn=use_varlen_attn)
+
+train_dataloader = dict(
+ batch_size=batch_size,
+ num_workers=dataloader_num_workers,
+ dataset=train_dataset,
+ sampler=dict(type=DefaultSampler, shuffle=True),
+ collate_fn=dict(type=default_collate_fn, use_varlen_attn=use_varlen_attn))
+
+#######################################################################
+# PART 4 Scheduler & Optimizer #
+#######################################################################
+# optimizer
+optim_wrapper = dict(
+ type=AmpOptimWrapper,
+ optimizer=dict(
+ type=optim_type, lr=lr, betas=betas, weight_decay=weight_decay),
+ clip_grad=dict(max_norm=max_norm, error_if_nonfinite=False),
+ accumulative_counts=accumulative_counts,
+ loss_scale='dynamic',
+ dtype='float16')
+
+# learning policy
+# More information: https://github.com/open-mmlab/mmengine/blob/main/docs/en/tutorials/param_scheduler.md # noqa: E501
+param_scheduler = [
+ dict(
+ type=LinearLR,
+ start_factor=1e-5,
+ by_epoch=True,
+ begin=0,
+ end=warmup_ratio * max_epochs,
+ convert_to_iter_based=True),
+ dict(
+ type=CosineAnnealingLR,
+ eta_min=0.0,
+ by_epoch=True,
+ begin=warmup_ratio * max_epochs,
+ end=max_epochs,
+ convert_to_iter_based=True)
+]
+
+# train, val, test setting
+train_cfg = dict(type=TrainLoop, max_epochs=max_epochs)
+
+#######################################################################
+# PART 5 Runtime #
+#######################################################################
+# Log the dialogue periodically during the training process, optional
+custom_hooks = [
+ dict(type=DatasetInfoHook, tokenizer=tokenizer),
+ dict(
+ type=EvaluateChatHook,
+ tokenizer=tokenizer,
+ every_n_iters=evaluation_freq,
+ evaluation_inputs=evaluation_inputs,
+ system=SYSTEM,
+ prompt_template=prompt_template)
+]
+
+if use_varlen_attn:
+ custom_hooks += [dict(type=VarlenAttnArgsToMessageHubHook)]
+
+# configure default hooks
+default_hooks = dict(
+ # record the time of every iteration.
+ timer=dict(type=IterTimerHook),
+ # print log every 10 iterations.
+ logger=dict(type=LoggerHook, log_metric_by_epoch=False, interval=10),
+ # enable the parameter scheduler.
+ param_scheduler=dict(type=ParamSchedulerHook),
+ # save checkpoint per `save_steps`.
+ checkpoint=dict(
+ type=CheckpointHook,
+ by_epoch=False,
+ interval=save_steps,
+ max_keep_ckpts=save_total_limit),
+ # set sampler seed in distributed evrionment.
+ sampler_seed=dict(type=DistSamplerSeedHook),
+)
+
+# configure environment
+env_cfg = dict(
+ # whether to enable cudnn benchmark
+ cudnn_benchmark=False,
+ # set multi process parameters
+ mp_cfg=dict(mp_start_method='fork', opencv_num_threads=0),
+ # set distributed parameters
+ dist_cfg=dict(backend='nccl'),
+)
+
+# set visualizer
+visualizer = None
+
+# set log level
+log_level = 'INFO'
+
+# load from which checkpoint
+load_from = None
+
+# whether to resume training from the loaded checkpoint
+resume = False
+
+# Defaults to use random seed and disable `deterministic`
+randomness = dict(seed=None, deterministic=False)
+
+# set log processor
+log_processor = dict(by_epoch=False)
diff --git a/xtuner/configs/custom_dataset/sft/internlm/internlm2_chat_20b_qlora_custom_sft_e1.py b/xtuner/configs/custom_dataset/sft/internlm/internlm2_chat_20b_qlora_custom_sft_e1.py
new file mode 100644
index 000000000..dfb423839
--- /dev/null
+++ b/xtuner/configs/custom_dataset/sft/internlm/internlm2_chat_20b_qlora_custom_sft_e1.py
@@ -0,0 +1,226 @@
+# Copyright (c) OpenMMLab. All rights reserved.
+"""Data format:
+
+[{
+ "messages": [
+ { "role": "system", "content": "xxx." },
+ { "role": "user", "content": "xxx." },
+ { "role": "assistant", "content": "xxx.", "loss": false},
+ { "role": "user", "content": "xxx." },
+ { "role": "assistant", "content": "xxx.", "loss": true}
+ ]
+},
+...
+]
+""" # noqa: E501
+import torch
+from datasets import load_dataset
+from mmengine.dataset import DefaultSampler
+from mmengine.hooks import (CheckpointHook, DistSamplerSeedHook, IterTimerHook,
+ LoggerHook, ParamSchedulerHook)
+from mmengine.optim import AmpOptimWrapper, CosineAnnealingLR, LinearLR
+from peft import LoraConfig
+from torch.optim import AdamW
+from transformers import (AutoModelForCausalLM, AutoTokenizer,
+ BitsAndBytesConfig)
+
+from xtuner.dataset import process_hf_dataset
+from xtuner.dataset.collate_fns import default_collate_fn
+from xtuner.dataset.map_fns import openai_map_fn, template_map_fn_factory
+from xtuner.engine.hooks import (DatasetInfoHook, EvaluateChatHook,
+ VarlenAttnArgsToMessageHubHook)
+from xtuner.engine.runner import TrainLoop
+from xtuner.model import SupervisedFinetune
+from xtuner.utils import PROMPT_TEMPLATE
+
+#######################################################################
+# PART 1 Settings #
+#######################################################################
+# Model
+pretrained_model_name_or_path = 'internlm/internlm2-chat-20b'
+use_varlen_attn = False
+
+# Data
+data_files = ['/path/to/json/file.json']
+prompt_template = PROMPT_TEMPLATE.internlm2_chat
+max_length = 2048
+pack_to_max_length = True
+
+# Scheduler & Optimizer
+batch_size = 1 # per_device
+accumulative_counts = 16 # bs = 1 GPU * 1 batch_size_per_device * 16 acc
+dataloader_num_workers = 0
+max_epochs = 1
+optim_type = AdamW
+lr = 2e-4
+betas = (0.9, 0.999)
+weight_decay = 0
+max_norm = 1 # grad clip
+warmup_ratio = 0.03
+
+# Save
+save_steps = 500
+save_total_limit = 2 # Maximum checkpoints to keep (-1 means unlimited)
+
+# Evaluate the generation performance during the training
+evaluation_freq = 500
+SYSTEM = ''
+evaluation_inputs = [
+ '请给我介绍五个上海的景点', 'Please tell me five scenic spots in Shanghai'
+]
+
+#######################################################################
+# PART 2 Model & Tokenizer #
+#######################################################################
+tokenizer = dict(
+ type=AutoTokenizer.from_pretrained,
+ pretrained_model_name_or_path=pretrained_model_name_or_path,
+ trust_remote_code=True,
+ padding_side='right')
+
+model = dict(
+ type=SupervisedFinetune,
+ use_varlen_attn=use_varlen_attn,
+ llm=dict(
+ type=AutoModelForCausalLM.from_pretrained,
+ pretrained_model_name_or_path=pretrained_model_name_or_path,
+ trust_remote_code=True,
+ torch_dtype=torch.float16,
+ quantization_config=dict(
+ type=BitsAndBytesConfig,
+ load_in_4bit=True,
+ load_in_8bit=False,
+ llm_int8_threshold=6.0,
+ llm_int8_has_fp16_weight=False,
+ bnb_4bit_compute_dtype=torch.float16,
+ bnb_4bit_use_double_quant=True,
+ bnb_4bit_quant_type='nf4')),
+ lora=dict(
+ type=LoraConfig,
+ r=64,
+ lora_alpha=16,
+ lora_dropout=0.1,
+ bias='none',
+ task_type='CAUSAL_LM'))
+
+#######################################################################
+# PART 3 Dataset & Dataloader #
+#######################################################################
+train_dataset = dict(
+ type=process_hf_dataset,
+ dataset=dict(type=load_dataset, path='json', data_files=data_files),
+ tokenizer=tokenizer,
+ max_length=max_length,
+ dataset_map_fn=openai_map_fn,
+ template_map_fn=dict(
+ type=template_map_fn_factory, template=prompt_template),
+ remove_unused_columns=True,
+ shuffle_before_pack=True,
+ pack_to_max_length=pack_to_max_length,
+ use_varlen_attn=use_varlen_attn)
+
+train_dataloader = dict(
+ batch_size=batch_size,
+ num_workers=dataloader_num_workers,
+ dataset=train_dataset,
+ sampler=dict(type=DefaultSampler, shuffle=True),
+ collate_fn=dict(type=default_collate_fn, use_varlen_attn=use_varlen_attn))
+
+#######################################################################
+# PART 4 Scheduler & Optimizer #
+#######################################################################
+# optimizer
+optim_wrapper = dict(
+ type=AmpOptimWrapper,
+ optimizer=dict(
+ type=optim_type, lr=lr, betas=betas, weight_decay=weight_decay),
+ clip_grad=dict(max_norm=max_norm, error_if_nonfinite=False),
+ accumulative_counts=accumulative_counts,
+ loss_scale='dynamic',
+ dtype='float16')
+
+# learning policy
+# More information: https://github.com/open-mmlab/mmengine/blob/main/docs/en/tutorials/param_scheduler.md # noqa: E501
+param_scheduler = [
+ dict(
+ type=LinearLR,
+ start_factor=1e-5,
+ by_epoch=True,
+ begin=0,
+ end=warmup_ratio * max_epochs,
+ convert_to_iter_based=True),
+ dict(
+ type=CosineAnnealingLR,
+ eta_min=0.0,
+ by_epoch=True,
+ begin=warmup_ratio * max_epochs,
+ end=max_epochs,
+ convert_to_iter_based=True)
+]
+
+# train, val, test setting
+train_cfg = dict(type=TrainLoop, max_epochs=max_epochs)
+
+#######################################################################
+# PART 5 Runtime #
+#######################################################################
+# Log the dialogue periodically during the training process, optional
+custom_hooks = [
+ dict(type=DatasetInfoHook, tokenizer=tokenizer),
+ dict(
+ type=EvaluateChatHook,
+ tokenizer=tokenizer,
+ every_n_iters=evaluation_freq,
+ evaluation_inputs=evaluation_inputs,
+ system=SYSTEM,
+ prompt_template=prompt_template)
+]
+
+if use_varlen_attn:
+ custom_hooks += [dict(type=VarlenAttnArgsToMessageHubHook)]
+
+# configure default hooks
+default_hooks = dict(
+ # record the time of every iteration.
+ timer=dict(type=IterTimerHook),
+ # print log every 10 iterations.
+ logger=dict(type=LoggerHook, log_metric_by_epoch=False, interval=10),
+ # enable the parameter scheduler.
+ param_scheduler=dict(type=ParamSchedulerHook),
+ # save checkpoint per `save_steps`.
+ checkpoint=dict(
+ type=CheckpointHook,
+ by_epoch=False,
+ interval=save_steps,
+ max_keep_ckpts=save_total_limit),
+ # set sampler seed in distributed evrionment.
+ sampler_seed=dict(type=DistSamplerSeedHook),
+)
+
+# configure environment
+env_cfg = dict(
+ # whether to enable cudnn benchmark
+ cudnn_benchmark=False,
+ # set multi process parameters
+ mp_cfg=dict(mp_start_method='fork', opencv_num_threads=0),
+ # set distributed parameters
+ dist_cfg=dict(backend='nccl'),
+)
+
+# set visualizer
+visualizer = None
+
+# set log level
+log_level = 'INFO'
+
+# load from which checkpoint
+load_from = None
+
+# whether to resume training from the loaded checkpoint
+resume = False
+
+# Defaults to use random seed and disable `deterministic`
+randomness = dict(seed=None, deterministic=False)
+
+# set log processor
+log_processor = dict(by_epoch=False)
diff --git a/xtuner/configs/custom_dataset/sft/internlm/internlm2_chat_7b_qlora_custom_sft_e1.py b/xtuner/configs/custom_dataset/sft/internlm/internlm2_chat_7b_qlora_custom_sft_e1.py
new file mode 100644
index 000000000..313103992
--- /dev/null
+++ b/xtuner/configs/custom_dataset/sft/internlm/internlm2_chat_7b_qlora_custom_sft_e1.py
@@ -0,0 +1,226 @@
+# Copyright (c) OpenMMLab. All rights reserved.
+"""Data format:
+
+[{
+ "messages": [
+ { "role": "system", "content": "xxx." },
+ { "role": "user", "content": "xxx." },
+ { "role": "assistant", "content": "xxx.", "loss": false},
+ { "role": "user", "content": "xxx." },
+ { "role": "assistant", "content": "xxx.", "loss": true}
+ ]
+},
+...
+]
+""" # noqa: E501
+import torch
+from datasets import load_dataset
+from mmengine.dataset import DefaultSampler
+from mmengine.hooks import (CheckpointHook, DistSamplerSeedHook, IterTimerHook,
+ LoggerHook, ParamSchedulerHook)
+from mmengine.optim import AmpOptimWrapper, CosineAnnealingLR, LinearLR
+from peft import LoraConfig
+from torch.optim import AdamW
+from transformers import (AutoModelForCausalLM, AutoTokenizer,
+ BitsAndBytesConfig)
+
+from xtuner.dataset import process_hf_dataset
+from xtuner.dataset.collate_fns import default_collate_fn
+from xtuner.dataset.map_fns import openai_map_fn, template_map_fn_factory
+from xtuner.engine.hooks import (DatasetInfoHook, EvaluateChatHook,
+ VarlenAttnArgsToMessageHubHook)
+from xtuner.engine.runner import TrainLoop
+from xtuner.model import SupervisedFinetune
+from xtuner.utils import PROMPT_TEMPLATE
+
+#######################################################################
+# PART 1 Settings #
+#######################################################################
+# Model
+pretrained_model_name_or_path = 'internlm/internlm2-chat-7b'
+use_varlen_attn = False
+
+# Data
+data_files = ['/path/to/json/file.json']
+prompt_template = PROMPT_TEMPLATE.internlm2_chat
+max_length = 2048
+pack_to_max_length = True
+
+# Scheduler & Optimizer
+batch_size = 1 # per_device
+accumulative_counts = 16 # bs = 1 GPU * 1 batch_size_per_device * 16 acc
+dataloader_num_workers = 0
+max_epochs = 1
+optim_type = AdamW
+lr = 2e-4
+betas = (0.9, 0.999)
+weight_decay = 0
+max_norm = 1 # grad clip
+warmup_ratio = 0.03
+
+# Save
+save_steps = 500
+save_total_limit = 2 # Maximum checkpoints to keep (-1 means unlimited)
+
+# Evaluate the generation performance during the training
+evaluation_freq = 500
+SYSTEM = ''
+evaluation_inputs = [
+ '请给我介绍五个上海的景点', 'Please tell me five scenic spots in Shanghai'
+]
+
+#######################################################################
+# PART 2 Model & Tokenizer #
+#######################################################################
+tokenizer = dict(
+ type=AutoTokenizer.from_pretrained,
+ pretrained_model_name_or_path=pretrained_model_name_or_path,
+ trust_remote_code=True,
+ padding_side='right')
+
+model = dict(
+ type=SupervisedFinetune,
+ use_varlen_attn=use_varlen_attn,
+ llm=dict(
+ type=AutoModelForCausalLM.from_pretrained,
+ pretrained_model_name_or_path=pretrained_model_name_or_path,
+ trust_remote_code=True,
+ torch_dtype=torch.float16,
+ quantization_config=dict(
+ type=BitsAndBytesConfig,
+ load_in_4bit=True,
+ load_in_8bit=False,
+ llm_int8_threshold=6.0,
+ llm_int8_has_fp16_weight=False,
+ bnb_4bit_compute_dtype=torch.float16,
+ bnb_4bit_use_double_quant=True,
+ bnb_4bit_quant_type='nf4')),
+ lora=dict(
+ type=LoraConfig,
+ r=64,
+ lora_alpha=16,
+ lora_dropout=0.1,
+ bias='none',
+ task_type='CAUSAL_LM'))
+
+#######################################################################
+# PART 3 Dataset & Dataloader #
+#######################################################################
+train_dataset = dict(
+ type=process_hf_dataset,
+ dataset=dict(type=load_dataset, path='json', data_files=data_files),
+ tokenizer=tokenizer,
+ max_length=max_length,
+ dataset_map_fn=openai_map_fn,
+ template_map_fn=dict(
+ type=template_map_fn_factory, template=prompt_template),
+ remove_unused_columns=True,
+ shuffle_before_pack=True,
+ pack_to_max_length=pack_to_max_length,
+ use_varlen_attn=use_varlen_attn)
+
+train_dataloader = dict(
+ batch_size=batch_size,
+ num_workers=dataloader_num_workers,
+ dataset=train_dataset,
+ sampler=dict(type=DefaultSampler, shuffle=True),
+ collate_fn=dict(type=default_collate_fn, use_varlen_attn=use_varlen_attn))
+
+#######################################################################
+# PART 4 Scheduler & Optimizer #
+#######################################################################
+# optimizer
+optim_wrapper = dict(
+ type=AmpOptimWrapper,
+ optimizer=dict(
+ type=optim_type, lr=lr, betas=betas, weight_decay=weight_decay),
+ clip_grad=dict(max_norm=max_norm, error_if_nonfinite=False),
+ accumulative_counts=accumulative_counts,
+ loss_scale='dynamic',
+ dtype='float16')
+
+# learning policy
+# More information: https://github.com/open-mmlab/mmengine/blob/main/docs/en/tutorials/param_scheduler.md # noqa: E501
+param_scheduler = [
+ dict(
+ type=LinearLR,
+ start_factor=1e-5,
+ by_epoch=True,
+ begin=0,
+ end=warmup_ratio * max_epochs,
+ convert_to_iter_based=True),
+ dict(
+ type=CosineAnnealingLR,
+ eta_min=0.0,
+ by_epoch=True,
+ begin=warmup_ratio * max_epochs,
+ end=max_epochs,
+ convert_to_iter_based=True)
+]
+
+# train, val, test setting
+train_cfg = dict(type=TrainLoop, max_epochs=max_epochs)
+
+#######################################################################
+# PART 5 Runtime #
+#######################################################################
+# Log the dialogue periodically during the training process, optional
+custom_hooks = [
+ dict(type=DatasetInfoHook, tokenizer=tokenizer),
+ dict(
+ type=EvaluateChatHook,
+ tokenizer=tokenizer,
+ every_n_iters=evaluation_freq,
+ evaluation_inputs=evaluation_inputs,
+ system=SYSTEM,
+ prompt_template=prompt_template)
+]
+
+if use_varlen_attn:
+ custom_hooks += [dict(type=VarlenAttnArgsToMessageHubHook)]
+
+# configure default hooks
+default_hooks = dict(
+ # record the time of every iteration.
+ timer=dict(type=IterTimerHook),
+ # print log every 10 iterations.
+ logger=dict(type=LoggerHook, log_metric_by_epoch=False, interval=10),
+ # enable the parameter scheduler.
+ param_scheduler=dict(type=ParamSchedulerHook),
+ # save checkpoint per `save_steps`.
+ checkpoint=dict(
+ type=CheckpointHook,
+ by_epoch=False,
+ interval=save_steps,
+ max_keep_ckpts=save_total_limit),
+ # set sampler seed in distributed evrionment.
+ sampler_seed=dict(type=DistSamplerSeedHook),
+)
+
+# configure environment
+env_cfg = dict(
+ # whether to enable cudnn benchmark
+ cudnn_benchmark=False,
+ # set multi process parameters
+ mp_cfg=dict(mp_start_method='fork', opencv_num_threads=0),
+ # set distributed parameters
+ dist_cfg=dict(backend='nccl'),
+)
+
+# set visualizer
+visualizer = None
+
+# set log level
+log_level = 'INFO'
+
+# load from which checkpoint
+load_from = None
+
+# whether to resume training from the loaded checkpoint
+resume = False
+
+# Defaults to use random seed and disable `deterministic`
+randomness = dict(seed=None, deterministic=False)
+
+# set log processor
+log_processor = dict(by_epoch=False)
diff --git a/xtuner/configs/custom_dataset/sft/llama/llama2_70b_qlora_custom_sft_e1.py b/xtuner/configs/custom_dataset/sft/llama/llama2_70b_qlora_custom_sft_e1.py
new file mode 100644
index 000000000..2b0f889b4
--- /dev/null
+++ b/xtuner/configs/custom_dataset/sft/llama/llama2_70b_qlora_custom_sft_e1.py
@@ -0,0 +1,227 @@
+# Copyright (c) OpenMMLab. All rights reserved.
+"""Data format:
+
+[{
+ "messages": [
+ { "role": "system", "content": "xxx." },
+ { "role": "user", "content": "xxx." },
+ { "role": "assistant", "content": "xxx.", "loss": false},
+ { "role": "user", "content": "xxx." },
+ { "role": "assistant", "content": "xxx.", "loss": true}
+ ]
+},
+...
+]
+""" # noqa: E501
+import torch
+from datasets import load_dataset
+from mmengine.dataset import DefaultSampler
+from mmengine.hooks import (CheckpointHook, DistSamplerSeedHook, IterTimerHook,
+ LoggerHook, ParamSchedulerHook)
+from mmengine.optim import AmpOptimWrapper, CosineAnnealingLR, LinearLR
+from peft import LoraConfig
+from torch.optim import AdamW
+from transformers import (AutoModelForCausalLM, AutoTokenizer,
+ BitsAndBytesConfig)
+
+from xtuner.dataset import process_hf_dataset
+from xtuner.dataset.collate_fns import default_collate_fn
+from xtuner.dataset.map_fns import openai_map_fn, template_map_fn_factory
+from xtuner.engine.hooks import (DatasetInfoHook, EvaluateChatHook,
+ VarlenAttnArgsToMessageHubHook)
+from xtuner.engine.runner import TrainLoop
+from xtuner.model import SupervisedFinetune
+from xtuner.utils import PROMPT_TEMPLATE
+
+#######################################################################
+# PART 1 Settings #
+#######################################################################
+# Model
+pretrained_model_name_or_path = 'meta-llama/Llama-2-70b-hf'
+use_varlen_attn = False
+
+# Data
+data_files = ['/path/to/json/file.json']
+prompt_template = PROMPT_TEMPLATE.llama2_chat
+max_length = 2048
+pack_to_max_length = True
+
+# Scheduler & Optimizer
+batch_size = 1 # per_device
+accumulative_counts = 16 # bs = 1 GPU * 1 batch_size_per_device * 16 acc
+dataloader_num_workers = 0
+max_epochs = 1
+optim_type = AdamW
+lr = 3e-4
+betas = (0.9, 0.999)
+weight_decay = 0
+max_norm = 1 # grad clip
+warmup_ratio = 0.03
+
+# Save
+save_steps = 500
+save_total_limit = 2 # Maximum checkpoints to keep (-1 means unlimited)
+
+# Evaluate the generation performance during the training
+evaluation_freq = 500
+SYSTEM = ''
+evaluation_inputs = [
+ '请给我介绍五个上海的景点', 'Please tell me five scenic spots in Shanghai'
+]
+
+#######################################################################
+# PART 2 Model & Tokenizer #
+#######################################################################
+tokenizer = dict(
+ type=AutoTokenizer.from_pretrained,
+ pretrained_model_name_or_path=pretrained_model_name_or_path,
+ trust_remote_code=True,
+ padding_side='right')
+
+model = dict(
+ type=SupervisedFinetune,
+ use_varlen_attn=use_varlen_attn,
+ llm=dict(
+ type=AutoModelForCausalLM.from_pretrained,
+ pretrained_model_name_or_path=pretrained_model_name_or_path,
+ trust_remote_code=True,
+ torch_dtype=torch.float16,
+ quantization_config=dict(
+ type=BitsAndBytesConfig,
+ load_in_4bit=True,
+ load_in_8bit=False,
+ llm_int8_threshold=6.0,
+ llm_int8_has_fp16_weight=False,
+ bnb_4bit_compute_dtype=torch.float16,
+ bnb_4bit_use_double_quant=True,
+ bnb_4bit_quant_type='nf4')),
+ lora=dict(
+ type=LoraConfig,
+ r=64,
+ lora_alpha=16,
+ lora_dropout=0.1,
+ target_modules=['gate_proj', 'down_proj', 'up_proj'],
+ bias='none',
+ task_type='CAUSAL_LM'))
+
+#######################################################################
+# PART 3 Dataset & Dataloader #
+#######################################################################
+train_dataset = dict(
+ type=process_hf_dataset,
+ dataset=dict(type=load_dataset, path='json', data_files=data_files),
+ tokenizer=tokenizer,
+ max_length=max_length,
+ dataset_map_fn=openai_map_fn,
+ template_map_fn=dict(
+ type=template_map_fn_factory, template=prompt_template),
+ remove_unused_columns=True,
+ shuffle_before_pack=True,
+ pack_to_max_length=pack_to_max_length,
+ use_varlen_attn=use_varlen_attn)
+
+train_dataloader = dict(
+ batch_size=batch_size,
+ num_workers=dataloader_num_workers,
+ dataset=train_dataset,
+ sampler=dict(type=DefaultSampler, shuffle=True),
+ collate_fn=dict(type=default_collate_fn, use_varlen_attn=use_varlen_attn))
+
+#######################################################################
+# PART 4 Scheduler & Optimizer #
+#######################################################################
+# optimizer
+optim_wrapper = dict(
+ type=AmpOptimWrapper,
+ optimizer=dict(
+ type=optim_type, lr=lr, betas=betas, weight_decay=weight_decay),
+ clip_grad=dict(max_norm=max_norm, error_if_nonfinite=False),
+ accumulative_counts=accumulative_counts,
+ loss_scale='dynamic',
+ dtype='float16')
+
+# learning policy
+# More information: https://github.com/open-mmlab/mmengine/blob/main/docs/en/tutorials/param_scheduler.md # noqa: E501
+param_scheduler = [
+ dict(
+ type=LinearLR,
+ start_factor=1e-5,
+ by_epoch=True,
+ begin=0,
+ end=warmup_ratio * max_epochs,
+ convert_to_iter_based=True),
+ dict(
+ type=CosineAnnealingLR,
+ eta_min=0.0,
+ by_epoch=True,
+ begin=warmup_ratio * max_epochs,
+ end=max_epochs,
+ convert_to_iter_based=True)
+]
+
+# train, val, test setting
+train_cfg = dict(type=TrainLoop, max_epochs=max_epochs)
+
+#######################################################################
+# PART 5 Runtime #
+#######################################################################
+# Log the dialogue periodically during the training process, optional
+custom_hooks = [
+ dict(type=DatasetInfoHook, tokenizer=tokenizer),
+ dict(
+ type=EvaluateChatHook,
+ tokenizer=tokenizer,
+ every_n_iters=evaluation_freq,
+ evaluation_inputs=evaluation_inputs,
+ system=SYSTEM,
+ prompt_template=prompt_template)
+]
+
+if use_varlen_attn:
+ custom_hooks += [dict(type=VarlenAttnArgsToMessageHubHook)]
+
+# configure default hooks
+default_hooks = dict(
+ # record the time of every iteration.
+ timer=dict(type=IterTimerHook),
+ # print log every 10 iterations.
+ logger=dict(type=LoggerHook, log_metric_by_epoch=False, interval=10),
+ # enable the parameter scheduler.
+ param_scheduler=dict(type=ParamSchedulerHook),
+ # save checkpoint per `save_steps`.
+ checkpoint=dict(
+ type=CheckpointHook,
+ by_epoch=False,
+ interval=save_steps,
+ max_keep_ckpts=save_total_limit),
+ # set sampler seed in distributed evrionment.
+ sampler_seed=dict(type=DistSamplerSeedHook),
+)
+
+# configure environment
+env_cfg = dict(
+ # whether to enable cudnn benchmark
+ cudnn_benchmark=False,
+ # set multi process parameters
+ mp_cfg=dict(mp_start_method='fork', opencv_num_threads=0),
+ # set distributed parameters
+ dist_cfg=dict(backend='nccl'),
+)
+
+# set visualizer
+visualizer = None
+
+# set log level
+log_level = 'INFO'
+
+# load from which checkpoint
+load_from = None
+
+# whether to resume training from the loaded checkpoint
+resume = False
+
+# Defaults to use random seed and disable `deterministic`
+randomness = dict(seed=None, deterministic=False)
+
+# set log processor
+log_processor = dict(by_epoch=False)
diff --git a/xtuner/configs/custom_dataset/sft/llama/llama2_7b_chat_qlora_custom_sft_e1.py b/xtuner/configs/custom_dataset/sft/llama/llama2_7b_chat_qlora_custom_sft_e1.py
new file mode 100644
index 000000000..9aa9b6362
--- /dev/null
+++ b/xtuner/configs/custom_dataset/sft/llama/llama2_7b_chat_qlora_custom_sft_e1.py
@@ -0,0 +1,226 @@
+# Copyright (c) OpenMMLab. All rights reserved.
+"""Data format:
+
+[{
+ "messages": [
+ { "role": "system", "content": "xxx." },
+ { "role": "user", "content": "xxx." },
+ { "role": "assistant", "content": "xxx.", "loss": false},
+ { "role": "user", "content": "xxx." },
+ { "role": "assistant", "content": "xxx.", "loss": true}
+ ]
+},
+...
+]
+""" # noqa: E501
+import torch
+from datasets import load_dataset
+from mmengine.dataset import DefaultSampler
+from mmengine.hooks import (CheckpointHook, DistSamplerSeedHook, IterTimerHook,
+ LoggerHook, ParamSchedulerHook)
+from mmengine.optim import AmpOptimWrapper, CosineAnnealingLR, LinearLR
+from peft import LoraConfig
+from torch.optim import AdamW
+from transformers import (AutoModelForCausalLM, AutoTokenizer,
+ BitsAndBytesConfig)
+
+from xtuner.dataset import process_hf_dataset
+from xtuner.dataset.collate_fns import default_collate_fn
+from xtuner.dataset.map_fns import openai_map_fn, template_map_fn_factory
+from xtuner.engine.hooks import (DatasetInfoHook, EvaluateChatHook,
+ VarlenAttnArgsToMessageHubHook)
+from xtuner.engine.runner import TrainLoop
+from xtuner.model import SupervisedFinetune
+from xtuner.utils import PROMPT_TEMPLATE
+
+#######################################################################
+# PART 1 Settings #
+#######################################################################
+# Model
+pretrained_model_name_or_path = 'meta-llama/Llama-2-7b-chat-hf'
+use_varlen_attn = False
+
+# Data
+data_files = ['/path/to/json/file.json']
+prompt_template = PROMPT_TEMPLATE.llama2_chat
+max_length = 2048
+pack_to_max_length = True
+
+# Scheduler & Optimizer
+batch_size = 1 # per_device
+accumulative_counts = 16 # bs = 1 GPU * 1 batch_size_per_device * 16 acc
+dataloader_num_workers = 0
+max_epochs = 1
+optim_type = AdamW
+lr = 2e-4
+betas = (0.9, 0.999)
+weight_decay = 0
+max_norm = 1 # grad clip
+warmup_ratio = 0.03
+
+# Save
+save_steps = 500
+save_total_limit = 2 # Maximum checkpoints to keep (-1 means unlimited)
+
+# Evaluate the generation performance during the training
+evaluation_freq = 500
+SYSTEM = ''
+evaluation_inputs = [
+ '请给我介绍五个上海的景点', 'Please tell me five scenic spots in Shanghai'
+]
+
+#######################################################################
+# PART 2 Model & Tokenizer #
+#######################################################################
+tokenizer = dict(
+ type=AutoTokenizer.from_pretrained,
+ pretrained_model_name_or_path=pretrained_model_name_or_path,
+ trust_remote_code=True,
+ padding_side='right')
+
+model = dict(
+ type=SupervisedFinetune,
+ use_varlen_attn=use_varlen_attn,
+ llm=dict(
+ type=AutoModelForCausalLM.from_pretrained,
+ pretrained_model_name_or_path=pretrained_model_name_or_path,
+ trust_remote_code=True,
+ torch_dtype=torch.float16,
+ quantization_config=dict(
+ type=BitsAndBytesConfig,
+ load_in_4bit=True,
+ load_in_8bit=False,
+ llm_int8_threshold=6.0,
+ llm_int8_has_fp16_weight=False,
+ bnb_4bit_compute_dtype=torch.float16,
+ bnb_4bit_use_double_quant=True,
+ bnb_4bit_quant_type='nf4')),
+ lora=dict(
+ type=LoraConfig,
+ r=64,
+ lora_alpha=16,
+ lora_dropout=0.1,
+ bias='none',
+ task_type='CAUSAL_LM'))
+
+#######################################################################
+# PART 3 Dataset & Dataloader #
+#######################################################################
+train_dataset = dict(
+ type=process_hf_dataset,
+ dataset=dict(type=load_dataset, path='json', data_files=data_files),
+ tokenizer=tokenizer,
+ max_length=max_length,
+ dataset_map_fn=openai_map_fn,
+ template_map_fn=dict(
+ type=template_map_fn_factory, template=prompt_template),
+ remove_unused_columns=True,
+ shuffle_before_pack=True,
+ pack_to_max_length=pack_to_max_length,
+ use_varlen_attn=use_varlen_attn)
+
+train_dataloader = dict(
+ batch_size=batch_size,
+ num_workers=dataloader_num_workers,
+ dataset=train_dataset,
+ sampler=dict(type=DefaultSampler, shuffle=True),
+ collate_fn=dict(type=default_collate_fn, use_varlen_attn=use_varlen_attn))
+
+#######################################################################
+# PART 4 Scheduler & Optimizer #
+#######################################################################
+# optimizer
+optim_wrapper = dict(
+ type=AmpOptimWrapper,
+ optimizer=dict(
+ type=optim_type, lr=lr, betas=betas, weight_decay=weight_decay),
+ clip_grad=dict(max_norm=max_norm, error_if_nonfinite=False),
+ accumulative_counts=accumulative_counts,
+ loss_scale='dynamic',
+ dtype='float16')
+
+# learning policy
+# More information: https://github.com/open-mmlab/mmengine/blob/main/docs/en/tutorials/param_scheduler.md # noqa: E501
+param_scheduler = [
+ dict(
+ type=LinearLR,
+ start_factor=1e-5,
+ by_epoch=True,
+ begin=0,
+ end=warmup_ratio * max_epochs,
+ convert_to_iter_based=True),
+ dict(
+ type=CosineAnnealingLR,
+ eta_min=0.0,
+ by_epoch=True,
+ begin=warmup_ratio * max_epochs,
+ end=max_epochs,
+ convert_to_iter_based=True)
+]
+
+# train, val, test setting
+train_cfg = dict(type=TrainLoop, max_epochs=max_epochs)
+
+#######################################################################
+# PART 5 Runtime #
+#######################################################################
+# Log the dialogue periodically during the training process, optional
+custom_hooks = [
+ dict(type=DatasetInfoHook, tokenizer=tokenizer),
+ dict(
+ type=EvaluateChatHook,
+ tokenizer=tokenizer,
+ every_n_iters=evaluation_freq,
+ evaluation_inputs=evaluation_inputs,
+ system=SYSTEM,
+ prompt_template=prompt_template)
+]
+
+if use_varlen_attn:
+ custom_hooks += [dict(type=VarlenAttnArgsToMessageHubHook)]
+
+# configure default hooks
+default_hooks = dict(
+ # record the time of every iteration.
+ timer=dict(type=IterTimerHook),
+ # print log every 10 iterations.
+ logger=dict(type=LoggerHook, log_metric_by_epoch=False, interval=10),
+ # enable the parameter scheduler.
+ param_scheduler=dict(type=ParamSchedulerHook),
+ # save checkpoint per `save_steps`.
+ checkpoint=dict(
+ type=CheckpointHook,
+ by_epoch=False,
+ interval=save_steps,
+ max_keep_ckpts=save_total_limit),
+ # set sampler seed in distributed evrionment.
+ sampler_seed=dict(type=DistSamplerSeedHook),
+)
+
+# configure environment
+env_cfg = dict(
+ # whether to enable cudnn benchmark
+ cudnn_benchmark=False,
+ # set multi process parameters
+ mp_cfg=dict(mp_start_method='fork', opencv_num_threads=0),
+ # set distributed parameters
+ dist_cfg=dict(backend='nccl'),
+)
+
+# set visualizer
+visualizer = None
+
+# set log level
+log_level = 'INFO'
+
+# load from which checkpoint
+load_from = None
+
+# whether to resume training from the loaded checkpoint
+resume = False
+
+# Defaults to use random seed and disable `deterministic`
+randomness = dict(seed=None, deterministic=False)
+
+# set log processor
+log_processor = dict(by_epoch=False)
diff --git a/xtuner/configs/custom_dataset/sft/minicpm/minicpm3_4b_chat_qlora_custom_sft_e1.py b/xtuner/configs/custom_dataset/sft/minicpm/minicpm3_4b_chat_qlora_custom_sft_e1.py
new file mode 100644
index 000000000..499d475fe
--- /dev/null
+++ b/xtuner/configs/custom_dataset/sft/minicpm/minicpm3_4b_chat_qlora_custom_sft_e1.py
@@ -0,0 +1,227 @@
+# Copyright (c) OpenMMLab. All rights reserved.
+"""Data format:
+
+[{
+ "messages": [
+ { "role": "system", "content": "xxx." },
+ { "role": "user", "content": "xxx." },
+ { "role": "assistant", "content": "xxx.", "loss": false},
+ { "role": "user", "content": "xxx." },
+ { "role": "assistant", "content": "xxx.", "loss": true}
+ ]
+},
+...
+]
+""" # noqa: E501
+import torch
+from datasets import load_dataset
+from mmengine.dataset import DefaultSampler
+from mmengine.hooks import (CheckpointHook, DistSamplerSeedHook, IterTimerHook,
+ LoggerHook, ParamSchedulerHook)
+from mmengine.optim import AmpOptimWrapper, CosineAnnealingLR, LinearLR
+from peft import LoraConfig
+from torch.optim import AdamW
+from transformers import (AutoModelForCausalLM, AutoTokenizer,
+ BitsAndBytesConfig)
+
+from xtuner.dataset import process_hf_dataset
+from xtuner.dataset.collate_fns import default_collate_fn
+from xtuner.dataset.map_fns import openai_map_fn, template_map_fn_factory
+from xtuner.engine.hooks import (DatasetInfoHook, EvaluateChatHook,
+ VarlenAttnArgsToMessageHubHook)
+from xtuner.engine.runner import TrainLoop
+from xtuner.model import SupervisedFinetune
+from xtuner.utils import PROMPT_TEMPLATE
+
+#######################################################################
+# PART 1 Settings #
+#######################################################################
+# Model
+pretrained_model_name_or_path = 'openbmb/MiniCPM3-4B'
+use_varlen_attn = False
+
+# Data
+data_files = ['/path/to/your.json']
+prompt_template = PROMPT_TEMPLATE.minicpm3
+max_length = 2048
+pack_to_max_length = True
+
+# Scheduler & Optimizer
+batch_size = 1 # per_device
+accumulative_counts = 16 # bs = 1 GPU * 1 batch_size_per_device * 16 acc
+dataloader_num_workers = 0
+max_steps = 10000
+optim_type = AdamW
+lr = 2e-4
+betas = (0.9, 0.999)
+weight_decay = 0
+max_norm = 1 # grad clip
+warmup_ratio = 0.03
+
+
+# Save
+save_steps = 500
+save_total_limit = 2 # Maximum checkpoints to keep (-1 means unlimited)
+
+# Evaluate the generation performance during the training
+evaluation_freq = 500
+SYSTEM = ''
+evaluation_inputs = [
+ '请给我介绍五个上海的景点', 'Please tell me five scenic spots in Shanghai'
+]
+
+#######################################################################
+# PART 2 Model & Tokenizer #
+#######################################################################
+tokenizer = dict(
+ type=AutoTokenizer.from_pretrained,
+ pretrained_model_name_or_path=pretrained_model_name_or_path,
+ trust_remote_code=True,
+ padding_side='right',
+ eos_token='<|im_end|>')
+
+model = dict(
+ type=SupervisedFinetune,
+ use_varlen_attn=use_varlen_attn,
+ llm=dict(
+ type=AutoModelForCausalLM.from_pretrained,
+ pretrained_model_name_or_path=pretrained_model_name_or_path,
+ trust_remote_code=True,
+ torch_dtype=torch.float16,
+ quantization_config=dict(
+ type=BitsAndBytesConfig,
+ load_in_4bit=True,
+ load_in_8bit=False,
+ llm_int8_threshold=6.0,
+ llm_int8_has_fp16_weight=False,
+ bnb_4bit_compute_dtype=torch.float16,
+ bnb_4bit_use_double_quant=True,
+ bnb_4bit_quant_type='nf4')),
+ lora=dict(
+ type=LoraConfig,
+ r=64,
+ lora_alpha=16,
+ lora_dropout=0.1,
+ bias='none',
+ task_type='CAUSAL_LM'))
+
+#######################################################################
+# PART 3 Dataset & Dataloader #
+#######################################################################
+train_dataset = dict(
+ type=process_hf_dataset,
+ dataset=dict(type=load_dataset, path='json', data_files=data_files),
+ tokenizer=tokenizer,
+ max_length=max_length,
+ dataset_map_fn=openai_map_fn,
+ template_map_fn=dict(
+ type=template_map_fn_factory, template=prompt_template),
+ remove_unused_columns=True,
+ shuffle_before_pack=True,
+ pack_to_max_length=pack_to_max_length,
+ use_varlen_attn=use_varlen_attn)
+
+train_dataloader = dict(
+ batch_size=batch_size,
+ num_workers=dataloader_num_workers,
+ dataset=train_dataset,
+ sampler=dict(type=DefaultSampler, shuffle=True),
+ collate_fn=dict(type=default_collate_fn, use_varlen_attn=use_varlen_attn))
+
+#######################################################################
+# PART 4 Scheduler & Optimizer #
+#######################################################################
+# optimizer
+optim_wrapper = dict(
+ type=AmpOptimWrapper,
+ optimizer=dict(
+ type=optim_type, lr=lr, betas=betas, weight_decay=weight_decay),
+ clip_grad=dict(max_norm=max_norm, error_if_nonfinite=False),
+ accumulative_counts=accumulative_counts,
+ loss_scale='dynamic',
+ dtype='float16')
+
+# learning policy
+param_scheduler = [
+ dict(
+ type=LinearLR,
+ start_factor=1e-5,
+ by_epoch=True,
+ begin=0,
+ end=warmup_ratio * max_steps,
+ convert_to_iter_based=True),
+ dict(
+ type=CosineAnnealingLR,
+ eta_min=0.0,
+ by_epoch=True,
+ begin=warmup_ratio * max_steps,
+ end=max_steps,
+ convert_to_iter_based=True)
+]
+
+# train, val, test setting
+train_cfg = dict(type=TrainLoop, max_iters=max_steps)
+
+#######################################################################
+# PART 5 Runtime #
+#######################################################################
+# Log the dialogue periodically during the training process, optional
+custom_hooks = [
+ dict(type=DatasetInfoHook, tokenizer=tokenizer),
+ dict(
+ type=EvaluateChatHook,
+ tokenizer=tokenizer,
+ every_n_iters=evaluation_freq,
+ evaluation_inputs=evaluation_inputs,
+ system=SYSTEM,
+ prompt_template=prompt_template)
+]
+
+if use_varlen_attn:
+ custom_hooks += [dict(type=VarlenAttnArgsToMessageHubHook)]
+
+# configure default hooks
+default_hooks = dict(
+ # record the time of every iteration.
+ timer=dict(type=IterTimerHook),
+ # print log every 10 iterations.
+ logger=dict(type=LoggerHook, log_metric_by_epoch=False, interval=10),
+ # enable the parameter scheduler.
+ param_scheduler=dict(type=ParamSchedulerHook),
+ # save checkpoint per `save_steps`.
+ checkpoint=dict(
+ type=CheckpointHook,
+ by_epoch=False,
+ interval=save_steps,
+ max_keep_ckpts=save_total_limit),
+ # set sampler seed in distributed evrionment.
+ sampler_seed=dict(type=DistSamplerSeedHook),
+)
+
+# configure environment
+env_cfg = dict(
+ # whether to enable cudnn benchmark
+ cudnn_benchmark=False,
+ # set multi process parameters
+ mp_cfg=dict(mp_start_method='fork', opencv_num_threads=0),
+ # set distributed parameters
+ dist_cfg=dict(backend='nccl'),
+)
+
+# set visualizer
+visualizer = None
+
+# set log level
+log_level = 'INFO'
+
+# load from which checkpoint
+load_from = None
+
+# whether to resume training from the loaded checkpoint
+resume = False
+
+# Defaults to use random seed and disable `deterministic`
+randomness = dict(seed=None, deterministic=False)
+
+# set log processor
+log_processor = dict(by_epoch=False)
diff --git a/xtuner/configs/custom_dataset/sft/minicpm/minicpm_1b_full_custom_pretrain_e1.py b/xtuner/configs/custom_dataset/sft/minicpm/minicpm_1b_full_custom_pretrain_e1.py
new file mode 100644
index 000000000..fc0da5ed3
--- /dev/null
+++ b/xtuner/configs/custom_dataset/sft/minicpm/minicpm_1b_full_custom_pretrain_e1.py
@@ -0,0 +1,200 @@
+# Copyright (c) OpenMMLab. All rights reserved.
+"""Data format:
+
+[
+ {
+ "text": "xxx"
+ },
+ {
+ "text": "xxx"
+ },
+ ...
+]
+""" # noqa: E501
+
+from datasets import load_dataset
+from mmengine.dataset import DefaultSampler
+from mmengine.hooks import (CheckpointHook, DistSamplerSeedHook, IterTimerHook,
+ LoggerHook, ParamSchedulerHook)
+from mmengine.optim import AmpOptimWrapper, CosineAnnealingLR, LinearLR
+from torch.optim import AdamW
+from transformers import AutoModelForCausalLM, AutoTokenizer
+
+from xtuner.dataset import process_hf_dataset
+from xtuner.dataset.collate_fns import default_collate_fn
+from xtuner.dataset.map_fns import pretrain_map_fn
+from xtuner.engine.hooks import (DatasetInfoHook, EvaluateChatHook,
+ VarlenAttnArgsToMessageHubHook)
+from xtuner.engine.runner import TrainLoop
+from xtuner.model import SupervisedFinetune
+
+#######################################################################
+# PART 1 Settings #
+#######################################################################
+# Model
+pretrained_model_name_or_path = 'openbmb/MiniCPM-1B-sft-bf16'
+use_varlen_attn = False
+
+# Data
+data_files = ['/path/to/json/file.json']
+max_length = 2048
+pack_to_max_length = True
+
+# Scheduler & Optimizer
+batch_size = 1 # per_device
+accumulative_counts = 1 # bs = 1 GPU * 1 batch_size_per_device * 16 acc
+dataloader_num_workers = 0
+max_epochs = 1
+optim_type = AdamW
+lr = 2e-5
+betas = (0.9, 0.999)
+weight_decay = 0
+max_norm = 1 # grad clip
+warmup_ratio = 0.03
+
+# Save
+save_steps = 500
+save_total_limit = 2 # Maximum checkpoints to keep (-1 means unlimited)
+
+# Evaluate the generation performance during the training
+evaluation_freq = 500
+SYSTEM = ''
+evaluation_inputs = ['上海是', 'Shanghai is']
+
+#######################################################################
+# PART 2 Model & Tokenizer #
+#######################################################################
+tokenizer = dict(
+ type=AutoTokenizer.from_pretrained,
+ pretrained_model_name_or_path=pretrained_model_name_or_path,
+ trust_remote_code=True,
+ padding_side='right',
+ eos_token='')
+
+model = dict(
+ type=SupervisedFinetune,
+ use_varlen_attn=use_varlen_attn,
+ llm=dict(
+ type=AutoModelForCausalLM.from_pretrained,
+ pretrained_model_name_or_path=pretrained_model_name_or_path,
+ trust_remote_code=True))
+
+#######################################################################
+# PART 3 Dataset & Dataloader #
+#######################################################################
+train_dataset = dict(
+ type=process_hf_dataset,
+ dataset=dict(type=load_dataset, path='json', data_files=data_files),
+ tokenizer=tokenizer,
+ max_length=max_length,
+ dataset_map_fn=pretrain_map_fn,
+ template_map_fn=None,
+ remove_unused_columns=True,
+ shuffle_before_pack=False,
+ pack_to_max_length=pack_to_max_length,
+ use_varlen_attn=use_varlen_attn)
+
+train_dataloader = dict(
+ batch_size=batch_size,
+ num_workers=dataloader_num_workers,
+ dataset=train_dataset,
+ sampler=dict(type=DefaultSampler, shuffle=True),
+ collate_fn=dict(type=default_collate_fn, use_varlen_attn=use_varlen_attn))
+
+#######################################################################
+# PART 4 Scheduler & Optimizer #
+#######################################################################
+# optimizer
+optim_wrapper = dict(
+ type=AmpOptimWrapper,
+ optimizer=dict(
+ type=optim_type, lr=lr, betas=betas, weight_decay=weight_decay),
+ clip_grad=dict(max_norm=max_norm, error_if_nonfinite=False),
+ accumulative_counts=accumulative_counts,
+ loss_scale='dynamic',
+ dtype='float16')
+
+# learning policy
+# More information: https://github.com/open-mmlab/mmengine/blob/main/docs/en/tutorials/param_scheduler.md # noqa: E501
+param_scheduler = [
+ dict(
+ type=LinearLR,
+ start_factor=1e-5,
+ by_epoch=True,
+ begin=0,
+ end=warmup_ratio * max_epochs,
+ convert_to_iter_based=True),
+ dict(
+ type=CosineAnnealingLR,
+ eta_min=0.0,
+ by_epoch=True,
+ begin=warmup_ratio * max_epochs,
+ end=max_epochs,
+ convert_to_iter_based=True)
+]
+
+# train, val, test setting
+train_cfg = dict(type=TrainLoop, max_epochs=max_epochs)
+
+#######################################################################
+# PART 5 Runtime #
+#######################################################################
+# Log the dialogue periodically during the training process, optional
+custom_hooks = [
+ dict(type=DatasetInfoHook, tokenizer=tokenizer),
+ dict(
+ type=EvaluateChatHook,
+ tokenizer=tokenizer,
+ every_n_iters=evaluation_freq,
+ evaluation_inputs=evaluation_inputs,
+ system=SYSTEM)
+]
+
+if use_varlen_attn:
+ custom_hooks += [dict(type=VarlenAttnArgsToMessageHubHook)]
+
+# configure default hooks
+default_hooks = dict(
+ # record the time of every iteration.
+ timer=dict(type=IterTimerHook),
+ # print log every 10 iterations.
+ logger=dict(type=LoggerHook, log_metric_by_epoch=False, interval=10),
+ # enable the parameter scheduler.
+ param_scheduler=dict(type=ParamSchedulerHook),
+ # save checkpoint per `save_steps`.
+ checkpoint=dict(
+ type=CheckpointHook,
+ by_epoch=False,
+ interval=save_steps,
+ max_keep_ckpts=save_total_limit),
+ # set sampler seed in distributed evrionment.
+ sampler_seed=dict(type=DistSamplerSeedHook),
+)
+
+# configure environment
+env_cfg = dict(
+ # whether to enable cudnn benchmark
+ cudnn_benchmark=False,
+ # set multi process parameters
+ mp_cfg=dict(mp_start_method='fork', opencv_num_threads=0),
+ # set distributed parameters
+ dist_cfg=dict(backend='nccl'),
+)
+
+# set visualizer
+visualizer = None
+
+# set log level
+log_level = 'INFO'
+
+# load from which checkpoint
+load_from = None
+
+# whether to resume training from the loaded checkpoint
+resume = False
+
+# Defaults to use random seed and disable `deterministic`
+randomness = dict(seed=None, deterministic=False)
+
+# set log processor
+log_processor = dict(by_epoch=False)
diff --git a/xtuner/configs/custom_dataset/sft/minicpm/minicpm_2b_full_custom_pretrain_e1.py b/xtuner/configs/custom_dataset/sft/minicpm/minicpm_2b_full_custom_pretrain_e1.py
new file mode 100644
index 000000000..160495a86
--- /dev/null
+++ b/xtuner/configs/custom_dataset/sft/minicpm/minicpm_2b_full_custom_pretrain_e1.py
@@ -0,0 +1,200 @@
+# Copyright (c) OpenMMLab. All rights reserved.
+"""Data format:
+
+[
+ {
+ "text": "xxx"
+ },
+ {
+ "text": "xxx"
+ },
+ ...
+]
+""" # noqa: E501
+
+from datasets import load_dataset
+from mmengine.dataset import DefaultSampler
+from mmengine.hooks import (CheckpointHook, DistSamplerSeedHook, IterTimerHook,
+ LoggerHook, ParamSchedulerHook)
+from mmengine.optim import AmpOptimWrapper, CosineAnnealingLR, LinearLR
+from torch.optim import AdamW
+from transformers import AutoModelForCausalLM, AutoTokenizer
+
+from xtuner.dataset import process_hf_dataset
+from xtuner.dataset.collate_fns import default_collate_fn
+from xtuner.dataset.map_fns import pretrain_map_fn
+from xtuner.engine.hooks import (DatasetInfoHook, EvaluateChatHook,
+ VarlenAttnArgsToMessageHubHook)
+from xtuner.engine.runner import TrainLoop
+from xtuner.model import SupervisedFinetune
+
+#######################################################################
+# PART 1 Settings #
+#######################################################################
+# Model
+pretrained_model_name_or_path = 'openbmb/MiniCPM-2B-sft-bf16'
+use_varlen_attn = False
+
+# Data
+data_files = ['/path/to/json/file.json']
+max_length = 2048
+pack_to_max_length = True
+
+# Scheduler & Optimizer
+batch_size = 1 # per_device
+accumulative_counts = 16 # bs = 1 GPU * 1 batch_size_per_device * 16 acc
+dataloader_num_workers = 0
+max_epochs = 1
+optim_type = AdamW
+lr = 2e-5
+betas = (0.9, 0.999)
+weight_decay = 0
+max_norm = 1 # grad clip
+warmup_ratio = 0.03
+
+# Save
+save_steps = 500
+save_total_limit = 2 # Maximum checkpoints to keep (-1 means unlimited)
+
+# Evaluate the generation performance during the training
+evaluation_freq = 500
+SYSTEM = ''
+evaluation_inputs = ['上海是', 'Shanghai is']
+
+#######################################################################
+# PART 2 Model & Tokenizer #
+#######################################################################
+tokenizer = dict(
+ type=AutoTokenizer.from_pretrained,
+ pretrained_model_name_or_path=pretrained_model_name_or_path,
+ trust_remote_code=True,
+ padding_side='right',
+ eos_token='')
+
+model = dict(
+ type=SupervisedFinetune,
+ use_varlen_attn=use_varlen_attn,
+ llm=dict(
+ type=AutoModelForCausalLM.from_pretrained,
+ pretrained_model_name_or_path=pretrained_model_name_or_path,
+ trust_remote_code=True))
+
+#######################################################################
+# PART 3 Dataset & Dataloader #
+#######################################################################
+train_dataset = dict(
+ type=process_hf_dataset,
+ dataset=dict(type=load_dataset, path='json', data_files=data_files),
+ tokenizer=tokenizer,
+ max_length=max_length,
+ dataset_map_fn=pretrain_map_fn,
+ template_map_fn=None,
+ remove_unused_columns=True,
+ shuffle_before_pack=False,
+ pack_to_max_length=pack_to_max_length,
+ use_varlen_attn=use_varlen_attn)
+
+train_dataloader = dict(
+ batch_size=batch_size,
+ num_workers=dataloader_num_workers,
+ dataset=train_dataset,
+ sampler=dict(type=DefaultSampler, shuffle=True),
+ collate_fn=dict(type=default_collate_fn, use_varlen_attn=use_varlen_attn))
+
+#######################################################################
+# PART 4 Scheduler & Optimizer #
+#######################################################################
+# optimizer
+optim_wrapper = dict(
+ type=AmpOptimWrapper,
+ optimizer=dict(
+ type=optim_type, lr=lr, betas=betas, weight_decay=weight_decay),
+ clip_grad=dict(max_norm=max_norm, error_if_nonfinite=False),
+ accumulative_counts=accumulative_counts,
+ loss_scale='dynamic',
+ dtype='float16')
+
+# learning policy
+# More information: https://github.com/open-mmlab/mmengine/blob/main/docs/en/tutorials/param_scheduler.md # noqa: E501
+param_scheduler = [
+ dict(
+ type=LinearLR,
+ start_factor=1e-5,
+ by_epoch=True,
+ begin=0,
+ end=warmup_ratio * max_epochs,
+ convert_to_iter_based=True),
+ dict(
+ type=CosineAnnealingLR,
+ eta_min=0.0,
+ by_epoch=True,
+ begin=warmup_ratio * max_epochs,
+ end=max_epochs,
+ convert_to_iter_based=True)
+]
+
+# train, val, test setting
+train_cfg = dict(type=TrainLoop, max_epochs=max_epochs)
+
+#######################################################################
+# PART 5 Runtime #
+#######################################################################
+# Log the dialogue periodically during the training process, optional
+custom_hooks = [
+ dict(type=DatasetInfoHook, tokenizer=tokenizer),
+ dict(
+ type=EvaluateChatHook,
+ tokenizer=tokenizer,
+ every_n_iters=evaluation_freq,
+ evaluation_inputs=evaluation_inputs,
+ system=SYSTEM)
+]
+
+if use_varlen_attn:
+ custom_hooks += [dict(type=VarlenAttnArgsToMessageHubHook)]
+
+# configure default hooks
+default_hooks = dict(
+ # record the time of every iteration.
+ timer=dict(type=IterTimerHook),
+ # print log every 10 iterations.
+ logger=dict(type=LoggerHook, log_metric_by_epoch=False, interval=10),
+ # enable the parameter scheduler.
+ param_scheduler=dict(type=ParamSchedulerHook),
+ # save checkpoint per `save_steps`.
+ checkpoint=dict(
+ type=CheckpointHook,
+ by_epoch=False,
+ interval=save_steps,
+ max_keep_ckpts=save_total_limit),
+ # set sampler seed in distributed evrionment.
+ sampler_seed=dict(type=DistSamplerSeedHook),
+)
+
+# configure environment
+env_cfg = dict(
+ # whether to enable cudnn benchmark
+ cudnn_benchmark=False,
+ # set multi process parameters
+ mp_cfg=dict(mp_start_method='fork', opencv_num_threads=0),
+ # set distributed parameters
+ dist_cfg=dict(backend='nccl'),
+)
+
+# set visualizer
+visualizer = None
+
+# set log level
+log_level = 'INFO'
+
+# load from which checkpoint
+load_from = None
+
+# whether to resume training from the loaded checkpoint
+resume = False
+
+# Defaults to use random seed and disable `deterministic`
+randomness = dict(seed=None, deterministic=False)
+
+# set log processor
+log_processor = dict(by_epoch=False)
diff --git a/xtuner/configs/custom_dataset/sft/mistral/mistral_7b_full_finetune_custom_sft_e1.py b/xtuner/configs/custom_dataset/sft/mistral/mistral_7b_full_finetune_custom_sft_e1.py
new file mode 100644
index 000000000..0af78f79f
--- /dev/null
+++ b/xtuner/configs/custom_dataset/sft/mistral/mistral_7b_full_finetune_custom_sft_e1.py
@@ -0,0 +1,234 @@
+# Copyright (c) OpenMMLab. All rights reserved.
+"""Data format:
+
+[{
+ "messages": [
+ { "role": "system", "content": "xxx." },
+ { "role": "user", "content": "xxx." },
+ { "role": "assistant", "content": "xxx.", "loss": false},
+ { "role": "user", "content": "xxx." },
+ { "role": "assistant", "content": "xxx.", "loss": true}
+ ]
+},
+...
+]
+""" # noqa: E501
+import torch
+from datasets import load_dataset
+from mmengine.hooks import (CheckpointHook, DistSamplerSeedHook, IterTimerHook,
+ LoggerHook, ParamSchedulerHook)
+from mmengine.optim import AmpOptimWrapper, CosineAnnealingLR, LinearLR
+from peft import LoraConfig
+from torch.optim import AdamW
+from torch.utils.data import BatchSampler
+from transformers import (AutoModelForCausalLM, AutoTokenizer,
+ BitsAndBytesConfig)
+
+from xtuner.dataset import process_hf_dataset
+from xtuner.dataset.collate_fns import default_collate_fn
+from xtuner.dataset.map_fns import openai_map_fn, template_map_fn_factory
+from xtuner.dataset.samplers import InternRepoSampler
+from xtuner.engine import (DatasetInfoHook, EvaluateChatHook, ThroughputHook,
+ VarlenAttnArgsToMessageHubHook)
+from xtuner.engine.runner import TrainLoop
+from xtuner.model import SupervisedFinetune
+from xtuner.utils import PROMPT_TEMPLATE
+
+#######################################################################
+# PART 1 Settings #
+#######################################################################
+# Model
+pretrained_model_name_or_path = 'mistralai/Mistral-7B-v0.1'
+use_varlen_attn = True
+
+# Data
+data_files = ['/path/to/json/file.json']
+prompt_template = PROMPT_TEMPLATE.mistral
+max_length = 32768
+pack_to_max_length = True
+
+# Scheduler & Optimizer
+batch_size = 1 # per_device
+accumulative_counts = 16 # bs = 1 GPU * 1 batch_size_per_device * 16 acc
+dataloader_num_workers = 0
+max_epochs = 1
+optim_type = AdamW
+lr = 2e-4
+betas = (0.9, 0.999)
+weight_decay = 0
+max_norm = 1 # grad clip
+warmup_ratio = 0.03
+
+# Save
+save_steps = 500
+save_total_limit = 2 # Maximum checkpoints to keep (-1 means unlimited)
+
+# Evaluate the generation performance during the training
+evaluation_freq = 500
+SYSTEM = ''
+evaluation_inputs = [
+ '请给我介绍五个上海的景点', 'Please tell me five scenic spots in Shanghai'
+]
+
+#######################################################################
+# PART 2 Model & Tokenizer #
+#######################################################################
+tokenizer = dict(
+ type=AutoTokenizer.from_pretrained,
+ pretrained_model_name_or_path=pretrained_model_name_or_path,
+ trust_remote_code=True,
+ padding_side='right')
+
+model = dict(
+ type=SupervisedFinetune,
+ use_varlen_attn=use_varlen_attn,
+ llm=dict(
+ type=AutoModelForCausalLM.from_pretrained,
+ pretrained_model_name_or_path=pretrained_model_name_or_path,
+ trust_remote_code=True,
+ torch_dtype=torch.bfloat16,
+ attn_implementation='flash_attention_2',
+ quantization_config=dict(
+ type=BitsAndBytesConfig,
+ load_in_4bit=True,
+ load_in_8bit=False,
+ llm_int8_threshold=6.0,
+ llm_int8_has_fp16_weight=False,
+ bnb_4bit_compute_dtype=torch.float16,
+ bnb_4bit_use_double_quant=True,
+ bnb_4bit_quant_type='nf4')),
+ lora=dict(
+ type=LoraConfig,
+ r=64,
+ lora_alpha=16,
+ lora_dropout=0.1,
+ bias='none',
+ task_type='CAUSAL_LM'))
+
+#######################################################################
+# PART 3 Dataset & Dataloader #
+#######################################################################
+train_dataset = dict(
+ type=process_hf_dataset,
+ use_varlen_attn=use_varlen_attn,
+ dataset=dict(type=load_dataset, path='json', data_files=data_files),
+ tokenizer=tokenizer,
+ max_length=max_length,
+ dataset_map_fn=openai_map_fn,
+ template_map_fn=dict(
+ type=template_map_fn_factory, template=prompt_template),
+ remove_unused_columns=True,
+ shuffle_before_pack=True,
+ pack_to_max_length=pack_to_max_length)
+
+train_dataloader = dict(
+ batch_size=batch_size,
+ num_workers=dataloader_num_workers,
+ dataset=train_dataset,
+ sampler=dict(type=InternRepoSampler, shuffle=True, seed=1024),
+ batch_sampler=dict(type=BatchSampler, drop_last=True, batch_size=1),
+ collate_fn=dict(type=default_collate_fn, use_varlen_attn=use_varlen_attn))
+
+#######################################################################
+# PART 4 Scheduler & Optimizer #
+#######################################################################
+# optimizer
+optim_wrapper = dict(
+ type=AmpOptimWrapper,
+ optimizer=dict(
+ type=optim_type, lr=lr, betas=betas, weight_decay=weight_decay),
+ clip_grad=dict(max_norm=max_norm, error_if_nonfinite=False),
+ accumulative_counts=accumulative_counts,
+ loss_scale='dynamic',
+)
+
+# learning policy
+# More information: https://github.com/open-mmlab/mmengine/blob/main/docs/en/tutorials/param_scheduler.md # noqa: E501
+param_scheduler = [
+ dict(
+ type=LinearLR,
+ start_factor=1e-5,
+ by_epoch=True,
+ begin=0,
+ end=warmup_ratio * max_epochs,
+ convert_to_iter_based=True),
+ dict(
+ type=CosineAnnealingLR,
+ eta_min=0.0,
+ by_epoch=True,
+ begin=warmup_ratio * max_epochs,
+ end=max_epochs,
+ convert_to_iter_based=True)
+]
+
+# train, val, test setting
+train_cfg = dict(type=TrainLoop, max_epochs=max_epochs)
+
+#######################################################################
+# PART 5 Runtime #
+#######################################################################
+# Log the dialogue periodically during the training process, optional
+custom_hooks = [
+ dict(
+ type=DatasetInfoHook, tokenizer=tokenizer,
+ is_intern_repo_dataset=True),
+ dict(
+ type=EvaluateChatHook,
+ tokenizer=tokenizer,
+ every_n_iters=evaluation_freq,
+ evaluation_inputs=evaluation_inputs,
+ system=SYSTEM,
+ prompt_template=prompt_template),
+ dict(type=ThroughputHook)
+]
+
+if use_varlen_attn:
+ custom_hooks += [dict(type=VarlenAttnArgsToMessageHubHook)]
+
+# configure default hooks
+default_hooks = dict(
+ # record the time of every iteration.
+ timer=dict(type=IterTimerHook),
+ # print log every 100 iterations.
+ logger=dict(type=LoggerHook, log_metric_by_epoch=False, interval=1),
+ # enable the parameter scheduler.
+ param_scheduler=dict(type=ParamSchedulerHook),
+ # save checkpoint per `save_steps`.
+ checkpoint=dict(
+ type=CheckpointHook,
+ by_epoch=False,
+ interval=save_steps,
+ max_keep_ckpts=save_total_limit),
+ # set sampler seed in distributed evrionment.
+ sampler_seed=dict(type=DistSamplerSeedHook),
+)
+
+# configure environment
+env_cfg = dict(
+ # whether to enable cudnn benchmark
+ cudnn_benchmark=False,
+ # set multi process parameters
+ mp_cfg=dict(mp_start_method='fork', opencv_num_threads=0),
+ # set distributed parameters
+ dist_cfg=dict(backend='nccl'),
+)
+
+# set visualizer
+visualizer = None
+
+# set log level
+log_level = 'INFO'
+
+# load from which checkpoint
+load_from = None
+
+# whether to resume training from the loaded checkpoint
+resume = False
+
+# Defaults to use random seed and disable `deterministic`
+randomness = dict(seed=None, deterministic=False)
+
+log_processor = dict(
+ by_epoch=False,
+ window_size=1,
+ mean_pattern=r'.*(loss|time|data_time|grad_norm|tflops).*')
diff --git a/xtuner/configs/custom_dataset/sft/mixtral/mixtral_8x7b_instruct_qlora_custom_sft_e1.py b/xtuner/configs/custom_dataset/sft/mixtral/mixtral_8x7b_instruct_qlora_custom_sft_e1.py
new file mode 100644
index 000000000..91cda57ec
--- /dev/null
+++ b/xtuner/configs/custom_dataset/sft/mixtral/mixtral_8x7b_instruct_qlora_custom_sft_e1.py
@@ -0,0 +1,229 @@
+# Copyright (c) OpenMMLab. All rights reserved.
+"""Data format:
+
+[{
+ "messages": [
+ { "role": "system", "content": "xxx." },
+ { "role": "user", "content": "xxx." },
+ { "role": "assistant", "content": "xxx.", "loss": false},
+ { "role": "user", "content": "xxx." },
+ { "role": "assistant", "content": "xxx.", "loss": true}
+ ]
+},
+...
+]
+""" # noqa: E501
+import torch
+from datasets import load_dataset
+from mmengine.dataset import DefaultSampler
+from mmengine.hooks import (CheckpointHook, DistSamplerSeedHook, IterTimerHook,
+ LoggerHook, ParamSchedulerHook)
+from mmengine.optim import AmpOptimWrapper, CosineAnnealingLR, LinearLR
+from peft import LoraConfig
+from torch.optim import AdamW
+from transformers import (AutoModelForCausalLM, AutoTokenizer,
+ BitsAndBytesConfig)
+
+from xtuner.dataset import process_hf_dataset
+from xtuner.dataset.collate_fns import default_collate_fn
+from xtuner.dataset.map_fns import openai_map_fn, template_map_fn_factory
+from xtuner.engine.hooks import (DatasetInfoHook, EvaluateChatHook,
+ VarlenAttnArgsToMessageHubHook)
+from xtuner.engine.runner import TrainLoop
+from xtuner.model import SupervisedFinetune
+from xtuner.utils import PROMPT_TEMPLATE
+
+#######################################################################
+# PART 1 Settings #
+#######################################################################
+# Model
+pretrained_model_name_or_path = 'mistralai/Mixtral-8x7B-Instruct-v0.1'
+use_varlen_attn = False
+
+# Data
+data_files = ['/path/to/json/file.json']
+prompt_template = PROMPT_TEMPLATE.mixtral
+max_length = 2048
+pack_to_max_length = True
+
+# Scheduler & Optimizer
+batch_size = 1 # per_device
+accumulative_counts = 16 # bs = 1 GPU * 1 batch_size_per_device * 16 acc
+dataloader_num_workers = 0
+max_epochs = 1
+optim_type = AdamW
+lr = 2e-4
+betas = (0.9, 0.999)
+weight_decay = 0
+max_norm = 1 # grad clip
+warmup_ratio = 0.03
+
+# Save
+save_steps = 500
+save_total_limit = 2 # Maximum checkpoints to keep (-1 means unlimited)
+
+# Evaluate the generation performance during the training
+evaluation_freq = 500
+SYSTEM = ''
+evaluation_inputs = [
+ '请给我介绍五个上海的景点', 'Please tell me five scenic spots in Shanghai'
+]
+
+#######################################################################
+# PART 2 Model & Tokenizer #
+#######################################################################
+tokenizer = dict(
+ type=AutoTokenizer.from_pretrained,
+ pretrained_model_name_or_path=pretrained_model_name_or_path,
+ trust_remote_code=True,
+ padding_side='right')
+
+model = dict(
+ type=SupervisedFinetune,
+ use_varlen_attn=use_varlen_attn,
+ llm=dict(
+ type=AutoModelForCausalLM.from_pretrained,
+ pretrained_model_name_or_path=pretrained_model_name_or_path,
+ trust_remote_code=True,
+ torch_dtype=torch.float16,
+ quantization_config=dict(
+ type=BitsAndBytesConfig,
+ load_in_4bit=True,
+ load_in_8bit=False,
+ llm_int8_threshold=6.0,
+ llm_int8_has_fp16_weight=False,
+ bnb_4bit_compute_dtype=torch.float16,
+ bnb_4bit_use_double_quant=True,
+ bnb_4bit_quant_type='nf4')),
+ lora=dict(
+ type=LoraConfig,
+ r=64,
+ lora_alpha=16,
+ lora_dropout=0.1,
+ target_modules=[
+ 'q_proj', 'k_proj', 'v_proj', 'o_proj', 'w1', 'w2', 'w3'
+ ],
+ bias='none',
+ task_type='CAUSAL_LM'))
+
+#######################################################################
+# PART 3 Dataset & Dataloader #
+#######################################################################
+train_dataset = dict(
+ type=process_hf_dataset,
+ dataset=dict(type=load_dataset, path='json', data_files=data_files),
+ tokenizer=tokenizer,
+ max_length=max_length,
+ dataset_map_fn=openai_map_fn,
+ template_map_fn=dict(
+ type=template_map_fn_factory, template=prompt_template),
+ remove_unused_columns=True,
+ shuffle_before_pack=True,
+ pack_to_max_length=pack_to_max_length,
+ use_varlen_attn=use_varlen_attn)
+
+train_dataloader = dict(
+ batch_size=batch_size,
+ num_workers=dataloader_num_workers,
+ dataset=train_dataset,
+ sampler=dict(type=DefaultSampler, shuffle=True),
+ collate_fn=dict(type=default_collate_fn, use_varlen_attn=use_varlen_attn))
+
+#######################################################################
+# PART 4 Scheduler & Optimizer #
+#######################################################################
+# optimizer
+optim_wrapper = dict(
+ type=AmpOptimWrapper,
+ optimizer=dict(
+ type=optim_type, lr=lr, betas=betas, weight_decay=weight_decay),
+ clip_grad=dict(max_norm=max_norm, error_if_nonfinite=False),
+ accumulative_counts=accumulative_counts,
+ loss_scale='dynamic',
+ dtype='float16')
+
+# learning policy
+# More information: https://github.com/open-mmlab/mmengine/blob/main/docs/en/tutorials/param_scheduler.md # noqa: E501
+param_scheduler = [
+ dict(
+ type=LinearLR,
+ start_factor=1e-5,
+ by_epoch=True,
+ begin=0,
+ end=warmup_ratio * max_epochs,
+ convert_to_iter_based=True),
+ dict(
+ type=CosineAnnealingLR,
+ eta_min=0.0,
+ by_epoch=True,
+ begin=warmup_ratio * max_epochs,
+ end=max_epochs,
+ convert_to_iter_based=True)
+]
+
+# train, val, test setting
+train_cfg = dict(type=TrainLoop, max_epochs=max_epochs)
+
+#######################################################################
+# PART 5 Runtime #
+#######################################################################
+# Log the dialogue periodically during the training process, optional
+custom_hooks = [
+ dict(type=DatasetInfoHook, tokenizer=tokenizer),
+ dict(
+ type=EvaluateChatHook,
+ tokenizer=tokenizer,
+ every_n_iters=evaluation_freq,
+ evaluation_inputs=evaluation_inputs,
+ system=SYSTEM,
+ prompt_template=prompt_template)
+]
+
+if use_varlen_attn:
+ custom_hooks += [dict(type=VarlenAttnArgsToMessageHubHook)]
+
+# configure default hooks
+default_hooks = dict(
+ # record the time of every iteration.
+ timer=dict(type=IterTimerHook),
+ # print log every 10 iterations.
+ logger=dict(type=LoggerHook, log_metric_by_epoch=False, interval=10),
+ # enable the parameter scheduler.
+ param_scheduler=dict(type=ParamSchedulerHook),
+ # save checkpoint per `save_steps`.
+ checkpoint=dict(
+ type=CheckpointHook,
+ by_epoch=False,
+ interval=save_steps,
+ max_keep_ckpts=save_total_limit),
+ # set sampler seed in distributed evrionment.
+ sampler_seed=dict(type=DistSamplerSeedHook),
+)
+
+# configure environment
+env_cfg = dict(
+ # whether to enable cudnn benchmark
+ cudnn_benchmark=False,
+ # set multi process parameters
+ mp_cfg=dict(mp_start_method='fork', opencv_num_threads=0),
+ # set distributed parameters
+ dist_cfg=dict(backend='nccl'),
+)
+
+# set visualizer
+visualizer = None
+
+# set log level
+log_level = 'INFO'
+
+# load from which checkpoint
+load_from = None
+
+# whether to resume training from the loaded checkpoint
+resume = False
+
+# Defaults to use random seed and disable `deterministic`
+randomness = dict(seed=None, deterministic=False)
+
+# set log processor
+log_processor = dict(by_epoch=False)
diff --git a/xtuner/configs/custom_dataset/sft/qwen/qwen1_5_0_5b_chat_qlora_custom_sft_e1.py b/xtuner/configs/custom_dataset/sft/qwen/qwen1_5_0_5b_chat_qlora_custom_sft_e1.py
new file mode 100644
index 000000000..3066f0be9
--- /dev/null
+++ b/xtuner/configs/custom_dataset/sft/qwen/qwen1_5_0_5b_chat_qlora_custom_sft_e1.py
@@ -0,0 +1,226 @@
+# Copyright (c) OpenMMLab. All rights reserved.
+"""Data format:
+
+[{
+ "messages": [
+ { "role": "system", "content": "xxx." },
+ { "role": "user", "content": "xxx." },
+ { "role": "assistant", "content": "xxx.", "loss": false},
+ { "role": "user", "content": "xxx." },
+ { "role": "assistant", "content": "xxx.", "loss": true}
+ ]
+},
+...
+]
+""" # noqa: E501
+import torch
+from datasets import load_dataset
+from mmengine.dataset import DefaultSampler
+from mmengine.hooks import (CheckpointHook, DistSamplerSeedHook, IterTimerHook,
+ LoggerHook, ParamSchedulerHook)
+from mmengine.optim import AmpOptimWrapper, CosineAnnealingLR, LinearLR
+from peft import LoraConfig
+from torch.optim import AdamW
+from transformers import (AutoModelForCausalLM, AutoTokenizer,
+ BitsAndBytesConfig)
+
+from xtuner.dataset import process_hf_dataset
+from xtuner.dataset.collate_fns import default_collate_fn
+from xtuner.dataset.map_fns import openai_map_fn, template_map_fn_factory
+from xtuner.engine.hooks import (DatasetInfoHook, EvaluateChatHook,
+ VarlenAttnArgsToMessageHubHook)
+from xtuner.engine.runner import TrainLoop
+from xtuner.model import SupervisedFinetune
+from xtuner.utils import PROMPT_TEMPLATE
+
+#######################################################################
+# PART 1 Settings #
+#######################################################################
+# Model
+pretrained_model_name_or_path = 'Qwen/Qwen1.5-0.5B-Chat'
+use_varlen_attn = False
+
+# Data
+data_files = ['/path/to/json/file.json']
+prompt_template = PROMPT_TEMPLATE.qwen_chat
+max_length = 2048
+pack_to_max_length = True
+
+# Scheduler & Optimizer
+batch_size = 1 # per_device
+accumulative_counts = 16 # bs = 1 GPU * 1 batch_size_per_device * 16 acc
+dataloader_num_workers = 0
+max_epochs = 1
+optim_type = AdamW
+lr = 2e-4
+betas = (0.9, 0.999)
+weight_decay = 0
+max_norm = 1 # grad clip
+warmup_ratio = 0.03
+
+# Save
+save_steps = 500
+save_total_limit = 2 # Maximum checkpoints to keep (-1 means unlimited)
+
+# Evaluate the generation performance during the training
+evaluation_freq = 500
+SYSTEM = ''
+evaluation_inputs = [
+ '请给我介绍五个上海的景点', 'Please tell me five scenic spots in Shanghai'
+]
+
+#######################################################################
+# PART 2 Model & Tokenizer #
+#######################################################################
+tokenizer = dict(
+ type=AutoTokenizer.from_pretrained,
+ pretrained_model_name_or_path=pretrained_model_name_or_path,
+ trust_remote_code=True,
+ padding_side='right')
+
+model = dict(
+ type=SupervisedFinetune,
+ use_varlen_attn=use_varlen_attn,
+ llm=dict(
+ type=AutoModelForCausalLM.from_pretrained,
+ pretrained_model_name_or_path=pretrained_model_name_or_path,
+ trust_remote_code=True,
+ torch_dtype=torch.float16,
+ quantization_config=dict(
+ type=BitsAndBytesConfig,
+ load_in_4bit=True,
+ load_in_8bit=False,
+ llm_int8_threshold=6.0,
+ llm_int8_has_fp16_weight=False,
+ bnb_4bit_compute_dtype=torch.float16,
+ bnb_4bit_use_double_quant=True,
+ bnb_4bit_quant_type='nf4')),
+ lora=dict(
+ type=LoraConfig,
+ r=64,
+ lora_alpha=16,
+ lora_dropout=0.1,
+ bias='none',
+ task_type='CAUSAL_LM'))
+
+#######################################################################
+# PART 3 Dataset & Dataloader #
+#######################################################################
+train_dataset = dict(
+ type=process_hf_dataset,
+ dataset=dict(type=load_dataset, path='json', data_files=data_files),
+ tokenizer=tokenizer,
+ max_length=max_length,
+ dataset_map_fn=openai_map_fn,
+ template_map_fn=dict(
+ type=template_map_fn_factory, template=prompt_template),
+ remove_unused_columns=True,
+ shuffle_before_pack=True,
+ pack_to_max_length=pack_to_max_length,
+ use_varlen_attn=use_varlen_attn)
+
+train_dataloader = dict(
+ batch_size=batch_size,
+ num_workers=dataloader_num_workers,
+ dataset=train_dataset,
+ sampler=dict(type=DefaultSampler, shuffle=True),
+ collate_fn=dict(type=default_collate_fn, use_varlen_attn=use_varlen_attn))
+
+#######################################################################
+# PART 4 Scheduler & Optimizer #
+#######################################################################
+# optimizer
+optim_wrapper = dict(
+ type=AmpOptimWrapper,
+ optimizer=dict(
+ type=optim_type, lr=lr, betas=betas, weight_decay=weight_decay),
+ clip_grad=dict(max_norm=max_norm, error_if_nonfinite=False),
+ accumulative_counts=accumulative_counts,
+ loss_scale='dynamic',
+ dtype='float16')
+
+# learning policy
+# More information: https://github.com/open-mmlab/mmengine/blob/main/docs/en/tutorials/param_scheduler.md # noqa: E501
+param_scheduler = [
+ dict(
+ type=LinearLR,
+ start_factor=1e-5,
+ by_epoch=True,
+ begin=0,
+ end=warmup_ratio * max_epochs,
+ convert_to_iter_based=True),
+ dict(
+ type=CosineAnnealingLR,
+ eta_min=0.0,
+ by_epoch=True,
+ begin=warmup_ratio * max_epochs,
+ end=max_epochs,
+ convert_to_iter_based=True)
+]
+
+# train, val, test setting
+train_cfg = dict(type=TrainLoop, max_epochs=max_epochs)
+
+#######################################################################
+# PART 5 Runtime #
+#######################################################################
+# Log the dialogue periodically during the training process, optional
+custom_hooks = [
+ dict(type=DatasetInfoHook, tokenizer=tokenizer),
+ dict(
+ type=EvaluateChatHook,
+ tokenizer=tokenizer,
+ every_n_iters=evaluation_freq,
+ evaluation_inputs=evaluation_inputs,
+ system=SYSTEM,
+ prompt_template=prompt_template)
+]
+
+if use_varlen_attn:
+ custom_hooks += [dict(type=VarlenAttnArgsToMessageHubHook)]
+
+# configure default hooks
+default_hooks = dict(
+ # record the time of every iteration.
+ timer=dict(type=IterTimerHook),
+ # print log every 10 iterations.
+ logger=dict(type=LoggerHook, log_metric_by_epoch=False, interval=10),
+ # enable the parameter scheduler.
+ param_scheduler=dict(type=ParamSchedulerHook),
+ # save checkpoint per `save_steps`.
+ checkpoint=dict(
+ type=CheckpointHook,
+ by_epoch=False,
+ interval=save_steps,
+ max_keep_ckpts=save_total_limit),
+ # set sampler seed in distributed evrionment.
+ sampler_seed=dict(type=DistSamplerSeedHook),
+)
+
+# configure environment
+env_cfg = dict(
+ # whether to enable cudnn benchmark
+ cudnn_benchmark=False,
+ # set multi process parameters
+ mp_cfg=dict(mp_start_method='fork', opencv_num_threads=0),
+ # set distributed parameters
+ dist_cfg=dict(backend='nccl'),
+)
+
+# set visualizer
+visualizer = None
+
+# set log level
+log_level = 'INFO'
+
+# load from which checkpoint
+load_from = None
+
+# whether to resume training from the loaded checkpoint
+resume = False
+
+# Defaults to use random seed and disable `deterministic`
+randomness = dict(seed=None, deterministic=False)
+
+# set log processor
+log_processor = dict(by_epoch=False)
diff --git a/xtuner/configs/custom_dataset/sft/qwen/qwen1_5_14b_chat_qlora_custom_sft_e1.py b/xtuner/configs/custom_dataset/sft/qwen/qwen1_5_14b_chat_qlora_custom_sft_e1.py
new file mode 100644
index 000000000..642592f0c
--- /dev/null
+++ b/xtuner/configs/custom_dataset/sft/qwen/qwen1_5_14b_chat_qlora_custom_sft_e1.py
@@ -0,0 +1,226 @@
+# Copyright (c) OpenMMLab. All rights reserved.
+"""Data format:
+
+[{
+ "messages": [
+ { "role": "system", "content": "xxx." },
+ { "role": "user", "content": "xxx." },
+ { "role": "assistant", "content": "xxx.", "loss": false},
+ { "role": "user", "content": "xxx." },
+ { "role": "assistant", "content": "xxx.", "loss": true}
+ ]
+},
+...
+]
+""" # noqa: E501
+import torch
+from datasets import load_dataset
+from mmengine.dataset import DefaultSampler
+from mmengine.hooks import (CheckpointHook, DistSamplerSeedHook, IterTimerHook,
+ LoggerHook, ParamSchedulerHook)
+from mmengine.optim import AmpOptimWrapper, CosineAnnealingLR, LinearLR
+from peft import LoraConfig
+from torch.optim import AdamW
+from transformers import (AutoModelForCausalLM, AutoTokenizer,
+ BitsAndBytesConfig)
+
+from xtuner.dataset import process_hf_dataset
+from xtuner.dataset.collate_fns import default_collate_fn
+from xtuner.dataset.map_fns import openai_map_fn, template_map_fn_factory
+from xtuner.engine.hooks import (DatasetInfoHook, EvaluateChatHook,
+ VarlenAttnArgsToMessageHubHook)
+from xtuner.engine.runner import TrainLoop
+from xtuner.model import SupervisedFinetune
+from xtuner.utils import PROMPT_TEMPLATE
+
+#######################################################################
+# PART 1 Settings #
+#######################################################################
+# Model
+pretrained_model_name_or_path = 'Qwen/Qwen1.5-14B-Chat'
+use_varlen_attn = False
+
+# Data
+data_files = ['/path/to/json/file.json']
+prompt_template = PROMPT_TEMPLATE.qwen_chat
+max_length = 2048
+pack_to_max_length = True
+
+# Scheduler & Optimizer
+batch_size = 1 # per_device
+accumulative_counts = 16 # bs = 1 GPU * 1 batch_size_per_device * 16 acc
+dataloader_num_workers = 0
+max_epochs = 1
+optim_type = AdamW
+lr = 2e-4
+betas = (0.9, 0.999)
+weight_decay = 0
+max_norm = 1 # grad clip
+warmup_ratio = 0.03
+
+# Save
+save_steps = 500
+save_total_limit = 2 # Maximum checkpoints to keep (-1 means unlimited)
+
+# Evaluate the generation performance during the training
+evaluation_freq = 500
+SYSTEM = ''
+evaluation_inputs = [
+ '请给我介绍五个上海的景点', 'Please tell me five scenic spots in Shanghai'
+]
+
+#######################################################################
+# PART 2 Model & Tokenizer #
+#######################################################################
+tokenizer = dict(
+ type=AutoTokenizer.from_pretrained,
+ pretrained_model_name_or_path=pretrained_model_name_or_path,
+ trust_remote_code=True,
+ padding_side='right')
+
+model = dict(
+ type=SupervisedFinetune,
+ use_varlen_attn=use_varlen_attn,
+ llm=dict(
+ type=AutoModelForCausalLM.from_pretrained,
+ pretrained_model_name_or_path=pretrained_model_name_or_path,
+ trust_remote_code=True,
+ torch_dtype=torch.float16,
+ quantization_config=dict(
+ type=BitsAndBytesConfig,
+ load_in_4bit=True,
+ load_in_8bit=False,
+ llm_int8_threshold=6.0,
+ llm_int8_has_fp16_weight=False,
+ bnb_4bit_compute_dtype=torch.float16,
+ bnb_4bit_use_double_quant=True,
+ bnb_4bit_quant_type='nf4')),
+ lora=dict(
+ type=LoraConfig,
+ r=64,
+ lora_alpha=16,
+ lora_dropout=0.1,
+ bias='none',
+ task_type='CAUSAL_LM'))
+
+#######################################################################
+# PART 3 Dataset & Dataloader #
+#######################################################################
+train_dataset = dict(
+ type=process_hf_dataset,
+ dataset=dict(type=load_dataset, path='json', data_files=data_files),
+ tokenizer=tokenizer,
+ max_length=max_length,
+ dataset_map_fn=openai_map_fn,
+ template_map_fn=dict(
+ type=template_map_fn_factory, template=prompt_template),
+ remove_unused_columns=True,
+ shuffle_before_pack=True,
+ pack_to_max_length=pack_to_max_length,
+ use_varlen_attn=use_varlen_attn)
+
+train_dataloader = dict(
+ batch_size=batch_size,
+ num_workers=dataloader_num_workers,
+ dataset=train_dataset,
+ sampler=dict(type=DefaultSampler, shuffle=True),
+ collate_fn=dict(type=default_collate_fn, use_varlen_attn=use_varlen_attn))
+
+#######################################################################
+# PART 4 Scheduler & Optimizer #
+#######################################################################
+# optimizer
+optim_wrapper = dict(
+ type=AmpOptimWrapper,
+ optimizer=dict(
+ type=optim_type, lr=lr, betas=betas, weight_decay=weight_decay),
+ clip_grad=dict(max_norm=max_norm, error_if_nonfinite=False),
+ accumulative_counts=accumulative_counts,
+ loss_scale='dynamic',
+ dtype='float16')
+
+# learning policy
+# More information: https://github.com/open-mmlab/mmengine/blob/main/docs/en/tutorials/param_scheduler.md # noqa: E501
+param_scheduler = [
+ dict(
+ type=LinearLR,
+ start_factor=1e-5,
+ by_epoch=True,
+ begin=0,
+ end=warmup_ratio * max_epochs,
+ convert_to_iter_based=True),
+ dict(
+ type=CosineAnnealingLR,
+ eta_min=0.0,
+ by_epoch=True,
+ begin=warmup_ratio * max_epochs,
+ end=max_epochs,
+ convert_to_iter_based=True)
+]
+
+# train, val, test setting
+train_cfg = dict(type=TrainLoop, max_epochs=max_epochs)
+
+#######################################################################
+# PART 5 Runtime #
+#######################################################################
+# Log the dialogue periodically during the training process, optional
+custom_hooks = [
+ dict(type=DatasetInfoHook, tokenizer=tokenizer),
+ dict(
+ type=EvaluateChatHook,
+ tokenizer=tokenizer,
+ every_n_iters=evaluation_freq,
+ evaluation_inputs=evaluation_inputs,
+ system=SYSTEM,
+ prompt_template=prompt_template)
+]
+
+if use_varlen_attn:
+ custom_hooks += [dict(type=VarlenAttnArgsToMessageHubHook)]
+
+# configure default hooks
+default_hooks = dict(
+ # record the time of every iteration.
+ timer=dict(type=IterTimerHook),
+ # print log every 10 iterations.
+ logger=dict(type=LoggerHook, log_metric_by_epoch=False, interval=10),
+ # enable the parameter scheduler.
+ param_scheduler=dict(type=ParamSchedulerHook),
+ # save checkpoint per `save_steps`.
+ checkpoint=dict(
+ type=CheckpointHook,
+ by_epoch=False,
+ interval=save_steps,
+ max_keep_ckpts=save_total_limit),
+ # set sampler seed in distributed evrionment.
+ sampler_seed=dict(type=DistSamplerSeedHook),
+)
+
+# configure environment
+env_cfg = dict(
+ # whether to enable cudnn benchmark
+ cudnn_benchmark=False,
+ # set multi process parameters
+ mp_cfg=dict(mp_start_method='fork', opencv_num_threads=0),
+ # set distributed parameters
+ dist_cfg=dict(backend='nccl'),
+)
+
+# set visualizer
+visualizer = None
+
+# set log level
+log_level = 'INFO'
+
+# load from which checkpoint
+load_from = None
+
+# whether to resume training from the loaded checkpoint
+resume = False
+
+# Defaults to use random seed and disable `deterministic`
+randomness = dict(seed=None, deterministic=False)
+
+# set log processor
+log_processor = dict(by_epoch=False)
diff --git a/xtuner/configs/custom_dataset/sft/qwen/qwen1_5_1_8b_chat_qlora_custom_sft_e1.py b/xtuner/configs/custom_dataset/sft/qwen/qwen1_5_1_8b_chat_qlora_custom_sft_e1.py
new file mode 100644
index 000000000..3790006d7
--- /dev/null
+++ b/xtuner/configs/custom_dataset/sft/qwen/qwen1_5_1_8b_chat_qlora_custom_sft_e1.py
@@ -0,0 +1,226 @@
+# Copyright (c) OpenMMLab. All rights reserved.
+"""Data format:
+
+[{
+ "messages": [
+ { "role": "system", "content": "xxx." },
+ { "role": "user", "content": "xxx." },
+ { "role": "assistant", "content": "xxx.", "loss": false},
+ { "role": "user", "content": "xxx." },
+ { "role": "assistant", "content": "xxx.", "loss": true}
+ ]
+},
+...
+]
+""" # noqa: E501
+import torch
+from datasets import load_dataset
+from mmengine.dataset import DefaultSampler
+from mmengine.hooks import (CheckpointHook, DistSamplerSeedHook, IterTimerHook,
+ LoggerHook, ParamSchedulerHook)
+from mmengine.optim import AmpOptimWrapper, CosineAnnealingLR, LinearLR
+from peft import LoraConfig
+from torch.optim import AdamW
+from transformers import (AutoModelForCausalLM, AutoTokenizer,
+ BitsAndBytesConfig)
+
+from xtuner.dataset import process_hf_dataset
+from xtuner.dataset.collate_fns import default_collate_fn
+from xtuner.dataset.map_fns import openai_map_fn, template_map_fn_factory
+from xtuner.engine.hooks import (DatasetInfoHook, EvaluateChatHook,
+ VarlenAttnArgsToMessageHubHook)
+from xtuner.engine.runner import TrainLoop
+from xtuner.model import SupervisedFinetune
+from xtuner.utils import PROMPT_TEMPLATE
+
+#######################################################################
+# PART 1 Settings #
+#######################################################################
+# Model
+pretrained_model_name_or_path = 'Qwen/Qwen1.5-1.8B-Chat'
+use_varlen_attn = False
+
+# Data
+data_files = ['/path/to/json/file.json']
+prompt_template = PROMPT_TEMPLATE.qwen_chat
+max_length = 2048
+pack_to_max_length = True
+
+# Scheduler & Optimizer
+batch_size = 1 # per_device
+accumulative_counts = 16 # bs = 1 GPU * 1 batch_size_per_device * 16 acc
+dataloader_num_workers = 0
+max_epochs = 1
+optim_type = AdamW
+lr = 2e-4
+betas = (0.9, 0.999)
+weight_decay = 0
+max_norm = 1 # grad clip
+warmup_ratio = 0.03
+
+# Save
+save_steps = 500
+save_total_limit = 2 # Maximum checkpoints to keep (-1 means unlimited)
+
+# Evaluate the generation performance during the training
+evaluation_freq = 500
+SYSTEM = ''
+evaluation_inputs = [
+ '请给我介绍五个上海的景点', 'Please tell me five scenic spots in Shanghai'
+]
+
+#######################################################################
+# PART 2 Model & Tokenizer #
+#######################################################################
+tokenizer = dict(
+ type=AutoTokenizer.from_pretrained,
+ pretrained_model_name_or_path=pretrained_model_name_or_path,
+ trust_remote_code=True,
+ padding_side='right')
+
+model = dict(
+ type=SupervisedFinetune,
+ use_varlen_attn=use_varlen_attn,
+ llm=dict(
+ type=AutoModelForCausalLM.from_pretrained,
+ pretrained_model_name_or_path=pretrained_model_name_or_path,
+ trust_remote_code=True,
+ torch_dtype=torch.float16,
+ quantization_config=dict(
+ type=BitsAndBytesConfig,
+ load_in_4bit=True,
+ load_in_8bit=False,
+ llm_int8_threshold=6.0,
+ llm_int8_has_fp16_weight=False,
+ bnb_4bit_compute_dtype=torch.float16,
+ bnb_4bit_use_double_quant=True,
+ bnb_4bit_quant_type='nf4')),
+ lora=dict(
+ type=LoraConfig,
+ r=64,
+ lora_alpha=16,
+ lora_dropout=0.1,
+ bias='none',
+ task_type='CAUSAL_LM'))
+
+#######################################################################
+# PART 3 Dataset & Dataloader #
+#######################################################################
+train_dataset = dict(
+ type=process_hf_dataset,
+ dataset=dict(type=load_dataset, path='json', data_files=data_files),
+ tokenizer=tokenizer,
+ max_length=max_length,
+ dataset_map_fn=openai_map_fn,
+ template_map_fn=dict(
+ type=template_map_fn_factory, template=prompt_template),
+ remove_unused_columns=True,
+ shuffle_before_pack=True,
+ pack_to_max_length=pack_to_max_length,
+ use_varlen_attn=use_varlen_attn)
+
+train_dataloader = dict(
+ batch_size=batch_size,
+ num_workers=dataloader_num_workers,
+ dataset=train_dataset,
+ sampler=dict(type=DefaultSampler, shuffle=True),
+ collate_fn=dict(type=default_collate_fn, use_varlen_attn=use_varlen_attn))
+
+#######################################################################
+# PART 4 Scheduler & Optimizer #
+#######################################################################
+# optimizer
+optim_wrapper = dict(
+ type=AmpOptimWrapper,
+ optimizer=dict(
+ type=optim_type, lr=lr, betas=betas, weight_decay=weight_decay),
+ clip_grad=dict(max_norm=max_norm, error_if_nonfinite=False),
+ accumulative_counts=accumulative_counts,
+ loss_scale='dynamic',
+ dtype='float16')
+
+# learning policy
+# More information: https://github.com/open-mmlab/mmengine/blob/main/docs/en/tutorials/param_scheduler.md # noqa: E501
+param_scheduler = [
+ dict(
+ type=LinearLR,
+ start_factor=1e-5,
+ by_epoch=True,
+ begin=0,
+ end=warmup_ratio * max_epochs,
+ convert_to_iter_based=True),
+ dict(
+ type=CosineAnnealingLR,
+ eta_min=0.0,
+ by_epoch=True,
+ begin=warmup_ratio * max_epochs,
+ end=max_epochs,
+ convert_to_iter_based=True)
+]
+
+# train, val, test setting
+train_cfg = dict(type=TrainLoop, max_epochs=max_epochs)
+
+#######################################################################
+# PART 5 Runtime #
+#######################################################################
+# Log the dialogue periodically during the training process, optional
+custom_hooks = [
+ dict(type=DatasetInfoHook, tokenizer=tokenizer),
+ dict(
+ type=EvaluateChatHook,
+ tokenizer=tokenizer,
+ every_n_iters=evaluation_freq,
+ evaluation_inputs=evaluation_inputs,
+ system=SYSTEM,
+ prompt_template=prompt_template)
+]
+
+if use_varlen_attn:
+ custom_hooks += [dict(type=VarlenAttnArgsToMessageHubHook)]
+
+# configure default hooks
+default_hooks = dict(
+ # record the time of every iteration.
+ timer=dict(type=IterTimerHook),
+ # print log every 10 iterations.
+ logger=dict(type=LoggerHook, log_metric_by_epoch=False, interval=10),
+ # enable the parameter scheduler.
+ param_scheduler=dict(type=ParamSchedulerHook),
+ # save checkpoint per `save_steps`.
+ checkpoint=dict(
+ type=CheckpointHook,
+ by_epoch=False,
+ interval=save_steps,
+ max_keep_ckpts=save_total_limit),
+ # set sampler seed in distributed evrionment.
+ sampler_seed=dict(type=DistSamplerSeedHook),
+)
+
+# configure environment
+env_cfg = dict(
+ # whether to enable cudnn benchmark
+ cudnn_benchmark=False,
+ # set multi process parameters
+ mp_cfg=dict(mp_start_method='fork', opencv_num_threads=0),
+ # set distributed parameters
+ dist_cfg=dict(backend='nccl'),
+)
+
+# set visualizer
+visualizer = None
+
+# set log level
+log_level = 'INFO'
+
+# load from which checkpoint
+load_from = None
+
+# whether to resume training from the loaded checkpoint
+resume = False
+
+# Defaults to use random seed and disable `deterministic`
+randomness = dict(seed=None, deterministic=False)
+
+# set log processor
+log_processor = dict(by_epoch=False)
diff --git a/xtuner/configs/custom_dataset/sft/qwen/qwen1_5_4b_chat_qlora_custom_sft_e1.py b/xtuner/configs/custom_dataset/sft/qwen/qwen1_5_4b_chat_qlora_custom_sft_e1.py
new file mode 100644
index 000000000..36d3e6cd0
--- /dev/null
+++ b/xtuner/configs/custom_dataset/sft/qwen/qwen1_5_4b_chat_qlora_custom_sft_e1.py
@@ -0,0 +1,226 @@
+# Copyright (c) OpenMMLab. All rights reserved.
+"""Data format:
+
+[{
+ "messages": [
+ { "role": "system", "content": "xxx." },
+ { "role": "user", "content": "xxx." },
+ { "role": "assistant", "content": "xxx.", "loss": false},
+ { "role": "user", "content": "xxx." },
+ { "role": "assistant", "content": "xxx.", "loss": true}
+ ]
+},
+...
+]
+""" # noqa: E501
+import torch
+from datasets import load_dataset
+from mmengine.dataset import DefaultSampler
+from mmengine.hooks import (CheckpointHook, DistSamplerSeedHook, IterTimerHook,
+ LoggerHook, ParamSchedulerHook)
+from mmengine.optim import AmpOptimWrapper, CosineAnnealingLR, LinearLR
+from peft import LoraConfig
+from torch.optim import AdamW
+from transformers import (AutoModelForCausalLM, AutoTokenizer,
+ BitsAndBytesConfig)
+
+from xtuner.dataset import process_hf_dataset
+from xtuner.dataset.collate_fns import default_collate_fn
+from xtuner.dataset.map_fns import openai_map_fn, template_map_fn_factory
+from xtuner.engine.hooks import (DatasetInfoHook, EvaluateChatHook,
+ VarlenAttnArgsToMessageHubHook)
+from xtuner.engine.runner import TrainLoop
+from xtuner.model import SupervisedFinetune
+from xtuner.utils import PROMPT_TEMPLATE
+
+#######################################################################
+# PART 1 Settings #
+#######################################################################
+# Model
+pretrained_model_name_or_path = 'Qwen/Qwen1.5-4B-Chat'
+use_varlen_attn = False
+
+# Data
+data_files = ['/path/to/json/file.json']
+prompt_template = PROMPT_TEMPLATE.qwen_chat
+max_length = 2048
+pack_to_max_length = True
+
+# Scheduler & Optimizer
+batch_size = 1 # per_device
+accumulative_counts = 16 # bs = 1 GPU * 1 batch_size_per_device * 16 acc
+dataloader_num_workers = 0
+max_epochs = 1
+optim_type = AdamW
+lr = 2e-4
+betas = (0.9, 0.999)
+weight_decay = 0
+max_norm = 1 # grad clip
+warmup_ratio = 0.03
+
+# Save
+save_steps = 500
+save_total_limit = 2 # Maximum checkpoints to keep (-1 means unlimited)
+
+# Evaluate the generation performance during the training
+evaluation_freq = 500
+SYSTEM = ''
+evaluation_inputs = [
+ '请给我介绍五个上海的景点', 'Please tell me five scenic spots in Shanghai'
+]
+
+#######################################################################
+# PART 2 Model & Tokenizer #
+#######################################################################
+tokenizer = dict(
+ type=AutoTokenizer.from_pretrained,
+ pretrained_model_name_or_path=pretrained_model_name_or_path,
+ trust_remote_code=True,
+ padding_side='right')
+
+model = dict(
+ type=SupervisedFinetune,
+ use_varlen_attn=use_varlen_attn,
+ llm=dict(
+ type=AutoModelForCausalLM.from_pretrained,
+ pretrained_model_name_or_path=pretrained_model_name_or_path,
+ trust_remote_code=True,
+ torch_dtype=torch.float16,
+ quantization_config=dict(
+ type=BitsAndBytesConfig,
+ load_in_4bit=True,
+ load_in_8bit=False,
+ llm_int8_threshold=6.0,
+ llm_int8_has_fp16_weight=False,
+ bnb_4bit_compute_dtype=torch.float16,
+ bnb_4bit_use_double_quant=True,
+ bnb_4bit_quant_type='nf4')),
+ lora=dict(
+ type=LoraConfig,
+ r=64,
+ lora_alpha=16,
+ lora_dropout=0.1,
+ bias='none',
+ task_type='CAUSAL_LM'))
+
+#######################################################################
+# PART 3 Dataset & Dataloader #
+#######################################################################
+train_dataset = dict(
+ type=process_hf_dataset,
+ dataset=dict(type=load_dataset, path='json', data_files=data_files),
+ tokenizer=tokenizer,
+ max_length=max_length,
+ dataset_map_fn=openai_map_fn,
+ template_map_fn=dict(
+ type=template_map_fn_factory, template=prompt_template),
+ remove_unused_columns=True,
+ shuffle_before_pack=True,
+ pack_to_max_length=pack_to_max_length,
+ use_varlen_attn=use_varlen_attn)
+
+train_dataloader = dict(
+ batch_size=batch_size,
+ num_workers=dataloader_num_workers,
+ dataset=train_dataset,
+ sampler=dict(type=DefaultSampler, shuffle=True),
+ collate_fn=dict(type=default_collate_fn, use_varlen_attn=use_varlen_attn))
+
+#######################################################################
+# PART 4 Scheduler & Optimizer #
+#######################################################################
+# optimizer
+optim_wrapper = dict(
+ type=AmpOptimWrapper,
+ optimizer=dict(
+ type=optim_type, lr=lr, betas=betas, weight_decay=weight_decay),
+ clip_grad=dict(max_norm=max_norm, error_if_nonfinite=False),
+ accumulative_counts=accumulative_counts,
+ loss_scale='dynamic',
+ dtype='float16')
+
+# learning policy
+# More information: https://github.com/open-mmlab/mmengine/blob/main/docs/en/tutorials/param_scheduler.md # noqa: E501
+param_scheduler = [
+ dict(
+ type=LinearLR,
+ start_factor=1e-5,
+ by_epoch=True,
+ begin=0,
+ end=warmup_ratio * max_epochs,
+ convert_to_iter_based=True),
+ dict(
+ type=CosineAnnealingLR,
+ eta_min=0.0,
+ by_epoch=True,
+ begin=warmup_ratio * max_epochs,
+ end=max_epochs,
+ convert_to_iter_based=True)
+]
+
+# train, val, test setting
+train_cfg = dict(type=TrainLoop, max_epochs=max_epochs)
+
+#######################################################################
+# PART 5 Runtime #
+#######################################################################
+# Log the dialogue periodically during the training process, optional
+custom_hooks = [
+ dict(type=DatasetInfoHook, tokenizer=tokenizer),
+ dict(
+ type=EvaluateChatHook,
+ tokenizer=tokenizer,
+ every_n_iters=evaluation_freq,
+ evaluation_inputs=evaluation_inputs,
+ system=SYSTEM,
+ prompt_template=prompt_template)
+]
+
+if use_varlen_attn:
+ custom_hooks += [dict(type=VarlenAttnArgsToMessageHubHook)]
+
+# configure default hooks
+default_hooks = dict(
+ # record the time of every iteration.
+ timer=dict(type=IterTimerHook),
+ # print log every 10 iterations.
+ logger=dict(type=LoggerHook, log_metric_by_epoch=False, interval=10),
+ # enable the parameter scheduler.
+ param_scheduler=dict(type=ParamSchedulerHook),
+ # save checkpoint per `save_steps`.
+ checkpoint=dict(
+ type=CheckpointHook,
+ by_epoch=False,
+ interval=save_steps,
+ max_keep_ckpts=save_total_limit),
+ # set sampler seed in distributed evrionment.
+ sampler_seed=dict(type=DistSamplerSeedHook),
+)
+
+# configure environment
+env_cfg = dict(
+ # whether to enable cudnn benchmark
+ cudnn_benchmark=False,
+ # set multi process parameters
+ mp_cfg=dict(mp_start_method='fork', opencv_num_threads=0),
+ # set distributed parameters
+ dist_cfg=dict(backend='nccl'),
+)
+
+# set visualizer
+visualizer = None
+
+# set log level
+log_level = 'INFO'
+
+# load from which checkpoint
+load_from = None
+
+# whether to resume training from the loaded checkpoint
+resume = False
+
+# Defaults to use random seed and disable `deterministic`
+randomness = dict(seed=None, deterministic=False)
+
+# set log processor
+log_processor = dict(by_epoch=False)
diff --git a/xtuner/configs/custom_dataset/sft/qwen/qwen1_5_72b_chat_qlora_custom_sft_e1.py b/xtuner/configs/custom_dataset/sft/qwen/qwen1_5_72b_chat_qlora_custom_sft_e1.py
new file mode 100644
index 000000000..d152c207d
--- /dev/null
+++ b/xtuner/configs/custom_dataset/sft/qwen/qwen1_5_72b_chat_qlora_custom_sft_e1.py
@@ -0,0 +1,226 @@
+# Copyright (c) OpenMMLab. All rights reserved.
+"""Data format:
+
+[{
+ "messages": [
+ { "role": "system", "content": "xxx." },
+ { "role": "user", "content": "xxx." },
+ { "role": "assistant", "content": "xxx.", "loss": false},
+ { "role": "user", "content": "xxx." },
+ { "role": "assistant", "content": "xxx.", "loss": true}
+ ]
+},
+...
+]
+""" # noqa: E501
+import torch
+from datasets import load_dataset
+from mmengine.dataset import DefaultSampler
+from mmengine.hooks import (CheckpointHook, DistSamplerSeedHook, IterTimerHook,
+ LoggerHook, ParamSchedulerHook)
+from mmengine.optim import AmpOptimWrapper, CosineAnnealingLR, LinearLR
+from peft import LoraConfig
+from torch.optim import AdamW
+from transformers import (AutoModelForCausalLM, AutoTokenizer,
+ BitsAndBytesConfig)
+
+from xtuner.dataset import process_hf_dataset
+from xtuner.dataset.collate_fns import default_collate_fn
+from xtuner.dataset.map_fns import openai_map_fn, template_map_fn_factory
+from xtuner.engine.hooks import (DatasetInfoHook, EvaluateChatHook,
+ VarlenAttnArgsToMessageHubHook)
+from xtuner.engine.runner import TrainLoop
+from xtuner.model import SupervisedFinetune
+from xtuner.utils import PROMPT_TEMPLATE
+
+#######################################################################
+# PART 1 Settings #
+#######################################################################
+# Model
+pretrained_model_name_or_path = 'Qwen/Qwen1.5-72B-Chat'
+use_varlen_attn = False
+
+# Data
+data_files = ['/path/to/json/file.json']
+prompt_template = PROMPT_TEMPLATE.qwen_chat
+max_length = 2048
+pack_to_max_length = True
+
+# Scheduler & Optimizer
+batch_size = 1 # per_device
+accumulative_counts = 16 # bs = 1 GPU * 1 batch_size_per_device * 16 acc
+dataloader_num_workers = 0
+max_epochs = 1
+optim_type = AdamW
+lr = 2e-4
+betas = (0.9, 0.999)
+weight_decay = 0
+max_norm = 1 # grad clip
+warmup_ratio = 0.03
+
+# Save
+save_steps = 500
+save_total_limit = 2 # Maximum checkpoints to keep (-1 means unlimited)
+
+# Evaluate the generation performance during the training
+evaluation_freq = 500
+SYSTEM = ''
+evaluation_inputs = [
+ '请给我介绍五个上海的景点', 'Please tell me five scenic spots in Shanghai'
+]
+
+#######################################################################
+# PART 2 Model & Tokenizer #
+#######################################################################
+tokenizer = dict(
+ type=AutoTokenizer.from_pretrained,
+ pretrained_model_name_or_path=pretrained_model_name_or_path,
+ trust_remote_code=True,
+ padding_side='right')
+
+model = dict(
+ type=SupervisedFinetune,
+ use_varlen_attn=use_varlen_attn,
+ llm=dict(
+ type=AutoModelForCausalLM.from_pretrained,
+ pretrained_model_name_or_path=pretrained_model_name_or_path,
+ trust_remote_code=True,
+ torch_dtype=torch.float16,
+ quantization_config=dict(
+ type=BitsAndBytesConfig,
+ load_in_4bit=True,
+ load_in_8bit=False,
+ llm_int8_threshold=6.0,
+ llm_int8_has_fp16_weight=False,
+ bnb_4bit_compute_dtype=torch.float16,
+ bnb_4bit_use_double_quant=True,
+ bnb_4bit_quant_type='nf4')),
+ lora=dict(
+ type=LoraConfig,
+ r=64,
+ lora_alpha=16,
+ lora_dropout=0.1,
+ bias='none',
+ task_type='CAUSAL_LM'))
+
+#######################################################################
+# PART 3 Dataset & Dataloader #
+#######################################################################
+train_dataset = dict(
+ type=process_hf_dataset,
+ dataset=dict(type=load_dataset, path='json', data_files=data_files),
+ tokenizer=tokenizer,
+ max_length=max_length,
+ dataset_map_fn=openai_map_fn,
+ template_map_fn=dict(
+ type=template_map_fn_factory, template=prompt_template),
+ remove_unused_columns=True,
+ shuffle_before_pack=True,
+ pack_to_max_length=pack_to_max_length,
+ use_varlen_attn=use_varlen_attn)
+
+train_dataloader = dict(
+ batch_size=batch_size,
+ num_workers=dataloader_num_workers,
+ dataset=train_dataset,
+ sampler=dict(type=DefaultSampler, shuffle=True),
+ collate_fn=dict(type=default_collate_fn, use_varlen_attn=use_varlen_attn))
+
+#######################################################################
+# PART 4 Scheduler & Optimizer #
+#######################################################################
+# optimizer
+optim_wrapper = dict(
+ type=AmpOptimWrapper,
+ optimizer=dict(
+ type=optim_type, lr=lr, betas=betas, weight_decay=weight_decay),
+ clip_grad=dict(max_norm=max_norm, error_if_nonfinite=False),
+ accumulative_counts=accumulative_counts,
+ loss_scale='dynamic',
+ dtype='float16')
+
+# learning policy
+# More information: https://github.com/open-mmlab/mmengine/blob/main/docs/en/tutorials/param_scheduler.md # noqa: E501
+param_scheduler = [
+ dict(
+ type=LinearLR,
+ start_factor=1e-5,
+ by_epoch=True,
+ begin=0,
+ end=warmup_ratio * max_epochs,
+ convert_to_iter_based=True),
+ dict(
+ type=CosineAnnealingLR,
+ eta_min=0.0,
+ by_epoch=True,
+ begin=warmup_ratio * max_epochs,
+ end=max_epochs,
+ convert_to_iter_based=True)
+]
+
+# train, val, test setting
+train_cfg = dict(type=TrainLoop, max_epochs=max_epochs)
+
+#######################################################################
+# PART 5 Runtime #
+#######################################################################
+# Log the dialogue periodically during the training process, optional
+custom_hooks = [
+ dict(type=DatasetInfoHook, tokenizer=tokenizer),
+ dict(
+ type=EvaluateChatHook,
+ tokenizer=tokenizer,
+ every_n_iters=evaluation_freq,
+ evaluation_inputs=evaluation_inputs,
+ system=SYSTEM,
+ prompt_template=prompt_template)
+]
+
+if use_varlen_attn:
+ custom_hooks += [dict(type=VarlenAttnArgsToMessageHubHook)]
+
+# configure default hooks
+default_hooks = dict(
+ # record the time of every iteration.
+ timer=dict(type=IterTimerHook),
+ # print log every 10 iterations.
+ logger=dict(type=LoggerHook, log_metric_by_epoch=False, interval=10),
+ # enable the parameter scheduler.
+ param_scheduler=dict(type=ParamSchedulerHook),
+ # save checkpoint per `save_steps`.
+ checkpoint=dict(
+ type=CheckpointHook,
+ by_epoch=False,
+ interval=save_steps,
+ max_keep_ckpts=save_total_limit),
+ # set sampler seed in distributed evrionment.
+ sampler_seed=dict(type=DistSamplerSeedHook),
+)
+
+# configure environment
+env_cfg = dict(
+ # whether to enable cudnn benchmark
+ cudnn_benchmark=False,
+ # set multi process parameters
+ mp_cfg=dict(mp_start_method='fork', opencv_num_threads=0),
+ # set distributed parameters
+ dist_cfg=dict(backend='nccl'),
+)
+
+# set visualizer
+visualizer = None
+
+# set log level
+log_level = 'INFO'
+
+# load from which checkpoint
+load_from = None
+
+# whether to resume training from the loaded checkpoint
+resume = False
+
+# Defaults to use random seed and disable `deterministic`
+randomness = dict(seed=None, deterministic=False)
+
+# set log processor
+log_processor = dict(by_epoch=False)
diff --git a/xtuner/configs/custom_dataset/sft/qwen/qwen1_5_7b_chat_qlora_custom_sft_e1.py b/xtuner/configs/custom_dataset/sft/qwen/qwen1_5_7b_chat_qlora_custom_sft_e1.py
new file mode 100644
index 000000000..1098c5ca8
--- /dev/null
+++ b/xtuner/configs/custom_dataset/sft/qwen/qwen1_5_7b_chat_qlora_custom_sft_e1.py
@@ -0,0 +1,226 @@
+# Copyright (c) OpenMMLab. All rights reserved.
+"""Data format:
+
+[{
+ "messages": [
+ { "role": "system", "content": "xxx." },
+ { "role": "user", "content": "xxx." },
+ { "role": "assistant", "content": "xxx.", "loss": false},
+ { "role": "user", "content": "xxx." },
+ { "role": "assistant", "content": "xxx.", "loss": true}
+ ]
+},
+...
+]
+""" # noqa: E501
+import torch
+from datasets import load_dataset
+from mmengine.dataset import DefaultSampler
+from mmengine.hooks import (CheckpointHook, DistSamplerSeedHook, IterTimerHook,
+ LoggerHook, ParamSchedulerHook)
+from mmengine.optim import AmpOptimWrapper, CosineAnnealingLR, LinearLR
+from peft import LoraConfig
+from torch.optim import AdamW
+from transformers import (AutoModelForCausalLM, AutoTokenizer,
+ BitsAndBytesConfig)
+
+from xtuner.dataset import process_hf_dataset
+from xtuner.dataset.collate_fns import default_collate_fn
+from xtuner.dataset.map_fns import openai_map_fn, template_map_fn_factory
+from xtuner.engine.hooks import (DatasetInfoHook, EvaluateChatHook,
+ VarlenAttnArgsToMessageHubHook)
+from xtuner.engine.runner import TrainLoop
+from xtuner.model import SupervisedFinetune
+from xtuner.utils import PROMPT_TEMPLATE
+
+#######################################################################
+# PART 1 Settings #
+#######################################################################
+# Model
+pretrained_model_name_or_path = 'Qwen/Qwen1.5-7B-Chat'
+use_varlen_attn = False
+
+# Data
+data_files = ['/path/to/json/file.json']
+prompt_template = PROMPT_TEMPLATE.qwen_chat
+max_length = 2048
+pack_to_max_length = True
+
+# Scheduler & Optimizer
+batch_size = 1 # per_device
+accumulative_counts = 16 # bs = 1 GPU * 1 batch_size_per_device * 16 acc
+dataloader_num_workers = 0
+max_epochs = 1
+optim_type = AdamW
+lr = 2e-4
+betas = (0.9, 0.999)
+weight_decay = 0
+max_norm = 1 # grad clip
+warmup_ratio = 0.03
+
+# Save
+save_steps = 500
+save_total_limit = 2 # Maximum checkpoints to keep (-1 means unlimited)
+
+# Evaluate the generation performance during the training
+evaluation_freq = 500
+SYSTEM = ''
+evaluation_inputs = [
+ '请给我介绍五个上海的景点', 'Please tell me five scenic spots in Shanghai'
+]
+
+#######################################################################
+# PART 2 Model & Tokenizer #
+#######################################################################
+tokenizer = dict(
+ type=AutoTokenizer.from_pretrained,
+ pretrained_model_name_or_path=pretrained_model_name_or_path,
+ trust_remote_code=True,
+ padding_side='right')
+
+model = dict(
+ type=SupervisedFinetune,
+ use_varlen_attn=use_varlen_attn,
+ llm=dict(
+ type=AutoModelForCausalLM.from_pretrained,
+ pretrained_model_name_or_path=pretrained_model_name_or_path,
+ trust_remote_code=True,
+ torch_dtype=torch.float16,
+ quantization_config=dict(
+ type=BitsAndBytesConfig,
+ load_in_4bit=True,
+ load_in_8bit=False,
+ llm_int8_threshold=6.0,
+ llm_int8_has_fp16_weight=False,
+ bnb_4bit_compute_dtype=torch.float16,
+ bnb_4bit_use_double_quant=True,
+ bnb_4bit_quant_type='nf4')),
+ lora=dict(
+ type=LoraConfig,
+ r=64,
+ lora_alpha=16,
+ lora_dropout=0.1,
+ bias='none',
+ task_type='CAUSAL_LM'))
+
+#######################################################################
+# PART 3 Dataset & Dataloader #
+#######################################################################
+train_dataset = dict(
+ type=process_hf_dataset,
+ dataset=dict(type=load_dataset, path='json', data_files=data_files),
+ tokenizer=tokenizer,
+ max_length=max_length,
+ dataset_map_fn=openai_map_fn,
+ template_map_fn=dict(
+ type=template_map_fn_factory, template=prompt_template),
+ remove_unused_columns=True,
+ shuffle_before_pack=True,
+ pack_to_max_length=pack_to_max_length,
+ use_varlen_attn=use_varlen_attn)
+
+train_dataloader = dict(
+ batch_size=batch_size,
+ num_workers=dataloader_num_workers,
+ dataset=train_dataset,
+ sampler=dict(type=DefaultSampler, shuffle=True),
+ collate_fn=dict(type=default_collate_fn, use_varlen_attn=use_varlen_attn))
+
+#######################################################################
+# PART 4 Scheduler & Optimizer #
+#######################################################################
+# optimizer
+optim_wrapper = dict(
+ type=AmpOptimWrapper,
+ optimizer=dict(
+ type=optim_type, lr=lr, betas=betas, weight_decay=weight_decay),
+ clip_grad=dict(max_norm=max_norm, error_if_nonfinite=False),
+ accumulative_counts=accumulative_counts,
+ loss_scale='dynamic',
+ dtype='float16')
+
+# learning policy
+# More information: https://github.com/open-mmlab/mmengine/blob/main/docs/en/tutorials/param_scheduler.md # noqa: E501
+param_scheduler = [
+ dict(
+ type=LinearLR,
+ start_factor=1e-5,
+ by_epoch=True,
+ begin=0,
+ end=warmup_ratio * max_epochs,
+ convert_to_iter_based=True),
+ dict(
+ type=CosineAnnealingLR,
+ eta_min=0.0,
+ by_epoch=True,
+ begin=warmup_ratio * max_epochs,
+ end=max_epochs,
+ convert_to_iter_based=True)
+]
+
+# train, val, test setting
+train_cfg = dict(type=TrainLoop, max_epochs=max_epochs)
+
+#######################################################################
+# PART 5 Runtime #
+#######################################################################
+# Log the dialogue periodically during the training process, optional
+custom_hooks = [
+ dict(type=DatasetInfoHook, tokenizer=tokenizer),
+ dict(
+ type=EvaluateChatHook,
+ tokenizer=tokenizer,
+ every_n_iters=evaluation_freq,
+ evaluation_inputs=evaluation_inputs,
+ system=SYSTEM,
+ prompt_template=prompt_template)
+]
+
+if use_varlen_attn:
+ custom_hooks += [dict(type=VarlenAttnArgsToMessageHubHook)]
+
+# configure default hooks
+default_hooks = dict(
+ # record the time of every iteration.
+ timer=dict(type=IterTimerHook),
+ # print log every 10 iterations.
+ logger=dict(type=LoggerHook, log_metric_by_epoch=False, interval=10),
+ # enable the parameter scheduler.
+ param_scheduler=dict(type=ParamSchedulerHook),
+ # save checkpoint per `save_steps`.
+ checkpoint=dict(
+ type=CheckpointHook,
+ by_epoch=False,
+ interval=save_steps,
+ max_keep_ckpts=save_total_limit),
+ # set sampler seed in distributed evrionment.
+ sampler_seed=dict(type=DistSamplerSeedHook),
+)
+
+# configure environment
+env_cfg = dict(
+ # whether to enable cudnn benchmark
+ cudnn_benchmark=False,
+ # set multi process parameters
+ mp_cfg=dict(mp_start_method='fork', opencv_num_threads=0),
+ # set distributed parameters
+ dist_cfg=dict(backend='nccl'),
+)
+
+# set visualizer
+visualizer = None
+
+# set log level
+log_level = 'INFO'
+
+# load from which checkpoint
+load_from = None
+
+# whether to resume training from the loaded checkpoint
+resume = False
+
+# Defaults to use random seed and disable `deterministic`
+randomness = dict(seed=None, deterministic=False)
+
+# set log processor
+log_processor = dict(by_epoch=False)
diff --git a/xtuner/configs/custom_dataset/sft/qwen/qwen_1_8b_chat_qlora_custom_sft_e1.py b/xtuner/configs/custom_dataset/sft/qwen/qwen_1_8b_chat_qlora_custom_sft_e1.py
new file mode 100644
index 000000000..2d517e897
--- /dev/null
+++ b/xtuner/configs/custom_dataset/sft/qwen/qwen_1_8b_chat_qlora_custom_sft_e1.py
@@ -0,0 +1,227 @@
+# Copyright (c) OpenMMLab. All rights reserved.
+"""Data format:
+
+[{
+ "messages": [
+ { "role": "system", "content": "xxx." },
+ { "role": "user", "content": "xxx." },
+ { "role": "assistant", "content": "xxx.", "loss": false},
+ { "role": "user", "content": "xxx." },
+ { "role": "assistant", "content": "xxx.", "loss": true}
+ ]
+},
+...
+]
+""" # noqa: E501
+import torch
+from datasets import load_dataset
+from mmengine.dataset import DefaultSampler
+from mmengine.hooks import (CheckpointHook, DistSamplerSeedHook, IterTimerHook,
+ LoggerHook, ParamSchedulerHook)
+from mmengine.optim import AmpOptimWrapper, CosineAnnealingLR, LinearLR
+from peft import LoraConfig
+from torch.optim import AdamW
+from transformers import (AutoModelForCausalLM, AutoTokenizer,
+ BitsAndBytesConfig)
+
+from xtuner.dataset import process_hf_dataset
+from xtuner.dataset.collate_fns import default_collate_fn
+from xtuner.dataset.map_fns import openai_map_fn, template_map_fn_factory
+from xtuner.engine.hooks import (DatasetInfoHook, EvaluateChatHook,
+ VarlenAttnArgsToMessageHubHook)
+from xtuner.engine.runner import TrainLoop
+from xtuner.model import SupervisedFinetune
+from xtuner.utils import PROMPT_TEMPLATE
+
+#######################################################################
+# PART 1 Settings #
+#######################################################################
+# Model
+pretrained_model_name_or_path = 'Qwen/Qwen-1_8B-Chat'
+use_varlen_attn = False
+
+# Data
+data_files = ['/path/to/json/file.json']
+prompt_template = PROMPT_TEMPLATE.qwen_chat
+max_length = 2048
+pack_to_max_length = True
+
+# Scheduler & Optimizer
+batch_size = 1 # per_device
+accumulative_counts = 16 # bs = 1 GPU * 1 batch_size_per_device * 16 acc
+dataloader_num_workers = 0
+max_epochs = 1
+optim_type = AdamW
+lr = 2e-4
+betas = (0.9, 0.999)
+weight_decay = 0
+max_norm = 1 # grad clip
+warmup_ratio = 0.03
+
+# Save
+save_steps = 500
+save_total_limit = 2 # Maximum checkpoints to keep (-1 means unlimited)
+
+# Evaluate the generation performance during the training
+evaluation_freq = 500
+SYSTEM = ''
+evaluation_inputs = [
+ '请给我介绍五个上海的景点', 'Please tell me five scenic spots in Shanghai'
+]
+
+#######################################################################
+# PART 2 Model & Tokenizer #
+#######################################################################
+tokenizer = dict(
+ type=AutoTokenizer.from_pretrained,
+ pretrained_model_name_or_path=pretrained_model_name_or_path,
+ trust_remote_code=True,
+ padding_side='right',
+ eos_token='<|im_end|>')
+
+model = dict(
+ type=SupervisedFinetune,
+ use_varlen_attn=use_varlen_attn,
+ llm=dict(
+ type=AutoModelForCausalLM.from_pretrained,
+ pretrained_model_name_or_path=pretrained_model_name_or_path,
+ trust_remote_code=True,
+ torch_dtype=torch.float16,
+ quantization_config=dict(
+ type=BitsAndBytesConfig,
+ load_in_4bit=True,
+ load_in_8bit=False,
+ llm_int8_threshold=6.0,
+ llm_int8_has_fp16_weight=False,
+ bnb_4bit_compute_dtype=torch.float16,
+ bnb_4bit_use_double_quant=True,
+ bnb_4bit_quant_type='nf4')),
+ lora=dict(
+ type=LoraConfig,
+ r=64,
+ lora_alpha=16,
+ lora_dropout=0.1,
+ bias='none',
+ task_type='CAUSAL_LM'))
+
+#######################################################################
+# PART 3 Dataset & Dataloader #
+#######################################################################
+train_dataset = dict(
+ type=process_hf_dataset,
+ dataset=dict(type=load_dataset, path='json', data_files=data_files),
+ tokenizer=tokenizer,
+ max_length=max_length,
+ dataset_map_fn=openai_map_fn,
+ template_map_fn=dict(
+ type=template_map_fn_factory, template=prompt_template),
+ remove_unused_columns=True,
+ shuffle_before_pack=True,
+ pack_to_max_length=pack_to_max_length,
+ use_varlen_attn=use_varlen_attn)
+
+train_dataloader = dict(
+ batch_size=batch_size,
+ num_workers=dataloader_num_workers,
+ dataset=train_dataset,
+ sampler=dict(type=DefaultSampler, shuffle=True),
+ collate_fn=dict(type=default_collate_fn, use_varlen_attn=use_varlen_attn))
+
+#######################################################################
+# PART 4 Scheduler & Optimizer #
+#######################################################################
+# optimizer
+optim_wrapper = dict(
+ type=AmpOptimWrapper,
+ optimizer=dict(
+ type=optim_type, lr=lr, betas=betas, weight_decay=weight_decay),
+ clip_grad=dict(max_norm=max_norm, error_if_nonfinite=False),
+ accumulative_counts=accumulative_counts,
+ loss_scale='dynamic',
+ dtype='float16')
+
+# learning policy
+# More information: https://github.com/open-mmlab/mmengine/blob/main/docs/en/tutorials/param_scheduler.md # noqa: E501
+param_scheduler = [
+ dict(
+ type=LinearLR,
+ start_factor=1e-5,
+ by_epoch=True,
+ begin=0,
+ end=warmup_ratio * max_epochs,
+ convert_to_iter_based=True),
+ dict(
+ type=CosineAnnealingLR,
+ eta_min=0.0,
+ by_epoch=True,
+ begin=warmup_ratio * max_epochs,
+ end=max_epochs,
+ convert_to_iter_based=True)
+]
+
+# train, val, test setting
+train_cfg = dict(type=TrainLoop, max_epochs=max_epochs)
+
+#######################################################################
+# PART 5 Runtime #
+#######################################################################
+# Log the dialogue periodically during the training process, optional
+custom_hooks = [
+ dict(type=DatasetInfoHook, tokenizer=tokenizer),
+ dict(
+ type=EvaluateChatHook,
+ tokenizer=tokenizer,
+ every_n_iters=evaluation_freq,
+ evaluation_inputs=evaluation_inputs,
+ system=SYSTEM,
+ prompt_template=prompt_template)
+]
+
+if use_varlen_attn:
+ custom_hooks += [dict(type=VarlenAttnArgsToMessageHubHook)]
+
+# configure default hooks
+default_hooks = dict(
+ # record the time of every iteration.
+ timer=dict(type=IterTimerHook),
+ # print log every 10 iterations.
+ logger=dict(type=LoggerHook, log_metric_by_epoch=False, interval=10),
+ # enable the parameter scheduler.
+ param_scheduler=dict(type=ParamSchedulerHook),
+ # save checkpoint per `save_steps`.
+ checkpoint=dict(
+ type=CheckpointHook,
+ by_epoch=False,
+ interval=save_steps,
+ max_keep_ckpts=save_total_limit),
+ # set sampler seed in distributed evrionment.
+ sampler_seed=dict(type=DistSamplerSeedHook),
+)
+
+# configure environment
+env_cfg = dict(
+ # whether to enable cudnn benchmark
+ cudnn_benchmark=False,
+ # set multi process parameters
+ mp_cfg=dict(mp_start_method='fork', opencv_num_threads=0),
+ # set distributed parameters
+ dist_cfg=dict(backend='nccl'),
+)
+
+# set visualizer
+visualizer = None
+
+# set log level
+log_level = 'INFO'
+
+# load from which checkpoint
+load_from = None
+
+# whether to resume training from the loaded checkpoint
+resume = False
+
+# Defaults to use random seed and disable `deterministic`
+randomness = dict(seed=None, deterministic=False)
+
+# set log processor
+log_processor = dict(by_epoch=False)
diff --git a/xtuner/configs/custom_dataset/sft/qwen/qwen_72b_qlora_custom_sft_e1.py b/xtuner/configs/custom_dataset/sft/qwen/qwen_72b_qlora_custom_sft_e1.py
new file mode 100644
index 000000000..e1156a1aa
--- /dev/null
+++ b/xtuner/configs/custom_dataset/sft/qwen/qwen_72b_qlora_custom_sft_e1.py
@@ -0,0 +1,227 @@
+# Copyright (c) OpenMMLab. All rights reserved.
+"""Data format:
+
+[{
+ "messages": [
+ { "role": "system", "content": "xxx." },
+ { "role": "user", "content": "xxx." },
+ { "role": "assistant", "content": "xxx.", "loss": false},
+ { "role": "user", "content": "xxx." },
+ { "role": "assistant", "content": "xxx.", "loss": true}
+ ]
+},
+...
+]
+""" # noqa: E501
+import torch
+from datasets import load_dataset
+from mmengine.dataset import DefaultSampler
+from mmengine.hooks import (CheckpointHook, DistSamplerSeedHook, IterTimerHook,
+ LoggerHook, ParamSchedulerHook)
+from mmengine.optim import AmpOptimWrapper, CosineAnnealingLR, LinearLR
+from peft import LoraConfig
+from torch.optim import AdamW
+from transformers import (AutoModelForCausalLM, AutoTokenizer,
+ BitsAndBytesConfig)
+
+from xtuner.dataset import process_hf_dataset
+from xtuner.dataset.collate_fns import default_collate_fn
+from xtuner.dataset.map_fns import openai_map_fn, template_map_fn_factory
+from xtuner.engine.hooks import (DatasetInfoHook, EvaluateChatHook,
+ VarlenAttnArgsToMessageHubHook)
+from xtuner.engine.runner import TrainLoop
+from xtuner.model import SupervisedFinetune
+from xtuner.utils import PROMPT_TEMPLATE
+
+#######################################################################
+# PART 1 Settings #
+#######################################################################
+# Model
+pretrained_model_name_or_path = 'Qwen/Qwen-72B'
+use_varlen_attn = False
+
+# Data
+data_files = ['/path/to/json/file.json']
+prompt_template = PROMPT_TEMPLATE.default
+max_length = 2048
+pack_to_max_length = True
+
+# Scheduler & Optimizer
+batch_size = 1 # per_device
+accumulative_counts = 16 # bs = 1 GPU * 1 batch_size_per_device * 16 acc
+dataloader_num_workers = 0
+max_epochs = 1
+optim_type = AdamW
+lr = 2e-4
+betas = (0.9, 0.999)
+weight_decay = 0
+max_norm = 1 # grad clip
+warmup_ratio = 0.03
+
+# Save
+save_steps = 500
+save_total_limit = 2 # Maximum checkpoints to keep (-1 means unlimited)
+
+# Evaluate the generation performance during the training
+evaluation_freq = 500
+SYSTEM = ''
+evaluation_inputs = [
+ '请给我介绍五个上海的景点', 'Please tell me five scenic spots in Shanghai'
+]
+
+#######################################################################
+# PART 2 Model & Tokenizer #
+#######################################################################
+tokenizer = dict(
+ type=AutoTokenizer.from_pretrained,
+ pretrained_model_name_or_path=pretrained_model_name_or_path,
+ trust_remote_code=True,
+ padding_side='right',
+ eos_token='<|endoftext|>')
+
+model = dict(
+ type=SupervisedFinetune,
+ use_varlen_attn=use_varlen_attn,
+ llm=dict(
+ type=AutoModelForCausalLM.from_pretrained,
+ pretrained_model_name_or_path=pretrained_model_name_or_path,
+ trust_remote_code=True,
+ torch_dtype=torch.float16,
+ quantization_config=dict(
+ type=BitsAndBytesConfig,
+ load_in_4bit=True,
+ load_in_8bit=False,
+ llm_int8_threshold=6.0,
+ llm_int8_has_fp16_weight=False,
+ bnb_4bit_compute_dtype=torch.float16,
+ bnb_4bit_use_double_quant=True,
+ bnb_4bit_quant_type='nf4')),
+ lora=dict(
+ type=LoraConfig,
+ r=64,
+ lora_alpha=16,
+ lora_dropout=0.1,
+ bias='none',
+ task_type='CAUSAL_LM'))
+
+#######################################################################
+# PART 3 Dataset & Dataloader #
+#######################################################################
+train_dataset = dict(
+ type=process_hf_dataset,
+ dataset=dict(type=load_dataset, path='json', data_files=data_files),
+ tokenizer=tokenizer,
+ max_length=max_length,
+ dataset_map_fn=openai_map_fn,
+ template_map_fn=dict(
+ type=template_map_fn_factory, template=prompt_template),
+ remove_unused_columns=True,
+ shuffle_before_pack=True,
+ pack_to_max_length=pack_to_max_length,
+ use_varlen_attn=use_varlen_attn)
+
+train_dataloader = dict(
+ batch_size=batch_size,
+ num_workers=dataloader_num_workers,
+ dataset=train_dataset,
+ sampler=dict(type=DefaultSampler, shuffle=True),
+ collate_fn=dict(type=default_collate_fn, use_varlen_attn=use_varlen_attn))
+
+#######################################################################
+# PART 4 Scheduler & Optimizer #
+#######################################################################
+# optimizer
+optim_wrapper = dict(
+ type=AmpOptimWrapper,
+ optimizer=dict(
+ type=optim_type, lr=lr, betas=betas, weight_decay=weight_decay),
+ clip_grad=dict(max_norm=max_norm, error_if_nonfinite=False),
+ accumulative_counts=accumulative_counts,
+ loss_scale='dynamic',
+ dtype='float16')
+
+# learning policy
+# More information: https://github.com/open-mmlab/mmengine/blob/main/docs/en/tutorials/param_scheduler.md # noqa: E501
+param_scheduler = [
+ dict(
+ type=LinearLR,
+ start_factor=1e-5,
+ by_epoch=True,
+ begin=0,
+ end=warmup_ratio * max_epochs,
+ convert_to_iter_based=True),
+ dict(
+ type=CosineAnnealingLR,
+ eta_min=0.0,
+ by_epoch=True,
+ begin=warmup_ratio * max_epochs,
+ end=max_epochs,
+ convert_to_iter_based=True)
+]
+
+# train, val, test setting
+train_cfg = dict(type=TrainLoop, max_epochs=max_epochs)
+
+#######################################################################
+# PART 5 Runtime #
+#######################################################################
+# Log the dialogue periodically during the training process, optional
+custom_hooks = [
+ dict(type=DatasetInfoHook, tokenizer=tokenizer),
+ dict(
+ type=EvaluateChatHook,
+ tokenizer=tokenizer,
+ every_n_iters=evaluation_freq,
+ evaluation_inputs=evaluation_inputs,
+ system=SYSTEM,
+ prompt_template=prompt_template)
+]
+
+if use_varlen_attn:
+ custom_hooks += [dict(type=VarlenAttnArgsToMessageHubHook)]
+
+# configure default hooks
+default_hooks = dict(
+ # record the time of every iteration.
+ timer=dict(type=IterTimerHook),
+ # print log every 10 iterations.
+ logger=dict(type=LoggerHook, log_metric_by_epoch=False, interval=10),
+ # enable the parameter scheduler.
+ param_scheduler=dict(type=ParamSchedulerHook),
+ # save checkpoint per `save_steps`.
+ checkpoint=dict(
+ type=CheckpointHook,
+ by_epoch=False,
+ interval=save_steps,
+ max_keep_ckpts=save_total_limit),
+ # set sampler seed in distributed evrionment.
+ sampler_seed=dict(type=DistSamplerSeedHook),
+)
+
+# configure environment
+env_cfg = dict(
+ # whether to enable cudnn benchmark
+ cudnn_benchmark=False,
+ # set multi process parameters
+ mp_cfg=dict(mp_start_method='fork', opencv_num_threads=0),
+ # set distributed parameters
+ dist_cfg=dict(backend='nccl'),
+)
+
+# set visualizer
+visualizer = None
+
+# set log level
+log_level = 'INFO'
+
+# load from which checkpoint
+load_from = None
+
+# whether to resume training from the loaded checkpoint
+resume = False
+
+# Defaults to use random seed and disable `deterministic`
+randomness = dict(seed=None, deterministic=False)
+
+# set log processor
+log_processor = dict(by_epoch=False)
diff --git a/xtuner/configs/custom_dataset/sft/qwen/qwen_7b_chat_qlora_custom_sft_e1.py b/xtuner/configs/custom_dataset/sft/qwen/qwen_7b_chat_qlora_custom_sft_e1.py
new file mode 100644
index 000000000..b6fcaacba
--- /dev/null
+++ b/xtuner/configs/custom_dataset/sft/qwen/qwen_7b_chat_qlora_custom_sft_e1.py
@@ -0,0 +1,227 @@
+# Copyright (c) OpenMMLab. All rights reserved.
+"""Data format:
+
+[{
+ "messages": [
+ { "role": "system", "content": "xxx." },
+ { "role": "user", "content": "xxx." },
+ { "role": "assistant", "content": "xxx.", "loss": false},
+ { "role": "user", "content": "xxx." },
+ { "role": "assistant", "content": "xxx.", "loss": true}
+ ]
+},
+...
+]
+""" # noqa: E501
+import torch
+from datasets import load_dataset
+from mmengine.dataset import DefaultSampler
+from mmengine.hooks import (CheckpointHook, DistSamplerSeedHook, IterTimerHook,
+ LoggerHook, ParamSchedulerHook)
+from mmengine.optim import AmpOptimWrapper, CosineAnnealingLR, LinearLR
+from peft import LoraConfig
+from torch.optim import AdamW
+from transformers import (AutoModelForCausalLM, AutoTokenizer,
+ BitsAndBytesConfig)
+
+from xtuner.dataset import process_hf_dataset
+from xtuner.dataset.collate_fns import default_collate_fn
+from xtuner.dataset.map_fns import openai_map_fn, template_map_fn_factory
+from xtuner.engine.hooks import (DatasetInfoHook, EvaluateChatHook,
+ VarlenAttnArgsToMessageHubHook)
+from xtuner.engine.runner import TrainLoop
+from xtuner.model import SupervisedFinetune
+from xtuner.utils import PROMPT_TEMPLATE
+
+#######################################################################
+# PART 1 Settings #
+#######################################################################
+# Model
+pretrained_model_name_or_path = 'Qwen/Qwen-7B-Chat'
+use_varlen_attn = False
+
+# Data
+data_files = ['/path/to/json/file.json']
+prompt_template = PROMPT_TEMPLATE.qwen_chat
+max_length = 2048
+pack_to_max_length = True
+
+# Scheduler & Optimizer
+batch_size = 1 # per_device
+accumulative_counts = 16 # bs = 1 GPU * 1 batch_size_per_device * 16 acc
+dataloader_num_workers = 0
+max_epochs = 1
+optim_type = AdamW
+lr = 2e-4
+betas = (0.9, 0.999)
+weight_decay = 0
+max_norm = 1 # grad clip
+warmup_ratio = 0.03
+
+# Save
+save_steps = 500
+save_total_limit = 2 # Maximum checkpoints to keep (-1 means unlimited)
+
+# Evaluate the generation performance during the training
+evaluation_freq = 500
+SYSTEM = ''
+evaluation_inputs = [
+ '请给我介绍五个上海的景点', 'Please tell me five scenic spots in Shanghai'
+]
+
+#######################################################################
+# PART 2 Model & Tokenizer #
+#######################################################################
+tokenizer = dict(
+ type=AutoTokenizer.from_pretrained,
+ pretrained_model_name_or_path=pretrained_model_name_or_path,
+ trust_remote_code=True,
+ padding_side='right',
+ eos_token='<|im_end|>')
+
+model = dict(
+ type=SupervisedFinetune,
+ use_varlen_attn=use_varlen_attn,
+ llm=dict(
+ type=AutoModelForCausalLM.from_pretrained,
+ pretrained_model_name_or_path=pretrained_model_name_or_path,
+ trust_remote_code=True,
+ torch_dtype=torch.float16,
+ quantization_config=dict(
+ type=BitsAndBytesConfig,
+ load_in_4bit=True,
+ load_in_8bit=False,
+ llm_int8_threshold=6.0,
+ llm_int8_has_fp16_weight=False,
+ bnb_4bit_compute_dtype=torch.float16,
+ bnb_4bit_use_double_quant=True,
+ bnb_4bit_quant_type='nf4')),
+ lora=dict(
+ type=LoraConfig,
+ r=64,
+ lora_alpha=16,
+ lora_dropout=0.1,
+ bias='none',
+ task_type='CAUSAL_LM'))
+
+#######################################################################
+# PART 3 Dataset & Dataloader #
+#######################################################################
+train_dataset = dict(
+ type=process_hf_dataset,
+ dataset=dict(type=load_dataset, path='json', data_files=data_files),
+ tokenizer=tokenizer,
+ max_length=max_length,
+ dataset_map_fn=openai_map_fn,
+ template_map_fn=dict(
+ type=template_map_fn_factory, template=prompt_template),
+ remove_unused_columns=True,
+ shuffle_before_pack=True,
+ pack_to_max_length=pack_to_max_length,
+ use_varlen_attn=use_varlen_attn)
+
+train_dataloader = dict(
+ batch_size=batch_size,
+ num_workers=dataloader_num_workers,
+ dataset=train_dataset,
+ sampler=dict(type=DefaultSampler, shuffle=True),
+ collate_fn=dict(type=default_collate_fn, use_varlen_attn=use_varlen_attn))
+
+#######################################################################
+# PART 4 Scheduler & Optimizer #
+#######################################################################
+# optimizer
+optim_wrapper = dict(
+ type=AmpOptimWrapper,
+ optimizer=dict(
+ type=optim_type, lr=lr, betas=betas, weight_decay=weight_decay),
+ clip_grad=dict(max_norm=max_norm, error_if_nonfinite=False),
+ accumulative_counts=accumulative_counts,
+ loss_scale='dynamic',
+ dtype='float16')
+
+# learning policy
+# More information: https://github.com/open-mmlab/mmengine/blob/main/docs/en/tutorials/param_scheduler.md # noqa: E501
+param_scheduler = [
+ dict(
+ type=LinearLR,
+ start_factor=1e-5,
+ by_epoch=True,
+ begin=0,
+ end=warmup_ratio * max_epochs,
+ convert_to_iter_based=True),
+ dict(
+ type=CosineAnnealingLR,
+ eta_min=0.0,
+ by_epoch=True,
+ begin=warmup_ratio * max_epochs,
+ end=max_epochs,
+ convert_to_iter_based=True)
+]
+
+# train, val, test setting
+train_cfg = dict(type=TrainLoop, max_epochs=max_epochs)
+
+#######################################################################
+# PART 5 Runtime #
+#######################################################################
+# Log the dialogue periodically during the training process, optional
+custom_hooks = [
+ dict(type=DatasetInfoHook, tokenizer=tokenizer),
+ dict(
+ type=EvaluateChatHook,
+ tokenizer=tokenizer,
+ every_n_iters=evaluation_freq,
+ evaluation_inputs=evaluation_inputs,
+ system=SYSTEM,
+ prompt_template=prompt_template)
+]
+
+if use_varlen_attn:
+ custom_hooks += [dict(type=VarlenAttnArgsToMessageHubHook)]
+
+# configure default hooks
+default_hooks = dict(
+ # record the time of every iteration.
+ timer=dict(type=IterTimerHook),
+ # print log every 10 iterations.
+ logger=dict(type=LoggerHook, log_metric_by_epoch=False, interval=10),
+ # enable the parameter scheduler.
+ param_scheduler=dict(type=ParamSchedulerHook),
+ # save checkpoint per `save_steps`.
+ checkpoint=dict(
+ type=CheckpointHook,
+ by_epoch=False,
+ interval=save_steps,
+ max_keep_ckpts=save_total_limit),
+ # set sampler seed in distributed evrionment.
+ sampler_seed=dict(type=DistSamplerSeedHook),
+)
+
+# configure environment
+env_cfg = dict(
+ # whether to enable cudnn benchmark
+ cudnn_benchmark=False,
+ # set multi process parameters
+ mp_cfg=dict(mp_start_method='fork', opencv_num_threads=0),
+ # set distributed parameters
+ dist_cfg=dict(backend='nccl'),
+)
+
+# set visualizer
+visualizer = None
+
+# set log level
+log_level = 'INFO'
+
+# load from which checkpoint
+load_from = None
+
+# whether to resume training from the loaded checkpoint
+resume = False
+
+# Defaults to use random seed and disable `deterministic`
+randomness = dict(seed=None, deterministic=False)
+
+# set log processor
+log_processor = dict(by_epoch=False)
diff --git a/xtuner/configs/custom_dataset/sft/starcoder/starcoder_qlora_custom_sft_e1.py b/xtuner/configs/custom_dataset/sft/starcoder/starcoder_qlora_custom_sft_e1.py
new file mode 100644
index 000000000..d79484dcf
--- /dev/null
+++ b/xtuner/configs/custom_dataset/sft/starcoder/starcoder_qlora_custom_sft_e1.py
@@ -0,0 +1,230 @@
+# Copyright (c) OpenMMLab. All rights reserved.
+"""Data format:
+
+[{
+ "messages": [
+ { "role": "system", "content": "xxx." },
+ { "role": "user", "content": "xxx." },
+ { "role": "assistant", "content": "xxx.", "loss": false},
+ { "role": "user", "content": "xxx." },
+ { "role": "assistant", "content": "xxx.", "loss": true}
+ ]
+},
+...
+]
+""" # noqa: E501
+import torch
+from datasets import load_dataset
+from mmengine.dataset import DefaultSampler
+from mmengine.hooks import (CheckpointHook, DistSamplerSeedHook, IterTimerHook,
+ LoggerHook, ParamSchedulerHook)
+from mmengine.optim import AmpOptimWrapper, CosineAnnealingLR, LinearLR
+from peft import LoraConfig
+from torch.optim import AdamW
+from transformers import (AutoModelForCausalLM, AutoTokenizer,
+ BitsAndBytesConfig)
+
+from xtuner.dataset import process_hf_dataset
+from xtuner.dataset.collate_fns import default_collate_fn
+from xtuner.dataset.map_fns import openai_map_fn, template_map_fn_factory
+from xtuner.engine.hooks import (DatasetInfoHook, EvaluateChatHook,
+ VarlenAttnArgsToMessageHubHook)
+from xtuner.engine.runner import TrainLoop
+from xtuner.model import SupervisedFinetune
+from xtuner.utils import PROMPT_TEMPLATE
+
+#######################################################################
+# PART 1 Settings #
+#######################################################################
+# Model
+pretrained_model_name_or_path = 'bigcode/starcoder'
+use_varlen_attn = False
+
+# Data
+data_files = ['/path/to/json/file.json']
+prompt_template = PROMPT_TEMPLATE.default
+max_length = 2048
+# randomly select 20000 samples from the original dataset
+max_dataset_length = 20000
+pack_to_max_length = True
+
+# Scheduler & Optimizer
+batch_size = 1 # per_device
+accumulative_counts = 16 # bs = 1 GPU * 1 batch_size_per_device * 16 acc
+dataloader_num_workers = 0
+max_epochs = 1
+optim_type = AdamW
+lr = 1e-4
+betas = (0.9, 0.999)
+weight_decay = 0.05
+max_norm = 1 # grad clip
+warmup_ratio = 0.03
+
+# Save
+save_steps = 500
+save_total_limit = 2 # Maximum checkpoints to keep (-1 means unlimited)
+
+# Evaluate the generation performance during the training
+evaluation_freq = 200
+SYSTEM = ''
+evaluation_inputs = [
+ 'from typing import List def has_close_elements(numbers: List[float], threshold: float) -> bool: """ Check if in given list of numbers, are any two numbers closer to each other than given threshold. >>> has_close_elements([1.0, 2.0, 3.0], 0.5) False >>> has_close_elements([1.0, 2.8, 3.0, 4.0, 5.0, 2.0], 0.3) True """' # noqa: E501
+]
+
+#######################################################################
+# PART 2 Model & Tokenizer #
+#######################################################################
+tokenizer = dict(
+ type=AutoTokenizer.from_pretrained,
+ pretrained_model_name_or_path=pretrained_model_name_or_path,
+ trust_remote_code=True,
+ padding_side='right')
+
+model = dict(
+ type=SupervisedFinetune,
+ use_varlen_attn=use_varlen_attn,
+ llm=dict(
+ type=AutoModelForCausalLM.from_pretrained,
+ pretrained_model_name_or_path=pretrained_model_name_or_path,
+ trust_remote_code=True,
+ torch_dtype=torch.float16,
+ quantization_config=dict(
+ type=BitsAndBytesConfig,
+ load_in_4bit=True,
+ load_in_8bit=False,
+ llm_int8_threshold=6.0,
+ llm_int8_has_fp16_weight=False,
+ bnb_4bit_compute_dtype=torch.float16,
+ bnb_4bit_use_double_quant=True,
+ bnb_4bit_quant_type='nf4')),
+ lora=dict(
+ type=LoraConfig,
+ r=16,
+ lora_alpha=32,
+ lora_dropout=0.05,
+ bias='none',
+ target_modules=['c_proj', 'c_attn', 'q_attn'],
+ task_type='CAUSAL_LM'))
+
+#######################################################################
+# PART 3 Dataset & Dataloader #
+#######################################################################
+train_dataset = dict(
+ type=process_hf_dataset,
+ dataset=dict(type=load_dataset, path='json', data_files=data_files),
+ tokenizer=tokenizer,
+ max_length=max_length,
+ dataset_map_fn=openai_map_fn,
+ template_map_fn=dict(
+ type=template_map_fn_factory, template=prompt_template),
+ max_dataset_length=max_dataset_length,
+ remove_unused_columns=True,
+ shuffle_before_pack=True,
+ pack_to_max_length=pack_to_max_length,
+ use_varlen_attn=use_varlen_attn)
+
+train_dataloader = dict(
+ batch_size=batch_size,
+ num_workers=dataloader_num_workers,
+ dataset=train_dataset,
+ sampler=dict(type=DefaultSampler, shuffle=True),
+ collate_fn=dict(type=default_collate_fn, use_varlen_attn=use_varlen_attn))
+
+#######################################################################
+# PART 4 Scheduler & Optimizer #
+#######################################################################
+# optimizer
+optim_wrapper = dict(
+ type=AmpOptimWrapper,
+ optimizer=dict(
+ type=optim_type, lr=lr, betas=betas, weight_decay=weight_decay),
+ clip_grad=dict(max_norm=max_norm, error_if_nonfinite=False),
+ accumulative_counts=accumulative_counts,
+ loss_scale='dynamic',
+ dtype='float16')
+
+# learning policy
+# More information: https://github.com/open-mmlab/mmengine/blob/main/docs/en/tutorials/param_scheduler.md # noqa: E501
+param_scheduler = [
+ dict(
+ type=LinearLR,
+ start_factor=1e-5,
+ by_epoch=True,
+ begin=0,
+ end=warmup_ratio * max_epochs,
+ convert_to_iter_based=True),
+ dict(
+ type=CosineAnnealingLR,
+ eta_min=0.0,
+ by_epoch=True,
+ begin=warmup_ratio * max_epochs,
+ end=max_epochs,
+ convert_to_iter_based=True)
+]
+
+# train, val, test setting
+train_cfg = dict(type=TrainLoop, max_epochs=max_epochs)
+
+#######################################################################
+# PART 5 Runtime #
+#######################################################################
+# Log the dialogue periodically during the training process, optional
+custom_hooks = [
+ dict(type=DatasetInfoHook, tokenizer=tokenizer),
+ dict(
+ type=EvaluateChatHook,
+ tokenizer=tokenizer,
+ every_n_iters=evaluation_freq,
+ evaluation_inputs=evaluation_inputs,
+ system=SYSTEM,
+ prompt_template=prompt_template)
+]
+
+if use_varlen_attn:
+ custom_hooks += [dict(type=VarlenAttnArgsToMessageHubHook)]
+
+# configure default hooks
+default_hooks = dict(
+ # record the time of every iteration.
+ timer=dict(type=IterTimerHook),
+ # print log every 10 iterations.
+ logger=dict(type=LoggerHook, log_metric_by_epoch=False, interval=10),
+ # enable the parameter scheduler.
+ param_scheduler=dict(type=ParamSchedulerHook),
+ # save checkpoint per `save_steps`.
+ checkpoint=dict(
+ type=CheckpointHook,
+ by_epoch=False,
+ interval=save_steps,
+ max_keep_ckpts=save_total_limit),
+ # set sampler seed in distributed evrionment.
+ sampler_seed=dict(type=DistSamplerSeedHook),
+)
+
+# configure environment
+env_cfg = dict(
+ # whether to enable cudnn benchmark
+ cudnn_benchmark=False,
+ # set multi process parameters
+ mp_cfg=dict(mp_start_method='fork', opencv_num_threads=0),
+ # set distributed parameters
+ dist_cfg=dict(backend='nccl'),
+)
+
+# set visualizer
+visualizer = None
+
+# set log level
+log_level = 'INFO'
+
+# load from which checkpoint
+load_from = None
+
+# whether to resume training from the loaded checkpoint
+resume = False
+
+# Defaults to use random seed and disable `deterministic`
+randomness = dict(seed=None, deterministic=False)
+
+# set log processor
+log_processor = dict(by_epoch=False)
diff --git a/xtuner/configs/custom_dataset/sft/yi/yi_34b_qlora_custom_sft_e1.py b/xtuner/configs/custom_dataset/sft/yi/yi_34b_qlora_custom_sft_e1.py
new file mode 100644
index 000000000..4906ab5f7
--- /dev/null
+++ b/xtuner/configs/custom_dataset/sft/yi/yi_34b_qlora_custom_sft_e1.py
@@ -0,0 +1,226 @@
+# Copyright (c) OpenMMLab. All rights reserved.
+"""Data format:
+
+[{
+ "messages": [
+ { "role": "system", "content": "xxx." },
+ { "role": "user", "content": "xxx." },
+ { "role": "assistant", "content": "xxx.", "loss": false},
+ { "role": "user", "content": "xxx." },
+ { "role": "assistant", "content": "xxx.", "loss": true}
+ ]
+},
+...
+]
+""" # noqa: E501
+import torch
+from datasets import load_dataset
+from mmengine.dataset import DefaultSampler
+from mmengine.hooks import (CheckpointHook, DistSamplerSeedHook, IterTimerHook,
+ LoggerHook, ParamSchedulerHook)
+from mmengine.optim import AmpOptimWrapper, CosineAnnealingLR, LinearLR
+from peft import LoraConfig
+from torch.optim import AdamW
+from transformers import (AutoModelForCausalLM, AutoTokenizer,
+ BitsAndBytesConfig)
+
+from xtuner.dataset import process_hf_dataset
+from xtuner.dataset.collate_fns import default_collate_fn
+from xtuner.dataset.map_fns import openai_map_fn, template_map_fn_factory
+from xtuner.engine.hooks import (DatasetInfoHook, EvaluateChatHook,
+ VarlenAttnArgsToMessageHubHook)
+from xtuner.engine.runner import TrainLoop
+from xtuner.model import SupervisedFinetune
+from xtuner.utils import PROMPT_TEMPLATE
+
+#######################################################################
+# PART 1 Settings #
+#######################################################################
+# Model
+pretrained_model_name_or_path = '01-ai/Yi-34B'
+use_varlen_attn = False
+
+# Data
+data_files = ['/path/to/json/file.json']
+prompt_template = PROMPT_TEMPLATE.default
+max_length = 2048
+pack_to_max_length = True
+
+# Scheduler & Optimizer
+batch_size = 1 # per_device
+accumulative_counts = 16 # bs = 1 GPU * 1 batch_size_per_device * 16 acc
+dataloader_num_workers = 0
+max_epochs = 1
+optim_type = AdamW
+lr = 2e-4
+betas = (0.9, 0.999)
+weight_decay = 0
+max_norm = 1 # grad clip
+warmup_ratio = 0.03
+
+# Save
+save_steps = 500
+save_total_limit = 2 # Maximum checkpoints to keep (-1 means unlimited)
+
+# Evaluate the generation performance during the training
+evaluation_freq = 500
+SYSTEM = ''
+evaluation_inputs = [
+ '请给我介绍五个上海的景点', 'Please tell me five scenic spots in Shanghai'
+]
+
+#######################################################################
+# PART 2 Model & Tokenizer #
+#######################################################################
+tokenizer = dict(
+ type=AutoTokenizer.from_pretrained,
+ pretrained_model_name_or_path=pretrained_model_name_or_path,
+ trust_remote_code=True,
+ padding_side='right')
+
+model = dict(
+ type=SupervisedFinetune,
+ use_varlen_attn=use_varlen_attn,
+ llm=dict(
+ type=AutoModelForCausalLM.from_pretrained,
+ pretrained_model_name_or_path=pretrained_model_name_or_path,
+ trust_remote_code=True,
+ torch_dtype=torch.float16,
+ quantization_config=dict(
+ type=BitsAndBytesConfig,
+ load_in_4bit=True,
+ load_in_8bit=False,
+ llm_int8_threshold=6.0,
+ llm_int8_has_fp16_weight=False,
+ bnb_4bit_compute_dtype=torch.float16,
+ bnb_4bit_use_double_quant=True,
+ bnb_4bit_quant_type='nf4')),
+ lora=dict(
+ type=LoraConfig,
+ r=64,
+ lora_alpha=16,
+ lora_dropout=0.1,
+ bias='none',
+ task_type='CAUSAL_LM'))
+
+#######################################################################
+# PART 3 Dataset & Dataloader #
+#######################################################################
+train_dataset = dict(
+ type=process_hf_dataset,
+ dataset=dict(type=load_dataset, path='json', data_files=data_files),
+ tokenizer=tokenizer,
+ max_length=max_length,
+ dataset_map_fn=openai_map_fn,
+ template_map_fn=dict(
+ type=template_map_fn_factory, template=prompt_template),
+ remove_unused_columns=True,
+ shuffle_before_pack=True,
+ pack_to_max_length=pack_to_max_length,
+ use_varlen_attn=use_varlen_attn)
+
+train_dataloader = dict(
+ batch_size=batch_size,
+ num_workers=dataloader_num_workers,
+ dataset=train_dataset,
+ sampler=dict(type=DefaultSampler, shuffle=True),
+ collate_fn=dict(type=default_collate_fn, use_varlen_attn=use_varlen_attn))
+
+#######################################################################
+# PART 4 Scheduler & Optimizer #
+#######################################################################
+# optimizer
+optim_wrapper = dict(
+ type=AmpOptimWrapper,
+ optimizer=dict(
+ type=optim_type, lr=lr, betas=betas, weight_decay=weight_decay),
+ clip_grad=dict(max_norm=max_norm, error_if_nonfinite=False),
+ accumulative_counts=accumulative_counts,
+ loss_scale='dynamic',
+ dtype='float16')
+
+# learning policy
+# More information: https://github.com/open-mmlab/mmengine/blob/main/docs/en/tutorials/param_scheduler.md # noqa: E501
+param_scheduler = [
+ dict(
+ type=LinearLR,
+ start_factor=1e-5,
+ by_epoch=True,
+ begin=0,
+ end=warmup_ratio * max_epochs,
+ convert_to_iter_based=True),
+ dict(
+ type=CosineAnnealingLR,
+ eta_min=0.0,
+ by_epoch=True,
+ begin=warmup_ratio * max_epochs,
+ end=max_epochs,
+ convert_to_iter_based=True)
+]
+
+# train, val, test setting
+train_cfg = dict(type=TrainLoop, max_epochs=max_epochs)
+
+#######################################################################
+# PART 5 Runtime #
+#######################################################################
+# Log the dialogue periodically during the training process, optional
+custom_hooks = [
+ dict(type=DatasetInfoHook, tokenizer=tokenizer),
+ dict(
+ type=EvaluateChatHook,
+ tokenizer=tokenizer,
+ every_n_iters=evaluation_freq,
+ evaluation_inputs=evaluation_inputs,
+ system=SYSTEM,
+ prompt_template=prompt_template)
+]
+
+if use_varlen_attn:
+ custom_hooks += [dict(type=VarlenAttnArgsToMessageHubHook)]
+
+# configure default hooks
+default_hooks = dict(
+ # record the time of every iteration.
+ timer=dict(type=IterTimerHook),
+ # print log every 10 iterations.
+ logger=dict(type=LoggerHook, log_metric_by_epoch=False, interval=10),
+ # enable the parameter scheduler.
+ param_scheduler=dict(type=ParamSchedulerHook),
+ # save checkpoint per `save_steps`.
+ checkpoint=dict(
+ type=CheckpointHook,
+ by_epoch=False,
+ interval=save_steps,
+ max_keep_ckpts=save_total_limit),
+ # set sampler seed in distributed evrionment.
+ sampler_seed=dict(type=DistSamplerSeedHook),
+)
+
+# configure environment
+env_cfg = dict(
+ # whether to enable cudnn benchmark
+ cudnn_benchmark=False,
+ # set multi process parameters
+ mp_cfg=dict(mp_start_method='fork', opencv_num_threads=0),
+ # set distributed parameters
+ dist_cfg=dict(backend='nccl'),
+)
+
+# set visualizer
+visualizer = None
+
+# set log level
+log_level = 'INFO'
+
+# load from which checkpoint
+load_from = None
+
+# whether to resume training from the loaded checkpoint
+resume = False
+
+# Defaults to use random seed and disable `deterministic`
+randomness = dict(seed=None, deterministic=False)
+
+# set log processor
+log_processor = dict(by_epoch=False)
diff --git a/xtuner/configs/custom_dataset/sft/yi/yi_6b_qlora_custom_sft_e1.py b/xtuner/configs/custom_dataset/sft/yi/yi_6b_qlora_custom_sft_e1.py
new file mode 100644
index 000000000..96a684a22
--- /dev/null
+++ b/xtuner/configs/custom_dataset/sft/yi/yi_6b_qlora_custom_sft_e1.py
@@ -0,0 +1,226 @@
+# Copyright (c) OpenMMLab. All rights reserved.
+"""Data format:
+
+[{
+ "messages": [
+ { "role": "system", "content": "xxx." },
+ { "role": "user", "content": "xxx." },
+ { "role": "assistant", "content": "xxx.", "loss": false},
+ { "role": "user", "content": "xxx." },
+ { "role": "assistant", "content": "xxx.", "loss": true}
+ ]
+},
+...
+]
+""" # noqa: E501
+import torch
+from datasets import load_dataset
+from mmengine.dataset import DefaultSampler
+from mmengine.hooks import (CheckpointHook, DistSamplerSeedHook, IterTimerHook,
+ LoggerHook, ParamSchedulerHook)
+from mmengine.optim import AmpOptimWrapper, CosineAnnealingLR, LinearLR
+from peft import LoraConfig
+from torch.optim import AdamW
+from transformers import (AutoModelForCausalLM, AutoTokenizer,
+ BitsAndBytesConfig)
+
+from xtuner.dataset import process_hf_dataset
+from xtuner.dataset.collate_fns import default_collate_fn
+from xtuner.dataset.map_fns import openai_map_fn, template_map_fn_factory
+from xtuner.engine.hooks import (DatasetInfoHook, EvaluateChatHook,
+ VarlenAttnArgsToMessageHubHook)
+from xtuner.engine.runner import TrainLoop
+from xtuner.model import SupervisedFinetune
+from xtuner.utils import PROMPT_TEMPLATE
+
+#######################################################################
+# PART 1 Settings #
+#######################################################################
+# Model
+pretrained_model_name_or_path = '01-ai/Yi-6B'
+use_varlen_attn = False
+
+# Data
+data_files = ['/path/to/json/file.json']
+prompt_template = PROMPT_TEMPLATE.default
+max_length = 2048
+pack_to_max_length = True
+
+# Scheduler & Optimizer
+batch_size = 1 # per_device
+accumulative_counts = 16 # bs = 1 GPU * 1 batch_size_per_device * 16 acc
+dataloader_num_workers = 0
+max_epochs = 1
+optim_type = AdamW
+lr = 2e-4
+betas = (0.9, 0.999)
+weight_decay = 0
+max_norm = 1 # grad clip
+warmup_ratio = 0.03
+
+# Save
+save_steps = 500
+save_total_limit = 2 # Maximum checkpoints to keep (-1 means unlimited)
+
+# Evaluate the generation performance during the training
+evaluation_freq = 500
+SYSTEM = ''
+evaluation_inputs = [
+ '请给我介绍五个上海的景点', 'Please tell me five scenic spots in Shanghai'
+]
+
+#######################################################################
+# PART 2 Model & Tokenizer #
+#######################################################################
+tokenizer = dict(
+ type=AutoTokenizer.from_pretrained,
+ pretrained_model_name_or_path=pretrained_model_name_or_path,
+ trust_remote_code=True,
+ padding_side='right')
+
+model = dict(
+ type=SupervisedFinetune,
+ use_varlen_attn=use_varlen_attn,
+ llm=dict(
+ type=AutoModelForCausalLM.from_pretrained,
+ pretrained_model_name_or_path=pretrained_model_name_or_path,
+ trust_remote_code=True,
+ torch_dtype=torch.float16,
+ quantization_config=dict(
+ type=BitsAndBytesConfig,
+ load_in_4bit=True,
+ load_in_8bit=False,
+ llm_int8_threshold=6.0,
+ llm_int8_has_fp16_weight=False,
+ bnb_4bit_compute_dtype=torch.float16,
+ bnb_4bit_use_double_quant=True,
+ bnb_4bit_quant_type='nf4')),
+ lora=dict(
+ type=LoraConfig,
+ r=64,
+ lora_alpha=16,
+ lora_dropout=0.1,
+ bias='none',
+ task_type='CAUSAL_LM'))
+
+#######################################################################
+# PART 3 Dataset & Dataloader #
+#######################################################################
+train_dataset = dict(
+ type=process_hf_dataset,
+ dataset=dict(type=load_dataset, path='json', data_files=data_files),
+ tokenizer=tokenizer,
+ max_length=max_length,
+ dataset_map_fn=openai_map_fn,
+ template_map_fn=dict(
+ type=template_map_fn_factory, template=prompt_template),
+ remove_unused_columns=True,
+ shuffle_before_pack=True,
+ pack_to_max_length=pack_to_max_length,
+ use_varlen_attn=use_varlen_attn)
+
+train_dataloader = dict(
+ batch_size=batch_size,
+ num_workers=dataloader_num_workers,
+ dataset=train_dataset,
+ sampler=dict(type=DefaultSampler, shuffle=True),
+ collate_fn=dict(type=default_collate_fn, use_varlen_attn=use_varlen_attn))
+
+#######################################################################
+# PART 4 Scheduler & Optimizer #
+#######################################################################
+# optimizer
+optim_wrapper = dict(
+ type=AmpOptimWrapper,
+ optimizer=dict(
+ type=optim_type, lr=lr, betas=betas, weight_decay=weight_decay),
+ clip_grad=dict(max_norm=max_norm, error_if_nonfinite=False),
+ accumulative_counts=accumulative_counts,
+ loss_scale='dynamic',
+ dtype='float16')
+
+# learning policy
+# More information: https://github.com/open-mmlab/mmengine/blob/main/docs/en/tutorials/param_scheduler.md # noqa: E501
+param_scheduler = [
+ dict(
+ type=LinearLR,
+ start_factor=1e-5,
+ by_epoch=True,
+ begin=0,
+ end=warmup_ratio * max_epochs,
+ convert_to_iter_based=True),
+ dict(
+ type=CosineAnnealingLR,
+ eta_min=0.0,
+ by_epoch=True,
+ begin=warmup_ratio * max_epochs,
+ end=max_epochs,
+ convert_to_iter_based=True)
+]
+
+# train, val, test setting
+train_cfg = dict(type=TrainLoop, max_epochs=max_epochs)
+
+#######################################################################
+# PART 5 Runtime #
+#######################################################################
+# Log the dialogue periodically during the training process, optional
+custom_hooks = [
+ dict(type=DatasetInfoHook, tokenizer=tokenizer),
+ dict(
+ type=EvaluateChatHook,
+ tokenizer=tokenizer,
+ every_n_iters=evaluation_freq,
+ evaluation_inputs=evaluation_inputs,
+ system=SYSTEM,
+ prompt_template=prompt_template)
+]
+
+if use_varlen_attn:
+ custom_hooks += [dict(type=VarlenAttnArgsToMessageHubHook)]
+
+# configure default hooks
+default_hooks = dict(
+ # record the time of every iteration.
+ timer=dict(type=IterTimerHook),
+ # print log every 10 iterations.
+ logger=dict(type=LoggerHook, log_metric_by_epoch=False, interval=10),
+ # enable the parameter scheduler.
+ param_scheduler=dict(type=ParamSchedulerHook),
+ # save checkpoint per `save_steps`.
+ checkpoint=dict(
+ type=CheckpointHook,
+ by_epoch=False,
+ interval=save_steps,
+ max_keep_ckpts=save_total_limit),
+ # set sampler seed in distributed evrionment.
+ sampler_seed=dict(type=DistSamplerSeedHook),
+)
+
+# configure environment
+env_cfg = dict(
+ # whether to enable cudnn benchmark
+ cudnn_benchmark=False,
+ # set multi process parameters
+ mp_cfg=dict(mp_start_method='fork', opencv_num_threads=0),
+ # set distributed parameters
+ dist_cfg=dict(backend='nccl'),
+)
+
+# set visualizer
+visualizer = None
+
+# set log level
+log_level = 'INFO'
+
+# load from which checkpoint
+load_from = None
+
+# whether to resume training from the loaded checkpoint
+resume = False
+
+# Defaults to use random seed and disable `deterministic`
+randomness = dict(seed=None, deterministic=False)
+
+# set log processor
+log_processor = dict(by_epoch=False)
diff --git a/xtuner/configs/custom_dataset/sft/zephyr/zephyr_7b_beta_qlora_custom_sft_e1.py b/xtuner/configs/custom_dataset/sft/zephyr/zephyr_7b_beta_qlora_custom_sft_e1.py
new file mode 100644
index 000000000..b2349c2da
--- /dev/null
+++ b/xtuner/configs/custom_dataset/sft/zephyr/zephyr_7b_beta_qlora_custom_sft_e1.py
@@ -0,0 +1,226 @@
+# Copyright (c) OpenMMLab. All rights reserved.
+"""Data format:
+
+[{
+ "messages": [
+ { "role": "system", "content": "xxx." },
+ { "role": "user", "content": "xxx." },
+ { "role": "assistant", "content": "xxx.", "loss": false},
+ { "role": "user", "content": "xxx." },
+ { "role": "assistant", "content": "xxx.", "loss": true}
+ ]
+},
+...
+]
+""" # noqa: E501
+import torch
+from datasets import load_dataset
+from mmengine.dataset import DefaultSampler
+from mmengine.hooks import (CheckpointHook, DistSamplerSeedHook, IterTimerHook,
+ LoggerHook, ParamSchedulerHook)
+from mmengine.optim import AmpOptimWrapper, CosineAnnealingLR, LinearLR
+from peft import LoraConfig
+from torch.optim import AdamW
+from transformers import (AutoModelForCausalLM, AutoTokenizer,
+ BitsAndBytesConfig)
+
+from xtuner.dataset import process_hf_dataset
+from xtuner.dataset.collate_fns import default_collate_fn
+from xtuner.dataset.map_fns import openai_map_fn, template_map_fn_factory
+from xtuner.engine.hooks import (DatasetInfoHook, EvaluateChatHook,
+ VarlenAttnArgsToMessageHubHook)
+from xtuner.engine.runner import TrainLoop
+from xtuner.model import SupervisedFinetune
+from xtuner.utils import PROMPT_TEMPLATE
+
+#######################################################################
+# PART 1 Settings #
+#######################################################################
+# Model
+pretrained_model_name_or_path = 'HuggingFaceH4/zephyr-7b-beta'
+use_varlen_attn = False
+
+# Data
+data_files = ['/path/to/json/file.json']
+prompt_template = PROMPT_TEMPLATE.zephyr
+max_length = 2048
+pack_to_max_length = True
+
+# Scheduler & Optimizer
+batch_size = 1 # per_device
+accumulative_counts = 16 # bs = 1 GPU * 1 batch_size_per_device * 16 acc
+dataloader_num_workers = 0
+max_epochs = 1
+optim_type = AdamW
+lr = 2e-4
+betas = (0.9, 0.999)
+weight_decay = 0
+max_norm = 1 # grad clip
+warmup_ratio = 0.03
+
+# Save
+save_steps = 500
+save_total_limit = 2 # Maximum checkpoints to keep (-1 means unlimited)
+
+# Evaluate the generation performance during the training
+evaluation_freq = 500
+SYSTEM = ''
+evaluation_inputs = [
+ '请给我介绍五个上海的景点', 'Please tell me five scenic spots in Shanghai'
+]
+
+#######################################################################
+# PART 2 Model & Tokenizer #
+#######################################################################
+tokenizer = dict(
+ type=AutoTokenizer.from_pretrained,
+ pretrained_model_name_or_path=pretrained_model_name_or_path,
+ trust_remote_code=True,
+ padding_side='right')
+
+model = dict(
+ type=SupervisedFinetune,
+ use_varlen_attn=use_varlen_attn,
+ llm=dict(
+ type=AutoModelForCausalLM.from_pretrained,
+ pretrained_model_name_or_path=pretrained_model_name_or_path,
+ trust_remote_code=True,
+ torch_dtype=torch.float16,
+ quantization_config=dict(
+ type=BitsAndBytesConfig,
+ load_in_4bit=True,
+ load_in_8bit=False,
+ llm_int8_threshold=6.0,
+ llm_int8_has_fp16_weight=False,
+ bnb_4bit_compute_dtype=torch.float16,
+ bnb_4bit_use_double_quant=True,
+ bnb_4bit_quant_type='nf4')),
+ lora=dict(
+ type=LoraConfig,
+ r=64,
+ lora_alpha=16,
+ lora_dropout=0.1,
+ bias='none',
+ task_type='CAUSAL_LM'))
+
+#######################################################################
+# PART 3 Dataset & Dataloader #
+#######################################################################
+train_dataset = dict(
+ type=process_hf_dataset,
+ dataset=dict(type=load_dataset, path='json', data_files=data_files),
+ tokenizer=tokenizer,
+ max_length=max_length,
+ dataset_map_fn=openai_map_fn,
+ template_map_fn=dict(
+ type=template_map_fn_factory, template=prompt_template),
+ remove_unused_columns=True,
+ shuffle_before_pack=True,
+ pack_to_max_length=pack_to_max_length,
+ use_varlen_attn=use_varlen_attn)
+
+train_dataloader = dict(
+ batch_size=batch_size,
+ num_workers=dataloader_num_workers,
+ dataset=train_dataset,
+ sampler=dict(type=DefaultSampler, shuffle=True),
+ collate_fn=dict(type=default_collate_fn, use_varlen_attn=use_varlen_attn))
+
+#######################################################################
+# PART 4 Scheduler & Optimizer #
+#######################################################################
+# optimizer
+optim_wrapper = dict(
+ type=AmpOptimWrapper,
+ optimizer=dict(
+ type=optim_type, lr=lr, betas=betas, weight_decay=weight_decay),
+ clip_grad=dict(max_norm=max_norm, error_if_nonfinite=False),
+ accumulative_counts=accumulative_counts,
+ loss_scale='dynamic',
+ dtype='float16')
+
+# learning policy
+# More information: https://github.com/open-mmlab/mmengine/blob/main/docs/en/tutorials/param_scheduler.md # noqa: E501
+param_scheduler = [
+ dict(
+ type=LinearLR,
+ start_factor=1e-5,
+ by_epoch=True,
+ begin=0,
+ end=warmup_ratio * max_epochs,
+ convert_to_iter_based=True),
+ dict(
+ type=CosineAnnealingLR,
+ eta_min=0.0,
+ by_epoch=True,
+ begin=warmup_ratio * max_epochs,
+ end=max_epochs,
+ convert_to_iter_based=True)
+]
+
+# train, val, test setting
+train_cfg = dict(type=TrainLoop, max_epochs=max_epochs)
+
+#######################################################################
+# PART 5 Runtime #
+#######################################################################
+# Log the dialogue periodically during the training process, optional
+custom_hooks = [
+ dict(type=DatasetInfoHook, tokenizer=tokenizer),
+ dict(
+ type=EvaluateChatHook,
+ tokenizer=tokenizer,
+ every_n_iters=evaluation_freq,
+ evaluation_inputs=evaluation_inputs,
+ system=SYSTEM,
+ prompt_template=prompt_template)
+]
+
+if use_varlen_attn:
+ custom_hooks += [dict(type=VarlenAttnArgsToMessageHubHook)]
+
+# configure default hooks
+default_hooks = dict(
+ # record the time of every iteration.
+ timer=dict(type=IterTimerHook),
+ # print log every 10 iterations.
+ logger=dict(type=LoggerHook, log_metric_by_epoch=False, interval=10),
+ # enable the parameter scheduler.
+ param_scheduler=dict(type=ParamSchedulerHook),
+ # save checkpoint per `save_steps`.
+ checkpoint=dict(
+ type=CheckpointHook,
+ by_epoch=False,
+ interval=save_steps,
+ max_keep_ckpts=save_total_limit),
+ # set sampler seed in distributed evrionment.
+ sampler_seed=dict(type=DistSamplerSeedHook),
+)
+
+# configure environment
+env_cfg = dict(
+ # whether to enable cudnn benchmark
+ cudnn_benchmark=False,
+ # set multi process parameters
+ mp_cfg=dict(mp_start_method='fork', opencv_num_threads=0),
+ # set distributed parameters
+ dist_cfg=dict(backend='nccl'),
+)
+
+# set visualizer
+visualizer = None
+
+# set log level
+log_level = 'INFO'
+
+# load from which checkpoint
+load_from = None
+
+# whether to resume training from the loaded checkpoint
+resume = False
+
+# Defaults to use random seed and disable `deterministic`
+randomness = dict(seed=None, deterministic=False)
+
+# set log processor
+log_processor = dict(by_epoch=False)
diff --git a/xtuner/configs/deepseek/README.md b/xtuner/configs/deepseek/README.md
new file mode 100644
index 000000000..dd16619c0
--- /dev/null
+++ b/xtuner/configs/deepseek/README.md
@@ -0,0 +1,59 @@
+# DeepSeek V2
+
+## Install
+
+```bash
+# Git clone the latest xtuner
+git clone https://github.com/InternLM/xtuner.git
+
+# Install the latest xtuner
+cd xtuner
+pip install -e '.[all]'
+
+# Mixtral requires flash-attn
+pip install flash-attn
+
+# install the latest transformers
+pip install -U transformers
+```
+
+## Full Parameter Fine-tune
+
+Full parameter fine-tune DeepSeek V2 236B needs at least 64 A100-80G. The full-tuned model will be saved to `${WORK_DIRS}/hf_model` by `HFCheckpointHook`.
+
+### slurm
+
+Note: `$PARTITION` means the virtual partition of slurm.
+
+```bash
+srun -p $PARTITION --job-name=mixtral --nodes=8 --gres=gpu:8 --ntasks-per-node=8 xtuner train deepseek_v2_chat_full_alpaca_e3 --deepspeed deepspeed_zero3 --launcher slurm
+```
+
+### torchrun
+
+Note: `$NODE_0_ADDR` means the ip address of the node_0 machine.
+
+```bash
+# excuete on node 0
+NPROC_PER_NODE=8 NNODES=8 PORT=29600 ADDR=$NODE_0_ADDR NODE_RANK=0 xtuner train deepseek_v2_chat_full_alpaca_e3 --deepspeed deepspeed_zero3 --launcher pytorch
+
+# excuete on node 1
+NPROC_PER_NODE=8 NNODES=8 PORT=29600 ADDR=$NODE_0_ADDR NODE_RANK=1 xtuner train deepseek_v2_chat_full_alpaca_e3 --deepspeed deepspeed_zero3 --launcher pytorch
+
+# excuete on node 2, 3, ..., 7
+```
+
+### Speed
+
+128 * A100 80G:
+
+| Model | Sequence Length | Use Varlen Attn | Sequence Parallel World Size | Tokens per Second |
+| :--------------------: | :-------------: | :-------------: | :--------------------------: | :---------------: |
+| deepseek v2 hf | 8k | False | 1 | 60 |
+| **deepseek v2 XTuner** | **8k** | **False** | **1** | **120 (2x)** |
+| deepseek v2 hf | 8k | True | 1 | 60 |
+| **deepseek v2 XTuner** | **8k** | **True** | **1** | **130 (2.2x)** |
+| deepseek v2 hf | 16k | False | 1 | OOM |
+| **deepseek v2 XTuner** | **16k** | **False** | **1** | **148** |
+| deepseek v2 hf | 16k | True | 1 | 95 |
+| **deepseek v2 XTuner** | **16k** | **True** | **1** | **180 (1.9x)** |
diff --git a/xtuner/configs/deepseek/deepseek_v2_chat/deepseek_v2_chat_full_alpaca_e3.py b/xtuner/configs/deepseek/deepseek_v2_chat/deepseek_v2_chat_full_alpaca_e3.py
new file mode 100644
index 000000000..016e7aed0
--- /dev/null
+++ b/xtuner/configs/deepseek/deepseek_v2_chat/deepseek_v2_chat_full_alpaca_e3.py
@@ -0,0 +1,198 @@
+# Copyright (c) OpenMMLab. All rights reserved.
+from datasets import load_dataset
+from mmengine.dataset import DefaultSampler
+from mmengine.hooks import (CheckpointHook, DistSamplerSeedHook, IterTimerHook,
+ LoggerHook, ParamSchedulerHook)
+from mmengine.optim import AmpOptimWrapper, CosineAnnealingLR
+from torch.optim import AdamW
+from transformers import AutoTokenizer
+
+from xtuner.dataset import process_hf_dataset
+from xtuner.dataset.collate_fns import default_collate_fn
+from xtuner.dataset.map_fns import alpaca_map_fn, template_map_fn_factory
+from xtuner.engine.hooks import (DatasetInfoHook, HFCheckpointHook,
+ ThroughputHook,
+ VarlenAttnArgsToMessageHubHook)
+from xtuner.engine.runner import TrainLoop
+from xtuner.model import SupervisedFinetune
+from xtuner.model.transformers_models.deepseek_v2 import DeepseekV2ForCausalLM
+from xtuner.parallel.sequence import SequenceParallelSampler
+from xtuner.utils import PROMPT_TEMPLATE, SYSTEM_TEMPLATE
+
+#######################################################################
+# PART 1 Settings #
+#######################################################################
+# Model
+pretrained_model_name_or_path = 'deepseek-ai/DeepSeek-V2-Chat'
+use_varlen_attn = False
+
+# Data
+data_path = 'tatsu-lab/alpaca'
+prompt_template = PROMPT_TEMPLATE.deepseek_v2
+max_length = 2048
+pack_to_max_length = True
+
+# parallel
+sequence_parallel_size = 1
+
+# Scheduler & Optimizer
+batch_size = 1 # per_device
+accumulative_counts = 1 # bs per device 1 * acc 1 * 128 gpus = 128 total bs
+accumulative_counts *= sequence_parallel_size
+dataloader_num_workers = 4
+max_epochs = 3
+optim_type = AdamW
+lr = 1e-5
+betas = (0.9, 0.999)
+weight_decay = 0
+max_norm = 1 # grad clip
+warmup_ratio = 0.03
+
+# Save
+save_steps = 50
+save_total_limit = 2 # Maximum checkpoints to keep (-1 means unlimited)
+# Save the optimizer states of deepseek v2 236B will require a lot of
+# storage space. It is recommended to set `save_optimizer` to False
+# (The training phase can not be resumed.)
+save_optimizer = True
+
+# Evaluate the generation performance during the training
+evaluation_freq = 25
+SYSTEM = SYSTEM_TEMPLATE.alpaca
+evaluation_inputs = [
+ '请给我介绍五个上海的景点', 'Please tell me five scenic spots in Shanghai'
+]
+
+#######################################################################
+# PART 2 Model & Tokenizer #
+#######################################################################
+tokenizer = dict(
+ type=AutoTokenizer.from_pretrained,
+ pretrained_model_name_or_path=pretrained_model_name_or_path,
+ trust_remote_code=True,
+ padding_side='right')
+
+model = dict(
+ type=SupervisedFinetune,
+ use_varlen_attn=use_varlen_attn,
+ llm=dict(
+ # Only full-finetune is supported in `DeepseekV2ForCausalLM``, XTuner.
+ # Please use `AutoModelForCausalLM` for lora or qlora finetune.
+ type=DeepseekV2ForCausalLM.from_pretrained,
+ pretrained_model_name_or_path=pretrained_model_name_or_path,
+ moe_implementation='shard',
+ expert_in_one_shard=10,
+ trust_remote_code=True))
+
+#######################################################################
+# PART 3 Dataset & Dataloader #
+#######################################################################
+train_dataset = dict(
+ type=process_hf_dataset,
+ dataset=dict(type=load_dataset, path=data_path),
+ tokenizer=tokenizer,
+ max_length=max_length,
+ dataset_map_fn=alpaca_map_fn,
+ template_map_fn=dict(
+ type=template_map_fn_factory, template=prompt_template),
+ remove_unused_columns=True,
+ shuffle_before_pack=True,
+ pack_to_max_length=pack_to_max_length,
+ use_varlen_attn=use_varlen_attn)
+
+sampler = SequenceParallelSampler \
+ if sequence_parallel_size > 1 else DefaultSampler
+
+train_dataloader = dict(
+ batch_size=batch_size,
+ num_workers=dataloader_num_workers,
+ dataset=train_dataset,
+ sampler=dict(type=sampler, shuffle=True),
+ collate_fn=dict(type=default_collate_fn, use_varlen_attn=use_varlen_attn))
+
+#######################################################################
+# PART 4 Scheduler & Optimizer #
+#######################################################################
+# optimizer
+optim_wrapper = dict(
+ type=AmpOptimWrapper,
+ optimizer=dict(
+ type=optim_type, lr=lr, betas=betas, weight_decay=weight_decay),
+ clip_grad=dict(max_norm=max_norm, error_if_nonfinite=False),
+ accumulative_counts=accumulative_counts,
+ loss_scale='dynamic',
+ dtype='float16')
+
+# learning policy
+# More information: https://github.com/open-mmlab/mmengine/blob/main/docs/en/tutorials/param_scheduler.md # noqa: E501
+param_scheduler = [
+ dict(
+ type=CosineAnnealingLR,
+ eta_min=0.0,
+ by_epoch=True,
+ begin=0,
+ end=max_epochs,
+ convert_to_iter_based=True)
+]
+
+# train, val, test setting
+train_cfg = dict(type=TrainLoop, max_epochs=max_epochs)
+
+#######################################################################
+# PART 5 Runtime #
+#######################################################################
+# Log the dialogue periodically during the training process, optional
+custom_hooks = [
+ dict(type=DatasetInfoHook, tokenizer=tokenizer),
+ dict(type=ThroughputHook),
+ dict(type=HFCheckpointHook)
+]
+
+if use_varlen_attn:
+ custom_hooks += [dict(type=VarlenAttnArgsToMessageHubHook)]
+
+# configure default hooks
+default_hooks = dict(
+ # record the time of every iteration.
+ timer=dict(type=IterTimerHook),
+ # print log every 10 iterations.
+ logger=dict(type=LoggerHook, log_metric_by_epoch=False, interval=1),
+ # enable the parameter scheduler.
+ param_scheduler=dict(type=ParamSchedulerHook),
+ # save checkpoint per `save_steps`.
+ checkpoint=dict(
+ type=CheckpointHook,
+ by_epoch=False,
+ interval=save_steps,
+ max_keep_ckpts=save_total_limit),
+ # set sampler seed in distributed evrionment.
+ sampler_seed=dict(type=DistSamplerSeedHook),
+)
+
+# configure environment
+env_cfg = dict(
+ # whether to enable cudnn benchmark
+ cudnn_benchmark=False,
+ # set multi process parameters
+ mp_cfg=dict(mp_start_method='fork', opencv_num_threads=0),
+ # set distributed parameters
+ dist_cfg=dict(backend='nccl'),
+)
+
+# set visualizer
+visualizer = None
+
+# set log level
+log_level = 'INFO'
+
+# load from which checkpoint
+load_from = None
+
+# whether to resume training from the loaded checkpoint
+resume = False
+
+# Defaults to use random seed and disable `deterministic`
+randomness = dict(seed=None, deterministic=False)
+
+# set log processor
+log_processor = dict(by_epoch=False, window_size=1)
diff --git a/xtuner/configs/deepseek/deepseek_v2_lite_chat/deepseek_v2_lite_chat_full_alpaca_e3.py b/xtuner/configs/deepseek/deepseek_v2_lite_chat/deepseek_v2_lite_chat_full_alpaca_e3.py
new file mode 100644
index 000000000..0d59ed45d
--- /dev/null
+++ b/xtuner/configs/deepseek/deepseek_v2_lite_chat/deepseek_v2_lite_chat_full_alpaca_e3.py
@@ -0,0 +1,195 @@
+# Copyright (c) OpenMMLab. All rights reserved.
+from datasets import load_dataset
+from mmengine.dataset import DefaultSampler
+from mmengine.hooks import (CheckpointHook, DistSamplerSeedHook, IterTimerHook,
+ LoggerHook, ParamSchedulerHook)
+from mmengine.optim import AmpOptimWrapper, CosineAnnealingLR
+from torch.optim import AdamW
+from transformers import AutoTokenizer
+
+from xtuner.dataset import process_hf_dataset
+from xtuner.dataset.collate_fns import default_collate_fn
+from xtuner.dataset.map_fns import alpaca_map_fn, template_map_fn_factory
+from xtuner.engine.hooks import (DatasetInfoHook, HFCheckpointHook,
+ ThroughputHook,
+ VarlenAttnArgsToMessageHubHook)
+from xtuner.engine.runner import TrainLoop
+from xtuner.model import SupervisedFinetune
+from xtuner.model.transformers_models.deepseek_v2 import DeepseekV2ForCausalLM
+from xtuner.parallel.sequence import SequenceParallelSampler
+from xtuner.utils import PROMPT_TEMPLATE, SYSTEM_TEMPLATE
+
+#######################################################################
+# PART 1 Settings #
+#######################################################################
+# Model
+pretrained_model_name_or_path = 'deepseek-ai/DeepSeek-V2-Lite-Chat'
+use_varlen_attn = False
+
+# Data
+data_path = 'tatsu-lab/alpaca'
+prompt_template = PROMPT_TEMPLATE.deepseek_v2
+max_length = 2048
+pack_to_max_length = True
+
+# parallel
+sequence_parallel_size = 1
+
+# Scheduler & Optimizer
+batch_size = 1 # per_device
+accumulative_counts = 1 # bs per device 1 * acc 1 * 128 gpus = 128 total bs
+accumulative_counts *= sequence_parallel_size
+dataloader_num_workers = 4
+max_epochs = 3
+optim_type = AdamW
+lr = 1e-5
+betas = (0.9, 0.999)
+weight_decay = 0
+max_norm = 1 # grad clip
+warmup_ratio = 0.03
+
+# Save
+save_steps = 50
+save_total_limit = 2 # Maximum checkpoints to keep (-1 means unlimited)
+save_optimizer = True
+
+# Evaluate the generation performance during the training
+evaluation_freq = 50
+SYSTEM = SYSTEM_TEMPLATE.alpaca
+evaluation_inputs = [
+ '请给我介绍五个上海的景点', 'Please tell me five scenic spots in Shanghai'
+]
+
+#######################################################################
+# PART 2 Model & Tokenizer #
+#######################################################################
+tokenizer = dict(
+ type=AutoTokenizer.from_pretrained,
+ pretrained_model_name_or_path=pretrained_model_name_or_path,
+ trust_remote_code=True,
+ padding_side='right')
+
+model = dict(
+ type=SupervisedFinetune,
+ use_varlen_attn=use_varlen_attn,
+ llm=dict(
+ # Only full-finetune is supported in `DeepseekV2ForCausalLM``, XTuner.
+ # Please use `AutoModelForCausalLM` for lora or qlora finetune.
+ type=DeepseekV2ForCausalLM.from_pretrained,
+ pretrained_model_name_or_path=pretrained_model_name_or_path,
+ moe_implementation='shard',
+ expert_in_one_shard=8,
+ trust_remote_code=True))
+
+#######################################################################
+# PART 3 Dataset & Dataloader #
+#######################################################################
+train_dataset = dict(
+ type=process_hf_dataset,
+ dataset=dict(type=load_dataset, path=data_path),
+ tokenizer=tokenizer,
+ max_length=max_length,
+ dataset_map_fn=alpaca_map_fn,
+ template_map_fn=dict(
+ type=template_map_fn_factory, template=prompt_template),
+ remove_unused_columns=True,
+ shuffle_before_pack=True,
+ pack_to_max_length=pack_to_max_length,
+ use_varlen_attn=use_varlen_attn)
+
+sampler = SequenceParallelSampler \
+ if sequence_parallel_size > 1 else DefaultSampler
+
+train_dataloader = dict(
+ batch_size=batch_size,
+ num_workers=dataloader_num_workers,
+ dataset=train_dataset,
+ sampler=dict(type=sampler, shuffle=True),
+ collate_fn=dict(type=default_collate_fn, use_varlen_attn=use_varlen_attn))
+
+#######################################################################
+# PART 4 Scheduler & Optimizer #
+#######################################################################
+# optimizer
+optim_wrapper = dict(
+ type=AmpOptimWrapper,
+ optimizer=dict(
+ type=optim_type, lr=lr, betas=betas, weight_decay=weight_decay),
+ clip_grad=dict(max_norm=max_norm, error_if_nonfinite=False),
+ accumulative_counts=accumulative_counts,
+ loss_scale='dynamic',
+ dtype='float16')
+
+# learning policy
+# More information: https://github.com/open-mmlab/mmengine/blob/main/docs/en/tutorials/param_scheduler.md # noqa: E501
+param_scheduler = [
+ dict(
+ type=CosineAnnealingLR,
+ eta_min=0.0,
+ by_epoch=True,
+ begin=0,
+ end=max_epochs,
+ convert_to_iter_based=True)
+]
+
+# train, val, test setting
+train_cfg = dict(type=TrainLoop, max_epochs=max_epochs)
+
+#######################################################################
+# PART 5 Runtime #
+#######################################################################
+# Log the dialogue periodically during the training process, optional
+custom_hooks = [
+ dict(type=DatasetInfoHook, tokenizer=tokenizer),
+ dict(type=ThroughputHook),
+ dict(type=HFCheckpointHook)
+]
+
+if use_varlen_attn:
+ custom_hooks += [dict(type=VarlenAttnArgsToMessageHubHook)]
+
+# configure default hooks
+default_hooks = dict(
+ # record the time of every iteration.
+ timer=dict(type=IterTimerHook),
+ # print log every 10 iterations.
+ logger=dict(type=LoggerHook, log_metric_by_epoch=False, interval=1),
+ # enable the parameter scheduler.
+ param_scheduler=dict(type=ParamSchedulerHook),
+ # save checkpoint per `save_steps`.
+ checkpoint=dict(
+ type=CheckpointHook,
+ by_epoch=False,
+ interval=save_steps,
+ max_keep_ckpts=save_total_limit),
+ # set sampler seed in distributed evrionment.
+ sampler_seed=dict(type=DistSamplerSeedHook),
+)
+
+# configure environment
+env_cfg = dict(
+ # whether to enable cudnn benchmark
+ cudnn_benchmark=False,
+ # set multi process parameters
+ mp_cfg=dict(mp_start_method='fork', opencv_num_threads=0),
+ # set distributed parameters
+ dist_cfg=dict(backend='nccl'),
+)
+
+# set visualizer
+visualizer = None
+
+# set log level
+log_level = 'INFO'
+
+# load from which checkpoint
+load_from = None
+
+# whether to resume training from the loaded checkpoint
+resume = False
+
+# Defaults to use random seed and disable `deterministic`
+randomness = dict(seed=None, deterministic=False)
+
+# set log processor
+log_processor = dict(by_epoch=False, window_size=1)
diff --git a/xtuner/configs/deepseek/deepseek_v2_lite_chat/deepseek_v2_lite_chat_full_alpaca_e3_32k_varlen.py b/xtuner/configs/deepseek/deepseek_v2_lite_chat/deepseek_v2_lite_chat_full_alpaca_e3_32k_varlen.py
new file mode 100644
index 000000000..03b042daf
--- /dev/null
+++ b/xtuner/configs/deepseek/deepseek_v2_lite_chat/deepseek_v2_lite_chat_full_alpaca_e3_32k_varlen.py
@@ -0,0 +1,195 @@
+# Copyright (c) OpenMMLab. All rights reserved.
+from datasets import load_dataset
+from mmengine.dataset import DefaultSampler
+from mmengine.hooks import (CheckpointHook, DistSamplerSeedHook, IterTimerHook,
+ LoggerHook, ParamSchedulerHook)
+from mmengine.optim import AmpOptimWrapper, CosineAnnealingLR
+from torch.optim import AdamW
+from transformers import AutoTokenizer
+
+from xtuner.dataset import process_hf_dataset
+from xtuner.dataset.collate_fns import default_collate_fn
+from xtuner.dataset.map_fns import alpaca_map_fn, template_map_fn_factory
+from xtuner.engine.hooks import (DatasetInfoHook, HFCheckpointHook,
+ ThroughputHook,
+ VarlenAttnArgsToMessageHubHook)
+from xtuner.engine.runner import TrainLoop
+from xtuner.model import SupervisedFinetune
+from xtuner.model.transformers_models.deepseek_v2 import DeepseekV2ForCausalLM
+from xtuner.parallel.sequence import SequenceParallelSampler
+from xtuner.utils import PROMPT_TEMPLATE, SYSTEM_TEMPLATE
+
+#######################################################################
+# PART 1 Settings #
+#######################################################################
+# Model
+pretrained_model_name_or_path = 'deepseek-ai/DeepSeek-V2-Lite-Chat'
+use_varlen_attn = True
+
+# Data
+data_path = 'tatsu-lab/alpaca'
+prompt_template = PROMPT_TEMPLATE.deepseek_v2
+max_length = 32768
+pack_to_max_length = True
+
+# parallel
+sequence_parallel_size = 1
+
+# Scheduler & Optimizer
+batch_size = 1 # per_device
+accumulative_counts = 1 # bs per device 1 * acc 1 * 128 gpus = 128 total bs
+accumulative_counts *= sequence_parallel_size
+dataloader_num_workers = 4
+max_epochs = 3
+optim_type = AdamW
+lr = 1e-5
+betas = (0.9, 0.999)
+weight_decay = 0
+max_norm = 1 # grad clip
+warmup_ratio = 0.03
+
+# Save
+save_steps = 50
+save_total_limit = 2 # Maximum checkpoints to keep (-1 means unlimited)
+save_optimizer = True
+
+# Evaluate the generation performance during the training
+evaluation_freq = 50
+SYSTEM = SYSTEM_TEMPLATE.alpaca
+evaluation_inputs = [
+ '请给我介绍五个上海的景点', 'Please tell me five scenic spots in Shanghai'
+]
+
+#######################################################################
+# PART 2 Model & Tokenizer #
+#######################################################################
+tokenizer = dict(
+ type=AutoTokenizer.from_pretrained,
+ pretrained_model_name_or_path=pretrained_model_name_or_path,
+ trust_remote_code=True,
+ padding_side='right')
+
+model = dict(
+ type=SupervisedFinetune,
+ use_varlen_attn=use_varlen_attn,
+ llm=dict(
+ # Only full-finetune is supported in `DeepseekV2ForCausalLM``, XTuner.
+ # Please use `AutoModelForCausalLM` for lora or qlora finetune.
+ type=DeepseekV2ForCausalLM.from_pretrained,
+ pretrained_model_name_or_path=pretrained_model_name_or_path,
+ moe_implementation='shard',
+ expert_in_one_shard=8,
+ trust_remote_code=True))
+
+#######################################################################
+# PART 3 Dataset & Dataloader #
+#######################################################################
+train_dataset = dict(
+ type=process_hf_dataset,
+ dataset=dict(type=load_dataset, path=data_path),
+ tokenizer=tokenizer,
+ max_length=max_length,
+ dataset_map_fn=alpaca_map_fn,
+ template_map_fn=dict(
+ type=template_map_fn_factory, template=prompt_template),
+ remove_unused_columns=True,
+ shuffle_before_pack=True,
+ pack_to_max_length=pack_to_max_length,
+ use_varlen_attn=use_varlen_attn)
+
+sampler = SequenceParallelSampler \
+ if sequence_parallel_size > 1 else DefaultSampler
+
+train_dataloader = dict(
+ batch_size=batch_size,
+ num_workers=dataloader_num_workers,
+ dataset=train_dataset,
+ sampler=dict(type=sampler, shuffle=True),
+ collate_fn=dict(type=default_collate_fn, use_varlen_attn=use_varlen_attn))
+
+#######################################################################
+# PART 4 Scheduler & Optimizer #
+#######################################################################
+# optimizer
+optim_wrapper = dict(
+ type=AmpOptimWrapper,
+ optimizer=dict(
+ type=optim_type, lr=lr, betas=betas, weight_decay=weight_decay),
+ clip_grad=dict(max_norm=max_norm, error_if_nonfinite=False),
+ accumulative_counts=accumulative_counts,
+ loss_scale='dynamic',
+ dtype='float16')
+
+# learning policy
+# More information: https://github.com/open-mmlab/mmengine/blob/main/docs/en/tutorials/param_scheduler.md # noqa: E501
+param_scheduler = [
+ dict(
+ type=CosineAnnealingLR,
+ eta_min=0.0,
+ by_epoch=True,
+ begin=0,
+ end=max_epochs,
+ convert_to_iter_based=True)
+]
+
+# train, val, test setting
+train_cfg = dict(type=TrainLoop, max_epochs=max_epochs)
+
+#######################################################################
+# PART 5 Runtime #
+#######################################################################
+# Log the dialogue periodically during the training process, optional
+custom_hooks = [
+ dict(type=DatasetInfoHook, tokenizer=tokenizer),
+ dict(type=ThroughputHook),
+ dict(type=HFCheckpointHook)
+]
+
+if use_varlen_attn:
+ custom_hooks += [dict(type=VarlenAttnArgsToMessageHubHook)]
+
+# configure default hooks
+default_hooks = dict(
+ # record the time of every iteration.
+ timer=dict(type=IterTimerHook),
+ # print log every 10 iterations.
+ logger=dict(type=LoggerHook, log_metric_by_epoch=False, interval=1),
+ # enable the parameter scheduler.
+ param_scheduler=dict(type=ParamSchedulerHook),
+ # save checkpoint per `save_steps`.
+ checkpoint=dict(
+ type=CheckpointHook,
+ by_epoch=False,
+ interval=save_steps,
+ max_keep_ckpts=save_total_limit),
+ # set sampler seed in distributed evrionment.
+ sampler_seed=dict(type=DistSamplerSeedHook),
+)
+
+# configure environment
+env_cfg = dict(
+ # whether to enable cudnn benchmark
+ cudnn_benchmark=False,
+ # set multi process parameters
+ mp_cfg=dict(mp_start_method='fork', opencv_num_threads=0),
+ # set distributed parameters
+ dist_cfg=dict(backend='nccl'),
+)
+
+# set visualizer
+visualizer = None
+
+# set log level
+log_level = 'INFO'
+
+# load from which checkpoint
+load_from = None
+
+# whether to resume training from the loaded checkpoint
+resume = False
+
+# Defaults to use random seed and disable `deterministic`
+randomness = dict(seed=None, deterministic=False)
+
+# set log processor
+log_processor = dict(by_epoch=False, window_size=1)
diff --git a/xtuner/configs/dpo/internlm/internlm2_chat_1_8b_dpo_full.py b/xtuner/configs/dpo/internlm/internlm2_chat_1_8b_dpo_full.py
new file mode 100644
index 000000000..908683fe6
--- /dev/null
+++ b/xtuner/configs/dpo/internlm/internlm2_chat_1_8b_dpo_full.py
@@ -0,0 +1,201 @@
+# Copyright (c) OpenMMLab. All rights reserved.
+from datasets import load_dataset
+from mmengine.dataset import DefaultSampler
+from mmengine.hooks import (CheckpointHook, DistSamplerSeedHook, IterTimerHook,
+ LoggerHook, ParamSchedulerHook)
+from mmengine.optim import AmpOptimWrapper, CosineAnnealingLR, LinearLR
+from torch.optim import AdamW
+from transformers import AutoModelForCausalLM, AutoTokenizer
+
+from xtuner.dataset.collate_fns.preference_collate_fn import \
+ preference_collate_fn
+from xtuner.dataset.preference_dataset import (build_preference_dataset,
+ orpo_dpo_mix_40k_map_fn)
+from xtuner.engine.hooks import (DatasetInfoHook, EvaluateChatHook,
+ VarlenAttnArgsToMessageHubHook)
+from xtuner.engine.runner import TrainLoop
+from xtuner.model.dpo import DPO
+from xtuner.utils import PROMPT_TEMPLATE, SYSTEM_TEMPLATE
+
+#######################################################################
+# PART 1 Settings #
+#######################################################################
+# Model
+pretrained_model_name_or_path = 'internlm/internlm2-chat-1_8b-sft'
+use_varlen_attn = False
+dpo_loss_type = 'sigmoid' # One of ['sigmoid', 'hinge', 'ipo', 'kto_pair', 'sppo_hard', 'nca_pair', 'robust'] # noqa: E501
+loss_beta = 0.1
+label_smoothing = 0.0
+
+# Data
+prompt_template = PROMPT_TEMPLATE.internlm2_chat
+max_length = 2048
+
+# Scheduler & Optimizer
+batch_size = 1 # per_device
+accumulative_counts = 16
+dataloader_num_workers = 0
+max_epochs = 3
+optim_type = AdamW
+lr = 5e-7 # refer to alignment handbook
+betas = (0.9, 0.999)
+weight_decay = 0
+max_norm = 1 # grad clip
+warmup_ratio = 0.03
+
+# Save
+save_steps = 500
+save_total_limit = 2 # Maximum checkpoints to keep (-1 means unlimited)
+
+# Evaluate the generation performance during the training
+evaluation_freq = 500
+SYSTEM = SYSTEM_TEMPLATE.alpaca
+evaluation_inputs = [
+ 'What famous British author, known for his tales of mystery and the macabre, shares his initials with a common abbreviation for "rest in peace"?', # noqa: E501
+ 'Please tell me five scenic spots in Shanghai',
+ '890729 - 425663? Only respond with math and no words.'
+]
+
+#######################################################################
+# PART 2 Model & Tokenizer #
+#######################################################################
+tokenizer = dict(
+ type=AutoTokenizer.from_pretrained,
+ pretrained_model_name_or_path=pretrained_model_name_or_path,
+ trust_remote_code=True,
+ padding_side='right')
+
+model = dict(
+ type=DPO,
+ use_varlen_attn=use_varlen_attn,
+ loss_type=dpo_loss_type,
+ beta=loss_beta,
+ label_smoothing=label_smoothing,
+ llm=dict(
+ type=AutoModelForCausalLM.from_pretrained,
+ pretrained_model_name_or_path=pretrained_model_name_or_path,
+ trust_remote_code=True))
+
+#######################################################################
+# PART 3 Dataset & Dataloader #
+#######################################################################
+train_dataset = dict(
+ type=build_preference_dataset,
+ dataset=dict(type=load_dataset, path='mlabonne/orpo-dpo-mix-40k'),
+ tokenizer=tokenizer,
+ max_length=max_length,
+ dataset_map_fn=orpo_dpo_mix_40k_map_fn,
+ is_dpo=True,
+ is_reward=False,
+ reward_token_id=-1,
+ num_proc=32,
+ use_varlen_attn=use_varlen_attn,
+ shuffle_before_pack=True,
+)
+
+train_dataloader = dict(
+ batch_size=batch_size,
+ num_workers=dataloader_num_workers,
+ dataset=train_dataset,
+ sampler=dict(type=DefaultSampler, shuffle=True),
+ collate_fn=dict(
+ type=preference_collate_fn, use_varlen_attn=use_varlen_attn))
+
+#######################################################################
+# PART 4 Scheduler & Optimizer #
+#######################################################################
+# optimizer
+optim_wrapper = dict(
+ type=AmpOptimWrapper,
+ optimizer=dict(
+ type=optim_type, lr=lr, betas=betas, weight_decay=weight_decay),
+ clip_grad=dict(max_norm=max_norm, error_if_nonfinite=False),
+ accumulative_counts=accumulative_counts,
+ loss_scale='dynamic',
+ dtype='float16')
+
+# learning policy
+# More information: https://github.com/open-mmlab/mmengine/blob/main/docs/en/tutorials/param_scheduler.md # noqa: E501
+param_scheduler = [
+ dict(
+ type=LinearLR,
+ start_factor=1e-5,
+ by_epoch=True,
+ begin=0,
+ end=warmup_ratio * max_epochs,
+ convert_to_iter_based=True),
+ dict(
+ type=CosineAnnealingLR,
+ eta_min=0.0,
+ by_epoch=True,
+ begin=warmup_ratio * max_epochs,
+ end=max_epochs,
+ convert_to_iter_based=True)
+]
+
+# train, val, test setting
+train_cfg = dict(type=TrainLoop, max_epochs=max_epochs)
+
+#######################################################################
+# PART 5 Runtime #
+#######################################################################
+# Log the dialogue periodically during the training process, optional
+custom_hooks = [
+ dict(type=DatasetInfoHook, tokenizer=tokenizer),
+ dict(
+ type=EvaluateChatHook,
+ tokenizer=tokenizer,
+ every_n_iters=evaluation_freq,
+ evaluation_inputs=evaluation_inputs,
+ system=SYSTEM,
+ prompt_template=prompt_template)
+]
+
+if use_varlen_attn:
+ custom_hooks += [dict(type=VarlenAttnArgsToMessageHubHook)]
+
+# configure default hooks
+default_hooks = dict(
+ # record the time of every iteration.
+ timer=dict(type=IterTimerHook),
+ # print log every 10 iterations.
+ logger=dict(type=LoggerHook, log_metric_by_epoch=False, interval=10),
+ # enable the parameter scheduler.
+ param_scheduler=dict(type=ParamSchedulerHook),
+ # save checkpoint per `save_steps`.
+ checkpoint=dict(
+ type=CheckpointHook,
+ by_epoch=False,
+ interval=save_steps,
+ max_keep_ckpts=save_total_limit),
+ # set sampler seed in distributed evrionment.
+ sampler_seed=dict(type=DistSamplerSeedHook),
+)
+
+# configure environment
+env_cfg = dict(
+ # whether to enable cudnn benchmark
+ cudnn_benchmark=False,
+ # set multi process parameters
+ mp_cfg=dict(mp_start_method='fork', opencv_num_threads=0),
+ # set distributed parameters
+ dist_cfg=dict(backend='nccl'),
+)
+
+# set visualizer
+visualizer = None
+
+# set log level
+log_level = 'INFO'
+
+# load from which checkpoint
+load_from = None
+
+# whether to resume training from the loaded checkpoint
+resume = False
+
+# Defaults to use random seed and disable `deterministic`
+randomness = dict(seed=None, deterministic=False)
+
+# set log processor
+log_processor = dict(by_epoch=False)
diff --git a/xtuner/configs/dpo/internlm/internlm2_chat_1_8b_dpo_full_varlenattn.py b/xtuner/configs/dpo/internlm/internlm2_chat_1_8b_dpo_full_varlenattn.py
new file mode 100644
index 000000000..787ad68bb
--- /dev/null
+++ b/xtuner/configs/dpo/internlm/internlm2_chat_1_8b_dpo_full_varlenattn.py
@@ -0,0 +1,211 @@
+# Copyright (c) OpenMMLab. All rights reserved.
+from datasets import load_dataset
+from mmengine.dataset import DefaultSampler
+from mmengine.hooks import (CheckpointHook, DistSamplerSeedHook, IterTimerHook,
+ LoggerHook, ParamSchedulerHook)
+from mmengine.optim import AmpOptimWrapper, CosineAnnealingLR, LinearLR
+from torch.optim import AdamW
+from transformers import AutoModelForCausalLM, AutoTokenizer
+
+from xtuner.dataset.collate_fns.preference_collate_fn import \
+ preference_collate_fn
+from xtuner.dataset.preference_dataset import (build_preference_dataset,
+ orpo_dpo_mix_40k_map_fn)
+from xtuner.engine.hooks import (DatasetInfoHook, EvaluateChatHook,
+ VarlenAttnArgsToMessageHubHook)
+from xtuner.engine.runner import TrainLoop
+from xtuner.model.dpo import DPO
+from xtuner.parallel.sequence import SequenceParallelSampler
+from xtuner.utils import PROMPT_TEMPLATE, SYSTEM_TEMPLATE
+
+#######################################################################
+# PART 1 Settings #
+#######################################################################
+# Model
+pretrained_model_name_or_path = 'internlm/internlm2-chat-1_8b-sft'
+use_varlen_attn = True
+dpo_loss_type = 'sigmoid' # One of ['sigmoid', 'hinge', 'ipo', 'kto_pair', 'sppo_hard', 'nca_pair', 'robust'] # noqa: E501
+loss_beta = 0.1
+label_smoothing = 0.0
+
+# Data
+prompt_template = PROMPT_TEMPLATE.internlm2_chat
+max_length = 2048
+max_packed_length = max_length * 2
+
+# parallel
+sequence_parallel_size = 1
+
+# Scheduler & Optimizer
+batch_size = 1 # per_device
+accumulative_counts = 16
+accumulative_counts *= sequence_parallel_size
+dataloader_num_workers = 0
+max_epochs = 3
+optim_type = AdamW
+lr = 5e-7 # refer to alignment handbook
+betas = (0.9, 0.999)
+weight_decay = 0
+max_norm = 1 # grad clip
+warmup_ratio = 0.03
+
+# Save
+save_steps = 500
+save_total_limit = 2 # Maximum checkpoints to keep (-1 means unlimited)
+
+# Evaluate the generation performance during the training
+evaluation_freq = 500
+SYSTEM = SYSTEM_TEMPLATE.alpaca
+evaluation_inputs = [
+ 'What famous British author, known for his tales of mystery and the macabre, shares his initials with a common abbreviation for "rest in peace"?', # noqa: E501
+ 'Please tell me five scenic spots in Shanghai',
+ '890729 - 425663? Only respond with math and no words.'
+]
+
+#######################################################################
+# PART 2 Model & Tokenizer #
+#######################################################################
+tokenizer = dict(
+ type=AutoTokenizer.from_pretrained,
+ pretrained_model_name_or_path=pretrained_model_name_or_path,
+ trust_remote_code=True,
+ padding_side='right')
+
+model = dict(
+ type=DPO,
+ use_varlen_attn=use_varlen_attn,
+ loss_type=dpo_loss_type,
+ beta=loss_beta,
+ label_smoothing=label_smoothing,
+ llm=dict(
+ type=AutoModelForCausalLM.from_pretrained,
+ pretrained_model_name_or_path=pretrained_model_name_or_path,
+ trust_remote_code=True))
+
+#######################################################################
+# PART 3 Dataset & Dataloader #
+#######################################################################
+sampler = SequenceParallelSampler \
+ if sequence_parallel_size > 1 else DefaultSampler
+
+train_dataset = dict(
+ type=build_preference_dataset,
+ dataset=dict(type=load_dataset, path='mlabonne/orpo-dpo-mix-40k'),
+ tokenizer=tokenizer,
+ max_length=max_length,
+ dataset_map_fn=orpo_dpo_mix_40k_map_fn,
+ is_dpo=True,
+ is_reward=False,
+ reward_token_id=-1,
+ num_proc=32,
+ use_varlen_attn=use_varlen_attn,
+ max_packed_length=max_packed_length,
+ shuffle_before_pack=True,
+)
+
+train_dataloader = dict(
+ batch_size=batch_size,
+ num_workers=dataloader_num_workers,
+ dataset=train_dataset,
+ sampler=dict(type=sampler, shuffle=True),
+ collate_fn=dict(
+ type=preference_collate_fn, use_varlen_attn=use_varlen_attn))
+
+#######################################################################
+# PART 4 Scheduler & Optimizer #
+#######################################################################
+# optimizer
+optim_wrapper = dict(
+ type=AmpOptimWrapper,
+ optimizer=dict(
+ type=optim_type, lr=lr, betas=betas, weight_decay=weight_decay),
+ clip_grad=dict(max_norm=max_norm, error_if_nonfinite=False),
+ accumulative_counts=accumulative_counts,
+ loss_scale='dynamic',
+ dtype='float16')
+
+# learning policy
+# More information: https://github.com/open-mmlab/mmengine/blob/main/docs/en/tutorials/param_scheduler.md # noqa: E501
+param_scheduler = [
+ dict(
+ type=LinearLR,
+ start_factor=1e-5,
+ by_epoch=True,
+ begin=0,
+ end=warmup_ratio * max_epochs,
+ convert_to_iter_based=True),
+ dict(
+ type=CosineAnnealingLR,
+ eta_min=0.0,
+ by_epoch=True,
+ begin=warmup_ratio * max_epochs,
+ end=max_epochs,
+ convert_to_iter_based=True)
+]
+
+# train, val, test setting
+train_cfg = dict(type=TrainLoop, max_epochs=max_epochs)
+
+#######################################################################
+# PART 5 Runtime #
+#######################################################################
+# Log the dialogue periodically during the training process, optional
+custom_hooks = [
+ dict(type=DatasetInfoHook, tokenizer=tokenizer),
+ dict(
+ type=EvaluateChatHook,
+ tokenizer=tokenizer,
+ every_n_iters=evaluation_freq,
+ evaluation_inputs=evaluation_inputs,
+ system=SYSTEM,
+ prompt_template=prompt_template)
+]
+
+if use_varlen_attn:
+ custom_hooks += [dict(type=VarlenAttnArgsToMessageHubHook)]
+
+# configure default hooks
+default_hooks = dict(
+ # record the time of every iteration.
+ timer=dict(type=IterTimerHook),
+ # print log every 10 iterations.
+ logger=dict(type=LoggerHook, log_metric_by_epoch=False, interval=10),
+ # enable the parameter scheduler.
+ param_scheduler=dict(type=ParamSchedulerHook),
+ # save checkpoint per `save_steps`.
+ checkpoint=dict(
+ type=CheckpointHook,
+ by_epoch=False,
+ interval=save_steps,
+ max_keep_ckpts=save_total_limit),
+ # set sampler seed in distributed evrionment.
+ sampler_seed=dict(type=DistSamplerSeedHook),
+)
+
+# configure environment
+env_cfg = dict(
+ # whether to enable cudnn benchmark
+ cudnn_benchmark=False,
+ # set multi process parameters
+ mp_cfg=dict(mp_start_method='fork', opencv_num_threads=0),
+ # set distributed parameters
+ dist_cfg=dict(backend='nccl'),
+)
+
+# set visualizer
+visualizer = None
+
+# set log level
+log_level = 'INFO'
+
+# load from which checkpoint
+load_from = None
+
+# whether to resume training from the loaded checkpoint
+resume = False
+
+# Defaults to use random seed and disable `deterministic`
+randomness = dict(seed=None, deterministic=False)
+
+# set log processor
+log_processor = dict(by_epoch=False)
diff --git a/xtuner/configs/dpo/internlm/internlm2_chat_1_8b_dpo_full_varlenattn_jsonl_dataset.py b/xtuner/configs/dpo/internlm/internlm2_chat_1_8b_dpo_full_varlenattn_jsonl_dataset.py
new file mode 100644
index 000000000..ae1a3cdca
--- /dev/null
+++ b/xtuner/configs/dpo/internlm/internlm2_chat_1_8b_dpo_full_varlenattn_jsonl_dataset.py
@@ -0,0 +1,215 @@
+# Copyright (c) OpenMMLab. All rights reserved.
+from mmengine.dataset import DefaultSampler
+from mmengine.hooks import (CheckpointHook, DistSamplerSeedHook, IterTimerHook,
+ LoggerHook, ParamSchedulerHook)
+from mmengine.optim import AmpOptimWrapper, CosineAnnealingLR, LinearLR
+from torch.optim import AdamW
+from transformers import AutoModelForCausalLM, AutoTokenizer
+
+from xtuner.dataset.collate_fns.preference_collate_fn import \
+ preference_collate_fn
+from xtuner.dataset.preference_dataset import (build_preference_dataset,
+ load_jsonl_dataset)
+from xtuner.engine.hooks import (DatasetInfoHook, EvaluateChatHook,
+ VarlenAttnArgsToMessageHubHook)
+from xtuner.engine.runner import TrainLoop
+from xtuner.model.dpo import DPO
+from xtuner.parallel.sequence import SequenceParallelSampler
+from xtuner.utils import PROMPT_TEMPLATE, SYSTEM_TEMPLATE
+
+#######################################################################
+# PART 1 Settings #
+#######################################################################
+# Model
+pretrained_model_name_or_path = 'internlm/internlm2-chat-1_8b-sft'
+use_varlen_attn = True
+dpo_loss_type = 'sigmoid' # One of ['sigmoid', 'hinge', 'ipo', 'kto_pair', 'sppo_hard', 'nca_pair', 'robust'] # noqa: E501
+loss_beta = 0.1
+label_smoothing = 0.0
+
+# Data
+prompt_template = PROMPT_TEMPLATE.internlm2_chat
+max_length = 2048
+max_packed_length = max_length * 2
+
+# parallel
+sequence_parallel_size = 1
+
+# Scheduler & Optimizer
+batch_size = 1 # per_device
+accumulative_counts = 16
+accumulative_counts *= sequence_parallel_size
+dataloader_num_workers = 0
+max_epochs = 3
+optim_type = AdamW
+lr = 5e-7 # refer to alignment handbook
+betas = (0.9, 0.999)
+weight_decay = 0
+max_norm = 1 # grad clip
+warmup_ratio = 0.03
+
+# Save
+save_steps = 500
+save_total_limit = 2 # Maximum checkpoints to keep (-1 means unlimited)
+
+# Evaluate the generation performance during the training
+evaluation_freq = 500
+SYSTEM = SYSTEM_TEMPLATE.alpaca
+evaluation_inputs = [
+ 'What famous British author, known for his tales of mystery and the macabre, shares his initials with a common abbreviation for "rest in peace"?', # noqa: E501
+ 'Please tell me five scenic spots in Shanghai',
+ '890729 - 425663? Only respond with math and no words.'
+]
+
+#######################################################################
+# PART 2 Model & Tokenizer #
+#######################################################################
+tokenizer = dict(
+ type=AutoTokenizer.from_pretrained,
+ pretrained_model_name_or_path=pretrained_model_name_or_path,
+ trust_remote_code=True,
+ padding_side='right')
+
+model = dict(
+ type=DPO,
+ use_varlen_attn=use_varlen_attn,
+ loss_type=dpo_loss_type,
+ beta=loss_beta,
+ label_smoothing=label_smoothing,
+ llm=dict(
+ type=AutoModelForCausalLM.from_pretrained,
+ pretrained_model_name_or_path=pretrained_model_name_or_path,
+ trust_remote_code=True))
+
+#######################################################################
+# PART 3 Dataset & Dataloader #
+#######################################################################
+sampler = SequenceParallelSampler \
+ if sequence_parallel_size > 1 else DefaultSampler
+
+train_dataset = dict(
+ type=build_preference_dataset,
+ dataset=dict(
+ type=load_jsonl_dataset,
+ data_files=[
+ '/your/jsonl/path/here.jsonl',
+ '/your/another/jsonl/path/here.jsonl'
+ ]),
+ tokenizer=tokenizer,
+ max_length=max_length,
+ dataset_map_fn=None,
+ is_dpo=True,
+ is_reward=False,
+ reward_token_id=-1,
+ num_proc=32,
+ use_varlen_attn=use_varlen_attn,
+ max_packed_length=max_packed_length,
+ shuffle_before_pack=True,
+)
+
+train_dataloader = dict(
+ batch_size=batch_size,
+ num_workers=dataloader_num_workers,
+ dataset=train_dataset,
+ sampler=dict(type=sampler, shuffle=True),
+ collate_fn=dict(
+ type=preference_collate_fn, use_varlen_attn=use_varlen_attn))
+
+#######################################################################
+# PART 4 Scheduler & Optimizer #
+#######################################################################
+# optimizer
+optim_wrapper = dict(
+ type=AmpOptimWrapper,
+ optimizer=dict(
+ type=optim_type, lr=lr, betas=betas, weight_decay=weight_decay),
+ clip_grad=dict(max_norm=max_norm, error_if_nonfinite=False),
+ accumulative_counts=accumulative_counts,
+ loss_scale='dynamic',
+ dtype='float16')
+
+# learning policy
+# More information: https://github.com/open-mmlab/mmengine/blob/main/docs/en/tutorials/param_scheduler.md # noqa: E501
+param_scheduler = [
+ dict(
+ type=LinearLR,
+ start_factor=1e-5,
+ by_epoch=True,
+ begin=0,
+ end=warmup_ratio * max_epochs,
+ convert_to_iter_based=True),
+ dict(
+ type=CosineAnnealingLR,
+ eta_min=0.0,
+ by_epoch=True,
+ begin=warmup_ratio * max_epochs,
+ end=max_epochs,
+ convert_to_iter_based=True)
+]
+
+# train, val, test setting
+train_cfg = dict(type=TrainLoop, max_epochs=max_epochs)
+
+#######################################################################
+# PART 5 Runtime #
+#######################################################################
+# Log the dialogue periodically during the training process, optional
+custom_hooks = [
+ dict(type=DatasetInfoHook, tokenizer=tokenizer),
+ dict(
+ type=EvaluateChatHook,
+ tokenizer=tokenizer,
+ every_n_iters=evaluation_freq,
+ evaluation_inputs=evaluation_inputs,
+ system=SYSTEM,
+ prompt_template=prompt_template)
+]
+
+if use_varlen_attn:
+ custom_hooks += [dict(type=VarlenAttnArgsToMessageHubHook)]
+
+# configure default hooks
+default_hooks = dict(
+ # record the time of every iteration.
+ timer=dict(type=IterTimerHook),
+ # print log every 10 iterations.
+ logger=dict(type=LoggerHook, log_metric_by_epoch=False, interval=10),
+ # enable the parameter scheduler.
+ param_scheduler=dict(type=ParamSchedulerHook),
+ # save checkpoint per `save_steps`.
+ checkpoint=dict(
+ type=CheckpointHook,
+ by_epoch=False,
+ interval=save_steps,
+ max_keep_ckpts=save_total_limit),
+ # set sampler seed in distributed evrionment.
+ sampler_seed=dict(type=DistSamplerSeedHook),
+)
+
+# configure environment
+env_cfg = dict(
+ # whether to enable cudnn benchmark
+ cudnn_benchmark=False,
+ # set multi process parameters
+ mp_cfg=dict(mp_start_method='fork', opencv_num_threads=0),
+ # set distributed parameters
+ dist_cfg=dict(backend='nccl'),
+)
+
+# set visualizer
+visualizer = None
+
+# set log level
+log_level = 'INFO'
+
+# load from which checkpoint
+load_from = None
+
+# whether to resume training from the loaded checkpoint
+resume = False
+
+# Defaults to use random seed and disable `deterministic`
+randomness = dict(seed=None, deterministic=False)
+
+# set log processor
+log_processor = dict(by_epoch=False)
diff --git a/xtuner/configs/dpo/internlm/internlm2_chat_7b_dpo_qlora_varlenattn.py b/xtuner/configs/dpo/internlm/internlm2_chat_7b_dpo_qlora_varlenattn.py
new file mode 100644
index 000000000..659d029b3
--- /dev/null
+++ b/xtuner/configs/dpo/internlm/internlm2_chat_7b_dpo_qlora_varlenattn.py
@@ -0,0 +1,230 @@
+# Copyright (c) OpenMMLab. All rights reserved.
+import torch
+from datasets import load_dataset
+from mmengine.dataset import DefaultSampler
+from mmengine.hooks import (CheckpointHook, DistSamplerSeedHook, IterTimerHook,
+ LoggerHook, ParamSchedulerHook)
+from mmengine.optim import AmpOptimWrapper, CosineAnnealingLR, LinearLR
+from peft import LoraConfig
+from torch.optim import AdamW
+from transformers import (AutoModelForCausalLM, AutoTokenizer,
+ BitsAndBytesConfig)
+
+from xtuner.dataset.collate_fns.preference_collate_fn import \
+ preference_collate_fn
+from xtuner.dataset.preference_dataset import (build_preference_dataset,
+ orpo_dpo_mix_40k_map_fn)
+from xtuner.engine.hooks import (DatasetInfoHook, EvaluateChatHook,
+ VarlenAttnArgsToMessageHubHook)
+from xtuner.engine.runner import TrainLoop
+from xtuner.model.dpo import DPO
+from xtuner.parallel.sequence import SequenceParallelSampler
+from xtuner.utils import PROMPT_TEMPLATE, SYSTEM_TEMPLATE
+
+#######################################################################
+# PART 1 Settings #
+#######################################################################
+# Model
+pretrained_model_name_or_path = 'internlm/internlm2-chat-7b-sft'
+use_varlen_attn = True
+dpo_loss_type = 'sigmoid' # One of ['sigmoid', 'hinge', 'ipo', 'kto_pair', 'sppo_hard', 'nca_pair', 'robust'] # noqa: E501
+loss_beta = 0.1
+label_smoothing = 0.0
+
+# Data
+prompt_template = PROMPT_TEMPLATE.internlm2_chat
+max_length = 2048
+max_packed_length = max_length * 2
+
+# parallel
+sequence_parallel_size = 1
+
+# Scheduler & Optimizer
+batch_size = 1 # per_device
+accumulative_counts = 16
+accumulative_counts *= sequence_parallel_size
+dataloader_num_workers = 0
+max_epochs = 3
+optim_type = AdamW
+lr = 5e-7 # refer to alignment handbook
+betas = (0.9, 0.999)
+weight_decay = 0
+max_norm = 1 # grad clip
+warmup_ratio = 0.03
+
+# Save
+save_steps = 500
+save_total_limit = 2 # Maximum checkpoints to keep (-1 means unlimited)
+
+# Evaluate the generation performance during the training
+evaluation_freq = 500
+SYSTEM = SYSTEM_TEMPLATE.alpaca
+evaluation_inputs = [
+ 'What famous British author, known for his tales of mystery and the macabre, shares his initials with a common abbreviation for "rest in peace"?', # noqa: E501
+ 'Please tell me five scenic spots in Shanghai',
+ '890729 - 425663? Only respond with math and no words.'
+]
+
+#######################################################################
+# PART 2 Model & Tokenizer #
+#######################################################################
+tokenizer = dict(
+ type=AutoTokenizer.from_pretrained,
+ pretrained_model_name_or_path=pretrained_model_name_or_path,
+ trust_remote_code=True,
+ padding_side='right')
+
+model = dict(
+ type=DPO,
+ use_varlen_attn=use_varlen_attn,
+ loss_type=dpo_loss_type,
+ beta=loss_beta,
+ label_smoothing=label_smoothing,
+ llm=dict(
+ type=AutoModelForCausalLM.from_pretrained,
+ pretrained_model_name_or_path=pretrained_model_name_or_path,
+ trust_remote_code=True,
+ torch_dtype=torch.float16,
+ quantization_config=dict(
+ type=BitsAndBytesConfig,
+ load_in_4bit=True,
+ load_in_8bit=False,
+ llm_int8_threshold=6.0,
+ llm_int8_has_fp16_weight=False,
+ bnb_4bit_compute_dtype=torch.float16,
+ bnb_4bit_use_double_quant=True,
+ bnb_4bit_quant_type='nf4')),
+ lora=dict(
+ type=LoraConfig,
+ r=64,
+ lora_alpha=16,
+ lora_dropout=0.1,
+ bias='none',
+ task_type='CAUSAL_LM'))
+#######################################################################
+# PART 3 Dataset & Dataloader #
+#######################################################################
+sampler = SequenceParallelSampler \
+ if sequence_parallel_size > 1 else DefaultSampler
+
+train_dataset = dict(
+ type=build_preference_dataset,
+ dataset=dict(type=load_dataset, path='mlabonne/orpo-dpo-mix-40k'),
+ tokenizer=tokenizer,
+ max_length=max_length,
+ dataset_map_fn=orpo_dpo_mix_40k_map_fn,
+ is_dpo=True,
+ is_reward=False,
+ reward_token_id=-1,
+ num_proc=32,
+ use_varlen_attn=use_varlen_attn,
+ max_packed_length=max_packed_length,
+ shuffle_before_pack=True,
+)
+
+train_dataloader = dict(
+ batch_size=batch_size,
+ num_workers=dataloader_num_workers,
+ dataset=train_dataset,
+ sampler=dict(type=sampler, shuffle=True),
+ collate_fn=dict(
+ type=preference_collate_fn, use_varlen_attn=use_varlen_attn))
+
+#######################################################################
+# PART 4 Scheduler & Optimizer #
+#######################################################################
+# optimizer
+optim_wrapper = dict(
+ type=AmpOptimWrapper,
+ optimizer=dict(
+ type=optim_type, lr=lr, betas=betas, weight_decay=weight_decay),
+ clip_grad=dict(max_norm=max_norm, error_if_nonfinite=False),
+ accumulative_counts=accumulative_counts,
+ loss_scale='dynamic',
+ dtype='float16')
+
+# learning policy
+# More information: https://github.com/open-mmlab/mmengine/blob/main/docs/en/tutorials/param_scheduler.md # noqa: E501
+param_scheduler = [
+ dict(
+ type=LinearLR,
+ start_factor=1e-5,
+ by_epoch=True,
+ begin=0,
+ end=warmup_ratio * max_epochs,
+ convert_to_iter_based=True),
+ dict(
+ type=CosineAnnealingLR,
+ eta_min=0.0,
+ by_epoch=True,
+ begin=warmup_ratio * max_epochs,
+ end=max_epochs,
+ convert_to_iter_based=True)
+]
+
+# train, val, test setting
+train_cfg = dict(type=TrainLoop, max_epochs=max_epochs)
+
+#######################################################################
+# PART 5 Runtime #
+#######################################################################
+# Log the dialogue periodically during the training process, optional
+custom_hooks = [
+ dict(type=DatasetInfoHook, tokenizer=tokenizer),
+ dict(
+ type=EvaluateChatHook,
+ tokenizer=tokenizer,
+ every_n_iters=evaluation_freq,
+ evaluation_inputs=evaluation_inputs,
+ system=SYSTEM,
+ prompt_template=prompt_template)
+]
+
+if use_varlen_attn:
+ custom_hooks += [dict(type=VarlenAttnArgsToMessageHubHook)]
+
+# configure default hooks
+default_hooks = dict(
+ # record the time of every iteration.
+ timer=dict(type=IterTimerHook),
+ # print log every 10 iterations.
+ logger=dict(type=LoggerHook, log_metric_by_epoch=False, interval=10),
+ # enable the parameter scheduler.
+ param_scheduler=dict(type=ParamSchedulerHook),
+ # save checkpoint per `save_steps`.
+ checkpoint=dict(
+ type=CheckpointHook,
+ by_epoch=False,
+ interval=save_steps,
+ max_keep_ckpts=save_total_limit),
+ # set sampler seed in distributed evrionment.
+ sampler_seed=dict(type=DistSamplerSeedHook),
+)
+
+# configure environment
+env_cfg = dict(
+ # whether to enable cudnn benchmark
+ cudnn_benchmark=False,
+ # set multi process parameters
+ mp_cfg=dict(mp_start_method='fork', opencv_num_threads=0),
+ # set distributed parameters
+ dist_cfg=dict(backend='nccl'),
+)
+
+# set visualizer
+visualizer = None
+
+# set log level
+log_level = 'INFO'
+
+# load from which checkpoint
+load_from = None
+
+# whether to resume training from the loaded checkpoint
+resume = False
+
+# Defaults to use random seed and disable `deterministic`
+randomness = dict(seed=None, deterministic=False)
+
+# set log processor
+log_processor = dict(by_epoch=False)
diff --git a/xtuner/configs/dpo/llama/llama3_8b_instruct_dpo_qlora_varlenattn.py b/xtuner/configs/dpo/llama/llama3_8b_instruct_dpo_qlora_varlenattn.py
new file mode 100644
index 000000000..e94b88fd0
--- /dev/null
+++ b/xtuner/configs/dpo/llama/llama3_8b_instruct_dpo_qlora_varlenattn.py
@@ -0,0 +1,230 @@
+# Copyright (c) OpenMMLab. All rights reserved.
+import torch
+from datasets import load_dataset
+from mmengine.dataset import DefaultSampler
+from mmengine.hooks import (CheckpointHook, DistSamplerSeedHook, IterTimerHook,
+ LoggerHook, ParamSchedulerHook)
+from mmengine.optim import AmpOptimWrapper, CosineAnnealingLR, LinearLR
+from peft import LoraConfig
+from torch.optim import AdamW
+from transformers import (AutoModelForCausalLM, AutoTokenizer,
+ BitsAndBytesConfig)
+
+from xtuner.dataset.collate_fns.preference_collate_fn import \
+ preference_collate_fn
+from xtuner.dataset.preference_dataset import (build_preference_dataset,
+ orpo_dpo_mix_40k_map_fn)
+from xtuner.engine.hooks import (DatasetInfoHook, EvaluateChatHook,
+ VarlenAttnArgsToMessageHubHook)
+from xtuner.engine.runner import TrainLoop
+from xtuner.model.dpo import DPO
+from xtuner.parallel.sequence import SequenceParallelSampler
+from xtuner.utils import PROMPT_TEMPLATE, SYSTEM_TEMPLATE
+
+#######################################################################
+# PART 1 Settings #
+#######################################################################
+# Model
+pretrained_model_name_or_path = 'meta-llama/Meta-Llama-3-8B-Instruct'
+use_varlen_attn = True
+dpo_loss_type = 'sigmoid' # One of ['sigmoid', 'hinge', 'ipo', 'kto_pair', 'sppo_hard', 'nca_pair', 'robust'] # noqa: E501
+loss_beta = 0.1
+label_smoothing = 0.0
+
+# Data
+prompt_template = PROMPT_TEMPLATE.llama3_chat
+max_length = 2048
+max_packed_length = max_length * 2
+
+# parallel
+sequence_parallel_size = 1
+
+# Scheduler & Optimizer
+batch_size = 1 # per_device
+accumulative_counts = 16
+accumulative_counts *= sequence_parallel_size
+dataloader_num_workers = 0
+max_epochs = 3
+optim_type = AdamW
+lr = 5e-7 # refer to alignment handbook
+betas = (0.9, 0.999)
+weight_decay = 0
+max_norm = 1 # grad clip
+warmup_ratio = 0.03
+
+# Save
+save_steps = 500
+save_total_limit = 2 # Maximum checkpoints to keep (-1 means unlimited)
+
+# Evaluate the generation performance during the training
+evaluation_freq = 500
+SYSTEM = SYSTEM_TEMPLATE.alpaca
+evaluation_inputs = [
+ 'What famous British author, known for his tales of mystery and the macabre, shares his initials with a common abbreviation for "rest in peace"?', # noqa: E501
+ 'Please tell me five scenic spots in Shanghai',
+ '890729 - 425663? Only respond with math and no words.'
+]
+
+#######################################################################
+# PART 2 Model & Tokenizer #
+#######################################################################
+tokenizer = dict(
+ type=AutoTokenizer.from_pretrained,
+ pretrained_model_name_or_path=pretrained_model_name_or_path,
+ trust_remote_code=True,
+ padding_side='right')
+
+model = dict(
+ type=DPO,
+ loss_type=dpo_loss_type,
+ use_varlen_attn=use_varlen_attn,
+ beta=loss_beta,
+ label_smoothing=label_smoothing,
+ llm=dict(
+ type=AutoModelForCausalLM.from_pretrained,
+ pretrained_model_name_or_path=pretrained_model_name_or_path,
+ trust_remote_code=True,
+ torch_dtype=torch.float16,
+ quantization_config=dict(
+ type=BitsAndBytesConfig,
+ load_in_4bit=True,
+ load_in_8bit=False,
+ llm_int8_threshold=6.0,
+ llm_int8_has_fp16_weight=False,
+ bnb_4bit_compute_dtype=torch.float16,
+ bnb_4bit_use_double_quant=True,
+ bnb_4bit_quant_type='nf4')),
+ lora=dict(
+ type=LoraConfig,
+ r=64,
+ lora_alpha=16,
+ lora_dropout=0.1,
+ bias='none',
+ task_type='CAUSAL_LM'))
+#######################################################################
+# PART 3 Dataset & Dataloader #
+#######################################################################
+sampler = SequenceParallelSampler \
+ if sequence_parallel_size > 1 else DefaultSampler
+
+train_dataset = dict(
+ type=build_preference_dataset,
+ dataset=dict(type=load_dataset, path='mlabonne/orpo-dpo-mix-40k'),
+ tokenizer=tokenizer,
+ max_length=max_length,
+ dataset_map_fn=orpo_dpo_mix_40k_map_fn,
+ is_dpo=True,
+ is_reward=False,
+ reward_token_id=-1,
+ num_proc=32,
+ use_varlen_attn=use_varlen_attn,
+ max_packed_length=max_packed_length,
+ shuffle_before_pack=True,
+)
+
+train_dataloader = dict(
+ batch_size=batch_size,
+ num_workers=dataloader_num_workers,
+ dataset=train_dataset,
+ sampler=dict(type=sampler, shuffle=True),
+ collate_fn=dict(
+ type=preference_collate_fn, use_varlen_attn=use_varlen_attn))
+
+#######################################################################
+# PART 4 Scheduler & Optimizer #
+#######################################################################
+# optimizer
+optim_wrapper = dict(
+ type=AmpOptimWrapper,
+ optimizer=dict(
+ type=optim_type, lr=lr, betas=betas, weight_decay=weight_decay),
+ clip_grad=dict(max_norm=max_norm, error_if_nonfinite=False),
+ accumulative_counts=accumulative_counts,
+ loss_scale='dynamic',
+ dtype='float16')
+
+# learning policy
+# More information: https://github.com/open-mmlab/mmengine/blob/main/docs/en/tutorials/param_scheduler.md # noqa: E501
+param_scheduler = [
+ dict(
+ type=LinearLR,
+ start_factor=1e-5,
+ by_epoch=True,
+ begin=0,
+ end=warmup_ratio * max_epochs,
+ convert_to_iter_based=True),
+ dict(
+ type=CosineAnnealingLR,
+ eta_min=0.0,
+ by_epoch=True,
+ begin=warmup_ratio * max_epochs,
+ end=max_epochs,
+ convert_to_iter_based=True)
+]
+
+# train, val, test setting
+train_cfg = dict(type=TrainLoop, max_epochs=max_epochs)
+
+#######################################################################
+# PART 5 Runtime #
+#######################################################################
+# Log the dialogue periodically during the training process, optional
+custom_hooks = [
+ dict(type=DatasetInfoHook, tokenizer=tokenizer),
+ dict(
+ type=EvaluateChatHook,
+ tokenizer=tokenizer,
+ every_n_iters=evaluation_freq,
+ evaluation_inputs=evaluation_inputs,
+ system=SYSTEM,
+ prompt_template=prompt_template)
+]
+
+if use_varlen_attn:
+ custom_hooks += [dict(type=VarlenAttnArgsToMessageHubHook)]
+
+# configure default hooks
+default_hooks = dict(
+ # record the time of every iteration.
+ timer=dict(type=IterTimerHook),
+ # print log every 10 iterations.
+ logger=dict(type=LoggerHook, log_metric_by_epoch=False, interval=10),
+ # enable the parameter scheduler.
+ param_scheduler=dict(type=ParamSchedulerHook),
+ # save checkpoint per `save_steps`.
+ checkpoint=dict(
+ type=CheckpointHook,
+ by_epoch=False,
+ interval=save_steps,
+ max_keep_ckpts=save_total_limit),
+ # set sampler seed in distributed evrionment.
+ sampler_seed=dict(type=DistSamplerSeedHook),
+)
+
+# configure environment
+env_cfg = dict(
+ # whether to enable cudnn benchmark
+ cudnn_benchmark=False,
+ # set multi process parameters
+ mp_cfg=dict(mp_start_method='fork', opencv_num_threads=0),
+ # set distributed parameters
+ dist_cfg=dict(backend='nccl'),
+)
+
+# set visualizer
+visualizer = None
+
+# set log level
+log_level = 'INFO'
+
+# load from which checkpoint
+load_from = None
+
+# whether to resume training from the loaded checkpoint
+resume = False
+
+# Defaults to use random seed and disable `deterministic`
+randomness = dict(seed=None, deterministic=False)
+
+# set log processor
+log_processor = dict(by_epoch=False)
diff --git a/xtuner/configs/internlm/internlm2_5_chat_20b/internlm2_5_chat_20b_alpaca_e3.py b/xtuner/configs/internlm/internlm2_5_chat_20b/internlm2_5_chat_20b_alpaca_e3.py
new file mode 100644
index 000000000..f67fc1a22
--- /dev/null
+++ b/xtuner/configs/internlm/internlm2_5_chat_20b/internlm2_5_chat_20b_alpaca_e3.py
@@ -0,0 +1,202 @@
+# Copyright (c) OpenMMLab. All rights reserved.
+import torch
+from datasets import load_dataset
+from mmengine.dataset import DefaultSampler
+from mmengine.hooks import (CheckpointHook, DistSamplerSeedHook, IterTimerHook,
+ LoggerHook, ParamSchedulerHook)
+from mmengine.optim import AmpOptimWrapper, CosineAnnealingLR, LinearLR
+from peft import LoraConfig
+from torch.optim import AdamW
+from transformers import (AutoModelForCausalLM, AutoTokenizer,
+ BitsAndBytesConfig)
+
+from xtuner.dataset import process_hf_dataset
+from xtuner.dataset.collate_fns import default_collate_fn
+from xtuner.dataset.map_fns import alpaca_map_fn, template_map_fn_factory
+from xtuner.engine.hooks import (DatasetInfoHook, EvaluateChatHook,
+ VarlenAttnArgsToMessageHubHook)
+from xtuner.engine.runner import TrainLoop
+from xtuner.model import SupervisedFinetune
+from xtuner.parallel.sequence import SequenceParallelSampler
+from xtuner.utils import PROMPT_TEMPLATE, SYSTEM_TEMPLATE
+
+#######################################################################
+# PART 1 Settings #
+#######################################################################
+# Model
+pretrained_model_name_or_path = 'internlm/internlm2_5-20b-chat'
+use_varlen_attn = False
+
+# Data
+alpaca_en_path = 'tatsu-lab/alpaca'
+prompt_template = PROMPT_TEMPLATE.internlm2_chat
+max_length = 2048
+pack_to_max_length = True
+
+# parallel
+sequence_parallel_size = 1
+
+# Scheduler & Optimizer
+batch_size = 1 # per_device
+accumulative_counts = 1
+accumulative_counts *= sequence_parallel_size
+dataloader_num_workers = 0
+max_epochs = 3
+optim_type = AdamW
+lr = 2e-5
+betas = (0.9, 0.999)
+weight_decay = 0
+max_norm = 1 # grad clip
+warmup_ratio = 0.03
+
+# Save
+save_steps = 500
+save_total_limit = 2 # Maximum checkpoints to keep (-1 means unlimited)
+
+# Evaluate the generation performance during the training
+evaluation_freq = 500
+SYSTEM = SYSTEM_TEMPLATE.alpaca
+evaluation_inputs = [
+ '请给我介绍五个上海的景点', 'Please tell me five scenic spots in Shanghai'
+]
+
+#######################################################################
+# PART 2 Model & Tokenizer #
+#######################################################################
+tokenizer = dict(
+ type=AutoTokenizer.from_pretrained,
+ pretrained_model_name_or_path=pretrained_model_name_or_path,
+ trust_remote_code=True,
+ padding_side='right')
+
+model = dict(
+ type=SupervisedFinetune,
+ use_varlen_attn=use_varlen_attn,
+ llm=dict(
+ type=AutoModelForCausalLM.from_pretrained,
+ pretrained_model_name_or_path=pretrained_model_name_or_path,
+ trust_remote_code=True))
+
+#######################################################################
+# PART 3 Dataset & Dataloader #
+#######################################################################
+alpaca_en = dict(
+ type=process_hf_dataset,
+ dataset=dict(type=load_dataset, path=alpaca_en_path),
+ tokenizer=tokenizer,
+ max_length=max_length,
+ dataset_map_fn=alpaca_map_fn,
+ template_map_fn=dict(
+ type=template_map_fn_factory, template=prompt_template),
+ remove_unused_columns=True,
+ shuffle_before_pack=True,
+ pack_to_max_length=pack_to_max_length,
+ use_varlen_attn=use_varlen_attn)
+
+sampler = SequenceParallelSampler \
+ if sequence_parallel_size > 1 else DefaultSampler
+train_dataloader = dict(
+ batch_size=batch_size,
+ num_workers=dataloader_num_workers,
+ dataset=alpaca_en,
+ sampler=dict(type=sampler, shuffle=True),
+ collate_fn=dict(type=default_collate_fn, use_varlen_attn=use_varlen_attn))
+
+#######################################################################
+# PART 4 Scheduler & Optimizer #
+#######################################################################
+# optimizer
+optim_wrapper = dict(
+ type=AmpOptimWrapper,
+ optimizer=dict(
+ type=optim_type, lr=lr, betas=betas, weight_decay=weight_decay),
+ clip_grad=dict(max_norm=max_norm, error_if_nonfinite=False),
+ accumulative_counts=accumulative_counts,
+ loss_scale='dynamic',
+ dtype='float16')
+
+# learning policy
+# More information: https://github.com/open-mmlab/mmengine/blob/main/docs/en/tutorials/param_scheduler.md # noqa: E501
+param_scheduler = [
+ dict(
+ type=LinearLR,
+ start_factor=1e-5,
+ by_epoch=True,
+ begin=0,
+ end=warmup_ratio * max_epochs,
+ convert_to_iter_based=True),
+ dict(
+ type=CosineAnnealingLR,
+ eta_min=0.0,
+ by_epoch=True,
+ begin=warmup_ratio * max_epochs,
+ end=max_epochs,
+ convert_to_iter_based=True)
+]
+
+# train, val, test setting
+train_cfg = dict(type=TrainLoop, max_epochs=max_epochs)
+
+#######################################################################
+# PART 5 Runtime #
+#######################################################################
+# Log the dialogue periodically during the training process, optional
+custom_hooks = [
+ dict(type=DatasetInfoHook, tokenizer=tokenizer),
+ dict(
+ type=EvaluateChatHook,
+ tokenizer=tokenizer,
+ every_n_iters=evaluation_freq,
+ evaluation_inputs=evaluation_inputs,
+ system=SYSTEM,
+ prompt_template=prompt_template)
+]
+
+if use_varlen_attn:
+ custom_hooks += [dict(type=VarlenAttnArgsToMessageHubHook)]
+
+# configure default hooks
+default_hooks = dict(
+ # record the time of every iteration.
+ timer=dict(type=IterTimerHook),
+ # print log every 10 iterations.
+ logger=dict(type=LoggerHook, log_metric_by_epoch=False, interval=10),
+ # enable the parameter scheduler.
+ param_scheduler=dict(type=ParamSchedulerHook),
+ # save checkpoint per `save_steps`.
+ checkpoint=dict(
+ type=CheckpointHook,
+ by_epoch=False,
+ interval=save_steps,
+ max_keep_ckpts=save_total_limit),
+ # set sampler seed in distributed evrionment.
+ sampler_seed=dict(type=DistSamplerSeedHook),
+)
+
+# configure environment
+env_cfg = dict(
+ # whether to enable cudnn benchmark
+ cudnn_benchmark=False,
+ # set multi process parameters
+ mp_cfg=dict(mp_start_method='fork', opencv_num_threads=0),
+ # set distributed parameters
+ dist_cfg=dict(backend='nccl'),
+)
+
+# set visualizer
+visualizer = None
+
+# set log level
+log_level = 'INFO'
+
+# load from which checkpoint
+load_from = None
+
+# whether to resume training from the loaded checkpoint
+resume = False
+
+# Defaults to use random seed and disable `deterministic`
+randomness = dict(seed=None, deterministic=False)
+
+# set log processor
+log_processor = dict(by_epoch=False)
diff --git a/xtuner/configs/internlm/internlm2_5_chat_20b/internlm2_5_chat_20b_qlora_alpaca_e3.py b/xtuner/configs/internlm/internlm2_5_chat_20b/internlm2_5_chat_20b_qlora_alpaca_e3.py
new file mode 100644
index 000000000..f695e7922
--- /dev/null
+++ b/xtuner/configs/internlm/internlm2_5_chat_20b/internlm2_5_chat_20b_qlora_alpaca_e3.py
@@ -0,0 +1,219 @@
+# Copyright (c) OpenMMLab. All rights reserved.
+import torch
+from datasets import load_dataset
+from mmengine.dataset import DefaultSampler
+from mmengine.hooks import (CheckpointHook, DistSamplerSeedHook, IterTimerHook,
+ LoggerHook, ParamSchedulerHook)
+from mmengine.optim import AmpOptimWrapper, CosineAnnealingLR, LinearLR
+from peft import LoraConfig
+from torch.optim import AdamW
+from transformers import (AutoModelForCausalLM, AutoTokenizer,
+ BitsAndBytesConfig)
+
+from xtuner.dataset import process_hf_dataset
+from xtuner.dataset.collate_fns import default_collate_fn
+from xtuner.dataset.map_fns import alpaca_map_fn, template_map_fn_factory
+from xtuner.engine.hooks import (DatasetInfoHook, EvaluateChatHook,
+ VarlenAttnArgsToMessageHubHook)
+from xtuner.engine.runner import TrainLoop
+from xtuner.model import SupervisedFinetune
+from xtuner.parallel.sequence import SequenceParallelSampler
+from xtuner.utils import PROMPT_TEMPLATE, SYSTEM_TEMPLATE
+
+#######################################################################
+# PART 1 Settings #
+#######################################################################
+# Model
+pretrained_model_name_or_path = 'internlm/internlm2_5-20b-chat'
+use_varlen_attn = False
+
+# Data
+alpaca_en_path = 'tatsu-lab/alpaca'
+prompt_template = PROMPT_TEMPLATE.internlm2_chat
+max_length = 2048
+pack_to_max_length = True
+
+# parallel
+sequence_parallel_size = 1
+
+# Scheduler & Optimizer
+batch_size = 1 # per_device
+accumulative_counts = 1
+accumulative_counts *= sequence_parallel_size
+dataloader_num_workers = 0
+max_epochs = 3
+optim_type = AdamW
+lr = 2e-4
+betas = (0.9, 0.999)
+weight_decay = 0
+max_norm = 1 # grad clip
+warmup_ratio = 0.03
+
+# Save
+save_steps = 500
+save_total_limit = 2 # Maximum checkpoints to keep (-1 means unlimited)
+
+# Evaluate the generation performance during the training
+evaluation_freq = 500
+SYSTEM = SYSTEM_TEMPLATE.alpaca
+evaluation_inputs = [
+ '请给我介绍五个上海的景点', 'Please tell me five scenic spots in Shanghai'
+]
+
+#######################################################################
+# PART 2 Model & Tokenizer #
+#######################################################################
+tokenizer = dict(
+ type=AutoTokenizer.from_pretrained,
+ pretrained_model_name_or_path=pretrained_model_name_or_path,
+ trust_remote_code=True,
+ padding_side='right')
+
+model = dict(
+ type=SupervisedFinetune,
+ use_varlen_attn=use_varlen_attn,
+ llm=dict(
+ type=AutoModelForCausalLM.from_pretrained,
+ pretrained_model_name_or_path=pretrained_model_name_or_path,
+ trust_remote_code=True,
+ torch_dtype=torch.float16,
+ quantization_config=dict(
+ type=BitsAndBytesConfig,
+ load_in_4bit=True,
+ load_in_8bit=False,
+ llm_int8_threshold=6.0,
+ llm_int8_has_fp16_weight=False,
+ bnb_4bit_compute_dtype=torch.float16,
+ bnb_4bit_use_double_quant=True,
+ bnb_4bit_quant_type='nf4')),
+ lora=dict(
+ type=LoraConfig,
+ r=64,
+ lora_alpha=16,
+ lora_dropout=0.1,
+ bias='none',
+ task_type='CAUSAL_LM'))
+
+#######################################################################
+# PART 3 Dataset & Dataloader #
+#######################################################################
+alpaca_en = dict(
+ type=process_hf_dataset,
+ dataset=dict(type=load_dataset, path=alpaca_en_path),
+ tokenizer=tokenizer,
+ max_length=max_length,
+ dataset_map_fn=alpaca_map_fn,
+ template_map_fn=dict(
+ type=template_map_fn_factory, template=prompt_template),
+ remove_unused_columns=True,
+ shuffle_before_pack=True,
+ pack_to_max_length=pack_to_max_length,
+ use_varlen_attn=use_varlen_attn)
+
+sampler = SequenceParallelSampler \
+ if sequence_parallel_size > 1 else DefaultSampler
+train_dataloader = dict(
+ batch_size=batch_size,
+ num_workers=dataloader_num_workers,
+ dataset=alpaca_en,
+ sampler=dict(type=sampler, shuffle=True),
+ collate_fn=dict(type=default_collate_fn, use_varlen_attn=use_varlen_attn))
+
+#######################################################################
+# PART 4 Scheduler & Optimizer #
+#######################################################################
+# optimizer
+optim_wrapper = dict(
+ type=AmpOptimWrapper,
+ optimizer=dict(
+ type=optim_type, lr=lr, betas=betas, weight_decay=weight_decay),
+ clip_grad=dict(max_norm=max_norm, error_if_nonfinite=False),
+ accumulative_counts=accumulative_counts,
+ loss_scale='dynamic',
+ dtype='float16')
+
+# learning policy
+# More information: https://github.com/open-mmlab/mmengine/blob/main/docs/en/tutorials/param_scheduler.md # noqa: E501
+param_scheduler = [
+ dict(
+ type=LinearLR,
+ start_factor=1e-5,
+ by_epoch=True,
+ begin=0,
+ end=warmup_ratio * max_epochs,
+ convert_to_iter_based=True),
+ dict(
+ type=CosineAnnealingLR,
+ eta_min=0.0,
+ by_epoch=True,
+ begin=warmup_ratio * max_epochs,
+ end=max_epochs,
+ convert_to_iter_based=True)
+]
+
+# train, val, test setting
+train_cfg = dict(type=TrainLoop, max_epochs=max_epochs)
+
+#######################################################################
+# PART 5 Runtime #
+#######################################################################
+# Log the dialogue periodically during the training process, optional
+custom_hooks = [
+ dict(type=DatasetInfoHook, tokenizer=tokenizer),
+ dict(
+ type=EvaluateChatHook,
+ tokenizer=tokenizer,
+ every_n_iters=evaluation_freq,
+ evaluation_inputs=evaluation_inputs,
+ system=SYSTEM,
+ prompt_template=prompt_template)
+]
+
+if use_varlen_attn:
+ custom_hooks += [dict(type=VarlenAttnArgsToMessageHubHook)]
+
+# configure default hooks
+default_hooks = dict(
+ # record the time of every iteration.
+ timer=dict(type=IterTimerHook),
+ # print log every 10 iterations.
+ logger=dict(type=LoggerHook, log_metric_by_epoch=False, interval=10),
+ # enable the parameter scheduler.
+ param_scheduler=dict(type=ParamSchedulerHook),
+ # save checkpoint per `save_steps`.
+ checkpoint=dict(
+ type=CheckpointHook,
+ by_epoch=False,
+ interval=save_steps,
+ max_keep_ckpts=save_total_limit),
+ # set sampler seed in distributed evrionment.
+ sampler_seed=dict(type=DistSamplerSeedHook),
+)
+
+# configure environment
+env_cfg = dict(
+ # whether to enable cudnn benchmark
+ cudnn_benchmark=False,
+ # set multi process parameters
+ mp_cfg=dict(mp_start_method='fork', opencv_num_threads=0),
+ # set distributed parameters
+ dist_cfg=dict(backend='nccl'),
+)
+
+# set visualizer
+visualizer = None
+
+# set log level
+log_level = 'INFO'
+
+# load from which checkpoint
+load_from = None
+
+# whether to resume training from the loaded checkpoint
+resume = False
+
+# Defaults to use random seed and disable `deterministic`
+randomness = dict(seed=None, deterministic=False)
+
+# set log processor
+log_processor = dict(by_epoch=False)
diff --git a/xtuner/configs/internlm/internlm2_5_chat_7b/internlm2_5_chat_7b_full_finetune_custom_dataset_e1.py b/xtuner/configs/internlm/internlm2_5_chat_7b/internlm2_5_chat_7b_full_finetune_custom_dataset_e1.py
new file mode 100644
index 000000000..bc8a2816a
--- /dev/null
+++ b/xtuner/configs/internlm/internlm2_5_chat_7b/internlm2_5_chat_7b_full_finetune_custom_dataset_e1.py
@@ -0,0 +1,226 @@
+# Copyright (c) OpenMMLab. All rights reserved.
+"""Data format:
+[
+ {
+ "conversation": [
+ {
+ "system": "",
+ "input": "xxx",
+ "output": "xxx"
+ },
+ {
+ "input": "xxx",
+ "output": "xxx"
+ }
+ ]
+ },
+...
+]
+Please refer to https://github.com/InternLM/xtuner/blob/main/docs/en/user_guides/dataset_format.md for details.
+""" # noqa: E501
+from datasets import load_dataset
+from mmengine.hooks import (CheckpointHook, DistSamplerSeedHook, IterTimerHook,
+ LoggerHook, ParamSchedulerHook)
+from mmengine.optim import AmpOptimWrapper, CosineAnnealingLR
+from torch.optim import AdamW
+from torch.utils.data import BatchSampler
+from transformers import AutoModelForCausalLM, AutoTokenizer
+
+from xtuner.dataset import process_hf_dataset
+from xtuner.dataset.collate_fns import default_collate_fn
+from xtuner.dataset.map_fns import template_map_fn_factory
+from xtuner.dataset.samplers import InternRepoSampler
+from xtuner.engine import (DatasetInfoHook, EvaluateChatHook, ThroughputHook,
+ VarlenAttnArgsToMessageHubHook)
+from xtuner.engine.runner import TrainLoop
+from xtuner.model import SupervisedFinetune
+from xtuner.utils import PROMPT_TEMPLATE
+
+#######################################################################
+# PART 1 Settings #
+#######################################################################
+# Model
+pretrained_model_name_or_path = 'internlm/internlm2_5-7b-chat'
+use_varlen_attn = True
+
+# Data
+data_files = ['/path/to/json/file.json']
+prompt_template = PROMPT_TEMPLATE.internlm2_chat
+max_length = 32768
+pack_to_max_length = True
+
+# parallel
+sequence_parallel_size = 1
+
+# Scheduler & Optimizer
+# batch size per device, set to 1 if `use_varlen_attn` = True
+# To clarify, enlarging the batch size essentially enlarges the `max_length`.
+# For example, doubling the max length is tantamount to doubling the batch size
+batch_size = 1
+accumulative_counts = 1 # 1bs * 1acc * 64gpu = 64 batchsize
+accumulative_counts *= sequence_parallel_size
+dataloader_num_workers = 4
+max_epochs = 1
+optim_type = AdamW
+lr = 4e-5
+betas = (0.9, 0.95)
+weight_decay = 0.01
+max_norm = 1 # grad clip
+warm_up_ratio = 0.025
+
+# Save
+save_steps = 500
+save_total_limit = 2 # Maximum checkpoints to keep (-1 means unlimited)
+
+# Evaluate the generation performance during the training
+evaluation_freq = 500
+SYSTEM = ''
+evaluation_inputs = [
+ '请给我介绍五个上海的景点', 'Please tell me five scenic spots in Shanghai'
+]
+
+#######################################################################
+# PART 2 Model & Tokenizer #
+#######################################################################
+tokenizer = dict(
+ type=AutoTokenizer.from_pretrained,
+ pretrained_model_name_or_path=pretrained_model_name_or_path,
+ trust_remote_code=True,
+ padding_side='right')
+
+model = dict(
+ type=SupervisedFinetune,
+ use_varlen_attn=use_varlen_attn,
+ llm=dict(
+ type=AutoModelForCausalLM.from_pretrained,
+ pretrained_model_name_or_path=pretrained_model_name_or_path,
+ trust_remote_code=True))
+
+#######################################################################
+# PART 3 Dataset & Dataloader #
+#######################################################################
+train_dataset = dict(
+ type=process_hf_dataset,
+ use_varlen_attn=use_varlen_attn,
+ dataset=dict(type=load_dataset, path='json', data_files=data_files),
+ tokenizer=tokenizer,
+ max_length=max_length,
+ dataset_map_fn=None,
+ template_map_fn=dict(
+ type=template_map_fn_factory, template=prompt_template),
+ remove_unused_columns=True,
+ shuffle_before_pack=True,
+ pack_to_max_length=pack_to_max_length)
+
+train_dataloader = dict(
+ batch_size=batch_size,
+ num_workers=dataloader_num_workers,
+ dataset=train_dataset,
+ sampler=dict(type=InternRepoSampler, shuffle=True, seed=1024),
+ batch_sampler=dict(
+ type=BatchSampler, drop_last=True, batch_size=batch_size),
+ collate_fn=dict(type=default_collate_fn, use_varlen_attn=use_varlen_attn))
+
+#######################################################################
+# PART 4 Scheduler & Optimizer #
+#######################################################################
+# optimizer
+optim_wrapper = dict(
+ type=AmpOptimWrapper,
+ optimizer=dict(
+ type=optim_type, lr=lr, betas=betas, weight_decay=weight_decay),
+ clip_grad=dict(max_norm=max_norm, error_if_nonfinite=False),
+ accumulative_counts=accumulative_counts,
+ loss_scale='dynamic',
+)
+
+# learning policy
+# More information: https://github.com/open-mmlab/mmengine/blob/main/docs/en/tutorials/param_scheduler.md # noqa: E501
+param_scheduler = [
+ dict(
+ type='LinearLR',
+ start_factor=1 / 40,
+ by_epoch=True,
+ begin=0,
+ end=warm_up_ratio * max_epochs,
+ convert_to_iter_based=True),
+ dict(
+ type=CosineAnnealingLR,
+ eta_min=lr * 0.15,
+ by_epoch=True,
+ begin=warm_up_ratio * max_epochs,
+ end=max_epochs,
+ convert_to_iter_based=True)
+]
+
+# train, val, test setting
+train_cfg = dict(type=TrainLoop, max_epochs=max_epochs)
+
+#######################################################################
+# PART 5 Runtime #
+#######################################################################
+# Log the dialogue periodically during the training process, optional
+custom_hooks = [
+ dict(
+ type=DatasetInfoHook, tokenizer=tokenizer,
+ is_intern_repo_dataset=True),
+ dict(
+ type=EvaluateChatHook,
+ tokenizer=tokenizer,
+ every_n_iters=evaluation_freq,
+ evaluation_inputs=evaluation_inputs,
+ system=SYSTEM,
+ prompt_template=prompt_template),
+ dict(type=ThroughputHook)
+]
+
+if use_varlen_attn:
+ custom_hooks += [dict(type=VarlenAttnArgsToMessageHubHook)]
+
+# configure default hooks
+default_hooks = dict(
+ # record the time of every iteration.
+ timer=dict(type=IterTimerHook),
+ # print log every 100 iterations.
+ logger=dict(type=LoggerHook, log_metric_by_epoch=False, interval=1),
+ # enable the parameter scheduler.
+ param_scheduler=dict(type=ParamSchedulerHook),
+ # save checkpoint per `save_steps`.
+ checkpoint=dict(
+ type=CheckpointHook,
+ by_epoch=False,
+ interval=save_steps,
+ max_keep_ckpts=save_total_limit),
+ # set sampler seed in distributed evrionment.
+ sampler_seed=dict(type=DistSamplerSeedHook),
+)
+
+# configure environment
+env_cfg = dict(
+ # whether to enable cudnn benchmark
+ cudnn_benchmark=False,
+ # set multi process parameters
+ mp_cfg=dict(mp_start_method='fork', opencv_num_threads=0),
+ # set distributed parameters
+ dist_cfg=dict(backend='nccl'),
+)
+
+# set visualizer
+visualizer = None
+
+# set log level
+log_level = 'INFO'
+
+# load from which checkpoint
+load_from = None
+
+# whether to resume training from the loaded checkpoint
+resume = False
+
+# Defaults to use random seed and disable `deterministic`
+randomness = dict(seed=None, deterministic=False)
+
+log_processor = dict(
+ by_epoch=False,
+ window_size=1,
+ mean_pattern=r'.*(loss|time|data_time|grad_norm|tflops).*')
diff --git a/xtuner/configs/internlm/internlm2_5_chat_7b/internlm2_5_chat_7b_qlora_alpaca_e3.py b/xtuner/configs/internlm/internlm2_5_chat_7b/internlm2_5_chat_7b_qlora_alpaca_e3.py
new file mode 100644
index 000000000..7dfc92617
--- /dev/null
+++ b/xtuner/configs/internlm/internlm2_5_chat_7b/internlm2_5_chat_7b_qlora_alpaca_e3.py
@@ -0,0 +1,219 @@
+# Copyright (c) OpenMMLab. All rights reserved.
+import torch
+from datasets import load_dataset
+from mmengine.dataset import DefaultSampler
+from mmengine.hooks import (CheckpointHook, DistSamplerSeedHook, IterTimerHook,
+ LoggerHook, ParamSchedulerHook)
+from mmengine.optim import AmpOptimWrapper, CosineAnnealingLR, LinearLR
+from peft import LoraConfig
+from torch.optim import AdamW
+from transformers import (AutoModelForCausalLM, AutoTokenizer,
+ BitsAndBytesConfig)
+
+from xtuner.dataset import process_hf_dataset
+from xtuner.dataset.collate_fns import default_collate_fn
+from xtuner.dataset.map_fns import alpaca_map_fn, template_map_fn_factory
+from xtuner.engine.hooks import (DatasetInfoHook, EvaluateChatHook,
+ VarlenAttnArgsToMessageHubHook)
+from xtuner.engine.runner import TrainLoop
+from xtuner.model import SupervisedFinetune
+from xtuner.parallel.sequence import SequenceParallelSampler
+from xtuner.utils import PROMPT_TEMPLATE, SYSTEM_TEMPLATE
+
+#######################################################################
+# PART 1 Settings #
+#######################################################################
+# Model
+pretrained_model_name_or_path = 'internlm/internlm2_5-7b-chat'
+use_varlen_attn = False
+
+# Data
+alpaca_en_path = 'tatsu-lab/alpaca'
+prompt_template = PROMPT_TEMPLATE.internlm2_chat
+max_length = 2048
+pack_to_max_length = True
+
+# parallel
+sequence_parallel_size = 1
+
+# Scheduler & Optimizer
+batch_size = 1 # per_device
+accumulative_counts = 1
+accumulative_counts *= sequence_parallel_size
+dataloader_num_workers = 0
+max_epochs = 3
+optim_type = AdamW
+lr = 2e-4
+betas = (0.9, 0.999)
+weight_decay = 0
+max_norm = 1 # grad clip
+warmup_ratio = 0.03
+
+# Save
+save_steps = 500
+save_total_limit = 2 # Maximum checkpoints to keep (-1 means unlimited)
+
+# Evaluate the generation performance during the training
+evaluation_freq = 500
+SYSTEM = SYSTEM_TEMPLATE.alpaca
+evaluation_inputs = [
+ '请给我介绍五个上海的景点', 'Please tell me five scenic spots in Shanghai'
+]
+
+#######################################################################
+# PART 2 Model & Tokenizer #
+#######################################################################
+tokenizer = dict(
+ type=AutoTokenizer.from_pretrained,
+ pretrained_model_name_or_path=pretrained_model_name_or_path,
+ trust_remote_code=True,
+ padding_side='right')
+
+model = dict(
+ type=SupervisedFinetune,
+ use_varlen_attn=use_varlen_attn,
+ llm=dict(
+ type=AutoModelForCausalLM.from_pretrained,
+ pretrained_model_name_or_path=pretrained_model_name_or_path,
+ trust_remote_code=True,
+ torch_dtype=torch.float16,
+ quantization_config=dict(
+ type=BitsAndBytesConfig,
+ load_in_4bit=True,
+ load_in_8bit=False,
+ llm_int8_threshold=6.0,
+ llm_int8_has_fp16_weight=False,
+ bnb_4bit_compute_dtype=torch.float16,
+ bnb_4bit_use_double_quant=True,
+ bnb_4bit_quant_type='nf4')),
+ lora=dict(
+ type=LoraConfig,
+ r=64,
+ lora_alpha=16,
+ lora_dropout=0.1,
+ bias='none',
+ task_type='CAUSAL_LM'))
+
+#######################################################################
+# PART 3 Dataset & Dataloader #
+#######################################################################
+alpaca_en = dict(
+ type=process_hf_dataset,
+ dataset=dict(type=load_dataset, path=alpaca_en_path),
+ tokenizer=tokenizer,
+ max_length=max_length,
+ dataset_map_fn=alpaca_map_fn,
+ template_map_fn=dict(
+ type=template_map_fn_factory, template=prompt_template),
+ remove_unused_columns=True,
+ shuffle_before_pack=True,
+ pack_to_max_length=pack_to_max_length,
+ use_varlen_attn=use_varlen_attn)
+
+sampler = SequenceParallelSampler \
+ if sequence_parallel_size > 1 else DefaultSampler
+train_dataloader = dict(
+ batch_size=batch_size,
+ num_workers=dataloader_num_workers,
+ dataset=alpaca_en,
+ sampler=dict(type=sampler, shuffle=True),
+ collate_fn=dict(type=default_collate_fn, use_varlen_attn=use_varlen_attn))
+
+#######################################################################
+# PART 4 Scheduler & Optimizer #
+#######################################################################
+# optimizer
+optim_wrapper = dict(
+ type=AmpOptimWrapper,
+ optimizer=dict(
+ type=optim_type, lr=lr, betas=betas, weight_decay=weight_decay),
+ clip_grad=dict(max_norm=max_norm, error_if_nonfinite=False),
+ accumulative_counts=accumulative_counts,
+ loss_scale='dynamic',
+ dtype='float16')
+
+# learning policy
+# More information: https://github.com/open-mmlab/mmengine/blob/main/docs/en/tutorials/param_scheduler.md # noqa: E501
+param_scheduler = [
+ dict(
+ type=LinearLR,
+ start_factor=1e-5,
+ by_epoch=True,
+ begin=0,
+ end=warmup_ratio * max_epochs,
+ convert_to_iter_based=True),
+ dict(
+ type=CosineAnnealingLR,
+ eta_min=0.0,
+ by_epoch=True,
+ begin=warmup_ratio * max_epochs,
+ end=max_epochs,
+ convert_to_iter_based=True)
+]
+
+# train, val, test setting
+train_cfg = dict(type=TrainLoop, max_epochs=max_epochs)
+
+#######################################################################
+# PART 5 Runtime #
+#######################################################################
+# Log the dialogue periodically during the training process, optional
+custom_hooks = [
+ dict(type=DatasetInfoHook, tokenizer=tokenizer),
+ dict(
+ type=EvaluateChatHook,
+ tokenizer=tokenizer,
+ every_n_iters=evaluation_freq,
+ evaluation_inputs=evaluation_inputs,
+ system=SYSTEM,
+ prompt_template=prompt_template)
+]
+
+if use_varlen_attn:
+ custom_hooks += [dict(type=VarlenAttnArgsToMessageHubHook)]
+
+# configure default hooks
+default_hooks = dict(
+ # record the time of every iteration.
+ timer=dict(type=IterTimerHook),
+ # print log every 10 iterations.
+ logger=dict(type=LoggerHook, log_metric_by_epoch=False, interval=10),
+ # enable the parameter scheduler.
+ param_scheduler=dict(type=ParamSchedulerHook),
+ # save checkpoint per `save_steps`.
+ checkpoint=dict(
+ type=CheckpointHook,
+ by_epoch=False,
+ interval=save_steps,
+ max_keep_ckpts=save_total_limit),
+ # set sampler seed in distributed evrionment.
+ sampler_seed=dict(type=DistSamplerSeedHook),
+)
+
+# configure environment
+env_cfg = dict(
+ # whether to enable cudnn benchmark
+ cudnn_benchmark=False,
+ # set multi process parameters
+ mp_cfg=dict(mp_start_method='fork', opencv_num_threads=0),
+ # set distributed parameters
+ dist_cfg=dict(backend='nccl'),
+)
+
+# set visualizer
+visualizer = None
+
+# set log level
+log_level = 'INFO'
+
+# load from which checkpoint
+load_from = None
+
+# whether to resume training from the loaded checkpoint
+resume = False
+
+# Defaults to use random seed and disable `deterministic`
+randomness = dict(seed=None, deterministic=False)
+
+# set log processor
+log_processor = dict(by_epoch=False)
diff --git a/xtuner/configs/internlm/internlm2_5_chat_7b/internlm2_5_chat_7b_qlora_oasst1_e3.py b/xtuner/configs/internlm/internlm2_5_chat_7b/internlm2_5_chat_7b_qlora_oasst1_e3.py
new file mode 100644
index 000000000..98b097efb
--- /dev/null
+++ b/xtuner/configs/internlm/internlm2_5_chat_7b/internlm2_5_chat_7b_qlora_oasst1_e3.py
@@ -0,0 +1,219 @@
+# Copyright (c) OpenMMLab. All rights reserved.
+import torch
+from datasets import load_dataset
+from mmengine.dataset import DefaultSampler
+from mmengine.hooks import (CheckpointHook, DistSamplerSeedHook, IterTimerHook,
+ LoggerHook, ParamSchedulerHook)
+from mmengine.optim import AmpOptimWrapper, CosineAnnealingLR, LinearLR
+from peft import LoraConfig
+from torch.optim import AdamW
+from transformers import (AutoModelForCausalLM, AutoTokenizer,
+ BitsAndBytesConfig)
+
+from xtuner.dataset import process_hf_dataset
+from xtuner.dataset.collate_fns import default_collate_fn
+from xtuner.dataset.map_fns import oasst1_map_fn, template_map_fn_factory
+from xtuner.engine.hooks import (DatasetInfoHook, EvaluateChatHook,
+ VarlenAttnArgsToMessageHubHook)
+from xtuner.engine.runner import TrainLoop
+from xtuner.model import SupervisedFinetune
+from xtuner.parallel.sequence import SequenceParallelSampler
+from xtuner.utils import PROMPT_TEMPLATE
+
+#######################################################################
+# PART 1 Settings #
+#######################################################################
+# Model
+pretrained_model_name_or_path = 'internlm/internlm2_5-7b-chat'
+use_varlen_attn = False
+
+# Data
+data_path = 'timdettmers/openassistant-guanaco'
+prompt_template = PROMPT_TEMPLATE.internlm2_chat
+max_length = 2048
+pack_to_max_length = True
+
+# parallel
+sequence_parallel_size = 1
+
+# Scheduler & Optimizer
+batch_size = 1 # per_device
+accumulative_counts = 16
+accumulative_counts *= sequence_parallel_size
+dataloader_num_workers = 0
+max_epochs = 3
+optim_type = AdamW
+lr = 2e-4
+betas = (0.9, 0.999)
+weight_decay = 0
+max_norm = 1 # grad clip
+warmup_ratio = 0.03
+
+# Save
+save_steps = 500
+save_total_limit = 2 # Maximum checkpoints to keep (-1 means unlimited)
+
+# Evaluate the generation performance during the training
+evaluation_freq = 500
+SYSTEM = ''
+evaluation_inputs = [
+ '请给我介绍五个上海的景点', 'Please tell me five scenic spots in Shanghai'
+]
+
+#######################################################################
+# PART 2 Model & Tokenizer #
+#######################################################################
+tokenizer = dict(
+ type=AutoTokenizer.from_pretrained,
+ pretrained_model_name_or_path=pretrained_model_name_or_path,
+ trust_remote_code=True,
+ padding_side='right')
+
+model = dict(
+ type=SupervisedFinetune,
+ use_varlen_attn=use_varlen_attn,
+ llm=dict(
+ type=AutoModelForCausalLM.from_pretrained,
+ pretrained_model_name_or_path=pretrained_model_name_or_path,
+ trust_remote_code=True,
+ torch_dtype=torch.float16,
+ quantization_config=dict(
+ type=BitsAndBytesConfig,
+ load_in_4bit=True,
+ load_in_8bit=False,
+ llm_int8_threshold=6.0,
+ llm_int8_has_fp16_weight=False,
+ bnb_4bit_compute_dtype=torch.float16,
+ bnb_4bit_use_double_quant=True,
+ bnb_4bit_quant_type='nf4')),
+ lora=dict(
+ type=LoraConfig,
+ r=64,
+ lora_alpha=16,
+ lora_dropout=0.1,
+ bias='none',
+ task_type='CAUSAL_LM'))
+
+#######################################################################
+# PART 3 Dataset & Dataloader #
+#######################################################################
+train_dataset = dict(
+ type=process_hf_dataset,
+ dataset=dict(type=load_dataset, path=data_path),
+ tokenizer=tokenizer,
+ max_length=max_length,
+ dataset_map_fn=oasst1_map_fn,
+ template_map_fn=dict(
+ type=template_map_fn_factory, template=prompt_template),
+ remove_unused_columns=True,
+ shuffle_before_pack=True,
+ pack_to_max_length=pack_to_max_length,
+ use_varlen_attn=use_varlen_attn)
+
+sampler = SequenceParallelSampler \
+ if sequence_parallel_size > 1 else DefaultSampler
+train_dataloader = dict(
+ batch_size=batch_size,
+ num_workers=dataloader_num_workers,
+ dataset=train_dataset,
+ sampler=dict(type=sampler, shuffle=True),
+ collate_fn=dict(type=default_collate_fn, use_varlen_attn=use_varlen_attn))
+
+#######################################################################
+# PART 4 Scheduler & Optimizer #
+#######################################################################
+# optimizer
+optim_wrapper = dict(
+ type=AmpOptimWrapper,
+ optimizer=dict(
+ type=optim_type, lr=lr, betas=betas, weight_decay=weight_decay),
+ clip_grad=dict(max_norm=max_norm, error_if_nonfinite=False),
+ accumulative_counts=accumulative_counts,
+ loss_scale='dynamic',
+ dtype='float16')
+
+# learning policy
+# More information: https://github.com/open-mmlab/mmengine/blob/main/docs/en/tutorials/param_scheduler.md # noqa: E501
+param_scheduler = [
+ dict(
+ type=LinearLR,
+ start_factor=1e-5,
+ by_epoch=True,
+ begin=0,
+ end=warmup_ratio * max_epochs,
+ convert_to_iter_based=True),
+ dict(
+ type=CosineAnnealingLR,
+ eta_min=0.0,
+ by_epoch=True,
+ begin=warmup_ratio * max_epochs,
+ end=max_epochs,
+ convert_to_iter_based=True)
+]
+
+# train, val, test setting
+train_cfg = dict(type=TrainLoop, max_epochs=max_epochs)
+
+#######################################################################
+# PART 5 Runtime #
+#######################################################################
+# Log the dialogue periodically during the training process, optional
+custom_hooks = [
+ dict(type=DatasetInfoHook, tokenizer=tokenizer),
+ dict(
+ type=EvaluateChatHook,
+ tokenizer=tokenizer,
+ every_n_iters=evaluation_freq,
+ evaluation_inputs=evaluation_inputs,
+ system=SYSTEM,
+ prompt_template=prompt_template)
+]
+
+if use_varlen_attn:
+ custom_hooks += [dict(type=VarlenAttnArgsToMessageHubHook)]
+
+# configure default hooks
+default_hooks = dict(
+ # record the time of every iteration.
+ timer=dict(type=IterTimerHook),
+ # print log every 10 iterations.
+ logger=dict(type=LoggerHook, log_metric_by_epoch=False, interval=10),
+ # enable the parameter scheduler.
+ param_scheduler=dict(type=ParamSchedulerHook),
+ # save checkpoint per `save_steps`.
+ checkpoint=dict(
+ type=CheckpointHook,
+ by_epoch=False,
+ interval=save_steps,
+ max_keep_ckpts=save_total_limit),
+ # set sampler seed in distributed evrionment.
+ sampler_seed=dict(type=DistSamplerSeedHook),
+)
+
+# configure environment
+env_cfg = dict(
+ # whether to enable cudnn benchmark
+ cudnn_benchmark=False,
+ # set multi process parameters
+ mp_cfg=dict(mp_start_method='fork', opencv_num_threads=0),
+ # set distributed parameters
+ dist_cfg=dict(backend='nccl'),
+)
+
+# set visualizer
+visualizer = None
+
+# set log level
+log_level = 'INFO'
+
+# load from which checkpoint
+load_from = None
+
+# whether to resume training from the loaded checkpoint
+resume = False
+
+# Defaults to use random seed and disable `deterministic`
+randomness = dict(seed=None, deterministic=False)
+
+# set log processor
+log_processor = dict(by_epoch=False)
diff --git a/xtuner/configs/internlm/internlm2_7b/internlm2_7b_w_internevo_dataset.py b/xtuner/configs/internlm/internlm2_7b/internlm2_7b_w_internevo_dataset.py
new file mode 100644
index 000000000..de45284b3
--- /dev/null
+++ b/xtuner/configs/internlm/internlm2_7b/internlm2_7b_w_internevo_dataset.py
@@ -0,0 +1,196 @@
+# Copyright (c) OpenMMLab. All rights reserved.
+from mmengine.hooks import (CheckpointHook, DistSamplerSeedHook, IterTimerHook,
+ LoggerHook, ParamSchedulerHook)
+from mmengine.optim import AmpOptimWrapper, CosineAnnealingLR
+from torch.optim import AdamW
+from torch.utils.data import BatchSampler
+from transformers import AutoModelForCausalLM, AutoTokenizer
+
+from xtuner.dataset.collate_fns import default_collate_fn
+from xtuner.dataset.intern_repo import (build_packed_dataset,
+ load_intern_repo_tokenized_dataset)
+from xtuner.dataset.samplers import InternRepoSampler
+from xtuner.engine import (DatasetInfoHook, EvaluateChatHook, ThroughputHook,
+ VarlenAttnArgsToMessageHubHook)
+from xtuner.engine.runner import TrainLoop
+from xtuner.model import SupervisedFinetune
+from xtuner.utils import PROMPT_TEMPLATE
+
+#######################################################################
+# PART 1 Settings #
+#######################################################################
+# Model
+pretrained_model_name_or_path = 'internlm/internlm2-7b'
+use_varlen_attn = True
+
+# Data
+dataset_folder = '/path/to/sft/data/folder' # noqa: E501
+prompt_template = PROMPT_TEMPLATE.internlm2_chat
+max_length = 32768
+pack_to_max_length = True
+
+# Scheduler & Optimizer
+batch_size = 1 # per_device
+accumulative_counts = 1 # 1bs * 1acc * 64gpu = 64 batchsize
+dataloader_num_workers = 4
+max_epochs = 1
+optim_type = AdamW
+lr = 4e-5
+betas = (0.9, 0.95)
+weight_decay = 0.01
+max_norm = 1 # grad clip
+warm_up_ratio = 0.025
+
+# Save
+save_steps = 500
+save_total_limit = 2 # Maximum checkpoints to keep (-1 means unlimited)
+
+# Evaluate the generation performance during the training
+evaluation_freq = 500
+SYSTEM = ''
+evaluation_inputs = [
+ '请给我介绍五个上海的景点', 'Please tell me five scenic spots in Shanghai'
+]
+
+#######################################################################
+# PART 2 Model & Tokenizer #
+#######################################################################
+tokenizer = dict(
+ type=AutoTokenizer.from_pretrained,
+ pretrained_model_name_or_path=pretrained_model_name_or_path,
+ trust_remote_code=True,
+ padding_side='right')
+
+model = dict(
+ type=SupervisedFinetune,
+ use_varlen_attn=use_varlen_attn,
+ llm=dict(
+ type=AutoModelForCausalLM.from_pretrained,
+ pretrained_model_name_or_path=pretrained_model_name_or_path,
+ trust_remote_code=True))
+
+#######################################################################
+# PART 3 Dataset & Dataloader #
+#######################################################################
+train_dataset = dict(
+ type=build_packed_dataset,
+ dataset_cfg=dict(
+ type=load_intern_repo_tokenized_dataset,
+ data_order_path=None,
+ folder=dataset_folder,
+ min_length=0,
+ file_type='.bin'),
+ packed_length=max_length,
+ seed=1024)
+
+train_dataloader = dict(
+ batch_size=batch_size,
+ num_workers=dataloader_num_workers,
+ dataset=train_dataset,
+ sampler=dict(type=InternRepoSampler, shuffle=True, seed=1024),
+ batch_sampler=dict(type=BatchSampler, drop_last=True, batch_size=1),
+ collate_fn=dict(type=default_collate_fn, use_varlen_attn=use_varlen_attn))
+
+#######################################################################
+# PART 4 Scheduler & Optimizer #
+#######################################################################
+# optimizer
+optim_wrapper = dict(
+ type=AmpOptimWrapper,
+ optimizer=dict(
+ type=optim_type, lr=lr, betas=betas, weight_decay=weight_decay),
+ clip_grad=dict(max_norm=max_norm, error_if_nonfinite=False),
+ accumulative_counts=accumulative_counts,
+ loss_scale='dynamic',
+)
+
+# learning policy
+# More information: https://github.com/open-mmlab/mmengine/blob/main/docs/en/tutorials/param_scheduler.md # noqa: E501
+param_scheduler = [
+ dict(
+ type='LinearLR',
+ start_factor=1 / 40,
+ by_epoch=True,
+ begin=0,
+ end=warm_up_ratio * max_epochs,
+ convert_to_iter_based=True),
+ dict(
+ type=CosineAnnealingLR,
+ eta_min=lr * 0.15,
+ by_epoch=True,
+ begin=warm_up_ratio * max_epochs,
+ end=max_epochs,
+ convert_to_iter_based=True)
+]
+
+# train, val, test setting
+train_cfg = dict(type=TrainLoop, max_epochs=max_epochs)
+
+#######################################################################
+# PART 5 Runtime #
+#######################################################################
+# Log the dialogue periodically during the training process, optional
+custom_hooks = [
+ dict(
+ type=DatasetInfoHook, tokenizer=tokenizer,
+ is_intern_repo_dataset=True),
+ dict(
+ type=EvaluateChatHook,
+ tokenizer=tokenizer,
+ every_n_iters=evaluation_freq,
+ evaluation_inputs=evaluation_inputs,
+ system=SYSTEM,
+ prompt_template=prompt_template),
+ dict(type=ThroughputHook)
+]
+
+if use_varlen_attn:
+ custom_hooks += [dict(type=VarlenAttnArgsToMessageHubHook)]
+
+# configure default hooks
+default_hooks = dict(
+ # record the time of every iteration.
+ timer=dict(type=IterTimerHook),
+ # print log every 100 iterations.
+ logger=dict(type=LoggerHook, log_metric_by_epoch=False, interval=1),
+ # enable the parameter scheduler.
+ param_scheduler=dict(type=ParamSchedulerHook),
+ # save checkpoint per `save_steps`.
+ checkpoint=dict(
+ type=CheckpointHook,
+ by_epoch=False,
+ interval=save_steps,
+ max_keep_ckpts=save_total_limit),
+ # set sampler seed in distributed evrionment.
+ sampler_seed=dict(type=DistSamplerSeedHook),
+)
+
+# configure environment
+env_cfg = dict(
+ # whether to enable cudnn benchmark
+ cudnn_benchmark=False,
+ # set multi process parameters
+ mp_cfg=dict(mp_start_method='fork', opencv_num_threads=0),
+ # set distributed parameters
+ dist_cfg=dict(backend='nccl'),
+)
+
+# set visualizer
+visualizer = None
+
+# set log level
+log_level = 'INFO'
+
+# load from which checkpoint
+load_from = None
+
+# whether to resume training from the loaded checkpoint
+resume = False
+
+# Defaults to use random seed and disable `deterministic`
+randomness = dict(seed=None, deterministic=False)
+
+log_processor = dict(
+ by_epoch=False,
+ window_size=1,
+ mean_pattern=r'.*(loss|time|data_time|grad_norm|tflops).*')
diff --git a/xtuner/configs/internvl/README.md b/xtuner/configs/internvl/README.md
new file mode 100644
index 000000000..1f1acf191
--- /dev/null
+++ b/xtuner/configs/internvl/README.md
@@ -0,0 +1,152 @@
+# InterVL Full Pipeline
+
+English | [简体中文](./README_zh-CN.md)
+
+## InterVL 2
+
+> [InternVL-2: Better than the Best—Expanding Performance Boundaries of Open-Source Multimodal Models with the Progressive Scaling Strategy](https://internvl.github.io/blog/2024-07-02-InternVL-2.0/)
+
+We introduce InternVL-2, currently the most powerful open-source Multimodal Large Language Model (MLLM). The InternVL-2 family includes models ranging from a 2B model, suitable for edge devices, to a 108B model, which is significantly more powerful. With larger-scale language models, InternVL-2-Pro demonstrates outstanding multimodal understanding capabilities, matching the performance of commercial closed-source models across various benchmarks.
+
+InternVL-2 family is built upon the following designs:
+
+- Progressive with larger language models: We introduce a progressive alignment training strategy, resulting in the first vision foundation model aligned with large language models. By employing the progressive training strategy where the model scales from small to large while the data refines from coarse to fine, we have completed the training of large models at a relatively low cost. This approach has demonstrated excellent performance with limited resources.
+- Multimodal input: With one set of parameters, our model supports multiple modalities of input, including text, images, video, audio, and 3D point clouds.
+- Multitask output: Our model supports various output formats, such as images, bounding boxes, and masks, demonstrating extensive versatility. By connecting the MLLM with multiple downstream task decoders, InternVL-2 can be generalized to hundreds of vision-language tasks while achieving performance comparable to expert models.
+
+
+

+
+
+### Basic Introduction
+
+- `./v2/` contains the configuration files for training InterVL 2
+- Supported fine-tuning of the InternVL 2B/4B/8B/26B model in full/LoRA/QLoRA single-image mode for now. We will support fine-tuning on multiple images and videos as soon as possible.
+- After training, you can use the `./v1_5/convert_to_official.py` script to convert the model trained by XTuner to the official format, so as to reuse all the official supported toolchains
+- All configurations are based on 8xA100 80G graphics cards, 2B/4B can use ZERO1 training, 8B models can use ZERO2, 26B models must run ZERO3, and there is no excessive adjustment of parameters, you can modify them according to your own needs
+- It is verified with LLaVA SFT data, which cannot fully reflect the fine-tuning performance. You can customize the data according to your own needs. We will provide a relatively fair fine-tuning dataset later
+
+### Data preparation
+
+If you also want to use the LLaVA SFT dataset for training, please refer to the [document](../../../docs/en/user_guides/dataset_prepare.md#llava-dataset) to prepare the data.
+
+For custom data, support multiple json and jsonl formats, the data organization can refer to the LLaVA SFT format, and support data sampling operations.
+
+**(1) Support multiple json or jsonl data**
+
+```text
+llava_dataset = dict(
+ type=InternVL_V1_5_Dataset,
+ model_path=path,
+ data_paths=['a.json','b.jsonl','c.json'],
+ image_folders=['a',None,'c'],
+ template=prompt_template,
+ max_length=max_length)
+```
+
+**(2) Support custom sampling**
+
+```text
+llava_dataset = dict(
+ type=InternVL_V1_5_Dataset,
+ model_path=path,
+ data_paths=['a.json','b.jsonl','c.json'],
+ image_folders=['a',None,'c'],
+ repeat_times=[2,0.5,3.5],
+ template=prompt_template,
+ max_length=max_length)
+```
+
+### Training
+
+The provided configuration is mainly used for fine-tuning based on the official weights. After preparing the data, you can use the following command to train:
+
+```bash
+NPROC_PER_NODE=8 xtuner train internvl_v2_internlm2_5_8b_lora_finetune --deepspeed deepspeed_zero2
+```
+
+Default saved in `./work_dirs/internvl_v2_internlm2_5_8b_lora_finetune/`.
+
+### Model Conversion
+
+After training, we will get a set of weights, that is `./work_dirs/internvl_v2_internlm2_5_8b_lora_finetune/iter_xxx.pth`, in order to facilitate evaluation and dialogue, we can convert it to official weights.
+
+```bash
+python xtuner/configs/internvl/v1_5/convert_to_official.py xtuner/configs/internvl/v2/internvl_v2_internlm2_5_8b_lora_finetune.py ./work_dirs/internvl_v2_internlm2_5_8b_lora_finetune/iter_xxx.pth ./work_dirs/internvl_v2_internlm2_5_8b_lora_finetune/convert_model/
+```
+
+Here, a complete set of official weights including configuration will be generated under `./work_dirs/internvl_v2_internlm2_5_8b_lora_finetune/convert_model`, you can use the [official toolchain](https://huggingface.co/OpenGVLab/InternVL2-8B) for evaluation and dialogue.
+
+If you encounter any problems during use, please feel free to contact us!!!
+
+## InterVL 1.5
+
+> [How Far Are We to GPT-4V? Closing the Gap to Commercial Multimodal Models with Open-Source Suites](https://arxiv.org/abs/2404.16821)
+
+In this report, we introduce InternVL 1.5, an open-source multimodal large language model (MLLM) to bridge the capability gap between open-source and proprietary commercial models in multimodal understanding. We introduce three simple improvements: (1) Strong Vision Encoder: we explored a continuous learning strategy for the large-scale vision foundation model -- InternViT-6B, boosting its visual understanding capabilities, and making it can be transferred and reused in different LLMs. (2) Dynamic High-Resolution: we divide images into tiles ranging from 1 to 40 of 448×448 pixels according to the aspect ratio and resolution of the input images, which supports up to 4K resolution input. (3) High-Quality Bilingual Dataset: we carefully collected a high-quality bilingual dataset that covers common scenes, document images, and annotated them with English and Chinese question-answer pairs, significantly enhancing performance in OCR- and Chinese-related tasks. We evaluate InternVL 1.5 through a series of benchmarks and comparative studies. Compared to both open-source and proprietary models, InternVL 1.5 shows competitive performance, achieving state-of-the-art results in 8 of 18 benchmarks.
+
+
+

+
+
+### Basic Introduction
+
+- `./v1_5/` contains the configuration files for training InterVL 1.5
+- Support InternVL 2B/4B/26B model full/LoRA/Qing efficiency and performance, it is recommended to choose the 4B model first
+- After training, you can use the `./v1_5/convert_to_official.py` script to convert the model trained by XTuner to the official format, so as to reuse all the official supported toolchains
+- All configurations are based on 8xA100 80G graphics cards, 2B/4B can use ZERO1 training, 8B models must run ZERO2, and there is no excessive adjustment of parameters, you can modify them according to your own needs
+- It is verified with LLaVA SFT data, which cannot fully reflect the fine-tuning performance. You can customize the data according to your own needs. We will provide a relatively fair fine-tuning dataset later
+
+### Data preparation
+
+If you also want to use the LLaVA SFT dataset for training, please refer to the [document](../../../docs/en/user_guides/dataset_prepare.md#llava-dataset) to prepare the data.
+
+For custom data, support multiple json and jsonl formats, the data organization can refer to the LLaVA SFT format, and support data sampling operations.
+
+**(1) Support multiple json or jsonl data**
+
+```text
+llava_dataset = dict(
+ type=InternVL_V1_5_Dataset,
+ model_path=path,
+ data_paths=['a.json','b.jsonl','c.json'],
+ image_folders=['a',None,'c'],
+ template=prompt_template,
+ max_length=max_length)
+```
+
+**(2) Support custom sampling**
+
+```text
+llava_dataset = dict(
+ type=InternVL_V1_5_Dataset,
+ model_path=path,
+ data_paths=['a.json','b.jsonl','c.json'],
+ image_folders=['a',None,'c'],
+ repeat_times=[2,0.5,3.5],
+ template=prompt_template,
+ max_length=max_length)
+```
+
+### Training
+
+The provided configuration is mainly used for fine-tuning based on the official weights. After preparing the data, you can use the following command to train:
+
+```bash
+NPROC_PER_NODE=8 xtuner train internvl_v1_5_phi3_4b_lora_finetune --deepspeed deepspeed_zero1
+# NPROC_PER_NODE=8 xtuner train internvl_v1_5_internlm2_26b_lora_finetune.py --deepspeed deepspeed_zero3
+```
+
+Default saved in `./work_dirs/internvl_v1_5_phi3_4b_lora_finetune/`.
+
+### Model Conversion
+
+After training, we will get a set of weights, that is `./work_dirs/internvl_v1_5_phi3_4b_lora_finetune/iter_xxx.pth`, in order to facilitate evaluation and dialogue, we can convert it to official weights.
+
+```bash
+python xtuner/configs/internvl/v1_5/convert_to_official.py xtuner/configs/internvl/v1_5/internvl_v1_5_phi3_4b_lora_finetune.py ./work_dirs/internvl_v1_5_phi3_4b_lora_finetune/iter_xxx.pth ./work_dirs/internvl_v1_5_phi3_4b_lora_finetune/internvl_v1_5_phi3_4b/
+```
+
+Here, a complete set of official weights including configuration will be generated under `./work_dirs/internvl_v1_5_phi3_4b_lora_finetune/internvl_v1_5_phi3_4b/`, you can use the [official toolchain](https://github.com/OpenGVLab/InternVL) for evaluation and dialogue.
+
+If you encounter any problems during use, please feel free to contact us!!!
diff --git a/xtuner/configs/internvl/README_zh-CN.md b/xtuner/configs/internvl/README_zh-CN.md
new file mode 100644
index 000000000..cdaa59348
--- /dev/null
+++ b/xtuner/configs/internvl/README_zh-CN.md
@@ -0,0 +1,152 @@
+# InterVL 全流程
+
+[English](./README.md) | 简体中文
+
+## InterVL 2
+
+> [InternVL-2: Better than the Best—Expanding Performance Boundaries of Open-Source Multimodal Models with the Progressive Scaling Strategy](https://internvl.github.io/blog/2024-07-02-InternVL-2.0/)
+
+我们引入了 InternVL-2,目前最强大的开源多模态大语言模型(MLLM)。InternVL-2 系列包括从适合于边缘设备的 2B 模型到强大的 108B 模型等多种规模的模型。借助更大规模的语言模型,InternVL-2-Pro 展现出了出色的多模态理解能力,在各种基准测试中的性能与商业闭源模型相匹配。
+
+InternVL-2 系列基于以下设计:
+
+- 渐进式的大型语言模型:我们引入了一种渐进式对齐训练策略,实现了首个与大型语言模型对齐的视觉基础模型。通过采用从小到大模型扩展、从粗到细数据优化的渐进式训练策略,我们以较低的成本完成了大模型的训练。这种方法已经展示了出色的性能,资源有限的情况下也能取得良好的结果。
+- 多模态输入:使用一套参数,我们的模型支持文本、图像、视频、音频和 3D 点云等多种输入模态。
+- 多任务输出:我们的模型支持图像、边界框和掩码等各种输出格式,展现出广泛的多功能性。通过将 MLLM 与多个下游任务解码器相连接,InternVL-2 可以泛化到数百个视觉语言任务,并取得与专家模型相当的性能。
+
+
+

+
+
+### 基本说明
+
+- `./v2/` 包含着 InterVL 2 训练配置的配置文件
+- 支持了 InternVL 2B/4B/8B/26B 模型全量/LoRA/QLoRA 单图模式的微调,会尽快支持多图和视频的微调。
+- 在训练完成后,可以使用 `./v1_5/convert_to_official.py` 脚本将 XTuner 训练的模型转换为官方格式,从而复用官方所支持的所有工具链
+- 目前所有配置都是以 8xA100 80G 显卡为基准,2B/4B 可以使用 ZERO1 训练,8B 模型要 ZERO2 运行,26B 模型必须要 ZERO3,并且没有对参数进行过多的调整,你可以按照你自己的需求进行修改
+- 目前是以 LLaVA SFT 数据进行验证,无法充分反应微调性能,你可以根据自己的需求进行数据自定义,后续我们会提供一个相对公平的微调数据集
+
+### 数据准备
+
+如果你也想使用 LLaVA SFT 数据集进行训练,请参考[文档](../../../docs/zh_cn/user_guides/dataset_prepare.md#llava-dataset) 准备数据。
+
+对于自定义数据,支持多种 json 和 jsonl 格式,内部数据组织可以参考 LLaVA SFT 格式,且支持数据采样操作。
+
+**(1) 支持多个 json 或者 jsonl 数据**
+
+```text
+llava_dataset = dict(
+ type=InternVL_V1_5_Dataset,
+ model_path=path,
+ data_paths=['a.json','b.jsonl','c.json'],
+ image_folders=['a',None,'c'],
+ template=prompt_template,
+ max_length=max_length)
+```
+
+**(2) 支持自定义采样**
+
+```text
+llava_dataset = dict(
+ type=InternVL_V1_5_Dataset,
+ model_path=path,
+ data_paths=['a.json','b.jsonl','c.json'],
+ image_folders=['a',None,'c'],
+ repeat_times=[2,0.5,3.5],
+ template=prompt_template,
+ max_length=max_length)
+```
+
+### 训练流程
+
+所提供的配置主要用于基于官方权重继续微调。在准备好数据后,你可以使用以下命令进行训练:
+
+```bash
+NPROC_PER_NODE=8 xtuner train internvl_v2_internlm2_5_8b_lora_finetune --deepspeed deepspeed_zero2
+```
+
+默认保存在 `./work_dirs/internvl_v2_internlm2_5_8b_lora_finetune/`。
+
+### 模型转换
+
+训练后,我们将获得一组权重即 `./work_dirs/internvl_v2_internlm2_5_8b_lora_finetune/iter_xxx.pth`,为了方便评测和对话,可以将其转换为官方权重。
+
+```bash
+python xtuner/configs/internvl/v1_5/convert_to_official.py xtuner/configs/internvl/v2/internvl_v2_internlm2_5_8b_lora_finetune.py ./work_dirs/internvl_v2_internlm2_5_8b_lora_finetune/iter_xxx.pth ./work_dirs/internvl_v2_internlm2_5_8b_lora_finetune/convert_model/
+```
+
+此时,会在 `./work_dirs/internvl_v2_internlm2_5_8b_lora_finetune/convert_model` 下生成一组包括配置的完整官方权重,你可以使用[官方工具链](https://huggingface.co/OpenGVLab/InternVL2-8B)进行评测和对话。
+
+如果你在使用中碰到任何问题,欢迎联系我们!!!
+
+## InterVL 1.5
+
+> [How Far Are We to GPT-4V? Closing the Gap to Commercial Multimodal Models with Open-Source Suites](https://arxiv.org/abs/2404.16821)
+
+在本报告中,我们介绍了开源多模态大语言模型 InternVL 1.5,以弥补开源模型与商业专有模型在多模态理解能力上的差距。我们引入了三项简单的改进:(1) 强大的视觉编码器:我们探索了大规模视觉基础模型 InternViT-6B 的连续学习策略,提升了其视觉理解能力,并使其可以在不同的大语言模型中进行迁移和重复利用。(2) 动态高分辨率:我们根据输入图像的长宽比和分辨率,将图像划分为从1到40个448×448像素的瓦片,支持高达4K分辨率的输入。(3) 高质量双语数据集:我们精心收集了一个高质量的双语数据集,涵盖了常见场景、文档图像,并用英语和中文问答对进行了注释,显著提升了在OCR和中文相关任务中的性能。我们通过一系列基准测试和对比研究评估了 InternVL 1.5。与开源和专有模型相比,InternVL 1.5 表现出了竞争力,在18个基准中的8个中取得了最先进的结果。
+
+
+

+
+
+### 基本说明
+
+- `./v1_5/` 包含着 InterVL 1.5 训练配置的配置文件
+- 支持 InternVL 2B/4B/26B 模型全量/LoRA/QLoRA 微调,综合考虑效率性能,建议你优先选择 4B 模型
+- 在训练完成后,可以使用 `./v1_5/convert_to_official.py` 脚本将 XTuner 训练的模型转换为官方格式,从而复用官方所支持的所有工具链
+- 目前所有配置都是以 8xA100 80G 显卡为基准,2B/4B 可以使用 ZERO1 训练,26B 模型必须要 ZERO3 运行,并且没有对参数进行过多的调整,你可以按照你自己的需求进行修改
+- 目前是以 LLaVA SFT 数据进行验证,无法充分反应微调性能,你可以根据自己的需求进行数据自定义,后续我们会提供一个相对公平的微调数据集
+
+### 数据准备
+
+如果你也想使用 LLaVA SFT 数据集进行训练,请参考[文档](../../../docs/zh_cn/user_guides/dataset_prepare.md#llava-dataset) 准备数据。
+
+对于自定义数据,支持多种 json 和 jsonl 格式,内部数据组织可以参考 LLaVA SFT 格式,且支持数据采样操作。
+
+**(1) 支持多个 json 或者 jsonl 数据**
+
+```text
+llava_dataset = dict(
+ type=InternVL_V1_5_Dataset,
+ model_path=path,
+ data_paths=['a.json','b.jsonl','c.json'],
+ image_folders=['a',None,'c'],
+ template=prompt_template,
+ max_length=max_length)
+```
+
+**(2) 支持自定义采样**
+
+```text
+llava_dataset = dict(
+ type=InternVL_V1_5_Dataset,
+ model_path=path,
+ data_paths=['a.json','b.jsonl','c.json'],
+ image_folders=['a',None,'c'],
+ repeat_times=[2,0.5,3.5],
+ template=prompt_template,
+ max_length=max_length)
+```
+
+### 训练流程
+
+所提供的配置主要用于基于官方权重继续微调。在准备好数据后,你可以使用以下命令进行训练:
+
+```bash
+NPROC_PER_NODE=8 xtuner train internvl_v1_5_phi3_4b_lora_finetune --deepspeed deepspeed_zero1
+# NPROC_PER_NODE=8 xtuner train internvl_v1_5_internlm2_26b_lora_finetune.py --deepspeed deepspeed_zero3
+```
+
+默认保存在 `./work_dirs/internvl_v1_5_phi3_4b_lora_finetune/`。
+
+### 模型转换
+
+训练后,我们将获得一组权重即 `./work_dirs/internvl_v1_5_phi3_4b_lora_finetune/iter_xxx.pth`,为了方便评测和对话,可以将其转换为官方权重。
+
+```bash
+python xtuner/configs/internvl/v1_5/convert_to_official.py xtuner/configs/internvl/v1_5/internvl_v1_5_phi3_4b_lora_finetune.py ./work_dirs/iter_xxx.pth ./work_dirs/internvl_v1_5_phi3_4b_lora_finetune/internvl_v1_5_phi3_4b/
+```
+
+此时,会在 `./work_dirs/internvl_v1_5_phi3_4b_lora_finetune/internvl_v1_5_phi3_4b/` 下生成一组包括配置的完整官方权重,你可以使用[官方工具链](https://github.com/OpenGVLab/InternVL)进行评测和对话。
+
+如果你在使用中碰到任何问题,欢迎联系我们!!!
diff --git a/xtuner/configs/internvl/v1_5/convert_to_official.py b/xtuner/configs/internvl/v1_5/convert_to_official.py
new file mode 100644
index 000000000..765855daa
--- /dev/null
+++ b/xtuner/configs/internvl/v1_5/convert_to_official.py
@@ -0,0 +1,56 @@
+import argparse
+import os.path as osp
+
+import torch
+from mmengine.config import Config
+from transformers import AutoTokenizer
+
+from xtuner.model.utils import LoadWoInit
+from xtuner.registry import BUILDER
+
+
+def convert_to_official(config, trained_path, save_path):
+ cfg = Config.fromfile(config)
+ cfg.model.pretrained_pth = trained_path
+ cfg.model.quantization_vit = False
+ cfg.model.quantization_llm = False
+
+ with LoadWoInit():
+ model = BUILDER.build(cfg.model)
+ model.to(torch.bfloat16)
+
+ if model.use_visual_encoder_lora:
+ vision_model = model.model.vision_model.merge_and_unload()
+ model.model.vision_model = vision_model
+
+ if model.use_llm_lora:
+ language_model = model.model.language_model.merge_and_unload()
+ model.model.language_model = language_model
+
+ model.model.save_pretrained(save_path)
+
+ tokenizer = AutoTokenizer.from_pretrained(
+ cfg.model.model_path, trust_remote_code=True)
+ tokenizer.save_pretrained(save_path)
+
+ print(model)
+
+
+def main():
+ parser = argparse.ArgumentParser(
+ description='Convert the pth model to HuggingFace model')
+ parser.add_argument('config', help='config file name or path.')
+ parser.add_argument('trained_model_pth', help='The trained model path.')
+ parser.add_argument(
+ 'save_path', help='The path to save the converted model.')
+ args = parser.parse_args()
+
+ if osp.realpath(args.trained_model_pth) == osp.realpath(args.save_path):
+ raise ValueError(
+ 'The trained path and save path should not be the same.')
+
+ convert_to_official(args.config, args.trained_model_pth, args.save_path)
+
+
+if __name__ == '__main__':
+ main()
diff --git a/xtuner/configs/internvl/v1_5/internvl_v1_5_internlm2_26b_finetune.py b/xtuner/configs/internvl/v1_5/internvl_v1_5_internlm2_26b_finetune.py
new file mode 100644
index 000000000..d5eec7829
--- /dev/null
+++ b/xtuner/configs/internvl/v1_5/internvl_v1_5_internlm2_26b_finetune.py
@@ -0,0 +1,170 @@
+# Copyright (c) OpenMMLab. All rights reserved.
+from mmengine.hooks import (CheckpointHook, DistSamplerSeedHook, IterTimerHook,
+ LoggerHook, ParamSchedulerHook)
+from mmengine.optim import AmpOptimWrapper, CosineAnnealingLR, LinearLR
+from torch.optim import AdamW
+from transformers import AutoTokenizer
+
+from xtuner.dataset import InternVL_V1_5_Dataset
+from xtuner.dataset.collate_fns import default_collate_fn
+from xtuner.dataset.samplers import LengthGroupedSampler
+from xtuner.engine.hooks import DatasetInfoHook
+from xtuner.engine.runner import TrainLoop
+from xtuner.model import InternVL_V1_5
+from xtuner.utils import PROMPT_TEMPLATE
+
+#######################################################################
+# PART 1 Settings #
+#######################################################################
+# Model
+path = 'OpenGVLab/InternVL-Chat-V1-5'
+prompt_template = PROMPT_TEMPLATE.internlm2_chat
+
+# Data
+data_root = './data/llava_data/'
+data_path = data_root + 'LLaVA-Instruct-150K/llava_v1_5_mix665k.json'
+image_folder = data_root + 'llava_images'
+max_length = 4096
+
+# Scheduler & Optimizer
+batch_size = 1 # per_device
+accumulative_counts = 8
+dataloader_num_workers = 4
+max_epochs = 1
+optim_type = AdamW
+# official 1024 -> 2e-5
+lr = 1e-6
+betas = (0.9, 0.999)
+weight_decay = 0.01
+max_norm = 1 # grad clip
+warmup_ratio = 0.03
+
+# Save
+save_steps = 1000
+save_total_limit = 1 # Maximum checkpoints to keep (-1 means unlimited)
+
+#######################################################################
+# PART 2 Model & Tokenizer & Image Processor #
+#######################################################################
+model = dict(
+ type=InternVL_V1_5,
+ model_path=path,
+ freeze_llm=False,
+ freeze_visual_encoder=True # or False
+)
+
+#######################################################################
+# PART 3 Dataset & Dataloader #
+#######################################################################
+llava_dataset = dict(
+ type=InternVL_V1_5_Dataset,
+ model_path=path,
+ data_paths=data_path,
+ image_folders=image_folder,
+ template=prompt_template,
+ max_length=max_length)
+
+train_dataloader = dict(
+ batch_size=batch_size,
+ num_workers=dataloader_num_workers,
+ dataset=llava_dataset,
+ sampler=dict(
+ type=LengthGroupedSampler,
+ length_property='modality_length',
+ per_device_batch_size=batch_size * accumulative_counts),
+ collate_fn=dict(type=default_collate_fn))
+
+#######################################################################
+# PART 4 Scheduler & Optimizer #
+#######################################################################
+# optimizer
+optim_wrapper = dict(
+ type=AmpOptimWrapper,
+ optimizer=dict(
+ type=optim_type, lr=lr, betas=betas, weight_decay=weight_decay),
+ clip_grad=dict(max_norm=max_norm, error_if_nonfinite=False),
+ accumulative_counts=accumulative_counts,
+ loss_scale='dynamic',
+ dtype='float16')
+
+# learning policy
+# More information: https://github.com/open-mmlab/mmengine/blob/main/docs/en/tutorials/param_scheduler.md # noqa: E501
+param_scheduler = [
+ dict(
+ type=LinearLR,
+ start_factor=1e-5,
+ by_epoch=True,
+ begin=0,
+ end=warmup_ratio * max_epochs,
+ convert_to_iter_based=True),
+ dict(
+ type=CosineAnnealingLR,
+ eta_min=0.0,
+ by_epoch=True,
+ begin=warmup_ratio * max_epochs,
+ end=max_epochs,
+ convert_to_iter_based=True)
+]
+
+# train, val, test setting
+train_cfg = dict(type=TrainLoop, max_epochs=max_epochs)
+
+#######################################################################
+# PART 5 Runtime #
+#######################################################################
+# Log the dialogue periodically during the training process, optional
+tokenizer = dict(
+ type=AutoTokenizer.from_pretrained,
+ pretrained_model_name_or_path=path,
+ trust_remote_code=True)
+
+custom_hooks = [
+ dict(type=DatasetInfoHook, tokenizer=tokenizer),
+]
+
+# configure default hooks
+default_hooks = dict(
+ # record the time of every iteration.
+ timer=dict(type=IterTimerHook),
+ # print log every 10 iterations.
+ logger=dict(type=LoggerHook, log_metric_by_epoch=False, interval=10),
+ # enable the parameter scheduler.
+ param_scheduler=dict(type=ParamSchedulerHook),
+ # save checkpoint per `save_steps`.
+ checkpoint=dict(
+ type=CheckpointHook,
+ save_optimizer=False,
+ by_epoch=False,
+ interval=save_steps,
+ max_keep_ckpts=save_total_limit),
+ # set sampler seed in distributed evrionment.
+ sampler_seed=dict(type=DistSamplerSeedHook),
+)
+
+# configure environment
+env_cfg = dict(
+ # whether to enable cudnn benchmark
+ cudnn_benchmark=False,
+ # set multi process parameters
+ mp_cfg=dict(mp_start_method='fork', opencv_num_threads=0),
+ # set distributed parameters
+ dist_cfg=dict(backend='nccl'),
+)
+
+# set visualizer
+visualizer = None
+
+# set log level
+log_level = 'INFO'
+
+# load from which checkpoint
+load_from = None
+
+# whether to resume training from the loaded checkpoint
+resume = False
+
+# Defaults to use random seed and disable `deterministic`
+randomness = dict(seed=None, deterministic=False)
+
+# set log processor
+log_processor = dict(by_epoch=False)
diff --git a/xtuner/configs/internvl/v1_5/internvl_v1_5_internlm2_26b_lora_finetune.py b/xtuner/configs/internvl/v1_5/internvl_v1_5_internlm2_26b_lora_finetune.py
new file mode 100644
index 000000000..0fb511d42
--- /dev/null
+++ b/xtuner/configs/internvl/v1_5/internvl_v1_5_internlm2_26b_lora_finetune.py
@@ -0,0 +1,183 @@
+# Copyright (c) OpenMMLab. All rights reserved.
+from mmengine.hooks import (CheckpointHook, DistSamplerSeedHook, IterTimerHook,
+ LoggerHook, ParamSchedulerHook)
+from mmengine.optim import AmpOptimWrapper, CosineAnnealingLR, LinearLR
+from peft import LoraConfig
+from torch.optim import AdamW
+from transformers import AutoTokenizer
+
+from xtuner.dataset import InternVL_V1_5_Dataset
+from xtuner.dataset.collate_fns import default_collate_fn
+from xtuner.dataset.samplers import LengthGroupedSampler
+from xtuner.engine.hooks import DatasetInfoHook
+from xtuner.engine.runner import TrainLoop
+from xtuner.model import InternVL_V1_5
+from xtuner.utils import PROMPT_TEMPLATE
+
+#######################################################################
+# PART 1 Settings #
+#######################################################################
+# Model
+path = 'OpenGVLab/InternVL-Chat-V1-5'
+prompt_template = PROMPT_TEMPLATE.internlm2_chat
+
+# Data
+data_root = './data/llava_data/'
+data_path = data_root + 'LLaVA-Instruct-150K/llava_v1_5_mix665k.json'
+image_folder = data_root + 'llava_images'
+max_length = 4096
+
+# Scheduler & Optimizer
+batch_size = 2 # per_device
+accumulative_counts = 4
+dataloader_num_workers = 4
+max_epochs = 1
+optim_type = AdamW
+# official 1024 -> 2e-5
+lr = 1e-6
+betas = (0.9, 0.999)
+weight_decay = 0.01
+max_norm = 1 # grad clip
+warmup_ratio = 0.03
+
+# Save
+save_steps = 1000
+save_total_limit = 1 # Maximum checkpoints to keep (-1 means unlimited)
+
+#######################################################################
+# PART 2 Model & Tokenizer & Image Processor #
+#######################################################################
+model = dict(
+ type=InternVL_V1_5,
+ model_path=path,
+ freeze_llm=True,
+ freeze_visual_encoder=True,
+ # comment the following lines if you don't want to use Lora in llm
+ llm_lora=dict(
+ type=LoraConfig,
+ r=128,
+ lora_alpha=256,
+ lora_dropout=0.05,
+ target_modules=None,
+ task_type='CAUSAL_LM'),
+ # uncomment the following lines if you don't want to use Lora in visual encoder # noqa
+ # visual_encoder_lora=dict(
+ # type=LoraConfig, r=64, lora_alpha=16, lora_dropout=0.05,
+ # target_modules=['attn.qkv', 'attn.proj', 'mlp.fc1', 'mlp.fc2'])
+)
+
+#######################################################################
+# PART 3 Dataset & Dataloader #
+#######################################################################
+llava_dataset = dict(
+ type=InternVL_V1_5_Dataset,
+ model_path=path,
+ data_paths=data_path,
+ image_folders=image_folder,
+ template=prompt_template,
+ max_length=max_length)
+
+train_dataloader = dict(
+ batch_size=batch_size,
+ num_workers=dataloader_num_workers,
+ dataset=llava_dataset,
+ sampler=dict(
+ type=LengthGroupedSampler,
+ length_property='modality_length',
+ per_device_batch_size=batch_size * accumulative_counts),
+ collate_fn=dict(type=default_collate_fn))
+
+#######################################################################
+# PART 4 Scheduler & Optimizer #
+#######################################################################
+# optimizer
+optim_wrapper = dict(
+ type=AmpOptimWrapper,
+ optimizer=dict(
+ type=optim_type, lr=lr, betas=betas, weight_decay=weight_decay),
+ clip_grad=dict(max_norm=max_norm, error_if_nonfinite=False),
+ accumulative_counts=accumulative_counts,
+ loss_scale='dynamic',
+ dtype='float16')
+
+# learning policy
+# More information: https://github.com/open-mmlab/mmengine/blob/main/docs/en/tutorials/param_scheduler.md # noqa: E501
+param_scheduler = [
+ dict(
+ type=LinearLR,
+ start_factor=1e-5,
+ by_epoch=True,
+ begin=0,
+ end=warmup_ratio * max_epochs,
+ convert_to_iter_based=True),
+ dict(
+ type=CosineAnnealingLR,
+ eta_min=0.0,
+ by_epoch=True,
+ begin=warmup_ratio * max_epochs,
+ end=max_epochs,
+ convert_to_iter_based=True)
+]
+
+# train, val, test setting
+train_cfg = dict(type=TrainLoop, max_epochs=max_epochs)
+
+#######################################################################
+# PART 5 Runtime #
+#######################################################################
+# Log the dialogue periodically during the training process, optional
+tokenizer = dict(
+ type=AutoTokenizer.from_pretrained,
+ pretrained_model_name_or_path=path,
+ trust_remote_code=True)
+
+custom_hooks = [
+ dict(type=DatasetInfoHook, tokenizer=tokenizer),
+]
+
+# configure default hooks
+default_hooks = dict(
+ # record the time of every iteration.
+ timer=dict(type=IterTimerHook),
+ # print log every 10 iterations.
+ logger=dict(type=LoggerHook, log_metric_by_epoch=False, interval=10),
+ # enable the parameter scheduler.
+ param_scheduler=dict(type=ParamSchedulerHook),
+ # save checkpoint per `save_steps`.
+ checkpoint=dict(
+ type=CheckpointHook,
+ save_optimizer=False,
+ by_epoch=False,
+ interval=save_steps,
+ max_keep_ckpts=save_total_limit),
+ # set sampler seed in distributed evrionment.
+ sampler_seed=dict(type=DistSamplerSeedHook),
+)
+
+# configure environment
+env_cfg = dict(
+ # whether to enable cudnn benchmark
+ cudnn_benchmark=False,
+ # set multi process parameters
+ mp_cfg=dict(mp_start_method='fork', opencv_num_threads=0),
+ # set distributed parameters
+ dist_cfg=dict(backend='nccl'),
+)
+
+# set visualizer
+visualizer = None
+
+# set log level
+log_level = 'INFO'
+
+# load from which checkpoint
+load_from = None
+
+# whether to resume training from the loaded checkpoint
+resume = False
+
+# Defaults to use random seed and disable `deterministic`
+randomness = dict(seed=None, deterministic=False)
+
+# set log processor
+log_processor = dict(by_epoch=False)
diff --git a/xtuner/configs/internvl/v1_5/internvl_v1_5_internlm2_26b_qlora_finetune.py b/xtuner/configs/internvl/v1_5/internvl_v1_5_internlm2_26b_qlora_finetune.py
new file mode 100644
index 000000000..8d994c81d
--- /dev/null
+++ b/xtuner/configs/internvl/v1_5/internvl_v1_5_internlm2_26b_qlora_finetune.py
@@ -0,0 +1,185 @@
+# Copyright (c) OpenMMLab. All rights reserved.
+from mmengine.hooks import (CheckpointHook, DistSamplerSeedHook, IterTimerHook,
+ LoggerHook, ParamSchedulerHook)
+from mmengine.optim import AmpOptimWrapper, CosineAnnealingLR, LinearLR
+from peft import LoraConfig
+from torch.optim import AdamW
+from transformers import AutoTokenizer
+
+from xtuner.dataset import InternVL_V1_5_Dataset
+from xtuner.dataset.collate_fns import default_collate_fn
+from xtuner.dataset.samplers import LengthGroupedSampler
+from xtuner.engine.hooks import DatasetInfoHook
+from xtuner.engine.runner import TrainLoop
+from xtuner.model import InternVL_V1_5
+from xtuner.utils import PROMPT_TEMPLATE
+
+#######################################################################
+# PART 1 Settings #
+#######################################################################
+# Model
+path = 'OpenGVLab/InternVL-Chat-V1-5'
+prompt_template = PROMPT_TEMPLATE.internlm2_chat
+
+# Data
+data_root = './data/llava_data/'
+data_path = data_root + 'LLaVA-Instruct-150K/llava_v1_5_mix665k.json'
+image_folder = data_root + 'llava_images'
+max_length = 4096
+
+# Scheduler & Optimizer
+batch_size = 2 # per_device
+accumulative_counts = 4
+dataloader_num_workers = 4
+max_epochs = 1
+optim_type = AdamW
+# official 1024 -> 2e-5
+lr = 1e-6
+betas = (0.9, 0.999)
+weight_decay = 0.01
+max_norm = 1 # grad clip
+warmup_ratio = 0.03
+
+# Save
+save_steps = 1000
+save_total_limit = 1 # Maximum checkpoints to keep (-1 means unlimited)
+
+#######################################################################
+# PART 2 Model & Tokenizer & Image Processor #
+#######################################################################
+model = dict(
+ type=InternVL_V1_5,
+ model_path=path,
+ freeze_llm=True,
+ freeze_visual_encoder=True,
+ quantization_llm=True, # or False
+ quantization_vit=False, # or True and uncomment visual_encoder_lora
+ # comment the following lines if you don't want to use Lora in llm
+ llm_lora=dict(
+ type=LoraConfig,
+ r=128,
+ lora_alpha=256,
+ lora_dropout=0.05,
+ target_modules=None,
+ task_type='CAUSAL_LM'),
+ # uncomment the following lines if you don't want to use Lora in visual encoder # noqa
+ # visual_encoder_lora=dict(
+ # type=LoraConfig, r=64, lora_alpha=16, lora_dropout=0.05,
+ # target_modules=['attn.qkv', 'attn.proj', 'mlp.fc1', 'mlp.fc2'])
+)
+
+#######################################################################
+# PART 3 Dataset & Dataloader #
+#######################################################################
+llava_dataset = dict(
+ type=InternVL_V1_5_Dataset,
+ model_path=path,
+ data_paths=data_path,
+ image_folders=image_folder,
+ template=prompt_template,
+ max_length=max_length)
+
+train_dataloader = dict(
+ batch_size=batch_size,
+ num_workers=dataloader_num_workers,
+ dataset=llava_dataset,
+ sampler=dict(
+ type=LengthGroupedSampler,
+ length_property='modality_length',
+ per_device_batch_size=batch_size * accumulative_counts),
+ collate_fn=dict(type=default_collate_fn))
+
+#######################################################################
+# PART 4 Scheduler & Optimizer #
+#######################################################################
+# optimizer
+optim_wrapper = dict(
+ type=AmpOptimWrapper,
+ optimizer=dict(
+ type=optim_type, lr=lr, betas=betas, weight_decay=weight_decay),
+ clip_grad=dict(max_norm=max_norm, error_if_nonfinite=False),
+ accumulative_counts=accumulative_counts,
+ loss_scale='dynamic',
+ dtype='float16')
+
+# learning policy
+# More information: https://github.com/open-mmlab/mmengine/blob/main/docs/en/tutorials/param_scheduler.md # noqa: E501
+param_scheduler = [
+ dict(
+ type=LinearLR,
+ start_factor=1e-5,
+ by_epoch=True,
+ begin=0,
+ end=warmup_ratio * max_epochs,
+ convert_to_iter_based=True),
+ dict(
+ type=CosineAnnealingLR,
+ eta_min=0.0,
+ by_epoch=True,
+ begin=warmup_ratio * max_epochs,
+ end=max_epochs,
+ convert_to_iter_based=True)
+]
+
+# train, val, test setting
+train_cfg = dict(type=TrainLoop, max_epochs=max_epochs)
+
+#######################################################################
+# PART 5 Runtime #
+#######################################################################
+# Log the dialogue periodically during the training process, optional
+tokenizer = dict(
+ type=AutoTokenizer.from_pretrained,
+ pretrained_model_name_or_path=path,
+ trust_remote_code=True)
+
+custom_hooks = [
+ dict(type=DatasetInfoHook, tokenizer=tokenizer),
+]
+
+# configure default hooks
+default_hooks = dict(
+ # record the time of every iteration.
+ timer=dict(type=IterTimerHook),
+ # print log every 10 iterations.
+ logger=dict(type=LoggerHook, log_metric_by_epoch=False, interval=10),
+ # enable the parameter scheduler.
+ param_scheduler=dict(type=ParamSchedulerHook),
+ # save checkpoint per `save_steps`.
+ checkpoint=dict(
+ type=CheckpointHook,
+ save_optimizer=False,
+ by_epoch=False,
+ interval=save_steps,
+ max_keep_ckpts=save_total_limit),
+ # set sampler seed in distributed evrionment.
+ sampler_seed=dict(type=DistSamplerSeedHook),
+)
+
+# configure environment
+env_cfg = dict(
+ # whether to enable cudnn benchmark
+ cudnn_benchmark=False,
+ # set multi process parameters
+ mp_cfg=dict(mp_start_method='fork', opencv_num_threads=0),
+ # set distributed parameters
+ dist_cfg=dict(backend='nccl'),
+)
+
+# set visualizer
+visualizer = None
+
+# set log level
+log_level = 'INFO'
+
+# load from which checkpoint
+load_from = None
+
+# whether to resume training from the loaded checkpoint
+resume = False
+
+# Defaults to use random seed and disable `deterministic`
+randomness = dict(seed=None, deterministic=False)
+
+# set log processor
+log_processor = dict(by_epoch=False)
diff --git a/xtuner/configs/internvl/v1_5/internvl_v1_5_internlm2_2b_finetune.py b/xtuner/configs/internvl/v1_5/internvl_v1_5_internlm2_2b_finetune.py
new file mode 100644
index 000000000..09fb01e3f
--- /dev/null
+++ b/xtuner/configs/internvl/v1_5/internvl_v1_5_internlm2_2b_finetune.py
@@ -0,0 +1,170 @@
+# Copyright (c) OpenMMLab. All rights reserved.
+from mmengine.hooks import (CheckpointHook, DistSamplerSeedHook, IterTimerHook,
+ LoggerHook, ParamSchedulerHook)
+from mmengine.optim import AmpOptimWrapper, CosineAnnealingLR, LinearLR
+from torch.optim import AdamW
+from transformers import AutoTokenizer
+
+from xtuner.dataset import InternVL_V1_5_Dataset
+from xtuner.dataset.collate_fns import default_collate_fn
+from xtuner.dataset.samplers import LengthGroupedSampler
+from xtuner.engine.hooks import DatasetInfoHook
+from xtuner.engine.runner import TrainLoop
+from xtuner.model import InternVL_V1_5
+from xtuner.utils import PROMPT_TEMPLATE
+
+#######################################################################
+# PART 1 Settings #
+#######################################################################
+# Model
+path = 'OpenGVLab/Mini-InternVL-Chat-2B-V1-5'
+prompt_template = PROMPT_TEMPLATE.internlm2_chat
+
+# Data
+data_root = './data/llava_data/'
+data_path = data_root + 'LLaVA-Instruct-150K/llava_v1_5_mix665k.json'
+image_folder = data_root + 'llava_images'
+max_length = 8192
+
+# Scheduler & Optimizer
+batch_size = 4 # per_device
+accumulative_counts = 4
+dataloader_num_workers = 4
+max_epochs = 1
+optim_type = AdamW
+# official 1024 -> 4e-5
+lr = 1e-6
+betas = (0.9, 0.999)
+weight_decay = 0.05
+max_norm = 1 # grad clip
+warmup_ratio = 0.03
+
+# Save
+save_steps = 1000
+save_total_limit = 1 # Maximum checkpoints to keep (-1 means unlimited)
+
+#######################################################################
+# PART 2 Model & Tokenizer & Image Processor #
+#######################################################################
+model = dict(
+ type=InternVL_V1_5,
+ model_path=path,
+ freeze_llm=False,
+ freeze_visual_encoder=True # or False
+)
+
+#######################################################################
+# PART 3 Dataset & Dataloader #
+#######################################################################
+llava_dataset = dict(
+ type=InternVL_V1_5_Dataset,
+ model_path=path,
+ data_paths=data_path,
+ image_folders=image_folder,
+ template=prompt_template,
+ max_length=max_length)
+
+train_dataloader = dict(
+ batch_size=batch_size,
+ num_workers=dataloader_num_workers,
+ dataset=llava_dataset,
+ sampler=dict(
+ type=LengthGroupedSampler,
+ length_property='modality_length',
+ per_device_batch_size=batch_size * accumulative_counts),
+ collate_fn=dict(type=default_collate_fn))
+
+#######################################################################
+# PART 4 Scheduler & Optimizer #
+#######################################################################
+# optimizer
+optim_wrapper = dict(
+ type=AmpOptimWrapper,
+ optimizer=dict(
+ type=optim_type, lr=lr, betas=betas, weight_decay=weight_decay),
+ clip_grad=dict(max_norm=max_norm, error_if_nonfinite=False),
+ accumulative_counts=accumulative_counts,
+ loss_scale='dynamic',
+ dtype='float16')
+
+# learning policy
+# More information: https://github.com/open-mmlab/mmengine/blob/main/docs/en/tutorials/param_scheduler.md # noqa: E501
+param_scheduler = [
+ dict(
+ type=LinearLR,
+ start_factor=1e-5,
+ by_epoch=True,
+ begin=0,
+ end=warmup_ratio * max_epochs,
+ convert_to_iter_based=True),
+ dict(
+ type=CosineAnnealingLR,
+ eta_min=0.0,
+ by_epoch=True,
+ begin=warmup_ratio * max_epochs,
+ end=max_epochs,
+ convert_to_iter_based=True)
+]
+
+# train, val, test setting
+train_cfg = dict(type=TrainLoop, max_epochs=max_epochs)
+
+#######################################################################
+# PART 5 Runtime #
+#######################################################################
+# Log the dialogue periodically during the training process, optional
+tokenizer = dict(
+ type=AutoTokenizer.from_pretrained,
+ pretrained_model_name_or_path=path,
+ trust_remote_code=True)
+
+custom_hooks = [
+ dict(type=DatasetInfoHook, tokenizer=tokenizer),
+]
+
+# configure default hooks
+default_hooks = dict(
+ # record the time of every iteration.
+ timer=dict(type=IterTimerHook),
+ # print log every 10 iterations.
+ logger=dict(type=LoggerHook, log_metric_by_epoch=False, interval=10),
+ # enable the parameter scheduler.
+ param_scheduler=dict(type=ParamSchedulerHook),
+ # save checkpoint per `save_steps`.
+ checkpoint=dict(
+ type=CheckpointHook,
+ save_optimizer=False,
+ by_epoch=False,
+ interval=save_steps,
+ max_keep_ckpts=save_total_limit),
+ # set sampler seed in distributed evrionment.
+ sampler_seed=dict(type=DistSamplerSeedHook),
+)
+
+# configure environment
+env_cfg = dict(
+ # whether to enable cudnn benchmark
+ cudnn_benchmark=False,
+ # set multi process parameters
+ mp_cfg=dict(mp_start_method='fork', opencv_num_threads=0),
+ # set distributed parameters
+ dist_cfg=dict(backend='nccl'),
+)
+
+# set visualizer
+visualizer = None
+
+# set log level
+log_level = 'INFO'
+
+# load from which checkpoint
+load_from = None
+
+# whether to resume training from the loaded checkpoint
+resume = False
+
+# Defaults to use random seed and disable `deterministic`
+randomness = dict(seed=None, deterministic=False)
+
+# set log processor
+log_processor = dict(by_epoch=False)
diff --git a/xtuner/configs/internvl/v1_5/internvl_v1_5_internlm2_2b_lora_finetune.py b/xtuner/configs/internvl/v1_5/internvl_v1_5_internlm2_2b_lora_finetune.py
new file mode 100644
index 000000000..193e2f269
--- /dev/null
+++ b/xtuner/configs/internvl/v1_5/internvl_v1_5_internlm2_2b_lora_finetune.py
@@ -0,0 +1,183 @@
+# Copyright (c) OpenMMLab. All rights reserved.
+from mmengine.hooks import (CheckpointHook, DistSamplerSeedHook, IterTimerHook,
+ LoggerHook, ParamSchedulerHook)
+from mmengine.optim import AmpOptimWrapper, CosineAnnealingLR, LinearLR
+from peft import LoraConfig
+from torch.optim import AdamW
+from transformers import AutoTokenizer
+
+from xtuner.dataset import InternVL_V1_5_Dataset
+from xtuner.dataset.collate_fns import default_collate_fn
+from xtuner.dataset.samplers import LengthGroupedSampler
+from xtuner.engine.hooks import DatasetInfoHook
+from xtuner.engine.runner import TrainLoop
+from xtuner.model import InternVL_V1_5
+from xtuner.utils import PROMPT_TEMPLATE
+
+#######################################################################
+# PART 1 Settings #
+#######################################################################
+# Model
+path = 'OpenGVLab/Mini-InternVL-Chat-2B-V1-5'
+prompt_template = PROMPT_TEMPLATE.internlm2_chat
+
+# Data
+data_root = './data/llava_data/'
+data_path = data_root + 'LLaVA-Instruct-150K/llava_v1_5_mix665k.json'
+image_folder = data_root + 'llava_images'
+max_length = 8192
+
+# Scheduler & Optimizer
+batch_size = 8 # per_device
+accumulative_counts = 2
+dataloader_num_workers = 4
+max_epochs = 1
+optim_type = AdamW
+# official 1024 -> 4e-5
+lr = 1e-6
+betas = (0.9, 0.999)
+weight_decay = 0.05
+max_norm = 1 # grad clip
+warmup_ratio = 0.03
+
+# Save
+save_steps = 1000
+save_total_limit = 1 # Maximum checkpoints to keep (-1 means unlimited)
+
+#######################################################################
+# PART 2 Model & Tokenizer & Image Processor #
+#######################################################################
+model = dict(
+ type=InternVL_V1_5,
+ model_path=path,
+ freeze_llm=True,
+ freeze_visual_encoder=True,
+ # comment the following lines if you don't want to use Lora in llm
+ llm_lora=dict(
+ type=LoraConfig,
+ r=128,
+ lora_alpha=256,
+ lora_dropout=0.05,
+ target_modules=None,
+ task_type='CAUSAL_LM'),
+ # uncomment the following lines if you don't want to use Lora in visual encoder # noqa
+ # visual_encoder_lora=dict(
+ # type=LoraConfig, r=64, lora_alpha=16, lora_dropout=0.05,
+ # target_modules=['attn.qkv', 'attn.proj', 'mlp.fc1', 'mlp.fc2'])
+)
+
+#######################################################################
+# PART 3 Dataset & Dataloader #
+#######################################################################
+llava_dataset = dict(
+ type=InternVL_V1_5_Dataset,
+ model_path=path,
+ data_paths=data_path,
+ image_folders=image_folder,
+ template=prompt_template,
+ max_length=max_length)
+
+train_dataloader = dict(
+ batch_size=batch_size,
+ num_workers=dataloader_num_workers,
+ dataset=llava_dataset,
+ sampler=dict(
+ type=LengthGroupedSampler,
+ length_property='modality_length',
+ per_device_batch_size=batch_size * accumulative_counts),
+ collate_fn=dict(type=default_collate_fn))
+
+#######################################################################
+# PART 4 Scheduler & Optimizer #
+#######################################################################
+# optimizer
+optim_wrapper = dict(
+ type=AmpOptimWrapper,
+ optimizer=dict(
+ type=optim_type, lr=lr, betas=betas, weight_decay=weight_decay),
+ clip_grad=dict(max_norm=max_norm, error_if_nonfinite=False),
+ accumulative_counts=accumulative_counts,
+ loss_scale='dynamic',
+ dtype='float16')
+
+# learning policy
+# More information: https://github.com/open-mmlab/mmengine/blob/main/docs/en/tutorials/param_scheduler.md # noqa: E501
+param_scheduler = [
+ dict(
+ type=LinearLR,
+ start_factor=1e-5,
+ by_epoch=True,
+ begin=0,
+ end=warmup_ratio * max_epochs,
+ convert_to_iter_based=True),
+ dict(
+ type=CosineAnnealingLR,
+ eta_min=0.0,
+ by_epoch=True,
+ begin=warmup_ratio * max_epochs,
+ end=max_epochs,
+ convert_to_iter_based=True)
+]
+
+# train, val, test setting
+train_cfg = dict(type=TrainLoop, max_epochs=max_epochs)
+
+#######################################################################
+# PART 5 Runtime #
+#######################################################################
+# Log the dialogue periodically during the training process, optional
+tokenizer = dict(
+ type=AutoTokenizer.from_pretrained,
+ pretrained_model_name_or_path=path,
+ trust_remote_code=True)
+
+custom_hooks = [
+ dict(type=DatasetInfoHook, tokenizer=tokenizer),
+]
+
+# configure default hooks
+default_hooks = dict(
+ # record the time of every iteration.
+ timer=dict(type=IterTimerHook),
+ # print log every 10 iterations.
+ logger=dict(type=LoggerHook, log_metric_by_epoch=False, interval=10),
+ # enable the parameter scheduler.
+ param_scheduler=dict(type=ParamSchedulerHook),
+ # save checkpoint per `save_steps`.
+ checkpoint=dict(
+ type=CheckpointHook,
+ save_optimizer=False,
+ by_epoch=False,
+ interval=save_steps,
+ max_keep_ckpts=save_total_limit),
+ # set sampler seed in distributed evrionment.
+ sampler_seed=dict(type=DistSamplerSeedHook),
+)
+
+# configure environment
+env_cfg = dict(
+ # whether to enable cudnn benchmark
+ cudnn_benchmark=False,
+ # set multi process parameters
+ mp_cfg=dict(mp_start_method='fork', opencv_num_threads=0),
+ # set distributed parameters
+ dist_cfg=dict(backend='nccl'),
+)
+
+# set visualizer
+visualizer = None
+
+# set log level
+log_level = 'INFO'
+
+# load from which checkpoint
+load_from = None
+
+# whether to resume training from the loaded checkpoint
+resume = False
+
+# Defaults to use random seed and disable `deterministic`
+randomness = dict(seed=None, deterministic=False)
+
+# set log processor
+log_processor = dict(by_epoch=False)
diff --git a/xtuner/configs/internvl/v1_5/internvl_v1_5_internlm2_2b_qlora_finetune.py b/xtuner/configs/internvl/v1_5/internvl_v1_5_internlm2_2b_qlora_finetune.py
new file mode 100644
index 000000000..6bb28e490
--- /dev/null
+++ b/xtuner/configs/internvl/v1_5/internvl_v1_5_internlm2_2b_qlora_finetune.py
@@ -0,0 +1,185 @@
+# Copyright (c) OpenMMLab. All rights reserved.
+from mmengine.hooks import (CheckpointHook, DistSamplerSeedHook, IterTimerHook,
+ LoggerHook, ParamSchedulerHook)
+from mmengine.optim import AmpOptimWrapper, CosineAnnealingLR, LinearLR
+from peft import LoraConfig
+from torch.optim import AdamW
+from transformers import AutoTokenizer
+
+from xtuner.dataset import InternVL_V1_5_Dataset
+from xtuner.dataset.collate_fns import default_collate_fn
+from xtuner.dataset.samplers import LengthGroupedSampler
+from xtuner.engine.hooks import DatasetInfoHook
+from xtuner.engine.runner import TrainLoop
+from xtuner.model import InternVL_V1_5
+from xtuner.utils import PROMPT_TEMPLATE
+
+#######################################################################
+# PART 1 Settings #
+#######################################################################
+# Model
+path = 'OpenGVLab/Mini-InternVL-Chat-2B-V1-5'
+prompt_template = PROMPT_TEMPLATE.internlm2_chat
+
+# Data
+data_root = './data/llava_data/'
+data_path = data_root + 'LLaVA-Instruct-150K/llava_v1_5_mix665k.json'
+image_folder = data_root + 'llava_images'
+max_length = 8192
+
+# Scheduler & Optimizer
+batch_size = 8 # per_device
+accumulative_counts = 2
+dataloader_num_workers = 4
+max_epochs = 1
+optim_type = AdamW
+# official 1024 -> 4e-5
+lr = 1e-6
+betas = (0.9, 0.999)
+weight_decay = 0.05
+max_norm = 1 # grad clip
+warmup_ratio = 0.03
+
+# Save
+save_steps = 1000
+save_total_limit = 1 # Maximum checkpoints to keep (-1 means unlimited)
+
+#######################################################################
+# PART 2 Model & Tokenizer & Image Processor #
+#######################################################################
+model = dict(
+ type=InternVL_V1_5,
+ model_path=path,
+ freeze_llm=True,
+ freeze_visual_encoder=True,
+ quantization_llm=True, # or False
+ quantization_vit=False, # or True and uncomment visual_encoder_lora
+ # comment the following lines if you don't want to use Lora in llm
+ llm_lora=dict(
+ type=LoraConfig,
+ r=128,
+ lora_alpha=256,
+ lora_dropout=0.05,
+ target_modules=None,
+ task_type='CAUSAL_LM'),
+ # uncomment the following lines if you don't want to use Lora in visual encoder # noqa
+ # visual_encoder_lora=dict(
+ # type=LoraConfig, r=64, lora_alpha=16, lora_dropout=0.05,
+ # target_modules=['attn.qkv', 'attn.proj', 'mlp.fc1', 'mlp.fc2'])
+)
+
+#######################################################################
+# PART 3 Dataset & Dataloader #
+#######################################################################
+llava_dataset = dict(
+ type=InternVL_V1_5_Dataset,
+ model_path=path,
+ data_paths=data_path,
+ image_folders=image_folder,
+ template=prompt_template,
+ max_length=max_length)
+
+train_dataloader = dict(
+ batch_size=batch_size,
+ num_workers=dataloader_num_workers,
+ dataset=llava_dataset,
+ sampler=dict(
+ type=LengthGroupedSampler,
+ length_property='modality_length',
+ per_device_batch_size=batch_size * accumulative_counts),
+ collate_fn=dict(type=default_collate_fn))
+
+#######################################################################
+# PART 4 Scheduler & Optimizer #
+#######################################################################
+# optimizer
+optim_wrapper = dict(
+ type=AmpOptimWrapper,
+ optimizer=dict(
+ type=optim_type, lr=lr, betas=betas, weight_decay=weight_decay),
+ clip_grad=dict(max_norm=max_norm, error_if_nonfinite=False),
+ accumulative_counts=accumulative_counts,
+ loss_scale='dynamic',
+ dtype='float16')
+
+# learning policy
+# More information: https://github.com/open-mmlab/mmengine/blob/main/docs/en/tutorials/param_scheduler.md # noqa: E501
+param_scheduler = [
+ dict(
+ type=LinearLR,
+ start_factor=1e-5,
+ by_epoch=True,
+ begin=0,
+ end=warmup_ratio * max_epochs,
+ convert_to_iter_based=True),
+ dict(
+ type=CosineAnnealingLR,
+ eta_min=0.0,
+ by_epoch=True,
+ begin=warmup_ratio * max_epochs,
+ end=max_epochs,
+ convert_to_iter_based=True)
+]
+
+# train, val, test setting
+train_cfg = dict(type=TrainLoop, max_epochs=max_epochs)
+
+#######################################################################
+# PART 5 Runtime #
+#######################################################################
+# Log the dialogue periodically during the training process, optional
+tokenizer = dict(
+ type=AutoTokenizer.from_pretrained,
+ pretrained_model_name_or_path=path,
+ trust_remote_code=True)
+
+custom_hooks = [
+ dict(type=DatasetInfoHook, tokenizer=tokenizer),
+]
+
+# configure default hooks
+default_hooks = dict(
+ # record the time of every iteration.
+ timer=dict(type=IterTimerHook),
+ # print log every 10 iterations.
+ logger=dict(type=LoggerHook, log_metric_by_epoch=False, interval=10),
+ # enable the parameter scheduler.
+ param_scheduler=dict(type=ParamSchedulerHook),
+ # save checkpoint per `save_steps`.
+ checkpoint=dict(
+ type=CheckpointHook,
+ save_optimizer=False,
+ by_epoch=False,
+ interval=save_steps,
+ max_keep_ckpts=save_total_limit),
+ # set sampler seed in distributed evrionment.
+ sampler_seed=dict(type=DistSamplerSeedHook),
+)
+
+# configure environment
+env_cfg = dict(
+ # whether to enable cudnn benchmark
+ cudnn_benchmark=False,
+ # set multi process parameters
+ mp_cfg=dict(mp_start_method='fork', opencv_num_threads=0),
+ # set distributed parameters
+ dist_cfg=dict(backend='nccl'),
+)
+
+# set visualizer
+visualizer = None
+
+# set log level
+log_level = 'INFO'
+
+# load from which checkpoint
+load_from = None
+
+# whether to resume training from the loaded checkpoint
+resume = False
+
+# Defaults to use random seed and disable `deterministic`
+randomness = dict(seed=None, deterministic=False)
+
+# set log processor
+log_processor = dict(by_epoch=False)
diff --git a/xtuner/configs/internvl/v1_5/internvl_v1_5_phi3_4b_finetune.py b/xtuner/configs/internvl/v1_5/internvl_v1_5_phi3_4b_finetune.py
new file mode 100644
index 000000000..5d34a928b
--- /dev/null
+++ b/xtuner/configs/internvl/v1_5/internvl_v1_5_phi3_4b_finetune.py
@@ -0,0 +1,170 @@
+# Copyright (c) OpenMMLab. All rights reserved.
+from mmengine.hooks import (CheckpointHook, DistSamplerSeedHook, IterTimerHook,
+ LoggerHook, ParamSchedulerHook)
+from mmengine.optim import AmpOptimWrapper, CosineAnnealingLR, LinearLR
+from torch.optim import AdamW
+from transformers import AutoTokenizer
+
+from xtuner.dataset import InternVL_V1_5_Dataset
+from xtuner.dataset.collate_fns import default_collate_fn
+from xtuner.dataset.samplers import LengthGroupedSampler
+from xtuner.engine.hooks import DatasetInfoHook
+from xtuner.engine.runner import TrainLoop
+from xtuner.model import InternVL_V1_5
+from xtuner.utils import PROMPT_TEMPLATE
+
+#######################################################################
+# PART 1 Settings #
+#######################################################################
+# Model
+path = 'OpenGVLab/Mini-InternVL-Chat-4B-V1-5'
+
+# Data
+data_root = './data/llava_data/'
+data_path = data_root + 'LLaVA-Instruct-150K/llava_v1_5_mix665k.json'
+image_folder = data_root + 'llava_images'
+prompt_template = PROMPT_TEMPLATE.phi3_chat
+max_length = 8192
+
+# Scheduler & Optimizer
+batch_size = 4 # per_device
+accumulative_counts = 4
+dataloader_num_workers = 4
+max_epochs = 1
+optim_type = AdamW
+# official 1024 -> 4e-5
+lr = 1e-6
+betas = (0.9, 0.999)
+weight_decay = 0.05
+max_norm = 1 # grad clip
+warmup_ratio = 0.03
+
+# Save
+save_steps = 1000
+save_total_limit = 1 # Maximum checkpoints to keep (-1 means unlimited)
+
+#######################################################################
+# PART 2 Model & Tokenizer & Image Processor #
+#######################################################################
+model = dict(
+ type=InternVL_V1_5,
+ model_path=path,
+ freeze_llm=False,
+ freeze_visual_encoder=True # or False
+)
+
+#######################################################################
+# PART 3 Dataset & Dataloader #
+#######################################################################
+llava_dataset = dict(
+ type=InternVL_V1_5_Dataset,
+ model_path=path,
+ data_paths=data_path,
+ image_folders=image_folder,
+ template=prompt_template,
+ max_length=max_length)
+
+train_dataloader = dict(
+ batch_size=batch_size,
+ num_workers=dataloader_num_workers,
+ dataset=llava_dataset,
+ sampler=dict(
+ type=LengthGroupedSampler,
+ length_property='modality_length',
+ per_device_batch_size=batch_size * accumulative_counts),
+ collate_fn=dict(type=default_collate_fn))
+
+#######################################################################
+# PART 4 Scheduler & Optimizer #
+#######################################################################
+# optimizer
+optim_wrapper = dict(
+ type=AmpOptimWrapper,
+ optimizer=dict(
+ type=optim_type, lr=lr, betas=betas, weight_decay=weight_decay),
+ clip_grad=dict(max_norm=max_norm, error_if_nonfinite=False),
+ accumulative_counts=accumulative_counts,
+ loss_scale='dynamic',
+ dtype='float16')
+
+# learning policy
+# More information: https://github.com/open-mmlab/mmengine/blob/main/docs/en/tutorials/param_scheduler.md # noqa: E501
+param_scheduler = [
+ dict(
+ type=LinearLR,
+ start_factor=1e-5,
+ by_epoch=True,
+ begin=0,
+ end=warmup_ratio * max_epochs,
+ convert_to_iter_based=True),
+ dict(
+ type=CosineAnnealingLR,
+ eta_min=0.0,
+ by_epoch=True,
+ begin=warmup_ratio * max_epochs,
+ end=max_epochs,
+ convert_to_iter_based=True)
+]
+
+# train, val, test setting
+train_cfg = dict(type=TrainLoop, max_epochs=max_epochs)
+
+#######################################################################
+# PART 5 Runtime #
+#######################################################################
+# Log the dialogue periodically during the training process, optional
+tokenizer = dict(
+ type=AutoTokenizer.from_pretrained,
+ pretrained_model_name_or_path=path,
+ trust_remote_code=True)
+
+custom_hooks = [
+ dict(type=DatasetInfoHook, tokenizer=tokenizer),
+]
+
+# configure default hooks
+default_hooks = dict(
+ # record the time of every iteration.
+ timer=dict(type=IterTimerHook),
+ # print log every 10 iterations.
+ logger=dict(type=LoggerHook, log_metric_by_epoch=False, interval=10),
+ # enable the parameter scheduler.
+ param_scheduler=dict(type=ParamSchedulerHook),
+ # save checkpoint per `save_steps`.
+ checkpoint=dict(
+ type=CheckpointHook,
+ save_optimizer=False,
+ by_epoch=False,
+ interval=save_steps,
+ max_keep_ckpts=save_total_limit),
+ # set sampler seed in distributed evrionment.
+ sampler_seed=dict(type=DistSamplerSeedHook),
+)
+
+# configure environment
+env_cfg = dict(
+ # whether to enable cudnn benchmark
+ cudnn_benchmark=False,
+ # set multi process parameters
+ mp_cfg=dict(mp_start_method='fork', opencv_num_threads=0),
+ # set distributed parameters
+ dist_cfg=dict(backend='nccl'),
+)
+
+# set visualizer
+visualizer = None
+
+# set log level
+log_level = 'INFO'
+
+# load from which checkpoint
+load_from = None
+
+# whether to resume training from the loaded checkpoint
+resume = False
+
+# Defaults to use random seed and disable `deterministic`
+randomness = dict(seed=None, deterministic=False)
+
+# set log processor
+log_processor = dict(by_epoch=False)
diff --git a/xtuner/configs/internvl/v1_5/internvl_v1_5_phi3_4b_lora_finetune.py b/xtuner/configs/internvl/v1_5/internvl_v1_5_phi3_4b_lora_finetune.py
new file mode 100644
index 000000000..19588cb95
--- /dev/null
+++ b/xtuner/configs/internvl/v1_5/internvl_v1_5_phi3_4b_lora_finetune.py
@@ -0,0 +1,183 @@
+# Copyright (c) OpenMMLab. All rights reserved.
+from mmengine.hooks import (CheckpointHook, DistSamplerSeedHook, IterTimerHook,
+ LoggerHook, ParamSchedulerHook)
+from mmengine.optim import AmpOptimWrapper, CosineAnnealingLR, LinearLR
+from peft import LoraConfig
+from torch.optim import AdamW
+from transformers import AutoTokenizer
+
+from xtuner.dataset import InternVL_V1_5_Dataset
+from xtuner.dataset.collate_fns import default_collate_fn
+from xtuner.dataset.samplers import LengthGroupedSampler
+from xtuner.engine.hooks import DatasetInfoHook
+from xtuner.engine.runner import TrainLoop
+from xtuner.model import InternVL_V1_5
+from xtuner.utils import PROMPT_TEMPLATE
+
+#######################################################################
+# PART 1 Settings #
+#######################################################################
+# Model
+path = 'OpenGVLab/Mini-InternVL-Chat-4B-V1-5'
+
+# Data
+data_root = './data/llava_data/'
+data_path = data_root + 'LLaVA-Instruct-150K/llava_v1_5_mix665k.json'
+image_folder = data_root + 'llava_images'
+prompt_template = PROMPT_TEMPLATE.phi3_chat
+max_length = 8192
+
+# Scheduler & Optimizer
+batch_size = 8 # per_device
+accumulative_counts = 2
+dataloader_num_workers = 4
+max_epochs = 1
+optim_type = AdamW
+# official 1024 -> 4e-5
+lr = 1e-6
+betas = (0.9, 0.999)
+weight_decay = 0.05
+max_norm = 1 # grad clip
+warmup_ratio = 0.03
+
+# Save
+save_steps = 1000
+save_total_limit = 1 # Maximum checkpoints to keep (-1 means unlimited)
+
+#######################################################################
+# PART 2 Model & Tokenizer & Image Processor #
+#######################################################################
+model = dict(
+ type=InternVL_V1_5,
+ model_path=path,
+ freeze_llm=True,
+ freeze_visual_encoder=True,
+ # comment the following lines if you don't want to use Lora in llm
+ llm_lora=dict(
+ type=LoraConfig,
+ r=128,
+ lora_alpha=256,
+ lora_dropout=0.05,
+ target_modules=None,
+ task_type='CAUSAL_LM'),
+ # uncomment the following lines if you don't want to use Lora in visual encoder # noqa
+ # visual_encoder_lora=dict(
+ # type=LoraConfig, r=64, lora_alpha=16, lora_dropout=0.05,
+ # target_modules=['attn.qkv', 'attn.proj', 'mlp.fc1', 'mlp.fc2'])
+)
+
+#######################################################################
+# PART 3 Dataset & Dataloader #
+#######################################################################
+llava_dataset = dict(
+ type=InternVL_V1_5_Dataset,
+ model_path=path,
+ data_paths=data_path,
+ image_folders=image_folder,
+ template=prompt_template,
+ max_length=max_length)
+
+train_dataloader = dict(
+ batch_size=batch_size,
+ num_workers=dataloader_num_workers,
+ dataset=llava_dataset,
+ sampler=dict(
+ type=LengthGroupedSampler,
+ length_property='modality_length',
+ per_device_batch_size=batch_size * accumulative_counts),
+ collate_fn=dict(type=default_collate_fn))
+
+#######################################################################
+# PART 4 Scheduler & Optimizer #
+#######################################################################
+# optimizer
+optim_wrapper = dict(
+ type=AmpOptimWrapper,
+ optimizer=dict(
+ type=optim_type, lr=lr, betas=betas, weight_decay=weight_decay),
+ clip_grad=dict(max_norm=max_norm, error_if_nonfinite=False),
+ accumulative_counts=accumulative_counts,
+ loss_scale='dynamic',
+ dtype='float16')
+
+# learning policy
+# More information: https://github.com/open-mmlab/mmengine/blob/main/docs/en/tutorials/param_scheduler.md # noqa: E501
+param_scheduler = [
+ dict(
+ type=LinearLR,
+ start_factor=1e-5,
+ by_epoch=True,
+ begin=0,
+ end=warmup_ratio * max_epochs,
+ convert_to_iter_based=True),
+ dict(
+ type=CosineAnnealingLR,
+ eta_min=0.0,
+ by_epoch=True,
+ begin=warmup_ratio * max_epochs,
+ end=max_epochs,
+ convert_to_iter_based=True)
+]
+
+# train, val, test setting
+train_cfg = dict(type=TrainLoop, max_epochs=max_epochs)
+
+#######################################################################
+# PART 5 Runtime #
+#######################################################################
+# Log the dialogue periodically during the training process, optional
+tokenizer = dict(
+ type=AutoTokenizer.from_pretrained,
+ pretrained_model_name_or_path=path,
+ trust_remote_code=True)
+
+custom_hooks = [
+ dict(type=DatasetInfoHook, tokenizer=tokenizer),
+]
+
+# configure default hooks
+default_hooks = dict(
+ # record the time of every iteration.
+ timer=dict(type=IterTimerHook),
+ # print log every 10 iterations.
+ logger=dict(type=LoggerHook, log_metric_by_epoch=False, interval=10),
+ # enable the parameter scheduler.
+ param_scheduler=dict(type=ParamSchedulerHook),
+ # save checkpoint per `save_steps`.
+ checkpoint=dict(
+ type=CheckpointHook,
+ save_optimizer=False,
+ by_epoch=False,
+ interval=save_steps,
+ max_keep_ckpts=save_total_limit),
+ # set sampler seed in distributed evrionment.
+ sampler_seed=dict(type=DistSamplerSeedHook),
+)
+
+# configure environment
+env_cfg = dict(
+ # whether to enable cudnn benchmark
+ cudnn_benchmark=False,
+ # set multi process parameters
+ mp_cfg=dict(mp_start_method='fork', opencv_num_threads=0),
+ # set distributed parameters
+ dist_cfg=dict(backend='nccl'),
+)
+
+# set visualizer
+visualizer = None
+
+# set log level
+log_level = 'INFO'
+
+# load from which checkpoint
+load_from = None
+
+# whether to resume training from the loaded checkpoint
+resume = False
+
+# Defaults to use random seed and disable `deterministic`
+randomness = dict(seed=None, deterministic=False)
+
+# set log processor
+log_processor = dict(by_epoch=False)
diff --git a/xtuner/configs/internvl/v1_5/internvl_v1_5_phi3_4b_qlora_finetune.py b/xtuner/configs/internvl/v1_5/internvl_v1_5_phi3_4b_qlora_finetune.py
new file mode 100644
index 000000000..cb150f0c4
--- /dev/null
+++ b/xtuner/configs/internvl/v1_5/internvl_v1_5_phi3_4b_qlora_finetune.py
@@ -0,0 +1,185 @@
+# Copyright (c) OpenMMLab. All rights reserved.
+from mmengine.hooks import (CheckpointHook, DistSamplerSeedHook, IterTimerHook,
+ LoggerHook, ParamSchedulerHook)
+from mmengine.optim import AmpOptimWrapper, CosineAnnealingLR, LinearLR
+from peft import LoraConfig
+from torch.optim import AdamW
+from transformers import AutoTokenizer
+
+from xtuner.dataset import InternVL_V1_5_Dataset
+from xtuner.dataset.collate_fns import default_collate_fn
+from xtuner.dataset.samplers import LengthGroupedSampler
+from xtuner.engine.hooks import DatasetInfoHook
+from xtuner.engine.runner import TrainLoop
+from xtuner.model import InternVL_V1_5
+from xtuner.utils import PROMPT_TEMPLATE
+
+#######################################################################
+# PART 1 Settings #
+#######################################################################
+# Model
+path = 'OpenGVLab/Mini-InternVL-Chat-4B-V1-5'
+
+# Data
+data_root = './data/llava_data/'
+data_path = data_root + 'LLaVA-Instruct-150K/llava_v1_5_mix665k.json'
+image_folder = data_root + 'llava_images'
+prompt_template = PROMPT_TEMPLATE.phi3_chat
+max_length = 8192
+
+# Scheduler & Optimizer
+batch_size = 8 # per_device
+accumulative_counts = 2
+dataloader_num_workers = 4
+max_epochs = 1
+optim_type = AdamW
+# official 1024 -> 4e-5
+lr = 1e-6
+betas = (0.9, 0.999)
+weight_decay = 0.05
+max_norm = 1 # grad clip
+warmup_ratio = 0.03
+
+# Save
+save_steps = 1000
+save_total_limit = 1 # Maximum checkpoints to keep (-1 means unlimited)
+
+#######################################################################
+# PART 2 Model & Tokenizer & Image Processor #
+#######################################################################
+model = dict(
+ type=InternVL_V1_5,
+ model_path=path,
+ freeze_llm=True,
+ freeze_visual_encoder=True,
+ quantization_llm=True, # or False
+ quantization_vit=False, # or True and uncomment visual_encoder_lora
+ # comment the following lines if you don't want to use Lora in llm
+ llm_lora=dict(
+ type=LoraConfig,
+ r=128,
+ lora_alpha=256,
+ lora_dropout=0.05,
+ target_modules=None,
+ task_type='CAUSAL_LM'),
+ # uncomment the following lines if you don't want to use Lora in visual encoder # noqa
+ # visual_encoder_lora=dict(
+ # type=LoraConfig, r=64, lora_alpha=16, lora_dropout=0.05,
+ # target_modules=['attn.qkv', 'attn.proj', 'mlp.fc1', 'mlp.fc2'])
+)
+
+#######################################################################
+# PART 3 Dataset & Dataloader #
+#######################################################################
+llava_dataset = dict(
+ type=InternVL_V1_5_Dataset,
+ model_path=path,
+ data_paths=data_path,
+ image_folders=image_folder,
+ template=prompt_template,
+ max_length=max_length)
+
+train_dataloader = dict(
+ batch_size=batch_size,
+ num_workers=dataloader_num_workers,
+ dataset=llava_dataset,
+ sampler=dict(
+ type=LengthGroupedSampler,
+ length_property='modality_length',
+ per_device_batch_size=batch_size * accumulative_counts),
+ collate_fn=dict(type=default_collate_fn))
+
+#######################################################################
+# PART 4 Scheduler & Optimizer #
+#######################################################################
+# optimizer
+optim_wrapper = dict(
+ type=AmpOptimWrapper,
+ optimizer=dict(
+ type=optim_type, lr=lr, betas=betas, weight_decay=weight_decay),
+ clip_grad=dict(max_norm=max_norm, error_if_nonfinite=False),
+ accumulative_counts=accumulative_counts,
+ loss_scale='dynamic',
+ dtype='float16')
+
+# learning policy
+# More information: https://github.com/open-mmlab/mmengine/blob/main/docs/en/tutorials/param_scheduler.md # noqa: E501
+param_scheduler = [
+ dict(
+ type=LinearLR,
+ start_factor=1e-5,
+ by_epoch=True,
+ begin=0,
+ end=warmup_ratio * max_epochs,
+ convert_to_iter_based=True),
+ dict(
+ type=CosineAnnealingLR,
+ eta_min=0.0,
+ by_epoch=True,
+ begin=warmup_ratio * max_epochs,
+ end=max_epochs,
+ convert_to_iter_based=True)
+]
+
+# train, val, test setting
+train_cfg = dict(type=TrainLoop, max_epochs=max_epochs)
+
+#######################################################################
+# PART 5 Runtime #
+#######################################################################
+# Log the dialogue periodically during the training process, optional
+tokenizer = dict(
+ type=AutoTokenizer.from_pretrained,
+ pretrained_model_name_or_path=path,
+ trust_remote_code=True)
+
+custom_hooks = [
+ dict(type=DatasetInfoHook, tokenizer=tokenizer),
+]
+
+# configure default hooks
+default_hooks = dict(
+ # record the time of every iteration.
+ timer=dict(type=IterTimerHook),
+ # print log every 10 iterations.
+ logger=dict(type=LoggerHook, log_metric_by_epoch=False, interval=10),
+ # enable the parameter scheduler.
+ param_scheduler=dict(type=ParamSchedulerHook),
+ # save checkpoint per `save_steps`.
+ checkpoint=dict(
+ type=CheckpointHook,
+ save_optimizer=False,
+ by_epoch=False,
+ interval=save_steps,
+ max_keep_ckpts=save_total_limit),
+ # set sampler seed in distributed evrionment.
+ sampler_seed=dict(type=DistSamplerSeedHook),
+)
+
+# configure environment
+env_cfg = dict(
+ # whether to enable cudnn benchmark
+ cudnn_benchmark=False,
+ # set multi process parameters
+ mp_cfg=dict(mp_start_method='fork', opencv_num_threads=0),
+ # set distributed parameters
+ dist_cfg=dict(backend='nccl'),
+)
+
+# set visualizer
+visualizer = None
+
+# set log level
+log_level = 'INFO'
+
+# load from which checkpoint
+load_from = None
+
+# whether to resume training from the loaded checkpoint
+resume = False
+
+# Defaults to use random seed and disable `deterministic`
+randomness = dict(seed=None, deterministic=False)
+
+# set log processor
+log_processor = dict(by_epoch=False)
diff --git a/xtuner/configs/internvl/v2/internvl_v2_internlm2_26b_finetune.py b/xtuner/configs/internvl/v2/internvl_v2_internlm2_26b_finetune.py
new file mode 100644
index 000000000..0916df44a
--- /dev/null
+++ b/xtuner/configs/internvl/v2/internvl_v2_internlm2_26b_finetune.py
@@ -0,0 +1,170 @@
+# Copyright (c) OpenMMLab. All rights reserved.
+from mmengine.hooks import (CheckpointHook, DistSamplerSeedHook, IterTimerHook,
+ LoggerHook, ParamSchedulerHook)
+from mmengine.optim import AmpOptimWrapper, CosineAnnealingLR, LinearLR
+from torch.optim import AdamW
+from transformers import AutoTokenizer
+
+from xtuner.dataset import InternVL_V1_5_Dataset
+from xtuner.dataset.collate_fns import default_collate_fn
+from xtuner.dataset.samplers import LengthGroupedSampler
+from xtuner.engine.hooks import DatasetInfoHook
+from xtuner.engine.runner import TrainLoop
+from xtuner.model import InternVL_V1_5
+from xtuner.utils import PROMPT_TEMPLATE
+
+#######################################################################
+# PART 1 Settings #
+#######################################################################
+# Model
+path = 'OpenGVLab/InternVL2-26B'
+
+# Data
+data_root = './data/llava_data/'
+data_path = data_root + 'LLaVA-Instruct-150K/llava_v1_5_mix665k.json'
+image_folder = data_root + 'llava_images'
+prompt_template = PROMPT_TEMPLATE.internlm2_chat
+max_length = 8192
+
+# Scheduler & Optimizer
+batch_size = 1 # per_device
+accumulative_counts = 8
+dataloader_num_workers = 4
+max_epochs = 1
+optim_type = AdamW
+# official 1024 -> 4e-5
+lr = 1e-6
+betas = (0.9, 0.999)
+weight_decay = 0.05
+max_norm = 1 # grad clip
+warmup_ratio = 0.03
+
+# Save
+save_steps = 1000
+save_total_limit = 1 # Maximum checkpoints to keep (-1 means unlimited)
+
+#######################################################################
+# PART 2 Model & Tokenizer & Image Processor #
+#######################################################################
+model = dict(
+ type=InternVL_V1_5,
+ model_path=path,
+ freeze_llm=False,
+ freeze_visual_encoder=True # or False
+)
+
+#######################################################################
+# PART 3 Dataset & Dataloader #
+#######################################################################
+llava_dataset = dict(
+ type=InternVL_V1_5_Dataset,
+ model_path=path,
+ data_paths=data_path,
+ image_folders=image_folder,
+ template=prompt_template,
+ max_length=max_length)
+
+train_dataloader = dict(
+ batch_size=batch_size,
+ num_workers=dataloader_num_workers,
+ dataset=llava_dataset,
+ sampler=dict(
+ type=LengthGroupedSampler,
+ length_property='modality_length',
+ per_device_batch_size=batch_size * accumulative_counts),
+ collate_fn=dict(type=default_collate_fn))
+
+#######################################################################
+# PART 4 Scheduler & Optimizer #
+#######################################################################
+# optimizer
+optim_wrapper = dict(
+ type=AmpOptimWrapper,
+ optimizer=dict(
+ type=optim_type, lr=lr, betas=betas, weight_decay=weight_decay),
+ clip_grad=dict(max_norm=max_norm, error_if_nonfinite=False),
+ accumulative_counts=accumulative_counts,
+ loss_scale='dynamic',
+ dtype='float16')
+
+# learning policy
+# More information: https://github.com/open-mmlab/mmengine/blob/main/docs/en/tutorials/param_scheduler.md # noqa: E501
+param_scheduler = [
+ dict(
+ type=LinearLR,
+ start_factor=1e-5,
+ by_epoch=True,
+ begin=0,
+ end=warmup_ratio * max_epochs,
+ convert_to_iter_based=True),
+ dict(
+ type=CosineAnnealingLR,
+ eta_min=0.0,
+ by_epoch=True,
+ begin=warmup_ratio * max_epochs,
+ end=max_epochs,
+ convert_to_iter_based=True)
+]
+
+# train, val, test setting
+train_cfg = dict(type=TrainLoop, max_epochs=max_epochs)
+
+#######################################################################
+# PART 5 Runtime #
+#######################################################################
+# Log the dialogue periodically during the training process, optional
+tokenizer = dict(
+ type=AutoTokenizer.from_pretrained,
+ pretrained_model_name_or_path=path,
+ trust_remote_code=True)
+
+custom_hooks = [
+ dict(type=DatasetInfoHook, tokenizer=tokenizer),
+]
+
+# configure default hooks
+default_hooks = dict(
+ # record the time of every iteration.
+ timer=dict(type=IterTimerHook),
+ # print log every 10 iterations.
+ logger=dict(type=LoggerHook, log_metric_by_epoch=False, interval=10),
+ # enable the parameter scheduler.
+ param_scheduler=dict(type=ParamSchedulerHook),
+ # save checkpoint per `save_steps`.
+ checkpoint=dict(
+ type=CheckpointHook,
+ save_optimizer=False,
+ by_epoch=False,
+ interval=save_steps,
+ max_keep_ckpts=save_total_limit),
+ # set sampler seed in distributed evrionment.
+ sampler_seed=dict(type=DistSamplerSeedHook),
+)
+
+# configure environment
+env_cfg = dict(
+ # whether to enable cudnn benchmark
+ cudnn_benchmark=False,
+ # set multi process parameters
+ mp_cfg=dict(mp_start_method='fork', opencv_num_threads=0),
+ # set distributed parameters
+ dist_cfg=dict(backend='nccl'),
+)
+
+# set visualizer
+visualizer = None
+
+# set log level
+log_level = 'INFO'
+
+# load from which checkpoint
+load_from = None
+
+# whether to resume training from the loaded checkpoint
+resume = False
+
+# Defaults to use random seed and disable `deterministic`
+randomness = dict(seed=None, deterministic=False)
+
+# set log processor
+log_processor = dict(by_epoch=False)
diff --git a/xtuner/configs/internvl/v2/internvl_v2_internlm2_26b_lora_finetune.py b/xtuner/configs/internvl/v2/internvl_v2_internlm2_26b_lora_finetune.py
new file mode 100644
index 000000000..045fd7055
--- /dev/null
+++ b/xtuner/configs/internvl/v2/internvl_v2_internlm2_26b_lora_finetune.py
@@ -0,0 +1,183 @@
+# Copyright (c) OpenMMLab. All rights reserved.
+from mmengine.hooks import (CheckpointHook, DistSamplerSeedHook, IterTimerHook,
+ LoggerHook, ParamSchedulerHook)
+from mmengine.optim import AmpOptimWrapper, CosineAnnealingLR, LinearLR
+from peft import LoraConfig
+from torch.optim import AdamW
+from transformers import AutoTokenizer
+
+from xtuner.dataset import InternVL_V1_5_Dataset
+from xtuner.dataset.collate_fns import default_collate_fn
+from xtuner.dataset.samplers import LengthGroupedSampler
+from xtuner.engine.hooks import DatasetInfoHook
+from xtuner.engine.runner import TrainLoop
+from xtuner.model import InternVL_V1_5
+from xtuner.utils import PROMPT_TEMPLATE
+
+#######################################################################
+# PART 1 Settings #
+#######################################################################
+# Model
+path = 'OpenGVLab/InternVL2-26B'
+
+# Data
+data_root = './data/llava_data/'
+data_path = data_root + 'LLaVA-Instruct-150K/llava_v1_5_mix665k.json'
+image_folder = data_root + 'llava_images'
+prompt_template = PROMPT_TEMPLATE.internlm2_chat
+max_length = 8192
+
+# Scheduler & Optimizer
+batch_size = 2 # per_device
+accumulative_counts = 4
+dataloader_num_workers = 4
+max_epochs = 1
+optim_type = AdamW
+# official 1024 -> 4e-5
+lr = 1e-6
+betas = (0.9, 0.999)
+weight_decay = 0.05
+max_norm = 1 # grad clip
+warmup_ratio = 0.03
+
+# Save
+save_steps = 1000
+save_total_limit = 1 # Maximum checkpoints to keep (-1 means unlimited)
+
+#######################################################################
+# PART 2 Model & Tokenizer & Image Processor #
+#######################################################################
+model = dict(
+ type=InternVL_V1_5,
+ model_path=path,
+ freeze_llm=True,
+ freeze_visual_encoder=True,
+ # comment the following lines if you don't want to use Lora in llm
+ llm_lora=dict(
+ type=LoraConfig,
+ r=128,
+ lora_alpha=256,
+ lora_dropout=0.05,
+ target_modules=None,
+ task_type='CAUSAL_LM'),
+ # uncomment the following lines if you don't want to use Lora in visual encoder # noqa
+ # visual_encoder_lora=dict(
+ # type=LoraConfig, r=64, lora_alpha=16, lora_dropout=0.05,
+ # target_modules=['attn.qkv', 'attn.proj', 'mlp.fc1', 'mlp.fc2'])
+)
+
+#######################################################################
+# PART 3 Dataset & Dataloader #
+#######################################################################
+llava_dataset = dict(
+ type=InternVL_V1_5_Dataset,
+ model_path=path,
+ data_paths=data_path,
+ image_folders=image_folder,
+ template=prompt_template,
+ max_length=max_length)
+
+train_dataloader = dict(
+ batch_size=batch_size,
+ num_workers=dataloader_num_workers,
+ dataset=llava_dataset,
+ sampler=dict(
+ type=LengthGroupedSampler,
+ length_property='modality_length',
+ per_device_batch_size=batch_size * accumulative_counts),
+ collate_fn=dict(type=default_collate_fn))
+
+#######################################################################
+# PART 4 Scheduler & Optimizer #
+#######################################################################
+# optimizer
+optim_wrapper = dict(
+ type=AmpOptimWrapper,
+ optimizer=dict(
+ type=optim_type, lr=lr, betas=betas, weight_decay=weight_decay),
+ clip_grad=dict(max_norm=max_norm, error_if_nonfinite=False),
+ accumulative_counts=accumulative_counts,
+ loss_scale='dynamic',
+ dtype='float16')
+
+# learning policy
+# More information: https://github.com/open-mmlab/mmengine/blob/main/docs/en/tutorials/param_scheduler.md # noqa: E501
+param_scheduler = [
+ dict(
+ type=LinearLR,
+ start_factor=1e-5,
+ by_epoch=True,
+ begin=0,
+ end=warmup_ratio * max_epochs,
+ convert_to_iter_based=True),
+ dict(
+ type=CosineAnnealingLR,
+ eta_min=0.0,
+ by_epoch=True,
+ begin=warmup_ratio * max_epochs,
+ end=max_epochs,
+ convert_to_iter_based=True)
+]
+
+# train, val, test setting
+train_cfg = dict(type=TrainLoop, max_epochs=max_epochs)
+
+#######################################################################
+# PART 5 Runtime #
+#######################################################################
+# Log the dialogue periodically during the training process, optional
+tokenizer = dict(
+ type=AutoTokenizer.from_pretrained,
+ pretrained_model_name_or_path=path,
+ trust_remote_code=True)
+
+custom_hooks = [
+ dict(type=DatasetInfoHook, tokenizer=tokenizer),
+]
+
+# configure default hooks
+default_hooks = dict(
+ # record the time of every iteration.
+ timer=dict(type=IterTimerHook),
+ # print log every 10 iterations.
+ logger=dict(type=LoggerHook, log_metric_by_epoch=False, interval=10),
+ # enable the parameter scheduler.
+ param_scheduler=dict(type=ParamSchedulerHook),
+ # save checkpoint per `save_steps`.
+ checkpoint=dict(
+ type=CheckpointHook,
+ save_optimizer=False,
+ by_epoch=False,
+ interval=save_steps,
+ max_keep_ckpts=save_total_limit),
+ # set sampler seed in distributed evrionment.
+ sampler_seed=dict(type=DistSamplerSeedHook),
+)
+
+# configure environment
+env_cfg = dict(
+ # whether to enable cudnn benchmark
+ cudnn_benchmark=False,
+ # set multi process parameters
+ mp_cfg=dict(mp_start_method='fork', opencv_num_threads=0),
+ # set distributed parameters
+ dist_cfg=dict(backend='nccl'),
+)
+
+# set visualizer
+visualizer = None
+
+# set log level
+log_level = 'INFO'
+
+# load from which checkpoint
+load_from = None
+
+# whether to resume training from the loaded checkpoint
+resume = False
+
+# Defaults to use random seed and disable `deterministic`
+randomness = dict(seed=None, deterministic=False)
+
+# set log processor
+log_processor = dict(by_epoch=False)
diff --git a/xtuner/configs/internvl/v2/internvl_v2_internlm2_26b_qlora_finetune.py b/xtuner/configs/internvl/v2/internvl_v2_internlm2_26b_qlora_finetune.py
new file mode 100644
index 000000000..60717b312
--- /dev/null
+++ b/xtuner/configs/internvl/v2/internvl_v2_internlm2_26b_qlora_finetune.py
@@ -0,0 +1,185 @@
+# Copyright (c) OpenMMLab. All rights reserved.
+from mmengine.hooks import (CheckpointHook, DistSamplerSeedHook, IterTimerHook,
+ LoggerHook, ParamSchedulerHook)
+from mmengine.optim import AmpOptimWrapper, CosineAnnealingLR, LinearLR
+from peft import LoraConfig
+from torch.optim import AdamW
+from transformers import AutoTokenizer
+
+from xtuner.dataset import InternVL_V1_5_Dataset
+from xtuner.dataset.collate_fns import default_collate_fn
+from xtuner.dataset.samplers import LengthGroupedSampler
+from xtuner.engine.hooks import DatasetInfoHook
+from xtuner.engine.runner import TrainLoop
+from xtuner.model import InternVL_V1_5
+from xtuner.utils import PROMPT_TEMPLATE
+
+#######################################################################
+# PART 1 Settings #
+#######################################################################
+# Model
+path = 'OpenGVLab/InternVL2-26B'
+
+# Data
+data_root = './data/llava_data/'
+data_path = data_root + 'LLaVA-Instruct-150K/llava_v1_5_mix665k.json'
+image_folder = data_root + 'llava_images'
+prompt_template = PROMPT_TEMPLATE.internlm2_chat
+max_length = 8192
+
+# Scheduler & Optimizer
+batch_size = 2 # per_device
+accumulative_counts = 4
+dataloader_num_workers = 4
+max_epochs = 1
+optim_type = AdamW
+# official 1024 -> 4e-5
+lr = 1e-6
+betas = (0.9, 0.999)
+weight_decay = 0.05
+max_norm = 1 # grad clip
+warmup_ratio = 0.03
+
+# Save
+save_steps = 1000
+save_total_limit = 1 # Maximum checkpoints to keep (-1 means unlimited)
+
+#######################################################################
+# PART 2 Model & Tokenizer & Image Processor #
+#######################################################################
+model = dict(
+ type=InternVL_V1_5,
+ model_path=path,
+ freeze_llm=True,
+ freeze_visual_encoder=True,
+ quantization_llm=True, # or False
+ quantization_vit=False, # or True and uncomment visual_encoder_lora
+ # comment the following lines if you don't want to use Lora in llm
+ llm_lora=dict(
+ type=LoraConfig,
+ r=128,
+ lora_alpha=256,
+ lora_dropout=0.05,
+ target_modules=None,
+ task_type='CAUSAL_LM'),
+ # uncomment the following lines if you don't want to use Lora in visual encoder # noqa
+ # visual_encoder_lora=dict(
+ # type=LoraConfig, r=64, lora_alpha=16, lora_dropout=0.05,
+ # target_modules=['attn.qkv', 'attn.proj', 'mlp.fc1', 'mlp.fc2'])
+)
+
+#######################################################################
+# PART 3 Dataset & Dataloader #
+#######################################################################
+llava_dataset = dict(
+ type=InternVL_V1_5_Dataset,
+ model_path=path,
+ data_paths=data_path,
+ image_folders=image_folder,
+ template=prompt_template,
+ max_length=max_length)
+
+train_dataloader = dict(
+ batch_size=batch_size,
+ num_workers=dataloader_num_workers,
+ dataset=llava_dataset,
+ sampler=dict(
+ type=LengthGroupedSampler,
+ length_property='modality_length',
+ per_device_batch_size=batch_size * accumulative_counts),
+ collate_fn=dict(type=default_collate_fn))
+
+#######################################################################
+# PART 4 Scheduler & Optimizer #
+#######################################################################
+# optimizer
+optim_wrapper = dict(
+ type=AmpOptimWrapper,
+ optimizer=dict(
+ type=optim_type, lr=lr, betas=betas, weight_decay=weight_decay),
+ clip_grad=dict(max_norm=max_norm, error_if_nonfinite=False),
+ accumulative_counts=accumulative_counts,
+ loss_scale='dynamic',
+ dtype='float16')
+
+# learning policy
+# More information: https://github.com/open-mmlab/mmengine/blob/main/docs/en/tutorials/param_scheduler.md # noqa: E501
+param_scheduler = [
+ dict(
+ type=LinearLR,
+ start_factor=1e-5,
+ by_epoch=True,
+ begin=0,
+ end=warmup_ratio * max_epochs,
+ convert_to_iter_based=True),
+ dict(
+ type=CosineAnnealingLR,
+ eta_min=0.0,
+ by_epoch=True,
+ begin=warmup_ratio * max_epochs,
+ end=max_epochs,
+ convert_to_iter_based=True)
+]
+
+# train, val, test setting
+train_cfg = dict(type=TrainLoop, max_epochs=max_epochs)
+
+#######################################################################
+# PART 5 Runtime #
+#######################################################################
+# Log the dialogue periodically during the training process, optional
+tokenizer = dict(
+ type=AutoTokenizer.from_pretrained,
+ pretrained_model_name_or_path=path,
+ trust_remote_code=True)
+
+custom_hooks = [
+ dict(type=DatasetInfoHook, tokenizer=tokenizer),
+]
+
+# configure default hooks
+default_hooks = dict(
+ # record the time of every iteration.
+ timer=dict(type=IterTimerHook),
+ # print log every 10 iterations.
+ logger=dict(type=LoggerHook, log_metric_by_epoch=False, interval=10),
+ # enable the parameter scheduler.
+ param_scheduler=dict(type=ParamSchedulerHook),
+ # save checkpoint per `save_steps`.
+ checkpoint=dict(
+ type=CheckpointHook,
+ save_optimizer=False,
+ by_epoch=False,
+ interval=save_steps,
+ max_keep_ckpts=save_total_limit),
+ # set sampler seed in distributed evrionment.
+ sampler_seed=dict(type=DistSamplerSeedHook),
+)
+
+# configure environment
+env_cfg = dict(
+ # whether to enable cudnn benchmark
+ cudnn_benchmark=False,
+ # set multi process parameters
+ mp_cfg=dict(mp_start_method='fork', opencv_num_threads=0),
+ # set distributed parameters
+ dist_cfg=dict(backend='nccl'),
+)
+
+# set visualizer
+visualizer = None
+
+# set log level
+log_level = 'INFO'
+
+# load from which checkpoint
+load_from = None
+
+# whether to resume training from the loaded checkpoint
+resume = False
+
+# Defaults to use random seed and disable `deterministic`
+randomness = dict(seed=None, deterministic=False)
+
+# set log processor
+log_processor = dict(by_epoch=False)
diff --git a/xtuner/configs/internvl/v2/internvl_v2_internlm2_2b_finetune.py b/xtuner/configs/internvl/v2/internvl_v2_internlm2_2b_finetune.py
new file mode 100644
index 000000000..a921cf0c0
--- /dev/null
+++ b/xtuner/configs/internvl/v2/internvl_v2_internlm2_2b_finetune.py
@@ -0,0 +1,170 @@
+# Copyright (c) OpenMMLab. All rights reserved.
+from mmengine.hooks import (CheckpointHook, DistSamplerSeedHook, IterTimerHook,
+ LoggerHook, ParamSchedulerHook)
+from mmengine.optim import AmpOptimWrapper, CosineAnnealingLR, LinearLR
+from torch.optim import AdamW
+from transformers import AutoTokenizer
+
+from xtuner.dataset import InternVL_V1_5_Dataset
+from xtuner.dataset.collate_fns import default_collate_fn
+from xtuner.dataset.samplers import LengthGroupedSampler
+from xtuner.engine.hooks import DatasetInfoHook
+from xtuner.engine.runner import TrainLoop
+from xtuner.model import InternVL_V1_5
+from xtuner.utils import PROMPT_TEMPLATE
+
+#######################################################################
+# PART 1 Settings #
+#######################################################################
+# Model
+path = 'OpenGVLab/InternVL2-2B'
+
+# Data
+data_root = './data/llava_data/'
+data_path = data_root + 'LLaVA-Instruct-150K/llava_v1_5_mix665k.json'
+image_folder = data_root + 'llava_images'
+prompt_template = PROMPT_TEMPLATE.internlm2_chat
+max_length = 8192
+
+# Scheduler & Optimizer
+batch_size = 4 # per_device
+accumulative_counts = 4
+dataloader_num_workers = 4
+max_epochs = 1
+optim_type = AdamW
+# official 1024 -> 4e-5
+lr = 1e-6
+betas = (0.9, 0.999)
+weight_decay = 0.05
+max_norm = 1 # grad clip
+warmup_ratio = 0.03
+
+# Save
+save_steps = 1000
+save_total_limit = 1 # Maximum checkpoints to keep (-1 means unlimited)
+
+#######################################################################
+# PART 2 Model & Tokenizer & Image Processor #
+#######################################################################
+model = dict(
+ type=InternVL_V1_5,
+ model_path=path,
+ freeze_llm=False,
+ freeze_visual_encoder=True # or False
+)
+
+#######################################################################
+# PART 3 Dataset & Dataloader #
+#######################################################################
+llava_dataset = dict(
+ type=InternVL_V1_5_Dataset,
+ model_path=path,
+ data_paths=data_path,
+ image_folders=image_folder,
+ template=prompt_template,
+ max_length=max_length)
+
+train_dataloader = dict(
+ batch_size=batch_size,
+ num_workers=dataloader_num_workers,
+ dataset=llava_dataset,
+ sampler=dict(
+ type=LengthGroupedSampler,
+ length_property='modality_length',
+ per_device_batch_size=batch_size * accumulative_counts),
+ collate_fn=dict(type=default_collate_fn))
+
+#######################################################################
+# PART 4 Scheduler & Optimizer #
+#######################################################################
+# optimizer
+optim_wrapper = dict(
+ type=AmpOptimWrapper,
+ optimizer=dict(
+ type=optim_type, lr=lr, betas=betas, weight_decay=weight_decay),
+ clip_grad=dict(max_norm=max_norm, error_if_nonfinite=False),
+ accumulative_counts=accumulative_counts,
+ loss_scale='dynamic',
+ dtype='float16')
+
+# learning policy
+# More information: https://github.com/open-mmlab/mmengine/blob/main/docs/en/tutorials/param_scheduler.md # noqa: E501
+param_scheduler = [
+ dict(
+ type=LinearLR,
+ start_factor=1e-5,
+ by_epoch=True,
+ begin=0,
+ end=warmup_ratio * max_epochs,
+ convert_to_iter_based=True),
+ dict(
+ type=CosineAnnealingLR,
+ eta_min=0.0,
+ by_epoch=True,
+ begin=warmup_ratio * max_epochs,
+ end=max_epochs,
+ convert_to_iter_based=True)
+]
+
+# train, val, test setting
+train_cfg = dict(type=TrainLoop, max_epochs=max_epochs)
+
+#######################################################################
+# PART 5 Runtime #
+#######################################################################
+# Log the dialogue periodically during the training process, optional
+tokenizer = dict(
+ type=AutoTokenizer.from_pretrained,
+ pretrained_model_name_or_path=path,
+ trust_remote_code=True)
+
+custom_hooks = [
+ dict(type=DatasetInfoHook, tokenizer=tokenizer),
+]
+
+# configure default hooks
+default_hooks = dict(
+ # record the time of every iteration.
+ timer=dict(type=IterTimerHook),
+ # print log every 10 iterations.
+ logger=dict(type=LoggerHook, log_metric_by_epoch=False, interval=10),
+ # enable the parameter scheduler.
+ param_scheduler=dict(type=ParamSchedulerHook),
+ # save checkpoint per `save_steps`.
+ checkpoint=dict(
+ type=CheckpointHook,
+ save_optimizer=False,
+ by_epoch=False,
+ interval=save_steps,
+ max_keep_ckpts=save_total_limit),
+ # set sampler seed in distributed evrionment.
+ sampler_seed=dict(type=DistSamplerSeedHook),
+)
+
+# configure environment
+env_cfg = dict(
+ # whether to enable cudnn benchmark
+ cudnn_benchmark=False,
+ # set multi process parameters
+ mp_cfg=dict(mp_start_method='fork', opencv_num_threads=0),
+ # set distributed parameters
+ dist_cfg=dict(backend='nccl'),
+)
+
+# set visualizer
+visualizer = None
+
+# set log level
+log_level = 'INFO'
+
+# load from which checkpoint
+load_from = None
+
+# whether to resume training from the loaded checkpoint
+resume = False
+
+# Defaults to use random seed and disable `deterministic`
+randomness = dict(seed=None, deterministic=False)
+
+# set log processor
+log_processor = dict(by_epoch=False)
diff --git a/xtuner/configs/internvl/v2/internvl_v2_internlm2_2b_lora_finetune.py b/xtuner/configs/internvl/v2/internvl_v2_internlm2_2b_lora_finetune.py
new file mode 100644
index 000000000..44b3c3944
--- /dev/null
+++ b/xtuner/configs/internvl/v2/internvl_v2_internlm2_2b_lora_finetune.py
@@ -0,0 +1,183 @@
+# Copyright (c) OpenMMLab. All rights reserved.
+from mmengine.hooks import (CheckpointHook, DistSamplerSeedHook, IterTimerHook,
+ LoggerHook, ParamSchedulerHook)
+from mmengine.optim import AmpOptimWrapper, CosineAnnealingLR, LinearLR
+from peft import LoraConfig
+from torch.optim import AdamW
+from transformers import AutoTokenizer
+
+from xtuner.dataset import InternVL_V1_5_Dataset
+from xtuner.dataset.collate_fns import default_collate_fn
+from xtuner.dataset.samplers import LengthGroupedSampler
+from xtuner.engine.hooks import DatasetInfoHook
+from xtuner.engine.runner import TrainLoop
+from xtuner.model import InternVL_V1_5
+from xtuner.utils import PROMPT_TEMPLATE
+
+#######################################################################
+# PART 1 Settings #
+#######################################################################
+# Model
+path = 'OpenGVLab/InternVL2-2B'
+
+# Data
+data_root = './data/llava_data/'
+data_path = data_root + 'LLaVA-Instruct-150K/llava_v1_5_mix665k.json'
+image_folder = data_root + 'llava_images'
+prompt_template = PROMPT_TEMPLATE.internlm2_chat
+max_length = 8192
+
+# Scheduler & Optimizer
+batch_size = 8 # per_device
+accumulative_counts = 2
+dataloader_num_workers = 4
+max_epochs = 1
+optim_type = AdamW
+# official 1024 -> 4e-5
+lr = 1e-6
+betas = (0.9, 0.999)
+weight_decay = 0.05
+max_norm = 1 # grad clip
+warmup_ratio = 0.03
+
+# Save
+save_steps = 1000
+save_total_limit = 1 # Maximum checkpoints to keep (-1 means unlimited)
+
+#######################################################################
+# PART 2 Model & Tokenizer & Image Processor #
+#######################################################################
+model = dict(
+ type=InternVL_V1_5,
+ model_path=path,
+ freeze_llm=True,
+ freeze_visual_encoder=True,
+ # comment the following lines if you don't want to use Lora in llm
+ llm_lora=dict(
+ type=LoraConfig,
+ r=128,
+ lora_alpha=256,
+ lora_dropout=0.05,
+ target_modules=None,
+ task_type='CAUSAL_LM'),
+ # uncomment the following lines if you don't want to use Lora in visual encoder # noqa
+ # visual_encoder_lora=dict(
+ # type=LoraConfig, r=64, lora_alpha=16, lora_dropout=0.05,
+ # target_modules=['attn.qkv', 'attn.proj', 'mlp.fc1', 'mlp.fc2'])
+)
+
+#######################################################################
+# PART 3 Dataset & Dataloader #
+#######################################################################
+llava_dataset = dict(
+ type=InternVL_V1_5_Dataset,
+ model_path=path,
+ data_paths=data_path,
+ image_folders=image_folder,
+ template=prompt_template,
+ max_length=max_length)
+
+train_dataloader = dict(
+ batch_size=batch_size,
+ num_workers=dataloader_num_workers,
+ dataset=llava_dataset,
+ sampler=dict(
+ type=LengthGroupedSampler,
+ length_property='modality_length',
+ per_device_batch_size=batch_size * accumulative_counts),
+ collate_fn=dict(type=default_collate_fn))
+
+#######################################################################
+# PART 4 Scheduler & Optimizer #
+#######################################################################
+# optimizer
+optim_wrapper = dict(
+ type=AmpOptimWrapper,
+ optimizer=dict(
+ type=optim_type, lr=lr, betas=betas, weight_decay=weight_decay),
+ clip_grad=dict(max_norm=max_norm, error_if_nonfinite=False),
+ accumulative_counts=accumulative_counts,
+ loss_scale='dynamic',
+ dtype='float16')
+
+# learning policy
+# More information: https://github.com/open-mmlab/mmengine/blob/main/docs/en/tutorials/param_scheduler.md # noqa: E501
+param_scheduler = [
+ dict(
+ type=LinearLR,
+ start_factor=1e-5,
+ by_epoch=True,
+ begin=0,
+ end=warmup_ratio * max_epochs,
+ convert_to_iter_based=True),
+ dict(
+ type=CosineAnnealingLR,
+ eta_min=0.0,
+ by_epoch=True,
+ begin=warmup_ratio * max_epochs,
+ end=max_epochs,
+ convert_to_iter_based=True)
+]
+
+# train, val, test setting
+train_cfg = dict(type=TrainLoop, max_epochs=max_epochs)
+
+#######################################################################
+# PART 5 Runtime #
+#######################################################################
+# Log the dialogue periodically during the training process, optional
+tokenizer = dict(
+ type=AutoTokenizer.from_pretrained,
+ pretrained_model_name_or_path=path,
+ trust_remote_code=True)
+
+custom_hooks = [
+ dict(type=DatasetInfoHook, tokenizer=tokenizer),
+]
+
+# configure default hooks
+default_hooks = dict(
+ # record the time of every iteration.
+ timer=dict(type=IterTimerHook),
+ # print log every 10 iterations.
+ logger=dict(type=LoggerHook, log_metric_by_epoch=False, interval=10),
+ # enable the parameter scheduler.
+ param_scheduler=dict(type=ParamSchedulerHook),
+ # save checkpoint per `save_steps`.
+ checkpoint=dict(
+ type=CheckpointHook,
+ save_optimizer=False,
+ by_epoch=False,
+ interval=save_steps,
+ max_keep_ckpts=save_total_limit),
+ # set sampler seed in distributed evrionment.
+ sampler_seed=dict(type=DistSamplerSeedHook),
+)
+
+# configure environment
+env_cfg = dict(
+ # whether to enable cudnn benchmark
+ cudnn_benchmark=False,
+ # set multi process parameters
+ mp_cfg=dict(mp_start_method='fork', opencv_num_threads=0),
+ # set distributed parameters
+ dist_cfg=dict(backend='nccl'),
+)
+
+# set visualizer
+visualizer = None
+
+# set log level
+log_level = 'INFO'
+
+# load from which checkpoint
+load_from = None
+
+# whether to resume training from the loaded checkpoint
+resume = False
+
+# Defaults to use random seed and disable `deterministic`
+randomness = dict(seed=None, deterministic=False)
+
+# set log processor
+log_processor = dict(by_epoch=False)
diff --git a/xtuner/configs/internvl/v2/internvl_v2_internlm2_2b_qlora_finetune.py b/xtuner/configs/internvl/v2/internvl_v2_internlm2_2b_qlora_finetune.py
new file mode 100644
index 000000000..5840a593f
--- /dev/null
+++ b/xtuner/configs/internvl/v2/internvl_v2_internlm2_2b_qlora_finetune.py
@@ -0,0 +1,185 @@
+# Copyright (c) OpenMMLab. All rights reserved.
+from mmengine.hooks import (CheckpointHook, DistSamplerSeedHook, IterTimerHook,
+ LoggerHook, ParamSchedulerHook)
+from mmengine.optim import AmpOptimWrapper, CosineAnnealingLR, LinearLR
+from peft import LoraConfig
+from torch.optim import AdamW
+from transformers import AutoTokenizer
+
+from xtuner.dataset import InternVL_V1_5_Dataset
+from xtuner.dataset.collate_fns import default_collate_fn
+from xtuner.dataset.samplers import LengthGroupedSampler
+from xtuner.engine.hooks import DatasetInfoHook
+from xtuner.engine.runner import TrainLoop
+from xtuner.model import InternVL_V1_5
+from xtuner.utils import PROMPT_TEMPLATE
+
+#######################################################################
+# PART 1 Settings #
+#######################################################################
+# Model
+path = 'OpenGVLab/InternVL2-2B'
+
+# Data
+data_root = './data/llava_data/'
+data_path = data_root + 'LLaVA-Instruct-150K/llava_v1_5_mix665k.json'
+image_folder = data_root + 'llava_images'
+prompt_template = PROMPT_TEMPLATE.internlm2_chat
+max_length = 8192
+
+# Scheduler & Optimizer
+batch_size = 8 # per_device
+accumulative_counts = 2
+dataloader_num_workers = 4
+max_epochs = 1
+optim_type = AdamW
+# official 1024 -> 4e-5
+lr = 1e-6
+betas = (0.9, 0.999)
+weight_decay = 0.05
+max_norm = 1 # grad clip
+warmup_ratio = 0.03
+
+# Save
+save_steps = 1000
+save_total_limit = 1 # Maximum checkpoints to keep (-1 means unlimited)
+
+#######################################################################
+# PART 2 Model & Tokenizer & Image Processor #
+#######################################################################
+model = dict(
+ type=InternVL_V1_5,
+ model_path=path,
+ freeze_llm=True,
+ freeze_visual_encoder=True,
+ quantization_llm=True, # or False
+ quantization_vit=False, # or True and uncomment visual_encoder_lora
+ # comment the following lines if you don't want to use Lora in llm
+ llm_lora=dict(
+ type=LoraConfig,
+ r=128,
+ lora_alpha=256,
+ lora_dropout=0.05,
+ target_modules=None,
+ task_type='CAUSAL_LM'),
+ # uncomment the following lines if you don't want to use Lora in visual encoder # noqa
+ # visual_encoder_lora=dict(
+ # type=LoraConfig, r=64, lora_alpha=16, lora_dropout=0.05,
+ # target_modules=['attn.qkv', 'attn.proj', 'mlp.fc1', 'mlp.fc2'])
+)
+
+#######################################################################
+# PART 3 Dataset & Dataloader #
+#######################################################################
+llava_dataset = dict(
+ type=InternVL_V1_5_Dataset,
+ model_path=path,
+ data_paths=data_path,
+ image_folders=image_folder,
+ template=prompt_template,
+ max_length=max_length)
+
+train_dataloader = dict(
+ batch_size=batch_size,
+ num_workers=dataloader_num_workers,
+ dataset=llava_dataset,
+ sampler=dict(
+ type=LengthGroupedSampler,
+ length_property='modality_length',
+ per_device_batch_size=batch_size * accumulative_counts),
+ collate_fn=dict(type=default_collate_fn))
+
+#######################################################################
+# PART 4 Scheduler & Optimizer #
+#######################################################################
+# optimizer
+optim_wrapper = dict(
+ type=AmpOptimWrapper,
+ optimizer=dict(
+ type=optim_type, lr=lr, betas=betas, weight_decay=weight_decay),
+ clip_grad=dict(max_norm=max_norm, error_if_nonfinite=False),
+ accumulative_counts=accumulative_counts,
+ loss_scale='dynamic',
+ dtype='float16')
+
+# learning policy
+# More information: https://github.com/open-mmlab/mmengine/blob/main/docs/en/tutorials/param_scheduler.md # noqa: E501
+param_scheduler = [
+ dict(
+ type=LinearLR,
+ start_factor=1e-5,
+ by_epoch=True,
+ begin=0,
+ end=warmup_ratio * max_epochs,
+ convert_to_iter_based=True),
+ dict(
+ type=CosineAnnealingLR,
+ eta_min=0.0,
+ by_epoch=True,
+ begin=warmup_ratio * max_epochs,
+ end=max_epochs,
+ convert_to_iter_based=True)
+]
+
+# train, val, test setting
+train_cfg = dict(type=TrainLoop, max_epochs=max_epochs)
+
+#######################################################################
+# PART 5 Runtime #
+#######################################################################
+# Log the dialogue periodically during the training process, optional
+tokenizer = dict(
+ type=AutoTokenizer.from_pretrained,
+ pretrained_model_name_or_path=path,
+ trust_remote_code=True)
+
+custom_hooks = [
+ dict(type=DatasetInfoHook, tokenizer=tokenizer),
+]
+
+# configure default hooks
+default_hooks = dict(
+ # record the time of every iteration.
+ timer=dict(type=IterTimerHook),
+ # print log every 10 iterations.
+ logger=dict(type=LoggerHook, log_metric_by_epoch=False, interval=10),
+ # enable the parameter scheduler.
+ param_scheduler=dict(type=ParamSchedulerHook),
+ # save checkpoint per `save_steps`.
+ checkpoint=dict(
+ type=CheckpointHook,
+ save_optimizer=False,
+ by_epoch=False,
+ interval=save_steps,
+ max_keep_ckpts=save_total_limit),
+ # set sampler seed in distributed evrionment.
+ sampler_seed=dict(type=DistSamplerSeedHook),
+)
+
+# configure environment
+env_cfg = dict(
+ # whether to enable cudnn benchmark
+ cudnn_benchmark=False,
+ # set multi process parameters
+ mp_cfg=dict(mp_start_method='fork', opencv_num_threads=0),
+ # set distributed parameters
+ dist_cfg=dict(backend='nccl'),
+)
+
+# set visualizer
+visualizer = None
+
+# set log level
+log_level = 'INFO'
+
+# load from which checkpoint
+load_from = None
+
+# whether to resume training from the loaded checkpoint
+resume = False
+
+# Defaults to use random seed and disable `deterministic`
+randomness = dict(seed=None, deterministic=False)
+
+# set log processor
+log_processor = dict(by_epoch=False)
diff --git a/xtuner/configs/internvl/v2/internvl_v2_internlm2_5_8b_finetune.py b/xtuner/configs/internvl/v2/internvl_v2_internlm2_5_8b_finetune.py
new file mode 100644
index 000000000..2a92c017f
--- /dev/null
+++ b/xtuner/configs/internvl/v2/internvl_v2_internlm2_5_8b_finetune.py
@@ -0,0 +1,170 @@
+# Copyright (c) OpenMMLab. All rights reserved.
+from mmengine.hooks import (CheckpointHook, DistSamplerSeedHook, IterTimerHook,
+ LoggerHook, ParamSchedulerHook)
+from mmengine.optim import AmpOptimWrapper, CosineAnnealingLR, LinearLR
+from torch.optim import AdamW
+from transformers import AutoTokenizer
+
+from xtuner.dataset import InternVL_V1_5_Dataset
+from xtuner.dataset.collate_fns import default_collate_fn
+from xtuner.dataset.samplers import LengthGroupedSampler
+from xtuner.engine.hooks import DatasetInfoHook
+from xtuner.engine.runner import TrainLoop
+from xtuner.model import InternVL_V1_5
+from xtuner.utils import PROMPT_TEMPLATE
+
+#######################################################################
+# PART 1 Settings #
+#######################################################################
+# Model
+path = 'OpenGVLab/InternVL2-8B'
+
+# Data
+data_root = './data/llava_data/'
+data_path = data_root + 'LLaVA-Instruct-150K/llava_v1_5_mix665k.json'
+image_folder = data_root + 'llava_images'
+prompt_template = PROMPT_TEMPLATE.internlm2_chat
+max_length = 8192
+
+# Scheduler & Optimizer
+batch_size = 4 # per_device
+accumulative_counts = 4
+dataloader_num_workers = 4
+max_epochs = 1
+optim_type = AdamW
+# official 1024 -> 4e-5
+lr = 1e-6
+betas = (0.9, 0.999)
+weight_decay = 0.05
+max_norm = 1 # grad clip
+warmup_ratio = 0.03
+
+# Save
+save_steps = 1000
+save_total_limit = 1 # Maximum checkpoints to keep (-1 means unlimited)
+
+#######################################################################
+# PART 2 Model & Tokenizer & Image Processor #
+#######################################################################
+model = dict(
+ type=InternVL_V1_5,
+ model_path=path,
+ freeze_llm=False,
+ freeze_visual_encoder=True # or False
+)
+
+#######################################################################
+# PART 3 Dataset & Dataloader #
+#######################################################################
+llava_dataset = dict(
+ type=InternVL_V1_5_Dataset,
+ model_path=path,
+ data_paths=data_path,
+ image_folders=image_folder,
+ template=prompt_template,
+ max_length=max_length)
+
+train_dataloader = dict(
+ batch_size=batch_size,
+ num_workers=dataloader_num_workers,
+ dataset=llava_dataset,
+ sampler=dict(
+ type=LengthGroupedSampler,
+ length_property='modality_length',
+ per_device_batch_size=batch_size * accumulative_counts),
+ collate_fn=dict(type=default_collate_fn))
+
+#######################################################################
+# PART 4 Scheduler & Optimizer #
+#######################################################################
+# optimizer
+optim_wrapper = dict(
+ type=AmpOptimWrapper,
+ optimizer=dict(
+ type=optim_type, lr=lr, betas=betas, weight_decay=weight_decay),
+ clip_grad=dict(max_norm=max_norm, error_if_nonfinite=False),
+ accumulative_counts=accumulative_counts,
+ loss_scale='dynamic',
+ dtype='float16')
+
+# learning policy
+# More information: https://github.com/open-mmlab/mmengine/blob/main/docs/en/tutorials/param_scheduler.md # noqa: E501
+param_scheduler = [
+ dict(
+ type=LinearLR,
+ start_factor=1e-5,
+ by_epoch=True,
+ begin=0,
+ end=warmup_ratio * max_epochs,
+ convert_to_iter_based=True),
+ dict(
+ type=CosineAnnealingLR,
+ eta_min=0.0,
+ by_epoch=True,
+ begin=warmup_ratio * max_epochs,
+ end=max_epochs,
+ convert_to_iter_based=True)
+]
+
+# train, val, test setting
+train_cfg = dict(type=TrainLoop, max_epochs=max_epochs)
+
+#######################################################################
+# PART 5 Runtime #
+#######################################################################
+# Log the dialogue periodically during the training process, optional
+tokenizer = dict(
+ type=AutoTokenizer.from_pretrained,
+ pretrained_model_name_or_path=path,
+ trust_remote_code=True)
+
+custom_hooks = [
+ dict(type=DatasetInfoHook, tokenizer=tokenizer),
+]
+
+# configure default hooks
+default_hooks = dict(
+ # record the time of every iteration.
+ timer=dict(type=IterTimerHook),
+ # print log every 10 iterations.
+ logger=dict(type=LoggerHook, log_metric_by_epoch=False, interval=10),
+ # enable the parameter scheduler.
+ param_scheduler=dict(type=ParamSchedulerHook),
+ # save checkpoint per `save_steps`.
+ checkpoint=dict(
+ type=CheckpointHook,
+ save_optimizer=False,
+ by_epoch=False,
+ interval=save_steps,
+ max_keep_ckpts=save_total_limit),
+ # set sampler seed in distributed evrionment.
+ sampler_seed=dict(type=DistSamplerSeedHook),
+)
+
+# configure environment
+env_cfg = dict(
+ # whether to enable cudnn benchmark
+ cudnn_benchmark=False,
+ # set multi process parameters
+ mp_cfg=dict(mp_start_method='fork', opencv_num_threads=0),
+ # set distributed parameters
+ dist_cfg=dict(backend='nccl'),
+)
+
+# set visualizer
+visualizer = None
+
+# set log level
+log_level = 'INFO'
+
+# load from which checkpoint
+load_from = None
+
+# whether to resume training from the loaded checkpoint
+resume = False
+
+# Defaults to use random seed and disable `deterministic`
+randomness = dict(seed=None, deterministic=False)
+
+# set log processor
+log_processor = dict(by_epoch=False)
diff --git a/xtuner/configs/internvl/v2/internvl_v2_internlm2_5_8b_lora_finetune.py b/xtuner/configs/internvl/v2/internvl_v2_internlm2_5_8b_lora_finetune.py
new file mode 100644
index 000000000..d9fa7ab3a
--- /dev/null
+++ b/xtuner/configs/internvl/v2/internvl_v2_internlm2_5_8b_lora_finetune.py
@@ -0,0 +1,183 @@
+# Copyright (c) OpenMMLab. All rights reserved.
+from mmengine.hooks import (CheckpointHook, DistSamplerSeedHook, IterTimerHook,
+ LoggerHook, ParamSchedulerHook)
+from mmengine.optim import AmpOptimWrapper, CosineAnnealingLR, LinearLR
+from peft import LoraConfig
+from torch.optim import AdamW
+from transformers import AutoTokenizer
+
+from xtuner.dataset import InternVL_V1_5_Dataset
+from xtuner.dataset.collate_fns import default_collate_fn
+from xtuner.dataset.samplers import LengthGroupedSampler
+from xtuner.engine.hooks import DatasetInfoHook
+from xtuner.engine.runner import TrainLoop
+from xtuner.model import InternVL_V1_5
+from xtuner.utils import PROMPT_TEMPLATE
+
+#######################################################################
+# PART 1 Settings #
+#######################################################################
+# Model
+path = 'OpenGVLab/InternVL2-8B'
+
+# Data
+data_root = './data/llava_data/'
+data_path = data_root + 'LLaVA-Instruct-150K/llava_v1_5_mix665k.json'
+image_folder = data_root + 'llava_images'
+prompt_template = PROMPT_TEMPLATE.internlm2_chat
+max_length = 8192
+
+# Scheduler & Optimizer
+batch_size = 8 # per_device
+accumulative_counts = 2
+dataloader_num_workers = 4
+max_epochs = 1
+optim_type = AdamW
+# official 1024 -> 4e-5
+lr = 1e-6
+betas = (0.9, 0.999)
+weight_decay = 0.05
+max_norm = 1 # grad clip
+warmup_ratio = 0.03
+
+# Save
+save_steps = 1000
+save_total_limit = 1 # Maximum checkpoints to keep (-1 means unlimited)
+
+#######################################################################
+# PART 2 Model & Tokenizer & Image Processor #
+#######################################################################
+model = dict(
+ type=InternVL_V1_5,
+ model_path=path,
+ freeze_llm=True,
+ freeze_visual_encoder=True,
+ # comment the following lines if you don't want to use Lora in llm
+ llm_lora=dict(
+ type=LoraConfig,
+ r=128,
+ lora_alpha=256,
+ lora_dropout=0.05,
+ target_modules=None,
+ task_type='CAUSAL_LM'),
+ # uncomment the following lines if you don't want to use Lora in visual encoder # noqa
+ # visual_encoder_lora=dict(
+ # type=LoraConfig, r=64, lora_alpha=16, lora_dropout=0.05,
+ # target_modules=['attn.qkv', 'attn.proj', 'mlp.fc1', 'mlp.fc2'])
+)
+
+#######################################################################
+# PART 3 Dataset & Dataloader #
+#######################################################################
+llava_dataset = dict(
+ type=InternVL_V1_5_Dataset,
+ model_path=path,
+ data_paths=data_path,
+ image_folders=image_folder,
+ template=prompt_template,
+ max_length=max_length)
+
+train_dataloader = dict(
+ batch_size=batch_size,
+ num_workers=dataloader_num_workers,
+ dataset=llava_dataset,
+ sampler=dict(
+ type=LengthGroupedSampler,
+ length_property='modality_length',
+ per_device_batch_size=batch_size * accumulative_counts),
+ collate_fn=dict(type=default_collate_fn))
+
+#######################################################################
+# PART 4 Scheduler & Optimizer #
+#######################################################################
+# optimizer
+optim_wrapper = dict(
+ type=AmpOptimWrapper,
+ optimizer=dict(
+ type=optim_type, lr=lr, betas=betas, weight_decay=weight_decay),
+ clip_grad=dict(max_norm=max_norm, error_if_nonfinite=False),
+ accumulative_counts=accumulative_counts,
+ loss_scale='dynamic',
+ dtype='float16')
+
+# learning policy
+# More information: https://github.com/open-mmlab/mmengine/blob/main/docs/en/tutorials/param_scheduler.md # noqa: E501
+param_scheduler = [
+ dict(
+ type=LinearLR,
+ start_factor=1e-5,
+ by_epoch=True,
+ begin=0,
+ end=warmup_ratio * max_epochs,
+ convert_to_iter_based=True),
+ dict(
+ type=CosineAnnealingLR,
+ eta_min=0.0,
+ by_epoch=True,
+ begin=warmup_ratio * max_epochs,
+ end=max_epochs,
+ convert_to_iter_based=True)
+]
+
+# train, val, test setting
+train_cfg = dict(type=TrainLoop, max_epochs=max_epochs)
+
+#######################################################################
+# PART 5 Runtime #
+#######################################################################
+# Log the dialogue periodically during the training process, optional
+tokenizer = dict(
+ type=AutoTokenizer.from_pretrained,
+ pretrained_model_name_or_path=path,
+ trust_remote_code=True)
+
+custom_hooks = [
+ dict(type=DatasetInfoHook, tokenizer=tokenizer),
+]
+
+# configure default hooks
+default_hooks = dict(
+ # record the time of every iteration.
+ timer=dict(type=IterTimerHook),
+ # print log every 10 iterations.
+ logger=dict(type=LoggerHook, log_metric_by_epoch=False, interval=10),
+ # enable the parameter scheduler.
+ param_scheduler=dict(type=ParamSchedulerHook),
+ # save checkpoint per `save_steps`.
+ checkpoint=dict(
+ type=CheckpointHook,
+ save_optimizer=False,
+ by_epoch=False,
+ interval=save_steps,
+ max_keep_ckpts=save_total_limit),
+ # set sampler seed in distributed evrionment.
+ sampler_seed=dict(type=DistSamplerSeedHook),
+)
+
+# configure environment
+env_cfg = dict(
+ # whether to enable cudnn benchmark
+ cudnn_benchmark=False,
+ # set multi process parameters
+ mp_cfg=dict(mp_start_method='fork', opencv_num_threads=0),
+ # set distributed parameters
+ dist_cfg=dict(backend='nccl'),
+)
+
+# set visualizer
+visualizer = None
+
+# set log level
+log_level = 'INFO'
+
+# load from which checkpoint
+load_from = None
+
+# whether to resume training from the loaded checkpoint
+resume = False
+
+# Defaults to use random seed and disable `deterministic`
+randomness = dict(seed=None, deterministic=False)
+
+# set log processor
+log_processor = dict(by_epoch=False)
diff --git a/xtuner/configs/internvl/v2/internvl_v2_internlm2_5_8b_qlora_finetune.py b/xtuner/configs/internvl/v2/internvl_v2_internlm2_5_8b_qlora_finetune.py
new file mode 100644
index 000000000..b3d04bb43
--- /dev/null
+++ b/xtuner/configs/internvl/v2/internvl_v2_internlm2_5_8b_qlora_finetune.py
@@ -0,0 +1,185 @@
+# Copyright (c) OpenMMLab. All rights reserved.
+from mmengine.hooks import (CheckpointHook, DistSamplerSeedHook, IterTimerHook,
+ LoggerHook, ParamSchedulerHook)
+from mmengine.optim import AmpOptimWrapper, CosineAnnealingLR, LinearLR
+from peft import LoraConfig
+from torch.optim import AdamW
+from transformers import AutoTokenizer
+
+from xtuner.dataset import InternVL_V1_5_Dataset
+from xtuner.dataset.collate_fns import default_collate_fn
+from xtuner.dataset.samplers import LengthGroupedSampler
+from xtuner.engine.hooks import DatasetInfoHook
+from xtuner.engine.runner import TrainLoop
+from xtuner.model import InternVL_V1_5
+from xtuner.utils import PROMPT_TEMPLATE
+
+#######################################################################
+# PART 1 Settings #
+#######################################################################
+# Model
+path = 'OpenGVLab/InternVL2-8B'
+
+# Data
+data_root = './data/llava_data/'
+data_path = data_root + 'LLaVA-Instruct-150K/llava_v1_5_mix665k.json'
+image_folder = data_root + 'llava_images'
+prompt_template = PROMPT_TEMPLATE.internlm2_chat
+max_length = 8192
+
+# Scheduler & Optimizer
+batch_size = 8 # per_device
+accumulative_counts = 2
+dataloader_num_workers = 4
+max_epochs = 1
+optim_type = AdamW
+# official 1024 -> 4e-5
+lr = 1e-6
+betas = (0.9, 0.999)
+weight_decay = 0.05
+max_norm = 1 # grad clip
+warmup_ratio = 0.03
+
+# Save
+save_steps = 1000
+save_total_limit = 1 # Maximum checkpoints to keep (-1 means unlimited)
+
+#######################################################################
+# PART 2 Model & Tokenizer & Image Processor #
+#######################################################################
+model = dict(
+ type=InternVL_V1_5,
+ model_path=path,
+ freeze_llm=True,
+ freeze_visual_encoder=True,
+ quantization_llm=True, # or False
+ quantization_vit=False, # or True and uncomment visual_encoder_lora
+ # comment the following lines if you don't want to use Lora in llm
+ llm_lora=dict(
+ type=LoraConfig,
+ r=128,
+ lora_alpha=256,
+ lora_dropout=0.05,
+ target_modules=None,
+ task_type='CAUSAL_LM'),
+ # uncomment the following lines if you don't want to use Lora in visual encoder # noqa
+ # visual_encoder_lora=dict(
+ # type=LoraConfig, r=64, lora_alpha=16, lora_dropout=0.05,
+ # target_modules=['attn.qkv', 'attn.proj', 'mlp.fc1', 'mlp.fc2'])
+)
+
+#######################################################################
+# PART 3 Dataset & Dataloader #
+#######################################################################
+llava_dataset = dict(
+ type=InternVL_V1_5_Dataset,
+ model_path=path,
+ data_paths=data_path,
+ image_folders=image_folder,
+ template=prompt_template,
+ max_length=max_length)
+
+train_dataloader = dict(
+ batch_size=batch_size,
+ num_workers=dataloader_num_workers,
+ dataset=llava_dataset,
+ sampler=dict(
+ type=LengthGroupedSampler,
+ length_property='modality_length',
+ per_device_batch_size=batch_size * accumulative_counts),
+ collate_fn=dict(type=default_collate_fn))
+
+#######################################################################
+# PART 4 Scheduler & Optimizer #
+#######################################################################
+# optimizer
+optim_wrapper = dict(
+ type=AmpOptimWrapper,
+ optimizer=dict(
+ type=optim_type, lr=lr, betas=betas, weight_decay=weight_decay),
+ clip_grad=dict(max_norm=max_norm, error_if_nonfinite=False),
+ accumulative_counts=accumulative_counts,
+ loss_scale='dynamic',
+ dtype='float16')
+
+# learning policy
+# More information: https://github.com/open-mmlab/mmengine/blob/main/docs/en/tutorials/param_scheduler.md # noqa: E501
+param_scheduler = [
+ dict(
+ type=LinearLR,
+ start_factor=1e-5,
+ by_epoch=True,
+ begin=0,
+ end=warmup_ratio * max_epochs,
+ convert_to_iter_based=True),
+ dict(
+ type=CosineAnnealingLR,
+ eta_min=0.0,
+ by_epoch=True,
+ begin=warmup_ratio * max_epochs,
+ end=max_epochs,
+ convert_to_iter_based=True)
+]
+
+# train, val, test setting
+train_cfg = dict(type=TrainLoop, max_epochs=max_epochs)
+
+#######################################################################
+# PART 5 Runtime #
+#######################################################################
+# Log the dialogue periodically during the training process, optional
+tokenizer = dict(
+ type=AutoTokenizer.from_pretrained,
+ pretrained_model_name_or_path=path,
+ trust_remote_code=True)
+
+custom_hooks = [
+ dict(type=DatasetInfoHook, tokenizer=tokenizer),
+]
+
+# configure default hooks
+default_hooks = dict(
+ # record the time of every iteration.
+ timer=dict(type=IterTimerHook),
+ # print log every 10 iterations.
+ logger=dict(type=LoggerHook, log_metric_by_epoch=False, interval=10),
+ # enable the parameter scheduler.
+ param_scheduler=dict(type=ParamSchedulerHook),
+ # save checkpoint per `save_steps`.
+ checkpoint=dict(
+ type=CheckpointHook,
+ save_optimizer=False,
+ by_epoch=False,
+ interval=save_steps,
+ max_keep_ckpts=save_total_limit),
+ # set sampler seed in distributed evrionment.
+ sampler_seed=dict(type=DistSamplerSeedHook),
+)
+
+# configure environment
+env_cfg = dict(
+ # whether to enable cudnn benchmark
+ cudnn_benchmark=False,
+ # set multi process parameters
+ mp_cfg=dict(mp_start_method='fork', opencv_num_threads=0),
+ # set distributed parameters
+ dist_cfg=dict(backend='nccl'),
+)
+
+# set visualizer
+visualizer = None
+
+# set log level
+log_level = 'INFO'
+
+# load from which checkpoint
+load_from = None
+
+# whether to resume training from the loaded checkpoint
+resume = False
+
+# Defaults to use random seed and disable `deterministic`
+randomness = dict(seed=None, deterministic=False)
+
+# set log processor
+log_processor = dict(by_epoch=False)
diff --git a/xtuner/configs/internvl/v2/internvl_v2_phi3_4b_finetune.py b/xtuner/configs/internvl/v2/internvl_v2_phi3_4b_finetune.py
new file mode 100644
index 000000000..41a712569
--- /dev/null
+++ b/xtuner/configs/internvl/v2/internvl_v2_phi3_4b_finetune.py
@@ -0,0 +1,170 @@
+# Copyright (c) OpenMMLab. All rights reserved.
+from mmengine.hooks import (CheckpointHook, DistSamplerSeedHook, IterTimerHook,
+ LoggerHook, ParamSchedulerHook)
+from mmengine.optim import AmpOptimWrapper, CosineAnnealingLR, LinearLR
+from torch.optim import AdamW
+from transformers import AutoTokenizer
+
+from xtuner.dataset import InternVL_V1_5_Dataset
+from xtuner.dataset.collate_fns import default_collate_fn
+from xtuner.dataset.samplers import LengthGroupedSampler
+from xtuner.engine.hooks import DatasetInfoHook
+from xtuner.engine.runner import TrainLoop
+from xtuner.model import InternVL_V1_5
+from xtuner.utils import PROMPT_TEMPLATE
+
+#######################################################################
+# PART 1 Settings #
+#######################################################################
+# Model
+path = 'OpenGVLab/InternVL2-4B'
+
+# Data
+data_root = './data/llava_data/'
+data_path = data_root + 'LLaVA-Instruct-150K/llava_v1_5_mix665k.json'
+image_folder = data_root + 'llava_images'
+prompt_template = PROMPT_TEMPLATE.phi3_chat
+max_length = 8192
+
+# Scheduler & Optimizer
+batch_size = 4 # per_device
+accumulative_counts = 4
+dataloader_num_workers = 4
+max_epochs = 1
+optim_type = AdamW
+# official 1024 -> 4e-5
+lr = 1e-6
+betas = (0.9, 0.999)
+weight_decay = 0.05
+max_norm = 1 # grad clip
+warmup_ratio = 0.03
+
+# Save
+save_steps = 1000
+save_total_limit = 1 # Maximum checkpoints to keep (-1 means unlimited)
+
+#######################################################################
+# PART 2 Model & Tokenizer & Image Processor #
+#######################################################################
+model = dict(
+ type=InternVL_V1_5,
+ model_path=path,
+ freeze_llm=False,
+ freeze_visual_encoder=True # or False
+)
+
+#######################################################################
+# PART 3 Dataset & Dataloader #
+#######################################################################
+llava_dataset = dict(
+ type=InternVL_V1_5_Dataset,
+ model_path=path,
+ data_paths=data_path,
+ image_folders=image_folder,
+ template=prompt_template,
+ max_length=max_length)
+
+train_dataloader = dict(
+ batch_size=batch_size,
+ num_workers=dataloader_num_workers,
+ dataset=llava_dataset,
+ sampler=dict(
+ type=LengthGroupedSampler,
+ length_property='modality_length',
+ per_device_batch_size=batch_size * accumulative_counts),
+ collate_fn=dict(type=default_collate_fn))
+
+#######################################################################
+# PART 4 Scheduler & Optimizer #
+#######################################################################
+# optimizer
+optim_wrapper = dict(
+ type=AmpOptimWrapper,
+ optimizer=dict(
+ type=optim_type, lr=lr, betas=betas, weight_decay=weight_decay),
+ clip_grad=dict(max_norm=max_norm, error_if_nonfinite=False),
+ accumulative_counts=accumulative_counts,
+ loss_scale='dynamic',
+ dtype='float16')
+
+# learning policy
+# More information: https://github.com/open-mmlab/mmengine/blob/main/docs/en/tutorials/param_scheduler.md # noqa: E501
+param_scheduler = [
+ dict(
+ type=LinearLR,
+ start_factor=1e-5,
+ by_epoch=True,
+ begin=0,
+ end=warmup_ratio * max_epochs,
+ convert_to_iter_based=True),
+ dict(
+ type=CosineAnnealingLR,
+ eta_min=0.0,
+ by_epoch=True,
+ begin=warmup_ratio * max_epochs,
+ end=max_epochs,
+ convert_to_iter_based=True)
+]
+
+# train, val, test setting
+train_cfg = dict(type=TrainLoop, max_epochs=max_epochs)
+
+#######################################################################
+# PART 5 Runtime #
+#######################################################################
+# Log the dialogue periodically during the training process, optional
+tokenizer = dict(
+ type=AutoTokenizer.from_pretrained,
+ pretrained_model_name_or_path=path,
+ trust_remote_code=True)
+
+custom_hooks = [
+ dict(type=DatasetInfoHook, tokenizer=tokenizer),
+]
+
+# configure default hooks
+default_hooks = dict(
+ # record the time of every iteration.
+ timer=dict(type=IterTimerHook),
+ # print log every 10 iterations.
+ logger=dict(type=LoggerHook, log_metric_by_epoch=False, interval=10),
+ # enable the parameter scheduler.
+ param_scheduler=dict(type=ParamSchedulerHook),
+ # save checkpoint per `save_steps`.
+ checkpoint=dict(
+ type=CheckpointHook,
+ save_optimizer=False,
+ by_epoch=False,
+ interval=save_steps,
+ max_keep_ckpts=save_total_limit),
+ # set sampler seed in distributed evrionment.
+ sampler_seed=dict(type=DistSamplerSeedHook),
+)
+
+# configure environment
+env_cfg = dict(
+ # whether to enable cudnn benchmark
+ cudnn_benchmark=False,
+ # set multi process parameters
+ mp_cfg=dict(mp_start_method='fork', opencv_num_threads=0),
+ # set distributed parameters
+ dist_cfg=dict(backend='nccl'),
+)
+
+# set visualizer
+visualizer = None
+
+# set log level
+log_level = 'INFO'
+
+# load from which checkpoint
+load_from = None
+
+# whether to resume training from the loaded checkpoint
+resume = False
+
+# Defaults to use random seed and disable `deterministic`
+randomness = dict(seed=None, deterministic=False)
+
+# set log processor
+log_processor = dict(by_epoch=False)
diff --git a/xtuner/configs/internvl/v2/internvl_v2_phi3_4b_lora_finetune.py b/xtuner/configs/internvl/v2/internvl_v2_phi3_4b_lora_finetune.py
new file mode 100644
index 000000000..64a20450f
--- /dev/null
+++ b/xtuner/configs/internvl/v2/internvl_v2_phi3_4b_lora_finetune.py
@@ -0,0 +1,183 @@
+# Copyright (c) OpenMMLab. All rights reserved.
+from mmengine.hooks import (CheckpointHook, DistSamplerSeedHook, IterTimerHook,
+ LoggerHook, ParamSchedulerHook)
+from mmengine.optim import AmpOptimWrapper, CosineAnnealingLR, LinearLR
+from peft import LoraConfig
+from torch.optim import AdamW
+from transformers import AutoTokenizer
+
+from xtuner.dataset import InternVL_V1_5_Dataset
+from xtuner.dataset.collate_fns import default_collate_fn
+from xtuner.dataset.samplers import LengthGroupedSampler
+from xtuner.engine.hooks import DatasetInfoHook
+from xtuner.engine.runner import TrainLoop
+from xtuner.model import InternVL_V1_5
+from xtuner.utils import PROMPT_TEMPLATE
+
+#######################################################################
+# PART 1 Settings #
+#######################################################################
+# Model
+path = 'OpenGVLab/InternVL2-4B'
+
+# Data
+data_root = './data/llava_data/'
+data_path = data_root + 'LLaVA-Instruct-150K/llava_v1_5_mix665k.json'
+image_folder = data_root + 'llava_images'
+prompt_template = PROMPT_TEMPLATE.phi3_chat
+max_length = 8192
+
+# Scheduler & Optimizer
+batch_size = 8 # per_device
+accumulative_counts = 2
+dataloader_num_workers = 4
+max_epochs = 1
+optim_type = AdamW
+# official 1024 -> 4e-5
+lr = 1e-6
+betas = (0.9, 0.999)
+weight_decay = 0.05
+max_norm = 1 # grad clip
+warmup_ratio = 0.03
+
+# Save
+save_steps = 1000
+save_total_limit = 1 # Maximum checkpoints to keep (-1 means unlimited)
+
+#######################################################################
+# PART 2 Model & Tokenizer & Image Processor #
+#######################################################################
+model = dict(
+ type=InternVL_V1_5,
+ model_path=path,
+ freeze_llm=True,
+ freeze_visual_encoder=True,
+ # comment the following lines if you don't want to use Lora in llm
+ llm_lora=dict(
+ type=LoraConfig,
+ r=128,
+ lora_alpha=256,
+ lora_dropout=0.05,
+ target_modules=None,
+ task_type='CAUSAL_LM'),
+ # uncomment the following lines if you don't want to use Lora in visual encoder # noqa
+ # visual_encoder_lora=dict(
+ # type=LoraConfig, r=64, lora_alpha=16, lora_dropout=0.05,
+ # target_modules=['attn.qkv', 'attn.proj', 'mlp.fc1', 'mlp.fc2'])
+)
+
+#######################################################################
+# PART 3 Dataset & Dataloader #
+#######################################################################
+llava_dataset = dict(
+ type=InternVL_V1_5_Dataset,
+ model_path=path,
+ data_paths=data_path,
+ image_folders=image_folder,
+ template=prompt_template,
+ max_length=max_length)
+
+train_dataloader = dict(
+ batch_size=batch_size,
+ num_workers=dataloader_num_workers,
+ dataset=llava_dataset,
+ sampler=dict(
+ type=LengthGroupedSampler,
+ length_property='modality_length',
+ per_device_batch_size=batch_size * accumulative_counts),
+ collate_fn=dict(type=default_collate_fn))
+
+#######################################################################
+# PART 4 Scheduler & Optimizer #
+#######################################################################
+# optimizer
+optim_wrapper = dict(
+ type=AmpOptimWrapper,
+ optimizer=dict(
+ type=optim_type, lr=lr, betas=betas, weight_decay=weight_decay),
+ clip_grad=dict(max_norm=max_norm, error_if_nonfinite=False),
+ accumulative_counts=accumulative_counts,
+ loss_scale='dynamic',
+ dtype='float16')
+
+# learning policy
+# More information: https://github.com/open-mmlab/mmengine/blob/main/docs/en/tutorials/param_scheduler.md # noqa: E501
+param_scheduler = [
+ dict(
+ type=LinearLR,
+ start_factor=1e-5,
+ by_epoch=True,
+ begin=0,
+ end=warmup_ratio * max_epochs,
+ convert_to_iter_based=True),
+ dict(
+ type=CosineAnnealingLR,
+ eta_min=0.0,
+ by_epoch=True,
+ begin=warmup_ratio * max_epochs,
+ end=max_epochs,
+ convert_to_iter_based=True)
+]
+
+# train, val, test setting
+train_cfg = dict(type=TrainLoop, max_epochs=max_epochs)
+
+#######################################################################
+# PART 5 Runtime #
+#######################################################################
+# Log the dialogue periodically during the training process, optional
+tokenizer = dict(
+ type=AutoTokenizer.from_pretrained,
+ pretrained_model_name_or_path=path,
+ trust_remote_code=True)
+
+custom_hooks = [
+ dict(type=DatasetInfoHook, tokenizer=tokenizer),
+]
+
+# configure default hooks
+default_hooks = dict(
+ # record the time of every iteration.
+ timer=dict(type=IterTimerHook),
+ # print log every 10 iterations.
+ logger=dict(type=LoggerHook, log_metric_by_epoch=False, interval=10),
+ # enable the parameter scheduler.
+ param_scheduler=dict(type=ParamSchedulerHook),
+ # save checkpoint per `save_steps`.
+ checkpoint=dict(
+ type=CheckpointHook,
+ save_optimizer=False,
+ by_epoch=False,
+ interval=save_steps,
+ max_keep_ckpts=save_total_limit),
+ # set sampler seed in distributed evrionment.
+ sampler_seed=dict(type=DistSamplerSeedHook),
+)
+
+# configure environment
+env_cfg = dict(
+ # whether to enable cudnn benchmark
+ cudnn_benchmark=False,
+ # set multi process parameters
+ mp_cfg=dict(mp_start_method='fork', opencv_num_threads=0),
+ # set distributed parameters
+ dist_cfg=dict(backend='nccl'),
+)
+
+# set visualizer
+visualizer = None
+
+# set log level
+log_level = 'INFO'
+
+# load from which checkpoint
+load_from = None
+
+# whether to resume training from the loaded checkpoint
+resume = False
+
+# Defaults to use random seed and disable `deterministic`
+randomness = dict(seed=None, deterministic=False)
+
+# set log processor
+log_processor = dict(by_epoch=False)
diff --git a/xtuner/configs/internvl/v2/internvl_v2_phi3_4b_qlora_finetune.py b/xtuner/configs/internvl/v2/internvl_v2_phi3_4b_qlora_finetune.py
new file mode 100644
index 000000000..8302fa5cc
--- /dev/null
+++ b/xtuner/configs/internvl/v2/internvl_v2_phi3_4b_qlora_finetune.py
@@ -0,0 +1,185 @@
+# Copyright (c) OpenMMLab. All rights reserved.
+from mmengine.hooks import (CheckpointHook, DistSamplerSeedHook, IterTimerHook,
+ LoggerHook, ParamSchedulerHook)
+from mmengine.optim import AmpOptimWrapper, CosineAnnealingLR, LinearLR
+from peft import LoraConfig
+from torch.optim import AdamW
+from transformers import AutoTokenizer
+
+from xtuner.dataset import InternVL_V1_5_Dataset
+from xtuner.dataset.collate_fns import default_collate_fn
+from xtuner.dataset.samplers import LengthGroupedSampler
+from xtuner.engine.hooks import DatasetInfoHook
+from xtuner.engine.runner import TrainLoop
+from xtuner.model import InternVL_V1_5
+from xtuner.utils import PROMPT_TEMPLATE
+
+#######################################################################
+# PART 1 Settings #
+#######################################################################
+# Model
+path = 'OpenGVLab/InternVL2-4B'
+
+# Data
+data_root = './data/llava_data/'
+data_path = data_root + 'LLaVA-Instruct-150K/llava_v1_5_mix665k.json'
+image_folder = data_root + 'llava_images'
+prompt_template = PROMPT_TEMPLATE.phi3_chat
+max_length = 8192
+
+# Scheduler & Optimizer
+batch_size = 8 # per_device
+accumulative_counts = 2
+dataloader_num_workers = 4
+max_epochs = 1
+optim_type = AdamW
+# official 1024 -> 4e-5
+lr = 1e-6
+betas = (0.9, 0.999)
+weight_decay = 0.05
+max_norm = 1 # grad clip
+warmup_ratio = 0.03
+
+# Save
+save_steps = 1000
+save_total_limit = 1 # Maximum checkpoints to keep (-1 means unlimited)
+
+#######################################################################
+# PART 2 Model & Tokenizer & Image Processor #
+#######################################################################
+model = dict(
+ type=InternVL_V1_5,
+ model_path=path,
+ freeze_llm=True,
+ freeze_visual_encoder=True,
+ quantization_llm=True, # or False
+ quantization_vit=False, # or True and uncomment visual_encoder_lora
+ # comment the following lines if you don't want to use Lora in llm
+ llm_lora=dict(
+ type=LoraConfig,
+ r=128,
+ lora_alpha=256,
+ lora_dropout=0.05,
+ target_modules=None,
+ task_type='CAUSAL_LM'),
+ # uncomment the following lines if you don't want to use Lora in visual encoder # noqa
+ # visual_encoder_lora=dict(
+ # type=LoraConfig, r=64, lora_alpha=16, lora_dropout=0.05,
+ # target_modules=['attn.qkv', 'attn.proj', 'mlp.fc1', 'mlp.fc2'])
+)
+
+#######################################################################
+# PART 3 Dataset & Dataloader #
+#######################################################################
+llava_dataset = dict(
+ type=InternVL_V1_5_Dataset,
+ model_path=path,
+ data_paths=data_path,
+ image_folders=image_folder,
+ template=prompt_template,
+ max_length=max_length)
+
+train_dataloader = dict(
+ batch_size=batch_size,
+ num_workers=dataloader_num_workers,
+ dataset=llava_dataset,
+ sampler=dict(
+ type=LengthGroupedSampler,
+ length_property='modality_length',
+ per_device_batch_size=batch_size * accumulative_counts),
+ collate_fn=dict(type=default_collate_fn))
+
+#######################################################################
+# PART 4 Scheduler & Optimizer #
+#######################################################################
+# optimizer
+optim_wrapper = dict(
+ type=AmpOptimWrapper,
+ optimizer=dict(
+ type=optim_type, lr=lr, betas=betas, weight_decay=weight_decay),
+ clip_grad=dict(max_norm=max_norm, error_if_nonfinite=False),
+ accumulative_counts=accumulative_counts,
+ loss_scale='dynamic',
+ dtype='float16')
+
+# learning policy
+# More information: https://github.com/open-mmlab/mmengine/blob/main/docs/en/tutorials/param_scheduler.md # noqa: E501
+param_scheduler = [
+ dict(
+ type=LinearLR,
+ start_factor=1e-5,
+ by_epoch=True,
+ begin=0,
+ end=warmup_ratio * max_epochs,
+ convert_to_iter_based=True),
+ dict(
+ type=CosineAnnealingLR,
+ eta_min=0.0,
+ by_epoch=True,
+ begin=warmup_ratio * max_epochs,
+ end=max_epochs,
+ convert_to_iter_based=True)
+]
+
+# train, val, test setting
+train_cfg = dict(type=TrainLoop, max_epochs=max_epochs)
+
+#######################################################################
+# PART 5 Runtime #
+#######################################################################
+# Log the dialogue periodically during the training process, optional
+tokenizer = dict(
+ type=AutoTokenizer.from_pretrained,
+ pretrained_model_name_or_path=path,
+ trust_remote_code=True)
+
+custom_hooks = [
+ dict(type=DatasetInfoHook, tokenizer=tokenizer),
+]
+
+# configure default hooks
+default_hooks = dict(
+ # record the time of every iteration.
+ timer=dict(type=IterTimerHook),
+ # print log every 10 iterations.
+ logger=dict(type=LoggerHook, log_metric_by_epoch=False, interval=10),
+ # enable the parameter scheduler.
+ param_scheduler=dict(type=ParamSchedulerHook),
+ # save checkpoint per `save_steps`.
+ checkpoint=dict(
+ type=CheckpointHook,
+ save_optimizer=False,
+ by_epoch=False,
+ interval=save_steps,
+ max_keep_ckpts=save_total_limit),
+ # set sampler seed in distributed evrionment.
+ sampler_seed=dict(type=DistSamplerSeedHook),
+)
+
+# configure environment
+env_cfg = dict(
+ # whether to enable cudnn benchmark
+ cudnn_benchmark=False,
+ # set multi process parameters
+ mp_cfg=dict(mp_start_method='fork', opencv_num_threads=0),
+ # set distributed parameters
+ dist_cfg=dict(backend='nccl'),
+)
+
+# set visualizer
+visualizer = None
+
+# set log level
+log_level = 'INFO'
+
+# load from which checkpoint
+load_from = None
+
+# whether to resume training from the loaded checkpoint
+resume = False
+
+# Defaults to use random seed and disable `deterministic`
+randomness = dict(seed=None, deterministic=False)
+
+# set log processor
+log_processor = dict(by_epoch=False)
diff --git a/xtuner/configs/llama/llama3_70b_instruct/llama3_70b_instruct_qlora_alpaca_e3_2k_gpu8.py b/xtuner/configs/llama/llama3_70b_instruct/llama3_70b_instruct_qlora_alpaca_e3_2k_gpu8.py
new file mode 100644
index 000000000..89feac44e
--- /dev/null
+++ b/xtuner/configs/llama/llama3_70b_instruct/llama3_70b_instruct_qlora_alpaca_e3_2k_gpu8.py
@@ -0,0 +1,220 @@
+# Copyright (c) OpenMMLab. All rights reserved.
+import torch
+from datasets import load_dataset
+from mmengine.dataset import DefaultSampler
+from mmengine.hooks import (CheckpointHook, DistSamplerSeedHook, IterTimerHook,
+ LoggerHook, ParamSchedulerHook)
+from mmengine.optim import AmpOptimWrapper, CosineAnnealingLR, LinearLR
+from peft import LoraConfig
+from torch.optim import AdamW
+from transformers import (AutoModelForCausalLM, AutoTokenizer,
+ BitsAndBytesConfig)
+
+from xtuner.dataset import process_hf_dataset
+from xtuner.dataset.collate_fns import default_collate_fn
+from xtuner.dataset.map_fns import alpaca_map_fn, template_map_fn_factory
+from xtuner.engine.hooks import (DatasetInfoHook, EvaluateChatHook,
+ VarlenAttnArgsToMessageHubHook)
+from xtuner.engine.runner import TrainLoop
+from xtuner.model import SupervisedFinetune
+from xtuner.parallel.sequence import SequenceParallelSampler
+from xtuner.utils import PROMPT_TEMPLATE, SYSTEM_TEMPLATE
+
+#######################################################################
+# PART 1 Settings #
+#######################################################################
+# Model
+pretrained_model_name_or_path = 'meta-llama/Meta-Llama-3-70B-Instruct'
+use_varlen_attn = False
+
+# Data
+alpaca_en_path = 'tatsu-lab/alpaca'
+prompt_template = PROMPT_TEMPLATE.llama3_chat
+max_length = 2048
+pack_to_max_length = True
+
+# parallel
+sequence_parallel_size = 1
+
+# Scheduler & Optimizer
+batch_size = 1 # per_device
+accumulative_counts = 2 # total bs = 1 bs_per_device * 8 gpus * 2 acc = 16
+accumulative_counts *= sequence_parallel_size
+dataloader_num_workers = 4
+max_epochs = 3
+optim_type = AdamW
+lr = 1e-4 # 70B model use smaller lr
+betas = (0.9, 0.999)
+weight_decay = 0
+max_norm = 1 # grad clip
+warmup_ratio = 0.03
+
+# Save
+save_steps = 500
+save_total_limit = 2 # Maximum checkpoints to keep (-1 means unlimited)
+
+# Evaluate the generation performance during the training
+evaluation_freq = 50
+SYSTEM = SYSTEM_TEMPLATE.alpaca
+evaluation_inputs = [
+ '请给我介绍五个上海的景点', 'Please tell me five scenic spots in Shanghai'
+]
+
+#######################################################################
+# PART 2 Model & Tokenizer #
+#######################################################################
+tokenizer = dict(
+ type=AutoTokenizer.from_pretrained,
+ pretrained_model_name_or_path=pretrained_model_name_or_path,
+ trust_remote_code=True,
+ padding_side='right')
+
+model = dict(
+ type=SupervisedFinetune,
+ use_varlen_attn=use_varlen_attn,
+ llm=dict(
+ type=AutoModelForCausalLM.from_pretrained,
+ pretrained_model_name_or_path=pretrained_model_name_or_path,
+ trust_remote_code=True,
+ torch_dtype=torch.float16,
+ quantization_config=dict(
+ type=BitsAndBytesConfig,
+ load_in_4bit=True,
+ load_in_8bit=False,
+ llm_int8_threshold=6.0,
+ llm_int8_has_fp16_weight=False,
+ bnb_4bit_compute_dtype=torch.float16,
+ bnb_4bit_use_double_quant=True,
+ bnb_4bit_quant_type='nf4',
+ bnb_4bit_quant_storage=torch.float16)),
+ lora=dict(
+ type=LoraConfig,
+ r=64,
+ lora_alpha=16,
+ lora_dropout=0.1,
+ bias='none',
+ task_type='CAUSAL_LM'))
+
+#######################################################################
+# PART 3 Dataset & Dataloader #
+#######################################################################
+alpaca_en = dict(
+ type=process_hf_dataset,
+ dataset=dict(type=load_dataset, path=alpaca_en_path),
+ tokenizer=tokenizer,
+ max_length=max_length,
+ dataset_map_fn=alpaca_map_fn,
+ template_map_fn=dict(
+ type=template_map_fn_factory, template=prompt_template),
+ remove_unused_columns=True,
+ shuffle_before_pack=True,
+ pack_to_max_length=pack_to_max_length,
+ use_varlen_attn=use_varlen_attn)
+
+sampler = SequenceParallelSampler \
+ if sequence_parallel_size > 1 else DefaultSampler
+train_dataloader = dict(
+ batch_size=batch_size,
+ num_workers=dataloader_num_workers,
+ dataset=alpaca_en,
+ sampler=dict(type=sampler, shuffle=True),
+ collate_fn=dict(type=default_collate_fn, use_varlen_attn=use_varlen_attn))
+
+#######################################################################
+# PART 4 Scheduler & Optimizer #
+#######################################################################
+# optimizer
+optim_wrapper = dict(
+ type=AmpOptimWrapper,
+ optimizer=dict(
+ type=optim_type, lr=lr, betas=betas, weight_decay=weight_decay),
+ clip_grad=dict(max_norm=max_norm, error_if_nonfinite=False),
+ accumulative_counts=accumulative_counts,
+ loss_scale='dynamic',
+ dtype='float16')
+
+# learning policy
+# More information: https://github.com/open-mmlab/mmengine/blob/main/docs/en/tutorials/param_scheduler.md # noqa: E501
+param_scheduler = [
+ dict(
+ type=LinearLR,
+ start_factor=1e-5,
+ by_epoch=True,
+ begin=0,
+ end=warmup_ratio * max_epochs,
+ convert_to_iter_based=True),
+ dict(
+ type=CosineAnnealingLR,
+ eta_min=0.0,
+ by_epoch=True,
+ begin=warmup_ratio * max_epochs,
+ end=max_epochs,
+ convert_to_iter_based=True)
+]
+
+# train, val, test setting
+train_cfg = dict(type=TrainLoop, max_epochs=max_epochs)
+
+#######################################################################
+# PART 5 Runtime #
+#######################################################################
+# Log the dialogue periodically during the training process, optional
+custom_hooks = [
+ dict(type=DatasetInfoHook, tokenizer=tokenizer),
+ dict(
+ type=EvaluateChatHook,
+ tokenizer=tokenizer,
+ every_n_iters=evaluation_freq,
+ evaluation_inputs=evaluation_inputs,
+ system=SYSTEM,
+ prompt_template=prompt_template)
+]
+
+if use_varlen_attn:
+ custom_hooks += [dict(type=VarlenAttnArgsToMessageHubHook)]
+
+# configure default hooks
+default_hooks = dict(
+ # record the time of every iteration.
+ timer=dict(type=IterTimerHook),
+ # print log every 10 iterations.
+ logger=dict(type=LoggerHook, log_metric_by_epoch=False, interval=10),
+ # enable the parameter scheduler.
+ param_scheduler=dict(type=ParamSchedulerHook),
+ # save checkpoint per `save_steps`.
+ checkpoint=dict(
+ type=CheckpointHook,
+ by_epoch=False,
+ interval=save_steps,
+ max_keep_ckpts=save_total_limit),
+ # set sampler seed in distributed evrionment.
+ sampler_seed=dict(type=DistSamplerSeedHook),
+)
+
+# configure environment
+env_cfg = dict(
+ # whether to enable cudnn benchmark
+ cudnn_benchmark=False,
+ # set multi process parameters
+ mp_cfg=dict(mp_start_method='fork', opencv_num_threads=0),
+ # set distributed parameters
+ dist_cfg=dict(backend='nccl'),
+)
+
+# set visualizer
+visualizer = None
+
+# set log level
+log_level = 'INFO'
+
+# load from which checkpoint
+load_from = None
+
+# whether to resume training from the loaded checkpoint
+resume = False
+
+# Defaults to use random seed and disable `deterministic`
+randomness = dict(seed=None, deterministic=False)
+
+# set log processor
+log_processor = dict(by_epoch=False)
diff --git a/xtuner/configs/llama/llama3_8b/README.md b/xtuner/configs/llama/llama3_8b/README.md
new file mode 100644
index 000000000..f77193dab
--- /dev/null
+++ b/xtuner/configs/llama/llama3_8b/README.md
@@ -0,0 +1,51 @@
+# Llama3 8B
+
+## Install
+
+```bash
+# Install the latest xtuner
+pip install -U 'xtuner[deepspeed]'
+
+# install the latest transformers
+pip install -U transformers
+```
+
+## QLoRA Fine-tune
+
+QLoRA only need a single A100-80G
+
+```bash
+xtuner train llama3_8b_instruct_qlora_alpaca_e3
+```
+
+## Full Parameter Fine-tune
+
+Full parameter fine-tune Llama3 8B in 8k context only requires 2 * A100-80G
+
+### torchrun
+
+```bash
+NPROC_PER_NODE=${GPU_NUM} xtuner train llama3_8b_instruct_full_alpaca_e3 --deepspeed deepspeed_zero2
+```
+
+### slurm
+
+```bash
+srun ${SRUN_ARGS} xtuner train llama3_8b_instruct_full_alpaca_e3 --launcher slurm --deepspeed deepspeed_zero3
+```
+
+### Speed
+
+| Model | Sequence Length | GPU Number | ZeRO | Sequence Parallel | Tokens per Second | TFLOPs |
+| :-------: | :-------------: | :--------: | :----: | :---------------: | :---------------: | :----: |
+| Llama3 8B | 8k | 2 | ZeRO-3 | 2 | 1037.0 | 76.8 |
+| Llama3 8B | 8k | 4 | ZeRO-3 | 1 | 2331.3 | 172.6 |
+| Llama3 8B | 8k | 8 | ZeRO-3 | 1 | 2771.2 | 205.1 |
+
+| Model | Sequence Length | GPU Number | ZeRO | Sequence Parallel | Tokens per Second | TFLOPs |
+| :-------: | :-------------: | :--------: | :----: | :---------------: | :---------------: | :----: |
+| Llama3 8B | 8k | 8 | ZeRO-3 | 1 | 2771.2 | 205.1 |
+| Llama3 8B | 16k | 8 | ZeRO-3 | 2 | 2320.7 | 191.7 |
+| Llama3 8B | 32k | 8 | ZeRO-3 | 4 | 1870.2 | 186.6 |
+| Llama3 8B | 64k | 8 | ZeRO-3 | 8 | 1356.4 | 182.0 |
+| Llama3 8B | 128k | 8 | ZeRO-3 | 8 | 875.7 | 177.7 |
diff --git a/xtuner/configs/llama/llama3_8b/llama3_8b_full_alpaca_e3.py b/xtuner/configs/llama/llama3_8b/llama3_8b_full_alpaca_e3.py
new file mode 100644
index 000000000..04f2e4dab
--- /dev/null
+++ b/xtuner/configs/llama/llama3_8b/llama3_8b_full_alpaca_e3.py
@@ -0,0 +1,199 @@
+# Copyright (c) OpenMMLab. All rights reserved.
+from datasets import load_dataset
+from mmengine.dataset import DefaultSampler
+from mmengine.hooks import (CheckpointHook, DistSamplerSeedHook, IterTimerHook,
+ LoggerHook, ParamSchedulerHook)
+from mmengine.optim import AmpOptimWrapper, CosineAnnealingLR, LinearLR
+from torch.optim import AdamW
+from transformers import AutoModelForCausalLM, AutoTokenizer
+
+from xtuner.dataset import process_hf_dataset
+from xtuner.dataset.collate_fns import default_collate_fn
+from xtuner.dataset.map_fns import alpaca_map_fn, template_map_fn_factory
+from xtuner.engine.hooks import (DatasetInfoHook, EvaluateChatHook,
+ VarlenAttnArgsToMessageHubHook)
+from xtuner.engine.runner import TrainLoop
+from xtuner.model import SupervisedFinetune
+from xtuner.parallel.sequence import SequenceParallelSampler
+from xtuner.utils import PROMPT_TEMPLATE, SYSTEM_TEMPLATE
+
+#######################################################################
+# PART 1 Settings #
+#######################################################################
+# Model
+pretrained_model_name_or_path = 'meta-llama/Meta-Llama-3-8B'
+use_varlen_attn = False
+
+# Data
+alpaca_en_path = 'tatsu-lab/alpaca'
+prompt_template = PROMPT_TEMPLATE.llama3_chat
+max_length = 2048
+pack_to_max_length = True
+
+# parallel
+sequence_parallel_size = 1
+
+# Scheduler & Optimizer
+batch_size = 1 # per_device
+accumulative_counts = 16
+accumulative_counts *= sequence_parallel_size
+dataloader_num_workers = 0
+max_epochs = 3
+optim_type = AdamW
+lr = 2e-5
+betas = (0.9, 0.999)
+weight_decay = 0
+max_norm = 1 # grad clip
+warmup_ratio = 0.03
+
+# Save
+save_steps = 500
+save_total_limit = 2 # Maximum checkpoints to keep (-1 means unlimited)
+
+# Evaluate the generation performance during the training
+evaluation_freq = 500
+SYSTEM = SYSTEM_TEMPLATE.alpaca
+evaluation_inputs = [
+ '请给我介绍五个上海的景点', 'Please tell me five scenic spots in Shanghai'
+]
+
+#######################################################################
+# PART 2 Model & Tokenizer #
+#######################################################################
+tokenizer = dict(
+ type=AutoTokenizer.from_pretrained,
+ pretrained_model_name_or_path=pretrained_model_name_or_path,
+ trust_remote_code=True,
+ padding_side='right')
+
+model = dict(
+ type=SupervisedFinetune,
+ use_varlen_attn=use_varlen_attn,
+ llm=dict(
+ type=AutoModelForCausalLM.from_pretrained,
+ pretrained_model_name_or_path=pretrained_model_name_or_path,
+ trust_remote_code=True))
+
+#######################################################################
+# PART 3 Dataset & Dataloader #
+#######################################################################
+alpaca_en = dict(
+ type=process_hf_dataset,
+ dataset=dict(type=load_dataset, path=alpaca_en_path),
+ tokenizer=tokenizer,
+ max_length=max_length,
+ dataset_map_fn=alpaca_map_fn,
+ template_map_fn=dict(
+ type=template_map_fn_factory, template=prompt_template),
+ remove_unused_columns=True,
+ shuffle_before_pack=True,
+ pack_to_max_length=pack_to_max_length,
+ use_varlen_attn=use_varlen_attn)
+
+sampler = SequenceParallelSampler \
+ if sequence_parallel_size > 1 else DefaultSampler
+train_dataloader = dict(
+ batch_size=batch_size,
+ num_workers=dataloader_num_workers,
+ dataset=alpaca_en,
+ sampler=dict(type=sampler, shuffle=True),
+ collate_fn=dict(type=default_collate_fn, use_varlen_attn=use_varlen_attn))
+
+#######################################################################
+# PART 4 Scheduler & Optimizer #
+#######################################################################
+# optimizer
+optim_wrapper = dict(
+ type=AmpOptimWrapper,
+ optimizer=dict(
+ type=optim_type, lr=lr, betas=betas, weight_decay=weight_decay),
+ clip_grad=dict(max_norm=max_norm, error_if_nonfinite=False),
+ accumulative_counts=accumulative_counts,
+ loss_scale='dynamic',
+ dtype='float16')
+
+# learning policy
+# More information: https://github.com/open-mmlab/mmengine/blob/main/docs/en/tutorials/param_scheduler.md # noqa: E501
+param_scheduler = [
+ dict(
+ type=LinearLR,
+ start_factor=1e-5,
+ by_epoch=True,
+ begin=0,
+ end=warmup_ratio * max_epochs,
+ convert_to_iter_based=True),
+ dict(
+ type=CosineAnnealingLR,
+ eta_min=0.0,
+ by_epoch=True,
+ begin=warmup_ratio * max_epochs,
+ end=max_epochs,
+ convert_to_iter_based=True)
+]
+
+# train, val, test setting
+train_cfg = dict(type=TrainLoop, max_epochs=max_epochs)
+
+#######################################################################
+# PART 5 Runtime #
+#######################################################################
+# Log the dialogue periodically during the training process, optional
+custom_hooks = [
+ dict(type=DatasetInfoHook, tokenizer=tokenizer),
+ dict(
+ type=EvaluateChatHook,
+ tokenizer=tokenizer,
+ every_n_iters=evaluation_freq,
+ evaluation_inputs=evaluation_inputs,
+ system=SYSTEM,
+ prompt_template=prompt_template)
+]
+
+if use_varlen_attn:
+ custom_hooks += [dict(type=VarlenAttnArgsToMessageHubHook)]
+
+# configure default hooks
+default_hooks = dict(
+ # record the time of every iteration.
+ timer=dict(type=IterTimerHook),
+ # print log every 10 iterations.
+ logger=dict(type=LoggerHook, log_metric_by_epoch=False, interval=10),
+ # enable the parameter scheduler.
+ param_scheduler=dict(type=ParamSchedulerHook),
+ # save checkpoint per `save_steps`.
+ checkpoint=dict(
+ type=CheckpointHook,
+ by_epoch=False,
+ interval=save_steps,
+ max_keep_ckpts=save_total_limit),
+ # set sampler seed in distributed evrionment.
+ sampler_seed=dict(type=DistSamplerSeedHook),
+)
+
+# configure environment
+env_cfg = dict(
+ # whether to enable cudnn benchmark
+ cudnn_benchmark=False,
+ # set multi process parameters
+ mp_cfg=dict(mp_start_method='fork', opencv_num_threads=0),
+ # set distributed parameters
+ dist_cfg=dict(backend='nccl'),
+)
+
+# set visualizer
+visualizer = None
+
+# set log level
+log_level = 'INFO'
+
+# load from which checkpoint
+load_from = None
+
+# whether to resume training from the loaded checkpoint
+resume = False
+
+# Defaults to use random seed and disable `deterministic`
+randomness = dict(seed=None, deterministic=False)
+
+# set log processor
+log_processor = dict(by_epoch=False)
diff --git a/xtuner/configs/llama/llama3_8b_instruct/llama3_8b_instruct_full_alpaca_e3.py b/xtuner/configs/llama/llama3_8b_instruct/llama3_8b_instruct_full_alpaca_e3.py
new file mode 100644
index 000000000..613ecad1e
--- /dev/null
+++ b/xtuner/configs/llama/llama3_8b_instruct/llama3_8b_instruct_full_alpaca_e3.py
@@ -0,0 +1,199 @@
+# Copyright (c) OpenMMLab. All rights reserved.
+from datasets import load_dataset
+from mmengine.dataset import DefaultSampler
+from mmengine.hooks import (CheckpointHook, DistSamplerSeedHook, IterTimerHook,
+ LoggerHook, ParamSchedulerHook)
+from mmengine.optim import AmpOptimWrapper, CosineAnnealingLR, LinearLR
+from torch.optim import AdamW
+from transformers import AutoModelForCausalLM, AutoTokenizer
+
+from xtuner.dataset import process_hf_dataset
+from xtuner.dataset.collate_fns import default_collate_fn
+from xtuner.dataset.map_fns import alpaca_map_fn, template_map_fn_factory
+from xtuner.engine.hooks import (DatasetInfoHook, EvaluateChatHook,
+ VarlenAttnArgsToMessageHubHook)
+from xtuner.engine.runner import TrainLoop
+from xtuner.model import SupervisedFinetune
+from xtuner.parallel.sequence import SequenceParallelSampler
+from xtuner.utils import PROMPT_TEMPLATE, SYSTEM_TEMPLATE
+
+#######################################################################
+# PART 1 Settings #
+#######################################################################
+# Model
+pretrained_model_name_or_path = 'meta-llama/Meta-Llama-3-8B-Instruct'
+use_varlen_attn = False
+
+# Data
+alpaca_en_path = 'tatsu-lab/alpaca'
+prompt_template = PROMPT_TEMPLATE.llama3_chat
+max_length = 2048
+pack_to_max_length = True
+
+# parallel
+sequence_parallel_size = 1
+
+# Scheduler & Optimizer
+batch_size = 1 # per_device
+accumulative_counts = 16
+accumulative_counts *= sequence_parallel_size
+dataloader_num_workers = 0
+max_epochs = 3
+optim_type = AdamW
+lr = 2e-5
+betas = (0.9, 0.999)
+weight_decay = 0
+max_norm = 1 # grad clip
+warmup_ratio = 0.03
+
+# Save
+save_steps = 500
+save_total_limit = 2 # Maximum checkpoints to keep (-1 means unlimited)
+
+# Evaluate the generation performance during the training
+evaluation_freq = 500
+SYSTEM = SYSTEM_TEMPLATE.alpaca
+evaluation_inputs = [
+ '请给我介绍五个上海的景点', 'Please tell me five scenic spots in Shanghai'
+]
+
+#######################################################################
+# PART 2 Model & Tokenizer #
+#######################################################################
+tokenizer = dict(
+ type=AutoTokenizer.from_pretrained,
+ pretrained_model_name_or_path=pretrained_model_name_or_path,
+ trust_remote_code=True,
+ padding_side='right')
+
+model = dict(
+ type=SupervisedFinetune,
+ use_varlen_attn=use_varlen_attn,
+ llm=dict(
+ type=AutoModelForCausalLM.from_pretrained,
+ pretrained_model_name_or_path=pretrained_model_name_or_path,
+ trust_remote_code=True))
+
+#######################################################################
+# PART 3 Dataset & Dataloader #
+#######################################################################
+alpaca_en = dict(
+ type=process_hf_dataset,
+ dataset=dict(type=load_dataset, path=alpaca_en_path),
+ tokenizer=tokenizer,
+ max_length=max_length,
+ dataset_map_fn=alpaca_map_fn,
+ template_map_fn=dict(
+ type=template_map_fn_factory, template=prompt_template),
+ remove_unused_columns=True,
+ shuffle_before_pack=True,
+ pack_to_max_length=pack_to_max_length,
+ use_varlen_attn=use_varlen_attn)
+
+sampler = SequenceParallelSampler \
+ if sequence_parallel_size > 1 else DefaultSampler
+train_dataloader = dict(
+ batch_size=batch_size,
+ num_workers=dataloader_num_workers,
+ dataset=alpaca_en,
+ sampler=dict(type=sampler, shuffle=True),
+ collate_fn=dict(type=default_collate_fn, use_varlen_attn=use_varlen_attn))
+
+#######################################################################
+# PART 4 Scheduler & Optimizer #
+#######################################################################
+# optimizer
+optim_wrapper = dict(
+ type=AmpOptimWrapper,
+ optimizer=dict(
+ type=optim_type, lr=lr, betas=betas, weight_decay=weight_decay),
+ clip_grad=dict(max_norm=max_norm, error_if_nonfinite=False),
+ accumulative_counts=accumulative_counts,
+ loss_scale='dynamic',
+ dtype='float16')
+
+# learning policy
+# More information: https://github.com/open-mmlab/mmengine/blob/main/docs/en/tutorials/param_scheduler.md # noqa: E501
+param_scheduler = [
+ dict(
+ type=LinearLR,
+ start_factor=1e-5,
+ by_epoch=True,
+ begin=0,
+ end=warmup_ratio * max_epochs,
+ convert_to_iter_based=True),
+ dict(
+ type=CosineAnnealingLR,
+ eta_min=0.0,
+ by_epoch=True,
+ begin=warmup_ratio * max_epochs,
+ end=max_epochs,
+ convert_to_iter_based=True)
+]
+
+# train, val, test setting
+train_cfg = dict(type=TrainLoop, max_epochs=max_epochs)
+
+#######################################################################
+# PART 5 Runtime #
+#######################################################################
+# Log the dialogue periodically during the training process, optional
+custom_hooks = [
+ dict(type=DatasetInfoHook, tokenizer=tokenizer),
+ dict(
+ type=EvaluateChatHook,
+ tokenizer=tokenizer,
+ every_n_iters=evaluation_freq,
+ evaluation_inputs=evaluation_inputs,
+ system=SYSTEM,
+ prompt_template=prompt_template)
+]
+
+if use_varlen_attn:
+ custom_hooks += [dict(type=VarlenAttnArgsToMessageHubHook)]
+
+# configure default hooks
+default_hooks = dict(
+ # record the time of every iteration.
+ timer=dict(type=IterTimerHook),
+ # print log every 10 iterations.
+ logger=dict(type=LoggerHook, log_metric_by_epoch=False, interval=10),
+ # enable the parameter scheduler.
+ param_scheduler=dict(type=ParamSchedulerHook),
+ # save checkpoint per `save_steps`.
+ checkpoint=dict(
+ type=CheckpointHook,
+ by_epoch=False,
+ interval=save_steps,
+ max_keep_ckpts=save_total_limit),
+ # set sampler seed in distributed evrionment.
+ sampler_seed=dict(type=DistSamplerSeedHook),
+)
+
+# configure environment
+env_cfg = dict(
+ # whether to enable cudnn benchmark
+ cudnn_benchmark=False,
+ # set multi process parameters
+ mp_cfg=dict(mp_start_method='fork', opencv_num_threads=0),
+ # set distributed parameters
+ dist_cfg=dict(backend='nccl'),
+)
+
+# set visualizer
+visualizer = None
+
+# set log level
+log_level = 'INFO'
+
+# load from which checkpoint
+load_from = None
+
+# whether to resume training from the loaded checkpoint
+resume = False
+
+# Defaults to use random seed and disable `deterministic`
+randomness = dict(seed=None, deterministic=False)
+
+# set log processor
+log_processor = dict(by_epoch=False)
diff --git a/xtuner/configs/llama/llama3_8b_instruct/llama3_8b_instruct_qlora_alpaca_e3.py b/xtuner/configs/llama/llama3_8b_instruct/llama3_8b_instruct_qlora_alpaca_e3.py
new file mode 100644
index 000000000..0373d41db
--- /dev/null
+++ b/xtuner/configs/llama/llama3_8b_instruct/llama3_8b_instruct_qlora_alpaca_e3.py
@@ -0,0 +1,219 @@
+# Copyright (c) OpenMMLab. All rights reserved.
+import torch
+from datasets import load_dataset
+from mmengine.dataset import DefaultSampler
+from mmengine.hooks import (CheckpointHook, DistSamplerSeedHook, IterTimerHook,
+ LoggerHook, ParamSchedulerHook)
+from mmengine.optim import AmpOptimWrapper, CosineAnnealingLR, LinearLR
+from peft import LoraConfig
+from torch.optim import AdamW
+from transformers import (AutoModelForCausalLM, AutoTokenizer,
+ BitsAndBytesConfig)
+
+from xtuner.dataset import process_hf_dataset
+from xtuner.dataset.collate_fns import default_collate_fn
+from xtuner.dataset.map_fns import alpaca_map_fn, template_map_fn_factory
+from xtuner.engine.hooks import (DatasetInfoHook, EvaluateChatHook,
+ VarlenAttnArgsToMessageHubHook)
+from xtuner.engine.runner import TrainLoop
+from xtuner.model import SupervisedFinetune
+from xtuner.parallel.sequence import SequenceParallelSampler
+from xtuner.utils import PROMPT_TEMPLATE, SYSTEM_TEMPLATE
+
+#######################################################################
+# PART 1 Settings #
+#######################################################################
+# Model
+pretrained_model_name_or_path = 'meta-llama/Meta-Llama-3-8B-Instruct'
+use_varlen_attn = False
+
+# Data
+alpaca_en_path = 'tatsu-lab/alpaca'
+prompt_template = PROMPT_TEMPLATE.llama3_chat
+max_length = 2048
+pack_to_max_length = True
+
+# parallel
+sequence_parallel_size = 1
+
+# Scheduler & Optimizer
+batch_size = 1 # per_device
+accumulative_counts = 16
+accumulative_counts *= sequence_parallel_size
+dataloader_num_workers = 0
+max_epochs = 3
+optim_type = AdamW
+lr = 2e-4
+betas = (0.9, 0.999)
+weight_decay = 0
+max_norm = 1 # grad clip
+warmup_ratio = 0.03
+
+# Save
+save_steps = 500
+save_total_limit = 2 # Maximum checkpoints to keep (-1 means unlimited)
+
+# Evaluate the generation performance during the training
+evaluation_freq = 500
+SYSTEM = SYSTEM_TEMPLATE.alpaca
+evaluation_inputs = [
+ '请给我介绍五个上海的景点', 'Please tell me five scenic spots in Shanghai'
+]
+
+#######################################################################
+# PART 2 Model & Tokenizer #
+#######################################################################
+tokenizer = dict(
+ type=AutoTokenizer.from_pretrained,
+ pretrained_model_name_or_path=pretrained_model_name_or_path,
+ trust_remote_code=True,
+ padding_side='right')
+
+model = dict(
+ type=SupervisedFinetune,
+ use_varlen_attn=use_varlen_attn,
+ llm=dict(
+ type=AutoModelForCausalLM.from_pretrained,
+ pretrained_model_name_or_path=pretrained_model_name_or_path,
+ trust_remote_code=True,
+ torch_dtype=torch.float16,
+ quantization_config=dict(
+ type=BitsAndBytesConfig,
+ load_in_4bit=True,
+ load_in_8bit=False,
+ llm_int8_threshold=6.0,
+ llm_int8_has_fp16_weight=False,
+ bnb_4bit_compute_dtype=torch.float16,
+ bnb_4bit_use_double_quant=True,
+ bnb_4bit_quant_type='nf4')),
+ lora=dict(
+ type=LoraConfig,
+ r=64,
+ lora_alpha=16,
+ lora_dropout=0.1,
+ bias='none',
+ task_type='CAUSAL_LM'))
+
+#######################################################################
+# PART 3 Dataset & Dataloader #
+#######################################################################
+alpaca_en = dict(
+ type=process_hf_dataset,
+ dataset=dict(type=load_dataset, path=alpaca_en_path),
+ tokenizer=tokenizer,
+ max_length=max_length,
+ dataset_map_fn=alpaca_map_fn,
+ template_map_fn=dict(
+ type=template_map_fn_factory, template=prompt_template),
+ remove_unused_columns=True,
+ shuffle_before_pack=True,
+ pack_to_max_length=pack_to_max_length,
+ use_varlen_attn=use_varlen_attn)
+
+sampler = SequenceParallelSampler \
+ if sequence_parallel_size > 1 else DefaultSampler
+train_dataloader = dict(
+ batch_size=batch_size,
+ num_workers=dataloader_num_workers,
+ dataset=alpaca_en,
+ sampler=dict(type=sampler, shuffle=True),
+ collate_fn=dict(type=default_collate_fn, use_varlen_attn=use_varlen_attn))
+
+#######################################################################
+# PART 4 Scheduler & Optimizer #
+#######################################################################
+# optimizer
+optim_wrapper = dict(
+ type=AmpOptimWrapper,
+ optimizer=dict(
+ type=optim_type, lr=lr, betas=betas, weight_decay=weight_decay),
+ clip_grad=dict(max_norm=max_norm, error_if_nonfinite=False),
+ accumulative_counts=accumulative_counts,
+ loss_scale='dynamic',
+ dtype='float16')
+
+# learning policy
+# More information: https://github.com/open-mmlab/mmengine/blob/main/docs/en/tutorials/param_scheduler.md # noqa: E501
+param_scheduler = [
+ dict(
+ type=LinearLR,
+ start_factor=1e-5,
+ by_epoch=True,
+ begin=0,
+ end=warmup_ratio * max_epochs,
+ convert_to_iter_based=True),
+ dict(
+ type=CosineAnnealingLR,
+ eta_min=0.0,
+ by_epoch=True,
+ begin=warmup_ratio * max_epochs,
+ end=max_epochs,
+ convert_to_iter_based=True)
+]
+
+# train, val, test setting
+train_cfg = dict(type=TrainLoop, max_epochs=max_epochs)
+
+#######################################################################
+# PART 5 Runtime #
+#######################################################################
+# Log the dialogue periodically during the training process, optional
+custom_hooks = [
+ dict(type=DatasetInfoHook, tokenizer=tokenizer),
+ dict(
+ type=EvaluateChatHook,
+ tokenizer=tokenizer,
+ every_n_iters=evaluation_freq,
+ evaluation_inputs=evaluation_inputs,
+ system=SYSTEM,
+ prompt_template=prompt_template)
+]
+
+if use_varlen_attn:
+ custom_hooks += [dict(type=VarlenAttnArgsToMessageHubHook)]
+
+# configure default hooks
+default_hooks = dict(
+ # record the time of every iteration.
+ timer=dict(type=IterTimerHook),
+ # print log every 10 iterations.
+ logger=dict(type=LoggerHook, log_metric_by_epoch=False, interval=10),
+ # enable the parameter scheduler.
+ param_scheduler=dict(type=ParamSchedulerHook),
+ # save checkpoint per `save_steps`.
+ checkpoint=dict(
+ type=CheckpointHook,
+ by_epoch=False,
+ interval=save_steps,
+ max_keep_ckpts=save_total_limit),
+ # set sampler seed in distributed evrionment.
+ sampler_seed=dict(type=DistSamplerSeedHook),
+)
+
+# configure environment
+env_cfg = dict(
+ # whether to enable cudnn benchmark
+ cudnn_benchmark=False,
+ # set multi process parameters
+ mp_cfg=dict(mp_start_method='fork', opencv_num_threads=0),
+ # set distributed parameters
+ dist_cfg=dict(backend='nccl'),
+)
+
+# set visualizer
+visualizer = None
+
+# set log level
+log_level = 'INFO'
+
+# load from which checkpoint
+load_from = None
+
+# whether to resume training from the loaded checkpoint
+resume = False
+
+# Defaults to use random seed and disable `deterministic`
+randomness = dict(seed=None, deterministic=False)
+
+# set log processor
+log_processor = dict(by_epoch=False)
diff --git a/xtuner/configs/llama_speed_benchmark/llama2_70b/llama2_70b_full_alpaca_enzh_128k_sp8.py b/xtuner/configs/llama_speed_benchmark/llama2_70b/llama2_70b_full_alpaca_enzh_128k_sp8.py
new file mode 100644
index 000000000..74554b469
--- /dev/null
+++ b/xtuner/configs/llama_speed_benchmark/llama2_70b/llama2_70b_full_alpaca_enzh_128k_sp8.py
@@ -0,0 +1,212 @@
+# Copyright (c) OpenMMLab. All rights reserved.
+from datasets import load_dataset
+from mmengine.hooks import (CheckpointHook, DistSamplerSeedHook, IterTimerHook,
+ LoggerHook, ParamSchedulerHook)
+from mmengine.optim import AmpOptimWrapper, CosineAnnealingLR, LinearLR
+from torch.optim import AdamW
+from transformers import AutoModelForCausalLM, AutoTokenizer
+
+from xtuner.dataset import ConcatDataset, process_hf_dataset
+from xtuner.dataset.collate_fns import default_collate_fn
+from xtuner.dataset.map_fns import (alpaca_map_fn, alpaca_zh_map_fn,
+ template_map_fn_factory)
+from xtuner.engine.hooks import ThroughputHook, VarlenAttnArgsToMessageHubHook
+from xtuner.engine.runner import TrainLoop
+from xtuner.model import SupervisedFinetune
+from xtuner.parallel.sequence import SequenceParallelSampler
+from xtuner.utils import PROMPT_TEMPLATE, SYSTEM_TEMPLATE
+
+#######################################################################
+# PART 1 Settings #
+#######################################################################
+# Model
+pretrained_model_name_or_path = 'meta-llama/Llama-2-70b-hf'
+use_varlen_attn = False
+sequence_parallel_size = 8
+
+# Data
+alpaca_zh_path = 'silk-road/alpaca-data-gpt4-chinese'
+alpaca_en_path = 'tatsu-lab/alpaca'
+prompt_template = PROMPT_TEMPLATE.llama2_chat
+max_length = 131072 # 128k
+pack_to_max_length = True
+
+# Scheduler & Optimizer
+batch_size = 1 # per_device
+# Suppose I aim to employ a training strategy using a batch size per device
+# of 1 with a maximum length of `max_length` on N GPUs.
+# Upon setting the sequence parallelism dimension to `SP`,
+# the accumulative counts have to be adjusted to `SP` times the original value.
+# This modification is essential to assure training equivalence,
+# as the sequence of `max_length` length will be segmented into `SP` parts,
+# with each part being allocated to its respective GPU among the `SP` GPUs
+# for parallelized training.
+# bs = 32 gpus * 1 batch_size_per_device * 8 acc / 8 sequence parallel
+accumulative_counts = 8
+dataloader_num_workers = 4
+max_epochs = 3
+optim_type = AdamW
+lr = 2e-5
+betas = (0.9, 0.999)
+weight_decay = 0
+max_norm = 1 # grad clip
+warmup_ratio = 0.03
+log_interval = 1
+
+# Save
+save_steps = -1 # speed only
+save_total_limit = 2 # Maximum checkpoints to keep (-1 means unlimited)
+
+# Evaluate the generation performance during the training
+evaluation_freq = 50
+SYSTEM = SYSTEM_TEMPLATE.alpaca
+evaluation_inputs = [
+ '请给我介绍五个上海的景点', 'Please tell me five scenic spots in Shanghai'
+]
+
+#######################################################################
+# PART 2 Model & Tokenizer #
+#######################################################################
+tokenizer = dict(
+ type=AutoTokenizer.from_pretrained,
+ pretrained_model_name_or_path=pretrained_model_name_or_path,
+ trust_remote_code=True,
+ padding_side='right')
+
+model = dict(
+ type=SupervisedFinetune,
+ use_varlen_attn=use_varlen_attn,
+ llm=dict(
+ type=AutoModelForCausalLM.from_pretrained,
+ pretrained_model_name_or_path=pretrained_model_name_or_path,
+ trust_remote_code=True))
+
+#######################################################################
+# PART 3 Dataset & Dataloader #
+#######################################################################
+alpaca_en = dict(
+ type=process_hf_dataset,
+ dataset=dict(type=load_dataset, path=alpaca_en_path),
+ tokenizer=tokenizer,
+ max_length=max_length,
+ dataset_map_fn=alpaca_map_fn,
+ template_map_fn=dict(
+ type=template_map_fn_factory, template=prompt_template),
+ remove_unused_columns=True,
+ shuffle_before_pack=True,
+ pack_to_max_length=pack_to_max_length,
+ use_varlen_attn=use_varlen_attn)
+
+alpaca_zh = dict(
+ type=process_hf_dataset,
+ dataset=dict(type=load_dataset, path=alpaca_zh_path),
+ tokenizer=tokenizer,
+ max_length=max_length,
+ dataset_map_fn=alpaca_zh_map_fn,
+ template_map_fn=dict(
+ type=template_map_fn_factory, template=prompt_template),
+ remove_unused_columns=True,
+ shuffle_before_pack=True,
+ pack_to_max_length=pack_to_max_length,
+ use_varlen_attn=use_varlen_attn)
+
+train_dataset = dict(type=ConcatDataset, datasets=[alpaca_en, alpaca_zh])
+
+train_dataloader = dict(
+ batch_size=batch_size,
+ num_workers=dataloader_num_workers,
+ dataset=train_dataset,
+ sampler=dict(type=SequenceParallelSampler, seed=1024),
+ collate_fn=dict(type=default_collate_fn, use_varlen_attn=use_varlen_attn))
+
+#######################################################################
+# PART 4 Scheduler & Optimizer #
+#######################################################################
+# optimizer
+optim_wrapper = dict(
+ type=AmpOptimWrapper,
+ optimizer=dict(
+ type=optim_type, lr=lr, betas=betas, weight_decay=weight_decay),
+ clip_grad=dict(max_norm=max_norm, error_if_nonfinite=False),
+ accumulative_counts=accumulative_counts,
+ loss_scale='dynamic',
+ dtype='float16')
+
+# learning policy
+# More information: https://github.com/open-mmlab/mmengine/blob/main/docs/en/tutorials/param_scheduler.md # noqa: E501
+param_scheduler = [
+ dict(
+ type=LinearLR,
+ start_factor=1e-5,
+ by_epoch=True,
+ begin=0,
+ end=warmup_ratio * max_epochs,
+ convert_to_iter_based=True),
+ dict(
+ type=CosineAnnealingLR,
+ eta_min=0.0,
+ by_epoch=True,
+ begin=warmup_ratio * max_epochs,
+ end=max_epochs,
+ convert_to_iter_based=True)
+]
+
+# train, val, test setting
+train_cfg = dict(type=TrainLoop, max_epochs=max_epochs)
+
+#######################################################################
+# PART 5 Runtime #
+#######################################################################
+# Log the dialogue periodically during the training process, optional
+custom_hooks = [dict(type=ThroughputHook)]
+
+if use_varlen_attn:
+ custom_hooks += [dict(type=VarlenAttnArgsToMessageHubHook)]
+
+# configure default hooks
+default_hooks = dict(
+ # record the time of every iteration.
+ timer=dict(type=IterTimerHook),
+ # print log every 10 iterations.
+ logger=dict(
+ type=LoggerHook, log_metric_by_epoch=False, interval=log_interval),
+ # enable the parameter scheduler.
+ param_scheduler=dict(type=ParamSchedulerHook),
+ # save checkpoint per `save_steps`.
+ checkpoint=dict(
+ type=CheckpointHook,
+ by_epoch=False,
+ interval=-1,
+ save_last=False,
+ max_keep_ckpts=save_total_limit),
+ # set sampler seed in distributed evrionment.
+ sampler_seed=dict(type=DistSamplerSeedHook),
+)
+
+# configure environment
+env_cfg = dict(
+ # whether to enable cudnn benchmark
+ cudnn_benchmark=False,
+ # set multi process parameters
+ mp_cfg=dict(mp_start_method='fork', opencv_num_threads=0),
+ # set distributed parameters
+ dist_cfg=dict(backend='nccl'),
+)
+
+# set visualizer
+visualizer = None
+
+# set log level
+log_level = 'INFO'
+
+# load from which checkpoint
+load_from = None
+
+# whether to resume training from the loaded checkpoint
+resume = False
+
+# Defaults to use random seed and disable `deterministic`
+randomness = dict(seed=None, deterministic=False)
+
+# set log processor
+log_processor = dict(by_epoch=False, window_size=log_interval)
diff --git a/xtuner/configs/llama_speed_benchmark/llama2_70b/llama2_70b_full_alpaca_enzh_256k_sp16.py b/xtuner/configs/llama_speed_benchmark/llama2_70b/llama2_70b_full_alpaca_enzh_256k_sp16.py
new file mode 100644
index 000000000..f0c213945
--- /dev/null
+++ b/xtuner/configs/llama_speed_benchmark/llama2_70b/llama2_70b_full_alpaca_enzh_256k_sp16.py
@@ -0,0 +1,212 @@
+# Copyright (c) OpenMMLab. All rights reserved.
+from datasets import load_dataset
+from mmengine.hooks import (CheckpointHook, DistSamplerSeedHook, IterTimerHook,
+ LoggerHook, ParamSchedulerHook)
+from mmengine.optim import AmpOptimWrapper, CosineAnnealingLR, LinearLR
+from torch.optim import AdamW
+from transformers import AutoModelForCausalLM, AutoTokenizer
+
+from xtuner.dataset import ConcatDataset, process_hf_dataset
+from xtuner.dataset.collate_fns import default_collate_fn
+from xtuner.dataset.map_fns import (alpaca_map_fn, alpaca_zh_map_fn,
+ template_map_fn_factory)
+from xtuner.engine.hooks import ThroughputHook, VarlenAttnArgsToMessageHubHook
+from xtuner.engine.runner import TrainLoop
+from xtuner.model import SupervisedFinetune
+from xtuner.parallel.sequence import SequenceParallelSampler
+from xtuner.utils import PROMPT_TEMPLATE, SYSTEM_TEMPLATE
+
+#######################################################################
+# PART 1 Settings #
+#######################################################################
+# Model
+pretrained_model_name_or_path = 'meta-llama/Llama-2-70b-hf'
+use_varlen_attn = False
+sequence_parallel_size = 16
+
+# Data
+alpaca_zh_path = 'silk-road/alpaca-data-gpt4-chinese'
+alpaca_en_path = 'tatsu-lab/alpaca'
+prompt_template = PROMPT_TEMPLATE.llama2_chat
+max_length = 262144 # 256k
+pack_to_max_length = True
+
+# Scheduler & Optimizer
+batch_size = 1 # per_device
+# Suppose I aim to employ a training strategy using a batch size per device
+# of 1 with a maximum length of `max_length` on N GPUs.
+# Upon setting the sequence parallelism dimension to `SP`,
+# the accumulative counts have to be adjusted to `SP` times the original value.
+# This modification is essential to assure training equivalence,
+# as the sequence of `max_length` length will be segmented into `SP` parts,
+# with each part being allocated to its respective GPU among the `SP` GPUs
+# for parallelized training.
+# bs = 32 gpus * 1 batch_size_per_device * 16 acc / 16 sequence parallel
+accumulative_counts = 16
+dataloader_num_workers = 4
+max_epochs = 3
+optim_type = AdamW
+lr = 2e-5
+betas = (0.9, 0.999)
+weight_decay = 0
+max_norm = 1 # grad clip
+warmup_ratio = 0.03
+log_interval = 1
+
+# Save
+save_steps = -1 # speed only
+save_total_limit = 2 # Maximum checkpoints to keep (-1 means unlimited)
+
+# Evaluate the generation performance during the training
+evaluation_freq = 50
+SYSTEM = SYSTEM_TEMPLATE.alpaca
+evaluation_inputs = [
+ '请给我介绍五个上海的景点', 'Please tell me five scenic spots in Shanghai'
+]
+
+#######################################################################
+# PART 2 Model & Tokenizer #
+#######################################################################
+tokenizer = dict(
+ type=AutoTokenizer.from_pretrained,
+ pretrained_model_name_or_path=pretrained_model_name_or_path,
+ trust_remote_code=True,
+ padding_side='right')
+
+model = dict(
+ type=SupervisedFinetune,
+ use_varlen_attn=use_varlen_attn,
+ llm=dict(
+ type=AutoModelForCausalLM.from_pretrained,
+ pretrained_model_name_or_path=pretrained_model_name_or_path,
+ trust_remote_code=True))
+
+#######################################################################
+# PART 3 Dataset & Dataloader #
+#######################################################################
+alpaca_en = dict(
+ type=process_hf_dataset,
+ dataset=dict(type=load_dataset, path=alpaca_en_path),
+ tokenizer=tokenizer,
+ max_length=max_length,
+ dataset_map_fn=alpaca_map_fn,
+ template_map_fn=dict(
+ type=template_map_fn_factory, template=prompt_template),
+ remove_unused_columns=True,
+ shuffle_before_pack=True,
+ pack_to_max_length=pack_to_max_length,
+ use_varlen_attn=use_varlen_attn)
+
+alpaca_zh = dict(
+ type=process_hf_dataset,
+ dataset=dict(type=load_dataset, path=alpaca_zh_path),
+ tokenizer=tokenizer,
+ max_length=max_length,
+ dataset_map_fn=alpaca_zh_map_fn,
+ template_map_fn=dict(
+ type=template_map_fn_factory, template=prompt_template),
+ remove_unused_columns=True,
+ shuffle_before_pack=True,
+ pack_to_max_length=pack_to_max_length,
+ use_varlen_attn=use_varlen_attn)
+
+train_dataset = dict(type=ConcatDataset, datasets=[alpaca_en, alpaca_zh])
+
+train_dataloader = dict(
+ batch_size=batch_size,
+ num_workers=dataloader_num_workers,
+ dataset=train_dataset,
+ sampler=dict(type=SequenceParallelSampler, seed=1024),
+ collate_fn=dict(type=default_collate_fn, use_varlen_attn=use_varlen_attn))
+
+#######################################################################
+# PART 4 Scheduler & Optimizer #
+#######################################################################
+# optimizer
+optim_wrapper = dict(
+ type=AmpOptimWrapper,
+ optimizer=dict(
+ type=optim_type, lr=lr, betas=betas, weight_decay=weight_decay),
+ clip_grad=dict(max_norm=max_norm, error_if_nonfinite=False),
+ accumulative_counts=accumulative_counts,
+ loss_scale='dynamic',
+ dtype='float16')
+
+# learning policy
+# More information: https://github.com/open-mmlab/mmengine/blob/main/docs/en/tutorials/param_scheduler.md # noqa: E501
+param_scheduler = [
+ dict(
+ type=LinearLR,
+ start_factor=1e-5,
+ by_epoch=True,
+ begin=0,
+ end=warmup_ratio * max_epochs,
+ convert_to_iter_based=True),
+ dict(
+ type=CosineAnnealingLR,
+ eta_min=0.0,
+ by_epoch=True,
+ begin=warmup_ratio * max_epochs,
+ end=max_epochs,
+ convert_to_iter_based=True)
+]
+
+# train, val, test setting
+train_cfg = dict(type=TrainLoop, max_epochs=max_epochs)
+
+#######################################################################
+# PART 5 Runtime #
+#######################################################################
+# Log the dialogue periodically during the training process, optional
+custom_hooks = [dict(type=ThroughputHook)]
+
+if use_varlen_attn:
+ custom_hooks += [dict(type=VarlenAttnArgsToMessageHubHook)]
+
+# configure default hooks
+default_hooks = dict(
+ # record the time of every iteration.
+ timer=dict(type=IterTimerHook),
+ # print log every 10 iterations.
+ logger=dict(
+ type=LoggerHook, log_metric_by_epoch=False, interval=log_interval),
+ # enable the parameter scheduler.
+ param_scheduler=dict(type=ParamSchedulerHook),
+ # save checkpoint per `save_steps`.
+ checkpoint=dict(
+ type=CheckpointHook,
+ by_epoch=False,
+ interval=-1,
+ save_last=False,
+ max_keep_ckpts=save_total_limit),
+ # set sampler seed in distributed evrionment.
+ sampler_seed=dict(type=DistSamplerSeedHook),
+)
+
+# configure environment
+env_cfg = dict(
+ # whether to enable cudnn benchmark
+ cudnn_benchmark=False,
+ # set multi process parameters
+ mp_cfg=dict(mp_start_method='fork', opencv_num_threads=0),
+ # set distributed parameters
+ dist_cfg=dict(backend='nccl'),
+)
+
+# set visualizer
+visualizer = None
+
+# set log level
+log_level = 'INFO'
+
+# load from which checkpoint
+load_from = None
+
+# whether to resume training from the loaded checkpoint
+resume = False
+
+# Defaults to use random seed and disable `deterministic`
+randomness = dict(seed=None, deterministic=False)
+
+# set log processor
+log_processor = dict(by_epoch=False, window_size=log_interval)
diff --git a/xtuner/configs/llama_speed_benchmark/llama2_70b/llama2_70b_full_alpaca_enzh_32k_sp4.py b/xtuner/configs/llama_speed_benchmark/llama2_70b/llama2_70b_full_alpaca_enzh_32k_sp4.py
new file mode 100644
index 000000000..679e89107
--- /dev/null
+++ b/xtuner/configs/llama_speed_benchmark/llama2_70b/llama2_70b_full_alpaca_enzh_32k_sp4.py
@@ -0,0 +1,212 @@
+# Copyright (c) OpenMMLab. All rights reserved.
+from datasets import load_dataset
+from mmengine.hooks import (CheckpointHook, DistSamplerSeedHook, IterTimerHook,
+ LoggerHook, ParamSchedulerHook)
+from mmengine.optim import AmpOptimWrapper, CosineAnnealingLR, LinearLR
+from torch.optim import AdamW
+from transformers import AutoModelForCausalLM, AutoTokenizer
+
+from xtuner.dataset import ConcatDataset, process_hf_dataset
+from xtuner.dataset.collate_fns import default_collate_fn
+from xtuner.dataset.map_fns import (alpaca_map_fn, alpaca_zh_map_fn,
+ template_map_fn_factory)
+from xtuner.engine.hooks import ThroughputHook, VarlenAttnArgsToMessageHubHook
+from xtuner.engine.runner import TrainLoop
+from xtuner.model import SupervisedFinetune
+from xtuner.parallel.sequence import SequenceParallelSampler
+from xtuner.utils import PROMPT_TEMPLATE, SYSTEM_TEMPLATE
+
+#######################################################################
+# PART 1 Settings #
+#######################################################################
+# Model
+pretrained_model_name_or_path = 'meta-llama/Llama-2-70b-hf'
+use_varlen_attn = False
+sequence_parallel_size = 4
+
+# Data
+alpaca_zh_path = 'silk-road/alpaca-data-gpt4-chinese'
+alpaca_en_path = 'tatsu-lab/alpaca'
+prompt_template = PROMPT_TEMPLATE.llama2_chat
+max_length = 32768
+pack_to_max_length = True
+
+# Scheduler & Optimizer
+batch_size = 1 # per_device
+# Suppose I aim to employ a training strategy using a batch size per device
+# of 1 with a maximum length of `max_length` on N GPUs.
+# Upon setting the sequence parallelism dimension to `SP`,
+# the accumulative counts have to be adjusted to `SP` times the original value.
+# This modification is essential to assure training equivalence,
+# as the sequence of `max_length` length will be segmented into `SP` parts,
+# with each part being allocated to its respective GPU among the `SP` GPUs
+# for parallelized training.
+# bs = 32 gpus * 1 batch_size_per_device * 4 acc / 4 sequence parallel
+accumulative_counts = 4
+dataloader_num_workers = 4
+max_epochs = 3
+optim_type = AdamW
+lr = 2e-5
+betas = (0.9, 0.999)
+weight_decay = 0
+max_norm = 1 # grad clip
+warmup_ratio = 0.03
+log_interval = 1
+
+# Save
+save_steps = -1 # speed only
+save_total_limit = 2 # Maximum checkpoints to keep (-1 means unlimited)
+
+# Evaluate the generation performance during the training
+evaluation_freq = 50
+SYSTEM = SYSTEM_TEMPLATE.alpaca
+evaluation_inputs = [
+ '请给我介绍五个上海的景点', 'Please tell me five scenic spots in Shanghai'
+]
+
+#######################################################################
+# PART 2 Model & Tokenizer #
+#######################################################################
+tokenizer = dict(
+ type=AutoTokenizer.from_pretrained,
+ pretrained_model_name_or_path=pretrained_model_name_or_path,
+ trust_remote_code=True,
+ padding_side='right')
+
+model = dict(
+ type=SupervisedFinetune,
+ use_varlen_attn=use_varlen_attn,
+ llm=dict(
+ type=AutoModelForCausalLM.from_pretrained,
+ pretrained_model_name_or_path=pretrained_model_name_or_path,
+ trust_remote_code=True))
+
+#######################################################################
+# PART 3 Dataset & Dataloader #
+#######################################################################
+alpaca_en = dict(
+ type=process_hf_dataset,
+ dataset=dict(type=load_dataset, path=alpaca_en_path),
+ tokenizer=tokenizer,
+ max_length=max_length,
+ dataset_map_fn=alpaca_map_fn,
+ template_map_fn=dict(
+ type=template_map_fn_factory, template=prompt_template),
+ remove_unused_columns=True,
+ shuffle_before_pack=True,
+ pack_to_max_length=pack_to_max_length,
+ use_varlen_attn=use_varlen_attn)
+
+alpaca_zh = dict(
+ type=process_hf_dataset,
+ dataset=dict(type=load_dataset, path=alpaca_zh_path),
+ tokenizer=tokenizer,
+ max_length=max_length,
+ dataset_map_fn=alpaca_zh_map_fn,
+ template_map_fn=dict(
+ type=template_map_fn_factory, template=prompt_template),
+ remove_unused_columns=True,
+ shuffle_before_pack=True,
+ pack_to_max_length=pack_to_max_length,
+ use_varlen_attn=use_varlen_attn)
+
+train_dataset = dict(type=ConcatDataset, datasets=[alpaca_en, alpaca_zh])
+
+train_dataloader = dict(
+ batch_size=batch_size,
+ num_workers=dataloader_num_workers,
+ dataset=train_dataset,
+ sampler=dict(type=SequenceParallelSampler, seed=1024),
+ collate_fn=dict(type=default_collate_fn, use_varlen_attn=use_varlen_attn))
+
+#######################################################################
+# PART 4 Scheduler & Optimizer #
+#######################################################################
+# optimizer
+optim_wrapper = dict(
+ type=AmpOptimWrapper,
+ optimizer=dict(
+ type=optim_type, lr=lr, betas=betas, weight_decay=weight_decay),
+ clip_grad=dict(max_norm=max_norm, error_if_nonfinite=False),
+ accumulative_counts=accumulative_counts,
+ loss_scale='dynamic',
+ dtype='float16')
+
+# learning policy
+# More information: https://github.com/open-mmlab/mmengine/blob/main/docs/en/tutorials/param_scheduler.md # noqa: E501
+param_scheduler = [
+ dict(
+ type=LinearLR,
+ start_factor=1e-5,
+ by_epoch=True,
+ begin=0,
+ end=warmup_ratio * max_epochs,
+ convert_to_iter_based=True),
+ dict(
+ type=CosineAnnealingLR,
+ eta_min=0.0,
+ by_epoch=True,
+ begin=warmup_ratio * max_epochs,
+ end=max_epochs,
+ convert_to_iter_based=True)
+]
+
+# train, val, test setting
+train_cfg = dict(type=TrainLoop, max_epochs=max_epochs)
+
+#######################################################################
+# PART 5 Runtime #
+#######################################################################
+# Log the dialogue periodically during the training process, optional
+custom_hooks = [dict(type=ThroughputHook)]
+
+if use_varlen_attn:
+ custom_hooks += [dict(type=VarlenAttnArgsToMessageHubHook)]
+
+# configure default hooks
+default_hooks = dict(
+ # record the time of every iteration.
+ timer=dict(type=IterTimerHook),
+ # print log every 10 iterations.
+ logger=dict(
+ type=LoggerHook, log_metric_by_epoch=False, interval=log_interval),
+ # enable the parameter scheduler.
+ param_scheduler=dict(type=ParamSchedulerHook),
+ # save checkpoint per `save_steps`.
+ checkpoint=dict(
+ type=CheckpointHook,
+ by_epoch=False,
+ interval=-1,
+ save_last=False,
+ max_keep_ckpts=save_total_limit),
+ # set sampler seed in distributed evrionment.
+ sampler_seed=dict(type=DistSamplerSeedHook),
+)
+
+# configure environment
+env_cfg = dict(
+ # whether to enable cudnn benchmark
+ cudnn_benchmark=False,
+ # set multi process parameters
+ mp_cfg=dict(mp_start_method='fork', opencv_num_threads=0),
+ # set distributed parameters
+ dist_cfg=dict(backend='nccl'),
+)
+
+# set visualizer
+visualizer = None
+
+# set log level
+log_level = 'INFO'
+
+# load from which checkpoint
+load_from = None
+
+# whether to resume training from the loaded checkpoint
+resume = False
+
+# Defaults to use random seed and disable `deterministic`
+randomness = dict(seed=None, deterministic=False)
+
+# set log processor
+log_processor = dict(by_epoch=False, window_size=log_interval)
diff --git a/xtuner/configs/llama_speed_benchmark/llama2_70b/llama2_70b_full_alpaca_enzh_8k_sp1.py b/xtuner/configs/llama_speed_benchmark/llama2_70b/llama2_70b_full_alpaca_enzh_8k_sp1.py
new file mode 100644
index 000000000..7ddc66215
--- /dev/null
+++ b/xtuner/configs/llama_speed_benchmark/llama2_70b/llama2_70b_full_alpaca_enzh_8k_sp1.py
@@ -0,0 +1,212 @@
+# Copyright (c) OpenMMLab. All rights reserved.
+from datasets import load_dataset
+from mmengine.hooks import (CheckpointHook, DistSamplerSeedHook, IterTimerHook,
+ LoggerHook, ParamSchedulerHook)
+from mmengine.optim import AmpOptimWrapper, CosineAnnealingLR, LinearLR
+from torch.optim import AdamW
+from transformers import AutoModelForCausalLM, AutoTokenizer
+
+from xtuner.dataset import ConcatDataset, process_hf_dataset
+from xtuner.dataset.collate_fns import default_collate_fn
+from xtuner.dataset.map_fns import (alpaca_map_fn, alpaca_zh_map_fn,
+ template_map_fn_factory)
+from xtuner.engine.hooks import ThroughputHook, VarlenAttnArgsToMessageHubHook
+from xtuner.engine.runner import TrainLoop
+from xtuner.model import SupervisedFinetune
+from xtuner.parallel.sequence import SequenceParallelSampler
+from xtuner.utils import PROMPT_TEMPLATE, SYSTEM_TEMPLATE
+
+#######################################################################
+# PART 1 Settings #
+#######################################################################
+# Model
+pretrained_model_name_or_path = 'meta-llama/Llama-2-70b-hf'
+use_varlen_attn = False
+sequence_parallel_size = 1
+
+# Data
+alpaca_zh_path = 'silk-road/alpaca-data-gpt4-chinese'
+alpaca_en_path = 'tatsu-lab/alpaca'
+prompt_template = PROMPT_TEMPLATE.llama2_chat
+max_length = 8192
+pack_to_max_length = True
+
+# Scheduler & Optimizer
+batch_size = 1 # per_device
+# Suppose I aim to employ a training strategy using a batch size per device
+# of 1 with a maximum length of `max_length` on N GPUs.
+# Upon setting the sequence parallelism dimension to `SP`,
+# the accumulative counts have to be adjusted to `SP` times the original value.
+# This modification is essential to assure training equivalence,
+# as the sequence of `max_length` length will be segmented into `SP` parts,
+# with each part being allocated to its respective GPU among the `SP` GPUs
+# for parallelized training.
+# bs = 32 gpus * 1 batch_size_per_device * 1 acc / 1 sequence parallel
+accumulative_counts = 1
+dataloader_num_workers = 4
+max_epochs = 3
+optim_type = AdamW
+lr = 2e-5
+betas = (0.9, 0.999)
+weight_decay = 0
+max_norm = 1 # grad clip
+warmup_ratio = 0.03
+log_interval = 1
+
+# Save
+save_steps = -1 # speed only
+save_total_limit = 2 # Maximum checkpoints to keep (-1 means unlimited)
+
+# Evaluate the generation performance during the training
+evaluation_freq = 50
+SYSTEM = SYSTEM_TEMPLATE.alpaca
+evaluation_inputs = [
+ '请给我介绍五个上海的景点', 'Please tell me five scenic spots in Shanghai'
+]
+
+#######################################################################
+# PART 2 Model & Tokenizer #
+#######################################################################
+tokenizer = dict(
+ type=AutoTokenizer.from_pretrained,
+ pretrained_model_name_or_path=pretrained_model_name_or_path,
+ trust_remote_code=True,
+ padding_side='right')
+
+model = dict(
+ type=SupervisedFinetune,
+ use_varlen_attn=use_varlen_attn,
+ llm=dict(
+ type=AutoModelForCausalLM.from_pretrained,
+ pretrained_model_name_or_path=pretrained_model_name_or_path,
+ trust_remote_code=True))
+
+#######################################################################
+# PART 3 Dataset & Dataloader #
+#######################################################################
+alpaca_en = dict(
+ type=process_hf_dataset,
+ dataset=dict(type=load_dataset, path=alpaca_en_path),
+ tokenizer=tokenizer,
+ max_length=max_length,
+ dataset_map_fn=alpaca_map_fn,
+ template_map_fn=dict(
+ type=template_map_fn_factory, template=prompt_template),
+ remove_unused_columns=True,
+ shuffle_before_pack=True,
+ pack_to_max_length=pack_to_max_length,
+ use_varlen_attn=use_varlen_attn)
+
+alpaca_zh = dict(
+ type=process_hf_dataset,
+ dataset=dict(type=load_dataset, path=alpaca_zh_path),
+ tokenizer=tokenizer,
+ max_length=max_length,
+ dataset_map_fn=alpaca_zh_map_fn,
+ template_map_fn=dict(
+ type=template_map_fn_factory, template=prompt_template),
+ remove_unused_columns=True,
+ shuffle_before_pack=True,
+ pack_to_max_length=pack_to_max_length,
+ use_varlen_attn=use_varlen_attn)
+
+train_dataset = dict(type=ConcatDataset, datasets=[alpaca_en, alpaca_zh])
+
+train_dataloader = dict(
+ batch_size=batch_size,
+ num_workers=dataloader_num_workers,
+ dataset=train_dataset,
+ sampler=dict(type=SequenceParallelSampler, seed=1024),
+ collate_fn=dict(type=default_collate_fn, use_varlen_attn=use_varlen_attn))
+
+#######################################################################
+# PART 4 Scheduler & Optimizer #
+#######################################################################
+# optimizer
+optim_wrapper = dict(
+ type=AmpOptimWrapper,
+ optimizer=dict(
+ type=optim_type, lr=lr, betas=betas, weight_decay=weight_decay),
+ clip_grad=dict(max_norm=max_norm, error_if_nonfinite=False),
+ accumulative_counts=accumulative_counts,
+ loss_scale='dynamic',
+ dtype='float16')
+
+# learning policy
+# More information: https://github.com/open-mmlab/mmengine/blob/main/docs/en/tutorials/param_scheduler.md # noqa: E501
+param_scheduler = [
+ dict(
+ type=LinearLR,
+ start_factor=1e-5,
+ by_epoch=True,
+ begin=0,
+ end=warmup_ratio * max_epochs,
+ convert_to_iter_based=True),
+ dict(
+ type=CosineAnnealingLR,
+ eta_min=0.0,
+ by_epoch=True,
+ begin=warmup_ratio * max_epochs,
+ end=max_epochs,
+ convert_to_iter_based=True)
+]
+
+# train, val, test setting
+train_cfg = dict(type=TrainLoop, max_epochs=max_epochs)
+
+#######################################################################
+# PART 5 Runtime #
+#######################################################################
+# Log the dialogue periodically during the training process, optional
+custom_hooks = [dict(type=ThroughputHook)]
+
+if use_varlen_attn:
+ custom_hooks += [dict(type=VarlenAttnArgsToMessageHubHook)]
+
+# configure default hooks
+default_hooks = dict(
+ # record the time of every iteration.
+ timer=dict(type=IterTimerHook),
+ # print log every 10 iterations.
+ logger=dict(
+ type=LoggerHook, log_metric_by_epoch=False, interval=log_interval),
+ # enable the parameter scheduler.
+ param_scheduler=dict(type=ParamSchedulerHook),
+ # save checkpoint per `save_steps`.
+ checkpoint=dict(
+ type=CheckpointHook,
+ by_epoch=False,
+ interval=-1,
+ save_last=False,
+ max_keep_ckpts=save_total_limit),
+ # set sampler seed in distributed evrionment.
+ sampler_seed=dict(type=DistSamplerSeedHook),
+)
+
+# configure environment
+env_cfg = dict(
+ # whether to enable cudnn benchmark
+ cudnn_benchmark=False,
+ # set multi process parameters
+ mp_cfg=dict(mp_start_method='fork', opencv_num_threads=0),
+ # set distributed parameters
+ dist_cfg=dict(backend='nccl'),
+)
+
+# set visualizer
+visualizer = None
+
+# set log level
+log_level = 'INFO'
+
+# load from which checkpoint
+load_from = None
+
+# whether to resume training from the loaded checkpoint
+resume = False
+
+# Defaults to use random seed and disable `deterministic`
+randomness = dict(seed=None, deterministic=False)
+
+# set log processor
+log_processor = dict(by_epoch=False, window_size=log_interval)
diff --git a/xtuner/configs/llama_speed_benchmark/llama2_7b/llama2_7b_full_alpaca_enzh_128k_sp8.py b/xtuner/configs/llama_speed_benchmark/llama2_7b/llama2_7b_full_alpaca_enzh_128k_sp8.py
new file mode 100644
index 000000000..6be9ef2df
--- /dev/null
+++ b/xtuner/configs/llama_speed_benchmark/llama2_7b/llama2_7b_full_alpaca_enzh_128k_sp8.py
@@ -0,0 +1,212 @@
+# Copyright (c) OpenMMLab. All rights reserved.
+from datasets import load_dataset
+from mmengine.hooks import (CheckpointHook, DistSamplerSeedHook, IterTimerHook,
+ LoggerHook, ParamSchedulerHook)
+from mmengine.optim import AmpOptimWrapper, CosineAnnealingLR, LinearLR
+from torch.optim import AdamW
+from transformers import AutoModelForCausalLM, AutoTokenizer
+
+from xtuner.dataset import ConcatDataset, process_hf_dataset
+from xtuner.dataset.collate_fns import default_collate_fn
+from xtuner.dataset.map_fns import (alpaca_map_fn, alpaca_zh_map_fn,
+ template_map_fn_factory)
+from xtuner.engine.hooks import ThroughputHook, VarlenAttnArgsToMessageHubHook
+from xtuner.engine.runner import TrainLoop
+from xtuner.model import SupervisedFinetune
+from xtuner.parallel.sequence import SequenceParallelSampler
+from xtuner.utils import PROMPT_TEMPLATE, SYSTEM_TEMPLATE
+
+#######################################################################
+# PART 1 Settings #
+#######################################################################
+# Model
+pretrained_model_name_or_path = 'meta-llama/Llama-2-7b'
+use_varlen_attn = False
+sequence_parallel_size = 8
+
+# Data
+alpaca_zh_path = 'silk-road/alpaca-data-gpt4-chinese'
+alpaca_en_path = 'tatsu-lab/alpaca'
+prompt_template = PROMPT_TEMPLATE.llama2_chat
+max_length = 131072 # 128k
+pack_to_max_length = True
+
+# Scheduler & Optimizer
+batch_size = 1 # per_device
+# Suppose I aim to employ a training strategy using a batch size per device
+# of 1 with a maximum length of `max_length` on N GPUs.
+# Upon setting the sequence parallelism dimension to `SP`,
+# the accumulative counts have to be adjusted to `SP` times the original value.
+# This modification is essential to assure training equivalence,
+# as the sequence of `max_length` length will be segmented into `SP` parts,
+# with each part being allocated to its respective GPU among the `SP` GPUs
+# for parallelized training.
+# bs = 8 gpus * 1 batch_size_per_device * 8 acc / 8 sequence parallel
+accumulative_counts = 8
+dataloader_num_workers = 4
+max_epochs = 3
+optim_type = AdamW
+lr = 2e-5
+betas = (0.9, 0.999)
+weight_decay = 0
+max_norm = 1 # grad clip
+warmup_ratio = 0.03
+log_interval = 1
+
+# Save
+save_steps = -1 # speed only
+save_total_limit = 2 # Maximum checkpoints to keep (-1 means unlimited)
+
+# Evaluate the generation performance during the training
+evaluation_freq = 50
+SYSTEM = SYSTEM_TEMPLATE.alpaca
+evaluation_inputs = [
+ '请给我介绍五个上海的景点', 'Please tell me five scenic spots in Shanghai'
+]
+
+#######################################################################
+# PART 2 Model & Tokenizer #
+#######################################################################
+tokenizer = dict(
+ type=AutoTokenizer.from_pretrained,
+ pretrained_model_name_or_path=pretrained_model_name_or_path,
+ trust_remote_code=True,
+ padding_side='right')
+
+model = dict(
+ type=SupervisedFinetune,
+ use_varlen_attn=use_varlen_attn,
+ llm=dict(
+ type=AutoModelForCausalLM.from_pretrained,
+ pretrained_model_name_or_path=pretrained_model_name_or_path,
+ trust_remote_code=True))
+
+#######################################################################
+# PART 3 Dataset & Dataloader #
+#######################################################################
+alpaca_en = dict(
+ type=process_hf_dataset,
+ dataset=dict(type=load_dataset, path=alpaca_en_path),
+ tokenizer=tokenizer,
+ max_length=max_length,
+ dataset_map_fn=alpaca_map_fn,
+ template_map_fn=dict(
+ type=template_map_fn_factory, template=prompt_template),
+ remove_unused_columns=True,
+ shuffle_before_pack=True,
+ pack_to_max_length=pack_to_max_length,
+ use_varlen_attn=use_varlen_attn)
+
+alpaca_zh = dict(
+ type=process_hf_dataset,
+ dataset=dict(type=load_dataset, path=alpaca_zh_path),
+ tokenizer=tokenizer,
+ max_length=max_length,
+ dataset_map_fn=alpaca_zh_map_fn,
+ template_map_fn=dict(
+ type=template_map_fn_factory, template=prompt_template),
+ remove_unused_columns=True,
+ shuffle_before_pack=True,
+ pack_to_max_length=pack_to_max_length,
+ use_varlen_attn=use_varlen_attn)
+
+train_dataset = dict(type=ConcatDataset, datasets=[alpaca_en, alpaca_zh])
+
+train_dataloader = dict(
+ batch_size=batch_size,
+ num_workers=dataloader_num_workers,
+ dataset=train_dataset,
+ sampler=dict(type=SequenceParallelSampler, seed=1024),
+ collate_fn=dict(type=default_collate_fn, use_varlen_attn=use_varlen_attn))
+
+#######################################################################
+# PART 4 Scheduler & Optimizer #
+#######################################################################
+# optimizer
+optim_wrapper = dict(
+ type=AmpOptimWrapper,
+ optimizer=dict(
+ type=optim_type, lr=lr, betas=betas, weight_decay=weight_decay),
+ clip_grad=dict(max_norm=max_norm, error_if_nonfinite=False),
+ accumulative_counts=accumulative_counts,
+ loss_scale='dynamic',
+ dtype='float16')
+
+# learning policy
+# More information: https://github.com/open-mmlab/mmengine/blob/main/docs/en/tutorials/param_scheduler.md # noqa: E501
+param_scheduler = [
+ dict(
+ type=LinearLR,
+ start_factor=1e-5,
+ by_epoch=True,
+ begin=0,
+ end=warmup_ratio * max_epochs,
+ convert_to_iter_based=True),
+ dict(
+ type=CosineAnnealingLR,
+ eta_min=0.0,
+ by_epoch=True,
+ begin=warmup_ratio * max_epochs,
+ end=max_epochs,
+ convert_to_iter_based=True)
+]
+
+# train, val, test setting
+train_cfg = dict(type=TrainLoop, max_epochs=max_epochs)
+
+#######################################################################
+# PART 5 Runtime #
+#######################################################################
+# Log the dialogue periodically during the training process, optional
+custom_hooks = [dict(type=ThroughputHook)]
+
+if use_varlen_attn:
+ custom_hooks += [dict(type=VarlenAttnArgsToMessageHubHook)]
+
+# configure default hooks
+default_hooks = dict(
+ # record the time of every iteration.
+ timer=dict(type=IterTimerHook),
+ # print log every 10 iterations.
+ logger=dict(
+ type=LoggerHook, log_metric_by_epoch=False, interval=log_interval),
+ # enable the parameter scheduler.
+ param_scheduler=dict(type=ParamSchedulerHook),
+ # save checkpoint per `save_steps`.
+ checkpoint=dict(
+ type=CheckpointHook,
+ by_epoch=False,
+ interval=-1,
+ save_last=False,
+ max_keep_ckpts=save_total_limit),
+ # set sampler seed in distributed evrionment.
+ sampler_seed=dict(type=DistSamplerSeedHook),
+)
+
+# configure environment
+env_cfg = dict(
+ # whether to enable cudnn benchmark
+ cudnn_benchmark=False,
+ # set multi process parameters
+ mp_cfg=dict(mp_start_method='fork', opencv_num_threads=0),
+ # set distributed parameters
+ dist_cfg=dict(backend='nccl'),
+)
+
+# set visualizer
+visualizer = None
+
+# set log level
+log_level = 'INFO'
+
+# load from which checkpoint
+load_from = None
+
+# whether to resume training from the loaded checkpoint
+resume = False
+
+# Defaults to use random seed and disable `deterministic`
+randomness = dict(seed=None, deterministic=False)
+
+# set log processor
+log_processor = dict(by_epoch=False, window_size=log_interval)
diff --git a/xtuner/configs/llama_speed_benchmark/llama2_7b/llama2_7b_full_alpaca_enzh_1M_sp16.py b/xtuner/configs/llama_speed_benchmark/llama2_7b/llama2_7b_full_alpaca_enzh_1M_sp16.py
new file mode 100644
index 000000000..7827c9dfb
--- /dev/null
+++ b/xtuner/configs/llama_speed_benchmark/llama2_7b/llama2_7b_full_alpaca_enzh_1M_sp16.py
@@ -0,0 +1,212 @@
+# Copyright (c) OpenMMLab. All rights reserved.
+from datasets import load_dataset
+from mmengine.hooks import (CheckpointHook, DistSamplerSeedHook, IterTimerHook,
+ LoggerHook, ParamSchedulerHook)
+from mmengine.optim import AmpOptimWrapper, CosineAnnealingLR, LinearLR
+from torch.optim import AdamW
+from transformers import AutoModelForCausalLM, AutoTokenizer
+
+from xtuner.dataset import ConcatDataset, process_hf_dataset
+from xtuner.dataset.collate_fns import default_collate_fn
+from xtuner.dataset.map_fns import (alpaca_map_fn, alpaca_zh_map_fn,
+ template_map_fn_factory)
+from xtuner.engine.hooks import ThroughputHook, VarlenAttnArgsToMessageHubHook
+from xtuner.engine.runner import TrainLoop
+from xtuner.model import SupervisedFinetune
+from xtuner.parallel.sequence import SequenceParallelSampler
+from xtuner.utils import PROMPT_TEMPLATE, SYSTEM_TEMPLATE
+
+#######################################################################
+# PART 1 Settings #
+#######################################################################
+# Model
+pretrained_model_name_or_path = 'meta-llama/Llama-2-7b'
+use_varlen_attn = False
+sequence_parallel_size = 16
+
+# Data
+alpaca_zh_path = 'silk-road/alpaca-data-gpt4-chinese'
+alpaca_en_path = 'tatsu-lab/alpaca'
+prompt_template = PROMPT_TEMPLATE.llama2_chat
+max_length = 1048576 # 1M
+pack_to_max_length = True
+
+# Scheduler & Optimizer
+batch_size = 1 # per_device
+# Suppose I aim to employ a training strategy using a batch size per device
+# of 1 with a maximum length of `max_length` on N GPUs.
+# Upon setting the sequence parallelism dimension to `SP`,
+# the accumulative counts have to be adjusted to `SP` times the original value.
+# This modification is essential to assure training equivalence,
+# as the sequence of `max_length` length will be segmented into `SP` parts,
+# with each part being allocated to its respective GPU among the `SP` GPUs
+# for parallelized training.
+# bs = 32 gpus * 1 batch_size_per_device * 16 acc / 16 sequence parallel
+accumulative_counts = 16
+dataloader_num_workers = 4
+max_epochs = 3
+optim_type = AdamW
+lr = 2e-5
+betas = (0.9, 0.999)
+weight_decay = 0
+max_norm = 1 # grad clip
+warmup_ratio = 0.03
+log_interval = 1
+
+# Save
+save_steps = -1 # speed only
+save_total_limit = 2 # Maximum checkpoints to keep (-1 means unlimited)
+
+# Evaluate the generation performance during the training
+evaluation_freq = 50
+SYSTEM = SYSTEM_TEMPLATE.alpaca
+evaluation_inputs = [
+ '请给我介绍五个上海的景点', 'Please tell me five scenic spots in Shanghai'
+]
+
+#######################################################################
+# PART 2 Model & Tokenizer #
+#######################################################################
+tokenizer = dict(
+ type=AutoTokenizer.from_pretrained,
+ pretrained_model_name_or_path=pretrained_model_name_or_path,
+ trust_remote_code=True,
+ padding_side='right')
+
+model = dict(
+ type=SupervisedFinetune,
+ use_varlen_attn=use_varlen_attn,
+ llm=dict(
+ type=AutoModelForCausalLM.from_pretrained,
+ pretrained_model_name_or_path=pretrained_model_name_or_path,
+ trust_remote_code=True))
+
+#######################################################################
+# PART 3 Dataset & Dataloader #
+#######################################################################
+alpaca_en = dict(
+ type=process_hf_dataset,
+ dataset=dict(type=load_dataset, path=alpaca_en_path),
+ tokenizer=tokenizer,
+ max_length=max_length,
+ dataset_map_fn=alpaca_map_fn,
+ template_map_fn=dict(
+ type=template_map_fn_factory, template=prompt_template),
+ remove_unused_columns=True,
+ shuffle_before_pack=True,
+ pack_to_max_length=pack_to_max_length,
+ use_varlen_attn=use_varlen_attn)
+
+alpaca_zh = dict(
+ type=process_hf_dataset,
+ dataset=dict(type=load_dataset, path=alpaca_zh_path),
+ tokenizer=tokenizer,
+ max_length=max_length,
+ dataset_map_fn=alpaca_zh_map_fn,
+ template_map_fn=dict(
+ type=template_map_fn_factory, template=prompt_template),
+ remove_unused_columns=True,
+ shuffle_before_pack=True,
+ pack_to_max_length=pack_to_max_length,
+ use_varlen_attn=use_varlen_attn)
+
+train_dataset = dict(type=ConcatDataset, datasets=[alpaca_en, alpaca_zh])
+
+train_dataloader = dict(
+ batch_size=batch_size,
+ num_workers=dataloader_num_workers,
+ dataset=train_dataset,
+ sampler=dict(type=SequenceParallelSampler, seed=1024),
+ collate_fn=dict(type=default_collate_fn, use_varlen_attn=use_varlen_attn))
+
+#######################################################################
+# PART 4 Scheduler & Optimizer #
+#######################################################################
+# optimizer
+optim_wrapper = dict(
+ type=AmpOptimWrapper,
+ optimizer=dict(
+ type=optim_type, lr=lr, betas=betas, weight_decay=weight_decay),
+ clip_grad=dict(max_norm=max_norm, error_if_nonfinite=False),
+ accumulative_counts=accumulative_counts,
+ loss_scale='dynamic',
+ dtype='float16')
+
+# learning policy
+# More information: https://github.com/open-mmlab/mmengine/blob/main/docs/en/tutorials/param_scheduler.md # noqa: E501
+param_scheduler = [
+ dict(
+ type=LinearLR,
+ start_factor=1e-5,
+ by_epoch=True,
+ begin=0,
+ end=warmup_ratio * max_epochs,
+ convert_to_iter_based=True),
+ dict(
+ type=CosineAnnealingLR,
+ eta_min=0.0,
+ by_epoch=True,
+ begin=warmup_ratio * max_epochs,
+ end=max_epochs,
+ convert_to_iter_based=True)
+]
+
+# train, val, test setting
+train_cfg = dict(type=TrainLoop, max_epochs=max_epochs)
+
+#######################################################################
+# PART 5 Runtime #
+#######################################################################
+# Log the dialogue periodically during the training process, optional
+custom_hooks = [dict(type=ThroughputHook)]
+
+if use_varlen_attn:
+ custom_hooks += [dict(type=VarlenAttnArgsToMessageHubHook)]
+
+# configure default hooks
+default_hooks = dict(
+ # record the time of every iteration.
+ timer=dict(type=IterTimerHook),
+ # print log every 10 iterations.
+ logger=dict(
+ type=LoggerHook, log_metric_by_epoch=False, interval=log_interval),
+ # enable the parameter scheduler.
+ param_scheduler=dict(type=ParamSchedulerHook),
+ # save checkpoint per `save_steps`.
+ checkpoint=dict(
+ type=CheckpointHook,
+ by_epoch=False,
+ interval=-1,
+ save_last=False,
+ max_keep_ckpts=save_total_limit),
+ # set sampler seed in distributed evrionment.
+ sampler_seed=dict(type=DistSamplerSeedHook),
+)
+
+# configure environment
+env_cfg = dict(
+ # whether to enable cudnn benchmark
+ cudnn_benchmark=False,
+ # set multi process parameters
+ mp_cfg=dict(mp_start_method='fork', opencv_num_threads=0),
+ # set distributed parameters
+ dist_cfg=dict(backend='nccl'),
+)
+
+# set visualizer
+visualizer = None
+
+# set log level
+log_level = 'INFO'
+
+# load from which checkpoint
+load_from = None
+
+# whether to resume training from the loaded checkpoint
+resume = False
+
+# Defaults to use random seed and disable `deterministic`
+randomness = dict(seed=None, deterministic=False)
+
+# set log processor
+log_processor = dict(by_epoch=False, window_size=log_interval)
diff --git a/xtuner/configs/llama_speed_benchmark/llama2_7b/llama2_7b_full_alpaca_enzh_256k_sp8.py b/xtuner/configs/llama_speed_benchmark/llama2_7b/llama2_7b_full_alpaca_enzh_256k_sp8.py
new file mode 100644
index 000000000..ba0c94bb6
--- /dev/null
+++ b/xtuner/configs/llama_speed_benchmark/llama2_7b/llama2_7b_full_alpaca_enzh_256k_sp8.py
@@ -0,0 +1,212 @@
+# Copyright (c) OpenMMLab. All rights reserved.
+from datasets import load_dataset
+from mmengine.hooks import (CheckpointHook, DistSamplerSeedHook, IterTimerHook,
+ LoggerHook, ParamSchedulerHook)
+from mmengine.optim import AmpOptimWrapper, CosineAnnealingLR, LinearLR
+from torch.optim import AdamW
+from transformers import AutoModelForCausalLM, AutoTokenizer
+
+from xtuner.dataset import ConcatDataset, process_hf_dataset
+from xtuner.dataset.collate_fns import default_collate_fn
+from xtuner.dataset.map_fns import (alpaca_map_fn, alpaca_zh_map_fn,
+ template_map_fn_factory)
+from xtuner.engine.hooks import ThroughputHook, VarlenAttnArgsToMessageHubHook
+from xtuner.engine.runner import TrainLoop
+from xtuner.model import SupervisedFinetune
+from xtuner.parallel.sequence import SequenceParallelSampler
+from xtuner.utils import PROMPT_TEMPLATE, SYSTEM_TEMPLATE
+
+#######################################################################
+# PART 1 Settings #
+#######################################################################
+# Model
+pretrained_model_name_or_path = 'meta-llama/Llama-2-7b'
+use_varlen_attn = False
+sequence_parallel_size = 8
+
+# Data
+alpaca_zh_path = 'silk-road/alpaca-data-gpt4-chinese'
+alpaca_en_path = 'tatsu-lab/alpaca'
+prompt_template = PROMPT_TEMPLATE.llama2_chat
+max_length = 262144 # 256k
+pack_to_max_length = True
+
+# Scheduler & Optimizer
+batch_size = 1 # per_device
+# Suppose I aim to employ a training strategy using a batch size per device
+# of 1 with a maximum length of `max_length` on N GPUs.
+# Upon setting the sequence parallelism dimension to `SP`,
+# the accumulative counts have to be adjusted to `SP` times the original value.
+# This modification is essential to assure training equivalence,
+# as the sequence of `max_length` length will be segmented into `SP` parts,
+# with each part being allocated to its respective GPU among the `SP` GPUs
+# for parallelized training.
+# bs = 8 gpus * 1 batch_size_per_device * 8 acc / 8 sequence parallel
+accumulative_counts = 8
+dataloader_num_workers = 4
+max_epochs = 3
+optim_type = AdamW
+lr = 2e-5
+betas = (0.9, 0.999)
+weight_decay = 0
+max_norm = 1 # grad clip
+warmup_ratio = 0.03
+log_interval = 1
+
+# Save
+save_steps = -1 # speed only
+save_total_limit = 2 # Maximum checkpoints to keep (-1 means unlimited)
+
+# Evaluate the generation performance during the training
+evaluation_freq = 50
+SYSTEM = SYSTEM_TEMPLATE.alpaca
+evaluation_inputs = [
+ '请给我介绍五个上海的景点', 'Please tell me five scenic spots in Shanghai'
+]
+
+#######################################################################
+# PART 2 Model & Tokenizer #
+#######################################################################
+tokenizer = dict(
+ type=AutoTokenizer.from_pretrained,
+ pretrained_model_name_or_path=pretrained_model_name_or_path,
+ trust_remote_code=True,
+ padding_side='right')
+
+model = dict(
+ type=SupervisedFinetune,
+ use_varlen_attn=use_varlen_attn,
+ llm=dict(
+ type=AutoModelForCausalLM.from_pretrained,
+ pretrained_model_name_or_path=pretrained_model_name_or_path,
+ trust_remote_code=True))
+
+#######################################################################
+# PART 3 Dataset & Dataloader #
+#######################################################################
+alpaca_en = dict(
+ type=process_hf_dataset,
+ dataset=dict(type=load_dataset, path=alpaca_en_path),
+ tokenizer=tokenizer,
+ max_length=max_length,
+ dataset_map_fn=alpaca_map_fn,
+ template_map_fn=dict(
+ type=template_map_fn_factory, template=prompt_template),
+ remove_unused_columns=True,
+ shuffle_before_pack=True,
+ pack_to_max_length=pack_to_max_length,
+ use_varlen_attn=use_varlen_attn)
+
+alpaca_zh = dict(
+ type=process_hf_dataset,
+ dataset=dict(type=load_dataset, path=alpaca_zh_path),
+ tokenizer=tokenizer,
+ max_length=max_length,
+ dataset_map_fn=alpaca_zh_map_fn,
+ template_map_fn=dict(
+ type=template_map_fn_factory, template=prompt_template),
+ remove_unused_columns=True,
+ shuffle_before_pack=True,
+ pack_to_max_length=pack_to_max_length,
+ use_varlen_attn=use_varlen_attn)
+
+train_dataset = dict(type=ConcatDataset, datasets=[alpaca_en, alpaca_zh])
+
+train_dataloader = dict(
+ batch_size=batch_size,
+ num_workers=dataloader_num_workers,
+ dataset=train_dataset,
+ sampler=dict(type=SequenceParallelSampler, seed=1024),
+ collate_fn=dict(type=default_collate_fn, use_varlen_attn=use_varlen_attn))
+
+#######################################################################
+# PART 4 Scheduler & Optimizer #
+#######################################################################
+# optimizer
+optim_wrapper = dict(
+ type=AmpOptimWrapper,
+ optimizer=dict(
+ type=optim_type, lr=lr, betas=betas, weight_decay=weight_decay),
+ clip_grad=dict(max_norm=max_norm, error_if_nonfinite=False),
+ accumulative_counts=accumulative_counts,
+ loss_scale='dynamic',
+ dtype='float16')
+
+# learning policy
+# More information: https://github.com/open-mmlab/mmengine/blob/main/docs/en/tutorials/param_scheduler.md # noqa: E501
+param_scheduler = [
+ dict(
+ type=LinearLR,
+ start_factor=1e-5,
+ by_epoch=True,
+ begin=0,
+ end=warmup_ratio * max_epochs,
+ convert_to_iter_based=True),
+ dict(
+ type=CosineAnnealingLR,
+ eta_min=0.0,
+ by_epoch=True,
+ begin=warmup_ratio * max_epochs,
+ end=max_epochs,
+ convert_to_iter_based=True)
+]
+
+# train, val, test setting
+train_cfg = dict(type=TrainLoop, max_epochs=max_epochs)
+
+#######################################################################
+# PART 5 Runtime #
+#######################################################################
+# Log the dialogue periodically during the training process, optional
+custom_hooks = [dict(type=ThroughputHook)]
+
+if use_varlen_attn:
+ custom_hooks += [dict(type=VarlenAttnArgsToMessageHubHook)]
+
+# configure default hooks
+default_hooks = dict(
+ # record the time of every iteration.
+ timer=dict(type=IterTimerHook),
+ # print log every 10 iterations.
+ logger=dict(
+ type=LoggerHook, log_metric_by_epoch=False, interval=log_interval),
+ # enable the parameter scheduler.
+ param_scheduler=dict(type=ParamSchedulerHook),
+ # save checkpoint per `save_steps`.
+ checkpoint=dict(
+ type=CheckpointHook,
+ by_epoch=False,
+ interval=-1,
+ save_last=False,
+ max_keep_ckpts=save_total_limit),
+ # set sampler seed in distributed evrionment.
+ sampler_seed=dict(type=DistSamplerSeedHook),
+)
+
+# configure environment
+env_cfg = dict(
+ # whether to enable cudnn benchmark
+ cudnn_benchmark=False,
+ # set multi process parameters
+ mp_cfg=dict(mp_start_method='fork', opencv_num_threads=0),
+ # set distributed parameters
+ dist_cfg=dict(backend='nccl'),
+)
+
+# set visualizer
+visualizer = None
+
+# set log level
+log_level = 'INFO'
+
+# load from which checkpoint
+load_from = None
+
+# whether to resume training from the loaded checkpoint
+resume = False
+
+# Defaults to use random seed and disable `deterministic`
+randomness = dict(seed=None, deterministic=False)
+
+# set log processor
+log_processor = dict(by_epoch=False, window_size=log_interval)
diff --git a/xtuner/configs/llama_speed_benchmark/llama2_7b/llama2_7b_full_alpaca_enzh_32k_sp1.py b/xtuner/configs/llama_speed_benchmark/llama2_7b/llama2_7b_full_alpaca_enzh_32k_sp1.py
new file mode 100644
index 000000000..b871ce6f5
--- /dev/null
+++ b/xtuner/configs/llama_speed_benchmark/llama2_7b/llama2_7b_full_alpaca_enzh_32k_sp1.py
@@ -0,0 +1,212 @@
+# Copyright (c) OpenMMLab. All rights reserved.
+from datasets import load_dataset
+from mmengine.hooks import (CheckpointHook, DistSamplerSeedHook, IterTimerHook,
+ LoggerHook, ParamSchedulerHook)
+from mmengine.optim import AmpOptimWrapper, CosineAnnealingLR, LinearLR
+from torch.optim import AdamW
+from transformers import AutoModelForCausalLM, AutoTokenizer
+
+from xtuner.dataset import ConcatDataset, process_hf_dataset
+from xtuner.dataset.collate_fns import default_collate_fn
+from xtuner.dataset.map_fns import (alpaca_map_fn, alpaca_zh_map_fn,
+ template_map_fn_factory)
+from xtuner.engine.hooks import ThroughputHook, VarlenAttnArgsToMessageHubHook
+from xtuner.engine.runner import TrainLoop
+from xtuner.model import SupervisedFinetune
+from xtuner.parallel.sequence import SequenceParallelSampler
+from xtuner.utils import PROMPT_TEMPLATE, SYSTEM_TEMPLATE
+
+#######################################################################
+# PART 1 Settings #
+#######################################################################
+# Model
+pretrained_model_name_or_path = 'meta-llama/Llama-2-7b'
+use_varlen_attn = False
+sequence_parallel_size = 1
+
+# Data
+alpaca_zh_path = 'silk-road/alpaca-data-gpt4-chinese'
+alpaca_en_path = 'tatsu-lab/alpaca'
+prompt_template = PROMPT_TEMPLATE.llama2_chat
+max_length = 32768
+pack_to_max_length = True
+
+# Scheduler & Optimizer
+batch_size = 1 # per_device
+# Suppose I aim to employ a training strategy using a batch size per device
+# of 1 with a maximum length of `max_length` on N GPUs.
+# Upon setting the sequence parallelism dimension to `SP`,
+# the accumulative counts have to be adjusted to `SP` times the original value.
+# This modification is essential to assure training equivalence,
+# as the sequence of `max_length` length will be segmented into `SP` parts,
+# with each part being allocated to its respective GPU among the `SP` GPUs
+# for parallelized training.
+# bs = 8 gpus * 1 batch_size_per_device * 1 acc / 1 sequence parallel
+accumulative_counts = 1
+dataloader_num_workers = 4
+max_epochs = 3
+optim_type = AdamW
+lr = 2e-5
+betas = (0.9, 0.999)
+weight_decay = 0
+max_norm = 1 # grad clip
+warmup_ratio = 0.03
+log_interval = 1
+
+# Save
+save_steps = -1 # speed only
+save_total_limit = 2 # Maximum checkpoints to keep (-1 means unlimited)
+
+# Evaluate the generation performance during the training
+evaluation_freq = 50
+SYSTEM = SYSTEM_TEMPLATE.alpaca
+evaluation_inputs = [
+ '请给我介绍五个上海的景点', 'Please tell me five scenic spots in Shanghai'
+]
+
+#######################################################################
+# PART 2 Model & Tokenizer #
+#######################################################################
+tokenizer = dict(
+ type=AutoTokenizer.from_pretrained,
+ pretrained_model_name_or_path=pretrained_model_name_or_path,
+ trust_remote_code=True,
+ padding_side='right')
+
+model = dict(
+ type=SupervisedFinetune,
+ use_varlen_attn=use_varlen_attn,
+ llm=dict(
+ type=AutoModelForCausalLM.from_pretrained,
+ pretrained_model_name_or_path=pretrained_model_name_or_path,
+ trust_remote_code=True))
+
+#######################################################################
+# PART 3 Dataset & Dataloader #
+#######################################################################
+alpaca_en = dict(
+ type=process_hf_dataset,
+ dataset=dict(type=load_dataset, path=alpaca_en_path),
+ tokenizer=tokenizer,
+ max_length=max_length,
+ dataset_map_fn=alpaca_map_fn,
+ template_map_fn=dict(
+ type=template_map_fn_factory, template=prompt_template),
+ remove_unused_columns=True,
+ shuffle_before_pack=True,
+ pack_to_max_length=pack_to_max_length,
+ use_varlen_attn=use_varlen_attn)
+
+alpaca_zh = dict(
+ type=process_hf_dataset,
+ dataset=dict(type=load_dataset, path=alpaca_zh_path),
+ tokenizer=tokenizer,
+ max_length=max_length,
+ dataset_map_fn=alpaca_zh_map_fn,
+ template_map_fn=dict(
+ type=template_map_fn_factory, template=prompt_template),
+ remove_unused_columns=True,
+ shuffle_before_pack=True,
+ pack_to_max_length=pack_to_max_length,
+ use_varlen_attn=use_varlen_attn)
+
+train_dataset = dict(type=ConcatDataset, datasets=[alpaca_en, alpaca_zh])
+
+train_dataloader = dict(
+ batch_size=batch_size,
+ num_workers=dataloader_num_workers,
+ dataset=train_dataset,
+ sampler=dict(type=SequenceParallelSampler, seed=1024),
+ collate_fn=dict(type=default_collate_fn, use_varlen_attn=use_varlen_attn))
+
+#######################################################################
+# PART 4 Scheduler & Optimizer #
+#######################################################################
+# optimizer
+optim_wrapper = dict(
+ type=AmpOptimWrapper,
+ optimizer=dict(
+ type=optim_type, lr=lr, betas=betas, weight_decay=weight_decay),
+ clip_grad=dict(max_norm=max_norm, error_if_nonfinite=False),
+ accumulative_counts=accumulative_counts,
+ loss_scale='dynamic',
+ dtype='float16')
+
+# learning policy
+# More information: https://github.com/open-mmlab/mmengine/blob/main/docs/en/tutorials/param_scheduler.md # noqa: E501
+param_scheduler = [
+ dict(
+ type=LinearLR,
+ start_factor=1e-5,
+ by_epoch=True,
+ begin=0,
+ end=warmup_ratio * max_epochs,
+ convert_to_iter_based=True),
+ dict(
+ type=CosineAnnealingLR,
+ eta_min=0.0,
+ by_epoch=True,
+ begin=warmup_ratio * max_epochs,
+ end=max_epochs,
+ convert_to_iter_based=True)
+]
+
+# train, val, test setting
+train_cfg = dict(type=TrainLoop, max_epochs=max_epochs)
+
+#######################################################################
+# PART 5 Runtime #
+#######################################################################
+# Log the dialogue periodically during the training process, optional
+custom_hooks = [dict(type=ThroughputHook)]
+
+if use_varlen_attn:
+ custom_hooks += [dict(type=VarlenAttnArgsToMessageHubHook)]
+
+# configure default hooks
+default_hooks = dict(
+ # record the time of every iteration.
+ timer=dict(type=IterTimerHook),
+ # print log every 10 iterations.
+ logger=dict(
+ type=LoggerHook, log_metric_by_epoch=False, interval=log_interval),
+ # enable the parameter scheduler.
+ param_scheduler=dict(type=ParamSchedulerHook),
+ # save checkpoint per `save_steps`.
+ checkpoint=dict(
+ type=CheckpointHook,
+ by_epoch=False,
+ interval=-1,
+ save_last=False,
+ max_keep_ckpts=save_total_limit),
+ # set sampler seed in distributed evrionment.
+ sampler_seed=dict(type=DistSamplerSeedHook),
+)
+
+# configure environment
+env_cfg = dict(
+ # whether to enable cudnn benchmark
+ cudnn_benchmark=False,
+ # set multi process parameters
+ mp_cfg=dict(mp_start_method='fork', opencv_num_threads=0),
+ # set distributed parameters
+ dist_cfg=dict(backend='nccl'),
+)
+
+# set visualizer
+visualizer = None
+
+# set log level
+log_level = 'INFO'
+
+# load from which checkpoint
+load_from = None
+
+# whether to resume training from the loaded checkpoint
+resume = False
+
+# Defaults to use random seed and disable `deterministic`
+randomness = dict(seed=None, deterministic=False)
+
+# set log processor
+log_processor = dict(by_epoch=False, window_size=log_interval)
diff --git a/xtuner/configs/llama_speed_benchmark/llama2_7b/llama2_7b_full_alpaca_enzh_8k_sp1.py b/xtuner/configs/llama_speed_benchmark/llama2_7b/llama2_7b_full_alpaca_enzh_8k_sp1.py
new file mode 100644
index 000000000..d6178015b
--- /dev/null
+++ b/xtuner/configs/llama_speed_benchmark/llama2_7b/llama2_7b_full_alpaca_enzh_8k_sp1.py
@@ -0,0 +1,212 @@
+# Copyright (c) OpenMMLab. All rights reserved.
+from datasets import load_dataset
+from mmengine.hooks import (CheckpointHook, DistSamplerSeedHook, IterTimerHook,
+ LoggerHook, ParamSchedulerHook)
+from mmengine.optim import AmpOptimWrapper, CosineAnnealingLR, LinearLR
+from torch.optim import AdamW
+from transformers import AutoModelForCausalLM, AutoTokenizer
+
+from xtuner.dataset import ConcatDataset, process_hf_dataset
+from xtuner.dataset.collate_fns import default_collate_fn
+from xtuner.dataset.map_fns import (alpaca_map_fn, alpaca_zh_map_fn,
+ template_map_fn_factory)
+from xtuner.engine.hooks import ThroughputHook, VarlenAttnArgsToMessageHubHook
+from xtuner.engine.runner import TrainLoop
+from xtuner.model import SupervisedFinetune
+from xtuner.parallel.sequence import SequenceParallelSampler
+from xtuner.utils import PROMPT_TEMPLATE, SYSTEM_TEMPLATE
+
+#######################################################################
+# PART 1 Settings #
+#######################################################################
+# Model
+pretrained_model_name_or_path = 'meta-llama/Llama-2-7b'
+use_varlen_attn = False
+sequence_parallel_size = 1
+
+# Data
+alpaca_zh_path = 'silk-road/alpaca-data-gpt4-chinese'
+alpaca_en_path = 'tatsu-lab/alpaca'
+prompt_template = PROMPT_TEMPLATE.llama2_chat
+max_length = 8192
+pack_to_max_length = True
+
+# Scheduler & Optimizer
+batch_size = 1 # per_device
+# Suppose I aim to employ a training strategy using a batch size per device
+# of 1 with a maximum length of `max_length` on N GPUs.
+# Upon setting the sequence parallelism dimension to `SP`,
+# the accumulative counts have to be adjusted to `SP` times the original value.
+# This modification is essential to assure training equivalence,
+# as the sequence of `max_length` length will be segmented into `SP` parts,
+# with each part being allocated to its respective GPU among the `SP` GPUs
+# for parallelized training.
+# bs = 8 gpus * 1 batch_size_per_device * 1 acc / 1 sequence parallel
+accumulative_counts = 1
+dataloader_num_workers = 4
+max_epochs = 3
+optim_type = AdamW
+lr = 2e-5
+betas = (0.9, 0.999)
+weight_decay = 0
+max_norm = 1 # grad clip
+warmup_ratio = 0.03
+log_interval = 1
+
+# Save
+save_steps = -1 # speed only
+save_total_limit = 2 # Maximum checkpoints to keep (-1 means unlimited)
+
+# Evaluate the generation performance during the training
+evaluation_freq = 50
+SYSTEM = SYSTEM_TEMPLATE.alpaca
+evaluation_inputs = [
+ '请给我介绍五个上海的景点', 'Please tell me five scenic spots in Shanghai'
+]
+
+#######################################################################
+# PART 2 Model & Tokenizer #
+#######################################################################
+tokenizer = dict(
+ type=AutoTokenizer.from_pretrained,
+ pretrained_model_name_or_path=pretrained_model_name_or_path,
+ trust_remote_code=True,
+ padding_side='right')
+
+model = dict(
+ type=SupervisedFinetune,
+ use_varlen_attn=use_varlen_attn,
+ llm=dict(
+ type=AutoModelForCausalLM.from_pretrained,
+ pretrained_model_name_or_path=pretrained_model_name_or_path,
+ trust_remote_code=True))
+
+#######################################################################
+# PART 3 Dataset & Dataloader #
+#######################################################################
+alpaca_en = dict(
+ type=process_hf_dataset,
+ dataset=dict(type=load_dataset, path=alpaca_en_path),
+ tokenizer=tokenizer,
+ max_length=max_length,
+ dataset_map_fn=alpaca_map_fn,
+ template_map_fn=dict(
+ type=template_map_fn_factory, template=prompt_template),
+ remove_unused_columns=True,
+ shuffle_before_pack=True,
+ pack_to_max_length=pack_to_max_length,
+ use_varlen_attn=use_varlen_attn)
+
+alpaca_zh = dict(
+ type=process_hf_dataset,
+ dataset=dict(type=load_dataset, path=alpaca_zh_path),
+ tokenizer=tokenizer,
+ max_length=max_length,
+ dataset_map_fn=alpaca_zh_map_fn,
+ template_map_fn=dict(
+ type=template_map_fn_factory, template=prompt_template),
+ remove_unused_columns=True,
+ shuffle_before_pack=True,
+ pack_to_max_length=pack_to_max_length,
+ use_varlen_attn=use_varlen_attn)
+
+train_dataset = dict(type=ConcatDataset, datasets=[alpaca_en, alpaca_zh])
+
+train_dataloader = dict(
+ batch_size=batch_size,
+ num_workers=dataloader_num_workers,
+ dataset=train_dataset,
+ sampler=dict(type=SequenceParallelSampler, seed=1024),
+ collate_fn=dict(type=default_collate_fn, use_varlen_attn=use_varlen_attn))
+
+#######################################################################
+# PART 4 Scheduler & Optimizer #
+#######################################################################
+# optimizer
+optim_wrapper = dict(
+ type=AmpOptimWrapper,
+ optimizer=dict(
+ type=optim_type, lr=lr, betas=betas, weight_decay=weight_decay),
+ clip_grad=dict(max_norm=max_norm, error_if_nonfinite=False),
+ accumulative_counts=accumulative_counts,
+ loss_scale='dynamic',
+ dtype='float16')
+
+# learning policy
+# More information: https://github.com/open-mmlab/mmengine/blob/main/docs/en/tutorials/param_scheduler.md # noqa: E501
+param_scheduler = [
+ dict(
+ type=LinearLR,
+ start_factor=1e-5,
+ by_epoch=True,
+ begin=0,
+ end=warmup_ratio * max_epochs,
+ convert_to_iter_based=True),
+ dict(
+ type=CosineAnnealingLR,
+ eta_min=0.0,
+ by_epoch=True,
+ begin=warmup_ratio * max_epochs,
+ end=max_epochs,
+ convert_to_iter_based=True)
+]
+
+# train, val, test setting
+train_cfg = dict(type=TrainLoop, max_epochs=max_epochs)
+
+#######################################################################
+# PART 5 Runtime #
+#######################################################################
+# Log the dialogue periodically during the training process, optional
+custom_hooks = [dict(type=ThroughputHook)]
+
+if use_varlen_attn:
+ custom_hooks += [dict(type=VarlenAttnArgsToMessageHubHook)]
+
+# configure default hooks
+default_hooks = dict(
+ # record the time of every iteration.
+ timer=dict(type=IterTimerHook),
+ # print log every 10 iterations.
+ logger=dict(
+ type=LoggerHook, log_metric_by_epoch=False, interval=log_interval),
+ # enable the parameter scheduler.
+ param_scheduler=dict(type=ParamSchedulerHook),
+ # save checkpoint per `save_steps`.
+ checkpoint=dict(
+ type=CheckpointHook,
+ by_epoch=False,
+ interval=-1,
+ save_last=False,
+ max_keep_ckpts=save_total_limit),
+ # set sampler seed in distributed evrionment.
+ sampler_seed=dict(type=DistSamplerSeedHook),
+)
+
+# configure environment
+env_cfg = dict(
+ # whether to enable cudnn benchmark
+ cudnn_benchmark=False,
+ # set multi process parameters
+ mp_cfg=dict(mp_start_method='fork', opencv_num_threads=0),
+ # set distributed parameters
+ dist_cfg=dict(backend='nccl'),
+)
+
+# set visualizer
+visualizer = None
+
+# set log level
+log_level = 'INFO'
+
+# load from which checkpoint
+load_from = None
+
+# whether to resume training from the loaded checkpoint
+resume = False
+
+# Defaults to use random seed and disable `deterministic`
+randomness = dict(seed=None, deterministic=False)
+
+# set log processor
+log_processor = dict(by_epoch=False, window_size=log_interval)
diff --git a/xtuner/configs/llama_speed_benchmark/yi_34b/yi_34b_200k_full_alpaca_enzh_128k_sp8.py b/xtuner/configs/llama_speed_benchmark/yi_34b/yi_34b_200k_full_alpaca_enzh_128k_sp8.py
new file mode 100644
index 000000000..60de99deb
--- /dev/null
+++ b/xtuner/configs/llama_speed_benchmark/yi_34b/yi_34b_200k_full_alpaca_enzh_128k_sp8.py
@@ -0,0 +1,212 @@
+# Copyright (c) OpenMMLab. All rights reserved.
+from datasets import load_dataset
+from mmengine.hooks import (CheckpointHook, DistSamplerSeedHook, IterTimerHook,
+ LoggerHook, ParamSchedulerHook)
+from mmengine.optim import AmpOptimWrapper, CosineAnnealingLR, LinearLR
+from torch.optim import AdamW
+from transformers import AutoModelForCausalLM, AutoTokenizer
+
+from xtuner.dataset import ConcatDataset, process_hf_dataset
+from xtuner.dataset.collate_fns import default_collate_fn
+from xtuner.dataset.map_fns import (alpaca_map_fn, alpaca_zh_map_fn,
+ template_map_fn_factory)
+from xtuner.engine.hooks import ThroughputHook, VarlenAttnArgsToMessageHubHook
+from xtuner.engine.runner import TrainLoop
+from xtuner.model import SupervisedFinetune
+from xtuner.parallel.sequence import SequenceParallelSampler
+from xtuner.utils import PROMPT_TEMPLATE, SYSTEM_TEMPLATE
+
+#######################################################################
+# PART 1 Settings #
+#######################################################################
+# Model
+pretrained_model_name_or_path = '01-ai/Yi-34B-200K'
+use_varlen_attn = False
+sequence_parallel_size = 8
+
+# Data
+alpaca_zh_path = 'silk-road/alpaca-data-gpt4-chinese'
+alpaca_en_path = 'tatsu-lab/alpaca'
+prompt_template = PROMPT_TEMPLATE.llama2_chat
+max_length = 131072 # 128k
+pack_to_max_length = True
+
+# Scheduler & Optimizer
+batch_size = 1 # per_device
+# Suppose I aim to employ a training strategy using a batch size per device
+# of 1 with a maximum length of `max_length` on N GPUs.
+# Upon setting the sequence parallelism dimension to `SP`,
+# the accumulative counts have to be adjusted to `SP` times the original value.
+# This modification is essential to assure training equivalence,
+# as the sequence of `max_length` length will be segmented into `SP` parts,
+# with each part being allocated to its respective GPU among the `SP` GPUs
+# for parallelized training.
+# bs = 32 gpus * 1 batch_size_per_device * 8 acc / 8 sequence parallel
+accumulative_counts = 8
+dataloader_num_workers = 4
+max_epochs = 3
+optim_type = AdamW
+lr = 2e-5
+betas = (0.9, 0.999)
+weight_decay = 0
+max_norm = 1 # grad clip
+warmup_ratio = 0.03
+log_interval = 1
+
+# Save
+save_steps = -1 # speed only
+save_total_limit = 2 # Maximum checkpoints to keep (-1 means unlimited)
+
+# Evaluate the generation performance during the training
+evaluation_freq = 50
+SYSTEM = SYSTEM_TEMPLATE.alpaca
+evaluation_inputs = [
+ '请给我介绍五个上海的景点', 'Please tell me five scenic spots in Shanghai'
+]
+
+#######################################################################
+# PART 2 Model & Tokenizer #
+#######################################################################
+tokenizer = dict(
+ type=AutoTokenizer.from_pretrained,
+ pretrained_model_name_or_path=pretrained_model_name_or_path,
+ trust_remote_code=True,
+ padding_side='right')
+
+model = dict(
+ type=SupervisedFinetune,
+ use_varlen_attn=use_varlen_attn,
+ llm=dict(
+ type=AutoModelForCausalLM.from_pretrained,
+ pretrained_model_name_or_path=pretrained_model_name_or_path,
+ trust_remote_code=True))
+
+#######################################################################
+# PART 3 Dataset & Dataloader #
+#######################################################################
+alpaca_en = dict(
+ type=process_hf_dataset,
+ dataset=dict(type=load_dataset, path=alpaca_en_path),
+ tokenizer=tokenizer,
+ max_length=max_length,
+ dataset_map_fn=alpaca_map_fn,
+ template_map_fn=dict(
+ type=template_map_fn_factory, template=prompt_template),
+ remove_unused_columns=True,
+ shuffle_before_pack=True,
+ pack_to_max_length=pack_to_max_length,
+ use_varlen_attn=use_varlen_attn)
+
+alpaca_zh = dict(
+ type=process_hf_dataset,
+ dataset=dict(type=load_dataset, path=alpaca_zh_path),
+ tokenizer=tokenizer,
+ max_length=max_length,
+ dataset_map_fn=alpaca_zh_map_fn,
+ template_map_fn=dict(
+ type=template_map_fn_factory, template=prompt_template),
+ remove_unused_columns=True,
+ shuffle_before_pack=True,
+ pack_to_max_length=pack_to_max_length,
+ use_varlen_attn=use_varlen_attn)
+
+train_dataset = dict(type=ConcatDataset, datasets=[alpaca_en, alpaca_zh])
+
+train_dataloader = dict(
+ batch_size=batch_size,
+ num_workers=dataloader_num_workers,
+ dataset=train_dataset,
+ sampler=dict(type=SequenceParallelSampler, seed=1024),
+ collate_fn=dict(type=default_collate_fn, use_varlen_attn=use_varlen_attn))
+
+#######################################################################
+# PART 4 Scheduler & Optimizer #
+#######################################################################
+# optimizer
+optim_wrapper = dict(
+ type=AmpOptimWrapper,
+ optimizer=dict(
+ type=optim_type, lr=lr, betas=betas, weight_decay=weight_decay),
+ clip_grad=dict(max_norm=max_norm, error_if_nonfinite=False),
+ accumulative_counts=accumulative_counts,
+ loss_scale='dynamic',
+ dtype='float16')
+
+# learning policy
+# More information: https://github.com/open-mmlab/mmengine/blob/main/docs/en/tutorials/param_scheduler.md # noqa: E501
+param_scheduler = [
+ dict(
+ type=LinearLR,
+ start_factor=1e-5,
+ by_epoch=True,
+ begin=0,
+ end=warmup_ratio * max_epochs,
+ convert_to_iter_based=True),
+ dict(
+ type=CosineAnnealingLR,
+ eta_min=0.0,
+ by_epoch=True,
+ begin=warmup_ratio * max_epochs,
+ end=max_epochs,
+ convert_to_iter_based=True)
+]
+
+# train, val, test setting
+train_cfg = dict(type=TrainLoop, max_epochs=max_epochs)
+
+#######################################################################
+# PART 5 Runtime #
+#######################################################################
+# Log the dialogue periodically during the training process, optional
+custom_hooks = [dict(type=ThroughputHook)]
+
+if use_varlen_attn:
+ custom_hooks += [dict(type=VarlenAttnArgsToMessageHubHook)]
+
+# configure default hooks
+default_hooks = dict(
+ # record the time of every iteration.
+ timer=dict(type=IterTimerHook),
+ # print log every 10 iterations.
+ logger=dict(
+ type=LoggerHook, log_metric_by_epoch=False, interval=log_interval),
+ # enable the parameter scheduler.
+ param_scheduler=dict(type=ParamSchedulerHook),
+ # save checkpoint per `save_steps`.
+ checkpoint=dict(
+ type=CheckpointHook,
+ by_epoch=False,
+ interval=-1,
+ save_last=False,
+ max_keep_ckpts=save_total_limit),
+ # set sampler seed in distributed evrionment.
+ sampler_seed=dict(type=DistSamplerSeedHook),
+)
+
+# configure environment
+env_cfg = dict(
+ # whether to enable cudnn benchmark
+ cudnn_benchmark=False,
+ # set multi process parameters
+ mp_cfg=dict(mp_start_method='fork', opencv_num_threads=0),
+ # set distributed parameters
+ dist_cfg=dict(backend='nccl'),
+)
+
+# set visualizer
+visualizer = None
+
+# set log level
+log_level = 'INFO'
+
+# load from which checkpoint
+load_from = None
+
+# whether to resume training from the loaded checkpoint
+resume = False
+
+# Defaults to use random seed and disable `deterministic`
+randomness = dict(seed=None, deterministic=False)
+
+# set log processor
+log_processor = dict(by_epoch=False, window_size=log_interval)
diff --git a/xtuner/configs/llama_speed_benchmark/yi_34b/yi_34b_200k_full_alpaca_enzh_256k_sp8.py b/xtuner/configs/llama_speed_benchmark/yi_34b/yi_34b_200k_full_alpaca_enzh_256k_sp8.py
new file mode 100644
index 000000000..86303fb52
--- /dev/null
+++ b/xtuner/configs/llama_speed_benchmark/yi_34b/yi_34b_200k_full_alpaca_enzh_256k_sp8.py
@@ -0,0 +1,212 @@
+# Copyright (c) OpenMMLab. All rights reserved.
+from datasets import load_dataset
+from mmengine.hooks import (CheckpointHook, DistSamplerSeedHook, IterTimerHook,
+ LoggerHook, ParamSchedulerHook)
+from mmengine.optim import AmpOptimWrapper, CosineAnnealingLR, LinearLR
+from torch.optim import AdamW
+from transformers import AutoModelForCausalLM, AutoTokenizer
+
+from xtuner.dataset import ConcatDataset, process_hf_dataset
+from xtuner.dataset.collate_fns import default_collate_fn
+from xtuner.dataset.map_fns import (alpaca_map_fn, alpaca_zh_map_fn,
+ template_map_fn_factory)
+from xtuner.engine.hooks import ThroughputHook, VarlenAttnArgsToMessageHubHook
+from xtuner.engine.runner import TrainLoop
+from xtuner.model import SupervisedFinetune
+from xtuner.parallel.sequence import SequenceParallelSampler
+from xtuner.utils import PROMPT_TEMPLATE, SYSTEM_TEMPLATE
+
+#######################################################################
+# PART 1 Settings #
+#######################################################################
+# Model
+pretrained_model_name_or_path = '01-ai/Yi-34B-200K'
+use_varlen_attn = False
+sequence_parallel_size = 8
+
+# Data
+alpaca_zh_path = 'silk-road/alpaca-data-gpt4-chinese'
+alpaca_en_path = 'tatsu-lab/alpaca'
+prompt_template = PROMPT_TEMPLATE.llama2_chat
+max_length = 262144 # 256k
+pack_to_max_length = True
+
+# Scheduler & Optimizer
+batch_size = 1 # per_device
+# Suppose I aim to employ a training strategy using a batch size per device
+# of 1 with a maximum length of `max_length` on N GPUs.
+# Upon setting the sequence parallelism dimension to `SP`,
+# the accumulative counts have to be adjusted to `SP` times the original value.
+# This modification is essential to assure training equivalence,
+# as the sequence of `max_length` length will be segmented into `SP` parts,
+# with each part being allocated to its respective GPU among the `SP` GPUs
+# for parallelized training.
+# bs = 32 gpus * 1 batch_size_per_device * 8 acc / 8 sequence parallel
+accumulative_counts = 8
+dataloader_num_workers = 4
+max_epochs = 3
+optim_type = AdamW
+lr = 2e-5
+betas = (0.9, 0.999)
+weight_decay = 0
+max_norm = 1 # grad clip
+warmup_ratio = 0.03
+log_interval = 1
+
+# Save
+save_steps = -1 # speed only
+save_total_limit = 2 # Maximum checkpoints to keep (-1 means unlimited)
+
+# Evaluate the generation performance during the training
+evaluation_freq = 50
+SYSTEM = SYSTEM_TEMPLATE.alpaca
+evaluation_inputs = [
+ '请给我介绍五个上海的景点', 'Please tell me five scenic spots in Shanghai'
+]
+
+#######################################################################
+# PART 2 Model & Tokenizer #
+#######################################################################
+tokenizer = dict(
+ type=AutoTokenizer.from_pretrained,
+ pretrained_model_name_or_path=pretrained_model_name_or_path,
+ trust_remote_code=True,
+ padding_side='right')
+
+model = dict(
+ type=SupervisedFinetune,
+ use_varlen_attn=use_varlen_attn,
+ llm=dict(
+ type=AutoModelForCausalLM.from_pretrained,
+ pretrained_model_name_or_path=pretrained_model_name_or_path,
+ trust_remote_code=True))
+
+#######################################################################
+# PART 3 Dataset & Dataloader #
+#######################################################################
+alpaca_en = dict(
+ type=process_hf_dataset,
+ dataset=dict(type=load_dataset, path=alpaca_en_path),
+ tokenizer=tokenizer,
+ max_length=max_length,
+ dataset_map_fn=alpaca_map_fn,
+ template_map_fn=dict(
+ type=template_map_fn_factory, template=prompt_template),
+ remove_unused_columns=True,
+ shuffle_before_pack=True,
+ pack_to_max_length=pack_to_max_length,
+ use_varlen_attn=use_varlen_attn)
+
+alpaca_zh = dict(
+ type=process_hf_dataset,
+ dataset=dict(type=load_dataset, path=alpaca_zh_path),
+ tokenizer=tokenizer,
+ max_length=max_length,
+ dataset_map_fn=alpaca_zh_map_fn,
+ template_map_fn=dict(
+ type=template_map_fn_factory, template=prompt_template),
+ remove_unused_columns=True,
+ shuffle_before_pack=True,
+ pack_to_max_length=pack_to_max_length,
+ use_varlen_attn=use_varlen_attn)
+
+train_dataset = dict(type=ConcatDataset, datasets=[alpaca_en, alpaca_zh])
+
+train_dataloader = dict(
+ batch_size=batch_size,
+ num_workers=dataloader_num_workers,
+ dataset=train_dataset,
+ sampler=dict(type=SequenceParallelSampler, seed=1024),
+ collate_fn=dict(type=default_collate_fn, use_varlen_attn=use_varlen_attn))
+
+#######################################################################
+# PART 4 Scheduler & Optimizer #
+#######################################################################
+# optimizer
+optim_wrapper = dict(
+ type=AmpOptimWrapper,
+ optimizer=dict(
+ type=optim_type, lr=lr, betas=betas, weight_decay=weight_decay),
+ clip_grad=dict(max_norm=max_norm, error_if_nonfinite=False),
+ accumulative_counts=accumulative_counts,
+ loss_scale='dynamic',
+ dtype='float16')
+
+# learning policy
+# More information: https://github.com/open-mmlab/mmengine/blob/main/docs/en/tutorials/param_scheduler.md # noqa: E501
+param_scheduler = [
+ dict(
+ type=LinearLR,
+ start_factor=1e-5,
+ by_epoch=True,
+ begin=0,
+ end=warmup_ratio * max_epochs,
+ convert_to_iter_based=True),
+ dict(
+ type=CosineAnnealingLR,
+ eta_min=0.0,
+ by_epoch=True,
+ begin=warmup_ratio * max_epochs,
+ end=max_epochs,
+ convert_to_iter_based=True)
+]
+
+# train, val, test setting
+train_cfg = dict(type=TrainLoop, max_epochs=max_epochs)
+
+#######################################################################
+# PART 5 Runtime #
+#######################################################################
+# Log the dialogue periodically during the training process, optional
+custom_hooks = [dict(type=ThroughputHook)]
+
+if use_varlen_attn:
+ custom_hooks += [dict(type=VarlenAttnArgsToMessageHubHook)]
+
+# configure default hooks
+default_hooks = dict(
+ # record the time of every iteration.
+ timer=dict(type=IterTimerHook),
+ # print log every 10 iterations.
+ logger=dict(
+ type=LoggerHook, log_metric_by_epoch=False, interval=log_interval),
+ # enable the parameter scheduler.
+ param_scheduler=dict(type=ParamSchedulerHook),
+ # save checkpoint per `save_steps`.
+ checkpoint=dict(
+ type=CheckpointHook,
+ by_epoch=False,
+ interval=-1,
+ save_last=False,
+ max_keep_ckpts=save_total_limit),
+ # set sampler seed in distributed evrionment.
+ sampler_seed=dict(type=DistSamplerSeedHook),
+)
+
+# configure environment
+env_cfg = dict(
+ # whether to enable cudnn benchmark
+ cudnn_benchmark=False,
+ # set multi process parameters
+ mp_cfg=dict(mp_start_method='fork', opencv_num_threads=0),
+ # set distributed parameters
+ dist_cfg=dict(backend='nccl'),
+)
+
+# set visualizer
+visualizer = None
+
+# set log level
+log_level = 'INFO'
+
+# load from which checkpoint
+load_from = None
+
+# whether to resume training from the loaded checkpoint
+resume = False
+
+# Defaults to use random seed and disable `deterministic`
+randomness = dict(seed=None, deterministic=False)
+
+# set log processor
+log_processor = dict(by_epoch=False, window_size=log_interval)
diff --git a/xtuner/configs/llama_speed_benchmark/yi_34b/yi_34b_200k_full_alpaca_enzh_32k_sp2.py b/xtuner/configs/llama_speed_benchmark/yi_34b/yi_34b_200k_full_alpaca_enzh_32k_sp2.py
new file mode 100644
index 000000000..452f999f6
--- /dev/null
+++ b/xtuner/configs/llama_speed_benchmark/yi_34b/yi_34b_200k_full_alpaca_enzh_32k_sp2.py
@@ -0,0 +1,212 @@
+# Copyright (c) OpenMMLab. All rights reserved.
+from datasets import load_dataset
+from mmengine.hooks import (CheckpointHook, DistSamplerSeedHook, IterTimerHook,
+ LoggerHook, ParamSchedulerHook)
+from mmengine.optim import AmpOptimWrapper, CosineAnnealingLR, LinearLR
+from torch.optim import AdamW
+from transformers import AutoModelForCausalLM, AutoTokenizer
+
+from xtuner.dataset import ConcatDataset, process_hf_dataset
+from xtuner.dataset.collate_fns import default_collate_fn
+from xtuner.dataset.map_fns import (alpaca_map_fn, alpaca_zh_map_fn,
+ template_map_fn_factory)
+from xtuner.engine.hooks import ThroughputHook, VarlenAttnArgsToMessageHubHook
+from xtuner.engine.runner import TrainLoop
+from xtuner.model import SupervisedFinetune
+from xtuner.parallel.sequence import SequenceParallelSampler
+from xtuner.utils import PROMPT_TEMPLATE, SYSTEM_TEMPLATE
+
+#######################################################################
+# PART 1 Settings #
+#######################################################################
+# Model
+pretrained_model_name_or_path = '01-ai/Yi-34B-200K'
+use_varlen_attn = False
+sequence_parallel_size = 2
+
+# Data
+alpaca_zh_path = 'silk-road/alpaca-data-gpt4-chinese'
+alpaca_en_path = 'tatsu-lab/alpaca'
+prompt_template = PROMPT_TEMPLATE.llama2_chat
+max_length = 32768
+pack_to_max_length = True
+
+# Scheduler & Optimizer
+batch_size = 1 # per_device
+# Suppose I aim to employ a training strategy using a batch size per device
+# of 1 with a maximum length of `max_length` on N GPUs.
+# Upon setting the sequence parallelism dimension to `SP`,
+# the accumulative counts have to be adjusted to `SP` times the original value.
+# This modification is essential to assure training equivalence,
+# as the sequence of `max_length` length will be segmented into `SP` parts,
+# with each part being allocated to its respective GPU among the `SP` GPUs
+# for parallelized training.
+# bs = 32 gpus * 1 batch_size_per_device * 2 acc / 2 sequence parallel
+accumulative_counts = 2
+dataloader_num_workers = 4
+max_epochs = 3
+optim_type = AdamW
+lr = 2e-5
+betas = (0.9, 0.999)
+weight_decay = 0
+max_norm = 1 # grad clip
+warmup_ratio = 0.03
+log_interval = 1
+
+# Save
+save_steps = -1 # speed only
+save_total_limit = 2 # Maximum checkpoints to keep (-1 means unlimited)
+
+# Evaluate the generation performance during the training
+evaluation_freq = 50
+SYSTEM = SYSTEM_TEMPLATE.alpaca
+evaluation_inputs = [
+ '请给我介绍五个上海的景点', 'Please tell me five scenic spots in Shanghai'
+]
+
+#######################################################################
+# PART 2 Model & Tokenizer #
+#######################################################################
+tokenizer = dict(
+ type=AutoTokenizer.from_pretrained,
+ pretrained_model_name_or_path=pretrained_model_name_or_path,
+ trust_remote_code=True,
+ padding_side='right')
+
+model = dict(
+ type=SupervisedFinetune,
+ use_varlen_attn=use_varlen_attn,
+ llm=dict(
+ type=AutoModelForCausalLM.from_pretrained,
+ pretrained_model_name_or_path=pretrained_model_name_or_path,
+ trust_remote_code=True))
+
+#######################################################################
+# PART 3 Dataset & Dataloader #
+#######################################################################
+alpaca_en = dict(
+ type=process_hf_dataset,
+ dataset=dict(type=load_dataset, path=alpaca_en_path),
+ tokenizer=tokenizer,
+ max_length=max_length,
+ dataset_map_fn=alpaca_map_fn,
+ template_map_fn=dict(
+ type=template_map_fn_factory, template=prompt_template),
+ remove_unused_columns=True,
+ shuffle_before_pack=True,
+ pack_to_max_length=pack_to_max_length,
+ use_varlen_attn=use_varlen_attn)
+
+alpaca_zh = dict(
+ type=process_hf_dataset,
+ dataset=dict(type=load_dataset, path=alpaca_zh_path),
+ tokenizer=tokenizer,
+ max_length=max_length,
+ dataset_map_fn=alpaca_zh_map_fn,
+ template_map_fn=dict(
+ type=template_map_fn_factory, template=prompt_template),
+ remove_unused_columns=True,
+ shuffle_before_pack=True,
+ pack_to_max_length=pack_to_max_length,
+ use_varlen_attn=use_varlen_attn)
+
+train_dataset = dict(type=ConcatDataset, datasets=[alpaca_en, alpaca_zh])
+
+train_dataloader = dict(
+ batch_size=batch_size,
+ num_workers=dataloader_num_workers,
+ dataset=train_dataset,
+ sampler=dict(type=SequenceParallelSampler, seed=1024),
+ collate_fn=dict(type=default_collate_fn, use_varlen_attn=use_varlen_attn))
+
+#######################################################################
+# PART 4 Scheduler & Optimizer #
+#######################################################################
+# optimizer
+optim_wrapper = dict(
+ type=AmpOptimWrapper,
+ optimizer=dict(
+ type=optim_type, lr=lr, betas=betas, weight_decay=weight_decay),
+ clip_grad=dict(max_norm=max_norm, error_if_nonfinite=False),
+ accumulative_counts=accumulative_counts,
+ loss_scale='dynamic',
+ dtype='float16')
+
+# learning policy
+# More information: https://github.com/open-mmlab/mmengine/blob/main/docs/en/tutorials/param_scheduler.md # noqa: E501
+param_scheduler = [
+ dict(
+ type=LinearLR,
+ start_factor=1e-5,
+ by_epoch=True,
+ begin=0,
+ end=warmup_ratio * max_epochs,
+ convert_to_iter_based=True),
+ dict(
+ type=CosineAnnealingLR,
+ eta_min=0.0,
+ by_epoch=True,
+ begin=warmup_ratio * max_epochs,
+ end=max_epochs,
+ convert_to_iter_based=True)
+]
+
+# train, val, test setting
+train_cfg = dict(type=TrainLoop, max_epochs=max_epochs)
+
+#######################################################################
+# PART 5 Runtime #
+#######################################################################
+# Log the dialogue periodically during the training process, optional
+custom_hooks = [dict(type=ThroughputHook)]
+
+if use_varlen_attn:
+ custom_hooks += [dict(type=VarlenAttnArgsToMessageHubHook)]
+
+# configure default hooks
+default_hooks = dict(
+ # record the time of every iteration.
+ timer=dict(type=IterTimerHook),
+ # print log every 10 iterations.
+ logger=dict(
+ type=LoggerHook, log_metric_by_epoch=False, interval=log_interval),
+ # enable the parameter scheduler.
+ param_scheduler=dict(type=ParamSchedulerHook),
+ # save checkpoint per `save_steps`.
+ checkpoint=dict(
+ type=CheckpointHook,
+ by_epoch=False,
+ interval=-1,
+ save_last=False,
+ max_keep_ckpts=save_total_limit),
+ # set sampler seed in distributed evrionment.
+ sampler_seed=dict(type=DistSamplerSeedHook),
+)
+
+# configure environment
+env_cfg = dict(
+ # whether to enable cudnn benchmark
+ cudnn_benchmark=False,
+ # set multi process parameters
+ mp_cfg=dict(mp_start_method='fork', opencv_num_threads=0),
+ # set distributed parameters
+ dist_cfg=dict(backend='nccl'),
+)
+
+# set visualizer
+visualizer = None
+
+# set log level
+log_level = 'INFO'
+
+# load from which checkpoint
+load_from = None
+
+# whether to resume training from the loaded checkpoint
+resume = False
+
+# Defaults to use random seed and disable `deterministic`
+randomness = dict(seed=None, deterministic=False)
+
+# set log processor
+log_processor = dict(by_epoch=False, window_size=log_interval)
diff --git a/xtuner/configs/llama_speed_benchmark/yi_34b/yi_34b_200k_full_alpaca_enzh_8k_sp1.py b/xtuner/configs/llama_speed_benchmark/yi_34b/yi_34b_200k_full_alpaca_enzh_8k_sp1.py
new file mode 100644
index 000000000..28e8c919c
--- /dev/null
+++ b/xtuner/configs/llama_speed_benchmark/yi_34b/yi_34b_200k_full_alpaca_enzh_8k_sp1.py
@@ -0,0 +1,212 @@
+# Copyright (c) OpenMMLab. All rights reserved.
+from datasets import load_dataset
+from mmengine.hooks import (CheckpointHook, DistSamplerSeedHook, IterTimerHook,
+ LoggerHook, ParamSchedulerHook)
+from mmengine.optim import AmpOptimWrapper, CosineAnnealingLR, LinearLR
+from torch.optim import AdamW
+from transformers import AutoModelForCausalLM, AutoTokenizer
+
+from xtuner.dataset import ConcatDataset, process_hf_dataset
+from xtuner.dataset.collate_fns import default_collate_fn
+from xtuner.dataset.map_fns import (alpaca_map_fn, alpaca_zh_map_fn,
+ template_map_fn_factory)
+from xtuner.engine.hooks import ThroughputHook, VarlenAttnArgsToMessageHubHook
+from xtuner.engine.runner import TrainLoop
+from xtuner.model import SupervisedFinetune
+from xtuner.parallel.sequence import SequenceParallelSampler
+from xtuner.utils import PROMPT_TEMPLATE, SYSTEM_TEMPLATE
+
+#######################################################################
+# PART 1 Settings #
+#######################################################################
+# Model
+pretrained_model_name_or_path = '01-ai/Yi-34B-200K'
+use_varlen_attn = False
+sequence_parallel_size = 1
+
+# Data
+alpaca_zh_path = 'silk-road/alpaca-data-gpt4-chinese'
+alpaca_en_path = 'tatsu-lab/alpaca'
+prompt_template = PROMPT_TEMPLATE.llama2_chat
+max_length = 8192
+pack_to_max_length = True
+
+# Scheduler & Optimizer
+batch_size = 1 # per_device
+# Suppose I aim to employ a training strategy using a batch size per device
+# of 1 with a maximum length of `max_length` on N GPUs.
+# Upon setting the sequence parallelism dimension to `SP`,
+# the accumulative counts have to be adjusted to `SP` times the original value.
+# This modification is essential to assure training equivalence,
+# as the sequence of `max_length` length will be segmented into `SP` parts,
+# with each part being allocated to its respective GPU among the `SP` GPUs
+# for parallelized training.
+# bs = 32 gpus * 1 batch_size_per_device * 1 acc / 1 sequence parallel
+accumulative_counts = 1
+dataloader_num_workers = 4
+max_epochs = 3
+optim_type = AdamW
+lr = 2e-5
+betas = (0.9, 0.999)
+weight_decay = 0
+max_norm = 1 # grad clip
+warmup_ratio = 0.03
+log_interval = 1
+
+# Save
+save_steps = -1 # speed only
+save_total_limit = 2 # Maximum checkpoints to keep (-1 means unlimited)
+
+# Evaluate the generation performance during the training
+evaluation_freq = 50
+SYSTEM = SYSTEM_TEMPLATE.alpaca
+evaluation_inputs = [
+ '请给我介绍五个上海的景点', 'Please tell me five scenic spots in Shanghai'
+]
+
+#######################################################################
+# PART 2 Model & Tokenizer #
+#######################################################################
+tokenizer = dict(
+ type=AutoTokenizer.from_pretrained,
+ pretrained_model_name_or_path=pretrained_model_name_or_path,
+ trust_remote_code=True,
+ padding_side='right')
+
+model = dict(
+ type=SupervisedFinetune,
+ use_varlen_attn=use_varlen_attn,
+ llm=dict(
+ type=AutoModelForCausalLM.from_pretrained,
+ pretrained_model_name_or_path=pretrained_model_name_or_path,
+ trust_remote_code=True))
+
+#######################################################################
+# PART 3 Dataset & Dataloader #
+#######################################################################
+alpaca_en = dict(
+ type=process_hf_dataset,
+ dataset=dict(type=load_dataset, path=alpaca_en_path),
+ tokenizer=tokenizer,
+ max_length=max_length,
+ dataset_map_fn=alpaca_map_fn,
+ template_map_fn=dict(
+ type=template_map_fn_factory, template=prompt_template),
+ remove_unused_columns=True,
+ shuffle_before_pack=True,
+ pack_to_max_length=pack_to_max_length,
+ use_varlen_attn=use_varlen_attn)
+
+alpaca_zh = dict(
+ type=process_hf_dataset,
+ dataset=dict(type=load_dataset, path=alpaca_zh_path),
+ tokenizer=tokenizer,
+ max_length=max_length,
+ dataset_map_fn=alpaca_zh_map_fn,
+ template_map_fn=dict(
+ type=template_map_fn_factory, template=prompt_template),
+ remove_unused_columns=True,
+ shuffle_before_pack=True,
+ pack_to_max_length=pack_to_max_length,
+ use_varlen_attn=use_varlen_attn)
+
+train_dataset = dict(type=ConcatDataset, datasets=[alpaca_en, alpaca_zh])
+
+train_dataloader = dict(
+ batch_size=batch_size,
+ num_workers=dataloader_num_workers,
+ dataset=train_dataset,
+ sampler=dict(type=SequenceParallelSampler, seed=1024),
+ collate_fn=dict(type=default_collate_fn, use_varlen_attn=use_varlen_attn))
+
+#######################################################################
+# PART 4 Scheduler & Optimizer #
+#######################################################################
+# optimizer
+optim_wrapper = dict(
+ type=AmpOptimWrapper,
+ optimizer=dict(
+ type=optim_type, lr=lr, betas=betas, weight_decay=weight_decay),
+ clip_grad=dict(max_norm=max_norm, error_if_nonfinite=False),
+ accumulative_counts=accumulative_counts,
+ loss_scale='dynamic',
+ dtype='float16')
+
+# learning policy
+# More information: https://github.com/open-mmlab/mmengine/blob/main/docs/en/tutorials/param_scheduler.md # noqa: E501
+param_scheduler = [
+ dict(
+ type=LinearLR,
+ start_factor=1e-5,
+ by_epoch=True,
+ begin=0,
+ end=warmup_ratio * max_epochs,
+ convert_to_iter_based=True),
+ dict(
+ type=CosineAnnealingLR,
+ eta_min=0.0,
+ by_epoch=True,
+ begin=warmup_ratio * max_epochs,
+ end=max_epochs,
+ convert_to_iter_based=True)
+]
+
+# train, val, test setting
+train_cfg = dict(type=TrainLoop, max_epochs=max_epochs)
+
+#######################################################################
+# PART 5 Runtime #
+#######################################################################
+# Log the dialogue periodically during the training process, optional
+custom_hooks = [dict(type=ThroughputHook)]
+
+if use_varlen_attn:
+ custom_hooks += [dict(type=VarlenAttnArgsToMessageHubHook)]
+
+# configure default hooks
+default_hooks = dict(
+ # record the time of every iteration.
+ timer=dict(type=IterTimerHook),
+ # print log every 10 iterations.
+ logger=dict(
+ type=LoggerHook, log_metric_by_epoch=False, interval=log_interval),
+ # enable the parameter scheduler.
+ param_scheduler=dict(type=ParamSchedulerHook),
+ # save checkpoint per `save_steps`.
+ checkpoint=dict(
+ type=CheckpointHook,
+ by_epoch=False,
+ interval=-1,
+ save_last=False,
+ max_keep_ckpts=save_total_limit),
+ # set sampler seed in distributed evrionment.
+ sampler_seed=dict(type=DistSamplerSeedHook),
+)
+
+# configure environment
+env_cfg = dict(
+ # whether to enable cudnn benchmark
+ cudnn_benchmark=False,
+ # set multi process parameters
+ mp_cfg=dict(mp_start_method='fork', opencv_num_threads=0),
+ # set distributed parameters
+ dist_cfg=dict(backend='nccl'),
+)
+
+# set visualizer
+visualizer = None
+
+# set log level
+log_level = 'INFO'
+
+# load from which checkpoint
+load_from = None
+
+# whether to resume training from the loaded checkpoint
+resume = False
+
+# Defaults to use random seed and disable `deterministic`
+randomness = dict(seed=None, deterministic=False)
+
+# set log processor
+log_processor = dict(by_epoch=False, window_size=log_interval)
diff --git a/xtuner/configs/llava/README.md b/xtuner/configs/llava/README.md
index 699c57b30..8d9db0f77 100644
--- a/xtuner/configs/llava/README.md
+++ b/xtuner/configs/llava/README.md
@@ -48,7 +48,7 @@ NPROC_PER_NODE=8 xtuner train llava_internlm2_chat_7b_clip_vit_large_p14_336_e1_
NPROC_PER_NODE=8 xtuner train llava_internlm2_chat_7b_qlora_clip_vit_large_p14_336_lora_e1_gpu8_finetune --deepspeed deepspeed_zero2
```
-## Model Convert (and Merge)
+## Model Conversion (and Merge)
After training, we will obtain a set of weights (*i.e.*, `iter_xxx.pth`), which are not in the universal HuggingFace format. We first need to convert them.
diff --git a/xtuner/configs/llava/internlm2_chat_1_8b_clip_vit_large_p14_336/finetune/llava_internlm2_chat_1_8b_qlora_clip_vit_large_p14_336_lora_e1_gpu8_finetune.py b/xtuner/configs/llava/internlm2_chat_1_8b_clip_vit_large_p14_336/finetune/llava_internlm2_chat_1_8b_qlora_clip_vit_large_p14_336_lora_e1_gpu8_finetune.py
index 3c84b4ce2..96e18e0e1 100644
--- a/xtuner/configs/llava/internlm2_chat_1_8b_clip_vit_large_p14_336/finetune/llava_internlm2_chat_1_8b_qlora_clip_vit_large_p14_336_lora_e1_gpu8_finetune.py
+++ b/xtuner/configs/llava/internlm2_chat_1_8b_clip_vit_large_p14_336/finetune/llava_internlm2_chat_1_8b_qlora_clip_vit_large_p14_336_lora_e1_gpu8_finetune.py
@@ -37,7 +37,7 @@
# Scheduler & Optimizer
batch_size = 16 # per_device
accumulative_counts = 1
-dataloader_num_workers = 0
+dataloader_num_workers = 4
max_epochs = 1
optim_type = AdamW
lr = 2e-4
@@ -120,6 +120,7 @@
train_dataloader = dict(
batch_size=batch_size,
num_workers=dataloader_num_workers,
+ pin_memory=True,
dataset=llava_dataset,
sampler=dict(
type=LengthGroupedSampler,
diff --git a/xtuner/configs/llava/internlm2_chat_1_8b_clip_vit_large_p14_336/pretrain/llava_internlm2_chat_1_8b_clip_vit_large_p14_336_e1_gpu8_pretrain.py b/xtuner/configs/llava/internlm2_chat_1_8b_clip_vit_large_p14_336/pretrain/llava_internlm2_chat_1_8b_clip_vit_large_p14_336_e1_gpu8_pretrain.py
index c40064a43..e14cdc91a 100644
--- a/xtuner/configs/llava/internlm2_chat_1_8b_clip_vit_large_p14_336/pretrain/llava_internlm2_chat_1_8b_clip_vit_large_p14_336_e1_gpu8_pretrain.py
+++ b/xtuner/configs/llava/internlm2_chat_1_8b_clip_vit_large_p14_336/pretrain/llava_internlm2_chat_1_8b_clip_vit_large_p14_336_e1_gpu8_pretrain.py
@@ -34,7 +34,7 @@
# Scheduler & Optimizer
batch_size = 32 # per_device
accumulative_counts = 1
-dataloader_num_workers = 0
+dataloader_num_workers = 4
max_epochs = 1
optim_type = AdamW
lr = 1e-3
@@ -107,6 +107,7 @@
train_dataloader = dict(
batch_size=batch_size,
num_workers=dataloader_num_workers,
+ pin_memory=True,
dataset=llava_dataset,
sampler=dict(type=DefaultSampler, shuffle=True),
collate_fn=dict(type=default_collate_fn))
diff --git a/xtuner/configs/llava/internlm2_chat_20b_clip_vit_large_p14_336/finetune/llava_internlm2_chat_20b_clip_vit_large_p14_336_e1_gpu8_finetune.py b/xtuner/configs/llava/internlm2_chat_20b_clip_vit_large_p14_336/finetune/llava_internlm2_chat_20b_clip_vit_large_p14_336_e1_gpu8_finetune.py
new file mode 100644
index 000000000..ff4e20ce3
--- /dev/null
+++ b/xtuner/configs/llava/internlm2_chat_20b_clip_vit_large_p14_336/finetune/llava_internlm2_chat_20b_clip_vit_large_p14_336_e1_gpu8_finetune.py
@@ -0,0 +1,207 @@
+# Copyright (c) OpenMMLab. All rights reserved.
+import torch
+from mmengine.hooks import (CheckpointHook, DistSamplerSeedHook, IterTimerHook,
+ LoggerHook, ParamSchedulerHook)
+from mmengine.optim import AmpOptimWrapper, CosineAnnealingLR, LinearLR
+from torch.optim import AdamW
+from transformers import (AutoModelForCausalLM, AutoTokenizer,
+ CLIPImageProcessor, CLIPVisionModel)
+
+from xtuner.dataset import LLaVADataset
+from xtuner.dataset.collate_fns import default_collate_fn
+from xtuner.dataset.map_fns import llava_map_fn, template_map_fn_factory
+from xtuner.dataset.samplers import LengthGroupedSampler
+from xtuner.engine.hooks import DatasetInfoHook, EvaluateChatHook
+from xtuner.engine.runner import TrainLoop
+from xtuner.model import LLaVAModel
+from xtuner.utils import PROMPT_TEMPLATE
+
+#######################################################################
+# PART 1 Settings #
+#######################################################################
+# Model
+llm_name_or_path = 'internlm/internlm2-chat-20b'
+visual_encoder_name_or_path = 'openai/clip-vit-large-patch14-336'
+# Specify the pretrained pth
+pretrained_pth = './work_dirs/llava_internlm2_chat_20b_clip_vit_large_p14_336_e1_gpu8_pretrain/iter_2181.pth' # noqa: E501
+
+# Data
+data_root = './data/llava_data/'
+data_path = data_root + 'LLaVA-Instruct-150K/llava_v1_5_mix665k.json'
+image_folder = data_root + 'llava_images'
+prompt_template = PROMPT_TEMPLATE.internlm2_chat
+max_length = int(2048 - (336 / 14)**2)
+
+# Scheduler & Optimizer
+batch_size = 4 # per_device
+accumulative_counts = 4
+
+dataloader_num_workers = 0
+max_epochs = 1
+optim_type = AdamW
+lr = 2e-5
+betas = (0.9, 0.999)
+weight_decay = 0
+max_norm = 1 # grad clip
+warmup_ratio = 0.03
+
+# Save
+save_steps = 500
+save_total_limit = 2 # Maximum checkpoints to keep (-1 means unlimited)
+
+# Evaluate the generation performance during the training
+evaluation_freq = 500
+SYSTEM = ''
+evaluation_images = 'https://llava-vl.github.io/static/images/view.jpg'
+evaluation_inputs = ['请描述一下这张照片', 'Please describe this picture']
+
+#######################################################################
+# PART 2 Model & Tokenizer & Image Processor #
+#######################################################################
+tokenizer = dict(
+ type=AutoTokenizer.from_pretrained,
+ pretrained_model_name_or_path=llm_name_or_path,
+ trust_remote_code=True,
+ padding_side='right')
+
+image_processor = dict(
+ type=CLIPImageProcessor.from_pretrained,
+ pretrained_model_name_or_path=visual_encoder_name_or_path,
+ trust_remote_code=True)
+
+model = dict(
+ type=LLaVAModel,
+ freeze_llm=False,
+ freeze_visual_encoder=True,
+ pretrained_pth=pretrained_pth,
+ llm=dict(
+ type=AutoModelForCausalLM.from_pretrained,
+ pretrained_model_name_or_path=llm_name_or_path,
+ trust_remote_code=True,
+ torch_dtype=torch.float32),
+ visual_encoder=dict(
+ type=CLIPVisionModel.from_pretrained,
+ pretrained_model_name_or_path=visual_encoder_name_or_path))
+
+#######################################################################
+# PART 3 Dataset & Dataloader #
+#######################################################################
+llava_dataset = dict(
+ type=LLaVADataset,
+ data_path=data_path,
+ image_folder=image_folder,
+ tokenizer=tokenizer,
+ image_processor=image_processor,
+ dataset_map_fn=llava_map_fn,
+ template_map_fn=dict(
+ type=template_map_fn_factory, template=prompt_template),
+ max_length=max_length,
+ pad_image_to_square=True)
+
+train_dataloader = dict(
+ batch_size=batch_size,
+ num_workers=dataloader_num_workers,
+ dataset=llava_dataset,
+ sampler=dict(
+ type=LengthGroupedSampler,
+ length_property='modality_length',
+ per_device_batch_size=batch_size * accumulative_counts),
+ collate_fn=dict(type=default_collate_fn))
+
+#######################################################################
+# PART 4 Scheduler & Optimizer #
+#######################################################################
+# optimizer
+optim_wrapper = dict(
+ type=AmpOptimWrapper,
+ optimizer=dict(
+ type=optim_type, lr=lr, betas=betas, weight_decay=weight_decay),
+ clip_grad=dict(max_norm=max_norm, error_if_nonfinite=False),
+ accumulative_counts=accumulative_counts,
+ loss_scale='dynamic',
+ dtype='float16')
+
+# learning policy
+# More information: https://github.com/open-mmlab/mmengine/blob/main/docs/en/tutorials/param_scheduler.md # noqa: E501
+param_scheduler = [
+ dict(
+ type=LinearLR,
+ start_factor=1e-5,
+ by_epoch=True,
+ begin=0,
+ end=warmup_ratio * max_epochs,
+ convert_to_iter_based=True),
+ dict(
+ type=CosineAnnealingLR,
+ eta_min=0.0,
+ by_epoch=True,
+ begin=warmup_ratio * max_epochs,
+ end=max_epochs,
+ convert_to_iter_based=True)
+]
+
+# train, val, test setting
+train_cfg = dict(type=TrainLoop, max_epochs=max_epochs)
+
+#######################################################################
+# PART 5 Runtime #
+#######################################################################
+# Log the dialogue periodically during the training process, optional
+custom_hooks = [
+ dict(type=DatasetInfoHook, tokenizer=tokenizer),
+ dict(
+ type=EvaluateChatHook,
+ tokenizer=tokenizer,
+ image_processor=image_processor,
+ every_n_iters=evaluation_freq,
+ evaluation_inputs=evaluation_inputs,
+ evaluation_images=evaluation_images,
+ system=SYSTEM,
+ prompt_template=prompt_template)
+]
+
+# configure default hooks
+default_hooks = dict(
+ # record the time of every iteration.
+ timer=dict(type=IterTimerHook),
+ # print log every 10 iterations.
+ logger=dict(type=LoggerHook, log_metric_by_epoch=False, interval=10),
+ # enable the parameter scheduler.
+ param_scheduler=dict(type=ParamSchedulerHook),
+ # save checkpoint per `save_steps`.
+ checkpoint=dict(
+ type=CheckpointHook,
+ by_epoch=False,
+ interval=save_steps,
+ max_keep_ckpts=save_total_limit),
+ # set sampler seed in distributed evrionment.
+ sampler_seed=dict(type=DistSamplerSeedHook),
+)
+
+# configure environment
+env_cfg = dict(
+ # whether to enable cudnn benchmark
+ cudnn_benchmark=False,
+ # set multi process parameters
+ mp_cfg=dict(mp_start_method='fork', opencv_num_threads=0),
+ # set distributed parameters
+ dist_cfg=dict(backend='nccl'),
+)
+
+# set visualizer
+visualizer = None
+
+# set log level
+log_level = 'INFO'
+
+# load from which checkpoint
+load_from = None
+
+# whether to resume training from the loaded checkpoint
+resume = False
+
+# Defaults to use random seed and disable `deterministic`
+randomness = dict(seed=None, deterministic=False)
+
+# set log processor
+log_processor = dict(by_epoch=False)
diff --git a/xtuner/configs/llava/internlm2_chat_20b_clip_vit_large_p14_336/finetune/llava_internlm2_chat_20b_qlora_clip_vit_large_p14_336_lora_e1_gpu8_finetune.py b/xtuner/configs/llava/internlm2_chat_20b_clip_vit_large_p14_336/finetune/llava_internlm2_chat_20b_qlora_clip_vit_large_p14_336_lora_e1_gpu8_finetune.py
index 2eec60373..1dacbeb92 100644
--- a/xtuner/configs/llava/internlm2_chat_20b_clip_vit_large_p14_336/finetune/llava_internlm2_chat_20b_qlora_clip_vit_large_p14_336_lora_e1_gpu8_finetune.py
+++ b/xtuner/configs/llava/internlm2_chat_20b_clip_vit_large_p14_336/finetune/llava_internlm2_chat_20b_qlora_clip_vit_large_p14_336_lora_e1_gpu8_finetune.py
@@ -37,7 +37,7 @@
# Scheduler & Optimizer
batch_size = 8 # per_device
accumulative_counts = 2
-dataloader_num_workers = 0
+dataloader_num_workers = 4
max_epochs = 1
optim_type = AdamW
lr = 2e-4
@@ -120,6 +120,7 @@
train_dataloader = dict(
batch_size=batch_size,
num_workers=dataloader_num_workers,
+ pin_memory=True,
dataset=llava_dataset,
sampler=dict(
type=LengthGroupedSampler,
diff --git a/xtuner/configs/llava/internlm2_chat_20b_clip_vit_large_p14_336/pretrain/llava_internlm2_chat_20b_clip_vit_large_p14_336_e1_gpu8_pretrain.py b/xtuner/configs/llava/internlm2_chat_20b_clip_vit_large_p14_336/pretrain/llava_internlm2_chat_20b_clip_vit_large_p14_336_e1_gpu8_pretrain.py
index 717a8a2a0..3cc2839a9 100644
--- a/xtuner/configs/llava/internlm2_chat_20b_clip_vit_large_p14_336/pretrain/llava_internlm2_chat_20b_clip_vit_large_p14_336_e1_gpu8_pretrain.py
+++ b/xtuner/configs/llava/internlm2_chat_20b_clip_vit_large_p14_336/pretrain/llava_internlm2_chat_20b_clip_vit_large_p14_336_e1_gpu8_pretrain.py
@@ -34,7 +34,7 @@
# Scheduler & Optimizer
batch_size = 32 # per_device
accumulative_counts = 1
-dataloader_num_workers = 0
+dataloader_num_workers = 4
max_epochs = 1
optim_type = AdamW
lr = 1e-3
@@ -107,6 +107,7 @@
train_dataloader = dict(
batch_size=batch_size,
num_workers=dataloader_num_workers,
+ pin_memory=True,
dataset=llava_dataset,
sampler=dict(type=DefaultSampler, shuffle=True),
collate_fn=dict(type=default_collate_fn))
diff --git a/xtuner/configs/llava/internlm2_chat_7b_clip_vit_large_p14_336/finetune/llava_internlm2_chat_7b_clip_vit_large_p14_336_e1_gpu8_finetune.py b/xtuner/configs/llava/internlm2_chat_7b_clip_vit_large_p14_336/finetune/llava_internlm2_chat_7b_clip_vit_large_p14_336_e1_gpu8_finetune.py
new file mode 100644
index 000000000..e9f4d8b5f
--- /dev/null
+++ b/xtuner/configs/llava/internlm2_chat_7b_clip_vit_large_p14_336/finetune/llava_internlm2_chat_7b_clip_vit_large_p14_336_e1_gpu8_finetune.py
@@ -0,0 +1,206 @@
+# Copyright (c) OpenMMLab. All rights reserved.
+import torch
+from mmengine.hooks import (CheckpointHook, DistSamplerSeedHook, IterTimerHook,
+ LoggerHook, ParamSchedulerHook)
+from mmengine.optim import AmpOptimWrapper, CosineAnnealingLR, LinearLR
+from torch.optim import AdamW
+from transformers import (AutoModelForCausalLM, AutoTokenizer,
+ CLIPImageProcessor, CLIPVisionModel)
+
+from xtuner.dataset import LLaVADataset
+from xtuner.dataset.collate_fns import default_collate_fn
+from xtuner.dataset.map_fns import llava_map_fn, template_map_fn_factory
+from xtuner.dataset.samplers import LengthGroupedSampler
+from xtuner.engine.hooks import DatasetInfoHook, EvaluateChatHook
+from xtuner.engine.runner import TrainLoop
+from xtuner.model import LLaVAModel
+from xtuner.utils import PROMPT_TEMPLATE
+
+#######################################################################
+# PART 1 Settings #
+#######################################################################
+# Model
+llm_name_or_path = 'internlm/internlm2-chat-7b'
+visual_encoder_name_or_path = 'openai/clip-vit-large-patch14-336'
+# Specify the pretrained pth
+pretrained_pth = './work_dirs/llava_internlm2_chat_7b_clip_vit_large_p14_336_e1_gpu8_pretrain/iter_2181.pth' # noqa: E501
+
+# Data
+data_root = './data/llava_data/'
+data_path = data_root + 'LLaVA-Instruct-150K/llava_v1_5_mix665k.json'
+image_folder = data_root + 'llava_images'
+prompt_template = PROMPT_TEMPLATE.internlm2_chat
+max_length = int(2048 - (336 / 14)**2)
+
+# Scheduler & Optimizer
+batch_size = 8 # per_device
+accumulative_counts = 2
+dataloader_num_workers = 0
+max_epochs = 1
+optim_type = AdamW
+lr = 2e-5
+betas = (0.9, 0.999)
+weight_decay = 0
+max_norm = 1 # grad clip
+warmup_ratio = 0.03
+
+# Save
+save_steps = 500
+save_total_limit = 2 # Maximum checkpoints to keep (-1 means unlimited)
+
+# Evaluate the generation performance during the training
+evaluation_freq = 500
+SYSTEM = ''
+evaluation_images = 'https://llava-vl.github.io/static/images/view.jpg'
+evaluation_inputs = ['请描述一下这张照片', 'Please describe this picture']
+
+#######################################################################
+# PART 2 Model & Tokenizer & Image Processor #
+#######################################################################
+tokenizer = dict(
+ type=AutoTokenizer.from_pretrained,
+ pretrained_model_name_or_path=llm_name_or_path,
+ trust_remote_code=True,
+ padding_side='right')
+
+image_processor = dict(
+ type=CLIPImageProcessor.from_pretrained,
+ pretrained_model_name_or_path=visual_encoder_name_or_path,
+ trust_remote_code=True)
+
+model = dict(
+ type=LLaVAModel,
+ freeze_llm=False,
+ freeze_visual_encoder=True,
+ pretrained_pth=pretrained_pth,
+ llm=dict(
+ type=AutoModelForCausalLM.from_pretrained,
+ pretrained_model_name_or_path=llm_name_or_path,
+ trust_remote_code=True,
+ torch_dtype=torch.float32),
+ visual_encoder=dict(
+ type=CLIPVisionModel.from_pretrained,
+ pretrained_model_name_or_path=visual_encoder_name_or_path))
+
+#######################################################################
+# PART 3 Dataset & Dataloader #
+#######################################################################
+llava_dataset = dict(
+ type=LLaVADataset,
+ data_path=data_path,
+ image_folder=image_folder,
+ tokenizer=tokenizer,
+ image_processor=image_processor,
+ dataset_map_fn=llava_map_fn,
+ template_map_fn=dict(
+ type=template_map_fn_factory, template=prompt_template),
+ max_length=max_length,
+ pad_image_to_square=True)
+
+train_dataloader = dict(
+ batch_size=batch_size,
+ num_workers=dataloader_num_workers,
+ dataset=llava_dataset,
+ sampler=dict(
+ type=LengthGroupedSampler,
+ length_property='modality_length',
+ per_device_batch_size=batch_size * accumulative_counts),
+ collate_fn=dict(type=default_collate_fn))
+
+#######################################################################
+# PART 4 Scheduler & Optimizer #
+#######################################################################
+# optimizer
+optim_wrapper = dict(
+ type=AmpOptimWrapper,
+ optimizer=dict(
+ type=optim_type, lr=lr, betas=betas, weight_decay=weight_decay),
+ clip_grad=dict(max_norm=max_norm, error_if_nonfinite=False),
+ accumulative_counts=accumulative_counts,
+ loss_scale='dynamic',
+ dtype='float16')
+
+# learning policy
+# More information: https://github.com/open-mmlab/mmengine/blob/main/docs/en/tutorials/param_scheduler.md # noqa: E501
+param_scheduler = [
+ dict(
+ type=LinearLR,
+ start_factor=1e-5,
+ by_epoch=True,
+ begin=0,
+ end=warmup_ratio * max_epochs,
+ convert_to_iter_based=True),
+ dict(
+ type=CosineAnnealingLR,
+ eta_min=0.0,
+ by_epoch=True,
+ begin=warmup_ratio * max_epochs,
+ end=max_epochs,
+ convert_to_iter_based=True)
+]
+
+# train, val, test setting
+train_cfg = dict(type=TrainLoop, max_epochs=max_epochs)
+
+#######################################################################
+# PART 5 Runtime #
+#######################################################################
+# Log the dialogue periodically during the training process, optional
+custom_hooks = [
+ dict(type=DatasetInfoHook, tokenizer=tokenizer),
+ dict(
+ type=EvaluateChatHook,
+ tokenizer=tokenizer,
+ image_processor=image_processor,
+ every_n_iters=evaluation_freq,
+ evaluation_inputs=evaluation_inputs,
+ evaluation_images=evaluation_images,
+ system=SYSTEM,
+ prompt_template=prompt_template)
+]
+
+# configure default hooks
+default_hooks = dict(
+ # record the time of every iteration.
+ timer=dict(type=IterTimerHook),
+ # print log every 10 iterations.
+ logger=dict(type=LoggerHook, log_metric_by_epoch=False, interval=10),
+ # enable the parameter scheduler.
+ param_scheduler=dict(type=ParamSchedulerHook),
+ # save checkpoint per `save_steps`.
+ checkpoint=dict(
+ type=CheckpointHook,
+ by_epoch=False,
+ interval=save_steps,
+ max_keep_ckpts=save_total_limit),
+ # set sampler seed in distributed evrionment.
+ sampler_seed=dict(type=DistSamplerSeedHook),
+)
+
+# configure environment
+env_cfg = dict(
+ # whether to enable cudnn benchmark
+ cudnn_benchmark=False,
+ # set multi process parameters
+ mp_cfg=dict(mp_start_method='fork', opencv_num_threads=0),
+ # set distributed parameters
+ dist_cfg=dict(backend='nccl'),
+)
+
+# set visualizer
+visualizer = None
+
+# set log level
+log_level = 'INFO'
+
+# load from which checkpoint
+load_from = None
+
+# whether to resume training from the loaded checkpoint
+resume = False
+
+# Defaults to use random seed and disable `deterministic`
+randomness = dict(seed=None, deterministic=False)
+
+# set log processor
+log_processor = dict(by_epoch=False)
diff --git a/xtuner/configs/llava/internlm2_chat_7b_clip_vit_large_p14_336/finetune/llava_internlm2_chat_7b_qlora_clip_vit_large_p14_336_lora_e1_gpu8_finetune.py b/xtuner/configs/llava/internlm2_chat_7b_clip_vit_large_p14_336/finetune/llava_internlm2_chat_7b_qlora_clip_vit_large_p14_336_lora_e1_gpu8_finetune.py
index e35e40bf4..3652333c9 100644
--- a/xtuner/configs/llava/internlm2_chat_7b_clip_vit_large_p14_336/finetune/llava_internlm2_chat_7b_qlora_clip_vit_large_p14_336_lora_e1_gpu8_finetune.py
+++ b/xtuner/configs/llava/internlm2_chat_7b_clip_vit_large_p14_336/finetune/llava_internlm2_chat_7b_qlora_clip_vit_large_p14_336_lora_e1_gpu8_finetune.py
@@ -37,7 +37,7 @@
# Scheduler & Optimizer
batch_size = 16 # per_device
accumulative_counts = 1
-dataloader_num_workers = 0
+dataloader_num_workers = 4
max_epochs = 1
optim_type = AdamW
lr = 2e-4
@@ -120,6 +120,7 @@
train_dataloader = dict(
batch_size=batch_size,
num_workers=dataloader_num_workers,
+ pin_memory=True,
dataset=llava_dataset,
sampler=dict(
type=LengthGroupedSampler,
diff --git a/xtuner/configs/llava/internlm2_chat_7b_clip_vit_large_p14_336/pretrain/llava_internlm2_chat_7b_clip_vit_large_p14_336_e1_gpu8_pretrain.py b/xtuner/configs/llava/internlm2_chat_7b_clip_vit_large_p14_336/pretrain/llava_internlm2_chat_7b_clip_vit_large_p14_336_e1_gpu8_pretrain.py
index 0edc43280..72d69b4b3 100644
--- a/xtuner/configs/llava/internlm2_chat_7b_clip_vit_large_p14_336/pretrain/llava_internlm2_chat_7b_clip_vit_large_p14_336_e1_gpu8_pretrain.py
+++ b/xtuner/configs/llava/internlm2_chat_7b_clip_vit_large_p14_336/pretrain/llava_internlm2_chat_7b_clip_vit_large_p14_336_e1_gpu8_pretrain.py
@@ -34,7 +34,7 @@
# Scheduler & Optimizer
batch_size = 32 # per_device
accumulative_counts = 1
-dataloader_num_workers = 0
+dataloader_num_workers = 4
max_epochs = 1
optim_type = AdamW
lr = 1e-3
@@ -107,6 +107,7 @@
train_dataloader = dict(
batch_size=batch_size,
num_workers=dataloader_num_workers,
+ pin_memory=True,
dataset=llava_dataset,
sampler=dict(type=DefaultSampler, shuffle=True),
collate_fn=dict(type=default_collate_fn))
diff --git a/xtuner/configs/llava/internlm_chat_7b_clip_vit_large_p14_336/finetune/llava_internlm_chat_7b_qlora_clip_vit_large_p14_336_lora_e1_gpu8_finetune.py b/xtuner/configs/llava/internlm_chat_7b_clip_vit_large_p14_336/finetune/llava_internlm_chat_7b_qlora_clip_vit_large_p14_336_lora_e1_gpu8_finetune.py
index 564799c75..e25dc4cc1 100644
--- a/xtuner/configs/llava/internlm_chat_7b_clip_vit_large_p14_336/finetune/llava_internlm_chat_7b_qlora_clip_vit_large_p14_336_lora_e1_gpu8_finetune.py
+++ b/xtuner/configs/llava/internlm_chat_7b_clip_vit_large_p14_336/finetune/llava_internlm_chat_7b_qlora_clip_vit_large_p14_336_lora_e1_gpu8_finetune.py
@@ -37,7 +37,7 @@
# Scheduler & Optimizer
batch_size = 16 # per_device
accumulative_counts = 1
-dataloader_num_workers = 0
+dataloader_num_workers = 4
max_epochs = 1
optim_type = AdamW
lr = 2e-4
@@ -120,6 +120,7 @@
train_dataloader = dict(
batch_size=batch_size,
num_workers=dataloader_num_workers,
+ pin_memory=True,
dataset=llava_dataset,
sampler=dict(
type=LengthGroupedSampler,
diff --git a/xtuner/configs/llava/internlm_chat_7b_clip_vit_large_p14_336/pretrain/llava_internlm_chat_7b_clip_vit_large_p14_336_e1_gpu8_pretrain.py b/xtuner/configs/llava/internlm_chat_7b_clip_vit_large_p14_336/pretrain/llava_internlm_chat_7b_clip_vit_large_p14_336_e1_gpu8_pretrain.py
index 00dd501c2..fbbbeb5ff 100644
--- a/xtuner/configs/llava/internlm_chat_7b_clip_vit_large_p14_336/pretrain/llava_internlm_chat_7b_clip_vit_large_p14_336_e1_gpu8_pretrain.py
+++ b/xtuner/configs/llava/internlm_chat_7b_clip_vit_large_p14_336/pretrain/llava_internlm_chat_7b_clip_vit_large_p14_336_e1_gpu8_pretrain.py
@@ -34,7 +34,7 @@
# Scheduler & Optimizer
batch_size = 32 # per_device
accumulative_counts = 1
-dataloader_num_workers = 0
+dataloader_num_workers = 4
max_epochs = 1
optim_type = AdamW
lr = 1e-3
@@ -107,6 +107,7 @@
train_dataloader = dict(
batch_size=batch_size,
num_workers=dataloader_num_workers,
+ pin_memory=True,
dataset=llava_dataset,
sampler=dict(type=DefaultSampler, shuffle=True),
collate_fn=dict(type=default_collate_fn))
diff --git a/xtuner/configs/llava/llama3_70b_instruct_clip_vit_large_p14_336/pretrain/llava_llama3_70b_instruct_quant_clip_vit_large_p14_336_e1_gpu8_pretrain.py b/xtuner/configs/llava/llama3_70b_instruct_clip_vit_large_p14_336/pretrain/llava_llama3_70b_instruct_quant_clip_vit_large_p14_336_e1_gpu8_pretrain.py
new file mode 100644
index 000000000..e3ef73297
--- /dev/null
+++ b/xtuner/configs/llava/llama3_70b_instruct_clip_vit_large_p14_336/pretrain/llava_llama3_70b_instruct_quant_clip_vit_large_p14_336_e1_gpu8_pretrain.py
@@ -0,0 +1,210 @@
+# Copyright (c) OpenMMLab. All rights reserved.
+import torch
+from mmengine.dataset import DefaultSampler
+from mmengine.hooks import (CheckpointHook, DistSamplerSeedHook, IterTimerHook,
+ LoggerHook, ParamSchedulerHook)
+from mmengine.optim import AmpOptimWrapper, CosineAnnealingLR, LinearLR
+from torch.optim import AdamW
+from transformers import (AutoModelForCausalLM, AutoTokenizer,
+ BitsAndBytesConfig, CLIPImageProcessor,
+ CLIPVisionModel)
+
+from xtuner.dataset import LLaVADataset
+from xtuner.dataset.collate_fns import default_collate_fn
+from xtuner.dataset.map_fns import llava_map_fn, template_map_fn_factory
+from xtuner.engine.hooks import DatasetInfoHook, EvaluateChatHook
+from xtuner.engine.runner import TrainLoop
+from xtuner.model import LLaVAModel
+from xtuner.utils import PROMPT_TEMPLATE
+
+#######################################################################
+# PART 1 Settings #
+#######################################################################
+# Model
+llm_name_or_path = 'meta-llama/Meta-Llama-3-8B-Instruct'
+visual_encoder_name_or_path = 'openai/clip-vit-large-patch14-336'
+
+# Data
+data_root = './data/llava_data/'
+data_path = data_root + 'LLaVA-Pretrain/blip_laion_cc_sbu_558k.json'
+image_folder = data_root + 'LLaVA-Pretrain/images'
+prompt_template = PROMPT_TEMPLATE.llama3_chat
+max_length = int(2048 - (336 / 14)**2)
+
+# Scheduler & Optimizer
+batch_size = 32 # per_device
+accumulative_counts = 1
+dataloader_num_workers = 4
+max_epochs = 1
+optim_type = AdamW
+lr = 5e-4
+betas = (0.9, 0.999)
+weight_decay = 0
+max_norm = 1 # grad clip
+warmup_ratio = 0.03
+
+# Save
+save_steps = 500
+save_total_limit = 2 # Maximum checkpoints to keep (-1 means unlimited)
+
+# Evaluate the generation performance during the training
+evaluation_freq = 500
+SYSTEM = ''
+evaluation_images = 'https://llava-vl.github.io/static/images/view.jpg'
+evaluation_inputs = ['请描述一下这张照片', 'Please describe this picture']
+
+#######################################################################
+# PART 2 Model & Tokenizer & Image Processor #
+#######################################################################
+tokenizer = dict(
+ type=AutoTokenizer.from_pretrained,
+ pretrained_model_name_or_path=llm_name_or_path,
+ trust_remote_code=True,
+ padding_side='right')
+
+image_processor = dict(
+ type=CLIPImageProcessor.from_pretrained,
+ pretrained_model_name_or_path=visual_encoder_name_or_path,
+ trust_remote_code=True)
+
+model = dict(
+ type=LLaVAModel,
+ freeze_llm=True,
+ freeze_visual_encoder=True,
+ llm=dict(
+ type=AutoModelForCausalLM.from_pretrained,
+ pretrained_model_name_or_path=llm_name_or_path,
+ trust_remote_code=True,
+ torch_dtype=torch.float16,
+ quantization_config=dict(
+ type=BitsAndBytesConfig,
+ load_in_4bit=True,
+ load_in_8bit=False,
+ llm_int8_threshold=6.0,
+ llm_int8_has_fp16_weight=False,
+ bnb_4bit_compute_dtype=torch.float16,
+ bnb_4bit_use_double_quant=True,
+ bnb_4bit_quant_type='nf4')),
+ visual_encoder=dict(
+ type=CLIPVisionModel.from_pretrained,
+ pretrained_model_name_or_path=visual_encoder_name_or_path))
+
+#######################################################################
+# PART 3 Dataset & Dataloader #
+#######################################################################
+llava_dataset = dict(
+ type=LLaVADataset,
+ data_path=data_path,
+ image_folder=image_folder,
+ tokenizer=tokenizer,
+ image_processor=image_processor,
+ dataset_map_fn=llava_map_fn,
+ template_map_fn=dict(
+ type=template_map_fn_factory, template=prompt_template),
+ max_length=max_length,
+ pad_image_to_square=False)
+
+train_dataloader = dict(
+ batch_size=batch_size,
+ num_workers=dataloader_num_workers,
+ dataset=llava_dataset,
+ sampler=dict(type=DefaultSampler, shuffle=True),
+ collate_fn=dict(type=default_collate_fn))
+
+#######################################################################
+# PART 4 Scheduler & Optimizer #
+#######################################################################
+# optimizer
+optim_wrapper = dict(
+ type=AmpOptimWrapper,
+ optimizer=dict(
+ type=optim_type, lr=lr, betas=betas, weight_decay=weight_decay),
+ clip_grad=dict(max_norm=max_norm, error_if_nonfinite=False),
+ accumulative_counts=accumulative_counts,
+ loss_scale='dynamic',
+ dtype='float16')
+
+# learning policy
+# More information: https://github.com/open-mmlab/mmengine/blob/main/docs/en/tutorials/param_scheduler.md # noqa: E501
+param_scheduler = [
+ dict(
+ type=LinearLR,
+ start_factor=1e-5,
+ by_epoch=True,
+ begin=0,
+ end=warmup_ratio * max_epochs,
+ convert_to_iter_based=True),
+ dict(
+ type=CosineAnnealingLR,
+ eta_min=0.0,
+ by_epoch=True,
+ begin=warmup_ratio * max_epochs,
+ end=max_epochs,
+ convert_to_iter_based=True)
+]
+
+# train, val, test setting
+train_cfg = dict(type=TrainLoop, max_epochs=max_epochs)
+
+#######################################################################
+# PART 5 Runtime #
+#######################################################################
+# Log the dialogue periodically during the training process, optional
+custom_hooks = [
+ dict(type=DatasetInfoHook, tokenizer=tokenizer),
+ dict(
+ type=EvaluateChatHook,
+ tokenizer=tokenizer,
+ image_processor=image_processor,
+ every_n_iters=evaluation_freq,
+ evaluation_inputs=evaluation_inputs,
+ evaluation_images=evaluation_images,
+ system=SYSTEM,
+ prompt_template=prompt_template)
+]
+
+# configure default hooks
+default_hooks = dict(
+ # record the time of every iteration.
+ timer=dict(type=IterTimerHook),
+ # print log every 10 iterations.
+ logger=dict(type=LoggerHook, log_metric_by_epoch=False, interval=10),
+ # enable the parameter scheduler.
+ param_scheduler=dict(type=ParamSchedulerHook),
+ # save checkpoint per `save_steps`.
+ checkpoint=dict(
+ type=CheckpointHook,
+ by_epoch=False,
+ interval=save_steps,
+ max_keep_ckpts=save_total_limit),
+ # set sampler seed in distributed evrionment.
+ sampler_seed=dict(type=DistSamplerSeedHook),
+)
+
+# configure environment
+env_cfg = dict(
+ # whether to enable cudnn benchmark
+ cudnn_benchmark=False,
+ # set multi process parameters
+ mp_cfg=dict(mp_start_method='fork', opencv_num_threads=0),
+ # set distributed parameters
+ dist_cfg=dict(backend='nccl'),
+)
+
+# set visualizer
+visualizer = None
+
+# set log level
+log_level = 'INFO'
+
+# load from which checkpoint
+load_from = None
+
+# whether to resume training from the loaded checkpoint
+resume = False
+
+# Defaults to use random seed and disable `deterministic`
+randomness = dict(seed=None, deterministic=False)
+
+# set log processor
+log_processor = dict(by_epoch=False)
diff --git a/xtuner/configs/llava/llama3_8b_instruct_clip_vit_large_p14_336/README.md b/xtuner/configs/llava/llama3_8b_instruct_clip_vit_large_p14_336/README.md
new file mode 100644
index 000000000..f0112fe57
--- /dev/null
+++ b/xtuner/configs/llava/llama3_8b_instruct_clip_vit_large_p14_336/README.md
@@ -0,0 +1,424 @@
+# LLaVA-Llama-3-8B
+
+## Results
+
+
+
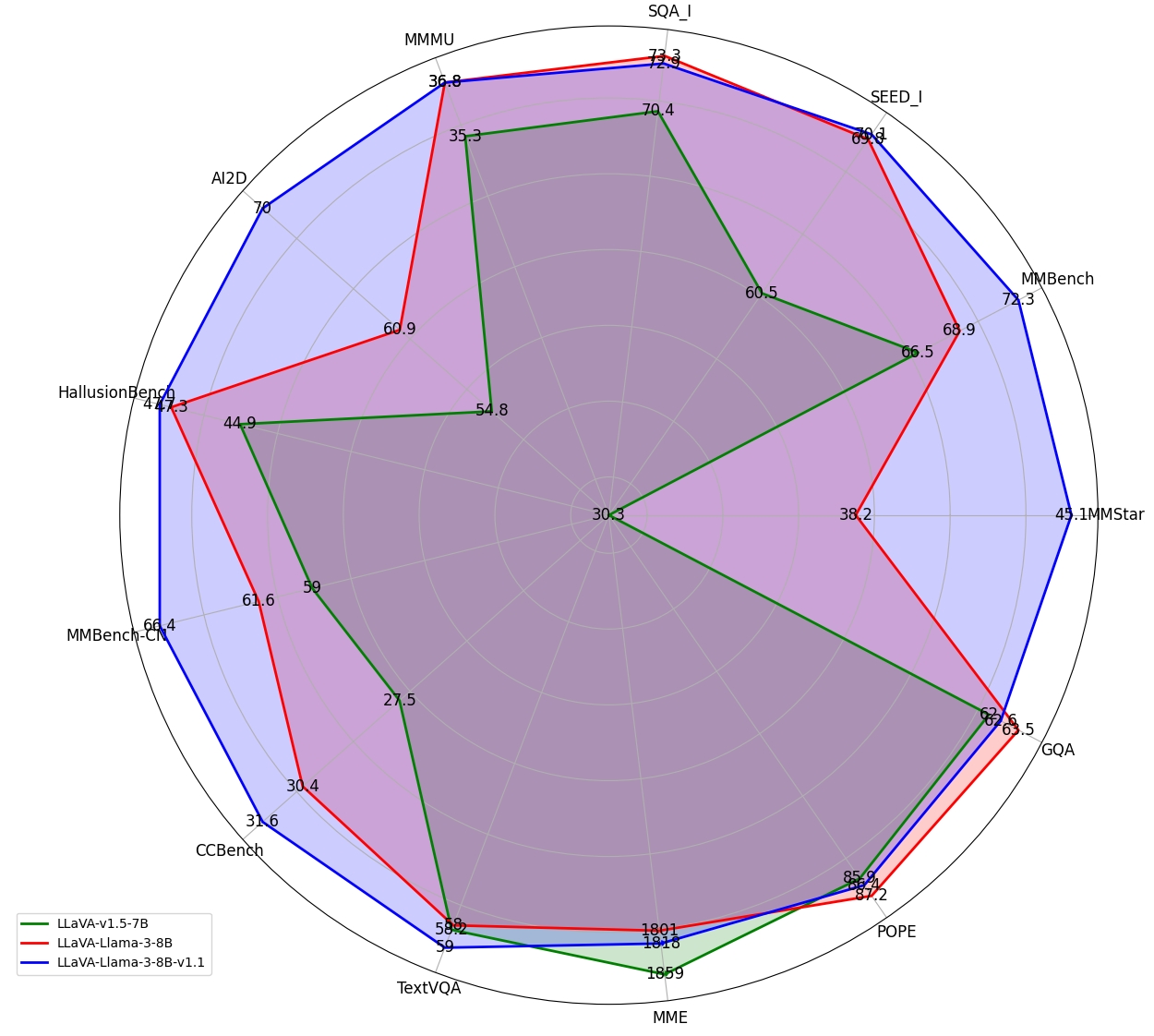
+
+
+| Model | MMBench Test (EN) | MMBench Test (CN) | CCBench Dev | MMMU Val | SEED-IMG | AI2D Test | ScienceQA Test | HallusionBench aAcc | POPE | GQA | TextVQA | MME | MMStar | Configs |
+| :-------------------- | :---------------: | :---------------: | :---------: | :-------: | :------: | :-------: | :------------: | :-----------------: | :--: | :--: | :-----: | :------: | :----: | :--------------------------------------------------------------------------------------------------------------------------------------------------------------------------------------------------------------------: |
+| LLaVA-v1.5-7B | 66.5 | 59.0 | 27.5 | 35.3 | 60.5 | 54.8 | 70.4 | 44.9 | 85.9 | 62.0 | 58.2 | 1511/348 | 30.3 | - |
+| LLaVA-Llama-3-8B | 68.9 | 61.6 | 30.4 | 36.8 | 69.8 | 60.9 | 73.3 | 47.3 | 87.2 | 63.5 | 58.0 | 1506/295 | 38.2 | [Pretrain](./pretrain/llava_llama3_8b_instruct_clip_vit_large_p14_336_e1_gpu8_pretrain.py) / [Fine-tune](./finetune/llava_llama3_8b_instruct_full_clip_vit_large_p14_336_lora_e1_gpu8_finetune.py) |
+| LLaVA-Llama-3-8B-v1.1 | 72.3 | 66.4 | 31.6 | 36.8 | 70.1 | 70.0 | 72.9 | 47.7 | 86.4 | 62.6 | 59.0 | 1469/349 | 45.1 | [Pretrain](./pretrain/llava_llama3_8b_instruct_clip_vit_large_p14_336_e1_gpu8_sharegpt4v_pretrain.py) / [Fine-tune](./finetune/llava_llama3_8b_instruct_full_clip_vit_large_p14_336_lora_e1_gpu8_internvl_finetune.py) |
+
+## Resources
+
+- LLaVA-Llama-3-8B-v1.1
+
+ - Official LLaVA format model (`xtuner/llava-llama-3-8b-v1_1-hf`): 🤗 [HuggingFace](https://huggingface.co/xtuner/llava-llama-3-8b-v1_1-hf) / 🤖 [ModelScope](https://modelscope.cn/models/xtuner/llava-llama-3-8b-v1_1-hf)
+ - HuggingFace LLaVA format model (`xtuner/llava-llama-3-8b-v1_1-transformers`): 🤗 [HuggingFace](https://huggingface.co/xtuner/llava-llama-3-8b-v1_1-transformers) / 🤖 [ModelScope](https://modelscope.cn/models/xtuner/llava-llama-3-8b-v1_1-transformers)
+ - XTuner LLaVA format model (`xtuner/llava-llama-3-8b-v1_1`): 🤗 [HuggingFace](https://huggingface.co/xtuner/llava-llama-3-8b-v1_1) / 🤖 [ModelScope](https://modelscope.cn/models/xtuner/llava-llama-3-8b-v1_1)
+ - GGUF model (`xtuner/llava-llama-3-8b-v1_1-gguf`): 🤗 [HuggingFace](https://huggingface.co/xtuner/llava-llama-3-8b-v1_1-gguf) / 🤖 [ModelScope](https://modelscope.cn/models/xtuner/llava-llama-3-8b-v1_1-gguf)
+ - Pretrained projector weights: 🤗 [HuggingFace](https://huggingface.co/xtuner/llava-llama-3-8b-v1_1-pretrain) / 🤖 [ModelScope](https://modelscope.cn/models/xtuner/llava-llama-3-8b-v1_1-pretrain)
+
+- LLaVA-Llama-3-8B
+
+ - Official LLaVA format model (`xtuner/llava-llama-3-8b-hf`): 🤗 [HuggingFace](https://huggingface.co/xtuner/llava-llama-3-8b-hf) / 🤖 [ModelScope](https://modelscope.cn/models/xtuner/llava-llama-3-8b-hf)
+ - HuggingFace LLaVA format model (`xtuner/llava-llama-3-8b-transformers`): 🤗 [HuggingFace](https://huggingface.co/xtuner/llava-llama-3-8b-transformers) / 🤖 [ModelScope](https://modelscope.cn/models/xtuner/llava-llama-3-8b-transformers)
+ - XTuner LLaVA format model (`xtuner/llava-llama-3-8b`): 🤗 [HuggingFace](https://huggingface.co/xtuner/llava-llama-3-8b) / 🤖 [ModelScope](https://modelscope.cn/models/xtuner/llava-llama-3-8b)
+ - Pretrained projector weights: 🤗 [HuggingFace](https://huggingface.co/xtuner/llava-llama-3-8b-pretrain) / 🤖 [ModelScope](https://modelscope.cn/models/xtuner/llava-llama-3-8b-pretrain)
+
+## Data Preparation
+
+### LLaVA dataset
+
+#### File structure
+
+```
+./data/llava_data
+├── LLaVA-Pretrain
+│ ├── blip_laion_cc_sbu_558k.json
+│ ├── blip_laion_cc_sbu_558k_meta.json
+│ └── images
+├── LLaVA-Instruct-150K
+│ └── llava_v1_5_mix665k.json
+└── llava_images
+ ├── coco
+ │ └── train2017
+ ├── gqa
+ │ └── images
+ ├── ocr_vqa
+ │ └── images
+ ├── textvqa
+ │ └── train_images
+ └── vg
+ ├── VG_100K
+ └── VG_100K_2
+```
+
+#### Pretrain
+
+LLaVA-Pretrain
+
+```shell
+# Make sure you have git-lfs installed (https://git-lfs.com)
+git lfs install
+git clone https://huggingface.co/datasets/liuhaotian/LLaVA-Pretrain --depth=1
+```
+
+#### Finetune
+
+1. Text data
+
+ 1. LLaVA-Instruct-150K
+
+ ```shell
+ # Make sure you have git-lfs installed (https://git-lfs.com)
+ git lfs install
+ git clone https://huggingface.co/datasets/liuhaotian/LLaVA-Instruct-150K --depth=1
+ ```
+
+2. Image data
+
+ 1. COCO (coco): [download url](http://images.cocodataset.org/zips/train2017.zip)
+
+ 2. GQA (gqa): [download url](https://downloads.cs.stanford.edu/nlp/data/gqa/images.zip)
+
+ 3. OCR-VQA (ocr_vqa): [download script](https://drive.google.com/drive/folders/1_GYPY5UkUy7HIcR0zq3ZCFgeZN7BAfm_?usp=sharing)
+
+ 1. ⚠️ Modify the name of OCR-VQA's images to keep the extension as `.jpg`!
+
+ ```shell
+ #!/bin/bash
+ ocr_vqa_path=""
+
+ find "$target_dir" -type f | while read file; do
+ extension="${file##*.}"
+ if [ "$extension" != "jpg" ]
+ then
+ cp -- "$file" "${file%.*}.jpg"
+ fi
+ done
+ ```
+
+ 4. TextVQA (textvqa): [download url](https://dl.fbaipublicfiles.com/textvqa/images/train_val_images.zip)
+
+ 5. VisualGenome (VG): [part1](https://cs.stanford.edu/people/rak248/VG_100K_2/images.zip), [part2](https://cs.stanford.edu/people/rak248/VG_100K_2/images2.zip)
+
+### ShareGPT4V dataset
+
+> Reference: https://github.com/InternLM/InternLM-XComposer/blob/main/projects/ShareGPT4V/docs/Data.md
+
+#### File structure
+
+```
+./data/sharegpt4v
+├── share-captioner_coco_lcs_sam_1246k_1107.json
+├── sharegpt4v_instruct_gpt4-vision_cap100k.json
+├── sharegpt4v_mix665k_cap23k_coco-ap9k_lcs3k_sam9k_div2k.json
+└── data
+ ├── sam
+ │ └── images
+ ├── share_textvqa
+ │ └── images
+ ├── web-celebrity
+ │ └── images
+ ├── web-landmark
+ │ └── images
+ ├── wikiart
+ │ └── images
+ ├── llava
+ │ └── llava_pretrain
+ │ └── images -> ../../../../llava_data/LLaVA-Pretrain/images
+ ├── coco -> ../../llava_data/llava_images/coco
+ ├── gqa -> ../../llava_data/llava_images/gqa
+ ├── ocr_vqa -> ../../llava_data/llava_images/ocr_vqa
+ ├── textvqa -> ../../llava_data/llava_images/textvqa
+ └── vg -> ../../llava_data/llava_images/vg
+```
+
+#### Download
+
+1. Text data
+
+ ```shell
+ wget https://huggingface.co/datasets/Lin-Chen/ShareGPT4V/blob/main/sharegpt4v_instruct_gpt4-vision_cap100k.json
+ wget https://huggingface.co/datasets/Lin-Chen/ShareGPT4V/blob/main/share-captioner_coco_lcs_sam_1246k_1107.json
+ wget https://huggingface.co/datasets/Lin-Chen/ShareGPT4V/blob/main/sharegpt4v_mix665k_cap23k_coco-ap9k_lcs3k_sam9k_div2k.json
+ ```
+
+2. Image data
+
+ 1. SAM (sam): [download url](https://drive.google.com/file/d/1dKumdOKSXtV7lIXdrG7jsIK_z2vZv2gs/view?usp=drive_link)
+
+ 2. ShareTextVQA (share_textvqa): [download url](https://drive.google.com/file/d/1f4v_3e1OJtyYqam1CEp6RenCNTU5_mG2/view?usp=share_link)
+
+ 3. Web-Celebrity (web-celebrity): [download url](https://drive.google.com/file/d/1-SB71C3j1mVg0kDDXwj2IWGEoBoRUD-J/view?usp=share_link)
+
+ 4. Web-Landmark (web-landmark): [download url](https://drive.google.com/file/d/1JpJkN7ZMA50xAhMx9O-rVb5yLhfGm3_o/view?usp=share_link)
+
+ 5. WikiArt (wikiart): [download url](https://drive.google.com/file/d/1FxB2Nw-vWUcTUSI_dBpPIykb-uGYoEqV/view?usp=share_link)
+
+ 6. llava, coco , gqa, ocr_vqa, textvqa, vg: Please refer to the preparation of LLaVA dataset.
+
+### InternVL-SFT
+
+> Reference: https://github.com/OpenGVLab/InternVL/tree/main/internvl_chat#prepare-training-datasets
+
+#### File structure
+
+```
+./data/internvl_sft
+├── sharegpt4v_instruct_gpt4-vision_cap100k.jsonl
+├── llava_instruct_150k_zh.jsonl
+├── sharegpt4v_mix665k_cap23k_coco-ap9k_lcs3k_sam9k_div2k.jsonl
+├── dvqa_train_200k.jsonl
+├── chartqa_train_18k.jsonl
+├── ai2d_train_12k.jsonl
+├── docvqa_train_10k.jsonl
+├── geoqa+.jsonl
+├── synthdog_en.jsonl
+└── data
+ ├── ai2d
+ │ ├── abc_images
+ │ └── images
+ ├── chartqa
+ │ ├── test
+ │ ├── train
+ │ └── val
+ ├── docvqa
+ │ ├── test
+ │ ├── train
+ │ └── val
+ ├── dvqa
+ │ └── images
+ ├── synthdog-en
+ │ └── images
+ ├── geoqa+
+ │ └── images
+ ├── llava
+ │ └── llava_pretrain
+ │ └── images -> ../../../../llava_data/LLaVA-Pretrain/images
+ ├── coco -> ../../llava_data/llava_images/coco
+ ├── gqa -> ../../llava_data/llava_images/gqa
+ ├── ocr_vqa -> ../../llava_data/llava_images/ocr_vqa
+ ├── textvqa -> ../../llava_data/llava_images/textvqa
+ ├── vg -> ../../llava_data/llava_images/vg
+ ├── sam -> ../../sharegpt4v/data/sam
+ ├── share_textvqa -> ../../sharegpt4v/data/share_textvqa
+ ├── web-celebrity -> ../../sharegpt4v/data/web-celebrity
+ ├── web-landmark -> ../../sharegpt4v/data/web-landmark
+ └── wikiart -> ../../sharegpt4v/data/wikiart
+```
+
+#### Download
+
+1. Text data
+
+ ```shell
+ wget https://huggingface.co/OpenGVLab/InternVL/resolve/main/playground.zip
+ unzip ./playground.zip
+ ```
+
+2. Image data
+
+ 1. AI2D (ai2d): [download url](https://drive.google.com/file/d/1dqqa3MnrxMXaU_K9JA6C83je32ibwdOY/view?usp=sharing)
+
+ 2. ChartQA (chartqa): [download url](https://huggingface.co/datasets/ahmed-masry/ChartQA/resolve/main/ChartQA%20Dataset.zip)
+
+ 3. DocVQA (docvqa): [train](https://datasets.cvc.uab.es/rrc/DocVQA/train.tar.gz), [val](https://datasets.cvc.uab.es/rrc/DocVQA/val.tar.gz), [test](https://datasets.cvc.uab.es/rrc/DocVQA/test.tar.gz)
+
+ 4. DVQA (dvqa): [download url](https://drive.google.com/file/d/1iKH2lTi1-QxtNUVRxTUWFvUvRHq6HAsZ/view)
+
+ 5. SynthDoG-EN (synthdog-en): [download url](https://huggingface.co/OpenGVLab/InternVL/resolve/main/synthdog-en-images.zip)
+
+ 6. GeoQA+ (geoqa+): [download url](https://huggingface.co/OpenGVLab/InternVL/resolve/main/geoqa%2B_images.zip)
+
+ 7. llava, coco, gqa, ocr_vqa, textvqa, vg: Please refer to the preparation of LLaVA dataset.
+
+ 8. sam, share_textvqa, web-celebrity, web-landmark, wikiart: Please refer to the preparation of ShareGPT4V dataset.
+
+## Training
+
+### LLaVA-LLama-3-8B
+
+1. Pretrain (saved by default in `./work_dirs/llava_llama3_8b_instruct_clip_vit_large_p14_336_e1_gpu8_pretrain/`)
+
+```bash
+NPROC_PER_NODE=8 xtuner train llava_llama3_8b_instruct_clip_vit_large_p14_336_e1_gpu8_pretrain --deepspeed deepspeed_zero2 --seed 1024
+```
+
+2. Fine-tune (saved by default in `./work_dirs/llava_llama3_8b_instruct_full_clip_vit_large_p14_336_lora_e1_gpu8_finetune/`)
+
+```bash
+NPROC_PER_NODE=8 xtuner train llava_llama3_8b_instruct_full_clip_vit_large_p14_336_lora_e1_gpu8_finetune --deepspeed deepspeed_zero2 --seed 1024
+```
+
+### LLaVA-LLama-3-8B-v1.1 (Recommended)
+
+1. Pretrain (saved by default in `./work_dirs/llava_llama3_8b_instruct_clip_vit_large_p14_336_e1_gpu8_sharegpt4v_pretrain/`)
+
+```bash
+NPROC_PER_NODE=8 xtuner train llava_llama3_8b_instruct_clip_vit_large_p14_336_e1_gpu8_sharegpt4v_pretrain --deepspeed deepspeed_zero2 --seed 1024
+```
+
+2. Fine-tune (saved by default in `./work_dirs/llava_llama3_8b_instruct_full_clip_vit_large_p14_336_lora_e1_gpu8_internvl_finetune/`)
+
+```bash
+NPROC_PER_NODE=8 xtuner train llava_llama3_8b_instruct_full_clip_vit_large_p14_336_lora_e1_gpu8_internvl_finetune --deepspeed deepspeed_zero2 --seed 1024
+```
+
+### Singlg card?
+
+XTuner also supports single-card training for LLaVA-Llama-3-8B (Youth Edition), requiring only a single card with 20GB to complete the entire process of multi-modal training.
+
+1. Pretrain (saved by default in `./work_dirs/llava_llama3_8b_instruct_quant_clip_vit_large_p14_336_e1_gpu1_pretrain/`)
+
+```bash
+xtuner train llava_llama3_8b_instruct_quant_clip_vit_large_p14_336_e1_gpu1_pretrain --deepspeed deepspeed_zero2 --seed 1024
+```
+
+2. Fine-tune (saved by default in `./work_dirs/llava_llama3_8b_instruct_qlora_clip_vit_large_p14_336_e1_gpu1_finetune/`)
+
+```bash
+xtuner train llava_llama3_8b_instruct_qlora_clip_vit_large_p14_336_e1_gpu1_finetune --deepspeed deepspeed_zero2 --seed 1024
+```
+
+## Model Conversion
+
+After training, we will obtain a set of weights (*i.e.*, `iter_xxx.pth`), which are not in the universal HuggingFace format. We first need to convert them to the LLaVA model.
+
+### Convert `.pth` file to LLaVA model in xtuner format ([xtuner/llava-llama-3-8b-v1_1](https://huggingface.co/xtuner/llava-llama-3-8b-v1_1))
+
+```bash
+xtuner convert pth_to_hf $FINETUNE_CFG $PTH_PATH $SAVE_PATH
+# e.g., xtuner convert pth_to_hf llava_llama3_8b_instruct_full_clip_vit_large_p14_336_lora_e1_gpu8_internvl_finetune ./iter_39620.pth ./iter_39620_xtuner
+```
+
+At this point, we have obtained the relevant model (LLM or the corresponding LoRA).
+If you use the default configuration of LLaVA-Llama-3-8B, you will obtain the following file structure after converting.
+It includes the full-finetuned LLM weights, projector weights, and LoRA weights of the visual encoder.
+
+```
+./iter_39620_xtuner
+├── config.json
+├── generation_config.json
+├── model-00001-of-00009.safetensors
+├── model-00002-of-00009.safetensors
+├── model-00003-of-00009.safetensors
+├── model-00004-of-00009.safetensors
+├── model-00005-of-00009.safetensors
+├── model-00006-of-00009.safetensors
+├── model-00007-of-00009.safetensors
+├── model-00008-of-00009.safetensors
+├── model-00009-of-00009.safetensors
+├── model.safetensors.index.json
+├── projector
+│ ├── config.json
+│ ├── configuration_projector.py
+│ ├── modeling_projector.py
+│ └── model.safetensors
+├── special_tokens_map.json
+├── tokenizer_config.json
+├── tokenizer.json
+└── visual_encoder_adapter
+ ├── adapter_config.json
+ ├── adapter_model.safetensors
+ └── README.md
+```
+
+LLaVA model in xtuner format can engage in conversation using xtuner chat, by
+
+```bash
+xtuner chat ./iter_39620_xtuner \
+ --visual-encoder openai/clip-vit-large-patch14-336 \
+ --llava ./iter_39620_xtuner \
+ --prompt-template llama3_chat \
+ --image $IMAGE_PATH
+```
+
+and in MMBench evaluation, by
+
+```bash
+xtuner mmbench ./iter_39620_xtuner \
+ --visual-encoder openai/clip-vit-large-patch14-336 \
+ --llava ./iter_39620_xtuner \
+ --prompt-template llama3_chat \
+ --data-path $DATA_PATH \
+ --work-dir $RESULT_PATH
+```
+
+Here, `$DATA_PATH` refers to one of the mmbench datasets. You can download the expected data by
+
+```bash
+wget https://opencompass.openxlab.space/utils/VLMEval/MMBench_DEV_EN.tsv
+wget https://opencompass.openxlab.space/utils/VLMEval/MMBench_TEST_EN.tsv
+wget https://opencompass.openxlab.space/utils/VLMEval/MMBench_DEV_CN.tsv
+wget https://opencompass.openxlab.space/utils/VLMEval/MMBench_TEST_CN.tsv
+wget https://opencompass.openxlab.space/utils/VLMEval/CCBench.tsv
+```
+
+### Convert `.pth` file to LLaVA model in official format ([xtuner/llava-llama-3-8b-v1_1-hf](https://huggingface.co/xtuner/llava-llama-3-8b-v1_1-hf))
+
+```bash
+xtuner convert pth_to_hf $FINETUNE_CFG $PTH_PATH $SAVE_PATH --save-format official
+# e.g., xtuner convert pth_to_hf llava_llama3_8b_instruct_full_clip_vit_large_p14_336_lora_e1_gpu8_internvl_finetune ./iter_39620.pth ./iter_39620_official --save-format official
+```
+
+Here, the converted LLaVA model in official LLaVA format is saved to `./iter_39620_official`.
+
+```
+./iter_39620_official
+├── config.json
+├── generation_config.json
+├── model-00001-of-00009.safetensors
+├── model-00002-of-00009.safetensors
+├── model-00003-of-00009.safetensors
+├── model-00004-of-00009.safetensors
+├── model-00005-of-00009.safetensors
+├── model-00006-of-00009.safetensors
+├── model-00007-of-00009.safetensors
+├── model-00008-of-00009.safetensors
+├── model-00009-of-00009.safetensors
+├── model.safetensors.index.json
+├── preprocessor_config.json
+├── special_tokens_map.json
+├── tokenizer_config.json
+└── tokenizer.json
+```
+
+### Convert `.pth` file to LLaVA model in HuggingFace format ([xtuner/llava-llama-3-8b-v1_1-transformers](https://huggingface.co/xtuner/llava-llama-3-8b-v1_1-transformers))
+
+```bash
+xtuner convert pth_to_hf $FINETUNE_CFG $PTH_PATH $SAVE_PATH --save-format huggingface
+# e.g., xtuner convert pth_to_hf llava_llama3_8b_instruct_full_clip_vit_large_p14_336_lora_e1_gpu8_internvl_finetune ./iter_39620.pth ./iter_39620_huggingface --save-format huggingface
+```
+
+Here, the converted LLaVA model in HuggingFace LLaVA format is saved to `./iter_39620_huggingface`.
+
+```
+./iter_39620_huggingface
+├── config.json
+├── generation_config.json
+├── model-00001-of-00004.safetensors
+├── model-00002-of-00004.safetensors
+├── model-00003-of-00004.safetensors
+├── model-00004-of-00004.safetensors
+├── model.safetensors.index.json
+├── preprocessor_config.json
+├── special_tokens_map.json
+├── tokenizer_config.json
+└── tokenizer.json
+```
+
+## Chat
+
+- XTuner LLaVA format [docs](https://huggingface.co/xtuner/llava-llama-3-8b-v1_1#quickstart)
+- Official LLaVA format [docs](https://huggingface.co/xtuner/llava-llama-3-8b-v1_1-hf#quickstart)
+- HuggingFace LLaVA format [docs](https://huggingface.co/xtuner/llava-llama-3-8b-v1_1-transformers#quickstart)
+- GGUF format [docs](https://huggingface.co/xtuner/llava-llama-3-8b-v1_1-gguf#quickstart)
+
+## Deployment
+
+[LMDeploy](https://github.com/InternLM/lmdeploy) now supports the deployment of official LLaVA format models (e.g.,[xtuner/llava-llama-3-8b-v1_1-hf](https://huggingface.co/xtuner/llava-llama-3-8b-v1_1-hf)). For specifics, please refer to [here](https://huggingface.co/xtuner/llava-llama-3-8b-v1_1-hf#chat-by-lmdeploy).
diff --git a/xtuner/configs/llava/llama3_8b_instruct_clip_vit_large_p14_336/convert_xtuner_weights_to_hf.py b/xtuner/configs/llava/llama3_8b_instruct_clip_vit_large_p14_336/convert_xtuner_weights_to_hf.py
new file mode 100644
index 000000000..17c5eb2ef
--- /dev/null
+++ b/xtuner/configs/llava/llama3_8b_instruct_clip_vit_large_p14_336/convert_xtuner_weights_to_hf.py
@@ -0,0 +1,143 @@
+# Copyright (c) OpenMMLab. All rights reserved.
+# Modified from https://github.com/huggingface/transformers/blob/v4.40.1/src/transformers/models/llava/convert_llava_weights_to_hf.py # noqa: E501
+import argparse
+
+import torch
+from safetensors import safe_open
+from transformers import (AddedToken, AutoConfig, AutoModelForCausalLM,
+ CLIPImageProcessor, CLIPVisionModel,
+ LlamaTokenizerFast, LlavaConfig,
+ LlavaForConditionalGeneration, LlavaProcessor)
+
+KEYS_TO_MODIFY_MAPPING_LLM = {
+ 'model': 'language_model.model',
+ 'lm_head': 'language_model.lm_head',
+}
+KEYS_TO_MODIFY_MAPPING_VIT = {
+ 'vision_model': 'vision_tower.vision_model',
+}
+KEYS_TO_MODIFY_MAPPING_PROJECTOR = {
+ 'model.0': 'multi_modal_projector.linear_1',
+ 'model.2': 'multi_modal_projector.linear_2',
+}
+
+
+def convert_state_dict_to_hf(state_dict, mapping):
+ new_state_dict = {}
+ for key, value in state_dict.items():
+ if key.endswith('.inv_freq'):
+ continue
+ for key_to_modify, new_key in mapping.items():
+ if key_to_modify in key:
+ key = key.replace(key_to_modify, new_key)
+
+ new_state_dict[key] = value
+ return new_state_dict
+
+
+def convert_to_hf(text_model_id, vision_model_id, projector_weight, save_path):
+ torch.set_default_dtype(torch.float16)
+ text_config = AutoConfig.from_pretrained(
+ text_model_id, trust_remote_code=True)
+ vision_config = AutoConfig.from_pretrained(vision_model_id)
+ if hasattr(vision_config, 'vision_config'):
+ vision_config = vision_config.vision_config
+
+ tokenizer = LlamaTokenizerFast.from_pretrained(text_model_id)
+ tokenizer.add_tokens(
+ AddedToken('', special=True, normalized=False),
+ special_tokens=True)
+ tokenizer.add_special_tokens({'pad_token': ''})
+
+ image_processor = CLIPImageProcessor.from_pretrained(vision_model_id)
+
+ processor = LlavaProcessor(
+ tokenizer=tokenizer, image_processor=image_processor)
+
+ config = LlavaConfig(
+ text_config=text_config,
+ vision_config=vision_config,
+ attn_implementation='eager')
+
+ with torch.device('meta'):
+ model = LlavaForConditionalGeneration(config)
+
+ # Pad to 64 for performance reasons
+ pad_shape = 64
+
+ projector_state_dict = {}
+ with safe_open(projector_weight, framework='pt', device='cpu') as f:
+ for key in f.keys():
+ projector_state_dict[key] = f.get_tensor(key)
+
+ ori_llm = AutoModelForCausalLM.from_pretrained(
+ text_model_id, trust_remote_code=True)
+ ori_vit = CLIPVisionModel.from_pretrained(vision_model_id)
+
+ llm_state_dict = ori_llm.state_dict()
+ vit_state_dict = ori_vit.state_dict()
+
+ projector_state_dict = convert_state_dict_to_hf(
+ projector_state_dict, KEYS_TO_MODIFY_MAPPING_PROJECTOR)
+ llm_state_dict = convert_state_dict_to_hf(llm_state_dict,
+ KEYS_TO_MODIFY_MAPPING_LLM)
+ vit_state_dict = convert_state_dict_to_hf(vit_state_dict,
+ KEYS_TO_MODIFY_MAPPING_VIT)
+ state_dict = {**projector_state_dict, **llm_state_dict, **vit_state_dict}
+ model.load_state_dict(state_dict, strict=True, assign=True)
+
+ pre_expansion_embeddings = \
+ model.language_model.model.embed_tokens.weight.data
+ mu = torch.mean(pre_expansion_embeddings, dim=0).float()
+ n = pre_expansion_embeddings.size()[0]
+ sigma = ((pre_expansion_embeddings - mu).T
+ @ (pre_expansion_embeddings - mu)) / n
+ dist = torch.distributions.multivariate_normal.MultivariateNormal(
+ mu, covariance_matrix=1e-5 * sigma)
+
+ # We add an image token so we resize the model
+ ori_vocab_size = config.text_config.vocab_size
+ tokenizer_vocab_size = tokenizer.encode('')[-1]
+ added_token = tokenizer_vocab_size - ori_vocab_size
+
+ if added_token > 0:
+ model.resize_token_embeddings(ori_vocab_size + added_token, pad_shape)
+ model.language_model.model.embed_tokens.weight.data[
+ ori_vocab_size:] = torch.stack(
+ tuple(dist.sample()
+ for _ in range(model.language_model.model.embed_tokens.
+ weight.data[ori_vocab_size:].shape[0])),
+ dim=0,
+ )
+ model.language_model.lm_head.weight.data[
+ ori_vocab_size:] = torch.stack(
+ tuple(dist.sample()
+ for _ in range(model.language_model.lm_head.weight.
+ data[ori_vocab_size:].shape[0])),
+ dim=0,
+ )
+
+ model.config.image_token_index = tokenizer.encode('')[-1]
+ model.config.pad_token_id = tokenizer.encode('')[-1]
+
+ if ori_vit.__class__.__name__ == 'SiglipVisionModel':
+ model.config.vision_feature_select_strategy = 'full'
+
+ model.save_pretrained(save_path)
+ processor.save_pretrained(save_path)
+ print(f'Saved to {save_path}')
+
+
+def main():
+ parser = argparse.ArgumentParser()
+ parser.add_argument('--text_model_id')
+ parser.add_argument('--vision_model_id')
+ parser.add_argument('--projector_weight')
+ parser.add_argument('--save_path')
+ args = parser.parse_args()
+ convert_to_hf(args.text_model_id, args.vision_model_id,
+ args.projector_weight, args.save_path)
+
+
+if __name__ == '__main__':
+ main()
diff --git a/xtuner/configs/llava/llama3_8b_instruct_clip_vit_large_p14_336/convert_xtuner_weights_to_llava.py b/xtuner/configs/llava/llama3_8b_instruct_clip_vit_large_p14_336/convert_xtuner_weights_to_llava.py
new file mode 100644
index 000000000..8a1df6233
--- /dev/null
+++ b/xtuner/configs/llava/llama3_8b_instruct_clip_vit_large_p14_336/convert_xtuner_weights_to_llava.py
@@ -0,0 +1,106 @@
+# Copyright (c) OpenMMLab. All rights reserved.
+import argparse
+
+import torch
+
+try:
+ from llava.model import LlavaConfig, LlavaLlamaForCausalLM
+ from llava.utils import disable_torch_init
+except ImportError:
+ raise ImportError(
+ 'Please install llava with '
+ '`pip install git+https://github.com/haotian-liu/LLaVA.git '
+ '--no-deps`.')
+from safetensors import safe_open
+from transformers import (AutoConfig, AutoModelForCausalLM, AutoTokenizer,
+ CLIPImageProcessor, CLIPVisionModel)
+
+KEYS_TO_MODIFY_MAPPING_VIT = {
+ 'vision_model': 'model.vision_tower.vision_tower.vision_model',
+}
+KEYS_TO_MODIFY_MAPPING_PROJECTOR = {
+ 'model.0': 'model.mm_projector.0',
+ 'model.2': 'model.mm_projector.2',
+}
+
+
+def convert_state_dict_to_hf(state_dict, mapping):
+ new_state_dict = {}
+ for key, value in state_dict.items():
+ if key.endswith('.inv_freq'):
+ continue
+ for key_to_modify, new_key in mapping.items():
+ if key_to_modify in key:
+ key = key.replace(key_to_modify, new_key)
+ new_state_dict[key] = value
+ return new_state_dict
+
+
+def convert_to_llava(text_model_id, vision_model_id, projector_weight,
+ save_path):
+ disable_torch_init()
+ torch.set_default_dtype(torch.float16)
+
+ projector_state_dict = {}
+ with safe_open(projector_weight, framework='pt', device='cpu') as f:
+ for key in f.keys():
+ projector_state_dict[key] = f.get_tensor(key)
+
+ ori_llm = AutoModelForCausalLM.from_pretrained(
+ text_model_id, trust_remote_code=True, device_map='auto')
+ ori_vit = CLIPVisionModel.from_pretrained(vision_model_id)
+ llm_state_dict = ori_llm.state_dict()
+ vit_state_dict = ori_vit.state_dict()
+
+ projector_state_dict = convert_state_dict_to_hf(
+ projector_state_dict, KEYS_TO_MODIFY_MAPPING_PROJECTOR)
+ vit_state_dict = convert_state_dict_to_hf(vit_state_dict,
+ KEYS_TO_MODIFY_MAPPING_VIT)
+ state_dict = {**projector_state_dict, **llm_state_dict, **vit_state_dict}
+
+ tokenizer = AutoTokenizer.from_pretrained(text_model_id)
+ text_config = AutoConfig.from_pretrained(
+ text_model_id, trust_remote_code=True)
+
+ ori_config = text_config.__dict__.copy()
+ ori_config.update(
+ dict(
+ image_aspect_ratio='pad',
+ mm_hidden_size=ori_vit.config.hidden_size,
+ mm_projector_type='mlp2x_gelu',
+ mm_use_im_patch_token=False,
+ mm_use_im_start_end=False,
+ mm_vision_select_feature='patch',
+ mm_vision_select_layer=-2,
+ mm_vision_tower=vision_model_id,
+ unfreeze_mm_vision_tower=True,
+ model_type='llava',
+ use_cache=True,
+ use_mm_proj=True))
+ config = LlavaConfig(**ori_config)
+
+ with torch.device('meta'):
+ model = LlavaLlamaForCausalLM(config)
+
+ image_processor = CLIPImageProcessor.from_pretrained(vision_model_id)
+
+ model.load_state_dict(state_dict, strict=True, assign=True)
+ model.save_pretrained(save_path, max_shard_size='2GB')
+ image_processor.save_pretrained(save_path)
+ tokenizer.save_pretrained(save_path)
+ print(f'Saved to {save_path}')
+
+
+def main():
+ parser = argparse.ArgumentParser()
+ parser.add_argument('--text_model_id')
+ parser.add_argument('--vision_model_id')
+ parser.add_argument('--projector_weight')
+ parser.add_argument('--save_path')
+ args = parser.parse_args()
+ convert_to_llava(args.text_model_id, args.vision_model_id,
+ args.projector_weight, args.save_path)
+
+
+if __name__ == '__main__':
+ main()
diff --git a/xtuner/configs/llava/llama3_8b_instruct_clip_vit_large_p14_336/finetune/llava_llama3_8b_instruct_full_clip_vit_large_p14_336_e1_gpu8_finetune.py b/xtuner/configs/llava/llama3_8b_instruct_clip_vit_large_p14_336/finetune/llava_llama3_8b_instruct_full_clip_vit_large_p14_336_e1_gpu8_finetune.py
new file mode 100644
index 000000000..6db8ed31b
--- /dev/null
+++ b/xtuner/configs/llava/llama3_8b_instruct_clip_vit_large_p14_336/finetune/llava_llama3_8b_instruct_full_clip_vit_large_p14_336_e1_gpu8_finetune.py
@@ -0,0 +1,205 @@
+# Copyright (c) OpenMMLab. All rights reserved.
+from mmengine.hooks import (CheckpointHook, DistSamplerSeedHook, IterTimerHook,
+ LoggerHook, ParamSchedulerHook)
+from mmengine.optim import AmpOptimWrapper, CosineAnnealingLR, LinearLR
+from torch.optim import AdamW
+from transformers import (AutoModelForCausalLM, AutoTokenizer,
+ CLIPImageProcessor, CLIPVisionModel)
+
+from xtuner.dataset import LLaVADataset
+from xtuner.dataset.collate_fns import default_collate_fn
+from xtuner.dataset.map_fns import llava_map_fn, template_map_fn_factory
+from xtuner.dataset.samplers import LengthGroupedSampler
+from xtuner.engine.hooks import DatasetInfoHook, EvaluateChatHook
+from xtuner.engine.runner import TrainLoop
+from xtuner.model import LLaVAModel
+from xtuner.utils import PROMPT_TEMPLATE
+
+#######################################################################
+# PART 1 Settings #
+#######################################################################
+# Model
+llm_name_or_path = 'meta-llama/Meta-Llama-3-8B-Instruct'
+visual_encoder_name_or_path = 'openai/clip-vit-large-patch14-336'
+# Specify the pretrained pth
+pretrained_pth = './work_dirs/llava_llama3_8b_instruct_clip_vit_large_p14_336_e1_gpu8_pretrain/iter_2181.pth' # noqa: E501
+
+# Data
+data_root = './data/llava_data/'
+data_path = data_root + 'LLaVA-Instruct-150K/llava_v1_5_mix665k.json'
+image_folder = data_root + 'llava_images'
+prompt_template = PROMPT_TEMPLATE.llama3_chat
+max_length = int(2048 - (336 / 14)**2)
+
+# Scheduler & Optimizer
+batch_size = 8 # per_device
+accumulative_counts = 2
+dataloader_num_workers = 4
+max_epochs = 1
+optim_type = AdamW
+lr = 2e-5
+betas = (0.9, 0.999)
+weight_decay = 0
+max_norm = 1 # grad clip
+warmup_ratio = 0.03
+
+# Save
+save_steps = 1000
+save_total_limit = 2 # Maximum checkpoints to keep (-1 means unlimited)
+
+# Evaluate the generation performance during the training
+evaluation_freq = 1000
+SYSTEM = ''
+evaluation_images = 'https://llava-vl.github.io/static/images/view.jpg'
+evaluation_inputs = ['请描述一下这张照片', 'Please describe this picture']
+
+#######################################################################
+# PART 2 Model & Tokenizer & Image Processor #
+#######################################################################
+tokenizer = dict(
+ type=AutoTokenizer.from_pretrained,
+ pretrained_model_name_or_path=llm_name_or_path,
+ trust_remote_code=True,
+ padding_side='right')
+
+image_processor = dict(
+ type=CLIPImageProcessor.from_pretrained,
+ pretrained_model_name_or_path=visual_encoder_name_or_path,
+ trust_remote_code=True)
+
+model = dict(
+ type=LLaVAModel,
+ freeze_llm=False,
+ freeze_visual_encoder=True,
+ pretrained_pth=pretrained_pth,
+ llm=dict(
+ type=AutoModelForCausalLM.from_pretrained,
+ pretrained_model_name_or_path=llm_name_or_path,
+ trust_remote_code=True),
+ visual_encoder=dict(
+ type=CLIPVisionModel.from_pretrained,
+ pretrained_model_name_or_path=visual_encoder_name_or_path))
+
+#######################################################################
+# PART 3 Dataset & Dataloader #
+#######################################################################
+llava_dataset = dict(
+ type=LLaVADataset,
+ data_path=data_path,
+ image_folder=image_folder,
+ tokenizer=tokenizer,
+ image_processor=image_processor,
+ dataset_map_fn=llava_map_fn,
+ template_map_fn=dict(
+ type=template_map_fn_factory, template=prompt_template),
+ max_length=max_length,
+ pad_image_to_square=True)
+
+train_dataloader = dict(
+ batch_size=batch_size,
+ num_workers=dataloader_num_workers,
+ pin_memory=True,
+ dataset=llava_dataset,
+ sampler=dict(
+ type=LengthGroupedSampler,
+ length_property='modality_length',
+ per_device_batch_size=batch_size * accumulative_counts),
+ collate_fn=dict(type=default_collate_fn))
+
+#######################################################################
+# PART 4 Scheduler & Optimizer #
+#######################################################################
+# optimizer
+optim_wrapper = dict(
+ type=AmpOptimWrapper,
+ optimizer=dict(
+ type=optim_type, lr=lr, betas=betas, weight_decay=weight_decay),
+ clip_grad=dict(max_norm=max_norm, error_if_nonfinite=False),
+ accumulative_counts=accumulative_counts,
+ loss_scale='dynamic',
+ dtype='float16')
+
+# learning policy
+# More information: https://github.com/open-mmlab/mmengine/blob/main/docs/en/tutorials/param_scheduler.md # noqa: E501
+param_scheduler = [
+ dict(
+ type=LinearLR,
+ start_factor=1e-5,
+ by_epoch=True,
+ begin=0,
+ end=warmup_ratio * max_epochs,
+ convert_to_iter_based=True),
+ dict(
+ type=CosineAnnealingLR,
+ eta_min=0.0,
+ by_epoch=True,
+ begin=warmup_ratio * max_epochs,
+ end=max_epochs,
+ convert_to_iter_based=True)
+]
+
+# train, val, test setting
+train_cfg = dict(type=TrainLoop, max_epochs=max_epochs)
+
+#######################################################################
+# PART 5 Runtime #
+#######################################################################
+# Log the dialogue periodically during the training process, optional
+custom_hooks = [
+ dict(type=DatasetInfoHook, tokenizer=tokenizer),
+ dict(
+ type=EvaluateChatHook,
+ tokenizer=tokenizer,
+ image_processor=image_processor,
+ every_n_iters=evaluation_freq,
+ evaluation_inputs=evaluation_inputs,
+ evaluation_images=evaluation_images,
+ system=SYSTEM,
+ prompt_template=prompt_template)
+]
+
+# configure default hooks
+default_hooks = dict(
+ # record the time of every iteration.
+ timer=dict(type=IterTimerHook),
+ # print log every 10 iterations.
+ logger=dict(type=LoggerHook, log_metric_by_epoch=False, interval=10),
+ # enable the parameter scheduler.
+ param_scheduler=dict(type=ParamSchedulerHook),
+ # save checkpoint per `save_steps`.
+ checkpoint=dict(
+ type=CheckpointHook,
+ by_epoch=False,
+ interval=save_steps,
+ max_keep_ckpts=save_total_limit),
+ # set sampler seed in distributed evrionment.
+ sampler_seed=dict(type=DistSamplerSeedHook),
+)
+
+# configure environment
+env_cfg = dict(
+ # whether to enable cudnn benchmark
+ cudnn_benchmark=False,
+ # set multi process parameters
+ mp_cfg=dict(mp_start_method='fork', opencv_num_threads=0),
+ # set distributed parameters
+ dist_cfg=dict(backend='nccl'),
+)
+
+# set visualizer
+visualizer = None
+
+# set log level
+log_level = 'INFO'
+
+# load from which checkpoint
+load_from = None
+
+# whether to resume training from the loaded checkpoint
+resume = False
+
+# Defaults to use random seed and disable `deterministic`
+randomness = dict(seed=None, deterministic=False)
+
+# set log processor
+log_processor = dict(by_epoch=False)
diff --git a/xtuner/configs/llava/llama3_8b_instruct_clip_vit_large_p14_336/finetune/llava_llama3_8b_instruct_full_clip_vit_large_p14_336_lora_e1_gpu8_finetune.py b/xtuner/configs/llava/llama3_8b_instruct_clip_vit_large_p14_336/finetune/llava_llama3_8b_instruct_full_clip_vit_large_p14_336_lora_e1_gpu8_finetune.py
new file mode 100644
index 000000000..e35984b5e
--- /dev/null
+++ b/xtuner/configs/llava/llama3_8b_instruct_clip_vit_large_p14_336/finetune/llava_llama3_8b_instruct_full_clip_vit_large_p14_336_lora_e1_gpu8_finetune.py
@@ -0,0 +1,208 @@
+# Copyright (c) OpenMMLab. All rights reserved.
+from mmengine.hooks import (CheckpointHook, DistSamplerSeedHook, IterTimerHook,
+ LoggerHook, ParamSchedulerHook)
+from mmengine.optim import AmpOptimWrapper, CosineAnnealingLR, LinearLR
+from peft import LoraConfig
+from torch.optim import AdamW
+from transformers import (AutoModelForCausalLM, AutoTokenizer,
+ CLIPImageProcessor, CLIPVisionModel)
+
+from xtuner.dataset import LLaVADataset
+from xtuner.dataset.collate_fns import default_collate_fn
+from xtuner.dataset.map_fns import llava_map_fn, template_map_fn_factory
+from xtuner.dataset.samplers import LengthGroupedSampler
+from xtuner.engine.hooks import DatasetInfoHook, EvaluateChatHook
+from xtuner.engine.runner import TrainLoop
+from xtuner.model import LLaVAModel
+from xtuner.utils import PROMPT_TEMPLATE
+
+#######################################################################
+# PART 1 Settings #
+#######################################################################
+# Model
+llm_name_or_path = 'meta-llama/Meta-Llama-3-8B-Instruct'
+visual_encoder_name_or_path = 'openai/clip-vit-large-patch14-336'
+# Specify the pretrained pth
+pretrained_pth = './work_dirs/llava_llama3_8b_instruct_clip_vit_large_p14_336_e1_gpu8_pretrain/iter_2181.pth' # noqa: E501
+
+# Data
+data_root = './data/llava_data/'
+data_path = data_root + 'LLaVA-Instruct-150K/llava_v1_5_mix665k.json'
+image_folder = data_root + 'llava_images'
+prompt_template = PROMPT_TEMPLATE.llama3_chat
+max_length = int(2048 - (336 / 14)**2)
+
+# Scheduler & Optimizer
+batch_size = 8 # per_device
+accumulative_counts = 2
+dataloader_num_workers = 4
+max_epochs = 1
+optim_type = AdamW
+lr = 2e-5
+betas = (0.9, 0.999)
+weight_decay = 0
+max_norm = 1 # grad clip
+warmup_ratio = 0.03
+
+# Save
+save_steps = 1000
+save_total_limit = 2 # Maximum checkpoints to keep (-1 means unlimited)
+
+# Evaluate the generation performance during the training
+evaluation_freq = 1000
+SYSTEM = ''
+evaluation_images = 'https://llava-vl.github.io/static/images/view.jpg'
+evaluation_inputs = ['请描述一下这张照片', 'Please describe this picture']
+
+#######################################################################
+# PART 2 Model & Tokenizer & Image Processor #
+#######################################################################
+tokenizer = dict(
+ type=AutoTokenizer.from_pretrained,
+ pretrained_model_name_or_path=llm_name_or_path,
+ trust_remote_code=True,
+ padding_side='right')
+
+image_processor = dict(
+ type=CLIPImageProcessor.from_pretrained,
+ pretrained_model_name_or_path=visual_encoder_name_or_path,
+ trust_remote_code=True)
+
+model = dict(
+ type=LLaVAModel,
+ freeze_llm=False,
+ freeze_visual_encoder=True,
+ pretrained_pth=pretrained_pth,
+ llm=dict(
+ type=AutoModelForCausalLM.from_pretrained,
+ pretrained_model_name_or_path=llm_name_or_path,
+ trust_remote_code=True),
+ visual_encoder=dict(
+ type=CLIPVisionModel.from_pretrained,
+ pretrained_model_name_or_path=visual_encoder_name_or_path),
+ visual_encoder_lora=dict(
+ type=LoraConfig, r=64, lora_alpha=16, lora_dropout=0.05, bias='none'))
+
+#######################################################################
+# PART 3 Dataset & Dataloader #
+#######################################################################
+llava_dataset = dict(
+ type=LLaVADataset,
+ data_path=data_path,
+ image_folder=image_folder,
+ tokenizer=tokenizer,
+ image_processor=image_processor,
+ dataset_map_fn=llava_map_fn,
+ template_map_fn=dict(
+ type=template_map_fn_factory, template=prompt_template),
+ max_length=max_length,
+ pad_image_to_square=True)
+
+train_dataloader = dict(
+ batch_size=batch_size,
+ num_workers=dataloader_num_workers,
+ pin_memory=True,
+ dataset=llava_dataset,
+ sampler=dict(
+ type=LengthGroupedSampler,
+ length_property='modality_length',
+ per_device_batch_size=batch_size * accumulative_counts),
+ collate_fn=dict(type=default_collate_fn))
+
+#######################################################################
+# PART 4 Scheduler & Optimizer #
+#######################################################################
+# optimizer
+optim_wrapper = dict(
+ type=AmpOptimWrapper,
+ optimizer=dict(
+ type=optim_type, lr=lr, betas=betas, weight_decay=weight_decay),
+ clip_grad=dict(max_norm=max_norm, error_if_nonfinite=False),
+ accumulative_counts=accumulative_counts,
+ loss_scale='dynamic',
+ dtype='float16')
+
+# learning policy
+# More information: https://github.com/open-mmlab/mmengine/blob/main/docs/en/tutorials/param_scheduler.md # noqa: E501
+param_scheduler = [
+ dict(
+ type=LinearLR,
+ start_factor=1e-5,
+ by_epoch=True,
+ begin=0,
+ end=warmup_ratio * max_epochs,
+ convert_to_iter_based=True),
+ dict(
+ type=CosineAnnealingLR,
+ eta_min=0.0,
+ by_epoch=True,
+ begin=warmup_ratio * max_epochs,
+ end=max_epochs,
+ convert_to_iter_based=True)
+]
+
+# train, val, test setting
+train_cfg = dict(type=TrainLoop, max_epochs=max_epochs)
+
+#######################################################################
+# PART 5 Runtime #
+#######################################################################
+# Log the dialogue periodically during the training process, optional
+custom_hooks = [
+ dict(type=DatasetInfoHook, tokenizer=tokenizer),
+ dict(
+ type=EvaluateChatHook,
+ tokenizer=tokenizer,
+ image_processor=image_processor,
+ every_n_iters=evaluation_freq,
+ evaluation_inputs=evaluation_inputs,
+ evaluation_images=evaluation_images,
+ system=SYSTEM,
+ prompt_template=prompt_template)
+]
+
+# configure default hooks
+default_hooks = dict(
+ # record the time of every iteration.
+ timer=dict(type=IterTimerHook),
+ # print log every 10 iterations.
+ logger=dict(type=LoggerHook, log_metric_by_epoch=False, interval=10),
+ # enable the parameter scheduler.
+ param_scheduler=dict(type=ParamSchedulerHook),
+ # save checkpoint per `save_steps`.
+ checkpoint=dict(
+ type=CheckpointHook,
+ by_epoch=False,
+ interval=save_steps,
+ max_keep_ckpts=save_total_limit),
+ # set sampler seed in distributed evrionment.
+ sampler_seed=dict(type=DistSamplerSeedHook),
+)
+
+# configure environment
+env_cfg = dict(
+ # whether to enable cudnn benchmark
+ cudnn_benchmark=False,
+ # set multi process parameters
+ mp_cfg=dict(mp_start_method='fork', opencv_num_threads=0),
+ # set distributed parameters
+ dist_cfg=dict(backend='nccl'),
+)
+
+# set visualizer
+visualizer = None
+
+# set log level
+log_level = 'INFO'
+
+# load from which checkpoint
+load_from = None
+
+# whether to resume training from the loaded checkpoint
+resume = False
+
+# Defaults to use random seed and disable `deterministic`
+randomness = dict(seed=None, deterministic=False)
+
+# set log processor
+log_processor = dict(by_epoch=False)
diff --git a/xtuner/configs/llava/llama3_8b_instruct_clip_vit_large_p14_336/finetune/llava_llama3_8b_instruct_full_clip_vit_large_p14_336_lora_e1_gpu8_internvl_finetune.py b/xtuner/configs/llava/llama3_8b_instruct_clip_vit_large_p14_336/finetune/llava_llama3_8b_instruct_full_clip_vit_large_p14_336_lora_e1_gpu8_internvl_finetune.py
new file mode 100644
index 000000000..98cddc939
--- /dev/null
+++ b/xtuner/configs/llava/llama3_8b_instruct_clip_vit_large_p14_336/finetune/llava_llama3_8b_instruct_full_clip_vit_large_p14_336_lora_e1_gpu8_internvl_finetune.py
@@ -0,0 +1,337 @@
+# Copyright (c) OpenMMLab. All rights reserved.
+from mmengine.hooks import (CheckpointHook, DistSamplerSeedHook, IterTimerHook,
+ LoggerHook, ParamSchedulerHook)
+from mmengine.optim import AmpOptimWrapper, CosineAnnealingLR, LinearLR
+from peft import LoraConfig
+from torch.optim import AdamW
+from transformers import (AutoModelForCausalLM, AutoTokenizer,
+ CLIPImageProcessor, CLIPVisionModel)
+
+from xtuner.dataset import ConcatDataset, LLaVADataset
+from xtuner.dataset.collate_fns import default_collate_fn
+from xtuner.dataset.map_fns import llava_map_fn, template_map_fn_factory
+from xtuner.dataset.samplers import LengthGroupedSampler
+from xtuner.engine.hooks import DatasetInfoHook, EvaluateChatHook
+from xtuner.engine.runner import TrainLoop
+from xtuner.model import LLaVAModel
+from xtuner.utils import PROMPT_TEMPLATE
+
+#######################################################################
+# PART 1 Settings #
+#######################################################################
+# Model
+llm_name_or_path = 'meta-llama/Meta-Llama-3-8B-Instruct'
+visual_encoder_name_or_path = 'openai/clip-vit-large-patch14-336'
+# Specify the pretrained pth
+pretrained_pth = './work_dirs/llava_llama3_8b_instruct_clip_vit_large_p14_336_e1_gpu8_sharegpt4v_pretrain/iter_9742.pth' # noqa: E501
+# Data
+data_root = './data/internvl_sft/'
+
+sharegpt4v_caption_data_path = data_root + 'sharegpt4v_instruct_gpt4-vision_cap100k.jsonl' # noqa: E501
+sharegpt4v_caption_image_folder = data_root + 'data'
+
+llava_data_path = data_root + 'llava_instruct_150k_zh.jsonl'
+llava_image_folder = data_root + 'data/coco'
+
+sharegpt4v_data_path = data_root + 'sharegpt4v_mix665k_cap23k_coco-ap9k_lcs3k_sam9k_div2k.jsonl' # noqa: E501
+sharegpt4v_image_folder = data_root + 'data'
+
+dvqa_data_path = data_root + 'dvqa_train_200k.jsonl'
+dvqa_image_folder = data_root + 'data/dvqa'
+
+chartqa_data_path = data_root + 'chartqa_train_18k.jsonl'
+chartqa_image_folder = data_root + 'data/chartqa'
+
+ai2d_data_path = data_root + 'ai2d_train_12k.jsonl'
+ai2d_image_folder = data_root + 'data/ai2d'
+
+docvqa_data_path = data_root + 'docvqa_train_10k.jsonl'
+docvqa_image_folder = data_root + 'data/docvqa'
+
+geoqa_data_path = data_root + 'geoqa+.jsonl'
+geoqa_image_folder = data_root + 'data/geoqa+'
+
+synthdog_data_path = data_root + 'synthdog_en.jsonl'
+synthdog_image_folder = data_root + 'data/synthdog-en'
+
+prompt_template = PROMPT_TEMPLATE.llama3_chat
+max_length = int(4096 - (336 / 14)**2)
+
+# Scheduler & Optimizer
+batch_size = 4 # per_device
+accumulative_counts = 4
+dataloader_num_workers = 4
+max_epochs = 1
+optim_type = AdamW
+lr = 2e-5
+betas = (0.9, 0.999)
+weight_decay = 0
+max_norm = 1 # grad clip
+warmup_ratio = 0.03
+
+# Save
+save_steps = 5000
+save_total_limit = 2 # Maximum checkpoints to keep (-1 means unlimited)
+
+# Evaluate the generation performance during the training
+evaluation_freq = 5000
+SYSTEM = ''
+evaluation_images = 'https://llava-vl.github.io/static/images/view.jpg'
+evaluation_inputs = ['请描述一下这张照片', 'Please describe this picture']
+
+#######################################################################
+# PART 2 Model & Tokenizer & Image Processor #
+#######################################################################
+tokenizer = dict(
+ type=AutoTokenizer.from_pretrained,
+ pretrained_model_name_or_path=llm_name_or_path,
+ trust_remote_code=True,
+ padding_side='right')
+
+image_processor = dict(
+ type=CLIPImageProcessor.from_pretrained,
+ pretrained_model_name_or_path=visual_encoder_name_or_path,
+ trust_remote_code=True)
+
+model = dict(
+ type=LLaVAModel,
+ freeze_llm=False,
+ freeze_visual_encoder=True,
+ pretrained_pth=pretrained_pth,
+ llm=dict(
+ type=AutoModelForCausalLM.from_pretrained,
+ pretrained_model_name_or_path=llm_name_or_path,
+ trust_remote_code=True),
+ visual_encoder=dict(
+ type=CLIPVisionModel.from_pretrained,
+ pretrained_model_name_or_path=visual_encoder_name_or_path),
+ visual_encoder_lora=dict(
+ type=LoraConfig, r=64, lora_alpha=16, lora_dropout=0.05, bias='none'))
+
+#######################################################################
+# PART 3 Dataset & Dataloader #
+#######################################################################
+sharegpt4v_caption_dataset = dict(
+ type=LLaVADataset,
+ data_path=sharegpt4v_caption_data_path,
+ image_folder=sharegpt4v_caption_image_folder,
+ tokenizer=tokenizer,
+ image_processor=image_processor,
+ dataset_map_fn=llava_map_fn,
+ template_map_fn=dict(
+ type=template_map_fn_factory, template=prompt_template),
+ max_length=max_length,
+ pad_image_to_square=True)
+
+llava_dataset = dict(
+ type=LLaVADataset,
+ data_path=llava_data_path,
+ image_folder=llava_image_folder,
+ tokenizer=tokenizer,
+ image_processor=image_processor,
+ dataset_map_fn=llava_map_fn,
+ template_map_fn=dict(
+ type=template_map_fn_factory, template=prompt_template),
+ max_length=max_length,
+ pad_image_to_square=True)
+
+sharegpt4v_dataset = dict(
+ type=LLaVADataset,
+ data_path=sharegpt4v_data_path,
+ image_folder=sharegpt4v_image_folder,
+ tokenizer=tokenizer,
+ image_processor=image_processor,
+ dataset_map_fn=llava_map_fn,
+ template_map_fn=dict(
+ type=template_map_fn_factory, template=prompt_template),
+ max_length=max_length,
+ pad_image_to_square=True)
+
+dvqa_dataset = dict(
+ type=LLaVADataset,
+ data_path=dvqa_data_path,
+ image_folder=dvqa_image_folder,
+ tokenizer=tokenizer,
+ image_processor=image_processor,
+ dataset_map_fn=llava_map_fn,
+ template_map_fn=dict(
+ type=template_map_fn_factory, template=prompt_template),
+ max_length=max_length,
+ pad_image_to_square=True)
+
+chartqa_dataset = dict(
+ type=LLaVADataset,
+ data_path=chartqa_data_path,
+ image_folder=chartqa_image_folder,
+ tokenizer=tokenizer,
+ image_processor=image_processor,
+ dataset_map_fn=llava_map_fn,
+ template_map_fn=dict(
+ type=template_map_fn_factory, template=prompt_template),
+ max_length=max_length,
+ pad_image_to_square=True)
+
+ai2d_dataset = dict(
+ type=LLaVADataset,
+ data_path=ai2d_data_path,
+ image_folder=ai2d_image_folder,
+ tokenizer=tokenizer,
+ image_processor=image_processor,
+ dataset_map_fn=llava_map_fn,
+ template_map_fn=dict(
+ type=template_map_fn_factory, template=prompt_template),
+ max_length=max_length,
+ pad_image_to_square=True)
+
+docvqa_dataset = dict(
+ type=LLaVADataset,
+ data_path=docvqa_data_path,
+ image_folder=docvqa_image_folder,
+ tokenizer=tokenizer,
+ image_processor=image_processor,
+ dataset_map_fn=llava_map_fn,
+ template_map_fn=dict(
+ type=template_map_fn_factory, template=prompt_template),
+ max_length=max_length,
+ pad_image_to_square=True)
+
+geoqa_dataset = dict(
+ type=LLaVADataset,
+ data_path=geoqa_data_path,
+ image_folder=geoqa_image_folder,
+ tokenizer=tokenizer,
+ image_processor=image_processor,
+ dataset_map_fn=llava_map_fn,
+ template_map_fn=dict(
+ type=template_map_fn_factory, template=prompt_template),
+ max_length=max_length,
+ pad_image_to_square=True)
+
+synthdog_dataset = dict(
+ type=LLaVADataset,
+ data_path=synthdog_data_path,
+ image_folder=synthdog_image_folder,
+ tokenizer=tokenizer,
+ image_processor=image_processor,
+ dataset_map_fn=llava_map_fn,
+ template_map_fn=dict(
+ type=template_map_fn_factory, template=prompt_template),
+ max_length=max_length,
+ pad_image_to_square=True)
+
+train_dataset = dict(
+ type=ConcatDataset,
+ datasets=[
+ sharegpt4v_caption_dataset, llava_dataset, sharegpt4v_dataset,
+ dvqa_dataset, chartqa_dataset, ai2d_dataset, docvqa_dataset,
+ geoqa_dataset, synthdog_dataset
+ ])
+
+train_dataloader = dict(
+ batch_size=batch_size,
+ num_workers=dataloader_num_workers,
+ pin_memory=True,
+ dataset=train_dataset,
+ sampler=dict(
+ type=LengthGroupedSampler,
+ length_property='modality_length',
+ per_device_batch_size=batch_size * accumulative_counts),
+ collate_fn=dict(type=default_collate_fn))
+
+#######################################################################
+# PART 4 Scheduler & Optimizer #
+#######################################################################
+# optimizer
+optim_wrapper = dict(
+ type=AmpOptimWrapper,
+ optimizer=dict(
+ type=optim_type, lr=lr, betas=betas, weight_decay=weight_decay),
+ clip_grad=dict(max_norm=max_norm, error_if_nonfinite=False),
+ accumulative_counts=accumulative_counts,
+ loss_scale='dynamic',
+ dtype='float16')
+
+# learning policy
+# More information: https://github.com/open-mmlab/mmengine/blob/main/docs/en/tutorials/param_scheduler.md # noqa: E501
+param_scheduler = [
+ dict(
+ type=LinearLR,
+ start_factor=1e-5,
+ by_epoch=True,
+ begin=0,
+ end=warmup_ratio * max_epochs,
+ convert_to_iter_based=True),
+ dict(
+ type=CosineAnnealingLR,
+ eta_min=0.0,
+ by_epoch=True,
+ begin=warmup_ratio * max_epochs,
+ end=max_epochs,
+ convert_to_iter_based=True)
+]
+
+# train, val, test setting
+train_cfg = dict(type=TrainLoop, max_epochs=max_epochs)
+
+#######################################################################
+# PART 5 Runtime #
+#######################################################################
+# Log the dialogue periodically during the training process, optional
+custom_hooks = [
+ dict(type=DatasetInfoHook, tokenizer=tokenizer),
+ dict(
+ type=EvaluateChatHook,
+ tokenizer=tokenizer,
+ image_processor=image_processor,
+ every_n_iters=evaluation_freq,
+ evaluation_inputs=evaluation_inputs,
+ evaluation_images=evaluation_images,
+ system=SYSTEM,
+ prompt_template=prompt_template)
+]
+
+# configure default hooks
+default_hooks = dict(
+ # record the time of every iteration.
+ timer=dict(type=IterTimerHook),
+ # print log every 10 iterations.
+ logger=dict(type=LoggerHook, log_metric_by_epoch=False, interval=10),
+ # enable the parameter scheduler.
+ param_scheduler=dict(type=ParamSchedulerHook),
+ # save checkpoint per `save_steps`.
+ checkpoint=dict(
+ type=CheckpointHook,
+ by_epoch=False,
+ interval=save_steps,
+ max_keep_ckpts=save_total_limit),
+ # set sampler seed in distributed evrionment.
+ sampler_seed=dict(type=DistSamplerSeedHook),
+)
+
+# configure environment
+env_cfg = dict(
+ # whether to enable cudnn benchmark
+ cudnn_benchmark=False,
+ # set multi process parameters
+ mp_cfg=dict(mp_start_method='fork', opencv_num_threads=0),
+ # set distributed parameters
+ dist_cfg=dict(backend='nccl'),
+)
+
+# set visualizer
+visualizer = None
+
+# set log level
+log_level = 'INFO'
+
+# load from which checkpoint
+load_from = None
+
+# whether to resume training from the loaded checkpoint
+resume = False
+
+# Defaults to use random seed and disable `deterministic`
+randomness = dict(seed=None, deterministic=False)
+
+# set log processor
+log_processor = dict(by_epoch=False)
diff --git a/xtuner/configs/llava/llama3_8b_instruct_clip_vit_large_p14_336/finetune/llava_llama3_8b_instruct_qlora_clip_vit_large_p14_336_e1_gpu1_finetune.py b/xtuner/configs/llava/llama3_8b_instruct_clip_vit_large_p14_336/finetune/llava_llama3_8b_instruct_qlora_clip_vit_large_p14_336_e1_gpu1_finetune.py
new file mode 100644
index 000000000..99d209005
--- /dev/null
+++ b/xtuner/configs/llava/llama3_8b_instruct_clip_vit_large_p14_336/finetune/llava_llama3_8b_instruct_qlora_clip_vit_large_p14_336_e1_gpu1_finetune.py
@@ -0,0 +1,224 @@
+# Copyright (c) OpenMMLab. All rights reserved.
+import torch
+from mmengine.hooks import (CheckpointHook, DistSamplerSeedHook, IterTimerHook,
+ LoggerHook, ParamSchedulerHook)
+from mmengine.optim import AmpOptimWrapper, CosineAnnealingLR, LinearLR
+from peft import LoraConfig
+from torch.optim import AdamW
+from transformers import (AutoModelForCausalLM, AutoTokenizer,
+ BitsAndBytesConfig, CLIPImageProcessor,
+ CLIPVisionModel)
+
+from xtuner.dataset import LLaVADataset
+from xtuner.dataset.collate_fns import default_collate_fn
+from xtuner.dataset.map_fns import llava_map_fn, template_map_fn_factory
+from xtuner.dataset.samplers import LengthGroupedSampler
+from xtuner.engine.hooks import DatasetInfoHook, EvaluateChatHook
+from xtuner.engine.runner import TrainLoop
+from xtuner.model import LLaVAModel
+from xtuner.utils import PROMPT_TEMPLATE
+
+#######################################################################
+# PART 1 Settings #
+#######################################################################
+# Model
+llm_name_or_path = 'meta-llama/Meta-Llama-3-8B-Instruct'
+visual_encoder_name_or_path = 'openai/clip-vit-large-patch14-336'
+# Specify the pretrained pth
+pretrained_pth = './work_dirs/llava_llama3_8b_instruct_quant_clip_vit_large_p14_336_e1_gpu1_pretrain/558128.pth' # noqa: E501
+
+# Data
+data_root = './data/llava_data/'
+data_path = data_root + 'LLaVA-Instruct-150K/llava_v1_5_mix665k.json'
+image_folder = data_root + 'llava_images'
+prompt_template = PROMPT_TEMPLATE.llama3_chat
+max_length = int(2048 - (336 / 14)**2)
+
+# Scheduler & Optimizer
+batch_size = 1 # per_device
+accumulative_counts = 128
+dataloader_num_workers = 0
+max_epochs = 1
+optim_type = AdamW
+lr = 2e-4
+betas = (0.9, 0.999)
+weight_decay = 0
+max_norm = 1 # grad clip
+warmup_ratio = 0.03
+
+# Save
+save_steps = 50000
+save_total_limit = 2 # Maximum checkpoints to keep (-1 means unlimited)
+
+# Evaluate the generation performance during the training
+evaluation_freq = 50000
+SYSTEM = ''
+evaluation_images = 'https://llava-vl.github.io/static/images/view.jpg'
+evaluation_inputs = ['请描述一下这张照片', 'Please describe this picture']
+
+#######################################################################
+# PART 2 Model & Tokenizer & Image Processor #
+#######################################################################
+tokenizer = dict(
+ type=AutoTokenizer.from_pretrained,
+ pretrained_model_name_or_path=llm_name_or_path,
+ trust_remote_code=True,
+ padding_side='right')
+
+image_processor = dict(
+ type=CLIPImageProcessor.from_pretrained,
+ pretrained_model_name_or_path=visual_encoder_name_or_path,
+ trust_remote_code=True)
+
+model = dict(
+ type=LLaVAModel,
+ freeze_llm=True,
+ freeze_visual_encoder=True,
+ pretrained_pth=pretrained_pth,
+ llm=dict(
+ type=AutoModelForCausalLM.from_pretrained,
+ pretrained_model_name_or_path=llm_name_or_path,
+ trust_remote_code=True,
+ torch_dtype=torch.float16,
+ quantization_config=dict(
+ type=BitsAndBytesConfig,
+ load_in_4bit=True,
+ load_in_8bit=False,
+ llm_int8_threshold=6.0,
+ llm_int8_has_fp16_weight=False,
+ bnb_4bit_compute_dtype=torch.float16,
+ bnb_4bit_use_double_quant=True,
+ bnb_4bit_quant_type='nf4')),
+ llm_lora=dict(
+ type=LoraConfig,
+ r=64,
+ lora_alpha=16,
+ lora_dropout=0.05,
+ bias='none',
+ task_type='CAUSAL_LM'),
+ visual_encoder=dict(
+ type=CLIPVisionModel.from_pretrained,
+ pretrained_model_name_or_path=visual_encoder_name_or_path))
+
+#######################################################################
+# PART 3 Dataset & Dataloader #
+#######################################################################
+llava_dataset = dict(
+ type=LLaVADataset,
+ data_path=data_path,
+ image_folder=image_folder,
+ tokenizer=tokenizer,
+ image_processor=image_processor,
+ dataset_map_fn=llava_map_fn,
+ template_map_fn=dict(
+ type=template_map_fn_factory, template=prompt_template),
+ max_length=max_length,
+ pad_image_to_square=True)
+
+train_dataloader = dict(
+ batch_size=batch_size,
+ num_workers=dataloader_num_workers,
+ dataset=llava_dataset,
+ sampler=dict(
+ type=LengthGroupedSampler,
+ length_property='modality_length',
+ per_device_batch_size=batch_size * accumulative_counts),
+ collate_fn=dict(type=default_collate_fn))
+
+#######################################################################
+# PART 4 Scheduler & Optimizer #
+#######################################################################
+# optimizer
+optim_wrapper = dict(
+ type=AmpOptimWrapper,
+ optimizer=dict(
+ type=optim_type, lr=lr, betas=betas, weight_decay=weight_decay),
+ clip_grad=dict(max_norm=max_norm, error_if_nonfinite=False),
+ accumulative_counts=accumulative_counts,
+ loss_scale='dynamic',
+ dtype='float16')
+
+# learning policy
+# More information: https://github.com/open-mmlab/mmengine/blob/main/docs/en/tutorials/param_scheduler.md # noqa: E501
+param_scheduler = [
+ dict(
+ type=LinearLR,
+ start_factor=1e-5,
+ by_epoch=True,
+ begin=0,
+ end=warmup_ratio * max_epochs,
+ convert_to_iter_based=True),
+ dict(
+ type=CosineAnnealingLR,
+ eta_min=0.0,
+ by_epoch=True,
+ begin=warmup_ratio * max_epochs,
+ end=max_epochs,
+ convert_to_iter_based=True)
+]
+
+# train, val, test setting
+train_cfg = dict(type=TrainLoop, max_epochs=max_epochs)
+
+#######################################################################
+# PART 5 Runtime #
+#######################################################################
+# Log the dialogue periodically during the training process, optional
+custom_hooks = [
+ dict(type=DatasetInfoHook, tokenizer=tokenizer),
+ dict(
+ type=EvaluateChatHook,
+ tokenizer=tokenizer,
+ image_processor=image_processor,
+ every_n_iters=evaluation_freq,
+ evaluation_inputs=evaluation_inputs,
+ evaluation_images=evaluation_images,
+ system=SYSTEM,
+ prompt_template=prompt_template)
+]
+
+# configure default hooks
+default_hooks = dict(
+ # record the time of every iteration.
+ timer=dict(type=IterTimerHook),
+ # print log every 10 iterations.
+ logger=dict(type=LoggerHook, log_metric_by_epoch=False, interval=10),
+ # enable the parameter scheduler.
+ param_scheduler=dict(type=ParamSchedulerHook),
+ # save checkpoint per `save_steps`.
+ checkpoint=dict(
+ type=CheckpointHook,
+ by_epoch=False,
+ interval=save_steps,
+ max_keep_ckpts=save_total_limit),
+ # set sampler seed in distributed evrionment.
+ sampler_seed=dict(type=DistSamplerSeedHook),
+)
+
+# configure environment
+env_cfg = dict(
+ # whether to enable cudnn benchmark
+ cudnn_benchmark=False,
+ # set multi process parameters
+ mp_cfg=dict(mp_start_method='fork', opencv_num_threads=0),
+ # set distributed parameters
+ dist_cfg=dict(backend='nccl'),
+)
+
+# set visualizer
+visualizer = None
+
+# set log level
+log_level = 'INFO'
+
+# load from which checkpoint
+load_from = None
+
+# whether to resume training from the loaded checkpoint
+resume = False
+
+# Defaults to use random seed and disable `deterministic`
+randomness = dict(seed=None, deterministic=False)
+
+# set log processor
+log_processor = dict(by_epoch=False)
diff --git a/xtuner/configs/llava/llama3_8b_instruct_clip_vit_large_p14_336/pretrain/llava_llama3_8b_instruct_clip_vit_large_p14_336_e1_gpu8_pretrain.py b/xtuner/configs/llava/llama3_8b_instruct_clip_vit_large_p14_336/pretrain/llava_llama3_8b_instruct_clip_vit_large_p14_336_e1_gpu8_pretrain.py
new file mode 100644
index 000000000..342348370
--- /dev/null
+++ b/xtuner/configs/llava/llama3_8b_instruct_clip_vit_large_p14_336/pretrain/llava_llama3_8b_instruct_clip_vit_large_p14_336_e1_gpu8_pretrain.py
@@ -0,0 +1,199 @@
+# Copyright (c) OpenMMLab. All rights reserved.
+from mmengine.dataset import DefaultSampler
+from mmengine.hooks import (CheckpointHook, DistSamplerSeedHook, IterTimerHook,
+ LoggerHook, ParamSchedulerHook)
+from mmengine.optim import AmpOptimWrapper, CosineAnnealingLR, LinearLR
+from torch.optim import AdamW
+from transformers import (AutoModelForCausalLM, AutoTokenizer,
+ CLIPImageProcessor, CLIPVisionModel)
+
+from xtuner.dataset import LLaVADataset
+from xtuner.dataset.collate_fns import default_collate_fn
+from xtuner.dataset.map_fns import llava_map_fn, template_map_fn_factory
+from xtuner.engine.hooks import DatasetInfoHook, EvaluateChatHook
+from xtuner.engine.runner import TrainLoop
+from xtuner.model import LLaVAModel
+from xtuner.utils import PROMPT_TEMPLATE
+
+#######################################################################
+# PART 1 Settings #
+#######################################################################
+# Model
+llm_name_or_path = 'meta-llama/Meta-Llama-3-8B-Instruct'
+visual_encoder_name_or_path = 'openai/clip-vit-large-patch14-336'
+
+# Data
+data_root = './data/llava_data/'
+data_path = data_root + 'LLaVA-Pretrain/blip_laion_cc_sbu_558k.json'
+image_folder = data_root + 'LLaVA-Pretrain/images'
+prompt_template = PROMPT_TEMPLATE.llama3_chat
+max_length = int(2048 - (336 / 14)**2)
+
+# Scheduler & Optimizer
+batch_size = 32 # per_device
+accumulative_counts = 1
+dataloader_num_workers = 4
+max_epochs = 1
+optim_type = AdamW
+lr = 1e-3
+betas = (0.9, 0.999)
+weight_decay = 0
+max_norm = 1 # grad clip
+warmup_ratio = 0.03
+
+# Save
+save_steps = 500
+save_total_limit = 2 # Maximum checkpoints to keep (-1 means unlimited)
+
+# Evaluate the generation performance during the training
+evaluation_freq = 500
+SYSTEM = ''
+evaluation_images = 'https://llava-vl.github.io/static/images/view.jpg'
+evaluation_inputs = ['请描述一下这张照片', 'Please describe this picture']
+
+#######################################################################
+# PART 2 Model & Tokenizer & Image Processor #
+#######################################################################
+tokenizer = dict(
+ type=AutoTokenizer.from_pretrained,
+ pretrained_model_name_or_path=llm_name_or_path,
+ trust_remote_code=True,
+ padding_side='right')
+
+image_processor = dict(
+ type=CLIPImageProcessor.from_pretrained,
+ pretrained_model_name_or_path=visual_encoder_name_or_path,
+ trust_remote_code=True)
+
+model = dict(
+ type=LLaVAModel,
+ freeze_llm=True,
+ freeze_visual_encoder=True,
+ llm=dict(
+ type=AutoModelForCausalLM.from_pretrained,
+ pretrained_model_name_or_path=llm_name_or_path,
+ trust_remote_code=True),
+ visual_encoder=dict(
+ type=CLIPVisionModel.from_pretrained,
+ pretrained_model_name_or_path=visual_encoder_name_or_path))
+
+#######################################################################
+# PART 3 Dataset & Dataloader #
+#######################################################################
+llava_dataset = dict(
+ type=LLaVADataset,
+ data_path=data_path,
+ image_folder=image_folder,
+ tokenizer=tokenizer,
+ image_processor=image_processor,
+ dataset_map_fn=llava_map_fn,
+ template_map_fn=dict(
+ type=template_map_fn_factory, template=prompt_template),
+ max_length=max_length,
+ pad_image_to_square=False)
+
+train_dataloader = dict(
+ batch_size=batch_size,
+ num_workers=dataloader_num_workers,
+ pin_memory=True,
+ dataset=llava_dataset,
+ sampler=dict(type=DefaultSampler, shuffle=True),
+ collate_fn=dict(type=default_collate_fn))
+
+#######################################################################
+# PART 4 Scheduler & Optimizer #
+#######################################################################
+# optimizer
+optim_wrapper = dict(
+ type=AmpOptimWrapper,
+ optimizer=dict(
+ type=optim_type, lr=lr, betas=betas, weight_decay=weight_decay),
+ clip_grad=dict(max_norm=max_norm, error_if_nonfinite=False),
+ accumulative_counts=accumulative_counts,
+ loss_scale='dynamic',
+ dtype='float16')
+
+# learning policy
+# More information: https://github.com/open-mmlab/mmengine/blob/main/docs/en/tutorials/param_scheduler.md # noqa: E501
+param_scheduler = [
+ dict(
+ type=LinearLR,
+ start_factor=1e-5,
+ by_epoch=True,
+ begin=0,
+ end=warmup_ratio * max_epochs,
+ convert_to_iter_based=True),
+ dict(
+ type=CosineAnnealingLR,
+ eta_min=0.0,
+ by_epoch=True,
+ begin=warmup_ratio * max_epochs,
+ end=max_epochs,
+ convert_to_iter_based=True)
+]
+
+# train, val, test setting
+train_cfg = dict(type=TrainLoop, max_epochs=max_epochs)
+
+#######################################################################
+# PART 5 Runtime #
+#######################################################################
+# Log the dialogue periodically during the training process, optional
+custom_hooks = [
+ dict(type=DatasetInfoHook, tokenizer=tokenizer),
+ dict(
+ type=EvaluateChatHook,
+ tokenizer=tokenizer,
+ image_processor=image_processor,
+ every_n_iters=evaluation_freq,
+ evaluation_inputs=evaluation_inputs,
+ evaluation_images=evaluation_images,
+ system=SYSTEM,
+ prompt_template=prompt_template)
+]
+
+# configure default hooks
+default_hooks = dict(
+ # record the time of every iteration.
+ timer=dict(type=IterTimerHook),
+ # print log every 10 iterations.
+ logger=dict(type=LoggerHook, log_metric_by_epoch=False, interval=10),
+ # enable the parameter scheduler.
+ param_scheduler=dict(type=ParamSchedulerHook),
+ # save checkpoint per `save_steps`.
+ checkpoint=dict(
+ type=CheckpointHook,
+ by_epoch=False,
+ interval=save_steps,
+ max_keep_ckpts=save_total_limit),
+ # set sampler seed in distributed evrionment.
+ sampler_seed=dict(type=DistSamplerSeedHook),
+)
+
+# configure environment
+env_cfg = dict(
+ # whether to enable cudnn benchmark
+ cudnn_benchmark=False,
+ # set multi process parameters
+ mp_cfg=dict(mp_start_method='fork', opencv_num_threads=0),
+ # set distributed parameters
+ dist_cfg=dict(backend='nccl'),
+)
+
+# set visualizer
+visualizer = None
+
+# set log level
+log_level = 'INFO'
+
+# load from which checkpoint
+load_from = None
+
+# whether to resume training from the loaded checkpoint
+resume = False
+
+# Defaults to use random seed and disable `deterministic`
+randomness = dict(seed=None, deterministic=False)
+
+# set log processor
+log_processor = dict(by_epoch=False)
diff --git a/xtuner/configs/llava/llama3_8b_instruct_clip_vit_large_p14_336/pretrain/llava_llama3_8b_instruct_clip_vit_large_p14_336_e1_gpu8_sharegpt4v_pretrain.py b/xtuner/configs/llava/llama3_8b_instruct_clip_vit_large_p14_336/pretrain/llava_llama3_8b_instruct_clip_vit_large_p14_336_e1_gpu8_sharegpt4v_pretrain.py
new file mode 100644
index 000000000..6e2e32431
--- /dev/null
+++ b/xtuner/configs/llava/llama3_8b_instruct_clip_vit_large_p14_336/pretrain/llava_llama3_8b_instruct_clip_vit_large_p14_336_e1_gpu8_sharegpt4v_pretrain.py
@@ -0,0 +1,199 @@
+# Copyright (c) OpenMMLab. All rights reserved.
+from mmengine.dataset import DefaultSampler
+from mmengine.hooks import (CheckpointHook, DistSamplerSeedHook, IterTimerHook,
+ LoggerHook, ParamSchedulerHook)
+from mmengine.optim import AmpOptimWrapper, CosineAnnealingLR, LinearLR
+from torch.optim import AdamW
+from transformers import (AutoModelForCausalLM, AutoTokenizer,
+ CLIPImageProcessor, CLIPVisionModel)
+
+from xtuner.dataset import LLaVADataset
+from xtuner.dataset.collate_fns import default_collate_fn
+from xtuner.dataset.map_fns import llava_map_fn, template_map_fn_factory
+from xtuner.engine.hooks import DatasetInfoHook, EvaluateChatHook
+from xtuner.engine.runner import TrainLoop
+from xtuner.model import LLaVAModel
+from xtuner.utils import PROMPT_TEMPLATE
+
+#######################################################################
+# PART 1 Settings #
+#######################################################################
+# Model
+llm_name_or_path = 'meta-llama/Meta-Llama-3-8B-Instruct'
+visual_encoder_name_or_path = 'openai/clip-vit-large-patch14-336'
+
+# Data
+data_root = './data/sharegpt4v/'
+data_path = data_root + 'share-captioner_coco_lcs_sam_1246k_1107.json'
+image_folder = data_root + 'data'
+prompt_template = PROMPT_TEMPLATE.llama3_chat
+max_length = int(4096 - (336 / 14)**2)
+
+# Scheduler & Optimizer
+batch_size = 16 # per_device
+accumulative_counts = 2
+dataloader_num_workers = 4
+max_epochs = 1
+optim_type = AdamW
+lr = 1e-3
+betas = (0.9, 0.999)
+weight_decay = 0
+max_norm = 1 # grad clip
+warmup_ratio = 0.03
+
+# Save
+save_steps = 1000
+save_total_limit = 2 # Maximum checkpoints to keep (-1 means unlimited)
+
+# Evaluate the generation performance during the training
+evaluation_freq = 1000
+SYSTEM = ''
+evaluation_images = 'https://llava-vl.github.io/static/images/view.jpg'
+evaluation_inputs = ['请描述一下这张照片', 'Please describe this picture']
+
+#######################################################################
+# PART 2 Model & Tokenizer & Image Processor #
+#######################################################################
+tokenizer = dict(
+ type=AutoTokenizer.from_pretrained,
+ pretrained_model_name_or_path=llm_name_or_path,
+ trust_remote_code=True,
+ padding_side='right')
+
+image_processor = dict(
+ type=CLIPImageProcessor.from_pretrained,
+ pretrained_model_name_or_path=visual_encoder_name_or_path,
+ trust_remote_code=True)
+
+model = dict(
+ type=LLaVAModel,
+ freeze_llm=True,
+ freeze_visual_encoder=True,
+ llm=dict(
+ type=AutoModelForCausalLM.from_pretrained,
+ pretrained_model_name_or_path=llm_name_or_path,
+ trust_remote_code=True),
+ visual_encoder=dict(
+ type=CLIPVisionModel.from_pretrained,
+ pretrained_model_name_or_path=visual_encoder_name_or_path))
+
+#######################################################################
+# PART 3 Dataset & Dataloader #
+#######################################################################
+llava_dataset = dict(
+ type=LLaVADataset,
+ data_path=data_path,
+ image_folder=image_folder,
+ tokenizer=tokenizer,
+ image_processor=image_processor,
+ dataset_map_fn=llava_map_fn,
+ template_map_fn=dict(
+ type=template_map_fn_factory, template=prompt_template),
+ max_length=max_length,
+ pad_image_to_square=False)
+
+train_dataloader = dict(
+ batch_size=batch_size,
+ num_workers=dataloader_num_workers,
+ pin_memory=True,
+ dataset=llava_dataset,
+ sampler=dict(type=DefaultSampler, shuffle=True),
+ collate_fn=dict(type=default_collate_fn))
+
+#######################################################################
+# PART 4 Scheduler & Optimizer #
+#######################################################################
+# optimizer
+optim_wrapper = dict(
+ type=AmpOptimWrapper,
+ optimizer=dict(
+ type=optim_type, lr=lr, betas=betas, weight_decay=weight_decay),
+ clip_grad=dict(max_norm=max_norm, error_if_nonfinite=False),
+ accumulative_counts=accumulative_counts,
+ loss_scale='dynamic',
+ dtype='float16')
+
+# learning policy
+# More information: https://github.com/open-mmlab/mmengine/blob/main/docs/en/tutorials/param_scheduler.md # noqa: E501
+param_scheduler = [
+ dict(
+ type=LinearLR,
+ start_factor=1e-5,
+ by_epoch=True,
+ begin=0,
+ end=warmup_ratio * max_epochs,
+ convert_to_iter_based=True),
+ dict(
+ type=CosineAnnealingLR,
+ eta_min=0.0,
+ by_epoch=True,
+ begin=warmup_ratio * max_epochs,
+ end=max_epochs,
+ convert_to_iter_based=True)
+]
+
+# train, val, test setting
+train_cfg = dict(type=TrainLoop, max_epochs=max_epochs)
+
+#######################################################################
+# PART 5 Runtime #
+#######################################################################
+# Log the dialogue periodically during the training process, optional
+custom_hooks = [
+ dict(type=DatasetInfoHook, tokenizer=tokenizer),
+ dict(
+ type=EvaluateChatHook,
+ tokenizer=tokenizer,
+ image_processor=image_processor,
+ every_n_iters=evaluation_freq,
+ evaluation_inputs=evaluation_inputs,
+ evaluation_images=evaluation_images,
+ system=SYSTEM,
+ prompt_template=prompt_template)
+]
+
+# configure default hooks
+default_hooks = dict(
+ # record the time of every iteration.
+ timer=dict(type=IterTimerHook),
+ # print log every 10 iterations.
+ logger=dict(type=LoggerHook, log_metric_by_epoch=False, interval=10),
+ # enable the parameter scheduler.
+ param_scheduler=dict(type=ParamSchedulerHook),
+ # save checkpoint per `save_steps`.
+ checkpoint=dict(
+ type=CheckpointHook,
+ by_epoch=False,
+ interval=save_steps,
+ max_keep_ckpts=save_total_limit),
+ # set sampler seed in distributed evrionment.
+ sampler_seed=dict(type=DistSamplerSeedHook),
+)
+
+# configure environment
+env_cfg = dict(
+ # whether to enable cudnn benchmark
+ cudnn_benchmark=False,
+ # set multi process parameters
+ mp_cfg=dict(mp_start_method='fork', opencv_num_threads=0),
+ # set distributed parameters
+ dist_cfg=dict(backend='nccl'),
+)
+
+# set visualizer
+visualizer = None
+
+# set log level
+log_level = 'INFO'
+
+# load from which checkpoint
+load_from = None
+
+# whether to resume training from the loaded checkpoint
+resume = False
+
+# Defaults to use random seed and disable `deterministic`
+randomness = dict(seed=None, deterministic=False)
+
+# set log processor
+log_processor = dict(by_epoch=False)
diff --git a/xtuner/configs/llava/llama3_8b_instruct_clip_vit_large_p14_336/pretrain/llava_llama3_8b_instruct_quant_clip_vit_large_p14_336_e1_gpu1_pretrain.py b/xtuner/configs/llava/llama3_8b_instruct_clip_vit_large_p14_336/pretrain/llava_llama3_8b_instruct_quant_clip_vit_large_p14_336_e1_gpu1_pretrain.py
new file mode 100644
index 000000000..98a4813e2
--- /dev/null
+++ b/xtuner/configs/llava/llama3_8b_instruct_clip_vit_large_p14_336/pretrain/llava_llama3_8b_instruct_quant_clip_vit_large_p14_336_e1_gpu1_pretrain.py
@@ -0,0 +1,210 @@
+# Copyright (c) OpenMMLab. All rights reserved.
+import torch
+from mmengine.dataset import DefaultSampler
+from mmengine.hooks import (CheckpointHook, DistSamplerSeedHook, IterTimerHook,
+ LoggerHook, ParamSchedulerHook)
+from mmengine.optim import AmpOptimWrapper, CosineAnnealingLR, LinearLR
+from torch.optim import AdamW
+from transformers import (AutoModelForCausalLM, AutoTokenizer,
+ BitsAndBytesConfig, CLIPImageProcessor,
+ CLIPVisionModel)
+
+from xtuner.dataset import LLaVADataset
+from xtuner.dataset.collate_fns import default_collate_fn
+from xtuner.dataset.map_fns import llava_map_fn, template_map_fn_factory
+from xtuner.engine.hooks import DatasetInfoHook, EvaluateChatHook
+from xtuner.engine.runner import TrainLoop
+from xtuner.model import LLaVAModel
+from xtuner.utils import PROMPT_TEMPLATE
+
+#######################################################################
+# PART 1 Settings #
+#######################################################################
+# Model
+llm_name_or_path = 'meta-llama/Meta-Llama-3-8B-Instruct'
+visual_encoder_name_or_path = 'openai/clip-vit-large-patch14-336'
+
+# Data
+data_root = './data/llava_data/'
+data_path = data_root + 'LLaVA-Pretrain/blip_laion_cc_sbu_558k.json'
+image_folder = data_root + 'LLaVA-Pretrain/images'
+prompt_template = PROMPT_TEMPLATE.llama3_chat
+max_length = int(2048 - (336 / 14)**2)
+
+# Scheduler & Optimizer
+batch_size = 1 # per_device
+accumulative_counts = 256
+dataloader_num_workers = 0
+max_epochs = 1
+optim_type = AdamW
+lr = 1e-3
+betas = (0.9, 0.999)
+weight_decay = 0
+max_norm = 1 # grad clip
+warmup_ratio = 0.03
+
+# Save
+save_steps = 50000
+save_total_limit = 2 # Maximum checkpoints to keep (-1 means unlimited)
+
+# Evaluate the generation performance during the training
+evaluation_freq = 50000
+SYSTEM = ''
+evaluation_images = 'https://llava-vl.github.io/static/images/view.jpg'
+evaluation_inputs = ['请描述一下这张照片', 'Please describe this picture']
+
+#######################################################################
+# PART 2 Model & Tokenizer & Image Processor #
+#######################################################################
+tokenizer = dict(
+ type=AutoTokenizer.from_pretrained,
+ pretrained_model_name_or_path=llm_name_or_path,
+ trust_remote_code=True,
+ padding_side='right')
+
+image_processor = dict(
+ type=CLIPImageProcessor.from_pretrained,
+ pretrained_model_name_or_path=visual_encoder_name_or_path,
+ trust_remote_code=True)
+
+model = dict(
+ type=LLaVAModel,
+ freeze_llm=True,
+ freeze_visual_encoder=True,
+ llm=dict(
+ type=AutoModelForCausalLM.from_pretrained,
+ pretrained_model_name_or_path=llm_name_or_path,
+ trust_remote_code=True,
+ torch_dtype=torch.float16,
+ quantization_config=dict(
+ type=BitsAndBytesConfig,
+ load_in_4bit=True,
+ load_in_8bit=False,
+ llm_int8_threshold=6.0,
+ llm_int8_has_fp16_weight=False,
+ bnb_4bit_compute_dtype=torch.float16,
+ bnb_4bit_use_double_quant=True,
+ bnb_4bit_quant_type='nf4')),
+ visual_encoder=dict(
+ type=CLIPVisionModel.from_pretrained,
+ pretrained_model_name_or_path=visual_encoder_name_or_path))
+
+#######################################################################
+# PART 3 Dataset & Dataloader #
+#######################################################################
+llava_dataset = dict(
+ type=LLaVADataset,
+ data_path=data_path,
+ image_folder=image_folder,
+ tokenizer=tokenizer,
+ image_processor=image_processor,
+ dataset_map_fn=llava_map_fn,
+ template_map_fn=dict(
+ type=template_map_fn_factory, template=prompt_template),
+ max_length=max_length,
+ pad_image_to_square=False)
+
+train_dataloader = dict(
+ batch_size=batch_size,
+ num_workers=dataloader_num_workers,
+ dataset=llava_dataset,
+ sampler=dict(type=DefaultSampler, shuffle=True),
+ collate_fn=dict(type=default_collate_fn))
+
+#######################################################################
+# PART 4 Scheduler & Optimizer #
+#######################################################################
+# optimizer
+optim_wrapper = dict(
+ type=AmpOptimWrapper,
+ optimizer=dict(
+ type=optim_type, lr=lr, betas=betas, weight_decay=weight_decay),
+ clip_grad=dict(max_norm=max_norm, error_if_nonfinite=False),
+ accumulative_counts=accumulative_counts,
+ loss_scale='dynamic',
+ dtype='float16')
+
+# learning policy
+# More information: https://github.com/open-mmlab/mmengine/blob/main/docs/en/tutorials/param_scheduler.md # noqa: E501
+param_scheduler = [
+ dict(
+ type=LinearLR,
+ start_factor=1e-5,
+ by_epoch=True,
+ begin=0,
+ end=warmup_ratio * max_epochs,
+ convert_to_iter_based=True),
+ dict(
+ type=CosineAnnealingLR,
+ eta_min=0.0,
+ by_epoch=True,
+ begin=warmup_ratio * max_epochs,
+ end=max_epochs,
+ convert_to_iter_based=True)
+]
+
+# train, val, test setting
+train_cfg = dict(type=TrainLoop, max_epochs=max_epochs)
+
+#######################################################################
+# PART 5 Runtime #
+#######################################################################
+# Log the dialogue periodically during the training process, optional
+custom_hooks = [
+ dict(type=DatasetInfoHook, tokenizer=tokenizer),
+ dict(
+ type=EvaluateChatHook,
+ tokenizer=tokenizer,
+ image_processor=image_processor,
+ every_n_iters=evaluation_freq,
+ evaluation_inputs=evaluation_inputs,
+ evaluation_images=evaluation_images,
+ system=SYSTEM,
+ prompt_template=prompt_template)
+]
+
+# configure default hooks
+default_hooks = dict(
+ # record the time of every iteration.
+ timer=dict(type=IterTimerHook),
+ # print log every 10 iterations.
+ logger=dict(type=LoggerHook, log_metric_by_epoch=False, interval=10),
+ # enable the parameter scheduler.
+ param_scheduler=dict(type=ParamSchedulerHook),
+ # save checkpoint per `save_steps`.
+ checkpoint=dict(
+ type=CheckpointHook,
+ by_epoch=False,
+ interval=save_steps,
+ max_keep_ckpts=save_total_limit),
+ # set sampler seed in distributed evrionment.
+ sampler_seed=dict(type=DistSamplerSeedHook),
+)
+
+# configure environment
+env_cfg = dict(
+ # whether to enable cudnn benchmark
+ cudnn_benchmark=False,
+ # set multi process parameters
+ mp_cfg=dict(mp_start_method='fork', opencv_num_threads=0),
+ # set distributed parameters
+ dist_cfg=dict(backend='nccl'),
+)
+
+# set visualizer
+visualizer = None
+
+# set log level
+log_level = 'INFO'
+
+# load from which checkpoint
+load_from = None
+
+# whether to resume training from the loaded checkpoint
+resume = False
+
+# Defaults to use random seed and disable `deterministic`
+randomness = dict(seed=None, deterministic=False)
+
+# set log processor
+log_processor = dict(by_epoch=False)
diff --git a/xtuner/configs/llava/official/llava_v15_13b/llava_v15_13b_finetune.py b/xtuner/configs/llava/official/llava_v15_13b/llava_v15_13b_finetune.py
index c7a4bb823..183b73a9e 100644
--- a/xtuner/configs/llava/official/llava_v15_13b/llava_v15_13b_finetune.py
+++ b/xtuner/configs/llava/official/llava_v15_13b/llava_v15_13b_finetune.py
@@ -34,7 +34,7 @@
# Scheduler & Optimizer
batch_size = 16 # per_device
accumulative_counts = 1
-dataloader_num_workers = 0
+dataloader_num_workers = 4
max_epochs = 1
optim_type = AdamW
lr = 2e-5
@@ -98,6 +98,7 @@
train_dataloader = dict(
batch_size=batch_size,
num_workers=dataloader_num_workers,
+ pin_memory=True,
dataset=llava_dataset,
sampler=dict(
type=LengthGroupedSampler,
diff --git a/xtuner/configs/llava/official/llava_v15_13b/llava_v15_13b_finetune_lora.py b/xtuner/configs/llava/official/llava_v15_13b/llava_v15_13b_finetune_lora.py
index 512651bce..2384bbf71 100644
--- a/xtuner/configs/llava/official/llava_v15_13b/llava_v15_13b_finetune_lora.py
+++ b/xtuner/configs/llava/official/llava_v15_13b/llava_v15_13b_finetune_lora.py
@@ -35,7 +35,7 @@
# Scheduler & Optimizer
batch_size = 16 # per_device
accumulative_counts = 1
-dataloader_num_workers = 0
+dataloader_num_workers = 4
max_epochs = 1
optim_type = AdamW
lr = 2e-4
@@ -70,7 +70,7 @@
model = dict(
type=LLaVAModel,
- freeze_llm=False,
+ freeze_llm=True,
freeze_visual_encoder=True,
pretrained_pth=pretrained_pth,
llm=dict(
@@ -106,6 +106,7 @@
train_dataloader = dict(
batch_size=batch_size,
num_workers=dataloader_num_workers,
+ pin_memory=True,
dataset=llava_dataset,
sampler=dict(
type=LengthGroupedSampler,
diff --git a/xtuner/configs/llava/official/llava_v15_13b/llava_v15_13b_pretrain.py b/xtuner/configs/llava/official/llava_v15_13b/llava_v15_13b_pretrain.py
index 5ee8b7c23..358f09934 100644
--- a/xtuner/configs/llava/official/llava_v15_13b/llava_v15_13b_pretrain.py
+++ b/xtuner/configs/llava/official/llava_v15_13b/llava_v15_13b_pretrain.py
@@ -32,7 +32,7 @@
# Scheduler & Optimizer
batch_size = 32 # per_device
accumulative_counts = 1
-dataloader_num_workers = 0
+dataloader_num_workers = 4
max_epochs = 1
optim_type = AdamW
lr = 1e-3
@@ -95,6 +95,7 @@
train_dataloader = dict(
batch_size=batch_size,
num_workers=dataloader_num_workers,
+ pin_memory=True,
dataset=llava_dataset,
sampler=dict(type=DefaultSampler, shuffle=True),
collate_fn=dict(type=default_collate_fn))
diff --git a/xtuner/configs/llava/official/llava_v15_7b/llava_v15_7b_finetune.py b/xtuner/configs/llava/official/llava_v15_7b/llava_v15_7b_finetune.py
index 25dc04104..7bef64a4e 100644
--- a/xtuner/configs/llava/official/llava_v15_7b/llava_v15_7b_finetune.py
+++ b/xtuner/configs/llava/official/llava_v15_7b/llava_v15_7b_finetune.py
@@ -34,7 +34,7 @@
# Scheduler & Optimizer
batch_size = 16 # per_device
accumulative_counts = 1
-dataloader_num_workers = 0
+dataloader_num_workers = 4
max_epochs = 1
optim_type = AdamW
lr = 2e-5
@@ -98,6 +98,7 @@
train_dataloader = dict(
batch_size=batch_size,
num_workers=dataloader_num_workers,
+ pin_memory=True,
dataset=llava_dataset,
sampler=dict(
type=LengthGroupedSampler,
diff --git a/xtuner/configs/llava/official/llava_v15_7b/llava_v15_7b_finetune_lora.py b/xtuner/configs/llava/official/llava_v15_7b/llava_v15_7b_finetune_lora.py
index 0f9c95c12..b17974f5d 100644
--- a/xtuner/configs/llava/official/llava_v15_7b/llava_v15_7b_finetune_lora.py
+++ b/xtuner/configs/llava/official/llava_v15_7b/llava_v15_7b_finetune_lora.py
@@ -35,7 +35,7 @@
# Scheduler & Optimizer
batch_size = 16 # per_device
accumulative_counts = 1
-dataloader_num_workers = 0
+dataloader_num_workers = 4
max_epochs = 1
optim_type = AdamW
lr = 2e-4
@@ -70,7 +70,7 @@
model = dict(
type=LLaVAModel,
- freeze_llm=False,
+ freeze_llm=True,
freeze_visual_encoder=True,
pretrained_pth=pretrained_pth,
llm=dict(
@@ -106,6 +106,7 @@
train_dataloader = dict(
batch_size=batch_size,
num_workers=dataloader_num_workers,
+ pin_memory=True,
dataset=llava_dataset,
sampler=dict(
type=LengthGroupedSampler,
diff --git a/xtuner/configs/llava/official/llava_v15_7b/llava_v15_7b_pretrain.py b/xtuner/configs/llava/official/llava_v15_7b/llava_v15_7b_pretrain.py
index 33c634e4a..a30457cf8 100644
--- a/xtuner/configs/llava/official/llava_v15_7b/llava_v15_7b_pretrain.py
+++ b/xtuner/configs/llava/official/llava_v15_7b/llava_v15_7b_pretrain.py
@@ -32,7 +32,7 @@
# Scheduler & Optimizer
batch_size = 32 # per_device
accumulative_counts = 1
-dataloader_num_workers = 0
+dataloader_num_workers = 4
max_epochs = 1
optim_type = AdamW
lr = 1e-3
@@ -95,6 +95,7 @@
train_dataloader = dict(
batch_size=batch_size,
num_workers=dataloader_num_workers,
+ pin_memory=True,
dataset=llava_dataset,
sampler=dict(type=DefaultSampler, shuffle=True),
collate_fn=dict(type=default_collate_fn))
diff --git a/xtuner/configs/llava/phi3_mini_4k_instruct_clip_vit_large_p14_336/README.md b/xtuner/configs/llava/phi3_mini_4k_instruct_clip_vit_large_p14_336/README.md
new file mode 100644
index 000000000..00c39b26c
--- /dev/null
+++ b/xtuner/configs/llava/phi3_mini_4k_instruct_clip_vit_large_p14_336/README.md
@@ -0,0 +1,179 @@
+# LLaVA-Phi-3-mini
+
+## Results
+
+
+
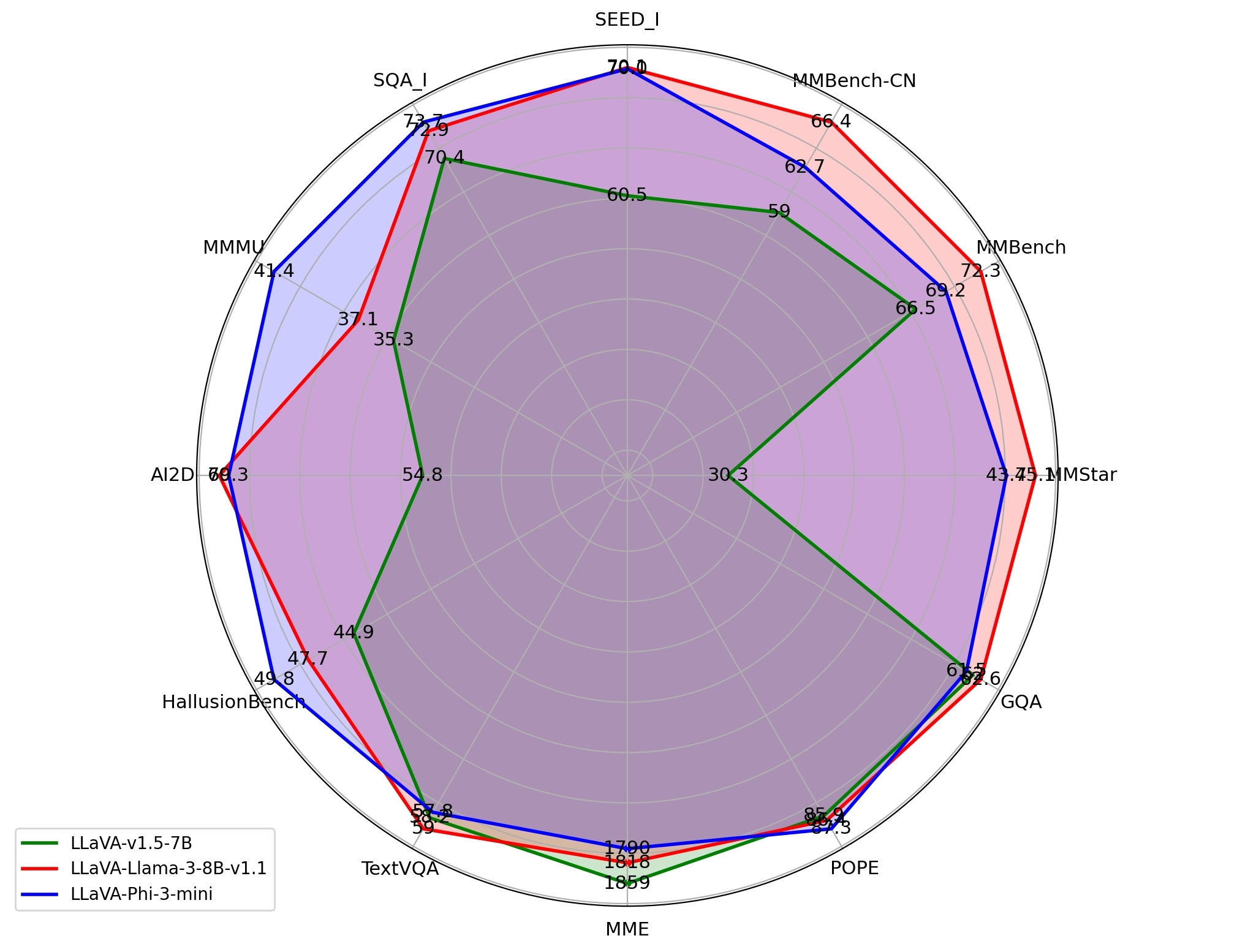
+
+
+| Model | MMBench Test (EN) | MMMU Val | SEED-IMG | AI2D Test | ScienceQA Test | HallusionBench aAcc | POPE | GQA | TextVQA | MME | MMStar | Configs |
+| :-------------------- | :---------------: | :-------: | :------: | :-------: | :------------: | :-----------------: | :--: | :--: | :-----: | :------: | :----: | :----------------------------------------------------------------------------------------------------------------------------------------------------------------------------------------------------------------------------------------------------------------------------------------------------------------------------------------------------------------------------------------------------------------------------------------: |
+| LLaVA-v1.5-7B | 66.5 | 35.3 | 60.5 | 54.8 | 70.4 | 44.9 | 85.9 | 62.0 | 58.2 | 1511/348 | 30.3 | - |
+| LLaVA-Llama-3-8B | 68.9 | 36.8 | 69.8 | 60.9 | 73.3 | 47.3 | 87.2 | 63.5 | 58.0 | 1506/295 | 38.2 | [Pretrain](https://github.com/InternLM/xtuner/blob/main/xtuner/configs/llava/llama3_8b_instruct_clip_vit_large_p14_336/pretrain/llava_llama3_8b_instruct_clip_vit_large_p14_336_e1_gpu8_pretrain.py) / [Fine-tune](https://github.com/InternLM/xtuner/blob/main/xtuner/configs/llava/llama3_8b_instruct_clip_vit_large_p14_336/finetune/llava_llama3_8b_instruct_full_clip_vit_large_p14_336_lora_e1_gpu8_finetune.py) |
+| LLaVA-Llama-3-8B-v1.1 | 72.3 | 37.1 | 70.1 | 70.0 | 72.9 | 47.7 | 86.4 | 62.6 | 59.0 | 1469/349 | 45.1 | [Pretrain](https://github.com/InternLM/xtuner/blob/main/xtuner/configs/llava/llama3_8b_instruct_clip_vit_large_p14_336/pretrain/llava_llama3_8b_instruct_clip_vit_large_p14_336_e1_gpu8_sharegpt4v_pretrain.py) / [Fine-tune](https://github.com/InternLM/xtuner/blob/main/xtuner/configs/llava/llama3_8b_instruct_clip_vit_large_p14_336/finetune/llava_llama3_8b_instruct_full_clip_vit_large_p14_336_lora_e1_gpu8_internvl_finetune.py) |
+| **LLaVA-Phi-3-mini** | 69.2 | 41.4 | 70.0 | 69.3 | 73.7 | 49.8 | 87.3 | 61.5 | 57.8 | 1477/313 | 43.7 | [Pretrain](./pretrain/llava_phi3_mini_4k_instruct_clip_vit_large_p14_336_e1_gpu8_sharegpt4v_pretrain.py) / [Fine-tune](./finetune/llava_phi3_mini_4k_instruct_full_clip_vit_large_p14_336_full_e2_gpu8_internvl_finetune.py) |
+
+## Resources
+
+- Official LLaVA format model (`xtuner/llava-phi-3-mini`): 🤗 [HuggingFace](https://huggingface.co/xtuner/llava-phi-3-mini) / 🤖 [ModelScope](https://modelscope.cn/models/xtuner/llava-phi-3-mini)
+- HuggingFace LLaVA format model (`xtuner/llava-phi-3-mini-hf`): 🤗 [HuggingFace](https://huggingface.co/xtuner/llava-phi-3-mini-hf) / 🤖 [ModelScope](https://modelscope.cn/models/xtuner/llava-phi-3-mini-hf)
+- XTuner LLaVA format model (`xtuner/llava-phi-3-mini-xtuner`): 🤗 [HuggingFace](https://huggingface.co/xtuner/llava-phi-3-mini-xtuner) / 🤖 [ModelScope](https://modelscope.cn/models/xtuner/llava-phi-3-mini-xtuner)
+- GGUF model (`xtuner/llava-phi-3-mini-gguf`): 🤗 [HuggingFace](https://huggingface.co/xtuner/llava-phi-3-mini-gguf) / 🤖 [ModelScope](https://modelscope.cn/models/xtuner/llava-phi-3-mini-gguf)
+- Pretrained projector weights: 🤗 [HuggingFace](https://huggingface.co/xtuner/llava-phi-3-mini-pretrain) / 🤖 [ModelScope](https://modelscope.cn/models/xtuner/llava-phi-3-mini-pretrain)
+
+## Data Preparation
+
+Please refer to [here](https://github.com/InternLM/xtuner/tree/main/xtuner/configs/llava/llama3_8b_instruct_clip_vit_large_p14_336#data-preparation).
+
+## Training
+
+### LLaVA-Phi-3-mini
+
+1. Pretrain
+
+```bash
+NPROC_PER_NODE=8 xtuner train llava_phi3_mini_4k_instruct_clip_vit_large_p14_336_e1_gpu8_sharegpt4v_pretrain --deepspeed deepspeed_zero2 --seed 1024
+```
+
+2. Fine-tune
+
+```bash
+NPROC_PER_NODE=8 xtuner train llava_phi3_mini_4k_instruct_full_clip_vit_large_p14_336_full_e2_gpu8_internvl_finetune --deepspeed deepspeed_zero2 --seed 1024
+```
+
+## Model Conversion
+
+### Step 0. Convert `.pth` file to LLaVA model in xtuner format ([LLaVA-Phi-3-mini-xtuner](https://huggingface.co/xtuner/llava-phi-3-mini-xtuner))
+
+After training, we will obtain a set of weights (*i.e.*, `iter_xxx.pth`), which are not in the universal HuggingFace format. We first need to convert them to the LLaVA model in xtuner format.
+
+```bash
+xtuner convert pth_to_hf $FINETUNE_CFG $PTH_PATH $SAVE_PATH
+# e.g., xtuner convert pth_to_hf llava_phi3_mini_4k_instruct_full_clip_vit_large_p14_336_full_e2_gpu8_internvl_finetune ./iter_39620.pth ./iter_39620_xtuner
+```
+
+```
+./iter_39620_xtuner
+├── added_tokens.json
+├── config.json
+├── model-00001-of-00004.safetensors
+├── model-00002-of-00004.safetensors
+├── model-00003-of-00004.safetensors
+├── model-00004-of-00004.safetensors
+├── model.safetensors.index.json
+├── projector
+│ ├── config.json
+│ ├── configuration_projector.py
+│ ├── modeling_projector.py
+│ └── model.safetensors
+├── special_tokens_map.json
+├── tokenizer_config.json
+├── tokenizer.json
+├── tokenizer.model
+└── visual_encoder
+ ├── config.json
+ ├── model.safetensors
+ └── preprocessor_config.json
+```
+
+At this time, the LLaVA model of xtuner-format can engage in conversation using xtuner chat, by
+
+```bash
+xtuner chat ./iter_39620_xtuner \
+ --llava ./iter_39620_xtuner \
+ --prompt-template phi3_chat \
+ --image $IMAGE_PATH
+```
+
+and in MMBench evaluation, by
+
+```bash
+xtuner mmbench ./iter_39620_xtuner \
+ --llava ./iter_39620_xtuner \
+ --prompt-template phi3_chat \
+ --data-path $DATA_PATH \
+ --work-dir $RESULT_PATH
+```
+
+Here, `$DATA_PATH` refers to one of the mmbench datasets. You can download the expected data by
+
+```bash
+wget https://opencompass.openxlab.space/utils/VLMEval/MMBench_DEV_EN.tsv
+wget https://opencompass.openxlab.space/utils/VLMEval/MMBench_TEST_EN.tsv
+wget https://opencompass.openxlab.space/utils/VLMEval/MMBench_DEV_CN.tsv
+wget https://opencompass.openxlab.space/utils/VLMEval/MMBench_TEST_CN.tsv
+wget https://opencompass.openxlab.space/utils/VLMEval/CCBench.tsv
+```
+
+### Step 1. Convert LLaVA in xtuner format to official LLaVA format or HuggingFace LLaVA format
+
+- The official LLaVA format is structured similarly to the architecture of the [liuhaotian/llava-v1.5-7b](https://huggingface.co/liuhaotian/llava-v1.5-7b) model.
+- The HuggingFace LLaVA format is structured similarly to the architecture of the [llava-hf/llava-1.5-7b-hf](https://huggingface.co/llava-hf/llava-1.5-7b-hf) model.
+
+Since the official LLaVA format and the HuggingFace LLaVA format only support Llama architecture as the LLM, we need to first convert the phi-3 model to an equivalent Llama LLM.
+
+```bash
+python ./convert_phi_to_llama.py --phi_path ./iter_39620_xtuner --save_path ./iter_39620_xtuner_llama_llm
+```
+
+Here, `--phi_path` should specify the path to phi-3, which is the path obtained from Step.0 for the xtuner-format LLaVA model. `--save_path` should specify the save path for the converted Llama LLM.
+
+#### To official LLaVA format ([LLaVA-Phi-3-mini](https://huggingface.co/xtuner/llava-phi-3-mini))
+
+We can utilize the following command to obtain the LLaVA model in the official LLaVA format.
+
+```bash
+python ./convert_xtuner_weights_to_llava.py --text_model_id ./iter_39620_xtuner_llama_llm --vision_model_id ./iter_39620_xtuner/visual_encoder --projector_weight ./iter_39620_xtuner/projector/model.safetensors --save_path ./iter_39620_llava
+```
+
+Here, the converted LLaVA model in official LLaVA format is saved to `./iter_39620_llava`.
+
+```
+./iter_39620_llava
+├── added_tokens.json
+├── config.json
+├── generation_config.json
+├── model-00001-of-00005.safetensors
+├── model-00002-of-00005.safetensors
+├── model-00003-of-00005.safetensors
+├── model-00004-of-00005.safetensors
+├── model-00005-of-00005.safetensors
+├── model.safetensors.index.json
+├── preprocessor_config.json
+├── special_tokens_map.json
+├── tokenizer_config.json
+├── tokenizer.json
+└── tokenizer.model
+```
+
+#### To HuggingFace LLaVA format ([LLaVA-Phi-3-mini-hf](https://huggingface.co/xtuner/llava-phi-3-mini-hf))
+
+We can utilize the following command to obtain the LLaVA model in the HuggingFace LLaVA format.
+
+```bash
+python ./convert_xtuner_weights_to_hf.py --text_model_id ./iter_39620_xtuner_llama_llm --vision_model_id ./iter_39620_xtuner/visual_encoder --projector_weight ./iter_39620_xtuner/projector/model.safetensors --save_path ./iter_39620_hf
+```
+
+Here, the converted LLaVA model in HuggingFace LLaVA format is saved to `./iter_39620_hf`.
+
+```
+./iter_39620_hf
+├── added_tokens.json
+├── config.json
+├── generation_config.json
+├── model-00001-of-00002.safetensors
+├── model-00002-of-00002.safetensors
+├── model.safetensors.index.json
+├── preprocessor_config.json
+├── special_tokens_map.json
+├── tokenizer_config.json
+├── tokenizer.json
+└── tokenizer.model
+```
+
+## Chat
+
+- XTuner LLaVA format [docs](https://huggingface.co/xtuner/llava-phi-3-mini-xtuner#quickstart)
+- Official LLaVA format [docs](https://huggingface.co/xtuner/llava-phi-3-mini#quickstart)
+- HuggingFace LLaVA format [docs](https://huggingface.co/xtuner/llava-phi-3-mini-hf#quickstart)
+- GGUF format [docs](https://huggingface.co/xtuner/llava-phi-3-mini-gguf#quickstart)
diff --git a/xtuner/configs/llava/phi3_mini_4k_instruct_clip_vit_large_p14_336/convert_phi_to_llama.py b/xtuner/configs/llava/phi3_mini_4k_instruct_clip_vit_large_p14_336/convert_phi_to_llama.py
new file mode 100644
index 000000000..fea4a58f9
--- /dev/null
+++ b/xtuner/configs/llava/phi3_mini_4k_instruct_clip_vit_large_p14_336/convert_phi_to_llama.py
@@ -0,0 +1,100 @@
+# Copyright (c) OpenMMLab. All rights reserved.
+import argparse
+import json
+import os
+
+from mmengine.utils import mkdir_or_exist
+from safetensors import safe_open
+from safetensors.torch import save_file
+from tqdm import tqdm
+from transformers import AutoTokenizer
+
+
+def convert_phi_to_llama(phi_path, save_path):
+ files = [f for f in os.listdir(phi_path) if f.endswith('safetensors')]
+ mkdir_or_exist(save_path)
+
+ index_json = os.path.join(phi_path, 'model.safetensors.index.json')
+ config_json = os.path.join(phi_path, 'config.json')
+
+ with open(index_json) as f:
+ index = json.load(f)
+
+ with open(config_json) as f:
+ config = json.load(f)
+
+ config.pop('_name_or_path')
+ if 'auto_map' in config:
+ config.pop('auto_map')
+ config.pop('embd_pdrop')
+ config.pop('resid_pdrop')
+ config['architectures'] = ['LlamaForCausalLM']
+ config['model_type'] = 'llama'
+
+ for file in tqdm(files, desc='Convert'):
+ tensors = {}
+ new_path = os.path.join(save_path, file)
+ old_path = os.path.join(phi_path, file)
+ with safe_open(old_path, framework='pt', device='cpu') as f:
+ for key in f.keys():
+
+ if 'qkv_proj' in key:
+ qkv = f.get_tensor(key)
+
+ q, k, v = qkv.chunk(3, dim=0)
+ q_name = key.replace('qkv_proj', 'q_proj')
+ k_name = key.replace('qkv_proj', 'k_proj')
+ v_name = key.replace('qkv_proj', 'v_proj')
+
+ tensors[q_name] = q
+ tensors[k_name] = k
+ tensors[v_name] = v
+
+ index['weight_map'].pop(key)
+
+ filename = os.path.basename(new_path)
+ index['weight_map'][q_name] = filename
+ index['weight_map'][k_name] = filename
+ index['weight_map'][v_name] = filename
+
+ elif 'gate_up_proj' in key:
+ gate_up_proj = f.get_tensor(key)
+ gate_proj, up_proj = gate_up_proj.chunk(2, dim=0)
+
+ gate_name = key.replace('gate_up_proj', 'gate_proj')
+ up_name = key.replace('gate_up_proj', 'up_proj')
+ tensors[gate_name] = gate_proj
+ tensors[up_name] = up_proj
+
+ index['weight_map'].pop(key)
+ filename = os.path.basename(new_path)
+ index['weight_map'][gate_name] = filename
+ index['weight_map'][up_name] = filename
+ else:
+ tensors[key] = f.get_tensor(key)
+ metadata = f.metadata()
+ save_file(tensors, new_path, metadata=metadata)
+
+ new_config_json = os.path.join(save_path, 'config.json')
+ with open(new_config_json, 'w') as f:
+ json.dump(config, f, indent=2)
+
+ new_index_json = os.path.join(save_path, 'model.safetensors.index.json')
+ with open(new_index_json, 'w') as f:
+ json.dump(index, f, indent=2)
+
+ tokenizer = AutoTokenizer.from_pretrained(phi_path, trust_remote_code=True)
+ tokenizer.save_pretrained(save_path)
+ print(f'Saved to {save_path}')
+
+
+def main():
+ parser = argparse.ArgumentParser()
+ parser.add_argument('--phi_path')
+ parser.add_argument('--save_path')
+ args = parser.parse_args()
+ convert_phi_to_llama(args.phi_path, args.save_path)
+
+
+if __name__ == '__main__':
+ main()
diff --git a/xtuner/configs/llava/phi3_mini_4k_instruct_clip_vit_large_p14_336/convert_xtuner_weights_to_hf.py b/xtuner/configs/llava/phi3_mini_4k_instruct_clip_vit_large_p14_336/convert_xtuner_weights_to_hf.py
new file mode 100644
index 000000000..e14ca29cd
--- /dev/null
+++ b/xtuner/configs/llava/phi3_mini_4k_instruct_clip_vit_large_p14_336/convert_xtuner_weights_to_hf.py
@@ -0,0 +1,140 @@
+# Copyright (c) OpenMMLab. All rights reserved.
+# Modified from https://github.com/huggingface/transformers/blob/v4.40.1/src/transformers/models/llava/convert_llava_weights_to_hf.py # noqa: E501
+import argparse
+
+import torch
+from safetensors import safe_open
+from transformers import (AddedToken, AutoConfig, AutoModel,
+ AutoModelForCausalLM, CLIPImageProcessor,
+ LlamaTokenizerFast, LlavaConfig,
+ LlavaForConditionalGeneration, LlavaProcessor)
+
+KEYS_TO_MODIFY_MAPPING_LLM = {
+ 'model': 'language_model.model',
+ 'lm_head': 'language_model.lm_head',
+}
+KEYS_TO_MODIFY_MAPPING_VIT = {
+ 'vision_model': 'vision_tower.vision_model',
+}
+KEYS_TO_MODIFY_MAPPING_PROJECTOR = {
+ 'model.0': 'multi_modal_projector.linear_1',
+ 'model.2': 'multi_modal_projector.linear_2',
+}
+
+
+def convert_state_dict_to_hf(state_dict, mapping):
+ new_state_dict = {}
+ for key, value in state_dict.items():
+ if key.endswith('.inv_freq'):
+ continue
+ for key_to_modify, new_key in mapping.items():
+ if key_to_modify in key:
+ key = key.replace(key_to_modify, new_key)
+
+ new_state_dict[key] = value
+ return new_state_dict
+
+
+def convert_to_hf(text_model_id, vision_model_id, projector_weight, save_path):
+ torch.set_default_dtype(torch.float16)
+ text_config = AutoConfig.from_pretrained(
+ text_model_id, trust_remote_code=True)
+ vision_config = AutoConfig.from_pretrained(vision_model_id)
+
+ tokenizer = LlamaTokenizerFast.from_pretrained(text_model_id)
+ tokenizer.add_tokens(
+ AddedToken('', special=True, normalized=False),
+ special_tokens=True)
+ tokenizer.add_special_tokens({'pad_token': ''})
+
+ image_processor = CLIPImageProcessor.from_pretrained(vision_model_id)
+
+ processor = LlavaProcessor(
+ tokenizer=tokenizer, image_processor=image_processor)
+
+ config = LlavaConfig(
+ text_config=text_config,
+ vision_config=vision_config,
+ attn_implementation='eager')
+
+ with torch.device('meta'):
+ model = LlavaForConditionalGeneration(config)
+
+ # Pad to 64 for performance reasons
+ pad_shape = 64
+
+ projector_state_dict = {}
+ with safe_open(projector_weight, framework='pt', device='cpu') as f:
+ for key in f.keys():
+ projector_state_dict[key] = f.get_tensor(key)
+
+ ori_llm = AutoModelForCausalLM.from_pretrained(
+ text_model_id, trust_remote_code=True)
+ ori_vit = AutoModel.from_pretrained(vision_model_id)
+ llm_state_dict = ori_llm.state_dict()
+ vit_state_dict = ori_vit.state_dict()
+
+ projector_state_dict = convert_state_dict_to_hf(
+ projector_state_dict, KEYS_TO_MODIFY_MAPPING_PROJECTOR)
+ llm_state_dict = convert_state_dict_to_hf(llm_state_dict,
+ KEYS_TO_MODIFY_MAPPING_LLM)
+ vit_state_dict = convert_state_dict_to_hf(vit_state_dict,
+ KEYS_TO_MODIFY_MAPPING_VIT)
+ state_dict = {**projector_state_dict, **llm_state_dict, **vit_state_dict}
+ model.load_state_dict(state_dict, strict=True, assign=True)
+
+ pre_expansion_embeddings = \
+ model.language_model.model.embed_tokens.weight.data
+ mu = torch.mean(pre_expansion_embeddings, dim=0).float()
+ n = pre_expansion_embeddings.size()[0]
+ sigma = ((pre_expansion_embeddings - mu).T
+ @ (pre_expansion_embeddings - mu)) / n
+ dist = torch.distributions.multivariate_normal.MultivariateNormal(
+ mu, covariance_matrix=1e-5 * sigma)
+
+ # We add an image token so we resize the model
+ ori_vocab_size = config.text_config.vocab_size
+ tokenizer_vocab_size = tokenizer.encode('')[-1]
+ added_token = tokenizer_vocab_size - ori_vocab_size
+
+ if added_token > 0:
+ model.resize_token_embeddings(ori_vocab_size + added_token, pad_shape)
+ model.language_model.model.embed_tokens.weight.data[
+ ori_vocab_size:] = torch.stack(
+ tuple(dist.sample()
+ for _ in range(model.language_model.model.embed_tokens.
+ weight.data[ori_vocab_size:].shape[0])),
+ dim=0,
+ )
+ model.language_model.lm_head.weight.data[
+ ori_vocab_size:] = torch.stack(
+ tuple(dist.sample()
+ for _ in range(model.language_model.lm_head.weight.
+ data[ori_vocab_size:].shape[0])),
+ dim=0,
+ )
+
+ model.config.image_token_index = tokenizer.encode('')[-1]
+ model.config.pad_token_id = tokenizer.encode('')[-1]
+
+ if ori_vit.__class__.__name__ == 'SiglipVisionModel':
+ model.config.vision_feature_select_strategy = 'full'
+
+ model.save_pretrained(save_path)
+ processor.save_pretrained(save_path)
+ print(f'Saved to {save_path}')
+
+
+def main():
+ parser = argparse.ArgumentParser()
+ parser.add_argument('--text_model_id')
+ parser.add_argument('--vision_model_id')
+ parser.add_argument('--projector_weight')
+ parser.add_argument('--save_path')
+ args = parser.parse_args()
+ convert_to_hf(args.text_model_id, args.vision_model_id,
+ args.projector_weight, args.save_path)
+
+
+if __name__ == '__main__':
+ main()
diff --git a/xtuner/configs/llava/phi3_mini_4k_instruct_clip_vit_large_p14_336/convert_xtuner_weights_to_llava.py b/xtuner/configs/llava/phi3_mini_4k_instruct_clip_vit_large_p14_336/convert_xtuner_weights_to_llava.py
new file mode 100644
index 000000000..8a1df6233
--- /dev/null
+++ b/xtuner/configs/llava/phi3_mini_4k_instruct_clip_vit_large_p14_336/convert_xtuner_weights_to_llava.py
@@ -0,0 +1,106 @@
+# Copyright (c) OpenMMLab. All rights reserved.
+import argparse
+
+import torch
+
+try:
+ from llava.model import LlavaConfig, LlavaLlamaForCausalLM
+ from llava.utils import disable_torch_init
+except ImportError:
+ raise ImportError(
+ 'Please install llava with '
+ '`pip install git+https://github.com/haotian-liu/LLaVA.git '
+ '--no-deps`.')
+from safetensors import safe_open
+from transformers import (AutoConfig, AutoModelForCausalLM, AutoTokenizer,
+ CLIPImageProcessor, CLIPVisionModel)
+
+KEYS_TO_MODIFY_MAPPING_VIT = {
+ 'vision_model': 'model.vision_tower.vision_tower.vision_model',
+}
+KEYS_TO_MODIFY_MAPPING_PROJECTOR = {
+ 'model.0': 'model.mm_projector.0',
+ 'model.2': 'model.mm_projector.2',
+}
+
+
+def convert_state_dict_to_hf(state_dict, mapping):
+ new_state_dict = {}
+ for key, value in state_dict.items():
+ if key.endswith('.inv_freq'):
+ continue
+ for key_to_modify, new_key in mapping.items():
+ if key_to_modify in key:
+ key = key.replace(key_to_modify, new_key)
+ new_state_dict[key] = value
+ return new_state_dict
+
+
+def convert_to_llava(text_model_id, vision_model_id, projector_weight,
+ save_path):
+ disable_torch_init()
+ torch.set_default_dtype(torch.float16)
+
+ projector_state_dict = {}
+ with safe_open(projector_weight, framework='pt', device='cpu') as f:
+ for key in f.keys():
+ projector_state_dict[key] = f.get_tensor(key)
+
+ ori_llm = AutoModelForCausalLM.from_pretrained(
+ text_model_id, trust_remote_code=True, device_map='auto')
+ ori_vit = CLIPVisionModel.from_pretrained(vision_model_id)
+ llm_state_dict = ori_llm.state_dict()
+ vit_state_dict = ori_vit.state_dict()
+
+ projector_state_dict = convert_state_dict_to_hf(
+ projector_state_dict, KEYS_TO_MODIFY_MAPPING_PROJECTOR)
+ vit_state_dict = convert_state_dict_to_hf(vit_state_dict,
+ KEYS_TO_MODIFY_MAPPING_VIT)
+ state_dict = {**projector_state_dict, **llm_state_dict, **vit_state_dict}
+
+ tokenizer = AutoTokenizer.from_pretrained(text_model_id)
+ text_config = AutoConfig.from_pretrained(
+ text_model_id, trust_remote_code=True)
+
+ ori_config = text_config.__dict__.copy()
+ ori_config.update(
+ dict(
+ image_aspect_ratio='pad',
+ mm_hidden_size=ori_vit.config.hidden_size,
+ mm_projector_type='mlp2x_gelu',
+ mm_use_im_patch_token=False,
+ mm_use_im_start_end=False,
+ mm_vision_select_feature='patch',
+ mm_vision_select_layer=-2,
+ mm_vision_tower=vision_model_id,
+ unfreeze_mm_vision_tower=True,
+ model_type='llava',
+ use_cache=True,
+ use_mm_proj=True))
+ config = LlavaConfig(**ori_config)
+
+ with torch.device('meta'):
+ model = LlavaLlamaForCausalLM(config)
+
+ image_processor = CLIPImageProcessor.from_pretrained(vision_model_id)
+
+ model.load_state_dict(state_dict, strict=True, assign=True)
+ model.save_pretrained(save_path, max_shard_size='2GB')
+ image_processor.save_pretrained(save_path)
+ tokenizer.save_pretrained(save_path)
+ print(f'Saved to {save_path}')
+
+
+def main():
+ parser = argparse.ArgumentParser()
+ parser.add_argument('--text_model_id')
+ parser.add_argument('--vision_model_id')
+ parser.add_argument('--projector_weight')
+ parser.add_argument('--save_path')
+ args = parser.parse_args()
+ convert_to_llava(args.text_model_id, args.vision_model_id,
+ args.projector_weight, args.save_path)
+
+
+if __name__ == '__main__':
+ main()
diff --git a/xtuner/configs/llava/phi3_mini_4k_instruct_clip_vit_large_p14_336/finetune/llava_phi3_mini_4k_instruct_full_clip_vit_large_p14_336_e1_gpu8_finetune.py b/xtuner/configs/llava/phi3_mini_4k_instruct_clip_vit_large_p14_336/finetune/llava_phi3_mini_4k_instruct_full_clip_vit_large_p14_336_e1_gpu8_finetune.py
new file mode 100644
index 000000000..a1d3cbcd8
--- /dev/null
+++ b/xtuner/configs/llava/phi3_mini_4k_instruct_clip_vit_large_p14_336/finetune/llava_phi3_mini_4k_instruct_full_clip_vit_large_p14_336_e1_gpu8_finetune.py
@@ -0,0 +1,205 @@
+# Copyright (c) OpenMMLab. All rights reserved.
+from mmengine.hooks import (CheckpointHook, DistSamplerSeedHook, IterTimerHook,
+ LoggerHook, ParamSchedulerHook)
+from mmengine.optim import AmpOptimWrapper, CosineAnnealingLR, LinearLR
+from torch.optim import AdamW
+from transformers import (AutoModelForCausalLM, AutoTokenizer,
+ CLIPImageProcessor, CLIPVisionModel)
+
+from xtuner.dataset import LLaVADataset
+from xtuner.dataset.collate_fns import default_collate_fn
+from xtuner.dataset.map_fns import llava_map_fn, template_map_fn_factory
+from xtuner.dataset.samplers import LengthGroupedSampler
+from xtuner.engine.hooks import DatasetInfoHook, EvaluateChatHook
+from xtuner.engine.runner import TrainLoop
+from xtuner.model import LLaVAModel
+from xtuner.utils import PROMPT_TEMPLATE
+
+#######################################################################
+# PART 1 Settings #
+#######################################################################
+# Model
+llm_name_or_path = 'microsoft/Phi-3-mini-4k-instruct'
+visual_encoder_name_or_path = 'openai/clip-vit-large-patch14-336'
+# Specify the pretrained pth
+pretrained_pth = './work_dirs/llava_phi3_mini_4k_instruct_clip_vit_large_p14_336_e1_gpu8_pretrain/iter_2181.pth' # noqa: E501
+
+# Data
+data_root = './data/llava_data/'
+data_path = data_root + 'LLaVA-Instruct-150K/llava_v1_5_mix665k.json'
+image_folder = data_root + 'llava_images'
+prompt_template = PROMPT_TEMPLATE.phi3_chat
+max_length = int(2048 - (336 / 14)**2)
+
+# Scheduler & Optimizer
+batch_size = 8 # per_device
+accumulative_counts = 2
+dataloader_num_workers = 4
+max_epochs = 1
+optim_type = AdamW
+lr = 2e-5
+betas = (0.9, 0.999)
+weight_decay = 0
+max_norm = 1 # grad clip
+warmup_ratio = 0.03
+
+# Save
+save_steps = 1000
+save_total_limit = 2 # Maximum checkpoints to keep (-1 means unlimited)
+
+# Evaluate the generation performance during the training
+evaluation_freq = 1000
+SYSTEM = ''
+evaluation_images = 'https://llava-vl.github.io/static/images/view.jpg'
+evaluation_inputs = ['请描述一下这张照片', 'Please describe this picture']
+
+#######################################################################
+# PART 2 Model & Tokenizer & Image Processor #
+#######################################################################
+tokenizer = dict(
+ type=AutoTokenizer.from_pretrained,
+ pretrained_model_name_or_path=llm_name_or_path,
+ trust_remote_code=True,
+ padding_side='right')
+
+image_processor = dict(
+ type=CLIPImageProcessor.from_pretrained,
+ pretrained_model_name_or_path=visual_encoder_name_or_path,
+ trust_remote_code=True)
+
+model = dict(
+ type=LLaVAModel,
+ freeze_llm=False,
+ freeze_visual_encoder=True,
+ pretrained_pth=pretrained_pth,
+ llm=dict(
+ type=AutoModelForCausalLM.from_pretrained,
+ pretrained_model_name_or_path=llm_name_or_path,
+ trust_remote_code=True),
+ visual_encoder=dict(
+ type=CLIPVisionModel.from_pretrained,
+ pretrained_model_name_or_path=visual_encoder_name_or_path))
+
+#######################################################################
+# PART 3 Dataset & Dataloader #
+#######################################################################
+llava_dataset = dict(
+ type=LLaVADataset,
+ data_path=data_path,
+ image_folder=image_folder,
+ tokenizer=tokenizer,
+ image_processor=image_processor,
+ dataset_map_fn=llava_map_fn,
+ template_map_fn=dict(
+ type=template_map_fn_factory, template=prompt_template),
+ max_length=max_length,
+ pad_image_to_square=True)
+
+train_dataloader = dict(
+ batch_size=batch_size,
+ num_workers=dataloader_num_workers,
+ pin_memory=True,
+ dataset=llava_dataset,
+ sampler=dict(
+ type=LengthGroupedSampler,
+ length_property='modality_length',
+ per_device_batch_size=batch_size * accumulative_counts),
+ collate_fn=dict(type=default_collate_fn))
+
+#######################################################################
+# PART 4 Scheduler & Optimizer #
+#######################################################################
+# optimizer
+optim_wrapper = dict(
+ type=AmpOptimWrapper,
+ optimizer=dict(
+ type=optim_type, lr=lr, betas=betas, weight_decay=weight_decay),
+ clip_grad=dict(max_norm=max_norm, error_if_nonfinite=False),
+ accumulative_counts=accumulative_counts,
+ loss_scale='dynamic',
+ dtype='float16')
+
+# learning policy
+# More information: https://github.com/open-mmlab/mmengine/blob/main/docs/en/tutorials/param_scheduler.md # noqa: E501
+param_scheduler = [
+ dict(
+ type=LinearLR,
+ start_factor=1e-5,
+ by_epoch=True,
+ begin=0,
+ end=warmup_ratio * max_epochs,
+ convert_to_iter_based=True),
+ dict(
+ type=CosineAnnealingLR,
+ eta_min=0.0,
+ by_epoch=True,
+ begin=warmup_ratio * max_epochs,
+ end=max_epochs,
+ convert_to_iter_based=True)
+]
+
+# train, val, test setting
+train_cfg = dict(type=TrainLoop, max_epochs=max_epochs)
+
+#######################################################################
+# PART 5 Runtime #
+#######################################################################
+# Log the dialogue periodically during the training process, optional
+custom_hooks = [
+ dict(type=DatasetInfoHook, tokenizer=tokenizer),
+ dict(
+ type=EvaluateChatHook,
+ tokenizer=tokenizer,
+ image_processor=image_processor,
+ every_n_iters=evaluation_freq,
+ evaluation_inputs=evaluation_inputs,
+ evaluation_images=evaluation_images,
+ system=SYSTEM,
+ prompt_template=prompt_template)
+]
+
+# configure default hooks
+default_hooks = dict(
+ # record the time of every iteration.
+ timer=dict(type=IterTimerHook),
+ # print log every 10 iterations.
+ logger=dict(type=LoggerHook, log_metric_by_epoch=False, interval=10),
+ # enable the parameter scheduler.
+ param_scheduler=dict(type=ParamSchedulerHook),
+ # save checkpoint per `save_steps`.
+ checkpoint=dict(
+ type=CheckpointHook,
+ by_epoch=False,
+ interval=save_steps,
+ max_keep_ckpts=save_total_limit),
+ # set sampler seed in distributed evrionment.
+ sampler_seed=dict(type=DistSamplerSeedHook),
+)
+
+# configure environment
+env_cfg = dict(
+ # whether to enable cudnn benchmark
+ cudnn_benchmark=False,
+ # set multi process parameters
+ mp_cfg=dict(mp_start_method='fork', opencv_num_threads=0),
+ # set distributed parameters
+ dist_cfg=dict(backend='nccl'),
+)
+
+# set visualizer
+visualizer = None
+
+# set log level
+log_level = 'INFO'
+
+# load from which checkpoint
+load_from = None
+
+# whether to resume training from the loaded checkpoint
+resume = False
+
+# Defaults to use random seed and disable `deterministic`
+randomness = dict(seed=None, deterministic=False)
+
+# set log processor
+log_processor = dict(by_epoch=False)
diff --git a/xtuner/configs/llava/phi3_mini_4k_instruct_clip_vit_large_p14_336/finetune/llava_phi3_mini_4k_instruct_full_clip_vit_large_p14_336_full_e2_gpu8_internvl_finetune.py b/xtuner/configs/llava/phi3_mini_4k_instruct_clip_vit_large_p14_336/finetune/llava_phi3_mini_4k_instruct_full_clip_vit_large_p14_336_full_e2_gpu8_internvl_finetune.py
new file mode 100644
index 000000000..7ba93bb24
--- /dev/null
+++ b/xtuner/configs/llava/phi3_mini_4k_instruct_clip_vit_large_p14_336/finetune/llava_phi3_mini_4k_instruct_full_clip_vit_large_p14_336_full_e2_gpu8_internvl_finetune.py
@@ -0,0 +1,334 @@
+# Copyright (c) OpenMMLab. All rights reserved.
+from mmengine.hooks import (CheckpointHook, DistSamplerSeedHook, IterTimerHook,
+ LoggerHook, ParamSchedulerHook)
+from mmengine.optim import AmpOptimWrapper, CosineAnnealingLR, LinearLR
+from torch.optim import AdamW
+from transformers import (AutoModelForCausalLM, AutoTokenizer,
+ CLIPImageProcessor, CLIPVisionModel)
+
+from xtuner.dataset import ConcatDataset, LLaVADataset
+from xtuner.dataset.collate_fns import default_collate_fn
+from xtuner.dataset.map_fns import llava_map_fn, template_map_fn_factory
+from xtuner.dataset.samplers import LengthGroupedSampler
+from xtuner.engine.hooks import DatasetInfoHook, EvaluateChatHook
+from xtuner.engine.runner import TrainLoop
+from xtuner.model import LLaVAModel
+from xtuner.utils import PROMPT_TEMPLATE
+
+#######################################################################
+# PART 1 Settings #
+#######################################################################
+# Model
+llm_name_or_path = 'microsoft/Phi-3-mini-4k-instruct'
+visual_encoder_name_or_path = 'openai/clip-vit-large-patch14-336'
+# Specify the pretrained pth
+pretrained_pth = './work_dirs/llava_phi3_mini_4k_instruct_clip_vit_large_p14_336_e1_gpu8_sharegpt4v_pretrain/iter_9742.pth' # noqa: E501
+# Data
+data_root = './data/internvl_sft/'
+
+sharegpt4v_caption_data_path = data_root + 'sharegpt4v_instruct_gpt4-vision_cap100k.jsonl' # noqa: E501
+sharegpt4v_caption_image_folder = data_root + 'data'
+
+llava_data_path = data_root + 'llava_instruct_150k_zh.jsonl'
+llava_image_folder = data_root + 'data/coco'
+
+sharegpt4v_data_path = data_root + 'sharegpt4v_mix665k_cap23k_coco-ap9k_lcs3k_sam9k_div2k.jsonl' # noqa: E501
+sharegpt4v_image_folder = data_root + 'data'
+
+dvqa_data_path = data_root + 'dvqa_train_200k.jsonl'
+dvqa_image_folder = data_root + 'data/dvqa'
+
+chartqa_data_path = data_root + 'chartqa_train_18k.jsonl'
+chartqa_image_folder = data_root + 'data/chartqa'
+
+ai2d_data_path = data_root + 'ai2d_train_12k.jsonl'
+ai2d_image_folder = data_root + 'data/ai2d'
+
+docvqa_data_path = data_root + 'docvqa_train_10k.jsonl'
+docvqa_image_folder = data_root + 'data/docvqa'
+
+geoqa_data_path = data_root + 'geoqa+.jsonl'
+geoqa_image_folder = data_root + 'data/geoqa+'
+
+synthdog_data_path = data_root + 'synthdog_en.jsonl'
+synthdog_image_folder = data_root + 'data/synthdog-en'
+
+prompt_template = PROMPT_TEMPLATE.phi3_chat
+max_length = int(4096 - (336 / 14)**2)
+
+# Scheduler & Optimizer
+batch_size = 8 # per_device
+accumulative_counts = 2
+dataloader_num_workers = 4
+max_epochs = 2
+optim_type = AdamW
+lr = 2e-5
+betas = (0.9, 0.999)
+weight_decay = 0
+max_norm = 1 # grad clip
+warmup_ratio = 0.03
+
+# Save
+save_steps = 5000
+save_total_limit = 2 # Maximum checkpoints to keep (-1 means unlimited)
+
+# Evaluate the generation performance during the training
+evaluation_freq = 5000
+SYSTEM = ''
+evaluation_images = 'https://llava-vl.github.io/static/images/view.jpg'
+evaluation_inputs = ['请描述一下这张照片', 'Please describe this picture']
+
+#######################################################################
+# PART 2 Model & Tokenizer & Image Processor #
+#######################################################################
+tokenizer = dict(
+ type=AutoTokenizer.from_pretrained,
+ pretrained_model_name_or_path=llm_name_or_path,
+ trust_remote_code=True,
+ padding_side='right')
+
+image_processor = dict(
+ type=CLIPImageProcessor.from_pretrained,
+ pretrained_model_name_or_path=visual_encoder_name_or_path,
+ trust_remote_code=True)
+
+model = dict(
+ type=LLaVAModel,
+ freeze_llm=False,
+ freeze_visual_encoder=False,
+ pretrained_pth=pretrained_pth,
+ llm=dict(
+ type=AutoModelForCausalLM.from_pretrained,
+ pretrained_model_name_or_path=llm_name_or_path,
+ trust_remote_code=True),
+ visual_encoder=dict(
+ type=CLIPVisionModel.from_pretrained,
+ pretrained_model_name_or_path=visual_encoder_name_or_path))
+
+#######################################################################
+# PART 3 Dataset & Dataloader #
+#######################################################################
+sharegpt4v_caption_dataset = dict(
+ type=LLaVADataset,
+ data_path=sharegpt4v_caption_data_path,
+ image_folder=sharegpt4v_caption_image_folder,
+ tokenizer=tokenizer,
+ image_processor=image_processor,
+ dataset_map_fn=llava_map_fn,
+ template_map_fn=dict(
+ type=template_map_fn_factory, template=prompt_template),
+ max_length=max_length,
+ pad_image_to_square=True)
+
+llava_dataset = dict(
+ type=LLaVADataset,
+ data_path=llava_data_path,
+ image_folder=llava_image_folder,
+ tokenizer=tokenizer,
+ image_processor=image_processor,
+ dataset_map_fn=llava_map_fn,
+ template_map_fn=dict(
+ type=template_map_fn_factory, template=prompt_template),
+ max_length=max_length,
+ pad_image_to_square=True)
+
+sharegpt4v_dataset = dict(
+ type=LLaVADataset,
+ data_path=sharegpt4v_data_path,
+ image_folder=sharegpt4v_image_folder,
+ tokenizer=tokenizer,
+ image_processor=image_processor,
+ dataset_map_fn=llava_map_fn,
+ template_map_fn=dict(
+ type=template_map_fn_factory, template=prompt_template),
+ max_length=max_length,
+ pad_image_to_square=True)
+
+dvqa_dataset = dict(
+ type=LLaVADataset,
+ data_path=dvqa_data_path,
+ image_folder=dvqa_image_folder,
+ tokenizer=tokenizer,
+ image_processor=image_processor,
+ dataset_map_fn=llava_map_fn,
+ template_map_fn=dict(
+ type=template_map_fn_factory, template=prompt_template),
+ max_length=max_length,
+ pad_image_to_square=True)
+
+chartqa_dataset = dict(
+ type=LLaVADataset,
+ data_path=chartqa_data_path,
+ image_folder=chartqa_image_folder,
+ tokenizer=tokenizer,
+ image_processor=image_processor,
+ dataset_map_fn=llava_map_fn,
+ template_map_fn=dict(
+ type=template_map_fn_factory, template=prompt_template),
+ max_length=max_length,
+ pad_image_to_square=True)
+
+ai2d_dataset = dict(
+ type=LLaVADataset,
+ data_path=ai2d_data_path,
+ image_folder=ai2d_image_folder,
+ tokenizer=tokenizer,
+ image_processor=image_processor,
+ dataset_map_fn=llava_map_fn,
+ template_map_fn=dict(
+ type=template_map_fn_factory, template=prompt_template),
+ max_length=max_length,
+ pad_image_to_square=True)
+
+docvqa_dataset = dict(
+ type=LLaVADataset,
+ data_path=docvqa_data_path,
+ image_folder=docvqa_image_folder,
+ tokenizer=tokenizer,
+ image_processor=image_processor,
+ dataset_map_fn=llava_map_fn,
+ template_map_fn=dict(
+ type=template_map_fn_factory, template=prompt_template),
+ max_length=max_length,
+ pad_image_to_square=True)
+
+geoqa_dataset = dict(
+ type=LLaVADataset,
+ data_path=geoqa_data_path,
+ image_folder=geoqa_image_folder,
+ tokenizer=tokenizer,
+ image_processor=image_processor,
+ dataset_map_fn=llava_map_fn,
+ template_map_fn=dict(
+ type=template_map_fn_factory, template=prompt_template),
+ max_length=max_length,
+ pad_image_to_square=True)
+
+synthdog_dataset = dict(
+ type=LLaVADataset,
+ data_path=synthdog_data_path,
+ image_folder=synthdog_image_folder,
+ tokenizer=tokenizer,
+ image_processor=image_processor,
+ dataset_map_fn=llava_map_fn,
+ template_map_fn=dict(
+ type=template_map_fn_factory, template=prompt_template),
+ max_length=max_length,
+ pad_image_to_square=True)
+
+train_dataset = dict(
+ type=ConcatDataset,
+ datasets=[
+ sharegpt4v_caption_dataset, llava_dataset, sharegpt4v_dataset,
+ dvqa_dataset, chartqa_dataset, ai2d_dataset, docvqa_dataset,
+ geoqa_dataset, synthdog_dataset
+ ])
+
+train_dataloader = dict(
+ batch_size=batch_size,
+ num_workers=dataloader_num_workers,
+ pin_memory=True,
+ dataset=train_dataset,
+ sampler=dict(
+ type=LengthGroupedSampler,
+ length_property='modality_length',
+ per_device_batch_size=batch_size * accumulative_counts),
+ collate_fn=dict(type=default_collate_fn))
+
+#######################################################################
+# PART 4 Scheduler & Optimizer #
+#######################################################################
+# optimizer
+optim_wrapper = dict(
+ type=AmpOptimWrapper,
+ optimizer=dict(
+ type=optim_type, lr=lr, betas=betas, weight_decay=weight_decay),
+ clip_grad=dict(max_norm=max_norm, error_if_nonfinite=False),
+ accumulative_counts=accumulative_counts,
+ loss_scale='dynamic',
+ dtype='float16')
+
+# learning policy
+# More information: https://github.com/open-mmlab/mmengine/blob/main/docs/en/tutorials/param_scheduler.md # noqa: E501
+param_scheduler = [
+ dict(
+ type=LinearLR,
+ start_factor=1e-5,
+ by_epoch=True,
+ begin=0,
+ end=warmup_ratio * max_epochs,
+ convert_to_iter_based=True),
+ dict(
+ type=CosineAnnealingLR,
+ eta_min=0.0,
+ by_epoch=True,
+ begin=warmup_ratio * max_epochs,
+ end=max_epochs,
+ convert_to_iter_based=True)
+]
+
+# train, val, test setting
+train_cfg = dict(type=TrainLoop, max_epochs=max_epochs)
+
+#######################################################################
+# PART 5 Runtime #
+#######################################################################
+# Log the dialogue periodically during the training process, optional
+custom_hooks = [
+ dict(type=DatasetInfoHook, tokenizer=tokenizer),
+ dict(
+ type=EvaluateChatHook,
+ tokenizer=tokenizer,
+ image_processor=image_processor,
+ every_n_iters=evaluation_freq,
+ evaluation_inputs=evaluation_inputs,
+ evaluation_images=evaluation_images,
+ system=SYSTEM,
+ prompt_template=prompt_template)
+]
+
+# configure default hooks
+default_hooks = dict(
+ # record the time of every iteration.
+ timer=dict(type=IterTimerHook),
+ # print log every 10 iterations.
+ logger=dict(type=LoggerHook, log_metric_by_epoch=False, interval=10),
+ # enable the parameter scheduler.
+ param_scheduler=dict(type=ParamSchedulerHook),
+ # save checkpoint per `save_steps`.
+ checkpoint=dict(
+ type=CheckpointHook,
+ by_epoch=False,
+ interval=save_steps,
+ max_keep_ckpts=save_total_limit),
+ # set sampler seed in distributed evrionment.
+ sampler_seed=dict(type=DistSamplerSeedHook),
+)
+
+# configure environment
+env_cfg = dict(
+ # whether to enable cudnn benchmark
+ cudnn_benchmark=False,
+ # set multi process parameters
+ mp_cfg=dict(mp_start_method='fork', opencv_num_threads=0),
+ # set distributed parameters
+ dist_cfg=dict(backend='nccl'),
+)
+
+# set visualizer
+visualizer = None
+
+# set log level
+log_level = 'INFO'
+
+# load from which checkpoint
+load_from = None
+
+# whether to resume training from the loaded checkpoint
+resume = False
+
+# Defaults to use random seed and disable `deterministic`
+randomness = dict(seed=None, deterministic=False)
+
+# set log processor
+log_processor = dict(by_epoch=False)
diff --git a/xtuner/configs/llava/phi3_mini_4k_instruct_clip_vit_large_p14_336/pretrain/llava_phi3_mini_4k_instruct_clip_vit_large_p14_336_e1_gpu8_pretrain.py b/xtuner/configs/llava/phi3_mini_4k_instruct_clip_vit_large_p14_336/pretrain/llava_phi3_mini_4k_instruct_clip_vit_large_p14_336_e1_gpu8_pretrain.py
new file mode 100644
index 000000000..cdd4bb484
--- /dev/null
+++ b/xtuner/configs/llava/phi3_mini_4k_instruct_clip_vit_large_p14_336/pretrain/llava_phi3_mini_4k_instruct_clip_vit_large_p14_336_e1_gpu8_pretrain.py
@@ -0,0 +1,199 @@
+# Copyright (c) OpenMMLab. All rights reserved.
+from mmengine.dataset import DefaultSampler
+from mmengine.hooks import (CheckpointHook, DistSamplerSeedHook, IterTimerHook,
+ LoggerHook, ParamSchedulerHook)
+from mmengine.optim import AmpOptimWrapper, CosineAnnealingLR, LinearLR
+from torch.optim import AdamW
+from transformers import (AutoModelForCausalLM, AutoTokenizer,
+ CLIPImageProcessor, CLIPVisionModel)
+
+from xtuner.dataset import LLaVADataset
+from xtuner.dataset.collate_fns import default_collate_fn
+from xtuner.dataset.map_fns import llava_map_fn, template_map_fn_factory
+from xtuner.engine.hooks import DatasetInfoHook, EvaluateChatHook
+from xtuner.engine.runner import TrainLoop
+from xtuner.model import LLaVAModel
+from xtuner.utils import PROMPT_TEMPLATE
+
+#######################################################################
+# PART 1 Settings #
+#######################################################################
+# Model
+llm_name_or_path = 'microsoft/Phi-3-mini-4k-instruct'
+visual_encoder_name_or_path = 'openai/clip-vit-large-patch14-336'
+
+# Data
+data_root = './data/llava_data/'
+data_path = data_root + 'LLaVA-Pretrain/blip_laion_cc_sbu_558k.json'
+image_folder = data_root + 'LLaVA-Pretrain/images'
+prompt_template = PROMPT_TEMPLATE.phi3_chat
+max_length = int(2048 - (336 / 14)**2)
+
+# Scheduler & Optimizer
+batch_size = 32 # per_device
+accumulative_counts = 1
+dataloader_num_workers = 4
+max_epochs = 1
+optim_type = AdamW
+lr = 1e-3
+betas = (0.9, 0.999)
+weight_decay = 0
+max_norm = 1 # grad clip
+warmup_ratio = 0.03
+
+# Save
+save_steps = 500
+save_total_limit = 2 # Maximum checkpoints to keep (-1 means unlimited)
+
+# Evaluate the generation performance during the training
+evaluation_freq = 500
+SYSTEM = ''
+evaluation_images = 'https://llava-vl.github.io/static/images/view.jpg'
+evaluation_inputs = ['请描述一下这张照片', 'Please describe this picture']
+
+#######################################################################
+# PART 2 Model & Tokenizer & Image Processor #
+#######################################################################
+tokenizer = dict(
+ type=AutoTokenizer.from_pretrained,
+ pretrained_model_name_or_path=llm_name_or_path,
+ trust_remote_code=True,
+ padding_side='right')
+
+image_processor = dict(
+ type=CLIPImageProcessor.from_pretrained,
+ pretrained_model_name_or_path=visual_encoder_name_or_path,
+ trust_remote_code=True)
+
+model = dict(
+ type=LLaVAModel,
+ freeze_llm=True,
+ freeze_visual_encoder=True,
+ llm=dict(
+ type=AutoModelForCausalLM.from_pretrained,
+ pretrained_model_name_or_path=llm_name_or_path,
+ trust_remote_code=True),
+ visual_encoder=dict(
+ type=CLIPVisionModel.from_pretrained,
+ pretrained_model_name_or_path=visual_encoder_name_or_path))
+
+#######################################################################
+# PART 3 Dataset & Dataloader #
+#######################################################################
+llava_dataset = dict(
+ type=LLaVADataset,
+ data_path=data_path,
+ image_folder=image_folder,
+ tokenizer=tokenizer,
+ image_processor=image_processor,
+ dataset_map_fn=llava_map_fn,
+ template_map_fn=dict(
+ type=template_map_fn_factory, template=prompt_template),
+ max_length=max_length,
+ pad_image_to_square=False)
+
+train_dataloader = dict(
+ batch_size=batch_size,
+ num_workers=dataloader_num_workers,
+ pin_memory=True,
+ dataset=llava_dataset,
+ sampler=dict(type=DefaultSampler, shuffle=True),
+ collate_fn=dict(type=default_collate_fn))
+
+#######################################################################
+# PART 4 Scheduler & Optimizer #
+#######################################################################
+# optimizer
+optim_wrapper = dict(
+ type=AmpOptimWrapper,
+ optimizer=dict(
+ type=optim_type, lr=lr, betas=betas, weight_decay=weight_decay),
+ clip_grad=dict(max_norm=max_norm, error_if_nonfinite=False),
+ accumulative_counts=accumulative_counts,
+ loss_scale='dynamic',
+ dtype='float16')
+
+# learning policy
+# More information: https://github.com/open-mmlab/mmengine/blob/main/docs/en/tutorials/param_scheduler.md # noqa: E501
+param_scheduler = [
+ dict(
+ type=LinearLR,
+ start_factor=1e-5,
+ by_epoch=True,
+ begin=0,
+ end=warmup_ratio * max_epochs,
+ convert_to_iter_based=True),
+ dict(
+ type=CosineAnnealingLR,
+ eta_min=0.0,
+ by_epoch=True,
+ begin=warmup_ratio * max_epochs,
+ end=max_epochs,
+ convert_to_iter_based=True)
+]
+
+# train, val, test setting
+train_cfg = dict(type=TrainLoop, max_epochs=max_epochs)
+
+#######################################################################
+# PART 5 Runtime #
+#######################################################################
+# Log the dialogue periodically during the training process, optional
+custom_hooks = [
+ dict(type=DatasetInfoHook, tokenizer=tokenizer),
+ dict(
+ type=EvaluateChatHook,
+ tokenizer=tokenizer,
+ image_processor=image_processor,
+ every_n_iters=evaluation_freq,
+ evaluation_inputs=evaluation_inputs,
+ evaluation_images=evaluation_images,
+ system=SYSTEM,
+ prompt_template=prompt_template)
+]
+
+# configure default hooks
+default_hooks = dict(
+ # record the time of every iteration.
+ timer=dict(type=IterTimerHook),
+ # print log every 10 iterations.
+ logger=dict(type=LoggerHook, log_metric_by_epoch=False, interval=10),
+ # enable the parameter scheduler.
+ param_scheduler=dict(type=ParamSchedulerHook),
+ # save checkpoint per `save_steps`.
+ checkpoint=dict(
+ type=CheckpointHook,
+ by_epoch=False,
+ interval=save_steps,
+ max_keep_ckpts=save_total_limit),
+ # set sampler seed in distributed evrionment.
+ sampler_seed=dict(type=DistSamplerSeedHook),
+)
+
+# configure environment
+env_cfg = dict(
+ # whether to enable cudnn benchmark
+ cudnn_benchmark=False,
+ # set multi process parameters
+ mp_cfg=dict(mp_start_method='fork', opencv_num_threads=0),
+ # set distributed parameters
+ dist_cfg=dict(backend='nccl'),
+)
+
+# set visualizer
+visualizer = None
+
+# set log level
+log_level = 'INFO'
+
+# load from which checkpoint
+load_from = None
+
+# whether to resume training from the loaded checkpoint
+resume = False
+
+# Defaults to use random seed and disable `deterministic`
+randomness = dict(seed=None, deterministic=False)
+
+# set log processor
+log_processor = dict(by_epoch=False)
diff --git a/xtuner/configs/llava/phi3_mini_4k_instruct_clip_vit_large_p14_336/pretrain/llava_phi3_mini_4k_instruct_clip_vit_large_p14_336_e1_gpu8_sharegpt4v_pretrain.py b/xtuner/configs/llava/phi3_mini_4k_instruct_clip_vit_large_p14_336/pretrain/llava_phi3_mini_4k_instruct_clip_vit_large_p14_336_e1_gpu8_sharegpt4v_pretrain.py
new file mode 100644
index 000000000..e74b12097
--- /dev/null
+++ b/xtuner/configs/llava/phi3_mini_4k_instruct_clip_vit_large_p14_336/pretrain/llava_phi3_mini_4k_instruct_clip_vit_large_p14_336_e1_gpu8_sharegpt4v_pretrain.py
@@ -0,0 +1,199 @@
+# Copyright (c) OpenMMLab. All rights reserved.
+from mmengine.dataset import DefaultSampler
+from mmengine.hooks import (CheckpointHook, DistSamplerSeedHook, IterTimerHook,
+ LoggerHook, ParamSchedulerHook)
+from mmengine.optim import AmpOptimWrapper, CosineAnnealingLR, LinearLR
+from torch.optim import AdamW
+from transformers import (AutoModelForCausalLM, AutoTokenizer,
+ CLIPImageProcessor, CLIPVisionModel)
+
+from xtuner.dataset import LLaVADataset
+from xtuner.dataset.collate_fns import default_collate_fn
+from xtuner.dataset.map_fns import llava_map_fn, template_map_fn_factory
+from xtuner.engine.hooks import DatasetInfoHook, EvaluateChatHook
+from xtuner.engine.runner import TrainLoop
+from xtuner.model import LLaVAModel
+from xtuner.utils import PROMPT_TEMPLATE
+
+#######################################################################
+# PART 1 Settings #
+#######################################################################
+# Model
+llm_name_or_path = 'microsoft/Phi-3-mini-4k-instruct'
+visual_encoder_name_or_path = 'openai/clip-vit-large-patch14-336'
+
+# Data
+data_root = './data/sharegpt4v/'
+data_path = data_root + 'share-captioner_coco_lcs_sam_1246k_1107.json'
+image_folder = data_root + 'data'
+prompt_template = PROMPT_TEMPLATE.phi3_chat
+max_length = int(4096 - (336 / 14)**2)
+
+# Scheduler & Optimizer
+batch_size = 16 # per_device
+accumulative_counts = 2
+dataloader_num_workers = 4
+max_epochs = 1
+optim_type = AdamW
+lr = 1e-3
+betas = (0.9, 0.999)
+weight_decay = 0
+max_norm = 1 # grad clip
+warmup_ratio = 0.03
+
+# Save
+save_steps = 1000
+save_total_limit = 2 # Maximum checkpoints to keep (-1 means unlimited)
+
+# Evaluate the generation performance during the training
+evaluation_freq = 1000
+SYSTEM = ''
+evaluation_images = 'https://llava-vl.github.io/static/images/view.jpg'
+evaluation_inputs = ['请描述一下这张照片', 'Please describe this picture']
+
+#######################################################################
+# PART 2 Model & Tokenizer & Image Processor #
+#######################################################################
+tokenizer = dict(
+ type=AutoTokenizer.from_pretrained,
+ pretrained_model_name_or_path=llm_name_or_path,
+ trust_remote_code=True,
+ padding_side='right')
+
+image_processor = dict(
+ type=CLIPImageProcessor.from_pretrained,
+ pretrained_model_name_or_path=visual_encoder_name_or_path,
+ trust_remote_code=True)
+
+model = dict(
+ type=LLaVAModel,
+ freeze_llm=True,
+ freeze_visual_encoder=True,
+ llm=dict(
+ type=AutoModelForCausalLM.from_pretrained,
+ pretrained_model_name_or_path=llm_name_or_path,
+ trust_remote_code=True),
+ visual_encoder=dict(
+ type=CLIPVisionModel.from_pretrained,
+ pretrained_model_name_or_path=visual_encoder_name_or_path))
+
+#######################################################################
+# PART 3 Dataset & Dataloader #
+#######################################################################
+llava_dataset = dict(
+ type=LLaVADataset,
+ data_path=data_path,
+ image_folder=image_folder,
+ tokenizer=tokenizer,
+ image_processor=image_processor,
+ dataset_map_fn=llava_map_fn,
+ template_map_fn=dict(
+ type=template_map_fn_factory, template=prompt_template),
+ max_length=max_length,
+ pad_image_to_square=False)
+
+train_dataloader = dict(
+ batch_size=batch_size,
+ num_workers=dataloader_num_workers,
+ pin_memory=True,
+ dataset=llava_dataset,
+ sampler=dict(type=DefaultSampler, shuffle=True),
+ collate_fn=dict(type=default_collate_fn))
+
+#######################################################################
+# PART 4 Scheduler & Optimizer #
+#######################################################################
+# optimizer
+optim_wrapper = dict(
+ type=AmpOptimWrapper,
+ optimizer=dict(
+ type=optim_type, lr=lr, betas=betas, weight_decay=weight_decay),
+ clip_grad=dict(max_norm=max_norm, error_if_nonfinite=False),
+ accumulative_counts=accumulative_counts,
+ loss_scale='dynamic',
+ dtype='float16')
+
+# learning policy
+# More information: https://github.com/open-mmlab/mmengine/blob/main/docs/en/tutorials/param_scheduler.md # noqa: E501
+param_scheduler = [
+ dict(
+ type=LinearLR,
+ start_factor=1e-5,
+ by_epoch=True,
+ begin=0,
+ end=warmup_ratio * max_epochs,
+ convert_to_iter_based=True),
+ dict(
+ type=CosineAnnealingLR,
+ eta_min=0.0,
+ by_epoch=True,
+ begin=warmup_ratio * max_epochs,
+ end=max_epochs,
+ convert_to_iter_based=True)
+]
+
+# train, val, test setting
+train_cfg = dict(type=TrainLoop, max_epochs=max_epochs)
+
+#######################################################################
+# PART 5 Runtime #
+#######################################################################
+# Log the dialogue periodically during the training process, optional
+custom_hooks = [
+ dict(type=DatasetInfoHook, tokenizer=tokenizer),
+ dict(
+ type=EvaluateChatHook,
+ tokenizer=tokenizer,
+ image_processor=image_processor,
+ every_n_iters=evaluation_freq,
+ evaluation_inputs=evaluation_inputs,
+ evaluation_images=evaluation_images,
+ system=SYSTEM,
+ prompt_template=prompt_template)
+]
+
+# configure default hooks
+default_hooks = dict(
+ # record the time of every iteration.
+ timer=dict(type=IterTimerHook),
+ # print log every 10 iterations.
+ logger=dict(type=LoggerHook, log_metric_by_epoch=False, interval=10),
+ # enable the parameter scheduler.
+ param_scheduler=dict(type=ParamSchedulerHook),
+ # save checkpoint per `save_steps`.
+ checkpoint=dict(
+ type=CheckpointHook,
+ by_epoch=False,
+ interval=save_steps,
+ max_keep_ckpts=save_total_limit),
+ # set sampler seed in distributed evrionment.
+ sampler_seed=dict(type=DistSamplerSeedHook),
+)
+
+# configure environment
+env_cfg = dict(
+ # whether to enable cudnn benchmark
+ cudnn_benchmark=False,
+ # set multi process parameters
+ mp_cfg=dict(mp_start_method='fork', opencv_num_threads=0),
+ # set distributed parameters
+ dist_cfg=dict(backend='nccl'),
+)
+
+# set visualizer
+visualizer = None
+
+# set log level
+log_level = 'INFO'
+
+# load from which checkpoint
+load_from = None
+
+# whether to resume training from the loaded checkpoint
+resume = False
+
+# Defaults to use random seed and disable `deterministic`
+randomness = dict(seed=None, deterministic=False)
+
+# set log processor
+log_processor = dict(by_epoch=False)
diff --git a/xtuner/configs/llava/vicuna_13b_v15_clip_vit_large_p14_336/finetune/llava_vicuna_13b_v15_qlora_clip_vit_large_p14_336_lora_e1_gpu8_finetune.py b/xtuner/configs/llava/vicuna_13b_v15_clip_vit_large_p14_336/finetune/llava_vicuna_13b_v15_qlora_clip_vit_large_p14_336_lora_e1_gpu8_finetune.py
index 20ca80759..a82c42c56 100644
--- a/xtuner/configs/llava/vicuna_13b_v15_clip_vit_large_p14_336/finetune/llava_vicuna_13b_v15_qlora_clip_vit_large_p14_336_lora_e1_gpu8_finetune.py
+++ b/xtuner/configs/llava/vicuna_13b_v15_clip_vit_large_p14_336/finetune/llava_vicuna_13b_v15_qlora_clip_vit_large_p14_336_lora_e1_gpu8_finetune.py
@@ -37,7 +37,7 @@
# Scheduler & Optimizer
batch_size = 16 # per_device
accumulative_counts = 1
-dataloader_num_workers = 0
+dataloader_num_workers = 4
max_epochs = 1
optim_type = AdamW
lr = 2e-4
@@ -120,6 +120,7 @@
train_dataloader = dict(
batch_size=batch_size,
num_workers=dataloader_num_workers,
+ pin_memory=True,
dataset=llava_dataset,
sampler=dict(
type=LengthGroupedSampler,
diff --git a/xtuner/configs/llava/vicuna_13b_v15_clip_vit_large_p14_336/pretrain/llava_vicuna_13b_v15_clip_vit_large_p14_336_e1_gpu8_pretrain.py b/xtuner/configs/llava/vicuna_13b_v15_clip_vit_large_p14_336/pretrain/llava_vicuna_13b_v15_clip_vit_large_p14_336_e1_gpu8_pretrain.py
index cda913ba6..d0620fe61 100644
--- a/xtuner/configs/llava/vicuna_13b_v15_clip_vit_large_p14_336/pretrain/llava_vicuna_13b_v15_clip_vit_large_p14_336_e1_gpu8_pretrain.py
+++ b/xtuner/configs/llava/vicuna_13b_v15_clip_vit_large_p14_336/pretrain/llava_vicuna_13b_v15_clip_vit_large_p14_336_e1_gpu8_pretrain.py
@@ -34,7 +34,7 @@
# Scheduler & Optimizer
batch_size = 32 # per_device
accumulative_counts = 1
-dataloader_num_workers = 0
+dataloader_num_workers = 4
max_epochs = 1
optim_type = AdamW
lr = 1e-3
@@ -107,6 +107,7 @@
train_dataloader = dict(
batch_size=batch_size,
num_workers=dataloader_num_workers,
+ pin_memory=True,
dataset=llava_dataset,
sampler=dict(type=DefaultSampler, shuffle=True),
collate_fn=dict(type=default_collate_fn))
diff --git a/xtuner/configs/llava/vicuna_7b_v15_clip_vit_large_p14_336/finetune/llava_vicuna_7b_v15_qlora_clip_vit_large_p14_336_lora_e1_gpu8_finetune.py b/xtuner/configs/llava/vicuna_7b_v15_clip_vit_large_p14_336/finetune/llava_vicuna_7b_v15_qlora_clip_vit_large_p14_336_lora_e1_gpu8_finetune.py
index b10c7547e..21d80a8ca 100644
--- a/xtuner/configs/llava/vicuna_7b_v15_clip_vit_large_p14_336/finetune/llava_vicuna_7b_v15_qlora_clip_vit_large_p14_336_lora_e1_gpu8_finetune.py
+++ b/xtuner/configs/llava/vicuna_7b_v15_clip_vit_large_p14_336/finetune/llava_vicuna_7b_v15_qlora_clip_vit_large_p14_336_lora_e1_gpu8_finetune.py
@@ -37,7 +37,7 @@
# Scheduler & Optimizer
batch_size = 16 # per_device
accumulative_counts = 1
-dataloader_num_workers = 0
+dataloader_num_workers = 4
max_epochs = 1
optim_type = AdamW
lr = 2e-4
@@ -120,6 +120,7 @@
train_dataloader = dict(
batch_size=batch_size,
num_workers=dataloader_num_workers,
+ pin_memory=True,
dataset=llava_dataset,
sampler=dict(
type=LengthGroupedSampler,
diff --git a/xtuner/configs/llava/vicuna_7b_v15_clip_vit_large_p14_336/finetune/llava_vicuna_7b_v15_qlora_clip_vit_large_p14_336_lora_e1_gpu8_finetune_refcoco.py b/xtuner/configs/llava/vicuna_7b_v15_clip_vit_large_p14_336/finetune/llava_vicuna_7b_v15_qlora_clip_vit_large_p14_336_lora_e1_gpu8_finetune_refcoco.py
index 803d54435..c3fb0f832 100644
--- a/xtuner/configs/llava/vicuna_7b_v15_clip_vit_large_p14_336/finetune/llava_vicuna_7b_v15_qlora_clip_vit_large_p14_336_lora_e1_gpu8_finetune_refcoco.py
+++ b/xtuner/configs/llava/vicuna_7b_v15_clip_vit_large_p14_336/finetune/llava_vicuna_7b_v15_qlora_clip_vit_large_p14_336_lora_e1_gpu8_finetune_refcoco.py
@@ -40,7 +40,7 @@
# Scheduler & Optimizer
batch_size = 16 # per_device
accumulative_counts = 1
-dataloader_num_workers = 0
+dataloader_num_workers = 4
max_epochs = 1
optim_type = AdamW
lr = 2e-4
@@ -157,6 +157,7 @@
train_dataloader = dict(
batch_size=batch_size,
num_workers=dataloader_num_workers,
+ pin_memory=True,
dataset=train_dataset,
sampler=dict(
type=LengthGroupedSampler,
diff --git a/xtuner/configs/llava/vicuna_7b_v15_clip_vit_large_p14_336/pretrain/llava_vicuna_7b_v15_clip_vit_large_p14_336_e1_gpu8_pretrain.py b/xtuner/configs/llava/vicuna_7b_v15_clip_vit_large_p14_336/pretrain/llava_vicuna_7b_v15_clip_vit_large_p14_336_e1_gpu8_pretrain.py
index bbe3b8c0f..46c6f4c9d 100644
--- a/xtuner/configs/llava/vicuna_7b_v15_clip_vit_large_p14_336/pretrain/llava_vicuna_7b_v15_clip_vit_large_p14_336_e1_gpu8_pretrain.py
+++ b/xtuner/configs/llava/vicuna_7b_v15_clip_vit_large_p14_336/pretrain/llava_vicuna_7b_v15_clip_vit_large_p14_336_e1_gpu8_pretrain.py
@@ -34,7 +34,7 @@
# Scheduler & Optimizer
batch_size = 32 # per_device
accumulative_counts = 1
-dataloader_num_workers = 0
+dataloader_num_workers = 4
max_epochs = 1
optim_type = AdamW
lr = 1e-3
@@ -107,6 +107,7 @@
train_dataloader = dict(
batch_size=batch_size,
num_workers=dataloader_num_workers,
+ pin_memory=True,
dataset=llava_dataset,
sampler=dict(type=DefaultSampler, shuffle=True),
collate_fn=dict(type=default_collate_fn))
diff --git a/xtuner/configs/minicpm/1_2b/minicpm_1b_dpo_qlora.py b/xtuner/configs/minicpm/1_2b/minicpm_1b_dpo_qlora.py
new file mode 100644
index 000000000..b0fc4556a
--- /dev/null
+++ b/xtuner/configs/minicpm/1_2b/minicpm_1b_dpo_qlora.py
@@ -0,0 +1,221 @@
+# Copyright (c) OpenMMLab. All rights reserved.
+import torch
+from datasets import load_dataset
+from mmengine.dataset import DefaultSampler
+from mmengine.hooks import (CheckpointHook, DistSamplerSeedHook, IterTimerHook,
+ LoggerHook, ParamSchedulerHook)
+from mmengine.optim import AmpOptimWrapper, CosineAnnealingLR, LinearLR
+from peft import LoraConfig
+from torch.optim import AdamW
+from transformers import (AutoModelForCausalLM, AutoTokenizer,
+ BitsAndBytesConfig)
+
+from xtuner.dataset.collate_fns.preference_collate_fn import \
+ preference_collate_fn
+from xtuner.dataset.preference_dataset import (build_preference_dataset,
+ orpo_dpo_mix_40k_map_fn)
+from xtuner.engine.hooks import (DatasetInfoHook, EvaluateChatHook,
+ VarlenAttnArgsToMessageHubHook)
+from xtuner.engine.runner import TrainLoop
+from xtuner.model.dpo import DPO
+from xtuner.utils import PROMPT_TEMPLATE, SYSTEM_TEMPLATE
+
+#######################################################################
+# PART 1 Settings #
+#######################################################################
+# Model
+pretrained_model_name_or_path = 'openbmb/MiniCPM-1B-sft-bf16'
+use_varlen_attn = False
+dpo_loss_type = 'sigmoid' # One of ['sigmoid', 'hinge', 'ipo', 'kto_pair', 'sppo_hard', 'nca_pair', 'robust'] # noqa: E501
+loss_beta = 0.1
+label_smoothing = 0.0
+
+# Data
+prompt_template = PROMPT_TEMPLATE.minicpm
+max_length = 2048
+
+# Scheduler & Optimizer
+batch_size = 1 # per_device
+accumulative_counts = 16
+dataloader_num_workers = 0
+max_epochs = 3
+optim_type = AdamW
+lr = 5e-7 # refer to alignment handbook
+betas = (0.9, 0.999)
+weight_decay = 0
+max_norm = 1 # grad clip
+warmup_ratio = 0.03
+
+# Save
+save_steps = 500
+save_total_limit = 2 # Maximum checkpoints to keep (-1 means unlimited)
+
+# Evaluate the generation performance during the training
+evaluation_freq = 500
+SYSTEM = SYSTEM_TEMPLATE.alpaca
+evaluation_inputs = [
+ 'What famous British author, known for his tales of mystery and the macabre, shares his initials with a common abbreviation for "rest in peace"?', # noqa: E501
+ 'Please tell me five scenic spots in Shanghai',
+ '890729 - 425663? Only respond with math and no words.'
+]
+
+#######################################################################
+# PART 2 Model & Tokenizer #
+#######################################################################
+tokenizer = dict(
+ type=AutoTokenizer.from_pretrained,
+ pretrained_model_name_or_path=pretrained_model_name_or_path,
+ trust_remote_code=True,
+ padding_side='right')
+
+model = dict(
+ type=DPO,
+ use_varlen_attn=use_varlen_attn,
+ loss_type=dpo_loss_type,
+ beta=loss_beta,
+ label_smoothing=label_smoothing,
+ llm=dict(
+ type=AutoModelForCausalLM.from_pretrained,
+ pretrained_model_name_or_path=pretrained_model_name_or_path,
+ trust_remote_code=True,
+ torch_dtype=torch.float16,
+ quantization_config=dict(
+ type=BitsAndBytesConfig,
+ load_in_4bit=True,
+ load_in_8bit=False,
+ llm_int8_threshold=6.0,
+ llm_int8_has_fp16_weight=False,
+ bnb_4bit_compute_dtype=torch.float16,
+ bnb_4bit_use_double_quant=True,
+ bnb_4bit_quant_type='nf4')),
+ lora=dict(
+ type=LoraConfig,
+ r=64,
+ lora_alpha=16,
+ lora_dropout=0.1,
+ bias='none',
+ task_type='CAUSAL_LM'))
+
+#######################################################################
+# PART 3 Dataset & Dataloader #
+#######################################################################
+train_dataset = dict(
+ type=build_preference_dataset,
+ dataset=dict(type=load_dataset, path='mlabonne/orpo-dpo-mix-40k'),
+ tokenizer=tokenizer,
+ max_length=max_length,
+ dataset_map_fn=orpo_dpo_mix_40k_map_fn,
+ is_dpo=True,
+ is_reward=False,
+ reward_token_id=-1,
+ num_proc=32,
+ use_varlen_attn=use_varlen_attn,
+ shuffle_before_pack=True,
+)
+
+train_dataloader = dict(
+ batch_size=batch_size,
+ num_workers=dataloader_num_workers,
+ dataset=train_dataset,
+ sampler=dict(type=DefaultSampler, shuffle=True),
+ collate_fn=dict(
+ type=preference_collate_fn, use_varlen_attn=use_varlen_attn))
+
+#######################################################################
+# PART 4 Scheduler & Optimizer #
+#######################################################################
+# optimizer
+optim_wrapper = dict(
+ type=AmpOptimWrapper,
+ optimizer=dict(
+ type=optim_type, lr=lr, betas=betas, weight_decay=weight_decay),
+ clip_grad=dict(max_norm=max_norm, error_if_nonfinite=False),
+ accumulative_counts=accumulative_counts,
+ loss_scale='dynamic',
+ dtype='float16')
+
+# learning policy
+# More information: https://github.com/open-mmlab/mmengine/blob/main/docs/en/tutorials/param_scheduler.md # noqa: E501
+param_scheduler = [
+ dict(
+ type=LinearLR,
+ start_factor=1e-5,
+ by_epoch=True,
+ begin=0,
+ end=warmup_ratio * max_epochs,
+ convert_to_iter_based=True),
+ dict(
+ type=CosineAnnealingLR,
+ eta_min=0.0,
+ by_epoch=True,
+ begin=warmup_ratio * max_epochs,
+ end=max_epochs,
+ convert_to_iter_based=True)
+]
+
+# train, val, test setting
+train_cfg = dict(type=TrainLoop, max_epochs=max_epochs)
+
+#######################################################################
+# PART 5 Runtime #
+#######################################################################
+# Log the dialogue periodically during the training process, optional
+custom_hooks = [
+ dict(type=DatasetInfoHook, tokenizer=tokenizer),
+ dict(
+ type=EvaluateChatHook,
+ tokenizer=tokenizer,
+ every_n_iters=evaluation_freq,
+ evaluation_inputs=evaluation_inputs,
+ system=SYSTEM,
+ prompt_template=prompt_template)
+]
+
+if use_varlen_attn:
+ custom_hooks += [dict(type=VarlenAttnArgsToMessageHubHook)]
+
+# configure default hooks
+default_hooks = dict(
+ # record the time of every iteration.
+ timer=dict(type=IterTimerHook),
+ # print log every 10 iterations.
+ logger=dict(type=LoggerHook, log_metric_by_epoch=False, interval=10),
+ # enable the parameter scheduler.
+ param_scheduler=dict(type=ParamSchedulerHook),
+ # save checkpoint per `save_steps`.
+ checkpoint=dict(
+ type=CheckpointHook,
+ by_epoch=False,
+ interval=save_steps,
+ max_keep_ckpts=save_total_limit),
+ # set sampler seed in distributed evrionment.
+ sampler_seed=dict(type=DistSamplerSeedHook),
+)
+
+# configure environment
+env_cfg = dict(
+ # whether to enable cudnn benchmark
+ cudnn_benchmark=False,
+ # set multi process parameters
+ mp_cfg=dict(mp_start_method='fork', opencv_num_threads=0),
+ # set distributed parameters
+ dist_cfg=dict(backend='nccl'),
+)
+
+# set visualizer
+visualizer = None
+
+# set log level
+log_level = 'INFO'
+
+# load from which checkpoint
+load_from = None
+
+# whether to resume training from the loaded checkpoint
+resume = False
+
+# Defaults to use random seed and disable `deterministic`
+randomness = dict(seed=None, deterministic=False)
+
+# set log processor
+log_processor = dict(by_epoch=False)
diff --git a/xtuner/configs/minicpm/1_2b/minicpm_1b_full_alpaca_zh_e3.py b/xtuner/configs/minicpm/1_2b/minicpm_1b_full_alpaca_zh_e3.py
new file mode 100644
index 000000000..2c1e37ff3
--- /dev/null
+++ b/xtuner/configs/minicpm/1_2b/minicpm_1b_full_alpaca_zh_e3.py
@@ -0,0 +1,201 @@
+# Copyright (c) OpenMMLab. All rights reserved.
+from datasets import load_dataset
+from mmengine.dataset import DefaultSampler
+from mmengine.hooks import (CheckpointHook, DistSamplerSeedHook, IterTimerHook,
+ LoggerHook, ParamSchedulerHook)
+from mmengine.optim import AmpOptimWrapper, CosineAnnealingLR, LinearLR
+from torch.optim import AdamW
+from transformers import AutoModelForCausalLM, AutoTokenizer
+
+from xtuner.dataset import process_hf_dataset
+from xtuner.dataset.collate_fns import default_collate_fn
+from xtuner.dataset.map_fns import alpaca_zh_map_fn, template_map_fn_factory
+from xtuner.engine.hooks import (DatasetInfoHook, EvaluateChatHook,
+ VarlenAttnArgsToMessageHubHook)
+from xtuner.engine.runner import TrainLoop
+from xtuner.model import SupervisedFinetune
+from xtuner.parallel.sequence import SequenceParallelSampler
+from xtuner.utils import PROMPT_TEMPLATE, SYSTEM_TEMPLATE
+
+#######################################################################
+# PART 1 Settings #
+#######################################################################
+# Model
+pretrained_model_name_or_path = 'openbmb/MiniCPM-1B-sft-bf16'
+use_varlen_attn = False
+
+# Data
+alpaca_en_path = 'silk-road/alpaca-data-gpt4-chinese'
+prompt_template = PROMPT_TEMPLATE.minicpm
+max_length = 2048
+pack_to_max_length = True
+
+# parallel
+sequence_parallel_size = 1
+
+# Scheduler & Optimizer
+batch_size = 1 # per_device
+accumulative_counts = 16
+accumulative_counts *= sequence_parallel_size
+dataloader_num_workers = 0
+max_epochs = 3
+optim_type = AdamW
+lr = 2e-5
+betas = (0.9, 0.999)
+weight_decay = 0
+max_norm = 1 # grad clip
+warmup_ratio = 0.03
+
+# Save
+save_steps = 500
+save_total_limit = 2 # Maximum checkpoints to keep (-1 means unlimited)
+
+# Evaluate the generation performance during the training
+evaluation_freq = 500
+SYSTEM = SYSTEM_TEMPLATE.alpaca
+evaluation_inputs = [
+ '请给我介绍五个上海的景点', 'Please tell me five scenic spots in Shanghai'
+]
+
+#######################################################################
+# PART 2 Model & Tokenizer #
+#######################################################################
+tokenizer = dict(
+ type=AutoTokenizer.from_pretrained,
+ pretrained_model_name_or_path=pretrained_model_name_or_path,
+ trust_remote_code=True,
+ padding_side='right',
+ eos_token='')
+
+model = dict(
+ type=SupervisedFinetune,
+ use_varlen_attn=use_varlen_attn,
+ llm=dict(
+ type=AutoModelForCausalLM.from_pretrained,
+ pretrained_model_name_or_path=pretrained_model_name_or_path,
+ trust_remote_code=True))
+
+#######################################################################
+# PART 3 Dataset & Dataloader #
+#######################################################################
+alpaca_en = dict(
+ type=process_hf_dataset,
+ dataset=dict(type=load_dataset, path=alpaca_en_path),
+ tokenizer=tokenizer,
+ max_length=max_length,
+ dataset_map_fn=alpaca_zh_map_fn,
+ template_map_fn=dict(
+ type=template_map_fn_factory, template=prompt_template),
+ remove_unused_columns=True,
+ shuffle_before_pack=True,
+ pack_to_max_length=pack_to_max_length,
+ use_varlen_attn=use_varlen_attn)
+
+sampler = SequenceParallelSampler \
+ if sequence_parallel_size > 1 else DefaultSampler
+
+train_dataloader = dict(
+ batch_size=batch_size,
+ num_workers=dataloader_num_workers,
+ dataset=alpaca_en,
+ sampler=dict(type=sampler, shuffle=True),
+ collate_fn=dict(type=default_collate_fn, use_varlen_attn=use_varlen_attn))
+
+#######################################################################
+# PART 4 Scheduler & Optimizer #
+#######################################################################
+# optimizer
+optim_wrapper = dict(
+ type=AmpOptimWrapper,
+ optimizer=dict(
+ type=optim_type, lr=lr, betas=betas, weight_decay=weight_decay),
+ clip_grad=dict(max_norm=max_norm, error_if_nonfinite=False),
+ accumulative_counts=accumulative_counts,
+ loss_scale='dynamic',
+ dtype='float16')
+
+# learning policy
+# More information: https://github.com/open-mmlab/mmengine/blob/main/docs/en/tutorials/param_scheduler.md # noqa: E501
+param_scheduler = [
+ dict(
+ type=LinearLR,
+ start_factor=1e-5,
+ by_epoch=True,
+ begin=0,
+ end=warmup_ratio * max_epochs,
+ convert_to_iter_based=True),
+ dict(
+ type=CosineAnnealingLR,
+ eta_min=0.0,
+ by_epoch=True,
+ begin=warmup_ratio * max_epochs,
+ end=max_epochs,
+ convert_to_iter_based=True)
+]
+
+# train, val, test setting
+train_cfg = dict(type=TrainLoop, max_epochs=max_epochs)
+
+#######################################################################
+# PART 5 Runtime #
+#######################################################################
+# Log the dialogue periodically during the training process, optional
+custom_hooks = [
+ dict(type=DatasetInfoHook, tokenizer=tokenizer),
+ dict(
+ type=EvaluateChatHook,
+ tokenizer=tokenizer,
+ every_n_iters=evaluation_freq,
+ evaluation_inputs=evaluation_inputs,
+ system=SYSTEM,
+ prompt_template=prompt_template)
+]
+
+if use_varlen_attn:
+ custom_hooks += [dict(type=VarlenAttnArgsToMessageHubHook)]
+
+# configure default hooks
+default_hooks = dict(
+ # record the time of every iteration.
+ timer=dict(type=IterTimerHook),
+ # print log every 10 iterations.
+ logger=dict(type=LoggerHook, log_metric_by_epoch=False, interval=10),
+ # enable the parameter scheduler.
+ param_scheduler=dict(type=ParamSchedulerHook),
+ # save checkpoint per `save_steps`.
+ checkpoint=dict(
+ type=CheckpointHook,
+ by_epoch=False,
+ interval=save_steps,
+ max_keep_ckpts=save_total_limit),
+ # set sampler seed in distributed evrionment.
+ sampler_seed=dict(type=DistSamplerSeedHook),
+)
+
+# configure environment
+env_cfg = dict(
+ # whether to enable cudnn benchmark
+ cudnn_benchmark=False,
+ # set multi process parameters
+ mp_cfg=dict(mp_start_method='fork', opencv_num_threads=0),
+ # set distributed parameters
+ dist_cfg=dict(backend='nccl'),
+)
+
+# set visualizer
+visualizer = None
+
+# set log level
+log_level = 'INFO'
+
+# load from which checkpoint
+load_from = None
+
+# whether to resume training from the loaded checkpoint
+resume = False
+
+# Defaults to use random seed and disable `deterministic`
+randomness = dict(seed=None, deterministic=False)
+
+# set log processor
+log_processor = dict(by_epoch=False)
diff --git a/xtuner/configs/minicpm/1_2b/minicpm_1b_lora_alpaca_zh_e3.py b/xtuner/configs/minicpm/1_2b/minicpm_1b_lora_alpaca_zh_e3.py
new file mode 100644
index 000000000..e0ed46147
--- /dev/null
+++ b/xtuner/configs/minicpm/1_2b/minicpm_1b_lora_alpaca_zh_e3.py
@@ -0,0 +1,212 @@
+# Copyright (c) OpenMMLab. All rights reserved.
+import torch
+from datasets import load_dataset
+from mmengine.dataset import DefaultSampler
+from mmengine.hooks import (CheckpointHook, DistSamplerSeedHook, IterTimerHook,
+ LoggerHook, ParamSchedulerHook)
+from mmengine.optim import AmpOptimWrapper, CosineAnnealingLR, LinearLR
+from peft import LoraConfig
+from torch.optim import AdamW
+from transformers import AutoModelForCausalLM, AutoTokenizer
+
+from xtuner.dataset import process_hf_dataset
+from xtuner.dataset.collate_fns import default_collate_fn
+from xtuner.dataset.map_fns import alpaca_zh_map_fn, template_map_fn_factory
+from xtuner.engine.hooks import (DatasetInfoHook, EvaluateChatHook,
+ VarlenAttnArgsToMessageHubHook)
+from xtuner.engine.runner import TrainLoop
+from xtuner.model import SupervisedFinetune
+from xtuner.parallel.sequence import SequenceParallelSampler
+from xtuner.utils import PROMPT_TEMPLATE, SYSTEM_TEMPLATE
+
+#######################################################################
+# PART 1 Settings #
+#######################################################################
+# Model
+pretrained_model_name_or_path = 'openbmb/MiniCPM-1B-sft-bf16'
+use_varlen_attn = False
+
+# Data
+alpaca_zh_path = 'silk-road/alpaca-data-gpt4-chinese'
+prompt_template = PROMPT_TEMPLATE.minicpm
+max_length = 2048
+pack_to_max_length = True
+
+# parallel
+sequence_parallel_size = 1
+
+# Scheduler & Optimizer
+batch_size = 1 # per_device
+accumulative_counts = 16
+accumulative_counts *= sequence_parallel_size
+dataloader_num_workers = 0
+max_epochs = 3
+optim_type = AdamW
+lr = 2e-4
+betas = (0.9, 0.999)
+weight_decay = 0
+max_norm = 1 # grad clip
+warmup_ratio = 0.03
+gradient_checkpointing = True
+# Save
+save_steps = 500
+save_total_limit = 2 # Maximum checkpoints to keep (-1 means unlimited)
+
+# Evaluate the generation performance during the training
+evaluation_freq = 500
+SYSTEM = SYSTEM_TEMPLATE.alpaca
+evaluation_inputs = [
+ '请给我介绍五个上海的景点', 'Please tell me five scenic spots in Shanghai'
+]
+
+#######################################################################
+# PART 2 Model & Tokenizer #
+#######################################################################
+tokenizer = dict(
+ type=AutoTokenizer.from_pretrained,
+ pretrained_model_name_or_path=pretrained_model_name_or_path,
+ trust_remote_code=True,
+ padding_side='right',
+ eos_token='')
+
+model = dict(
+ type=SupervisedFinetune,
+ use_varlen_attn=use_varlen_attn,
+ llm=dict(
+ type=AutoModelForCausalLM.from_pretrained,
+ pretrained_model_name_or_path=pretrained_model_name_or_path,
+ trust_remote_code=True,
+ torch_dtype=torch.float16,
+ ),
+ lora=dict(
+ type=LoraConfig,
+ r=64,
+ lora_alpha=16,
+ lora_dropout=0.1,
+ bias='none',
+ task_type='CAUSAL_LM'))
+
+#######################################################################
+# PART 3 Dataset & Dataloader #
+#######################################################################
+alpaca_zh = dict(
+ type=process_hf_dataset,
+ dataset=dict(type=load_dataset, path=alpaca_zh_path),
+ tokenizer=tokenizer,
+ max_length=max_length,
+ dataset_map_fn=alpaca_zh_map_fn,
+ template_map_fn=dict(
+ type=template_map_fn_factory, template=prompt_template),
+ remove_unused_columns=True,
+ shuffle_before_pack=True,
+ pack_to_max_length=pack_to_max_length,
+ use_varlen_attn=use_varlen_attn)
+
+sampler = SequenceParallelSampler \
+ if sequence_parallel_size > 1 else DefaultSampler
+
+train_dataloader = dict(
+ batch_size=batch_size,
+ num_workers=dataloader_num_workers,
+ dataset=alpaca_zh,
+ sampler=dict(type=sampler, shuffle=True),
+ collate_fn=dict(type=default_collate_fn, use_varlen_attn=use_varlen_attn))
+
+#######################################################################
+# PART 4 Scheduler & Optimizer #
+#######################################################################
+# optimizer
+optim_wrapper = dict(
+ type=AmpOptimWrapper,
+ optimizer=dict(
+ type=optim_type, lr=lr, betas=betas, weight_decay=weight_decay),
+ clip_grad=dict(max_norm=max_norm, error_if_nonfinite=False),
+ accumulative_counts=accumulative_counts,
+ loss_scale='dynamic',
+ dtype='float16')
+
+# learning policy
+# More information: https://github.com/open-mmlab/mmengine/blob/main/docs/en/tutorials/param_scheduler.md # noqa: E501
+param_scheduler = [
+ dict(
+ type=LinearLR,
+ start_factor=1e-5,
+ by_epoch=True,
+ begin=0,
+ end=warmup_ratio * max_epochs,
+ convert_to_iter_based=True),
+ dict(
+ type=CosineAnnealingLR,
+ eta_min=0.0,
+ by_epoch=True,
+ begin=warmup_ratio * max_epochs,
+ end=max_epochs,
+ convert_to_iter_based=True)
+]
+
+# train, val, test setting
+train_cfg = dict(type=TrainLoop, max_epochs=max_epochs)
+
+#######################################################################
+# PART 5 Runtime #
+#######################################################################
+# Log the dialogue periodically during the training process, optional
+custom_hooks = [
+ dict(type=DatasetInfoHook, tokenizer=tokenizer),
+ dict(
+ type=EvaluateChatHook,
+ tokenizer=tokenizer,
+ every_n_iters=evaluation_freq,
+ evaluation_inputs=evaluation_inputs,
+ system=SYSTEM,
+ prompt_template=prompt_template)
+]
+
+if use_varlen_attn:
+ custom_hooks += [dict(type=VarlenAttnArgsToMessageHubHook)]
+
+# configure default hooks
+default_hooks = dict(
+ # record the time of every iteration.
+ timer=dict(type=IterTimerHook),
+ # print log every 10 iterations.
+ logger=dict(type=LoggerHook, log_metric_by_epoch=False, interval=10),
+ # enable the parameter scheduler.
+ param_scheduler=dict(type=ParamSchedulerHook),
+ # save checkpoint per `save_steps`.
+ checkpoint=dict(
+ type=CheckpointHook,
+ by_epoch=False,
+ interval=save_steps,
+ max_keep_ckpts=save_total_limit),
+ # set sampler seed in distributed evrionment.
+ sampler_seed=dict(type=DistSamplerSeedHook),
+)
+
+# configure environment
+env_cfg = dict(
+ # whether to enable cudnn benchmark
+ cudnn_benchmark=False,
+ # set multi process parameters
+ mp_cfg=dict(mp_start_method='fork', opencv_num_threads=0),
+ # set distributed parameters
+ dist_cfg=dict(backend='nccl'),
+)
+
+# set visualizer
+visualizer = None
+
+# set log level
+log_level = 'INFO'
+
+# load from which checkpoint
+load_from = None
+
+# whether to resume training from the loaded checkpoint
+resume = False
+
+# Defaults to use random seed and disable `deterministic`
+randomness = dict(seed=None, deterministic=False)
+
+# set log processor
+log_processor = dict(by_epoch=False)
diff --git a/xtuner/configs/minicpm/1_2b/minicpm_1b_qlora_alpaca_enzh_e3.py b/xtuner/configs/minicpm/1_2b/minicpm_1b_qlora_alpaca_enzh_e3.py
new file mode 100644
index 000000000..0adc91aec
--- /dev/null
+++ b/xtuner/configs/minicpm/1_2b/minicpm_1b_qlora_alpaca_enzh_e3.py
@@ -0,0 +1,238 @@
+# Copyright (c) OpenMMLab. All rights reserved.
+import torch
+from datasets import load_dataset
+from mmengine.dataset import DefaultSampler
+from mmengine.hooks import (CheckpointHook, DistSamplerSeedHook, IterTimerHook,
+ LoggerHook, ParamSchedulerHook)
+from mmengine.optim import AmpOptimWrapper, CosineAnnealingLR, LinearLR
+from peft import LoraConfig
+from torch.optim import AdamW
+from transformers import (AutoModelForCausalLM, AutoTokenizer,
+ BitsAndBytesConfig)
+
+from xtuner.dataset import ConcatDataset, process_hf_dataset
+from xtuner.dataset.collate_fns import default_collate_fn
+from xtuner.dataset.map_fns import (alpaca_map_fn, alpaca_zh_map_fn,
+ template_map_fn_factory)
+from xtuner.engine.hooks import (DatasetInfoHook, EvaluateChatHook,
+ VarlenAttnArgsToMessageHubHook)
+from xtuner.engine.runner import TrainLoop
+from xtuner.model import SupervisedFinetune
+from xtuner.parallel.sequence import SequenceParallelSampler
+from xtuner.utils import PROMPT_TEMPLATE, SYSTEM_TEMPLATE
+
+#######################################################################
+# PART 1 Settings #
+#######################################################################
+# Model
+pretrained_model_name_or_path = 'openbmb/MiniCPM-1B-sft-bf16'
+use_varlen_attn = False
+
+# Data
+alpaca_zh_path = 'silk-road/alpaca-data-gpt4-chinese'
+alpaca_en_path = 'tatsu-lab/alpaca'
+prompt_template = PROMPT_TEMPLATE.minicpm
+max_length = 2048
+pack_to_max_length = True
+
+# parallel
+sequence_parallel_size = 1
+
+# Scheduler & Optimizer
+batch_size = 1 # per_device
+accumulative_counts = 16
+accumulative_counts *= sequence_parallel_size
+dataloader_num_workers = 0
+max_epochs = 3
+optim_type = AdamW
+lr = 2e-4
+betas = (0.9, 0.999)
+weight_decay = 0
+max_norm = 1 # grad clip
+warmup_ratio = 0.03
+
+# Save
+save_steps = 500
+save_total_limit = 2 # Maximum checkpoints to keep (-1 means unlimited)
+
+# Evaluate the generation performance during the training
+evaluation_freq = 500
+SYSTEM = SYSTEM_TEMPLATE.alpaca
+evaluation_inputs = [
+ '请给我介绍五个上海的景点', 'Please tell me five scenic spots in Shanghai'
+]
+
+#######################################################################
+# PART 2 Model & Tokenizer #
+#######################################################################
+tokenizer = dict(
+ type=AutoTokenizer.from_pretrained,
+ pretrained_model_name_or_path=pretrained_model_name_or_path,
+ trust_remote_code=True,
+ padding_side='right',
+ eos_token='')
+
+model = dict(
+ type=SupervisedFinetune,
+ use_varlen_attn=use_varlen_attn,
+ llm=dict(
+ type=AutoModelForCausalLM.from_pretrained,
+ pretrained_model_name_or_path=pretrained_model_name_or_path,
+ trust_remote_code=True,
+ torch_dtype=torch.float16,
+ quantization_config=dict(
+ type=BitsAndBytesConfig,
+ load_in_4bit=True,
+ load_in_8bit=False,
+ llm_int8_threshold=6.0,
+ llm_int8_has_fp16_weight=False,
+ bnb_4bit_compute_dtype=torch.float16,
+ bnb_4bit_use_double_quant=True,
+ bnb_4bit_quant_type='nf4')),
+ lora=dict(
+ type=LoraConfig,
+ r=64,
+ lora_alpha=16,
+ lora_dropout=0.1,
+ bias='none',
+ task_type='CAUSAL_LM'))
+
+#######################################################################
+# PART 3 Dataset & Dataloader #
+#######################################################################
+alpaca_en = dict(
+ type=process_hf_dataset,
+ dataset=dict(type=load_dataset, path=alpaca_en_path),
+ tokenizer=tokenizer,
+ max_length=max_length,
+ dataset_map_fn=alpaca_map_fn,
+ template_map_fn=dict(
+ type=template_map_fn_factory, template=prompt_template),
+ remove_unused_columns=True,
+ shuffle_before_pack=True,
+ pack_to_max_length=pack_to_max_length,
+ use_varlen_attn=use_varlen_attn)
+
+alpaca_zh = dict(
+ type=process_hf_dataset,
+ dataset=dict(type=load_dataset, path=alpaca_zh_path),
+ tokenizer=tokenizer,
+ max_length=max_length,
+ dataset_map_fn=alpaca_zh_map_fn,
+ template_map_fn=dict(
+ type=template_map_fn_factory, template=prompt_template),
+ remove_unused_columns=True,
+ shuffle_before_pack=True,
+ pack_to_max_length=pack_to_max_length,
+ use_varlen_attn=use_varlen_attn)
+
+train_dataset = dict(type=ConcatDataset, datasets=[alpaca_en, alpaca_zh])
+
+sampler = SequenceParallelSampler \
+ if sequence_parallel_size > 1 else DefaultSampler
+
+train_dataloader = dict(
+ batch_size=batch_size,
+ num_workers=dataloader_num_workers,
+ dataset=train_dataset,
+ sampler=dict(type=sampler, shuffle=True),
+ collate_fn=dict(type=default_collate_fn, use_varlen_attn=use_varlen_attn))
+
+#######################################################################
+# PART 4 Scheduler & Optimizer #
+#######################################################################
+# optimizer
+optim_wrapper = dict(
+ type=AmpOptimWrapper,
+ optimizer=dict(
+ type=optim_type, lr=lr, betas=betas, weight_decay=weight_decay),
+ clip_grad=dict(max_norm=max_norm, error_if_nonfinite=False),
+ accumulative_counts=accumulative_counts,
+ loss_scale='dynamic',
+ dtype='float16')
+
+# learning policy
+# More information: https://github.com/open-mmlab/mmengine/blob/main/docs/en/tutorials/param_scheduler.md # noqa: E501
+param_scheduler = [
+ dict(
+ type=LinearLR,
+ start_factor=1e-5,
+ by_epoch=True,
+ begin=0,
+ end=warmup_ratio * max_epochs,
+ convert_to_iter_based=True),
+ dict(
+ type=CosineAnnealingLR,
+ eta_min=0.0,
+ by_epoch=True,
+ begin=warmup_ratio * max_epochs,
+ end=max_epochs,
+ convert_to_iter_based=True)
+]
+
+# train, val, test setting
+train_cfg = dict(type=TrainLoop, max_epochs=max_epochs)
+
+#######################################################################
+# PART 5 Runtime #
+#######################################################################
+# Log the dialogue periodically during the training process, optional
+custom_hooks = [
+ dict(type=DatasetInfoHook, tokenizer=tokenizer),
+ dict(
+ type=EvaluateChatHook,
+ tokenizer=tokenizer,
+ every_n_iters=evaluation_freq,
+ evaluation_inputs=evaluation_inputs,
+ system=SYSTEM,
+ prompt_template=prompt_template)
+]
+
+if use_varlen_attn:
+ custom_hooks += [dict(type=VarlenAttnArgsToMessageHubHook)]
+
+# configure default hooks
+default_hooks = dict(
+ # record the time of every iteration.
+ timer=dict(type=IterTimerHook),
+ # print log every 10 iterations.
+ logger=dict(type=LoggerHook, log_metric_by_epoch=False, interval=10),
+ # enable the parameter scheduler.
+ param_scheduler=dict(type=ParamSchedulerHook),
+ # save checkpoint per `save_steps`.
+ checkpoint=dict(
+ type=CheckpointHook,
+ by_epoch=False,
+ interval=save_steps,
+ max_keep_ckpts=save_total_limit),
+ # set sampler seed in distributed evrionment.
+ sampler_seed=dict(type=DistSamplerSeedHook),
+)
+
+# configure environment
+env_cfg = dict(
+ # whether to enable cudnn benchmark
+ cudnn_benchmark=False,
+ # set multi process parameters
+ mp_cfg=dict(mp_start_method='fork', opencv_num_threads=0),
+ # set distributed parameters
+ dist_cfg=dict(backend='nccl'),
+)
+
+# set visualizer
+visualizer = None
+
+# set log level
+log_level = 'INFO'
+
+# load from which checkpoint
+load_from = None
+
+# whether to resume training from the loaded checkpoint
+resume = False
+
+# Defaults to use random seed and disable `deterministic`
+randomness = dict(seed=None, deterministic=False)
+
+# set log processor
+log_processor = dict(by_epoch=False)
diff --git a/xtuner/configs/minicpm/1_2b/minicpm_1b_qlora_alpaca_zh_e3.py b/xtuner/configs/minicpm/1_2b/minicpm_1b_qlora_alpaca_zh_e3.py
new file mode 100644
index 000000000..ca7816c0a
--- /dev/null
+++ b/xtuner/configs/minicpm/1_2b/minicpm_1b_qlora_alpaca_zh_e3.py
@@ -0,0 +1,221 @@
+# Copyright (c) OpenMMLab. All rights reserved.
+import torch
+from datasets import load_dataset
+from mmengine.dataset import DefaultSampler
+from mmengine.hooks import (CheckpointHook, DistSamplerSeedHook, IterTimerHook,
+ LoggerHook, ParamSchedulerHook)
+from mmengine.optim import AmpOptimWrapper, CosineAnnealingLR, LinearLR
+from peft import LoraConfig
+from torch.optim import AdamW
+from transformers import (AutoModelForCausalLM, AutoTokenizer,
+ BitsAndBytesConfig)
+
+from xtuner.dataset import process_hf_dataset
+from xtuner.dataset.collate_fns import default_collate_fn
+from xtuner.dataset.map_fns import alpaca_zh_map_fn, template_map_fn_factory
+from xtuner.engine.hooks import (DatasetInfoHook, EvaluateChatHook,
+ VarlenAttnArgsToMessageHubHook)
+from xtuner.engine.runner import TrainLoop
+from xtuner.model import SupervisedFinetune
+from xtuner.parallel.sequence import SequenceParallelSampler
+from xtuner.utils import PROMPT_TEMPLATE, SYSTEM_TEMPLATE
+
+#######################################################################
+# PART 1 Settings #
+#######################################################################
+# Model
+pretrained_model_name_or_path = 'openbmb/MiniCPM-1B-sft-bf16'
+use_varlen_attn = False
+
+# Data
+alpaca_zh_path = 'silk-road/alpaca-data-gpt4-chinese'
+prompt_template = PROMPT_TEMPLATE.minicpm
+max_length = 2048
+pack_to_max_length = True
+
+# parallel
+sequence_parallel_size = 1
+
+# Scheduler & Optimizer
+batch_size = 1 # per_device
+accumulative_counts = 16
+accumulative_counts *= sequence_parallel_size
+dataloader_num_workers = 0
+max_epochs = 3
+optim_type = AdamW
+lr = 2e-4
+betas = (0.9, 0.999)
+weight_decay = 0
+max_norm = 1 # grad clip
+warmup_ratio = 0.03
+gradient_checkpointing = True
+# Save
+save_steps = 500
+save_total_limit = 2 # Maximum checkpoints to keep (-1 means unlimited)
+
+# Evaluate the generation performance during the training
+evaluation_freq = 500
+SYSTEM = SYSTEM_TEMPLATE.alpaca
+evaluation_inputs = [
+ '请给我介绍五个上海的景点', 'Please tell me five scenic spots in Shanghai'
+]
+
+#######################################################################
+# PART 2 Model & Tokenizer #
+#######################################################################
+tokenizer = dict(
+ type=AutoTokenizer.from_pretrained,
+ pretrained_model_name_or_path=pretrained_model_name_or_path,
+ trust_remote_code=True,
+ padding_side='right',
+ eos_token='')
+
+model = dict(
+ type=SupervisedFinetune,
+ use_varlen_attn=use_varlen_attn,
+ llm=dict(
+ type=AutoModelForCausalLM.from_pretrained,
+ pretrained_model_name_or_path=pretrained_model_name_or_path,
+ trust_remote_code=True,
+ torch_dtype=torch.float16,
+ quantization_config=dict(
+ type=BitsAndBytesConfig,
+ load_in_4bit=True,
+ load_in_8bit=False,
+ llm_int8_threshold=6.0,
+ llm_int8_has_fp16_weight=False,
+ bnb_4bit_compute_dtype=torch.float16,
+ bnb_4bit_use_double_quant=True,
+ bnb_4bit_quant_type='nf4')),
+ lora=dict(
+ type=LoraConfig,
+ r=64,
+ lora_alpha=16,
+ lora_dropout=0.1,
+ bias='none',
+ task_type='CAUSAL_LM'))
+
+#######################################################################
+# PART 3 Dataset & Dataloader #
+#######################################################################
+alpaca_zh = dict(
+ type=process_hf_dataset,
+ dataset=dict(type=load_dataset, path=alpaca_zh_path),
+ tokenizer=tokenizer,
+ max_length=max_length,
+ dataset_map_fn=alpaca_zh_map_fn,
+ template_map_fn=dict(
+ type=template_map_fn_factory, template=prompt_template),
+ remove_unused_columns=True,
+ shuffle_before_pack=True,
+ pack_to_max_length=pack_to_max_length,
+ use_varlen_attn=use_varlen_attn)
+
+sampler = SequenceParallelSampler \
+ if sequence_parallel_size > 1 else DefaultSampler
+
+train_dataloader = dict(
+ batch_size=batch_size,
+ num_workers=dataloader_num_workers,
+ dataset=alpaca_zh,
+ sampler=dict(type=sampler, shuffle=True),
+ collate_fn=dict(type=default_collate_fn, use_varlen_attn=use_varlen_attn))
+
+#######################################################################
+# PART 4 Scheduler & Optimizer #
+#######################################################################
+# optimizer
+optim_wrapper = dict(
+ type=AmpOptimWrapper,
+ optimizer=dict(
+ type=optim_type, lr=lr, betas=betas, weight_decay=weight_decay),
+ clip_grad=dict(max_norm=max_norm, error_if_nonfinite=False),
+ accumulative_counts=accumulative_counts,
+ loss_scale='dynamic',
+ dtype='float16')
+
+# learning policy
+# More information: https://github.com/open-mmlab/mmengine/blob/main/docs/en/tutorials/param_scheduler.md # noqa: E501
+param_scheduler = [
+ dict(
+ type=LinearLR,
+ start_factor=1e-5,
+ by_epoch=True,
+ begin=0,
+ end=warmup_ratio * max_epochs,
+ convert_to_iter_based=True),
+ dict(
+ type=CosineAnnealingLR,
+ eta_min=0.0,
+ by_epoch=True,
+ begin=warmup_ratio * max_epochs,
+ end=max_epochs,
+ convert_to_iter_based=True)
+]
+
+# train, val, test setting
+train_cfg = dict(type=TrainLoop, max_epochs=max_epochs)
+
+#######################################################################
+# PART 5 Runtime #
+#######################################################################
+# Log the dialogue periodically during the training process, optional
+custom_hooks = [
+ dict(type=DatasetInfoHook, tokenizer=tokenizer),
+ dict(
+ type=EvaluateChatHook,
+ tokenizer=tokenizer,
+ every_n_iters=evaluation_freq,
+ evaluation_inputs=evaluation_inputs,
+ system=SYSTEM,
+ prompt_template=prompt_template)
+]
+
+if use_varlen_attn:
+ custom_hooks += [dict(type=VarlenAttnArgsToMessageHubHook)]
+
+# configure default hooks
+default_hooks = dict(
+ # record the time of every iteration.
+ timer=dict(type=IterTimerHook),
+ # print log every 10 iterations.
+ logger=dict(type=LoggerHook, log_metric_by_epoch=False, interval=10),
+ # enable the parameter scheduler.
+ param_scheduler=dict(type=ParamSchedulerHook),
+ # save checkpoint per `save_steps`.
+ checkpoint=dict(
+ type=CheckpointHook,
+ by_epoch=False,
+ interval=save_steps,
+ max_keep_ckpts=save_total_limit),
+ # set sampler seed in distributed evrionment.
+ sampler_seed=dict(type=DistSamplerSeedHook),
+)
+
+# configure environment
+env_cfg = dict(
+ # whether to enable cudnn benchmark
+ cudnn_benchmark=False,
+ # set multi process parameters
+ mp_cfg=dict(mp_start_method='fork', opencv_num_threads=0),
+ # set distributed parameters
+ dist_cfg=dict(backend='nccl'),
+)
+
+# set visualizer
+visualizer = None
+
+# set log level
+log_level = 'INFO'
+
+# load from which checkpoint
+load_from = None
+
+# whether to resume training from the loaded checkpoint
+resume = False
+
+# Defaults to use random seed and disable `deterministic`
+randomness = dict(seed=None, deterministic=False)
+
+# set log processor
+log_processor = dict(by_epoch=False)
diff --git a/xtuner/configs/minicpm/2b/minicpm_2b_dpo_qlora.py b/xtuner/configs/minicpm/2b/minicpm_2b_dpo_qlora.py
new file mode 100644
index 000000000..abf1e7ef9
--- /dev/null
+++ b/xtuner/configs/minicpm/2b/minicpm_2b_dpo_qlora.py
@@ -0,0 +1,221 @@
+# Copyright (c) OpenMMLab. All rights reserved.
+import torch
+from datasets import load_dataset
+from mmengine.dataset import DefaultSampler
+from mmengine.hooks import (CheckpointHook, DistSamplerSeedHook, IterTimerHook,
+ LoggerHook, ParamSchedulerHook)
+from mmengine.optim import AmpOptimWrapper, CosineAnnealingLR, LinearLR
+from peft import LoraConfig
+from torch.optim import AdamW
+from transformers import (AutoModelForCausalLM, AutoTokenizer,
+ BitsAndBytesConfig)
+
+from xtuner.dataset.collate_fns.preference_collate_fn import \
+ preference_collate_fn
+from xtuner.dataset.preference_dataset import (build_preference_dataset,
+ orpo_dpo_mix_40k_map_fn)
+from xtuner.engine.hooks import (DatasetInfoHook, EvaluateChatHook,
+ VarlenAttnArgsToMessageHubHook)
+from xtuner.engine.runner import TrainLoop
+from xtuner.model.dpo import DPO
+from xtuner.utils import PROMPT_TEMPLATE, SYSTEM_TEMPLATE
+
+#######################################################################
+# PART 1 Settings #
+#######################################################################
+# Model
+pretrained_model_name_or_path = 'openbmb/MiniCPM-2B-sft-bf16'
+use_varlen_attn = False
+dpo_loss_type = 'sigmoid' # One of ['sigmoid', 'hinge', 'ipo', 'kto_pair', 'sppo_hard', 'nca_pair', 'robust'] # noqa: E501
+loss_beta = 0.1
+label_smoothing = 0.0
+
+# Data
+prompt_template = PROMPT_TEMPLATE.minicpm
+max_length = 2048
+
+# Scheduler & Optimizer
+batch_size = 1 # per_device
+accumulative_counts = 16
+dataloader_num_workers = 0
+max_epochs = 3
+optim_type = AdamW
+lr = 5e-7 # refer to alignment handbook
+betas = (0.9, 0.999)
+weight_decay = 0
+max_norm = 1 # grad clip
+warmup_ratio = 0.03
+
+# Save
+save_steps = 500
+save_total_limit = 2 # Maximum checkpoints to keep (-1 means unlimited)
+
+# Evaluate the generation performance during the training
+evaluation_freq = 500
+SYSTEM = SYSTEM_TEMPLATE.alpaca
+evaluation_inputs = [
+ 'What famous British author, known for his tales of mystery and the macabre, shares his initials with a common abbreviation for "rest in peace"?', # noqa: E501
+ 'Please tell me five scenic spots in Shanghai',
+ '890729 - 425663? Only respond with math and no words.'
+]
+
+#######################################################################
+# PART 2 Model & Tokenizer #
+#######################################################################
+tokenizer = dict(
+ type=AutoTokenizer.from_pretrained,
+ pretrained_model_name_or_path=pretrained_model_name_or_path,
+ trust_remote_code=True,
+ padding_side='right')
+
+model = dict(
+ type=DPO,
+ use_varlen_attn=use_varlen_attn,
+ loss_type=dpo_loss_type,
+ beta=loss_beta,
+ label_smoothing=label_smoothing,
+ llm=dict(
+ type=AutoModelForCausalLM.from_pretrained,
+ pretrained_model_name_or_path=pretrained_model_name_or_path,
+ trust_remote_code=True,
+ torch_dtype=torch.float16,
+ quantization_config=dict(
+ type=BitsAndBytesConfig,
+ load_in_4bit=True,
+ load_in_8bit=False,
+ llm_int8_threshold=6.0,
+ llm_int8_has_fp16_weight=False,
+ bnb_4bit_compute_dtype=torch.float16,
+ bnb_4bit_use_double_quant=True,
+ bnb_4bit_quant_type='nf4')),
+ lora=dict(
+ type=LoraConfig,
+ r=64,
+ lora_alpha=16,
+ lora_dropout=0.1,
+ bias='none',
+ task_type='CAUSAL_LM'))
+
+#######################################################################
+# PART 3 Dataset & Dataloader #
+#######################################################################
+train_dataset = dict(
+ type=build_preference_dataset,
+ dataset=dict(type=load_dataset, path='mlabonne/orpo-dpo-mix-40k'),
+ tokenizer=tokenizer,
+ max_length=max_length,
+ dataset_map_fn=orpo_dpo_mix_40k_map_fn,
+ is_dpo=True,
+ is_reward=False,
+ reward_token_id=-1,
+ num_proc=32,
+ use_varlen_attn=use_varlen_attn,
+ shuffle_before_pack=True,
+)
+
+train_dataloader = dict(
+ batch_size=batch_size,
+ num_workers=dataloader_num_workers,
+ dataset=train_dataset,
+ sampler=dict(type=DefaultSampler, shuffle=True),
+ collate_fn=dict(
+ type=preference_collate_fn, use_varlen_attn=use_varlen_attn))
+
+#######################################################################
+# PART 4 Scheduler & Optimizer #
+#######################################################################
+# optimizer
+optim_wrapper = dict(
+ type=AmpOptimWrapper,
+ optimizer=dict(
+ type=optim_type, lr=lr, betas=betas, weight_decay=weight_decay),
+ clip_grad=dict(max_norm=max_norm, error_if_nonfinite=False),
+ accumulative_counts=accumulative_counts,
+ loss_scale='dynamic',
+ dtype='float16')
+
+# learning policy
+# More information: https://github.com/open-mmlab/mmengine/blob/main/docs/en/tutorials/param_scheduler.md # noqa: E501
+param_scheduler = [
+ dict(
+ type=LinearLR,
+ start_factor=1e-5,
+ by_epoch=True,
+ begin=0,
+ end=warmup_ratio * max_epochs,
+ convert_to_iter_based=True),
+ dict(
+ type=CosineAnnealingLR,
+ eta_min=0.0,
+ by_epoch=True,
+ begin=warmup_ratio * max_epochs,
+ end=max_epochs,
+ convert_to_iter_based=True)
+]
+
+# train, val, test setting
+train_cfg = dict(type=TrainLoop, max_epochs=max_epochs)
+
+#######################################################################
+# PART 5 Runtime #
+#######################################################################
+# Log the dialogue periodically during the training process, optional
+custom_hooks = [
+ dict(type=DatasetInfoHook, tokenizer=tokenizer),
+ dict(
+ type=EvaluateChatHook,
+ tokenizer=tokenizer,
+ every_n_iters=evaluation_freq,
+ evaluation_inputs=evaluation_inputs,
+ system=SYSTEM,
+ prompt_template=prompt_template)
+]
+
+if use_varlen_attn:
+ custom_hooks += [dict(type=VarlenAttnArgsToMessageHubHook)]
+
+# configure default hooks
+default_hooks = dict(
+ # record the time of every iteration.
+ timer=dict(type=IterTimerHook),
+ # print log every 10 iterations.
+ logger=dict(type=LoggerHook, log_metric_by_epoch=False, interval=10),
+ # enable the parameter scheduler.
+ param_scheduler=dict(type=ParamSchedulerHook),
+ # save checkpoint per `save_steps`.
+ checkpoint=dict(
+ type=CheckpointHook,
+ by_epoch=False,
+ interval=save_steps,
+ max_keep_ckpts=save_total_limit),
+ # set sampler seed in distributed evrionment.
+ sampler_seed=dict(type=DistSamplerSeedHook),
+)
+
+# configure environment
+env_cfg = dict(
+ # whether to enable cudnn benchmark
+ cudnn_benchmark=False,
+ # set multi process parameters
+ mp_cfg=dict(mp_start_method='fork', opencv_num_threads=0),
+ # set distributed parameters
+ dist_cfg=dict(backend='nccl'),
+)
+
+# set visualizer
+visualizer = None
+
+# set log level
+log_level = 'INFO'
+
+# load from which checkpoint
+load_from = None
+
+# whether to resume training from the loaded checkpoint
+resume = False
+
+# Defaults to use random seed and disable `deterministic`
+randomness = dict(seed=None, deterministic=False)
+
+# set log processor
+log_processor = dict(by_epoch=False)
diff --git a/xtuner/configs/minicpm/2b/minicpm_2b_full_alpaca_zh_e3.py b/xtuner/configs/minicpm/2b/minicpm_2b_full_alpaca_zh_e3.py
new file mode 100644
index 000000000..c699ff876
--- /dev/null
+++ b/xtuner/configs/minicpm/2b/minicpm_2b_full_alpaca_zh_e3.py
@@ -0,0 +1,201 @@
+# Copyright (c) OpenMMLab. All rights reserved.
+from datasets import load_dataset
+from mmengine.dataset import DefaultSampler
+from mmengine.hooks import (CheckpointHook, DistSamplerSeedHook, IterTimerHook,
+ LoggerHook, ParamSchedulerHook)
+from mmengine.optim import AmpOptimWrapper, CosineAnnealingLR, LinearLR
+from torch.optim import AdamW
+from transformers import AutoModelForCausalLM, AutoTokenizer
+
+from xtuner.dataset import process_hf_dataset
+from xtuner.dataset.collate_fns import default_collate_fn
+from xtuner.dataset.map_fns import alpaca_zh_map_fn, template_map_fn_factory
+from xtuner.engine.hooks import (DatasetInfoHook, EvaluateChatHook,
+ VarlenAttnArgsToMessageHubHook)
+from xtuner.engine.runner import TrainLoop
+from xtuner.model import SupervisedFinetune
+from xtuner.parallel.sequence import SequenceParallelSampler
+from xtuner.utils import PROMPT_TEMPLATE, SYSTEM_TEMPLATE
+
+#######################################################################
+# PART 1 Settings #
+#######################################################################
+# Model
+pretrained_model_name_or_path = 'openbmb/MiniCPM-2B-sft-bf16'
+use_varlen_attn = False
+
+# Data
+alpaca_en_path = 'silk-road/alpaca-data-gpt4-chinese'
+prompt_template = PROMPT_TEMPLATE.minicpm
+max_length = 2048
+pack_to_max_length = True
+
+# parallel
+sequence_parallel_size = 1
+
+# Scheduler & Optimizer
+batch_size = 1 # per_device
+accumulative_counts = 16
+accumulative_counts *= sequence_parallel_size
+dataloader_num_workers = 0
+max_epochs = 3
+optim_type = AdamW
+lr = 2e-5
+betas = (0.9, 0.999)
+weight_decay = 0
+max_norm = 1 # grad clip
+warmup_ratio = 0.03
+
+# Save
+save_steps = 500
+save_total_limit = 2 # Maximum checkpoints to keep (-1 means unlimited)
+
+# Evaluate the generation performance during the training
+evaluation_freq = 500
+SYSTEM = SYSTEM_TEMPLATE.alpaca
+evaluation_inputs = [
+ '请给我介绍五个上海的景点', 'Please tell me five scenic spots in Shanghai'
+]
+
+#######################################################################
+# PART 2 Model & Tokenizer #
+#######################################################################
+tokenizer = dict(
+ type=AutoTokenizer.from_pretrained,
+ pretrained_model_name_or_path=pretrained_model_name_or_path,
+ trust_remote_code=True,
+ padding_side='right',
+ eos_token='')
+
+model = dict(
+ type=SupervisedFinetune,
+ use_varlen_attn=use_varlen_attn,
+ llm=dict(
+ type=AutoModelForCausalLM.from_pretrained,
+ pretrained_model_name_or_path=pretrained_model_name_or_path,
+ trust_remote_code=True))
+
+#######################################################################
+# PART 3 Dataset & Dataloader #
+#######################################################################
+alpaca_en = dict(
+ type=process_hf_dataset,
+ dataset=dict(type=load_dataset, path=alpaca_en_path),
+ tokenizer=tokenizer,
+ max_length=max_length,
+ dataset_map_fn=alpaca_zh_map_fn,
+ template_map_fn=dict(
+ type=template_map_fn_factory, template=prompt_template),
+ remove_unused_columns=True,
+ shuffle_before_pack=True,
+ pack_to_max_length=pack_to_max_length,
+ use_varlen_attn=use_varlen_attn)
+
+sampler = SequenceParallelSampler \
+ if sequence_parallel_size > 1 else DefaultSampler
+
+train_dataloader = dict(
+ batch_size=batch_size,
+ num_workers=dataloader_num_workers,
+ dataset=alpaca_en,
+ sampler=dict(type=sampler, shuffle=True),
+ collate_fn=dict(type=default_collate_fn, use_varlen_attn=use_varlen_attn))
+
+#######################################################################
+# PART 4 Scheduler & Optimizer #
+#######################################################################
+# optimizer
+optim_wrapper = dict(
+ type=AmpOptimWrapper,
+ optimizer=dict(
+ type=optim_type, lr=lr, betas=betas, weight_decay=weight_decay),
+ clip_grad=dict(max_norm=max_norm, error_if_nonfinite=False),
+ accumulative_counts=accumulative_counts,
+ loss_scale='dynamic',
+ dtype='float16')
+
+# learning policy
+# More information: https://github.com/open-mmlab/mmengine/blob/main/docs/en/tutorials/param_scheduler.md # noqa: E501
+param_scheduler = [
+ dict(
+ type=LinearLR,
+ start_factor=1e-5,
+ by_epoch=True,
+ begin=0,
+ end=warmup_ratio * max_epochs,
+ convert_to_iter_based=True),
+ dict(
+ type=CosineAnnealingLR,
+ eta_min=0.0,
+ by_epoch=True,
+ begin=warmup_ratio * max_epochs,
+ end=max_epochs,
+ convert_to_iter_based=True)
+]
+
+# train, val, test setting
+train_cfg = dict(type=TrainLoop, max_epochs=max_epochs)
+
+#######################################################################
+# PART 5 Runtime #
+#######################################################################
+# Log the dialogue periodically during the training process, optional
+custom_hooks = [
+ dict(type=DatasetInfoHook, tokenizer=tokenizer),
+ dict(
+ type=EvaluateChatHook,
+ tokenizer=tokenizer,
+ every_n_iters=evaluation_freq,
+ evaluation_inputs=evaluation_inputs,
+ system=SYSTEM,
+ prompt_template=prompt_template)
+]
+
+if use_varlen_attn:
+ custom_hooks += [dict(type=VarlenAttnArgsToMessageHubHook)]
+
+# configure default hooks
+default_hooks = dict(
+ # record the time of every iteration.
+ timer=dict(type=IterTimerHook),
+ # print log every 10 iterations.
+ logger=dict(type=LoggerHook, log_metric_by_epoch=False, interval=10),
+ # enable the parameter scheduler.
+ param_scheduler=dict(type=ParamSchedulerHook),
+ # save checkpoint per `save_steps`.
+ checkpoint=dict(
+ type=CheckpointHook,
+ by_epoch=False,
+ interval=save_steps,
+ max_keep_ckpts=save_total_limit),
+ # set sampler seed in distributed evrionment.
+ sampler_seed=dict(type=DistSamplerSeedHook),
+)
+
+# configure environment
+env_cfg = dict(
+ # whether to enable cudnn benchmark
+ cudnn_benchmark=False,
+ # set multi process parameters
+ mp_cfg=dict(mp_start_method='fork', opencv_num_threads=0),
+ # set distributed parameters
+ dist_cfg=dict(backend='nccl'),
+)
+
+# set visualizer
+visualizer = None
+
+# set log level
+log_level = 'INFO'
+
+# load from which checkpoint
+load_from = None
+
+# whether to resume training from the loaded checkpoint
+resume = False
+
+# Defaults to use random seed and disable `deterministic`
+randomness = dict(seed=None, deterministic=False)
+
+# set log processor
+log_processor = dict(by_epoch=False)
diff --git a/xtuner/configs/minicpm/2b/minicpm_2b_lora_alpaca_zh_e3.py b/xtuner/configs/minicpm/2b/minicpm_2b_lora_alpaca_zh_e3.py
new file mode 100644
index 000000000..a50fe91ab
--- /dev/null
+++ b/xtuner/configs/minicpm/2b/minicpm_2b_lora_alpaca_zh_e3.py
@@ -0,0 +1,212 @@
+# Copyright (c) OpenMMLab. All rights reserved.
+import torch
+from datasets import load_dataset
+from mmengine.dataset import DefaultSampler
+from mmengine.hooks import (CheckpointHook, DistSamplerSeedHook, IterTimerHook,
+ LoggerHook, ParamSchedulerHook)
+from mmengine.optim import AmpOptimWrapper, CosineAnnealingLR, LinearLR
+from peft import LoraConfig
+from torch.optim import AdamW
+from transformers import AutoModelForCausalLM, AutoTokenizer
+
+from xtuner.dataset import process_hf_dataset
+from xtuner.dataset.collate_fns import default_collate_fn
+from xtuner.dataset.map_fns import alpaca_zh_map_fn, template_map_fn_factory
+from xtuner.engine.hooks import (DatasetInfoHook, EvaluateChatHook,
+ VarlenAttnArgsToMessageHubHook)
+from xtuner.engine.runner import TrainLoop
+from xtuner.model import SupervisedFinetune
+from xtuner.parallel.sequence import SequenceParallelSampler
+from xtuner.utils import PROMPT_TEMPLATE, SYSTEM_TEMPLATE
+
+#######################################################################
+# PART 1 Settings #
+#######################################################################
+# Model
+pretrained_model_name_or_path = 'openbmb/MiniCPM-2B-sft-bf16'
+use_varlen_attn = False
+
+# Data
+alpaca_zh_path = 'silk-road/alpaca-data-gpt4-chinese'
+prompt_template = PROMPT_TEMPLATE.minicpm
+max_length = 2048
+pack_to_max_length = True
+
+# parallel
+sequence_parallel_size = 1
+
+# Scheduler & Optimizer
+batch_size = 1 # per_device
+accumulative_counts = 16
+accumulative_counts *= sequence_parallel_size
+dataloader_num_workers = 0
+max_epochs = 3
+optim_type = AdamW
+lr = 2e-4
+betas = (0.9, 0.999)
+weight_decay = 0
+max_norm = 1 # grad clip
+warmup_ratio = 0.03
+gradient_checkpointing = True
+# Save
+save_steps = 500
+save_total_limit = 2 # Maximum checkpoints to keep (-1 means unlimited)
+
+# Evaluate the generation performance during the training
+evaluation_freq = 500
+SYSTEM = SYSTEM_TEMPLATE.alpaca
+evaluation_inputs = [
+ '请给我介绍五个上海的景点', 'Please tell me five scenic spots in Shanghai'
+]
+
+#######################################################################
+# PART 2 Model & Tokenizer #
+#######################################################################
+tokenizer = dict(
+ type=AutoTokenizer.from_pretrained,
+ pretrained_model_name_or_path=pretrained_model_name_or_path,
+ trust_remote_code=True,
+ padding_side='right',
+ eos_token='')
+
+model = dict(
+ type=SupervisedFinetune,
+ use_varlen_attn=use_varlen_attn,
+ llm=dict(
+ type=AutoModelForCausalLM.from_pretrained,
+ pretrained_model_name_or_path=pretrained_model_name_or_path,
+ trust_remote_code=True,
+ torch_dtype=torch.float16,
+ ),
+ lora=dict(
+ type=LoraConfig,
+ r=64,
+ lora_alpha=16,
+ lora_dropout=0.1,
+ bias='none',
+ task_type='CAUSAL_LM'))
+
+#######################################################################
+# PART 3 Dataset & Dataloader #
+#######################################################################
+alpaca_zh = dict(
+ type=process_hf_dataset,
+ dataset=dict(type=load_dataset, path=alpaca_zh_path),
+ tokenizer=tokenizer,
+ max_length=max_length,
+ dataset_map_fn=alpaca_zh_map_fn,
+ template_map_fn=dict(
+ type=template_map_fn_factory, template=prompt_template),
+ remove_unused_columns=True,
+ shuffle_before_pack=True,
+ pack_to_max_length=pack_to_max_length,
+ use_varlen_attn=use_varlen_attn)
+
+sampler = SequenceParallelSampler \
+ if sequence_parallel_size > 1 else DefaultSampler
+
+train_dataloader = dict(
+ batch_size=batch_size,
+ num_workers=dataloader_num_workers,
+ dataset=alpaca_zh,
+ sampler=dict(type=sampler, shuffle=True),
+ collate_fn=dict(type=default_collate_fn, use_varlen_attn=use_varlen_attn))
+
+#######################################################################
+# PART 4 Scheduler & Optimizer #
+#######################################################################
+# optimizer
+optim_wrapper = dict(
+ type=AmpOptimWrapper,
+ optimizer=dict(
+ type=optim_type, lr=lr, betas=betas, weight_decay=weight_decay),
+ clip_grad=dict(max_norm=max_norm, error_if_nonfinite=False),
+ accumulative_counts=accumulative_counts,
+ loss_scale='dynamic',
+ dtype='float16')
+
+# learning policy
+# More information: https://github.com/open-mmlab/mmengine/blob/main/docs/en/tutorials/param_scheduler.md # noqa: E501
+param_scheduler = [
+ dict(
+ type=LinearLR,
+ start_factor=1e-5,
+ by_epoch=True,
+ begin=0,
+ end=warmup_ratio * max_epochs,
+ convert_to_iter_based=True),
+ dict(
+ type=CosineAnnealingLR,
+ eta_min=0.0,
+ by_epoch=True,
+ begin=warmup_ratio * max_epochs,
+ end=max_epochs,
+ convert_to_iter_based=True)
+]
+
+# train, val, test setting
+train_cfg = dict(type=TrainLoop, max_epochs=max_epochs)
+
+#######################################################################
+# PART 5 Runtime #
+#######################################################################
+# Log the dialogue periodically during the training process, optional
+custom_hooks = [
+ dict(type=DatasetInfoHook, tokenizer=tokenizer),
+ dict(
+ type=EvaluateChatHook,
+ tokenizer=tokenizer,
+ every_n_iters=evaluation_freq,
+ evaluation_inputs=evaluation_inputs,
+ system=SYSTEM,
+ prompt_template=prompt_template)
+]
+
+if use_varlen_attn:
+ custom_hooks += [dict(type=VarlenAttnArgsToMessageHubHook)]
+
+# configure default hooks
+default_hooks = dict(
+ # record the time of every iteration.
+ timer=dict(type=IterTimerHook),
+ # print log every 10 iterations.
+ logger=dict(type=LoggerHook, log_metric_by_epoch=False, interval=10),
+ # enable the parameter scheduler.
+ param_scheduler=dict(type=ParamSchedulerHook),
+ # save checkpoint per `save_steps`.
+ checkpoint=dict(
+ type=CheckpointHook,
+ by_epoch=False,
+ interval=save_steps,
+ max_keep_ckpts=save_total_limit),
+ # set sampler seed in distributed evrionment.
+ sampler_seed=dict(type=DistSamplerSeedHook),
+)
+
+# configure environment
+env_cfg = dict(
+ # whether to enable cudnn benchmark
+ cudnn_benchmark=False,
+ # set multi process parameters
+ mp_cfg=dict(mp_start_method='fork', opencv_num_threads=0),
+ # set distributed parameters
+ dist_cfg=dict(backend='nccl'),
+)
+
+# set visualizer
+visualizer = None
+
+# set log level
+log_level = 'INFO'
+
+# load from which checkpoint
+load_from = None
+
+# whether to resume training from the loaded checkpoint
+resume = False
+
+# Defaults to use random seed and disable `deterministic`
+randomness = dict(seed=None, deterministic=False)
+
+# set log processor
+log_processor = dict(by_epoch=False)
diff --git a/xtuner/configs/minicpm/2b/minicpm_2b_qlora_alpaca_enzh_e3.py b/xtuner/configs/minicpm/2b/minicpm_2b_qlora_alpaca_enzh_e3.py
new file mode 100644
index 000000000..2082e4c24
--- /dev/null
+++ b/xtuner/configs/minicpm/2b/minicpm_2b_qlora_alpaca_enzh_e3.py
@@ -0,0 +1,238 @@
+# Copyright (c) OpenMMLab. All rights reserved.
+import torch
+from datasets import load_dataset
+from mmengine.dataset import DefaultSampler
+from mmengine.hooks import (CheckpointHook, DistSamplerSeedHook, IterTimerHook,
+ LoggerHook, ParamSchedulerHook)
+from mmengine.optim import AmpOptimWrapper, CosineAnnealingLR, LinearLR
+from peft import LoraConfig
+from torch.optim import AdamW
+from transformers import (AutoModelForCausalLM, AutoTokenizer,
+ BitsAndBytesConfig)
+
+from xtuner.dataset import ConcatDataset, process_hf_dataset
+from xtuner.dataset.collate_fns import default_collate_fn
+from xtuner.dataset.map_fns import (alpaca_map_fn, alpaca_zh_map_fn,
+ template_map_fn_factory)
+from xtuner.engine.hooks import (DatasetInfoHook, EvaluateChatHook,
+ VarlenAttnArgsToMessageHubHook)
+from xtuner.engine.runner import TrainLoop
+from xtuner.model import SupervisedFinetune
+from xtuner.parallel.sequence import SequenceParallelSampler
+from xtuner.utils import PROMPT_TEMPLATE, SYSTEM_TEMPLATE
+
+#######################################################################
+# PART 1 Settings #
+#######################################################################
+# Model
+pretrained_model_name_or_path = 'openbmb/MiniCPM-2B-sft-bf16'
+use_varlen_attn = False
+
+# Data
+alpaca_zh_path = 'silk-road/alpaca-data-gpt4-chinese'
+alpaca_en_path = 'tatsu-lab/alpaca'
+prompt_template = PROMPT_TEMPLATE.minicpm
+max_length = 2048
+pack_to_max_length = True
+
+# parallel
+sequence_parallel_size = 1
+
+# Scheduler & Optimizer
+batch_size = 1 # per_device
+accumulative_counts = 16
+accumulative_counts *= sequence_parallel_size
+dataloader_num_workers = 0
+max_epochs = 3
+optim_type = AdamW
+lr = 2e-4
+betas = (0.9, 0.999)
+weight_decay = 0
+max_norm = 1 # grad clip
+warmup_ratio = 0.03
+
+# Save
+save_steps = 500
+save_total_limit = 2 # Maximum checkpoints to keep (-1 means unlimited)
+
+# Evaluate the generation performance during the training
+evaluation_freq = 500
+SYSTEM = SYSTEM_TEMPLATE.alpaca
+evaluation_inputs = [
+ '请给我介绍五个上海的景点', 'Please tell me five scenic spots in Shanghai'
+]
+
+#######################################################################
+# PART 2 Model & Tokenizer #
+#######################################################################
+tokenizer = dict(
+ type=AutoTokenizer.from_pretrained,
+ pretrained_model_name_or_path=pretrained_model_name_or_path,
+ trust_remote_code=True,
+ padding_side='right',
+ eos_token='')
+
+model = dict(
+ type=SupervisedFinetune,
+ use_varlen_attn=use_varlen_attn,
+ llm=dict(
+ type=AutoModelForCausalLM.from_pretrained,
+ pretrained_model_name_or_path=pretrained_model_name_or_path,
+ trust_remote_code=True,
+ torch_dtype=torch.float16,
+ quantization_config=dict(
+ type=BitsAndBytesConfig,
+ load_in_4bit=True,
+ load_in_8bit=False,
+ llm_int8_threshold=6.0,
+ llm_int8_has_fp16_weight=False,
+ bnb_4bit_compute_dtype=torch.float16,
+ bnb_4bit_use_double_quant=True,
+ bnb_4bit_quant_type='nf4')),
+ lora=dict(
+ type=LoraConfig,
+ r=64,
+ lora_alpha=16,
+ lora_dropout=0.1,
+ bias='none',
+ task_type='CAUSAL_LM'))
+
+#######################################################################
+# PART 3 Dataset & Dataloader #
+#######################################################################
+alpaca_en = dict(
+ type=process_hf_dataset,
+ dataset=dict(type=load_dataset, path=alpaca_en_path),
+ tokenizer=tokenizer,
+ max_length=max_length,
+ dataset_map_fn=alpaca_map_fn,
+ template_map_fn=dict(
+ type=template_map_fn_factory, template=prompt_template),
+ remove_unused_columns=True,
+ shuffle_before_pack=True,
+ pack_to_max_length=pack_to_max_length,
+ use_varlen_attn=use_varlen_attn)
+
+alpaca_zh = dict(
+ type=process_hf_dataset,
+ dataset=dict(type=load_dataset, path=alpaca_zh_path),
+ tokenizer=tokenizer,
+ max_length=max_length,
+ dataset_map_fn=alpaca_zh_map_fn,
+ template_map_fn=dict(
+ type=template_map_fn_factory, template=prompt_template),
+ remove_unused_columns=True,
+ shuffle_before_pack=True,
+ pack_to_max_length=pack_to_max_length,
+ use_varlen_attn=use_varlen_attn)
+
+train_dataset = dict(type=ConcatDataset, datasets=[alpaca_en, alpaca_zh])
+
+sampler = SequenceParallelSampler \
+ if sequence_parallel_size > 1 else DefaultSampler
+
+train_dataloader = dict(
+ batch_size=batch_size,
+ num_workers=dataloader_num_workers,
+ dataset=train_dataset,
+ sampler=dict(type=sampler, shuffle=True),
+ collate_fn=dict(type=default_collate_fn, use_varlen_attn=use_varlen_attn))
+
+#######################################################################
+# PART 4 Scheduler & Optimizer #
+#######################################################################
+# optimizer
+optim_wrapper = dict(
+ type=AmpOptimWrapper,
+ optimizer=dict(
+ type=optim_type, lr=lr, betas=betas, weight_decay=weight_decay),
+ clip_grad=dict(max_norm=max_norm, error_if_nonfinite=False),
+ accumulative_counts=accumulative_counts,
+ loss_scale='dynamic',
+ dtype='float16')
+
+# learning policy
+# More information: https://github.com/open-mmlab/mmengine/blob/main/docs/en/tutorials/param_scheduler.md # noqa: E501
+param_scheduler = [
+ dict(
+ type=LinearLR,
+ start_factor=1e-5,
+ by_epoch=True,
+ begin=0,
+ end=warmup_ratio * max_epochs,
+ convert_to_iter_based=True),
+ dict(
+ type=CosineAnnealingLR,
+ eta_min=0.0,
+ by_epoch=True,
+ begin=warmup_ratio * max_epochs,
+ end=max_epochs,
+ convert_to_iter_based=True)
+]
+
+# train, val, test setting
+train_cfg = dict(type=TrainLoop, max_epochs=max_epochs)
+
+#######################################################################
+# PART 5 Runtime #
+#######################################################################
+# Log the dialogue periodically during the training process, optional
+custom_hooks = [
+ dict(type=DatasetInfoHook, tokenizer=tokenizer),
+ dict(
+ type=EvaluateChatHook,
+ tokenizer=tokenizer,
+ every_n_iters=evaluation_freq,
+ evaluation_inputs=evaluation_inputs,
+ system=SYSTEM,
+ prompt_template=prompt_template)
+]
+
+if use_varlen_attn:
+ custom_hooks += [dict(type=VarlenAttnArgsToMessageHubHook)]
+
+# configure default hooks
+default_hooks = dict(
+ # record the time of every iteration.
+ timer=dict(type=IterTimerHook),
+ # print log every 10 iterations.
+ logger=dict(type=LoggerHook, log_metric_by_epoch=False, interval=10),
+ # enable the parameter scheduler.
+ param_scheduler=dict(type=ParamSchedulerHook),
+ # save checkpoint per `save_steps`.
+ checkpoint=dict(
+ type=CheckpointHook,
+ by_epoch=False,
+ interval=save_steps,
+ max_keep_ckpts=save_total_limit),
+ # set sampler seed in distributed evrionment.
+ sampler_seed=dict(type=DistSamplerSeedHook),
+)
+
+# configure environment
+env_cfg = dict(
+ # whether to enable cudnn benchmark
+ cudnn_benchmark=False,
+ # set multi process parameters
+ mp_cfg=dict(mp_start_method='fork', opencv_num_threads=0),
+ # set distributed parameters
+ dist_cfg=dict(backend='nccl'),
+)
+
+# set visualizer
+visualizer = None
+
+# set log level
+log_level = 'INFO'
+
+# load from which checkpoint
+load_from = None
+
+# whether to resume training from the loaded checkpoint
+resume = False
+
+# Defaults to use random seed and disable `deterministic`
+randomness = dict(seed=None, deterministic=False)
+
+# set log processor
+log_processor = dict(by_epoch=False)
diff --git a/xtuner/configs/minicpm/2b/minicpm_2b_qlora_alpaca_zh_e3.py b/xtuner/configs/minicpm/2b/minicpm_2b_qlora_alpaca_zh_e3.py
new file mode 100644
index 000000000..86d3564da
--- /dev/null
+++ b/xtuner/configs/minicpm/2b/minicpm_2b_qlora_alpaca_zh_e3.py
@@ -0,0 +1,221 @@
+# Copyright (c) OpenMMLab. All rights reserved.
+import torch
+from datasets import load_dataset
+from mmengine.dataset import DefaultSampler
+from mmengine.hooks import (CheckpointHook, DistSamplerSeedHook, IterTimerHook,
+ LoggerHook, ParamSchedulerHook)
+from mmengine.optim import AmpOptimWrapper, CosineAnnealingLR, LinearLR
+from peft import LoraConfig
+from torch.optim import AdamW
+from transformers import (AutoModelForCausalLM, AutoTokenizer,
+ BitsAndBytesConfig)
+
+from xtuner.dataset import process_hf_dataset
+from xtuner.dataset.collate_fns import default_collate_fn
+from xtuner.dataset.map_fns import alpaca_zh_map_fn, template_map_fn_factory
+from xtuner.engine.hooks import (DatasetInfoHook, EvaluateChatHook,
+ VarlenAttnArgsToMessageHubHook)
+from xtuner.engine.runner import TrainLoop
+from xtuner.model import SupervisedFinetune
+from xtuner.parallel.sequence import SequenceParallelSampler
+from xtuner.utils import PROMPT_TEMPLATE, SYSTEM_TEMPLATE
+
+#######################################################################
+# PART 1 Settings #
+#######################################################################
+# Model
+pretrained_model_name_or_path = 'openbmb/MiniCPM-2B-sft-bf16'
+use_varlen_attn = False
+
+# Data
+alpaca_zh_path = 'silk-road/alpaca-data-gpt4-chinese'
+prompt_template = PROMPT_TEMPLATE.minicpm
+max_length = 2048
+pack_to_max_length = True
+
+# parallel
+sequence_parallel_size = 1
+
+# Scheduler & Optimizer
+batch_size = 1 # per_device
+accumulative_counts = 16
+accumulative_counts *= sequence_parallel_size
+dataloader_num_workers = 0
+max_epochs = 3
+optim_type = AdamW
+lr = 2e-4
+betas = (0.9, 0.999)
+weight_decay = 0
+max_norm = 1 # grad clip
+warmup_ratio = 0.03
+gradient_checkpointing = True
+# Save
+save_steps = 500
+save_total_limit = 2 # Maximum checkpoints to keep (-1 means unlimited)
+
+# Evaluate the generation performance during the training
+evaluation_freq = 500
+SYSTEM = SYSTEM_TEMPLATE.alpaca
+evaluation_inputs = [
+ '请给我介绍五个上海的景点', 'Please tell me five scenic spots in Shanghai'
+]
+
+#######################################################################
+# PART 2 Model & Tokenizer #
+#######################################################################
+tokenizer = dict(
+ type=AutoTokenizer.from_pretrained,
+ pretrained_model_name_or_path=pretrained_model_name_or_path,
+ trust_remote_code=True,
+ padding_side='right',
+ eos_token='')
+
+model = dict(
+ type=SupervisedFinetune,
+ use_varlen_attn=use_varlen_attn,
+ llm=dict(
+ type=AutoModelForCausalLM.from_pretrained,
+ pretrained_model_name_or_path=pretrained_model_name_or_path,
+ trust_remote_code=True,
+ torch_dtype=torch.float16,
+ quantization_config=dict(
+ type=BitsAndBytesConfig,
+ load_in_4bit=True,
+ load_in_8bit=False,
+ llm_int8_threshold=6.0,
+ llm_int8_has_fp16_weight=False,
+ bnb_4bit_compute_dtype=torch.float16,
+ bnb_4bit_use_double_quant=True,
+ bnb_4bit_quant_type='nf4')),
+ lora=dict(
+ type=LoraConfig,
+ r=64,
+ lora_alpha=16,
+ lora_dropout=0.1,
+ bias='none',
+ task_type='CAUSAL_LM'))
+
+#######################################################################
+# PART 3 Dataset & Dataloader #
+#######################################################################
+alpaca_zh = dict(
+ type=process_hf_dataset,
+ dataset=dict(type=load_dataset, path=alpaca_zh_path),
+ tokenizer=tokenizer,
+ max_length=max_length,
+ dataset_map_fn=alpaca_zh_map_fn,
+ template_map_fn=dict(
+ type=template_map_fn_factory, template=prompt_template),
+ remove_unused_columns=True,
+ shuffle_before_pack=True,
+ pack_to_max_length=pack_to_max_length,
+ use_varlen_attn=use_varlen_attn)
+
+sampler = SequenceParallelSampler \
+ if sequence_parallel_size > 1 else DefaultSampler
+
+train_dataloader = dict(
+ batch_size=batch_size,
+ num_workers=dataloader_num_workers,
+ dataset=alpaca_zh,
+ sampler=dict(type=sampler, shuffle=True),
+ collate_fn=dict(type=default_collate_fn, use_varlen_attn=use_varlen_attn))
+
+#######################################################################
+# PART 4 Scheduler & Optimizer #
+#######################################################################
+# optimizer
+optim_wrapper = dict(
+ type=AmpOptimWrapper,
+ optimizer=dict(
+ type=optim_type, lr=lr, betas=betas, weight_decay=weight_decay),
+ clip_grad=dict(max_norm=max_norm, error_if_nonfinite=False),
+ accumulative_counts=accumulative_counts,
+ loss_scale='dynamic',
+ dtype='float16')
+
+# learning policy
+# More information: https://github.com/open-mmlab/mmengine/blob/main/docs/en/tutorials/param_scheduler.md # noqa: E501
+param_scheduler = [
+ dict(
+ type=LinearLR,
+ start_factor=1e-5,
+ by_epoch=True,
+ begin=0,
+ end=warmup_ratio * max_epochs,
+ convert_to_iter_based=True),
+ dict(
+ type=CosineAnnealingLR,
+ eta_min=0.0,
+ by_epoch=True,
+ begin=warmup_ratio * max_epochs,
+ end=max_epochs,
+ convert_to_iter_based=True)
+]
+
+# train, val, test setting
+train_cfg = dict(type=TrainLoop, max_epochs=max_epochs)
+
+#######################################################################
+# PART 5 Runtime #
+#######################################################################
+# Log the dialogue periodically during the training process, optional
+custom_hooks = [
+ dict(type=DatasetInfoHook, tokenizer=tokenizer),
+ dict(
+ type=EvaluateChatHook,
+ tokenizer=tokenizer,
+ every_n_iters=evaluation_freq,
+ evaluation_inputs=evaluation_inputs,
+ system=SYSTEM,
+ prompt_template=prompt_template)
+]
+
+if use_varlen_attn:
+ custom_hooks += [dict(type=VarlenAttnArgsToMessageHubHook)]
+
+# configure default hooks
+default_hooks = dict(
+ # record the time of every iteration.
+ timer=dict(type=IterTimerHook),
+ # print log every 10 iterations.
+ logger=dict(type=LoggerHook, log_metric_by_epoch=False, interval=10),
+ # enable the parameter scheduler.
+ param_scheduler=dict(type=ParamSchedulerHook),
+ # save checkpoint per `save_steps`.
+ checkpoint=dict(
+ type=CheckpointHook,
+ by_epoch=False,
+ interval=save_steps,
+ max_keep_ckpts=save_total_limit),
+ # set sampler seed in distributed evrionment.
+ sampler_seed=dict(type=DistSamplerSeedHook),
+)
+
+# configure environment
+env_cfg = dict(
+ # whether to enable cudnn benchmark
+ cudnn_benchmark=False,
+ # set multi process parameters
+ mp_cfg=dict(mp_start_method='fork', opencv_num_threads=0),
+ # set distributed parameters
+ dist_cfg=dict(backend='nccl'),
+)
+
+# set visualizer
+visualizer = None
+
+# set log level
+log_level = 'INFO'
+
+# load from which checkpoint
+load_from = None
+
+# whether to resume training from the loaded checkpoint
+resume = False
+
+# Defaults to use random seed and disable `deterministic`
+randomness = dict(seed=None, deterministic=False)
+
+# set log processor
+log_processor = dict(by_epoch=False)
diff --git a/xtuner/configs/minicpm/minicpm3_4b/minicpm3_4b_dpo_qlora.py b/xtuner/configs/minicpm/minicpm3_4b/minicpm3_4b_dpo_qlora.py
new file mode 100644
index 000000000..dcb3344db
--- /dev/null
+++ b/xtuner/configs/minicpm/minicpm3_4b/minicpm3_4b_dpo_qlora.py
@@ -0,0 +1,221 @@
+# Copyright (c) OpenMMLab. All rights reserved.
+import torch
+from datasets import load_dataset
+from mmengine.dataset import DefaultSampler
+from mmengine.hooks import (CheckpointHook, DistSamplerSeedHook, IterTimerHook,
+ LoggerHook, ParamSchedulerHook)
+from mmengine.optim import AmpOptimWrapper, CosineAnnealingLR, LinearLR
+from peft import LoraConfig
+from torch.optim import AdamW
+from transformers import (AutoModelForCausalLM, AutoTokenizer,
+ BitsAndBytesConfig)
+
+from xtuner.dataset.collate_fns.preference_collate_fn import \
+ preference_collate_fn
+from xtuner.dataset.preference_dataset import (build_preference_dataset,
+ orpo_dpo_mix_40k_map_fn)
+from xtuner.engine.hooks import (DatasetInfoHook, EvaluateChatHook,
+ VarlenAttnArgsToMessageHubHook)
+from xtuner.engine.runner import TrainLoop
+from xtuner.model.dpo import DPO
+from xtuner.utils import PROMPT_TEMPLATE, SYSTEM_TEMPLATE
+
+#######################################################################
+# PART 1 Settings #
+#######################################################################
+# Model
+pretrained_model_name_or_path = 'openbmb/MiniCPM3-4B'
+use_varlen_attn = False
+dpo_loss_type = 'sigmoid' # One of ['sigmoid', 'hinge', 'ipo', 'kto_pair', 'sppo_hard', 'nca_pair', 'robust'] # noqa: E501
+loss_beta = 0.1
+label_smoothing = 0.0
+
+# Data
+prompt_template = PROMPT_TEMPLATE.minicpm
+max_length = 2048
+
+# Scheduler & Optimizer
+batch_size = 1 # per_device
+accumulative_counts = 16
+dataloader_num_workers = 0
+max_steps = 3
+optim_type = AdamW
+lr = 5e-7 # refer to alignment handbook
+betas = (0.9, 0.999)
+weight_decay = 0
+max_norm = 1 # grad clip
+warmup_ratio = 0.03
+
+# Save
+save_steps = 500
+save_total_limit = 2 # Maximum checkpoints to keep (-1 means unlimited)
+
+# Evaluate the generation performance during the training
+evaluation_freq = 500
+SYSTEM = SYSTEM_TEMPLATE.alpaca
+evaluation_inputs = [
+ 'What famous British author, known for his tales of mystery and the macabre, shares his initials with a common abbreviation for "rest in peace"?', # noqa: E501
+ 'Please tell me five scenic spots in Shanghai',
+ '890729 - 425663? Only respond with math and no words.'
+]
+
+#######################################################################
+# PART 2 Model & Tokenizer #
+#######################################################################
+tokenizer = dict(
+ type=AutoTokenizer.from_pretrained,
+ pretrained_model_name_or_path=pretrained_model_name_or_path,
+ trust_remote_code=True,
+ padding_side='right')
+
+model = dict(
+ type=DPO,
+ use_varlen_attn=use_varlen_attn,
+ loss_type=dpo_loss_type,
+ beta=loss_beta,
+ label_smoothing=label_smoothing,
+ llm=dict(
+ type=AutoModelForCausalLM.from_pretrained,
+ pretrained_model_name_or_path=pretrained_model_name_or_path,
+ trust_remote_code=True,
+ torch_dtype=torch.float16,
+ quantization_config=dict(
+ type=BitsAndBytesConfig,
+ load_in_4bit=True,
+ load_in_8bit=False,
+ llm_int8_threshold=6.0,
+ llm_int8_has_fp16_weight=False,
+ bnb_4bit_compute_dtype=torch.float16,
+ bnb_4bit_use_double_quant=True,
+ bnb_4bit_quant_type='nf4')),
+ lora=dict(
+ type=LoraConfig,
+ r=64,
+ lora_alpha=16,
+ lora_dropout=0.1,
+ bias='none',
+ task_type='CAUSAL_LM'))
+
+#######################################################################
+# PART 3 Dataset & Dataloader #
+#######################################################################
+train_dataset = dict(
+ type=build_preference_dataset,
+ dataset=dict(type=load_dataset, path='mlabonne/orpo-dpo-mix-40k'),
+ tokenizer=tokenizer,
+ max_length=max_length,
+ dataset_map_fn=orpo_dpo_mix_40k_map_fn,
+ is_dpo=True,
+ is_reward=False,
+ reward_token_id=-1,
+ num_proc=32,
+ use_varlen_attn=use_varlen_attn,
+ shuffle_before_pack=True,
+)
+
+train_dataloader = dict(
+ batch_size=batch_size,
+ num_workers=dataloader_num_workers,
+ dataset=train_dataset,
+ sampler=dict(type=DefaultSampler, shuffle=True),
+ collate_fn=dict(
+ type=preference_collate_fn, use_varlen_attn=use_varlen_attn))
+
+#######################################################################
+# PART 4 Scheduler & Optimizer #
+#######################################################################
+# optimizer
+optim_wrapper = dict(
+ type=AmpOptimWrapper,
+ optimizer=dict(
+ type=optim_type, lr=lr, betas=betas, weight_decay=weight_decay),
+ clip_grad=dict(max_norm=max_norm, error_if_nonfinite=False),
+ accumulative_counts=accumulative_counts,
+ loss_scale='dynamic',
+ dtype='float16')
+
+# learning policy
+# More information: https://github.com/open-mmlab/mmengine/blob/main/docs/en/tutorials/param_scheduler.md # noqa: E501
+param_scheduler = [
+ dict(
+ type=LinearLR,
+ start_factor=1e-5,
+ by_epoch=True,
+ begin=0,
+ end=warmup_ratio * max_steps,
+ convert_to_iter_based=True),
+ dict(
+ type=CosineAnnealingLR,
+ eta_min=0.0,
+ by_epoch=True,
+ begin=warmup_ratio * max_steps,
+ end=max_steps,
+ convert_to_iter_based=True)
+]
+
+# train, val, test setting
+train_cfg = dict(type=TrainLoop, max_iters=max_steps)
+
+#######################################################################
+# PART 5 Runtime #
+#######################################################################
+# Log the dialogue periodically during the training process, optional
+custom_hooks = [
+ dict(type=DatasetInfoHook, tokenizer=tokenizer),
+ dict(
+ type=EvaluateChatHook,
+ tokenizer=tokenizer,
+ every_n_iters=evaluation_freq,
+ evaluation_inputs=evaluation_inputs,
+ system=SYSTEM,
+ prompt_template=prompt_template)
+]
+
+if use_varlen_attn:
+ custom_hooks += [dict(type=VarlenAttnArgsToMessageHubHook)]
+
+# configure default hooks
+default_hooks = dict(
+ # record the time of every iteration.
+ timer=dict(type=IterTimerHook),
+ # print log every 10 iterations.
+ logger=dict(type=LoggerHook, log_metric_by_epoch=False, interval=10),
+ # enable the parameter scheduler.
+ param_scheduler=dict(type=ParamSchedulerHook),
+ # save checkpoint per `save_steps`.
+ checkpoint=dict(
+ type=CheckpointHook,
+ by_epoch=False,
+ interval=save_steps,
+ max_keep_ckpts=save_total_limit),
+ # set sampler seed in distributed evrionment.
+ sampler_seed=dict(type=DistSamplerSeedHook),
+)
+
+# configure environment
+env_cfg = dict(
+ # whether to enable cudnn benchmark
+ cudnn_benchmark=False,
+ # set multi process parameters
+ mp_cfg=dict(mp_start_method='fork', opencv_num_threads=0),
+ # set distributed parameters
+ dist_cfg=dict(backend='nccl'),
+)
+
+# set visualizer
+visualizer = None
+
+# set log level
+log_level = 'INFO'
+
+# load from which checkpoint
+load_from = None
+
+# whether to resume training from the loaded checkpoint
+resume = False
+
+# Defaults to use random seed and disable `deterministic`
+randomness = dict(seed=None, deterministic=False)
+
+# set log processor
+log_processor = dict(by_epoch=False)
diff --git a/xtuner/configs/minicpm/minicpm3_4b/minicpm3_4b_full_alpaca_zh_e3.py b/xtuner/configs/minicpm/minicpm3_4b/minicpm3_4b_full_alpaca_zh_e3.py
new file mode 100644
index 000000000..1a9e249a6
--- /dev/null
+++ b/xtuner/configs/minicpm/minicpm3_4b/minicpm3_4b_full_alpaca_zh_e3.py
@@ -0,0 +1,201 @@
+# Copyright (c) OpenMMLab. All rights reserved.
+from datasets import load_dataset
+from mmengine.dataset import DefaultSampler
+from mmengine.hooks import (CheckpointHook, DistSamplerSeedHook, IterTimerHook,
+ LoggerHook, ParamSchedulerHook)
+from mmengine.optim import AmpOptimWrapper, CosineAnnealingLR, LinearLR
+from torch.optim import AdamW
+from transformers import AutoModelForCausalLM, AutoTokenizer
+
+from xtuner.dataset import process_hf_dataset
+from xtuner.dataset.collate_fns import default_collate_fn
+from xtuner.dataset.map_fns import alpaca_zh_map_fn, template_map_fn_factory
+from xtuner.engine.hooks import (DatasetInfoHook, EvaluateChatHook,
+ VarlenAttnArgsToMessageHubHook)
+from xtuner.engine.runner import TrainLoop
+from xtuner.model import SupervisedFinetune
+from xtuner.parallel.sequence import SequenceParallelSampler
+from xtuner.utils import PROMPT_TEMPLATE, SYSTEM_TEMPLATE
+
+#######################################################################
+# PART 1 Settings #
+#######################################################################
+# Model
+pretrained_model_name_or_path = 'openbmb/MiniCPM3-4B'
+use_varlen_attn = False
+
+# Data
+alpaca_en_path = 'silk-road/alpaca-data-gpt4-chinese'
+prompt_template = PROMPT_TEMPLATE.minicpm3
+max_length = 2048
+pack_to_max_length = True
+
+# parallel
+sequence_parallel_size = 1
+
+# Scheduler & Optimizer
+batch_size = 1 # per_device
+accumulative_counts = 16
+accumulative_counts *= sequence_parallel_size
+dataloader_num_workers = 0
+max_steps = 10000
+optim_type = AdamW
+lr = 2e-5
+betas = (0.9, 0.999)
+weight_decay = 0
+max_norm = 1 # grad clip
+warmup_ratio = 0.03
+
+# Save
+save_steps = 500
+save_total_limit = 2 # Maximum checkpoints to keep (-1 means unlimited)
+
+# Evaluate the generation performance during the training
+evaluation_freq = 500
+SYSTEM = SYSTEM_TEMPLATE.alpaca
+evaluation_inputs = [
+ '请给我介绍五个上海的景点', 'Please tell me five scenic spots in Shanghai'
+]
+
+#######################################################################
+# PART 2 Model & Tokenizer #
+#######################################################################
+tokenizer = dict(
+ type=AutoTokenizer.from_pretrained,
+ pretrained_model_name_or_path=pretrained_model_name_or_path,
+ trust_remote_code=True,
+ padding_side='right',
+ eos_token='')
+
+model = dict(
+ type=SupervisedFinetune,
+ use_varlen_attn=use_varlen_attn,
+ llm=dict(
+ type=AutoModelForCausalLM.from_pretrained,
+ pretrained_model_name_or_path=pretrained_model_name_or_path,
+ trust_remote_code=True))
+
+#######################################################################
+# PART 3 Dataset & Dataloader #
+#######################################################################
+alpaca_en = dict(
+ type=process_hf_dataset,
+ dataset=dict(type=load_dataset, path=alpaca_en_path),
+ tokenizer=tokenizer,
+ max_length=max_length,
+ dataset_map_fn=alpaca_zh_map_fn,
+ template_map_fn=dict(
+ type=template_map_fn_factory, template=prompt_template),
+ remove_unused_columns=True,
+ shuffle_before_pack=True,
+ pack_to_max_length=pack_to_max_length,
+ use_varlen_attn=use_varlen_attn)
+
+sampler = SequenceParallelSampler \
+ if sequence_parallel_size > 1 else DefaultSampler
+
+train_dataloader = dict(
+ batch_size=batch_size,
+ num_workers=dataloader_num_workers,
+ dataset=alpaca_en,
+ sampler=dict(type=sampler, shuffle=True),
+ collate_fn=dict(type=default_collate_fn, use_varlen_attn=use_varlen_attn))
+
+#######################################################################
+# PART 4 Scheduler & Optimizer #
+#######################################################################
+# optimizer
+optim_wrapper = dict(
+ type=AmpOptimWrapper,
+ optimizer=dict(
+ type=optim_type, lr=lr, betas=betas, weight_decay=weight_decay),
+ clip_grad=dict(max_norm=max_norm, error_if_nonfinite=False),
+ accumulative_counts=accumulative_counts,
+ loss_scale='dynamic',
+ dtype='float16')
+
+# learning policy
+# More information: https://github.com/open-mmlab/mmengine/blob/main/docs/en/tutorials/param_scheduler.md # noqa: E501
+param_scheduler = [
+ dict(
+ type=LinearLR,
+ start_factor=1e-5,
+ by_epoch=True,
+ begin=0,
+ end=warmup_ratio * max_steps,
+ convert_to_iter_based=True),
+ dict(
+ type=CosineAnnealingLR,
+ eta_min=0.0,
+ by_epoch=True,
+ begin=warmup_ratio * max_steps,
+ end=max_steps,
+ convert_to_iter_based=True)
+]
+
+# train, val, test setting
+train_cfg = dict(type=TrainLoop, max_iters=max_steps)
+
+#######################################################################
+# PART 5 Runtime #
+#######################################################################
+# Log the dialogue periodically during the training process, optional
+custom_hooks = [
+ dict(type=DatasetInfoHook, tokenizer=tokenizer),
+ dict(
+ type=EvaluateChatHook,
+ tokenizer=tokenizer,
+ every_n_iters=evaluation_freq,
+ evaluation_inputs=evaluation_inputs,
+ system=SYSTEM,
+ prompt_template=prompt_template)
+]
+
+if use_varlen_attn:
+ custom_hooks += [dict(type=VarlenAttnArgsToMessageHubHook)]
+
+# configure default hooks
+default_hooks = dict(
+ # record the time of every iteration.
+ timer=dict(type=IterTimerHook),
+ # print log every 10 iterations.
+ logger=dict(type=LoggerHook, log_metric_by_epoch=False, interval=10),
+ # enable the parameter scheduler.
+ param_scheduler=dict(type=ParamSchedulerHook),
+ # save checkpoint per `save_steps`.
+ checkpoint=dict(
+ type=CheckpointHook,
+ by_epoch=False,
+ interval=save_steps,
+ max_keep_ckpts=save_total_limit),
+ # set sampler seed in distributed evrionment.
+ sampler_seed=dict(type=DistSamplerSeedHook),
+)
+
+# configure environment
+env_cfg = dict(
+ # whether to enable cudnn benchmark
+ cudnn_benchmark=False,
+ # set multi process parameters
+ mp_cfg=dict(mp_start_method='fork', opencv_num_threads=0),
+ # set distributed parameters
+ dist_cfg=dict(backend='nccl'),
+)
+
+# set visualizer
+visualizer = None
+
+# set log level
+log_level = 'INFO'
+
+# load from which checkpoint
+load_from = None
+
+# whether to resume training from the loaded checkpoint
+resume = False
+
+# Defaults to use random seed and disable `deterministic`
+randomness = dict(seed=None, deterministic=False)
+
+# set log processor
+log_processor = dict(by_epoch=False)
diff --git a/xtuner/configs/mistral/mistral_7b_full_finetune_custom_dataset_e1.py b/xtuner/configs/mistral/mistral_7b_full_finetune_custom_dataset_e1.py
index cf1034f3d..72c7a50aa 100644
--- a/xtuner/configs/mistral/mistral_7b_full_finetune_custom_dataset_e1.py
+++ b/xtuner/configs/mistral/mistral_7b_full_finetune_custom_dataset_e1.py
@@ -50,12 +50,16 @@
max_length = 32768
pack_to_max_length = True
+# parallel
+sequence_parallel_size = 1
+
# Scheduler & Optimizer
# batch size per device, set to 1 if `use_varlen_attn` = True
# To clarify, enlarging the batch size essentially enlarges the `max_length`.
# For example, doubling the max length is tantamount to doubling the batch size
batch_size = 1
accumulative_counts = 1 # 1bs * 1acc * 64gpu = 64 batchsize
+accumulative_counts *= sequence_parallel_size
dataloader_num_workers = 4
max_epochs = 1
optim_type = AdamW
diff --git a/xtuner/configs/mistral/mistral_7b_qlora_skypile_pretrain_e1.py b/xtuner/configs/mistral/mistral_7b_qlora_skypile_pretrain_e1.py
index 515c8c653..e1260fe5b 100644
--- a/xtuner/configs/mistral/mistral_7b_qlora_skypile_pretrain_e1.py
+++ b/xtuner/configs/mistral/mistral_7b_qlora_skypile_pretrain_e1.py
@@ -16,6 +16,7 @@
VarlenAttnArgsToMessageHubHook)
from xtuner.engine.runner import TrainLoop
from xtuner.model import SupervisedFinetune
+from xtuner.parallel.sequence import SequenceParallelSampler
#######################################################################
# PART 1 Settings #
@@ -29,9 +30,13 @@
max_length = 2048
pack_to_max_length = True
+# parallel
+sequence_parallel_size = 1
+
# Scheduler & Optimizer
batch_size = 1 # per_device
accumulative_counts = 16
+accumulative_counts *= sequence_parallel_size
dataloader_num_workers = 0
max_epochs = 1
optim_type = AdamW
@@ -98,11 +103,14 @@
pack_to_max_length=pack_to_max_length,
use_varlen_attn=use_varlen_attn)
+sampler = SequenceParallelSampler \
+ if sequence_parallel_size > 1 else DefaultSampler
+
train_dataloader = dict(
batch_size=batch_size,
num_workers=dataloader_num_workers,
dataset=train_dataset,
- sampler=dict(type=DefaultSampler, shuffle=True),
+ sampler=dict(type=sampler, shuffle=True),
collate_fn=dict(type=default_collate_fn, use_varlen_attn=use_varlen_attn))
#######################################################################
diff --git a/xtuner/configs/mistral/mistral_7b_w_tokenized_dataset.py b/xtuner/configs/mistral/mistral_7b_w_tokenized_dataset.py
index 12cceb950..660a023cc 100644
--- a/xtuner/configs/mistral/mistral_7b_w_tokenized_dataset.py
+++ b/xtuner/configs/mistral/mistral_7b_w_tokenized_dataset.py
@@ -35,12 +35,16 @@
max_length = 32768
pack_to_max_length = True
+# parallel
+sequence_parallel_size = 1
+
# Scheduler & Optimizer
# batch size per device, set to 1 if `use_varlen_attn` = True
# To clarify, enlarging the batch size essentially enlarges the `max_length`.
# For example, doubling the max length is tantamount to doubling the batch size
batch_size = 1
accumulative_counts = 1
+accumulative_counts *= sequence_parallel_size
dataloader_num_workers = 0
max_epochs = 1
optim_type = AdamW
diff --git a/xtuner/configs/mistral/mistral_7b_w_untokenized_dataset.py b/xtuner/configs/mistral/mistral_7b_w_untokenized_dataset.py
index 2096771a8..e1bbe9304 100644
--- a/xtuner/configs/mistral/mistral_7b_w_untokenized_dataset.py
+++ b/xtuner/configs/mistral/mistral_7b_w_untokenized_dataset.py
@@ -31,12 +31,16 @@
max_length = 32768
pack_to_max_length = True
+# parallel
+sequence_parallel_size = 1
+
# Scheduler & Optimizer
# batch size per device, set to 1 if `use_varlen_attn` = True
# To clarify, enlarging the batch size essentially enlarges the `max_length`.
# For example, doubling the max length is tantamount to doubling the batch size
batch_size = 1
accumulative_counts = 1
+accumulative_counts *= sequence_parallel_size
dataloader_num_workers = 0
max_epochs = 1
optim_type = AdamW
diff --git a/xtuner/configs/mixtral/README.md b/xtuner/configs/mixtral/README.md
index 3a98ea978..eaee3324d 100644
--- a/xtuner/configs/mixtral/README.md
+++ b/xtuner/configs/mixtral/README.md
@@ -6,9 +6,6 @@
# Install the latest xtuner
pip install -U 'xtuner[deepspeed]'
-# Mixtral requires the latest version of transformers.
-pip install git+https://github.com/huggingface/transformers.git
-
# Mixtral requires flash-attn
pip install flash-attn
@@ -47,3 +44,14 @@ NPROC_PER_NODE=8 NNODES=2 PORT=29600 ADDR=$NODE_0_ADDR NODE_RANK=0 xtuner train
# excuete on node 1
NPROC_PER_NODE=8 NNODES=2 PORT=29600 ADDR=$NODE_0_ADDR NODE_RANK=1 xtuner train mixtral_8x7b_instruct_full_oasst1_e3 --deepspeed deepspeed_zero3
```
+
+### Speed
+
+16 * A100 80G:
+
+| Model | Sequence Length | Use Varlen Attn | Sequence Parallel World Size | Tokens per Second |
+| :----------: | :-------------: | :-------------: | :--------------------------: | :---------------: |
+| mixtral_8x7b | 32k | False | 1 | 853.7 |
+| mixtral_8x7b | 32k | True | 1 | 910.1 |
+| mixtral_8x7b | 32k | False | 2 | 635.2 |
+| mixtral_8x7b | 32k | True | 2 | 650.9 |
diff --git a/xtuner/configs/mixtral/mixtral_8x7b/mixtral_8x7b_full_oasst1_e3.py b/xtuner/configs/mixtral/mixtral_8x7b/mixtral_8x7b_full_oasst1_e3.py
index 9b3057b88..784879ac2 100644
--- a/xtuner/configs/mixtral/mixtral_8x7b/mixtral_8x7b_full_oasst1_e3.py
+++ b/xtuner/configs/mixtral/mixtral_8x7b/mixtral_8x7b_full_oasst1_e3.py
@@ -15,6 +15,7 @@
VarlenAttnArgsToMessageHubHook)
from xtuner.engine.runner import TrainLoop
from xtuner.model import SupervisedFinetune
+from xtuner.parallel.sequence import SequenceParallelSampler
from xtuner.utils import PROMPT_TEMPLATE
#######################################################################
@@ -30,9 +31,13 @@
max_length = 2048
pack_to_max_length = True
+# parallel
+sequence_parallel_size = 1
+
# Scheduler & Optimizer
batch_size = 1 # per_device
accumulative_counts = 16
+accumulative_counts *= sequence_parallel_size
dataloader_num_workers = 0
max_epochs = 3
optim_type = AdamW
@@ -86,11 +91,14 @@
pack_to_max_length=pack_to_max_length,
use_varlen_attn=use_varlen_attn)
+sampler = SequenceParallelSampler \
+ if sequence_parallel_size > 1 else DefaultSampler
+
train_dataloader = dict(
batch_size=batch_size,
num_workers=dataloader_num_workers,
dataset=train_dataset,
- sampler=dict(type=DefaultSampler, shuffle=True),
+ sampler=dict(type=sampler, shuffle=True),
collate_fn=dict(type=default_collate_fn, use_varlen_attn=use_varlen_attn))
#######################################################################
diff --git a/xtuner/configs/mixtral/mixtral_8x7b/mixtral_8x7b_qlora_oasst1_e3.py b/xtuner/configs/mixtral/mixtral_8x7b/mixtral_8x7b_qlora_oasst1_e3.py
index 6e0991832..cb11f102f 100644
--- a/xtuner/configs/mixtral/mixtral_8x7b/mixtral_8x7b_qlora_oasst1_e3.py
+++ b/xtuner/configs/mixtral/mixtral_8x7b/mixtral_8x7b_qlora_oasst1_e3.py
@@ -17,6 +17,7 @@
VarlenAttnArgsToMessageHubHook)
from xtuner.engine.runner import TrainLoop
from xtuner.model import SupervisedFinetune
+from xtuner.parallel.sequence import SequenceParallelSampler
from xtuner.utils import PROMPT_TEMPLATE
#######################################################################
@@ -32,9 +33,13 @@
max_length = 2048
pack_to_max_length = True
+# parallel
+sequence_parallel_size = 1
+
# Scheduler & Optimizer
batch_size = 1 # per_device
accumulative_counts = 16
+accumulative_counts *= sequence_parallel_size
dataloader_num_workers = 0
max_epochs = 3
optim_type = AdamW
@@ -108,11 +113,14 @@
pack_to_max_length=pack_to_max_length,
use_varlen_attn=use_varlen_attn)
+sampler = SequenceParallelSampler \
+ if sequence_parallel_size > 1 else DefaultSampler
+
train_dataloader = dict(
batch_size=batch_size,
num_workers=dataloader_num_workers,
dataset=train_dataset,
- sampler=dict(type=DefaultSampler, shuffle=True),
+ sampler=dict(type=sampler, shuffle=True),
collate_fn=dict(type=default_collate_fn, use_varlen_attn=use_varlen_attn))
#######################################################################
diff --git a/xtuner/configs/mixtral/mixtral_8x7b_instruct/mixtral_8x7b_instruct_full_oasst1_e3.py b/xtuner/configs/mixtral/mixtral_8x7b_instruct/mixtral_8x7b_instruct_full_oasst1_e3.py
index ac1107d47..0093d0d9a 100644
--- a/xtuner/configs/mixtral/mixtral_8x7b_instruct/mixtral_8x7b_instruct_full_oasst1_e3.py
+++ b/xtuner/configs/mixtral/mixtral_8x7b_instruct/mixtral_8x7b_instruct_full_oasst1_e3.py
@@ -15,6 +15,7 @@
VarlenAttnArgsToMessageHubHook)
from xtuner.engine.runner import TrainLoop
from xtuner.model import SupervisedFinetune
+from xtuner.parallel.sequence import SequenceParallelSampler
from xtuner.utils import PROMPT_TEMPLATE
#######################################################################
@@ -30,9 +31,13 @@
max_length = 2048
pack_to_max_length = True
+# parallel
+sequence_parallel_size = 1
+
# Scheduler & Optimizer
batch_size = 1 # per_device
accumulative_counts = 16
+accumulative_counts *= sequence_parallel_size
dataloader_num_workers = 0
max_epochs = 3
optim_type = AdamW
@@ -86,11 +91,14 @@
pack_to_max_length=pack_to_max_length,
use_varlen_attn=use_varlen_attn)
+sampler = SequenceParallelSampler \
+ if sequence_parallel_size > 1 else DefaultSampler
+
train_dataloader = dict(
batch_size=batch_size,
num_workers=dataloader_num_workers,
dataset=train_dataset,
- sampler=dict(type=DefaultSampler, shuffle=True),
+ sampler=dict(type=sampler, shuffle=True),
collate_fn=dict(type=default_collate_fn, use_varlen_attn=use_varlen_attn))
#######################################################################
diff --git a/xtuner/configs/mixtral/mixtral_8x7b_instruct/mixtral_8x7b_instruct_qlora_oasst1_e3.py b/xtuner/configs/mixtral/mixtral_8x7b_instruct/mixtral_8x7b_instruct_qlora_oasst1_e3.py
index 9530d26d8..3f348f9d9 100644
--- a/xtuner/configs/mixtral/mixtral_8x7b_instruct/mixtral_8x7b_instruct_qlora_oasst1_e3.py
+++ b/xtuner/configs/mixtral/mixtral_8x7b_instruct/mixtral_8x7b_instruct_qlora_oasst1_e3.py
@@ -17,6 +17,7 @@
VarlenAttnArgsToMessageHubHook)
from xtuner.engine.runner import TrainLoop
from xtuner.model import SupervisedFinetune
+from xtuner.parallel.sequence import SequenceParallelSampler
from xtuner.utils import PROMPT_TEMPLATE
#######################################################################
@@ -32,9 +33,13 @@
max_length = 2048
pack_to_max_length = True
+# parallel
+sequence_parallel_size = 1
+
# Scheduler & Optimizer
batch_size = 1 # per_device
accumulative_counts = 16
+accumulative_counts *= sequence_parallel_size
dataloader_num_workers = 0
max_epochs = 3
optim_type = AdamW
@@ -108,11 +113,14 @@
pack_to_max_length=pack_to_max_length,
use_varlen_attn=use_varlen_attn)
+sampler = SequenceParallelSampler \
+ if sequence_parallel_size > 1 else DefaultSampler
+
train_dataloader = dict(
batch_size=batch_size,
num_workers=dataloader_num_workers,
dataset=train_dataset,
- sampler=dict(type=DefaultSampler, shuffle=True),
+ sampler=dict(type=sampler, shuffle=True),
collate_fn=dict(type=default_collate_fn, use_varlen_attn=use_varlen_attn))
#######################################################################
diff --git a/xtuner/configs/orpo/internlm/internlm2_chat_1_8b_orpo_full.py b/xtuner/configs/orpo/internlm/internlm2_chat_1_8b_orpo_full.py
new file mode 100644
index 000000000..52881739a
--- /dev/null
+++ b/xtuner/configs/orpo/internlm/internlm2_chat_1_8b_orpo_full.py
@@ -0,0 +1,197 @@
+# Copyright (c) OpenMMLab. All rights reserved.
+from datasets import load_dataset
+from mmengine.dataset import DefaultSampler
+from mmengine.hooks import (CheckpointHook, DistSamplerSeedHook, IterTimerHook,
+ LoggerHook, ParamSchedulerHook)
+from mmengine.optim import AmpOptimWrapper, CosineAnnealingLR, LinearLR
+from torch.optim import AdamW
+from transformers import AutoModelForCausalLM, AutoTokenizer
+
+from xtuner.dataset.collate_fns.preference_collate_fn import \
+ preference_collate_fn
+from xtuner.dataset.preference_dataset import (build_preference_dataset,
+ orpo_dpo_mix_40k_map_fn)
+from xtuner.engine.hooks import (EvaluateChatHook,
+ VarlenAttnArgsToMessageHubHook)
+from xtuner.engine.runner import TrainLoop
+from xtuner.model.orpo import ORPO
+from xtuner.utils import PROMPT_TEMPLATE, SYSTEM_TEMPLATE
+
+#######################################################################
+# PART 1 Settings #
+#######################################################################
+# Model
+pretrained_model_name_or_path = 'internlm/internlm2-chat-1_8b-sft'
+use_varlen_attn = False
+loss_beta = 0.1
+
+# Data
+prompt_template = PROMPT_TEMPLATE.internlm2_chat
+max_length = 2048
+
+# Scheduler & Optimizer
+batch_size = 1 # per_device
+accumulative_counts = 16
+dataloader_num_workers = 0
+max_epochs = 3
+optim_type = AdamW
+lr = 5e-6
+betas = (0.9, 0.999)
+weight_decay = 0
+max_norm = 1 # grad clip
+warmup_ratio = 0.03
+
+# Save
+save_steps = 500
+save_total_limit = 2 # Maximum checkpoints to keep (-1 means unlimited)
+
+# Evaluate the generation performance during the training
+evaluation_freq = 500
+SYSTEM = SYSTEM_TEMPLATE.alpaca
+evaluation_inputs = [
+ 'What famous British author, known for his tales of mystery and the macabre, shares his initials with a common abbreviation for "rest in peace"?', # noqa: E501
+ 'Please tell me five scenic spots in Shanghai',
+ '890729 - 425663? Only respond with math and no words.'
+]
+
+#######################################################################
+# PART 2 Model & Tokenizer #
+#######################################################################
+tokenizer = dict(
+ type=AutoTokenizer.from_pretrained,
+ pretrained_model_name_or_path=pretrained_model_name_or_path,
+ trust_remote_code=True,
+ padding_side='right')
+
+model = dict(
+ type=ORPO,
+ beta=loss_beta,
+ use_varlen_attn=use_varlen_attn,
+ llm=dict(
+ type=AutoModelForCausalLM.from_pretrained,
+ pretrained_model_name_or_path=pretrained_model_name_or_path,
+ trust_remote_code=True))
+
+#######################################################################
+# PART 3 Dataset & Dataloader #
+#######################################################################
+train_dataset = dict(
+ type=build_preference_dataset,
+ dataset=dict(type=load_dataset, path='mlabonne/orpo-dpo-mix-40k'),
+ tokenizer=tokenizer,
+ max_length=max_length,
+ dataset_map_fn=orpo_dpo_mix_40k_map_fn,
+ is_dpo=True,
+ is_reward=False,
+ reward_token_id=-1,
+ num_proc=32,
+ use_varlen_attn=use_varlen_attn,
+ shuffle_before_pack=True,
+)
+
+train_dataloader = dict(
+ batch_size=batch_size,
+ num_workers=dataloader_num_workers,
+ dataset=train_dataset,
+ sampler=dict(type=DefaultSampler, shuffle=True),
+ collate_fn=dict(
+ type=preference_collate_fn, use_varlen_attn=use_varlen_attn))
+
+#######################################################################
+# PART 4 Scheduler & Optimizer #
+#######################################################################
+# optimizer
+optim_wrapper = dict(
+ type=AmpOptimWrapper,
+ optimizer=dict(
+ type=optim_type, lr=lr, betas=betas, weight_decay=weight_decay),
+ clip_grad=dict(max_norm=max_norm, error_if_nonfinite=False),
+ accumulative_counts=accumulative_counts,
+ loss_scale='dynamic',
+ dtype='float16')
+
+# learning policy
+# More information: https://github.com/open-mmlab/mmengine/blob/main/docs/en/tutorials/param_scheduler.md # noqa: E501
+param_scheduler = [
+ dict(
+ type=LinearLR,
+ start_factor=1e-5,
+ by_epoch=True,
+ begin=0,
+ end=warmup_ratio * max_epochs,
+ convert_to_iter_based=True),
+ dict(
+ type=CosineAnnealingLR,
+ eta_min=0.0,
+ by_epoch=True,
+ begin=warmup_ratio * max_epochs,
+ end=max_epochs,
+ convert_to_iter_based=True)
+]
+
+# train, val, test setting
+train_cfg = dict(type=TrainLoop, max_epochs=max_epochs)
+
+#######################################################################
+# PART 5 Runtime #
+#######################################################################
+# Log the dialogue periodically during the training process, optional
+custom_hooks = [
+ # dict(type=DatasetInfoHook, tokenizer=tokenizer),
+ dict(
+ type=EvaluateChatHook,
+ tokenizer=tokenizer,
+ every_n_iters=evaluation_freq,
+ evaluation_inputs=evaluation_inputs,
+ system=SYSTEM,
+ prompt_template=prompt_template)
+]
+
+if use_varlen_attn:
+ custom_hooks += [dict(type=VarlenAttnArgsToMessageHubHook)]
+
+# configure default hooks
+default_hooks = dict(
+ # record the time of every iteration.
+ timer=dict(type=IterTimerHook),
+ # print log every 10 iterations.
+ logger=dict(type=LoggerHook, log_metric_by_epoch=False, interval=10),
+ # enable the parameter scheduler.
+ param_scheduler=dict(type=ParamSchedulerHook),
+ # save checkpoint per `save_steps`.
+ checkpoint=dict(
+ type=CheckpointHook,
+ by_epoch=False,
+ interval=save_steps,
+ max_keep_ckpts=save_total_limit),
+ # set sampler seed in distributed evrionment.
+ sampler_seed=dict(type=DistSamplerSeedHook),
+)
+
+# configure environment
+env_cfg = dict(
+ # whether to enable cudnn benchmark
+ cudnn_benchmark=False,
+ # set multi process parameters
+ mp_cfg=dict(mp_start_method='fork', opencv_num_threads=0),
+ # set distributed parameters
+ dist_cfg=dict(backend='nccl'),
+)
+
+# set visualizer
+visualizer = None
+
+# set log level
+log_level = 'INFO'
+
+# load from which checkpoint
+load_from = None
+
+# whether to resume training from the loaded checkpoint
+resume = False
+
+# Defaults to use random seed and disable `deterministic`
+randomness = dict(seed=None, deterministic=False)
+
+# set log processor
+log_processor = dict(by_epoch=False)
diff --git a/xtuner/configs/orpo/internlm/internlm2_chat_1_8b_orpo_full_varlenattn.py b/xtuner/configs/orpo/internlm/internlm2_chat_1_8b_orpo_full_varlenattn.py
new file mode 100644
index 000000000..d4cf3d65a
--- /dev/null
+++ b/xtuner/configs/orpo/internlm/internlm2_chat_1_8b_orpo_full_varlenattn.py
@@ -0,0 +1,207 @@
+# Copyright (c) OpenMMLab. All rights reserved.
+from datasets import load_dataset
+from mmengine.dataset import DefaultSampler
+from mmengine.hooks import (CheckpointHook, DistSamplerSeedHook, IterTimerHook,
+ LoggerHook, ParamSchedulerHook)
+from mmengine.optim import AmpOptimWrapper, CosineAnnealingLR, LinearLR
+from torch.optim import AdamW
+from transformers import AutoModelForCausalLM, AutoTokenizer
+
+from xtuner.dataset.collate_fns.preference_collate_fn import \
+ preference_collate_fn
+from xtuner.dataset.preference_dataset import (build_preference_dataset,
+ orpo_dpo_mix_40k_map_fn)
+from xtuner.engine.hooks import (EvaluateChatHook,
+ VarlenAttnArgsToMessageHubHook)
+from xtuner.engine.runner import TrainLoop
+from xtuner.model.orpo import ORPO
+from xtuner.parallel.sequence import SequenceParallelSampler
+from xtuner.utils import PROMPT_TEMPLATE, SYSTEM_TEMPLATE
+
+#######################################################################
+# PART 1 Settings #
+#######################################################################
+# Model
+pretrained_model_name_or_path = 'internlm/internlm2-chat-1_8b-sft'
+use_varlen_attn = True
+loss_beta = 0.1
+
+# parallel
+sequence_parallel_size = 1
+
+# Data
+prompt_template = PROMPT_TEMPLATE.internlm2_chat
+max_length = 2048
+max_packed_length = max_length * 2
+
+# Scheduler & Optimizer
+batch_size = 1 # per_device
+accumulative_counts = 16
+accumulative_counts *= sequence_parallel_size
+dataloader_num_workers = 0
+max_epochs = 3
+optim_type = AdamW
+lr = 5e-6
+betas = (0.9, 0.999)
+weight_decay = 0
+max_norm = 1 # grad clip
+warmup_ratio = 0.03
+
+# Save
+save_steps = 500
+save_total_limit = 2 # Maximum checkpoints to keep (-1 means unlimited)
+
+# Evaluate the generation performance during the training
+evaluation_freq = 500
+SYSTEM = SYSTEM_TEMPLATE.alpaca
+evaluation_inputs = [
+ 'What famous British author, known for his tales of mystery and the macabre, shares his initials with a common abbreviation for "rest in peace"?', # noqa: E501
+ 'Please tell me five scenic spots in Shanghai',
+ '890729 - 425663? Only respond with math and no words.'
+]
+
+#######################################################################
+# PART 2 Model & Tokenizer #
+#######################################################################
+tokenizer = dict(
+ type=AutoTokenizer.from_pretrained,
+ pretrained_model_name_or_path=pretrained_model_name_or_path,
+ trust_remote_code=True,
+ padding_side='right')
+
+model = dict(
+ type=ORPO,
+ use_varlen_attn=use_varlen_attn,
+ beta=loss_beta,
+ llm=dict(
+ type=AutoModelForCausalLM.from_pretrained,
+ pretrained_model_name_or_path=pretrained_model_name_or_path,
+ trust_remote_code=True))
+
+#######################################################################
+# PART 3 Dataset & Dataloader #
+#######################################################################
+sampler = SequenceParallelSampler \
+ if sequence_parallel_size > 1 else DefaultSampler
+
+train_dataset = dict(
+ type=build_preference_dataset,
+ dataset=dict(type=load_dataset, path='mlabonne/orpo-dpo-mix-40k'),
+ tokenizer=tokenizer,
+ max_length=max_length,
+ dataset_map_fn=orpo_dpo_mix_40k_map_fn,
+ is_dpo=True,
+ is_reward=False,
+ reward_token_id=-1,
+ num_proc=32,
+ use_varlen_attn=use_varlen_attn,
+ max_packed_length=max_packed_length,
+ shuffle_before_pack=True,
+)
+
+train_dataloader = dict(
+ batch_size=batch_size,
+ num_workers=dataloader_num_workers,
+ dataset=train_dataset,
+ sampler=dict(type=sampler, shuffle=True),
+ collate_fn=dict(
+ type=preference_collate_fn, use_varlen_attn=use_varlen_attn))
+
+#######################################################################
+# PART 4 Scheduler & Optimizer #
+#######################################################################
+# optimizer
+optim_wrapper = dict(
+ type=AmpOptimWrapper,
+ optimizer=dict(
+ type=optim_type, lr=lr, betas=betas, weight_decay=weight_decay),
+ clip_grad=dict(max_norm=max_norm, error_if_nonfinite=False),
+ accumulative_counts=accumulative_counts,
+ loss_scale='dynamic',
+ dtype='float16')
+
+# learning policy
+# More information: https://github.com/open-mmlab/mmengine/blob/main/docs/en/tutorials/param_scheduler.md # noqa: E501
+param_scheduler = [
+ dict(
+ type=LinearLR,
+ start_factor=1e-5,
+ by_epoch=True,
+ begin=0,
+ end=warmup_ratio * max_epochs,
+ convert_to_iter_based=True),
+ dict(
+ type=CosineAnnealingLR,
+ eta_min=0.0,
+ by_epoch=True,
+ begin=warmup_ratio * max_epochs,
+ end=max_epochs,
+ convert_to_iter_based=True)
+]
+
+# train, val, test setting
+train_cfg = dict(type=TrainLoop, max_epochs=max_epochs)
+
+#######################################################################
+# PART 5 Runtime #
+#######################################################################
+# Log the dialogue periodically during the training process, optional
+custom_hooks = [
+ # dict(type=DatasetInfoHook, tokenizer=tokenizer),
+ dict(
+ type=EvaluateChatHook,
+ tokenizer=tokenizer,
+ every_n_iters=evaluation_freq,
+ evaluation_inputs=evaluation_inputs,
+ system=SYSTEM,
+ prompt_template=prompt_template)
+]
+
+if use_varlen_attn:
+ custom_hooks += [dict(type=VarlenAttnArgsToMessageHubHook)]
+
+# configure default hooks
+default_hooks = dict(
+ # record the time of every iteration.
+ timer=dict(type=IterTimerHook),
+ # print log every 10 iterations.
+ logger=dict(type=LoggerHook, log_metric_by_epoch=False, interval=10),
+ # enable the parameter scheduler.
+ param_scheduler=dict(type=ParamSchedulerHook),
+ # save checkpoint per `save_steps`.
+ checkpoint=dict(
+ type=CheckpointHook,
+ by_epoch=False,
+ interval=save_steps,
+ max_keep_ckpts=save_total_limit),
+ # set sampler seed in distributed evrionment.
+ sampler_seed=dict(type=DistSamplerSeedHook),
+)
+
+# configure environment
+env_cfg = dict(
+ # whether to enable cudnn benchmark
+ cudnn_benchmark=False,
+ # set multi process parameters
+ mp_cfg=dict(mp_start_method='fork', opencv_num_threads=0),
+ # set distributed parameters
+ dist_cfg=dict(backend='nccl'),
+)
+
+# set visualizer
+visualizer = None
+
+# set log level
+log_level = 'INFO'
+
+# load from which checkpoint
+load_from = None
+
+# whether to resume training from the loaded checkpoint
+resume = False
+
+# Defaults to use random seed and disable `deterministic`
+randomness = dict(seed=None, deterministic=False)
+
+# set log processor
+log_processor = dict(by_epoch=False)
diff --git a/xtuner/configs/orpo/internlm/internlm2_chat_1_8b_orpo_full_varlenattn_jsonl_dataset.py b/xtuner/configs/orpo/internlm/internlm2_chat_1_8b_orpo_full_varlenattn_jsonl_dataset.py
new file mode 100644
index 000000000..126ff4bd8
--- /dev/null
+++ b/xtuner/configs/orpo/internlm/internlm2_chat_1_8b_orpo_full_varlenattn_jsonl_dataset.py
@@ -0,0 +1,211 @@
+# Copyright (c) OpenMMLab. All rights reserved.
+from mmengine.dataset import DefaultSampler
+from mmengine.hooks import (CheckpointHook, DistSamplerSeedHook, IterTimerHook,
+ LoggerHook, ParamSchedulerHook)
+from mmengine.optim import AmpOptimWrapper, CosineAnnealingLR, LinearLR
+from torch.optim import AdamW
+from transformers import AutoModelForCausalLM, AutoTokenizer
+
+from xtuner.dataset.collate_fns.preference_collate_fn import \
+ preference_collate_fn
+from xtuner.dataset.preference_dataset import (build_preference_dataset,
+ load_jsonl_dataset)
+from xtuner.engine.hooks import (EvaluateChatHook,
+ VarlenAttnArgsToMessageHubHook)
+from xtuner.engine.runner import TrainLoop
+from xtuner.model.orpo import ORPO
+from xtuner.parallel.sequence import SequenceParallelSampler
+from xtuner.utils import PROMPT_TEMPLATE, SYSTEM_TEMPLATE
+
+#######################################################################
+# PART 1 Settings #
+#######################################################################
+# Model
+pretrained_model_name_or_path = 'internlm/internlm2-chat-1_8b-sft'
+use_varlen_attn = True
+loss_beta = 0.1
+
+# Data
+prompt_template = PROMPT_TEMPLATE.internlm2_chat
+max_length = 2048
+max_packed_length = max_length * 2
+
+# parallel
+sequence_parallel_size = 1
+
+# Scheduler & Optimizer
+batch_size = 1 # per_device
+accumulative_counts = 16
+accumulative_counts *= sequence_parallel_size
+dataloader_num_workers = 0
+max_epochs = 3
+optim_type = AdamW
+lr = 5e-6
+betas = (0.9, 0.999)
+weight_decay = 0
+max_norm = 1 # grad clip
+warmup_ratio = 0.03
+
+# Save
+save_steps = 500
+save_total_limit = 2 # Maximum checkpoints to keep (-1 means unlimited)
+
+# Evaluate the generation performance during the training
+evaluation_freq = 500
+SYSTEM = SYSTEM_TEMPLATE.alpaca
+evaluation_inputs = [
+ 'What famous British author, known for his tales of mystery and the macabre, shares his initials with a common abbreviation for "rest in peace"?', # noqa: E501
+ 'Please tell me five scenic spots in Shanghai',
+ '890729 - 425663? Only respond with math and no words.'
+]
+
+#######################################################################
+# PART 2 Model & Tokenizer #
+#######################################################################
+tokenizer = dict(
+ type=AutoTokenizer.from_pretrained,
+ pretrained_model_name_or_path=pretrained_model_name_or_path,
+ trust_remote_code=True,
+ padding_side='right')
+
+model = dict(
+ type=ORPO,
+ use_varlen_attn=use_varlen_attn,
+ beta=loss_beta,
+ llm=dict(
+ type=AutoModelForCausalLM.from_pretrained,
+ pretrained_model_name_or_path=pretrained_model_name_or_path,
+ trust_remote_code=True))
+
+#######################################################################
+# PART 3 Dataset & Dataloader #
+#######################################################################
+sampler = SequenceParallelSampler \
+ if sequence_parallel_size > 1 else DefaultSampler
+
+train_dataset = dict(
+ type=build_preference_dataset,
+ dataset=dict(
+ type=load_jsonl_dataset,
+ data_files=[
+ '/your/jsonl/path/here.jsonl',
+ '/your/another/jsonl/path/here.jsonl'
+ ]),
+ tokenizer=tokenizer,
+ max_length=max_length,
+ dataset_map_fn=None,
+ is_dpo=True,
+ is_reward=False,
+ reward_token_id=-1,
+ num_proc=32,
+ use_varlen_attn=use_varlen_attn,
+ max_packed_length=max_packed_length,
+ shuffle_before_pack=True,
+)
+
+train_dataloader = dict(
+ batch_size=batch_size,
+ num_workers=dataloader_num_workers,
+ dataset=train_dataset,
+ sampler=dict(type=DefaultSampler, shuffle=True),
+ collate_fn=dict(
+ type=preference_collate_fn, use_varlen_attn=use_varlen_attn))
+
+#######################################################################
+# PART 4 Scheduler & Optimizer #
+#######################################################################
+# optimizer
+optim_wrapper = dict(
+ type=AmpOptimWrapper,
+ optimizer=dict(
+ type=optim_type, lr=lr, betas=betas, weight_decay=weight_decay),
+ clip_grad=dict(max_norm=max_norm, error_if_nonfinite=False),
+ accumulative_counts=accumulative_counts,
+ loss_scale='dynamic',
+ dtype='float16')
+
+# learning policy
+# More information: https://github.com/open-mmlab/mmengine/blob/main/docs/en/tutorials/param_scheduler.md # noqa: E501
+param_scheduler = [
+ dict(
+ type=LinearLR,
+ start_factor=1e-5,
+ by_epoch=True,
+ begin=0,
+ end=warmup_ratio * max_epochs,
+ convert_to_iter_based=True),
+ dict(
+ type=CosineAnnealingLR,
+ eta_min=0.0,
+ by_epoch=True,
+ begin=warmup_ratio * max_epochs,
+ end=max_epochs,
+ convert_to_iter_based=True)
+]
+
+# train, val, test setting
+train_cfg = dict(type=TrainLoop, max_epochs=max_epochs)
+
+#######################################################################
+# PART 5 Runtime #
+#######################################################################
+# Log the dialogue periodically during the training process, optional
+custom_hooks = [
+ # dict(type=DatasetInfoHook, tokenizer=tokenizer),
+ dict(
+ type=EvaluateChatHook,
+ tokenizer=tokenizer,
+ every_n_iters=evaluation_freq,
+ evaluation_inputs=evaluation_inputs,
+ system=SYSTEM,
+ prompt_template=prompt_template)
+]
+
+if use_varlen_attn:
+ custom_hooks += [dict(type=VarlenAttnArgsToMessageHubHook)]
+
+# configure default hooks
+default_hooks = dict(
+ # record the time of every iteration.
+ timer=dict(type=IterTimerHook),
+ # print log every 10 iterations.
+ logger=dict(type=LoggerHook, log_metric_by_epoch=False, interval=10),
+ # enable the parameter scheduler.
+ param_scheduler=dict(type=ParamSchedulerHook),
+ # save checkpoint per `save_steps`.
+ checkpoint=dict(
+ type=CheckpointHook,
+ by_epoch=False,
+ interval=save_steps,
+ max_keep_ckpts=save_total_limit),
+ # set sampler seed in distributed evrionment.
+ sampler_seed=dict(type=DistSamplerSeedHook),
+)
+
+# configure environment
+env_cfg = dict(
+ # whether to enable cudnn benchmark
+ cudnn_benchmark=False,
+ # set multi process parameters
+ mp_cfg=dict(mp_start_method='fork', opencv_num_threads=0),
+ # set distributed parameters
+ dist_cfg=dict(backend='nccl'),
+)
+
+# set visualizer
+visualizer = None
+
+# set log level
+log_level = 'INFO'
+
+# load from which checkpoint
+load_from = None
+
+# whether to resume training from the loaded checkpoint
+resume = False
+
+# Defaults to use random seed and disable `deterministic`
+randomness = dict(seed=None, deterministic=False)
+
+# set log processor
+log_processor = dict(by_epoch=False)
diff --git a/xtuner/configs/orpo/internlm/internlm2_chat_7b_orpo_qlora_varlenattn_ultrafeedback_e5.py b/xtuner/configs/orpo/internlm/internlm2_chat_7b_orpo_qlora_varlenattn_ultrafeedback_e5.py
new file mode 100644
index 000000000..2e7cdaa0a
--- /dev/null
+++ b/xtuner/configs/orpo/internlm/internlm2_chat_7b_orpo_qlora_varlenattn_ultrafeedback_e5.py
@@ -0,0 +1,229 @@
+# Copyright (c) OpenMMLab. All rights reserved.
+import torch
+from datasets import load_dataset
+from mmengine.dataset import DefaultSampler
+from mmengine.hooks import (CheckpointHook, DistSamplerSeedHook, IterTimerHook,
+ LoggerHook, ParamSchedulerHook)
+from mmengine.optim import AmpOptimWrapper, CosineAnnealingLR, LinearLR
+from peft import LoraConfig
+from torch.optim import AdamW
+from transformers import (AutoModelForCausalLM, AutoTokenizer,
+ BitsAndBytesConfig)
+
+from xtuner.dataset.collate_fns.preference_collate_fn import \
+ preference_collate_fn
+from xtuner.dataset.preference_dataset import (build_preference_dataset,
+ orpo_dpo_mix_40k_map_fn)
+from xtuner.engine.hooks import (EvaluateChatHook,
+ VarlenAttnArgsToMessageHubHook)
+from xtuner.engine.runner import TrainLoop
+from xtuner.model.orpo import ORPO
+from xtuner.parallel.sequence import SequenceParallelSampler
+from xtuner.utils import PROMPT_TEMPLATE, SYSTEM_TEMPLATE
+
+#######################################################################
+# PART 1 Settings #
+#######################################################################
+# Model
+pretrained_model_name_or_path = 'internlm/internlm2-chat-1_8b-sft'
+use_varlen_attn = True
+loss_beta = 0.1
+
+# Data
+prompt_template = PROMPT_TEMPLATE.internlm2_chat
+max_length = 2048
+max_packed_length = max_length * 2
+
+# parallel
+sequence_parallel_size = 1
+
+# Scheduler & Optimizer
+batch_size = 1 # per_device
+accumulative_counts = 16
+accumulative_counts *= sequence_parallel_size
+dataloader_num_workers = 0
+max_epochs = 5 # refer to orpo repo
+optim_type = AdamW
+lr = 5e-6
+betas = (0.9, 0.999)
+weight_decay = 0
+max_norm = 1 # grad clip
+warmup_ratio = 0.01
+
+# Save
+save_steps = 500
+save_total_limit = 2 # Maximum checkpoints to keep (-1 means unlimited)
+
+# Evaluate the generation performance during the training
+evaluation_freq = 500
+SYSTEM = SYSTEM_TEMPLATE.alpaca
+evaluation_inputs = [
+ 'What famous British author, known for his tales of mystery and the macabre, shares his initials with a common abbreviation for "rest in peace"?', # noqa: E501
+ 'Please tell me five scenic spots in Shanghai',
+ '890729 - 425663? Only respond with math and no words.'
+]
+
+#######################################################################
+# PART 2 Model & Tokenizer #
+#######################################################################
+tokenizer = dict(
+ type=AutoTokenizer.from_pretrained,
+ pretrained_model_name_or_path=pretrained_model_name_or_path,
+ trust_remote_code=True,
+ padding_side='right')
+
+model = dict(
+ type=ORPO,
+ use_varlen_attn=use_varlen_attn,
+ beta=loss_beta,
+ llm=dict(
+ type=AutoModelForCausalLM.from_pretrained,
+ pretrained_model_name_or_path=pretrained_model_name_or_path,
+ trust_remote_code=True,
+ torch_dtype=torch.float16,
+ quantization_config=dict(
+ type=BitsAndBytesConfig,
+ load_in_4bit=True,
+ load_in_8bit=False,
+ llm_int8_threshold=6.0,
+ llm_int8_has_fp16_weight=False,
+ bnb_4bit_compute_dtype=torch.float16,
+ bnb_4bit_use_double_quant=True,
+ bnb_4bit_quant_type='nf4')),
+ lora=dict(
+ type=LoraConfig,
+ r=64,
+ lora_alpha=16,
+ lora_dropout=0.1,
+ bias='none',
+ task_type='CAUSAL_LM'))
+
+#######################################################################
+# PART 3 Dataset & Dataloader #
+#######################################################################
+sampler = SequenceParallelSampler \
+ if sequence_parallel_size > 1 else DefaultSampler
+
+train_dataset = dict(
+ type=build_preference_dataset,
+ dataset=dict(
+ type=load_dataset,
+ path='argilla/ultrafeedback-binarized-preferences-cleaned'),
+ tokenizer=tokenizer,
+ max_length=max_length,
+ dataset_map_fn=orpo_dpo_mix_40k_map_fn,
+ is_dpo=True,
+ is_reward=False,
+ reward_token_id=-1,
+ num_proc=32,
+ use_varlen_attn=use_varlen_attn,
+ max_packed_length=max_packed_length,
+ shuffle_before_pack=True,
+)
+
+train_dataloader = dict(
+ batch_size=batch_size,
+ num_workers=dataloader_num_workers,
+ dataset=train_dataset,
+ sampler=dict(type=sampler, shuffle=True),
+ collate_fn=dict(
+ type=preference_collate_fn, use_varlen_attn=use_varlen_attn))
+
+#######################################################################
+# PART 4 Scheduler & Optimizer #
+#######################################################################
+# optimizer
+optim_wrapper = dict(
+ type=AmpOptimWrapper,
+ optimizer=dict(
+ type=optim_type, lr=lr, betas=betas, weight_decay=weight_decay),
+ clip_grad=dict(max_norm=max_norm, error_if_nonfinite=False),
+ accumulative_counts=accumulative_counts,
+ loss_scale='dynamic',
+ dtype='float16')
+
+# learning policy
+# More information: https://github.com/open-mmlab/mmengine/blob/main/docs/en/tutorials/param_scheduler.md # noqa: E501
+param_scheduler = [
+ dict(
+ type=LinearLR,
+ start_factor=1e-5,
+ by_epoch=True,
+ begin=0,
+ end=warmup_ratio * max_epochs,
+ convert_to_iter_based=True),
+ dict(
+ type=CosineAnnealingLR,
+ eta_min=0.0,
+ by_epoch=True,
+ begin=warmup_ratio * max_epochs,
+ end=max_epochs,
+ convert_to_iter_based=True)
+]
+
+# train, val, test setting
+train_cfg = dict(type=TrainLoop, max_epochs=max_epochs)
+
+#######################################################################
+# PART 5 Runtime #
+#######################################################################
+# Log the dialogue periodically during the training process, optional
+custom_hooks = [
+ # dict(type=DatasetInfoHook, tokenizer=tokenizer),
+ dict(
+ type=EvaluateChatHook,
+ tokenizer=tokenizer,
+ every_n_iters=evaluation_freq,
+ evaluation_inputs=evaluation_inputs,
+ system=SYSTEM,
+ prompt_template=prompt_template)
+]
+
+if use_varlen_attn:
+ custom_hooks += [dict(type=VarlenAttnArgsToMessageHubHook)]
+
+# configure default hooks
+default_hooks = dict(
+ # record the time of every iteration.
+ timer=dict(type=IterTimerHook),
+ # print log every 10 iterations.
+ logger=dict(type=LoggerHook, log_metric_by_epoch=False, interval=10),
+ # enable the parameter scheduler.
+ param_scheduler=dict(type=ParamSchedulerHook),
+ # save checkpoint per `save_steps`.
+ checkpoint=dict(
+ type=CheckpointHook,
+ by_epoch=False,
+ interval=save_steps,
+ max_keep_ckpts=save_total_limit),
+ # set sampler seed in distributed evrionment.
+ sampler_seed=dict(type=DistSamplerSeedHook),
+)
+
+# configure environment
+env_cfg = dict(
+ # whether to enable cudnn benchmark
+ cudnn_benchmark=False,
+ # set multi process parameters
+ mp_cfg=dict(mp_start_method='fork', opencv_num_threads=0),
+ # set distributed parameters
+ dist_cfg=dict(backend='nccl'),
+)
+
+# set visualizer
+visualizer = None
+
+# set log level
+log_level = 'INFO'
+
+# load from which checkpoint
+load_from = None
+
+# whether to resume training from the loaded checkpoint
+resume = False
+
+# Defaults to use random seed and disable `deterministic`
+randomness = dict(seed=None, deterministic=False)
+
+# set log processor
+log_processor = dict(by_epoch=False)
diff --git a/xtuner/configs/orpo/llama/llama3_8b_instruct_orpo_qlora_varlenattn_ultrafeedback_e5.py b/xtuner/configs/orpo/llama/llama3_8b_instruct_orpo_qlora_varlenattn_ultrafeedback_e5.py
new file mode 100644
index 000000000..00608c621
--- /dev/null
+++ b/xtuner/configs/orpo/llama/llama3_8b_instruct_orpo_qlora_varlenattn_ultrafeedback_e5.py
@@ -0,0 +1,229 @@
+# Copyright (c) OpenMMLab. All rights reserved.
+import torch
+from datasets import load_dataset
+from mmengine.dataset import DefaultSampler
+from mmengine.hooks import (CheckpointHook, DistSamplerSeedHook, IterTimerHook,
+ LoggerHook, ParamSchedulerHook)
+from mmengine.optim import AmpOptimWrapper, CosineAnnealingLR, LinearLR
+from peft import LoraConfig
+from torch.optim import AdamW
+from transformers import (AutoModelForCausalLM, AutoTokenizer,
+ BitsAndBytesConfig)
+
+from xtuner.dataset.collate_fns.preference_collate_fn import \
+ preference_collate_fn
+from xtuner.dataset.preference_dataset import (build_preference_dataset,
+ orpo_dpo_mix_40k_map_fn)
+from xtuner.engine.hooks import (EvaluateChatHook,
+ VarlenAttnArgsToMessageHubHook)
+from xtuner.engine.runner import TrainLoop
+from xtuner.model.orpo import ORPO
+from xtuner.parallel.sequence import SequenceParallelSampler
+from xtuner.utils import PROMPT_TEMPLATE, SYSTEM_TEMPLATE
+
+#######################################################################
+# PART 1 Settings #
+#######################################################################
+# Model
+pretrained_model_name_or_path = 'meta-llama/Meta-Llama-3-8B-Instruct'
+use_varlen_attn = True
+loss_beta = 0.1
+
+# Data
+prompt_template = PROMPT_TEMPLATE.llama3_chat
+max_length = 2048
+max_packed_length = max_length * 2
+
+# parallel
+sequence_parallel_size = 1
+
+# Scheduler & Optimizer
+batch_size = 1 # per_device
+accumulative_counts = 16
+accumulative_counts *= sequence_parallel_size
+dataloader_num_workers = 0
+max_epochs = 5 # refer to orpo repo
+optim_type = AdamW
+lr = 5e-6
+betas = (0.9, 0.999)
+weight_decay = 0
+max_norm = 1 # grad clip
+warmup_ratio = 0.01
+
+# Save
+save_steps = 500
+save_total_limit = 2 # Maximum checkpoints to keep (-1 means unlimited)
+
+# Evaluate the generation performance during the training
+evaluation_freq = 500
+SYSTEM = SYSTEM_TEMPLATE.alpaca
+evaluation_inputs = [
+ 'What famous British author, known for his tales of mystery and the macabre, shares his initials with a common abbreviation for "rest in peace"?', # noqa: E501
+ 'Please tell me five scenic spots in Shanghai',
+ '890729 - 425663? Only respond with math and no words.'
+]
+
+#######################################################################
+# PART 2 Model & Tokenizer #
+#######################################################################
+tokenizer = dict(
+ type=AutoTokenizer.from_pretrained,
+ pretrained_model_name_or_path=pretrained_model_name_or_path,
+ trust_remote_code=True,
+ padding_side='right')
+
+model = dict(
+ type=ORPO,
+ use_varlen_attn=use_varlen_attn,
+ beta=loss_beta,
+ llm=dict(
+ type=AutoModelForCausalLM.from_pretrained,
+ pretrained_model_name_or_path=pretrained_model_name_or_path,
+ trust_remote_code=True,
+ torch_dtype=torch.float16,
+ quantization_config=dict(
+ type=BitsAndBytesConfig,
+ load_in_4bit=True,
+ load_in_8bit=False,
+ llm_int8_threshold=6.0,
+ llm_int8_has_fp16_weight=False,
+ bnb_4bit_compute_dtype=torch.float16,
+ bnb_4bit_use_double_quant=True,
+ bnb_4bit_quant_type='nf4')),
+ lora=dict(
+ type=LoraConfig,
+ r=64,
+ lora_alpha=16,
+ lora_dropout=0.1,
+ bias='none',
+ task_type='CAUSAL_LM'))
+
+#######################################################################
+# PART 3 Dataset & Dataloader #
+#######################################################################
+sampler = SequenceParallelSampler \
+ if sequence_parallel_size > 1 else DefaultSampler
+
+train_dataset = dict(
+ type=build_preference_dataset,
+ dataset=dict(
+ type=load_dataset,
+ path='argilla/ultrafeedback-binarized-preferences-cleaned'),
+ tokenizer=tokenizer,
+ max_length=max_length,
+ dataset_map_fn=orpo_dpo_mix_40k_map_fn,
+ is_dpo=True,
+ is_reward=False,
+ reward_token_id=-1,
+ num_proc=32,
+ use_varlen_attn=use_varlen_attn,
+ max_packed_length=max_packed_length,
+ shuffle_before_pack=True,
+)
+
+train_dataloader = dict(
+ batch_size=batch_size,
+ num_workers=dataloader_num_workers,
+ dataset=train_dataset,
+ sampler=dict(type=sampler, shuffle=True),
+ collate_fn=dict(
+ type=preference_collate_fn, use_varlen_attn=use_varlen_attn))
+
+#######################################################################
+# PART 4 Scheduler & Optimizer #
+#######################################################################
+# optimizer
+optim_wrapper = dict(
+ type=AmpOptimWrapper,
+ optimizer=dict(
+ type=optim_type, lr=lr, betas=betas, weight_decay=weight_decay),
+ clip_grad=dict(max_norm=max_norm, error_if_nonfinite=False),
+ accumulative_counts=accumulative_counts,
+ loss_scale='dynamic',
+ dtype='float16')
+
+# learning policy
+# More information: https://github.com/open-mmlab/mmengine/blob/main/docs/en/tutorials/param_scheduler.md # noqa: E501
+param_scheduler = [
+ dict(
+ type=LinearLR,
+ start_factor=1e-5,
+ by_epoch=True,
+ begin=0,
+ end=warmup_ratio * max_epochs,
+ convert_to_iter_based=True),
+ dict(
+ type=CosineAnnealingLR,
+ eta_min=0.0,
+ by_epoch=True,
+ begin=warmup_ratio * max_epochs,
+ end=max_epochs,
+ convert_to_iter_based=True)
+]
+
+# train, val, test setting
+train_cfg = dict(type=TrainLoop, max_epochs=max_epochs)
+
+#######################################################################
+# PART 5 Runtime #
+#######################################################################
+# Log the dialogue periodically during the training process, optional
+custom_hooks = [
+ # dict(type=DatasetInfoHook, tokenizer=tokenizer),
+ dict(
+ type=EvaluateChatHook,
+ tokenizer=tokenizer,
+ every_n_iters=evaluation_freq,
+ evaluation_inputs=evaluation_inputs,
+ system=SYSTEM,
+ prompt_template=prompt_template)
+]
+
+if use_varlen_attn:
+ custom_hooks += [dict(type=VarlenAttnArgsToMessageHubHook)]
+
+# configure default hooks
+default_hooks = dict(
+ # record the time of every iteration.
+ timer=dict(type=IterTimerHook),
+ # print log every 10 iterations.
+ logger=dict(type=LoggerHook, log_metric_by_epoch=False, interval=10),
+ # enable the parameter scheduler.
+ param_scheduler=dict(type=ParamSchedulerHook),
+ # save checkpoint per `save_steps`.
+ checkpoint=dict(
+ type=CheckpointHook,
+ by_epoch=False,
+ interval=save_steps,
+ max_keep_ckpts=save_total_limit),
+ # set sampler seed in distributed evrionment.
+ sampler_seed=dict(type=DistSamplerSeedHook),
+)
+
+# configure environment
+env_cfg = dict(
+ # whether to enable cudnn benchmark
+ cudnn_benchmark=False,
+ # set multi process parameters
+ mp_cfg=dict(mp_start_method='fork', opencv_num_threads=0),
+ # set distributed parameters
+ dist_cfg=dict(backend='nccl'),
+)
+
+# set visualizer
+visualizer = None
+
+# set log level
+log_level = 'INFO'
+
+# load from which checkpoint
+load_from = None
+
+# whether to resume training from the loaded checkpoint
+resume = False
+
+# Defaults to use random seed and disable `deterministic`
+randomness = dict(seed=None, deterministic=False)
+
+# set log processor
+log_processor = dict(by_epoch=False)
diff --git a/xtuner/configs/phi/phi3/phi3_mini_128k_instruct_full_alpaca_e3.py b/xtuner/configs/phi/phi3/phi3_mini_128k_instruct_full_alpaca_e3.py
new file mode 100644
index 000000000..d60f67533
--- /dev/null
+++ b/xtuner/configs/phi/phi3/phi3_mini_128k_instruct_full_alpaca_e3.py
@@ -0,0 +1,199 @@
+# Copyright (c) OpenMMLab. All rights reserved.
+from datasets import load_dataset
+from mmengine.dataset import DefaultSampler
+from mmengine.hooks import (CheckpointHook, DistSamplerSeedHook, IterTimerHook,
+ LoggerHook, ParamSchedulerHook)
+from mmengine.optim import AmpOptimWrapper, CosineAnnealingLR, LinearLR
+from torch.optim import AdamW
+from transformers import AutoModelForCausalLM, AutoTokenizer
+
+from xtuner.dataset import process_hf_dataset
+from xtuner.dataset.collate_fns import default_collate_fn
+from xtuner.dataset.map_fns import alpaca_map_fn, template_map_fn_factory
+from xtuner.engine.hooks import (DatasetInfoHook, EvaluateChatHook,
+ VarlenAttnArgsToMessageHubHook)
+from xtuner.engine.runner import TrainLoop
+from xtuner.model import SupervisedFinetune
+from xtuner.parallel.sequence import SequenceParallelSampler
+from xtuner.utils import PROMPT_TEMPLATE, SYSTEM_TEMPLATE
+
+#######################################################################
+# PART 1 Settings #
+#######################################################################
+# Model
+pretrained_model_name_or_path = 'microsoft/Phi-3-mini-128k-instruct'
+use_varlen_attn = False
+
+# Data
+alpaca_en_path = 'tatsu-lab/alpaca'
+prompt_template = PROMPT_TEMPLATE.phi3_chat
+max_length = 128 * 1024
+pack_to_max_length = True
+
+# parallel
+sequence_parallel_size = 1
+
+# Scheduler & Optimizer
+batch_size = 1 # per_device
+accumulative_counts = 16
+accumulative_counts *= sequence_parallel_size
+dataloader_num_workers = 0
+max_epochs = 3
+optim_type = AdamW
+lr = 2e-5
+betas = (0.9, 0.999)
+weight_decay = 0
+max_norm = 1 # grad clip
+warmup_ratio = 0.03
+
+# Save
+save_steps = 500
+save_total_limit = 2 # Maximum checkpoints to keep (-1 means unlimited)
+
+# Evaluate the generation performance during the training
+evaluation_freq = 500
+SYSTEM = SYSTEM_TEMPLATE.alpaca
+evaluation_inputs = [
+ '请给我介绍五个上海的景点', 'Please tell me five scenic spots in Shanghai'
+]
+
+#######################################################################
+# PART 2 Model & Tokenizer #
+#######################################################################
+tokenizer = dict(
+ type=AutoTokenizer.from_pretrained,
+ pretrained_model_name_or_path=pretrained_model_name_or_path,
+ trust_remote_code=True,
+ padding_side='right')
+
+model = dict(
+ type=SupervisedFinetune,
+ use_varlen_attn=use_varlen_attn,
+ llm=dict(
+ type=AutoModelForCausalLM.from_pretrained,
+ pretrained_model_name_or_path=pretrained_model_name_or_path,
+ trust_remote_code=True))
+
+#######################################################################
+# PART 3 Dataset & Dataloader #
+#######################################################################
+alpaca_en = dict(
+ type=process_hf_dataset,
+ dataset=dict(type=load_dataset, path=alpaca_en_path),
+ tokenizer=tokenizer,
+ max_length=max_length,
+ dataset_map_fn=alpaca_map_fn,
+ template_map_fn=dict(
+ type=template_map_fn_factory, template=prompt_template),
+ remove_unused_columns=True,
+ shuffle_before_pack=True,
+ pack_to_max_length=pack_to_max_length,
+ use_varlen_attn=use_varlen_attn)
+
+sampler = SequenceParallelSampler \
+ if sequence_parallel_size > 1 else DefaultSampler
+train_dataloader = dict(
+ batch_size=batch_size,
+ num_workers=dataloader_num_workers,
+ dataset=alpaca_en,
+ sampler=dict(type=sampler, shuffle=True),
+ collate_fn=dict(type=default_collate_fn, use_varlen_attn=use_varlen_attn))
+
+#######################################################################
+# PART 4 Scheduler & Optimizer #
+#######################################################################
+# optimizer
+optim_wrapper = dict(
+ type=AmpOptimWrapper,
+ optimizer=dict(
+ type=optim_type, lr=lr, betas=betas, weight_decay=weight_decay),
+ clip_grad=dict(max_norm=max_norm, error_if_nonfinite=False),
+ accumulative_counts=accumulative_counts,
+ loss_scale='dynamic',
+ dtype='float16')
+
+# learning policy
+# More information: https://github.com/open-mmlab/mmengine/blob/main/docs/en/tutorials/param_scheduler.md # noqa: E501
+param_scheduler = [
+ dict(
+ type=LinearLR,
+ start_factor=1e-5,
+ by_epoch=True,
+ begin=0,
+ end=warmup_ratio * max_epochs,
+ convert_to_iter_based=True),
+ dict(
+ type=CosineAnnealingLR,
+ eta_min=0.0,
+ by_epoch=True,
+ begin=warmup_ratio * max_epochs,
+ end=max_epochs,
+ convert_to_iter_based=True)
+]
+
+# train, val, test setting
+train_cfg = dict(type=TrainLoop, max_epochs=max_epochs)
+
+#######################################################################
+# PART 5 Runtime #
+#######################################################################
+# Log the dialogue periodically during the training process, optional
+custom_hooks = [
+ dict(type=DatasetInfoHook, tokenizer=tokenizer),
+ dict(
+ type=EvaluateChatHook,
+ tokenizer=tokenizer,
+ every_n_iters=evaluation_freq,
+ evaluation_inputs=evaluation_inputs,
+ system=SYSTEM,
+ prompt_template=prompt_template)
+]
+
+if use_varlen_attn:
+ custom_hooks += [dict(type=VarlenAttnArgsToMessageHubHook)]
+
+# configure default hooks
+default_hooks = dict(
+ # record the time of every iteration.
+ timer=dict(type=IterTimerHook),
+ # print log every 10 iterations.
+ logger=dict(type=LoggerHook, log_metric_by_epoch=False, interval=10),
+ # enable the parameter scheduler.
+ param_scheduler=dict(type=ParamSchedulerHook),
+ # save checkpoint per `save_steps`.
+ checkpoint=dict(
+ type=CheckpointHook,
+ by_epoch=False,
+ interval=save_steps,
+ max_keep_ckpts=save_total_limit),
+ # set sampler seed in distributed evrionment.
+ sampler_seed=dict(type=DistSamplerSeedHook),
+)
+
+# configure environment
+env_cfg = dict(
+ # whether to enable cudnn benchmark
+ cudnn_benchmark=False,
+ # set multi process parameters
+ mp_cfg=dict(mp_start_method='fork', opencv_num_threads=0),
+ # set distributed parameters
+ dist_cfg=dict(backend='nccl'),
+)
+
+# set visualizer
+visualizer = None
+
+# set log level
+log_level = 'INFO'
+
+# load from which checkpoint
+load_from = None
+
+# whether to resume training from the loaded checkpoint
+resume = False
+
+# Defaults to use random seed and disable `deterministic`
+randomness = dict(seed=None, deterministic=False)
+
+# set log processor
+log_processor = dict(by_epoch=False)
diff --git a/xtuner/configs/phi/phi3/phi3_mini_128k_instruct_qlora_alpaca_e3.py b/xtuner/configs/phi/phi3/phi3_mini_128k_instruct_qlora_alpaca_e3.py
new file mode 100644
index 000000000..f528da716
--- /dev/null
+++ b/xtuner/configs/phi/phi3/phi3_mini_128k_instruct_qlora_alpaca_e3.py
@@ -0,0 +1,219 @@
+# Copyright (c) OpenMMLab. All rights reserved.
+import torch
+from datasets import load_dataset
+from mmengine.dataset import DefaultSampler
+from mmengine.hooks import (CheckpointHook, DistSamplerSeedHook, IterTimerHook,
+ LoggerHook, ParamSchedulerHook)
+from mmengine.optim import AmpOptimWrapper, CosineAnnealingLR, LinearLR
+from peft import LoraConfig
+from torch.optim import AdamW
+from transformers import (AutoModelForCausalLM, AutoTokenizer,
+ BitsAndBytesConfig)
+
+from xtuner.dataset import process_hf_dataset
+from xtuner.dataset.collate_fns import default_collate_fn
+from xtuner.dataset.map_fns import alpaca_map_fn, template_map_fn_factory
+from xtuner.engine.hooks import (DatasetInfoHook, EvaluateChatHook,
+ VarlenAttnArgsToMessageHubHook)
+from xtuner.engine.runner import TrainLoop
+from xtuner.model import SupervisedFinetune
+from xtuner.parallel.sequence import SequenceParallelSampler
+from xtuner.utils import PROMPT_TEMPLATE, SYSTEM_TEMPLATE
+
+#######################################################################
+# PART 1 Settings #
+#######################################################################
+# Model
+pretrained_model_name_or_path = 'microsoft/Phi-3-mini-128k-instruct'
+use_varlen_attn = False
+
+# Data
+alpaca_en_path = 'tatsu-lab/alpaca'
+prompt_template = PROMPT_TEMPLATE.phi3_chat
+max_length = 128 * 1024
+pack_to_max_length = True
+
+# parallel
+sequence_parallel_size = 1
+
+# Scheduler & Optimizer
+batch_size = 1 # per_device
+accumulative_counts = 16
+accumulative_counts *= sequence_parallel_size
+dataloader_num_workers = 0
+max_epochs = 3
+optim_type = AdamW
+lr = 2e-4
+betas = (0.9, 0.999)
+weight_decay = 0
+max_norm = 1 # grad clip
+warmup_ratio = 0.03
+
+# Save
+save_steps = 500
+save_total_limit = 2 # Maximum checkpoints to keep (-1 means unlimited)
+
+# Evaluate the generation performance during the training
+evaluation_freq = 500
+SYSTEM = SYSTEM_TEMPLATE.alpaca
+evaluation_inputs = [
+ '请给我介绍五个上海的景点', 'Please tell me five scenic spots in Shanghai'
+]
+
+#######################################################################
+# PART 2 Model & Tokenizer #
+#######################################################################
+tokenizer = dict(
+ type=AutoTokenizer.from_pretrained,
+ pretrained_model_name_or_path=pretrained_model_name_or_path,
+ trust_remote_code=True,
+ padding_side='right')
+
+model = dict(
+ type=SupervisedFinetune,
+ use_varlen_attn=use_varlen_attn,
+ llm=dict(
+ type=AutoModelForCausalLM.from_pretrained,
+ pretrained_model_name_or_path=pretrained_model_name_or_path,
+ trust_remote_code=True,
+ torch_dtype=torch.float16,
+ quantization_config=dict(
+ type=BitsAndBytesConfig,
+ load_in_4bit=True,
+ load_in_8bit=False,
+ llm_int8_threshold=6.0,
+ llm_int8_has_fp16_weight=False,
+ bnb_4bit_compute_dtype=torch.float16,
+ bnb_4bit_use_double_quant=True,
+ bnb_4bit_quant_type='nf4')),
+ lora=dict(
+ type=LoraConfig,
+ r=64,
+ lora_alpha=16,
+ lora_dropout=0.1,
+ bias='none',
+ task_type='CAUSAL_LM'))
+
+#######################################################################
+# PART 3 Dataset & Dataloader #
+#######################################################################
+alpaca_en = dict(
+ type=process_hf_dataset,
+ dataset=dict(type=load_dataset, path=alpaca_en_path),
+ tokenizer=tokenizer,
+ max_length=max_length,
+ dataset_map_fn=alpaca_map_fn,
+ template_map_fn=dict(
+ type=template_map_fn_factory, template=prompt_template),
+ remove_unused_columns=True,
+ shuffle_before_pack=True,
+ pack_to_max_length=pack_to_max_length,
+ use_varlen_attn=use_varlen_attn)
+
+sampler = SequenceParallelSampler \
+ if sequence_parallel_size > 1 else DefaultSampler
+train_dataloader = dict(
+ batch_size=batch_size,
+ num_workers=dataloader_num_workers,
+ dataset=alpaca_en,
+ sampler=dict(type=sampler, shuffle=True),
+ collate_fn=dict(type=default_collate_fn, use_varlen_attn=use_varlen_attn))
+
+#######################################################################
+# PART 4 Scheduler & Optimizer #
+#######################################################################
+# optimizer
+optim_wrapper = dict(
+ type=AmpOptimWrapper,
+ optimizer=dict(
+ type=optim_type, lr=lr, betas=betas, weight_decay=weight_decay),
+ clip_grad=dict(max_norm=max_norm, error_if_nonfinite=False),
+ accumulative_counts=accumulative_counts,
+ loss_scale='dynamic',
+ dtype='float16')
+
+# learning policy
+# More information: https://github.com/open-mmlab/mmengine/blob/main/docs/en/tutorials/param_scheduler.md # noqa: E501
+param_scheduler = [
+ dict(
+ type=LinearLR,
+ start_factor=1e-5,
+ by_epoch=True,
+ begin=0,
+ end=warmup_ratio * max_epochs,
+ convert_to_iter_based=True),
+ dict(
+ type=CosineAnnealingLR,
+ eta_min=0.0,
+ by_epoch=True,
+ begin=warmup_ratio * max_epochs,
+ end=max_epochs,
+ convert_to_iter_based=True)
+]
+
+# train, val, test setting
+train_cfg = dict(type=TrainLoop, max_epochs=max_epochs)
+
+#######################################################################
+# PART 5 Runtime #
+#######################################################################
+# Log the dialogue periodically during the training process, optional
+custom_hooks = [
+ dict(type=DatasetInfoHook, tokenizer=tokenizer),
+ dict(
+ type=EvaluateChatHook,
+ tokenizer=tokenizer,
+ every_n_iters=evaluation_freq,
+ evaluation_inputs=evaluation_inputs,
+ system=SYSTEM,
+ prompt_template=prompt_template)
+]
+
+if use_varlen_attn:
+ custom_hooks += [dict(type=VarlenAttnArgsToMessageHubHook)]
+
+# configure default hooks
+default_hooks = dict(
+ # record the time of every iteration.
+ timer=dict(type=IterTimerHook),
+ # print log every 10 iterations.
+ logger=dict(type=LoggerHook, log_metric_by_epoch=False, interval=10),
+ # enable the parameter scheduler.
+ param_scheduler=dict(type=ParamSchedulerHook),
+ # save checkpoint per `save_steps`.
+ checkpoint=dict(
+ type=CheckpointHook,
+ by_epoch=False,
+ interval=save_steps,
+ max_keep_ckpts=save_total_limit),
+ # set sampler seed in distributed evrionment.
+ sampler_seed=dict(type=DistSamplerSeedHook),
+)
+
+# configure environment
+env_cfg = dict(
+ # whether to enable cudnn benchmark
+ cudnn_benchmark=False,
+ # set multi process parameters
+ mp_cfg=dict(mp_start_method='fork', opencv_num_threads=0),
+ # set distributed parameters
+ dist_cfg=dict(backend='nccl'),
+)
+
+# set visualizer
+visualizer = None
+
+# set log level
+log_level = 'INFO'
+
+# load from which checkpoint
+load_from = None
+
+# whether to resume training from the loaded checkpoint
+resume = False
+
+# Defaults to use random seed and disable `deterministic`
+randomness = dict(seed=None, deterministic=False)
+
+# set log processor
+log_processor = dict(by_epoch=False)
diff --git a/xtuner/configs/phi/phi3/phi3_mini_4k_instruct_full_alpaca_e3.py b/xtuner/configs/phi/phi3/phi3_mini_4k_instruct_full_alpaca_e3.py
new file mode 100644
index 000000000..64f198d34
--- /dev/null
+++ b/xtuner/configs/phi/phi3/phi3_mini_4k_instruct_full_alpaca_e3.py
@@ -0,0 +1,199 @@
+# Copyright (c) OpenMMLab. All rights reserved.
+from datasets import load_dataset
+from mmengine.dataset import DefaultSampler
+from mmengine.hooks import (CheckpointHook, DistSamplerSeedHook, IterTimerHook,
+ LoggerHook, ParamSchedulerHook)
+from mmengine.optim import AmpOptimWrapper, CosineAnnealingLR, LinearLR
+from torch.optim import AdamW
+from transformers import AutoModelForCausalLM, AutoTokenizer
+
+from xtuner.dataset import process_hf_dataset
+from xtuner.dataset.collate_fns import default_collate_fn
+from xtuner.dataset.map_fns import alpaca_map_fn, template_map_fn_factory
+from xtuner.engine.hooks import (DatasetInfoHook, EvaluateChatHook,
+ VarlenAttnArgsToMessageHubHook)
+from xtuner.engine.runner import TrainLoop
+from xtuner.model import SupervisedFinetune
+from xtuner.parallel.sequence import SequenceParallelSampler
+from xtuner.utils import PROMPT_TEMPLATE, SYSTEM_TEMPLATE
+
+#######################################################################
+# PART 1 Settings #
+#######################################################################
+# Model
+pretrained_model_name_or_path = 'microsoft/Phi-3-mini-4k-instruct'
+use_varlen_attn = False
+
+# Data
+alpaca_en_path = 'tatsu-lab/alpaca'
+prompt_template = PROMPT_TEMPLATE.phi3_chat
+max_length = 4096
+pack_to_max_length = True
+
+# parallel
+sequence_parallel_size = 1
+
+# Scheduler & Optimizer
+batch_size = 1 # per_device
+accumulative_counts = 16
+accumulative_counts *= sequence_parallel_size
+dataloader_num_workers = 0
+max_epochs = 3
+optim_type = AdamW
+lr = 2e-5
+betas = (0.9, 0.999)
+weight_decay = 0
+max_norm = 1 # grad clip
+warmup_ratio = 0.03
+
+# Save
+save_steps = 500
+save_total_limit = 2 # Maximum checkpoints to keep (-1 means unlimited)
+
+# Evaluate the generation performance during the training
+evaluation_freq = 500
+SYSTEM = SYSTEM_TEMPLATE.alpaca
+evaluation_inputs = [
+ '请给我介绍五个上海的景点', 'Please tell me five scenic spots in Shanghai'
+]
+
+#######################################################################
+# PART 2 Model & Tokenizer #
+#######################################################################
+tokenizer = dict(
+ type=AutoTokenizer.from_pretrained,
+ pretrained_model_name_or_path=pretrained_model_name_or_path,
+ trust_remote_code=True,
+ padding_side='right')
+
+model = dict(
+ type=SupervisedFinetune,
+ use_varlen_attn=use_varlen_attn,
+ llm=dict(
+ type=AutoModelForCausalLM.from_pretrained,
+ pretrained_model_name_or_path=pretrained_model_name_or_path,
+ trust_remote_code=True))
+
+#######################################################################
+# PART 3 Dataset & Dataloader #
+#######################################################################
+alpaca_en = dict(
+ type=process_hf_dataset,
+ dataset=dict(type=load_dataset, path=alpaca_en_path),
+ tokenizer=tokenizer,
+ max_length=max_length,
+ dataset_map_fn=alpaca_map_fn,
+ template_map_fn=dict(
+ type=template_map_fn_factory, template=prompt_template),
+ remove_unused_columns=True,
+ shuffle_before_pack=True,
+ pack_to_max_length=pack_to_max_length,
+ use_varlen_attn=use_varlen_attn)
+
+sampler = SequenceParallelSampler \
+ if sequence_parallel_size > 1 else DefaultSampler
+train_dataloader = dict(
+ batch_size=batch_size,
+ num_workers=dataloader_num_workers,
+ dataset=alpaca_en,
+ sampler=dict(type=sampler, shuffle=True),
+ collate_fn=dict(type=default_collate_fn, use_varlen_attn=use_varlen_attn))
+
+#######################################################################
+# PART 4 Scheduler & Optimizer #
+#######################################################################
+# optimizer
+optim_wrapper = dict(
+ type=AmpOptimWrapper,
+ optimizer=dict(
+ type=optim_type, lr=lr, betas=betas, weight_decay=weight_decay),
+ clip_grad=dict(max_norm=max_norm, error_if_nonfinite=False),
+ accumulative_counts=accumulative_counts,
+ loss_scale='dynamic',
+ dtype='float16')
+
+# learning policy
+# More information: https://github.com/open-mmlab/mmengine/blob/main/docs/en/tutorials/param_scheduler.md # noqa: E501
+param_scheduler = [
+ dict(
+ type=LinearLR,
+ start_factor=1e-5,
+ by_epoch=True,
+ begin=0,
+ end=warmup_ratio * max_epochs,
+ convert_to_iter_based=True),
+ dict(
+ type=CosineAnnealingLR,
+ eta_min=0.0,
+ by_epoch=True,
+ begin=warmup_ratio * max_epochs,
+ end=max_epochs,
+ convert_to_iter_based=True)
+]
+
+# train, val, test setting
+train_cfg = dict(type=TrainLoop, max_epochs=max_epochs)
+
+#######################################################################
+# PART 5 Runtime #
+#######################################################################
+# Log the dialogue periodically during the training process, optional
+custom_hooks = [
+ dict(type=DatasetInfoHook, tokenizer=tokenizer),
+ dict(
+ type=EvaluateChatHook,
+ tokenizer=tokenizer,
+ every_n_iters=evaluation_freq,
+ evaluation_inputs=evaluation_inputs,
+ system=SYSTEM,
+ prompt_template=prompt_template)
+]
+
+if use_varlen_attn:
+ custom_hooks += [dict(type=VarlenAttnArgsToMessageHubHook)]
+
+# configure default hooks
+default_hooks = dict(
+ # record the time of every iteration.
+ timer=dict(type=IterTimerHook),
+ # print log every 10 iterations.
+ logger=dict(type=LoggerHook, log_metric_by_epoch=False, interval=10),
+ # enable the parameter scheduler.
+ param_scheduler=dict(type=ParamSchedulerHook),
+ # save checkpoint per `save_steps`.
+ checkpoint=dict(
+ type=CheckpointHook,
+ by_epoch=False,
+ interval=save_steps,
+ max_keep_ckpts=save_total_limit),
+ # set sampler seed in distributed evrionment.
+ sampler_seed=dict(type=DistSamplerSeedHook),
+)
+
+# configure environment
+env_cfg = dict(
+ # whether to enable cudnn benchmark
+ cudnn_benchmark=False,
+ # set multi process parameters
+ mp_cfg=dict(mp_start_method='fork', opencv_num_threads=0),
+ # set distributed parameters
+ dist_cfg=dict(backend='nccl'),
+)
+
+# set visualizer
+visualizer = None
+
+# set log level
+log_level = 'INFO'
+
+# load from which checkpoint
+load_from = None
+
+# whether to resume training from the loaded checkpoint
+resume = False
+
+# Defaults to use random seed and disable `deterministic`
+randomness = dict(seed=None, deterministic=False)
+
+# set log processor
+log_processor = dict(by_epoch=False)
diff --git a/xtuner/configs/phi/phi3/phi3_mini_4k_instruct_qlora_alpaca_e3.py b/xtuner/configs/phi/phi3/phi3_mini_4k_instruct_qlora_alpaca_e3.py
new file mode 100644
index 000000000..e90e17a14
--- /dev/null
+++ b/xtuner/configs/phi/phi3/phi3_mini_4k_instruct_qlora_alpaca_e3.py
@@ -0,0 +1,219 @@
+# Copyright (c) OpenMMLab. All rights reserved.
+import torch
+from datasets import load_dataset
+from mmengine.dataset import DefaultSampler
+from mmengine.hooks import (CheckpointHook, DistSamplerSeedHook, IterTimerHook,
+ LoggerHook, ParamSchedulerHook)
+from mmengine.optim import AmpOptimWrapper, CosineAnnealingLR, LinearLR
+from peft import LoraConfig
+from torch.optim import AdamW
+from transformers import (AutoModelForCausalLM, AutoTokenizer,
+ BitsAndBytesConfig)
+
+from xtuner.dataset import process_hf_dataset
+from xtuner.dataset.collate_fns import default_collate_fn
+from xtuner.dataset.map_fns import alpaca_map_fn, template_map_fn_factory
+from xtuner.engine.hooks import (DatasetInfoHook, EvaluateChatHook,
+ VarlenAttnArgsToMessageHubHook)
+from xtuner.engine.runner import TrainLoop
+from xtuner.model import SupervisedFinetune
+from xtuner.parallel.sequence import SequenceParallelSampler
+from xtuner.utils import PROMPT_TEMPLATE, SYSTEM_TEMPLATE
+
+#######################################################################
+# PART 1 Settings #
+#######################################################################
+# Model
+pretrained_model_name_or_path = 'microsoft/Phi-3-mini-4k-instruct'
+use_varlen_attn = False
+
+# Data
+alpaca_en_path = 'tatsu-lab/alpaca'
+prompt_template = PROMPT_TEMPLATE.phi3_chat
+max_length = 4096
+pack_to_max_length = True
+
+# parallel
+sequence_parallel_size = 1
+
+# Scheduler & Optimizer
+batch_size = 1 # per_device
+accumulative_counts = 16
+accumulative_counts *= sequence_parallel_size
+dataloader_num_workers = 0
+max_epochs = 3
+optim_type = AdamW
+lr = 2e-4
+betas = (0.9, 0.999)
+weight_decay = 0
+max_norm = 1 # grad clip
+warmup_ratio = 0.03
+
+# Save
+save_steps = 500
+save_total_limit = 2 # Maximum checkpoints to keep (-1 means unlimited)
+
+# Evaluate the generation performance during the training
+evaluation_freq = 500
+SYSTEM = SYSTEM_TEMPLATE.alpaca
+evaluation_inputs = [
+ '请给我介绍五个上海的景点', 'Please tell me five scenic spots in Shanghai'
+]
+
+#######################################################################
+# PART 2 Model & Tokenizer #
+#######################################################################
+tokenizer = dict(
+ type=AutoTokenizer.from_pretrained,
+ pretrained_model_name_or_path=pretrained_model_name_or_path,
+ trust_remote_code=True,
+ padding_side='right')
+
+model = dict(
+ type=SupervisedFinetune,
+ use_varlen_attn=use_varlen_attn,
+ llm=dict(
+ type=AutoModelForCausalLM.from_pretrained,
+ pretrained_model_name_or_path=pretrained_model_name_or_path,
+ trust_remote_code=True,
+ torch_dtype=torch.float16,
+ quantization_config=dict(
+ type=BitsAndBytesConfig,
+ load_in_4bit=True,
+ load_in_8bit=False,
+ llm_int8_threshold=6.0,
+ llm_int8_has_fp16_weight=False,
+ bnb_4bit_compute_dtype=torch.float16,
+ bnb_4bit_use_double_quant=True,
+ bnb_4bit_quant_type='nf4')),
+ lora=dict(
+ type=LoraConfig,
+ r=64,
+ lora_alpha=16,
+ lora_dropout=0.1,
+ bias='none',
+ task_type='CAUSAL_LM'))
+
+#######################################################################
+# PART 3 Dataset & Dataloader #
+#######################################################################
+alpaca_en = dict(
+ type=process_hf_dataset,
+ dataset=dict(type=load_dataset, path=alpaca_en_path),
+ tokenizer=tokenizer,
+ max_length=max_length,
+ dataset_map_fn=alpaca_map_fn,
+ template_map_fn=dict(
+ type=template_map_fn_factory, template=prompt_template),
+ remove_unused_columns=True,
+ shuffle_before_pack=True,
+ pack_to_max_length=pack_to_max_length,
+ use_varlen_attn=use_varlen_attn)
+
+sampler = SequenceParallelSampler \
+ if sequence_parallel_size > 1 else DefaultSampler
+train_dataloader = dict(
+ batch_size=batch_size,
+ num_workers=dataloader_num_workers,
+ dataset=alpaca_en,
+ sampler=dict(type=sampler, shuffle=True),
+ collate_fn=dict(type=default_collate_fn, use_varlen_attn=use_varlen_attn))
+
+#######################################################################
+# PART 4 Scheduler & Optimizer #
+#######################################################################
+# optimizer
+optim_wrapper = dict(
+ type=AmpOptimWrapper,
+ optimizer=dict(
+ type=optim_type, lr=lr, betas=betas, weight_decay=weight_decay),
+ clip_grad=dict(max_norm=max_norm, error_if_nonfinite=False),
+ accumulative_counts=accumulative_counts,
+ loss_scale='dynamic',
+ dtype='float16')
+
+# learning policy
+# More information: https://github.com/open-mmlab/mmengine/blob/main/docs/en/tutorials/param_scheduler.md # noqa: E501
+param_scheduler = [
+ dict(
+ type=LinearLR,
+ start_factor=1e-5,
+ by_epoch=True,
+ begin=0,
+ end=warmup_ratio * max_epochs,
+ convert_to_iter_based=True),
+ dict(
+ type=CosineAnnealingLR,
+ eta_min=0.0,
+ by_epoch=True,
+ begin=warmup_ratio * max_epochs,
+ end=max_epochs,
+ convert_to_iter_based=True)
+]
+
+# train, val, test setting
+train_cfg = dict(type=TrainLoop, max_epochs=max_epochs)
+
+#######################################################################
+# PART 5 Runtime #
+#######################################################################
+# Log the dialogue periodically during the training process, optional
+custom_hooks = [
+ dict(type=DatasetInfoHook, tokenizer=tokenizer),
+ dict(
+ type=EvaluateChatHook,
+ tokenizer=tokenizer,
+ every_n_iters=evaluation_freq,
+ evaluation_inputs=evaluation_inputs,
+ system=SYSTEM,
+ prompt_template=prompt_template)
+]
+
+if use_varlen_attn:
+ custom_hooks += [dict(type=VarlenAttnArgsToMessageHubHook)]
+
+# configure default hooks
+default_hooks = dict(
+ # record the time of every iteration.
+ timer=dict(type=IterTimerHook),
+ # print log every 10 iterations.
+ logger=dict(type=LoggerHook, log_metric_by_epoch=False, interval=10),
+ # enable the parameter scheduler.
+ param_scheduler=dict(type=ParamSchedulerHook),
+ # save checkpoint per `save_steps`.
+ checkpoint=dict(
+ type=CheckpointHook,
+ by_epoch=False,
+ interval=save_steps,
+ max_keep_ckpts=save_total_limit),
+ # set sampler seed in distributed evrionment.
+ sampler_seed=dict(type=DistSamplerSeedHook),
+)
+
+# configure environment
+env_cfg = dict(
+ # whether to enable cudnn benchmark
+ cudnn_benchmark=False,
+ # set multi process parameters
+ mp_cfg=dict(mp_start_method='fork', opencv_num_threads=0),
+ # set distributed parameters
+ dist_cfg=dict(backend='nccl'),
+)
+
+# set visualizer
+visualizer = None
+
+# set log level
+log_level = 'INFO'
+
+# load from which checkpoint
+load_from = None
+
+# whether to resume training from the loaded checkpoint
+resume = False
+
+# Defaults to use random seed and disable `deterministic`
+randomness = dict(seed=None, deterministic=False)
+
+# set log processor
+log_processor = dict(by_epoch=False)
diff --git a/xtuner/configs/qwen/qwen1/qwen_1_8b/qwen_1_8b_qlora_alpaca_e3.py b/xtuner/configs/qwen/qwen1/qwen_1_8b/qwen_1_8b_qlora_alpaca_e3.py
index 187e3f421..9245722b6 100644
--- a/xtuner/configs/qwen/qwen1/qwen_1_8b/qwen_1_8b_qlora_alpaca_e3.py
+++ b/xtuner/configs/qwen/qwen1/qwen_1_8b/qwen_1_8b_qlora_alpaca_e3.py
@@ -17,6 +17,7 @@
VarlenAttnArgsToMessageHubHook)
from xtuner.engine.runner import TrainLoop
from xtuner.model import SupervisedFinetune
+from xtuner.parallel.sequence import SequenceParallelSampler
from xtuner.utils import PROMPT_TEMPLATE, SYSTEM_TEMPLATE
#######################################################################
@@ -32,9 +33,13 @@
max_length = 2048
pack_to_max_length = True
+# parallel
+sequence_parallel_size = 1
+
# Scheduler & Optimizer
batch_size = 1 # per_device
accumulative_counts = 16
+accumulative_counts *= sequence_parallel_size
dataloader_num_workers = 0
max_epochs = 3
optim_type = AdamW
@@ -106,11 +111,14 @@
pack_to_max_length=pack_to_max_length,
use_varlen_attn=use_varlen_attn)
+sampler = SequenceParallelSampler \
+ if sequence_parallel_size > 1 else DefaultSampler
+
train_dataloader = dict(
batch_size=batch_size,
num_workers=dataloader_num_workers,
dataset=alpaca_en,
- sampler=dict(type=DefaultSampler, shuffle=True),
+ sampler=dict(type=sampler, shuffle=True),
collate_fn=dict(type=default_collate_fn, use_varlen_attn=use_varlen_attn))
#######################################################################
diff --git a/xtuner/configs/qwen/qwen1/qwen_1_8b/qwen_1_8b_qlora_alpaca_enzh_e3.py b/xtuner/configs/qwen/qwen1/qwen_1_8b/qwen_1_8b_qlora_alpaca_enzh_e3.py
index 6d9a3c564..88b822514 100644
--- a/xtuner/configs/qwen/qwen1/qwen_1_8b/qwen_1_8b_qlora_alpaca_enzh_e3.py
+++ b/xtuner/configs/qwen/qwen1/qwen_1_8b/qwen_1_8b_qlora_alpaca_enzh_e3.py
@@ -18,6 +18,7 @@
VarlenAttnArgsToMessageHubHook)
from xtuner.engine.runner import TrainLoop
from xtuner.model import SupervisedFinetune
+from xtuner.parallel.sequence import SequenceParallelSampler
from xtuner.utils import PROMPT_TEMPLATE, SYSTEM_TEMPLATE
#######################################################################
@@ -34,9 +35,13 @@
max_length = 2048
pack_to_max_length = True
+# parallel
+sequence_parallel_size = 1
+
# Scheduler & Optimizer
batch_size = 1 # per_device
accumulative_counts = 16
+accumulative_counts *= sequence_parallel_size
dataloader_num_workers = 0
max_epochs = 3
optim_type = AdamW
@@ -123,11 +128,14 @@
train_dataset = dict(type=ConcatDataset, datasets=[alpaca_en, alpaca_zh])
+sampler = SequenceParallelSampler \
+ if sequence_parallel_size > 1 else DefaultSampler
+
train_dataloader = dict(
batch_size=batch_size,
num_workers=dataloader_num_workers,
dataset=train_dataset,
- sampler=dict(type=DefaultSampler, shuffle=True),
+ sampler=dict(type=sampler, shuffle=True),
collate_fn=dict(type=default_collate_fn, use_varlen_attn=use_varlen_attn))
#######################################################################
diff --git a/xtuner/configs/qwen/qwen1/qwen_1_8b/qwen_1_8b_qlora_alpaca_enzh_oasst1_e3.py b/xtuner/configs/qwen/qwen1/qwen_1_8b/qwen_1_8b_qlora_alpaca_enzh_oasst1_e3.py
index 3ab872734..bce103128 100644
--- a/xtuner/configs/qwen/qwen1/qwen_1_8b/qwen_1_8b_qlora_alpaca_enzh_oasst1_e3.py
+++ b/xtuner/configs/qwen/qwen1/qwen_1_8b/qwen_1_8b_qlora_alpaca_enzh_oasst1_e3.py
@@ -18,6 +18,7 @@
VarlenAttnArgsToMessageHubHook)
from xtuner.engine.runner import TrainLoop
from xtuner.model import SupervisedFinetune
+from xtuner.parallel.sequence import SequenceParallelSampler
from xtuner.utils import PROMPT_TEMPLATE, SYSTEM_TEMPLATE
#######################################################################
@@ -35,9 +36,13 @@
max_length = 2048
pack_to_max_length = True
+# parallel
+sequence_parallel_size = 1
+
# Scheduler & Optimizer
batch_size = 1 # per_device
accumulative_counts = 16
+accumulative_counts *= sequence_parallel_size
dataloader_num_workers = 0
max_epochs = 3
optim_type = AdamW
@@ -138,11 +143,14 @@
train_dataset = dict(
type=ConcatDataset, datasets=[alpaca_en, alpaca_zh, oasst1])
+sampler = SequenceParallelSampler \
+ if sequence_parallel_size > 1 else DefaultSampler
+
train_dataloader = dict(
batch_size=batch_size,
num_workers=dataloader_num_workers,
dataset=train_dataset,
- sampler=dict(type=DefaultSampler, shuffle=True),
+ sampler=dict(type=sampler, shuffle=True),
collate_fn=dict(type=default_collate_fn, use_varlen_attn=use_varlen_attn))
#######################################################################
diff --git a/xtuner/configs/qwen/qwen1/qwen_1_8b/qwen_1_8b_qlora_alpaca_zh_e3.py b/xtuner/configs/qwen/qwen1/qwen_1_8b/qwen_1_8b_qlora_alpaca_zh_e3.py
index 13b005ccc..332cff37b 100644
--- a/xtuner/configs/qwen/qwen1/qwen_1_8b/qwen_1_8b_qlora_alpaca_zh_e3.py
+++ b/xtuner/configs/qwen/qwen1/qwen_1_8b/qwen_1_8b_qlora_alpaca_zh_e3.py
@@ -17,6 +17,7 @@
VarlenAttnArgsToMessageHubHook)
from xtuner.engine.runner import TrainLoop
from xtuner.model import SupervisedFinetune
+from xtuner.parallel.sequence import SequenceParallelSampler
from xtuner.utils import PROMPT_TEMPLATE, SYSTEM_TEMPLATE
#######################################################################
@@ -32,9 +33,13 @@
max_length = 2048
pack_to_max_length = True
+# parallel
+sequence_parallel_size = 1
+
# Scheduler & Optimizer
batch_size = 1 # per_device
accumulative_counts = 16
+accumulative_counts *= sequence_parallel_size
dataloader_num_workers = 0
max_epochs = 3
optim_type = AdamW
@@ -106,11 +111,14 @@
pack_to_max_length=pack_to_max_length,
use_varlen_attn=use_varlen_attn)
+sampler = SequenceParallelSampler \
+ if sequence_parallel_size > 1 else DefaultSampler
+
train_dataloader = dict(
batch_size=batch_size,
num_workers=dataloader_num_workers,
dataset=alpaca_zh,
- sampler=dict(type=DefaultSampler, shuffle=True),
+ sampler=dict(type=sampler, shuffle=True),
collate_fn=dict(type=default_collate_fn, use_varlen_attn=use_varlen_attn))
#######################################################################
diff --git a/xtuner/configs/qwen/qwen1/qwen_1_8b/qwen_1_8b_qlora_code_alpaca_e3.py b/xtuner/configs/qwen/qwen1/qwen_1_8b/qwen_1_8b_qlora_code_alpaca_e3.py
index fd6fa3c42..d7c087735 100644
--- a/xtuner/configs/qwen/qwen1/qwen_1_8b/qwen_1_8b_qlora_code_alpaca_e3.py
+++ b/xtuner/configs/qwen/qwen1/qwen_1_8b/qwen_1_8b_qlora_code_alpaca_e3.py
@@ -17,6 +17,7 @@
VarlenAttnArgsToMessageHubHook)
from xtuner.engine.runner import TrainLoop
from xtuner.model import SupervisedFinetune
+from xtuner.parallel.sequence import SequenceParallelSampler
from xtuner.utils import PROMPT_TEMPLATE, SYSTEM_TEMPLATE
#######################################################################
@@ -32,9 +33,13 @@
max_length = 2048
pack_to_max_length = True
+# parallel
+sequence_parallel_size = 1
+
# Scheduler & Optimizer
batch_size = 1 # per_device
accumulative_counts = 16
+accumulative_counts *= sequence_parallel_size
dataloader_num_workers = 0
max_epochs = 3
optim_type = AdamW
@@ -110,11 +115,14 @@
pack_to_max_length=pack_to_max_length,
use_varlen_attn=use_varlen_attn)
+sampler = SequenceParallelSampler \
+ if sequence_parallel_size > 1 else DefaultSampler
+
train_dataloader = dict(
batch_size=batch_size,
num_workers=dataloader_num_workers,
dataset=train_dataset,
- sampler=dict(type=DefaultSampler, shuffle=True),
+ sampler=dict(type=sampler, shuffle=True),
collate_fn=dict(type=default_collate_fn, use_varlen_attn=use_varlen_attn))
#######################################################################
diff --git a/xtuner/configs/qwen/qwen1/qwen_1_8b_chat/qwen_1_8b_chat_qlora_alpaca_e3.py b/xtuner/configs/qwen/qwen1/qwen_1_8b_chat/qwen_1_8b_chat_qlora_alpaca_e3.py
index d039f8a54..24c0040fa 100644
--- a/xtuner/configs/qwen/qwen1/qwen_1_8b_chat/qwen_1_8b_chat_qlora_alpaca_e3.py
+++ b/xtuner/configs/qwen/qwen1/qwen_1_8b_chat/qwen_1_8b_chat_qlora_alpaca_e3.py
@@ -17,6 +17,7 @@
VarlenAttnArgsToMessageHubHook)
from xtuner.engine.runner import TrainLoop
from xtuner.model import SupervisedFinetune
+from xtuner.parallel.sequence import SequenceParallelSampler
from xtuner.utils import PROMPT_TEMPLATE, SYSTEM_TEMPLATE
#######################################################################
@@ -32,9 +33,13 @@
max_length = 2048
pack_to_max_length = True
+# parallel
+sequence_parallel_size = 1
+
# Scheduler & Optimizer
batch_size = 1 # per_device
accumulative_counts = 16
+accumulative_counts *= sequence_parallel_size
dataloader_num_workers = 0
max_epochs = 3
optim_type = AdamW
@@ -106,11 +111,14 @@
pack_to_max_length=pack_to_max_length,
use_varlen_attn=use_varlen_attn)
+sampler = SequenceParallelSampler \
+ if sequence_parallel_size > 1 else DefaultSampler
+
train_dataloader = dict(
batch_size=batch_size,
num_workers=dataloader_num_workers,
dataset=alpaca_en,
- sampler=dict(type=DefaultSampler, shuffle=True),
+ sampler=dict(type=sampler, shuffle=True),
collate_fn=dict(type=default_collate_fn, use_varlen_attn=use_varlen_attn))
#######################################################################
diff --git a/xtuner/configs/qwen/qwen1/qwen_1_8b_chat/qwen_1_8b_chat_qlora_alpaca_enzh_e3.py b/xtuner/configs/qwen/qwen1/qwen_1_8b_chat/qwen_1_8b_chat_qlora_alpaca_enzh_e3.py
index 682bc6cd2..366958d49 100644
--- a/xtuner/configs/qwen/qwen1/qwen_1_8b_chat/qwen_1_8b_chat_qlora_alpaca_enzh_e3.py
+++ b/xtuner/configs/qwen/qwen1/qwen_1_8b_chat/qwen_1_8b_chat_qlora_alpaca_enzh_e3.py
@@ -18,6 +18,7 @@
VarlenAttnArgsToMessageHubHook)
from xtuner.engine.runner import TrainLoop
from xtuner.model import SupervisedFinetune
+from xtuner.parallel.sequence import SequenceParallelSampler
from xtuner.utils import PROMPT_TEMPLATE, SYSTEM_TEMPLATE
#######################################################################
@@ -34,9 +35,13 @@
max_length = 2048
pack_to_max_length = True
+# parallel
+sequence_parallel_size = 1
+
# Scheduler & Optimizer
batch_size = 1 # per_device
accumulative_counts = 16
+accumulative_counts *= sequence_parallel_size
dataloader_num_workers = 0
max_epochs = 3
optim_type = AdamW
@@ -123,11 +128,14 @@
train_dataset = dict(type=ConcatDataset, datasets=[alpaca_en, alpaca_zh])
+sampler = SequenceParallelSampler \
+ if sequence_parallel_size > 1 else DefaultSampler
+
train_dataloader = dict(
batch_size=batch_size,
num_workers=dataloader_num_workers,
dataset=train_dataset,
- sampler=dict(type=DefaultSampler, shuffle=True),
+ sampler=dict(type=sampler, shuffle=True),
collate_fn=dict(type=default_collate_fn, use_varlen_attn=use_varlen_attn))
#######################################################################
diff --git a/xtuner/configs/qwen/qwen1/qwen_1_8b_chat/qwen_1_8b_chat_qlora_alpaca_enzh_oasst1_e3.py b/xtuner/configs/qwen/qwen1/qwen_1_8b_chat/qwen_1_8b_chat_qlora_alpaca_enzh_oasst1_e3.py
index 4a5924b34..60bdd3dca 100644
--- a/xtuner/configs/qwen/qwen1/qwen_1_8b_chat/qwen_1_8b_chat_qlora_alpaca_enzh_oasst1_e3.py
+++ b/xtuner/configs/qwen/qwen1/qwen_1_8b_chat/qwen_1_8b_chat_qlora_alpaca_enzh_oasst1_e3.py
@@ -18,6 +18,7 @@
VarlenAttnArgsToMessageHubHook)
from xtuner.engine.runner import TrainLoop
from xtuner.model import SupervisedFinetune
+from xtuner.parallel.sequence import SequenceParallelSampler
from xtuner.utils import PROMPT_TEMPLATE, SYSTEM_TEMPLATE
#######################################################################
@@ -35,9 +36,13 @@
max_length = 2048
pack_to_max_length = True
+# parallel
+sequence_parallel_size = 1
+
# Scheduler & Optimizer
batch_size = 1 # per_device
accumulative_counts = 16
+accumulative_counts *= sequence_parallel_size
dataloader_num_workers = 0
max_epochs = 3
optim_type = AdamW
@@ -138,11 +143,14 @@
train_dataset = dict(
type=ConcatDataset, datasets=[alpaca_en, alpaca_zh, oasst1])
+sampler = SequenceParallelSampler \
+ if sequence_parallel_size > 1 else DefaultSampler
+
train_dataloader = dict(
batch_size=batch_size,
num_workers=dataloader_num_workers,
dataset=train_dataset,
- sampler=dict(type=DefaultSampler, shuffle=True),
+ sampler=dict(type=sampler, shuffle=True),
collate_fn=dict(type=default_collate_fn, use_varlen_attn=use_varlen_attn))
#######################################################################
diff --git a/xtuner/configs/qwen/qwen1/qwen_1_8b_chat/qwen_1_8b_chat_qlora_alpaca_zh_e3.py b/xtuner/configs/qwen/qwen1/qwen_1_8b_chat/qwen_1_8b_chat_qlora_alpaca_zh_e3.py
index dd00de5ca..058e200ee 100644
--- a/xtuner/configs/qwen/qwen1/qwen_1_8b_chat/qwen_1_8b_chat_qlora_alpaca_zh_e3.py
+++ b/xtuner/configs/qwen/qwen1/qwen_1_8b_chat/qwen_1_8b_chat_qlora_alpaca_zh_e3.py
@@ -17,6 +17,7 @@
VarlenAttnArgsToMessageHubHook)
from xtuner.engine.runner import TrainLoop
from xtuner.model import SupervisedFinetune
+from xtuner.parallel.sequence import SequenceParallelSampler
from xtuner.utils import PROMPT_TEMPLATE, SYSTEM_TEMPLATE
#######################################################################
@@ -32,9 +33,13 @@
max_length = 2048
pack_to_max_length = True
+# parallel
+sequence_parallel_size = 1
+
# Scheduler & Optimizer
batch_size = 1 # per_device
accumulative_counts = 16
+accumulative_counts *= sequence_parallel_size
dataloader_num_workers = 0
max_epochs = 3
optim_type = AdamW
@@ -106,11 +111,14 @@
pack_to_max_length=pack_to_max_length,
use_varlen_attn=use_varlen_attn)
+sampler = SequenceParallelSampler \
+ if sequence_parallel_size > 1 else DefaultSampler
+
train_dataloader = dict(
batch_size=batch_size,
num_workers=dataloader_num_workers,
dataset=alpaca_zh,
- sampler=dict(type=DefaultSampler, shuffle=True),
+ sampler=dict(type=sampler, shuffle=True),
collate_fn=dict(type=default_collate_fn, use_varlen_attn=use_varlen_attn))
#######################################################################
diff --git a/xtuner/configs/qwen/qwen1/qwen_1_8b_chat/qwen_1_8b_chat_qlora_code_alpaca_e3.py b/xtuner/configs/qwen/qwen1/qwen_1_8b_chat/qwen_1_8b_chat_qlora_code_alpaca_e3.py
index 92affd0b6..c50519930 100644
--- a/xtuner/configs/qwen/qwen1/qwen_1_8b_chat/qwen_1_8b_chat_qlora_code_alpaca_e3.py
+++ b/xtuner/configs/qwen/qwen1/qwen_1_8b_chat/qwen_1_8b_chat_qlora_code_alpaca_e3.py
@@ -17,6 +17,7 @@
VarlenAttnArgsToMessageHubHook)
from xtuner.engine.runner import TrainLoop
from xtuner.model import SupervisedFinetune
+from xtuner.parallel.sequence import SequenceParallelSampler
from xtuner.utils import PROMPT_TEMPLATE, SYSTEM_TEMPLATE
#######################################################################
@@ -32,9 +33,13 @@
max_length = 2048
pack_to_max_length = True
+# parallel
+sequence_parallel_size = 1
+
# Scheduler & Optimizer
batch_size = 1 # per_device
accumulative_counts = 16
+accumulative_counts *= sequence_parallel_size
dataloader_num_workers = 0
max_epochs = 3
optim_type = AdamW
@@ -110,11 +115,14 @@
pack_to_max_length=pack_to_max_length,
use_varlen_attn=use_varlen_attn)
+sampler = SequenceParallelSampler \
+ if sequence_parallel_size > 1 else DefaultSampler
+
train_dataloader = dict(
batch_size=batch_size,
num_workers=dataloader_num_workers,
dataset=train_dataset,
- sampler=dict(type=DefaultSampler, shuffle=True),
+ sampler=dict(type=sampler, shuffle=True),
collate_fn=dict(type=default_collate_fn, use_varlen_attn=use_varlen_attn))
#######################################################################
diff --git a/xtuner/configs/qwen/qwen1/qwen_72b/qwen_72b_qlora_alpaca_e3.py b/xtuner/configs/qwen/qwen1/qwen_72b/qwen_72b_qlora_alpaca_e3.py
index ece45c353..9f4d5ceb9 100644
--- a/xtuner/configs/qwen/qwen1/qwen_72b/qwen_72b_qlora_alpaca_e3.py
+++ b/xtuner/configs/qwen/qwen1/qwen_72b/qwen_72b_qlora_alpaca_e3.py
@@ -17,6 +17,7 @@
VarlenAttnArgsToMessageHubHook)
from xtuner.engine.runner import TrainLoop
from xtuner.model import SupervisedFinetune
+from xtuner.parallel.sequence import SequenceParallelSampler
from xtuner.utils import PROMPT_TEMPLATE, SYSTEM_TEMPLATE
#######################################################################
@@ -32,9 +33,13 @@
max_length = 2048
pack_to_max_length = True
+# parallel
+sequence_parallel_size = 1
+
# Scheduler & Optimizer
batch_size = 1 # per_device
accumulative_counts = 16
+accumulative_counts *= sequence_parallel_size
dataloader_num_workers = 0
max_epochs = 3
optim_type = AdamW
@@ -106,11 +111,14 @@
pack_to_max_length=pack_to_max_length,
use_varlen_attn=use_varlen_attn)
+sampler = SequenceParallelSampler \
+ if sequence_parallel_size > 1 else DefaultSampler
+
train_dataloader = dict(
batch_size=batch_size,
num_workers=dataloader_num_workers,
dataset=alpaca_en,
- sampler=dict(type=DefaultSampler, shuffle=True),
+ sampler=dict(type=sampler, shuffle=True),
collate_fn=dict(type=default_collate_fn, use_varlen_attn=use_varlen_attn))
#######################################################################
diff --git a/xtuner/configs/qwen/qwen1/qwen_72b/qwen_72b_qlora_alpaca_enzh_e3.py b/xtuner/configs/qwen/qwen1/qwen_72b/qwen_72b_qlora_alpaca_enzh_e3.py
index aa8306f2f..f985d04c4 100644
--- a/xtuner/configs/qwen/qwen1/qwen_72b/qwen_72b_qlora_alpaca_enzh_e3.py
+++ b/xtuner/configs/qwen/qwen1/qwen_72b/qwen_72b_qlora_alpaca_enzh_e3.py
@@ -18,6 +18,7 @@
VarlenAttnArgsToMessageHubHook)
from xtuner.engine.runner import TrainLoop
from xtuner.model import SupervisedFinetune
+from xtuner.parallel.sequence import SequenceParallelSampler
from xtuner.utils import PROMPT_TEMPLATE, SYSTEM_TEMPLATE
#######################################################################
@@ -34,9 +35,13 @@
max_length = 2048
pack_to_max_length = True
+# parallel
+sequence_parallel_size = 1
+
# Scheduler & Optimizer
batch_size = 1 # per_device
accumulative_counts = 16
+accumulative_counts *= sequence_parallel_size
dataloader_num_workers = 0
max_epochs = 3
optim_type = AdamW
@@ -123,11 +128,14 @@
train_dataset = dict(type=ConcatDataset, datasets=[alpaca_en, alpaca_zh])
+sampler = SequenceParallelSampler \
+ if sequence_parallel_size > 1 else DefaultSampler
+
train_dataloader = dict(
batch_size=batch_size,
num_workers=dataloader_num_workers,
dataset=train_dataset,
- sampler=dict(type=DefaultSampler, shuffle=True),
+ sampler=dict(type=sampler, shuffle=True),
collate_fn=dict(type=default_collate_fn, use_varlen_attn=use_varlen_attn))
#######################################################################
diff --git a/xtuner/configs/qwen/qwen1/qwen_72b/qwen_72b_qlora_alpaca_enzh_oasst1_e3.py b/xtuner/configs/qwen/qwen1/qwen_72b/qwen_72b_qlora_alpaca_enzh_oasst1_e3.py
index 5c5dbe391..2c5b951b0 100644
--- a/xtuner/configs/qwen/qwen1/qwen_72b/qwen_72b_qlora_alpaca_enzh_oasst1_e3.py
+++ b/xtuner/configs/qwen/qwen1/qwen_72b/qwen_72b_qlora_alpaca_enzh_oasst1_e3.py
@@ -18,6 +18,7 @@
VarlenAttnArgsToMessageHubHook)
from xtuner.engine.runner import TrainLoop
from xtuner.model import SupervisedFinetune
+from xtuner.parallel.sequence import SequenceParallelSampler
from xtuner.utils import PROMPT_TEMPLATE, SYSTEM_TEMPLATE
#######################################################################
@@ -35,9 +36,13 @@
max_length = 2048
pack_to_max_length = True
+# parallel
+sequence_parallel_size = 1
+
# Scheduler & Optimizer
batch_size = 1 # per_device
accumulative_counts = 16
+accumulative_counts *= sequence_parallel_size
dataloader_num_workers = 0
max_epochs = 3
optim_type = AdamW
@@ -138,11 +143,14 @@
train_dataset = dict(
type=ConcatDataset, datasets=[alpaca_en, alpaca_zh, oasst1])
+sampler = SequenceParallelSampler \
+ if sequence_parallel_size > 1 else DefaultSampler
+
train_dataloader = dict(
batch_size=batch_size,
num_workers=dataloader_num_workers,
dataset=train_dataset,
- sampler=dict(type=DefaultSampler, shuffle=True),
+ sampler=dict(type=sampler, shuffle=True),
collate_fn=dict(type=default_collate_fn, use_varlen_attn=use_varlen_attn))
#######################################################################
diff --git a/xtuner/configs/qwen/qwen1/qwen_72b/qwen_72b_qlora_alpaca_zh_e3.py b/xtuner/configs/qwen/qwen1/qwen_72b/qwen_72b_qlora_alpaca_zh_e3.py
index c235efe23..4c3f85eb4 100644
--- a/xtuner/configs/qwen/qwen1/qwen_72b/qwen_72b_qlora_alpaca_zh_e3.py
+++ b/xtuner/configs/qwen/qwen1/qwen_72b/qwen_72b_qlora_alpaca_zh_e3.py
@@ -17,6 +17,7 @@
VarlenAttnArgsToMessageHubHook)
from xtuner.engine.runner import TrainLoop
from xtuner.model import SupervisedFinetune
+from xtuner.parallel.sequence import SequenceParallelSampler
from xtuner.utils import PROMPT_TEMPLATE, SYSTEM_TEMPLATE
#######################################################################
@@ -32,9 +33,13 @@
max_length = 2048
pack_to_max_length = True
+# parallel
+sequence_parallel_size = 1
+
# Scheduler & Optimizer
batch_size = 1 # per_device
accumulative_counts = 16
+accumulative_counts *= sequence_parallel_size
dataloader_num_workers = 0
max_epochs = 3
optim_type = AdamW
@@ -106,11 +111,14 @@
pack_to_max_length=pack_to_max_length,
use_varlen_attn=use_varlen_attn)
+sampler = SequenceParallelSampler \
+ if sequence_parallel_size > 1 else DefaultSampler
+
train_dataloader = dict(
batch_size=batch_size,
num_workers=dataloader_num_workers,
dataset=alpaca_zh,
- sampler=dict(type=DefaultSampler, shuffle=True),
+ sampler=dict(type=sampler, shuffle=True),
collate_fn=dict(type=default_collate_fn, use_varlen_attn=use_varlen_attn))
#######################################################################
diff --git a/xtuner/configs/qwen/qwen1/qwen_72b/qwen_72b_qlora_code_alpaca_e3.py b/xtuner/configs/qwen/qwen1/qwen_72b/qwen_72b_qlora_code_alpaca_e3.py
index 40ead9c36..5cc74fe06 100644
--- a/xtuner/configs/qwen/qwen1/qwen_72b/qwen_72b_qlora_code_alpaca_e3.py
+++ b/xtuner/configs/qwen/qwen1/qwen_72b/qwen_72b_qlora_code_alpaca_e3.py
@@ -17,6 +17,7 @@
VarlenAttnArgsToMessageHubHook)
from xtuner.engine.runner import TrainLoop
from xtuner.model import SupervisedFinetune
+from xtuner.parallel.sequence import SequenceParallelSampler
from xtuner.utils import PROMPT_TEMPLATE, SYSTEM_TEMPLATE
#######################################################################
@@ -32,9 +33,13 @@
max_length = 2048
pack_to_max_length = True
+# parallel
+sequence_parallel_size = 1
+
# Scheduler & Optimizer
batch_size = 1 # per_device
accumulative_counts = 16
+accumulative_counts *= sequence_parallel_size
dataloader_num_workers = 0
max_epochs = 3
optim_type = AdamW
@@ -110,11 +115,14 @@
pack_to_max_length=pack_to_max_length,
use_varlen_attn=use_varlen_attn)
+sampler = SequenceParallelSampler \
+ if sequence_parallel_size > 1 else DefaultSampler
+
train_dataloader = dict(
batch_size=batch_size,
num_workers=dataloader_num_workers,
dataset=train_dataset,
- sampler=dict(type=DefaultSampler, shuffle=True),
+ sampler=dict(type=sampler, shuffle=True),
collate_fn=dict(type=default_collate_fn, use_varlen_attn=use_varlen_attn))
#######################################################################
diff --git a/xtuner/configs/qwen/qwen1/qwen_7b/qwen_7b_qlora_alpaca_e3.py b/xtuner/configs/qwen/qwen1/qwen_7b/qwen_7b_qlora_alpaca_e3.py
index a7e8f5bf3..c2e267f0c 100644
--- a/xtuner/configs/qwen/qwen1/qwen_7b/qwen_7b_qlora_alpaca_e3.py
+++ b/xtuner/configs/qwen/qwen1/qwen_7b/qwen_7b_qlora_alpaca_e3.py
@@ -17,6 +17,7 @@
VarlenAttnArgsToMessageHubHook)
from xtuner.engine.runner import TrainLoop
from xtuner.model import SupervisedFinetune
+from xtuner.parallel.sequence import SequenceParallelSampler
from xtuner.utils import PROMPT_TEMPLATE, SYSTEM_TEMPLATE
#######################################################################
@@ -32,9 +33,13 @@
max_length = 2048
pack_to_max_length = True
+# parallel
+sequence_parallel_size = 1
+
# Scheduler & Optimizer
batch_size = 1 # per_device
accumulative_counts = 16
+accumulative_counts *= sequence_parallel_size
dataloader_num_workers = 0
max_epochs = 3
optim_type = AdamW
@@ -106,11 +111,14 @@
pack_to_max_length=pack_to_max_length,
use_varlen_attn=use_varlen_attn)
+sampler = SequenceParallelSampler \
+ if sequence_parallel_size > 1 else DefaultSampler
+
train_dataloader = dict(
batch_size=batch_size,
num_workers=dataloader_num_workers,
dataset=alpaca_en,
- sampler=dict(type=DefaultSampler, shuffle=True),
+ sampler=dict(type=sampler, shuffle=True),
collate_fn=dict(type=default_collate_fn, use_varlen_attn=use_varlen_attn))
#######################################################################
diff --git a/xtuner/configs/qwen/qwen1/qwen_7b/qwen_7b_qlora_alpaca_enzh_e3.py b/xtuner/configs/qwen/qwen1/qwen_7b/qwen_7b_qlora_alpaca_enzh_e3.py
index 1bb75341d..77af4d903 100644
--- a/xtuner/configs/qwen/qwen1/qwen_7b/qwen_7b_qlora_alpaca_enzh_e3.py
+++ b/xtuner/configs/qwen/qwen1/qwen_7b/qwen_7b_qlora_alpaca_enzh_e3.py
@@ -18,6 +18,7 @@
VarlenAttnArgsToMessageHubHook)
from xtuner.engine.runner import TrainLoop
from xtuner.model import SupervisedFinetune
+from xtuner.parallel.sequence import SequenceParallelSampler
from xtuner.utils import PROMPT_TEMPLATE, SYSTEM_TEMPLATE
#######################################################################
@@ -34,9 +35,13 @@
max_length = 2048
pack_to_max_length = True
+# parallel
+sequence_parallel_size = 1
+
# Scheduler & Optimizer
batch_size = 1 # per_device
accumulative_counts = 16
+accumulative_counts *= sequence_parallel_size
dataloader_num_workers = 0
max_epochs = 3
optim_type = AdamW
@@ -123,11 +128,14 @@
train_dataset = dict(type=ConcatDataset, datasets=[alpaca_en, alpaca_zh])
+sampler = SequenceParallelSampler \
+ if sequence_parallel_size > 1 else DefaultSampler
+
train_dataloader = dict(
batch_size=batch_size,
num_workers=dataloader_num_workers,
dataset=train_dataset,
- sampler=dict(type=DefaultSampler, shuffle=True),
+ sampler=dict(type=sampler, shuffle=True),
collate_fn=dict(type=default_collate_fn, use_varlen_attn=use_varlen_attn))
#######################################################################
diff --git a/xtuner/configs/qwen/qwen1/qwen_7b/qwen_7b_qlora_alpaca_enzh_oasst1_e3.py b/xtuner/configs/qwen/qwen1/qwen_7b/qwen_7b_qlora_alpaca_enzh_oasst1_e3.py
index b970590b8..9a84fa1bf 100644
--- a/xtuner/configs/qwen/qwen1/qwen_7b/qwen_7b_qlora_alpaca_enzh_oasst1_e3.py
+++ b/xtuner/configs/qwen/qwen1/qwen_7b/qwen_7b_qlora_alpaca_enzh_oasst1_e3.py
@@ -18,6 +18,7 @@
VarlenAttnArgsToMessageHubHook)
from xtuner.engine.runner import TrainLoop
from xtuner.model import SupervisedFinetune
+from xtuner.parallel.sequence import SequenceParallelSampler
from xtuner.utils import PROMPT_TEMPLATE, SYSTEM_TEMPLATE
#######################################################################
@@ -35,9 +36,13 @@
max_length = 2048
pack_to_max_length = True
+# parallel
+sequence_parallel_size = 1
+
# Scheduler & Optimizer
batch_size = 1 # per_device
accumulative_counts = 16
+accumulative_counts *= sequence_parallel_size
dataloader_num_workers = 0
max_epochs = 3
optim_type = AdamW
@@ -138,11 +143,14 @@
train_dataset = dict(
type=ConcatDataset, datasets=[alpaca_en, alpaca_zh, oasst1])
+sampler = SequenceParallelSampler \
+ if sequence_parallel_size > 1 else DefaultSampler
+
train_dataloader = dict(
batch_size=batch_size,
num_workers=dataloader_num_workers,
dataset=train_dataset,
- sampler=dict(type=DefaultSampler, shuffle=True),
+ sampler=dict(type=sampler, shuffle=True),
collate_fn=dict(type=default_collate_fn, use_varlen_attn=use_varlen_attn))
#######################################################################
diff --git a/xtuner/configs/qwen/qwen1/qwen_7b/qwen_7b_qlora_alpaca_zh_e3.py b/xtuner/configs/qwen/qwen1/qwen_7b/qwen_7b_qlora_alpaca_zh_e3.py
index 44629c921..e4967ac51 100644
--- a/xtuner/configs/qwen/qwen1/qwen_7b/qwen_7b_qlora_alpaca_zh_e3.py
+++ b/xtuner/configs/qwen/qwen1/qwen_7b/qwen_7b_qlora_alpaca_zh_e3.py
@@ -17,6 +17,7 @@
VarlenAttnArgsToMessageHubHook)
from xtuner.engine.runner import TrainLoop
from xtuner.model import SupervisedFinetune
+from xtuner.parallel.sequence import SequenceParallelSampler
from xtuner.utils import PROMPT_TEMPLATE, SYSTEM_TEMPLATE
#######################################################################
@@ -32,9 +33,13 @@
max_length = 2048
pack_to_max_length = True
+# parallel
+sequence_parallel_size = 1
+
# Scheduler & Optimizer
batch_size = 1 # per_device
accumulative_counts = 16
+accumulative_counts *= sequence_parallel_size
dataloader_num_workers = 0
max_epochs = 3
optim_type = AdamW
@@ -106,11 +111,14 @@
pack_to_max_length=pack_to_max_length,
use_varlen_attn=use_varlen_attn)
+sampler = SequenceParallelSampler \
+ if sequence_parallel_size > 1 else DefaultSampler
+
train_dataloader = dict(
batch_size=batch_size,
num_workers=dataloader_num_workers,
dataset=alpaca_zh,
- sampler=dict(type=DefaultSampler, shuffle=True),
+ sampler=dict(type=sampler, shuffle=True),
collate_fn=dict(type=default_collate_fn, use_varlen_attn=use_varlen_attn))
#######################################################################
diff --git a/xtuner/configs/qwen/qwen1/qwen_7b/qwen_7b_qlora_arxiv_gentitle_e3.py b/xtuner/configs/qwen/qwen1/qwen_7b/qwen_7b_qlora_arxiv_gentitle_e3.py
index 5449bbed6..256a2dfc3 100644
--- a/xtuner/configs/qwen/qwen1/qwen_7b/qwen_7b_qlora_arxiv_gentitle_e3.py
+++ b/xtuner/configs/qwen/qwen1/qwen_7b/qwen_7b_qlora_arxiv_gentitle_e3.py
@@ -17,6 +17,7 @@
VarlenAttnArgsToMessageHubHook)
from xtuner.engine.runner import TrainLoop
from xtuner.model import SupervisedFinetune
+from xtuner.parallel.sequence import SequenceParallelSampler
from xtuner.utils import PROMPT_TEMPLATE, SYSTEM_TEMPLATE
#######################################################################
@@ -34,9 +35,13 @@
max_length = 2048
pack_to_max_length = True
+# parallel
+sequence_parallel_size = 1
+
# Scheduler & Optimizer
batch_size = 1 # per_device
accumulative_counts = 16
+accumulative_counts *= sequence_parallel_size
dataloader_num_workers = 0
max_epochs = 3
optim_type = AdamW
@@ -141,11 +146,14 @@
pack_to_max_length=pack_to_max_length,
use_varlen_attn=use_varlen_attn)
+sampler = SequenceParallelSampler \
+ if sequence_parallel_size > 1 else DefaultSampler
+
train_dataloader = dict(
batch_size=batch_size,
num_workers=dataloader_num_workers,
dataset=train_dataset,
- sampler=dict(type=DefaultSampler, shuffle=True),
+ sampler=dict(type=sampler, shuffle=True),
collate_fn=dict(type=default_collate_fn, use_varlen_attn=use_varlen_attn))
#######################################################################
diff --git a/xtuner/configs/qwen/qwen1/qwen_7b/qwen_7b_qlora_code_alpaca_e3.py b/xtuner/configs/qwen/qwen1/qwen_7b/qwen_7b_qlora_code_alpaca_e3.py
index 9c028fe8a..853cd63bc 100644
--- a/xtuner/configs/qwen/qwen1/qwen_7b/qwen_7b_qlora_code_alpaca_e3.py
+++ b/xtuner/configs/qwen/qwen1/qwen_7b/qwen_7b_qlora_code_alpaca_e3.py
@@ -17,6 +17,7 @@
VarlenAttnArgsToMessageHubHook)
from xtuner.engine.runner import TrainLoop
from xtuner.model import SupervisedFinetune
+from xtuner.parallel.sequence import SequenceParallelSampler
from xtuner.utils import PROMPT_TEMPLATE, SYSTEM_TEMPLATE
#######################################################################
@@ -32,9 +33,13 @@
max_length = 2048
pack_to_max_length = True
+# parallel
+sequence_parallel_size = 1
+
# Scheduler & Optimizer
batch_size = 1 # per_device
accumulative_counts = 16
+accumulative_counts *= sequence_parallel_size
dataloader_num_workers = 0
max_epochs = 3
optim_type = AdamW
@@ -110,11 +115,14 @@
pack_to_max_length=pack_to_max_length,
use_varlen_attn=use_varlen_attn)
+sampler = SequenceParallelSampler \
+ if sequence_parallel_size > 1 else DefaultSampler
+
train_dataloader = dict(
batch_size=batch_size,
num_workers=dataloader_num_workers,
dataset=train_dataset,
- sampler=dict(type=DefaultSampler, shuffle=True),
+ sampler=dict(type=sampler, shuffle=True),
collate_fn=dict(type=default_collate_fn, use_varlen_attn=use_varlen_attn))
#######################################################################
diff --git a/xtuner/configs/qwen/qwen1/qwen_7b/qwen_7b_qlora_colorist_e5.py b/xtuner/configs/qwen/qwen1/qwen_7b/qwen_7b_qlora_colorist_e5.py
index bdab0575b..631441e76 100644
--- a/xtuner/configs/qwen/qwen1/qwen_7b/qwen_7b_qlora_colorist_e5.py
+++ b/xtuner/configs/qwen/qwen1/qwen_7b/qwen_7b_qlora_colorist_e5.py
@@ -17,6 +17,7 @@
VarlenAttnArgsToMessageHubHook)
from xtuner.engine.runner import TrainLoop
from xtuner.model import SupervisedFinetune
+from xtuner.parallel.sequence import SequenceParallelSampler
from xtuner.utils import PROMPT_TEMPLATE, SYSTEM_TEMPLATE
#######################################################################
@@ -32,9 +33,13 @@
max_length = 2048
pack_to_max_length = True
+# parallel
+sequence_parallel_size = 1
+
# Scheduler & Optimizer
batch_size = 1 # per_device
accumulative_counts = 16
+accumulative_counts *= sequence_parallel_size
dataloader_num_workers = 0
max_epochs = 5
optim_type = AdamW
@@ -106,11 +111,14 @@
pack_to_max_length=pack_to_max_length,
use_varlen_attn=use_varlen_attn)
+sampler = SequenceParallelSampler \
+ if sequence_parallel_size > 1 else DefaultSampler
+
train_dataloader = dict(
batch_size=batch_size,
num_workers=dataloader_num_workers,
dataset=train_dataset,
- sampler=dict(type=DefaultSampler, shuffle=True),
+ sampler=dict(type=sampler, shuffle=True),
collate_fn=dict(type=default_collate_fn, use_varlen_attn=use_varlen_attn))
#######################################################################
diff --git a/xtuner/configs/qwen/qwen1/qwen_7b/qwen_7b_qlora_lawyer_e3.py b/xtuner/configs/qwen/qwen1/qwen_7b/qwen_7b_qlora_lawyer_e3.py
index 4697bb8d2..9c1b64f84 100644
--- a/xtuner/configs/qwen/qwen1/qwen_7b/qwen_7b_qlora_lawyer_e3.py
+++ b/xtuner/configs/qwen/qwen1/qwen_7b/qwen_7b_qlora_lawyer_e3.py
@@ -19,6 +19,7 @@
VarlenAttnArgsToMessageHubHook)
from xtuner.engine.runner import TrainLoop
from xtuner.model import SupervisedFinetune
+from xtuner.parallel.sequence import SequenceParallelSampler
from xtuner.utils import PROMPT_TEMPLATE, SYSTEM_TEMPLATE
#######################################################################
@@ -36,9 +37,13 @@
max_length = 2048
pack_to_max_length = True
+# parallel
+sequence_parallel_size = 1
+
# Scheduler & Optimizer
batch_size = 1 # per_device
accumulative_counts = 16
+accumulative_counts *= sequence_parallel_size
dataloader_num_workers = 0
max_epochs = 3
optim_type = AdamW
@@ -127,6 +132,9 @@
pack_to_max_length=pack_to_max_length,
use_varlen_attn=use_varlen_attn)
+sampler = SequenceParallelSampler \
+ if sequence_parallel_size > 1 else DefaultSampler
+
train_dataset = dict(
type=ConcatDataset, datasets=[crime_kg_assitant, law_reference_data])
@@ -134,7 +142,7 @@
batch_size=batch_size,
num_workers=dataloader_num_workers,
dataset=train_dataset,
- sampler=dict(type=DefaultSampler, shuffle=True),
+ sampler=dict(type=sampler, shuffle=True),
collate_fn=dict(type=default_collate_fn, use_varlen_attn=use_varlen_attn))
#######################################################################
diff --git a/xtuner/configs/qwen/qwen1/qwen_7b/qwen_7b_qlora_medical_e1.py b/xtuner/configs/qwen/qwen1/qwen_7b/qwen_7b_qlora_medical_e1.py
index 4913069fa..c8b657d03 100644
--- a/xtuner/configs/qwen/qwen1/qwen_7b/qwen_7b_qlora_medical_e1.py
+++ b/xtuner/configs/qwen/qwen1/qwen_7b/qwen_7b_qlora_medical_e1.py
@@ -17,6 +17,7 @@
VarlenAttnArgsToMessageHubHook)
from xtuner.engine.runner import TrainLoop
from xtuner.model import SupervisedFinetune
+from xtuner.parallel.sequence import SequenceParallelSampler
from xtuner.utils import PROMPT_TEMPLATE, SYSTEM_TEMPLATE
#######################################################################
@@ -33,9 +34,13 @@
max_length = 2048
pack_to_max_length = True
+# parallel
+sequence_parallel_size = 1
+
# Scheduler & Optimizer
batch_size = 1 # per_device
accumulative_counts = 16
+accumulative_counts *= sequence_parallel_size
dataloader_num_workers = 0
max_epochs = 1
optim_type = AdamW
@@ -108,11 +113,14 @@
pack_to_max_length=pack_to_max_length,
use_varlen_attn=use_varlen_attn)
+sampler = SequenceParallelSampler \
+ if sequence_parallel_size > 1 else DefaultSampler
+
train_dataloader = dict(
batch_size=batch_size,
num_workers=dataloader_num_workers,
dataset=train_dataset,
- sampler=dict(type=DefaultSampler, shuffle=True),
+ sampler=dict(type=sampler, shuffle=True),
collate_fn=dict(type=default_collate_fn, use_varlen_attn=use_varlen_attn))
#######################################################################
diff --git a/xtuner/configs/qwen/qwen1/qwen_7b/qwen_7b_qlora_moss_sft_all_e1.py b/xtuner/configs/qwen/qwen1/qwen_7b/qwen_7b_qlora_moss_sft_all_e1.py
index aefd2124a..6ae00805c 100644
--- a/xtuner/configs/qwen/qwen1/qwen_7b/qwen_7b_qlora_moss_sft_all_e1.py
+++ b/xtuner/configs/qwen/qwen1/qwen_7b/qwen_7b_qlora_moss_sft_all_e1.py
@@ -15,6 +15,7 @@
VarlenAttnArgsToMessageHubHook)
from xtuner.engine.runner import TrainLoop
from xtuner.model import SupervisedFinetune
+from xtuner.parallel.sequence import SequenceParallelSampler
from xtuner.utils import PROMPT_TEMPLATE, SYSTEM_TEMPLATE
#######################################################################
@@ -31,9 +32,13 @@
moss_sft_plugins_path = './data/conversations_with_tools_with_inner_instruction_no_text2image_train_all_random_meta0.5_0.1_0.01_moss_0709.jsonl' # noqa: E501
max_length = 2048
+# parallel
+sequence_parallel_size = 1
+
# Scheduler & Optimizer
batch_size = 1 # per_device
accumulative_counts = 16
+accumulative_counts *= sequence_parallel_size
dataloader_num_workers = 0
max_epochs = 1
optim_type = AdamW
@@ -110,11 +115,14 @@
train_dataset = dict(
type=ConcatDataset, datasets=[moss_sft_no_plugins, moss_sft_plugins])
+sampler = SequenceParallelSampler \
+ if sequence_parallel_size > 1 else DefaultSampler
+
train_dataloader = dict(
batch_size=batch_size,
num_workers=dataloader_num_workers,
dataset=train_dataset,
- sampler=dict(type=DefaultSampler, shuffle=True),
+ sampler=dict(type=sampler, shuffle=True),
collate_fn=dict(type=default_collate_fn, use_varlen_attn=use_varlen_attn))
#######################################################################
diff --git a/xtuner/configs/qwen/qwen1/qwen_7b/qwen_7b_qlora_moss_sft_all_e2_gpu8.py b/xtuner/configs/qwen/qwen1/qwen_7b/qwen_7b_qlora_moss_sft_all_e2_gpu8.py
index b4ec27589..99cfdc985 100644
--- a/xtuner/configs/qwen/qwen1/qwen_7b/qwen_7b_qlora_moss_sft_all_e2_gpu8.py
+++ b/xtuner/configs/qwen/qwen1/qwen_7b/qwen_7b_qlora_moss_sft_all_e2_gpu8.py
@@ -15,6 +15,7 @@
VarlenAttnArgsToMessageHubHook)
from xtuner.engine.runner import TrainLoop
from xtuner.model import SupervisedFinetune
+from xtuner.parallel.sequence import SequenceParallelSampler
from xtuner.utils import PROMPT_TEMPLATE, SYSTEM_TEMPLATE
#######################################################################
@@ -31,6 +32,9 @@
moss_sft_plugins_path = './data/conversations_with_tools_with_inner_instruction_no_text2image_train_all_random_meta0.5_0.1_0.01_moss_0709.jsonl' # noqa: E501
max_length = 2048
+# parallel
+sequence_parallel_size = 1
+
# Scheduler & Optimizer
batch_size = 8 # per_device
accumulative_counts = 1
@@ -110,11 +114,14 @@
train_dataset = dict(
type=ConcatDataset, datasets=[moss_sft_no_plugins, moss_sft_plugins])
+sampler = SequenceParallelSampler \
+ if sequence_parallel_size > 1 else DefaultSampler
+
train_dataloader = dict(
batch_size=batch_size,
num_workers=dataloader_num_workers,
dataset=train_dataset,
- sampler=dict(type=DefaultSampler, shuffle=True),
+ sampler=dict(type=sampler, shuffle=True),
collate_fn=dict(type=default_collate_fn, use_varlen_attn=use_varlen_attn))
#######################################################################
diff --git a/xtuner/configs/qwen/qwen1/qwen_7b/qwen_7b_qlora_moss_sft_plugins_e1.py b/xtuner/configs/qwen/qwen1/qwen_7b/qwen_7b_qlora_moss_sft_plugins_e1.py
index d25a22b23..3f391dc33 100644
--- a/xtuner/configs/qwen/qwen1/qwen_7b/qwen_7b_qlora_moss_sft_plugins_e1.py
+++ b/xtuner/configs/qwen/qwen1/qwen_7b/qwen_7b_qlora_moss_sft_plugins_e1.py
@@ -15,6 +15,7 @@
VarlenAttnArgsToMessageHubHook)
from xtuner.engine.runner import TrainLoop
from xtuner.model import SupervisedFinetune
+from xtuner.parallel.sequence import SequenceParallelSampler
from xtuner.utils import PROMPT_TEMPLATE, SYSTEM_TEMPLATE
#######################################################################
@@ -30,9 +31,13 @@
moss_sft_plugins_path = './data/conversations_with_tools_with_inner_instruction_no_text2image_train_all_random_meta0.5_0.1_0.01_moss_0709.jsonl' # noqa: E501
max_length = 2048
+# parallel
+sequence_parallel_size = 1
+
# Scheduler & Optimizer
batch_size = 1 # per_device
accumulative_counts = 16
+accumulative_counts *= sequence_parallel_size
dataloader_num_workers = 0
max_epochs = 1
optim_type = AdamW
@@ -99,11 +104,14 @@
tokenizer=tokenizer,
max_length=max_length)
+sampler = SequenceParallelSampler \
+ if sequence_parallel_size > 1 else DefaultSampler
+
train_dataloader = dict(
batch_size=batch_size,
num_workers=dataloader_num_workers,
dataset=train_dataset,
- sampler=dict(type=DefaultSampler, shuffle=True),
+ sampler=dict(type=sampler, shuffle=True),
collate_fn=dict(type=default_collate_fn, use_varlen_attn=use_varlen_attn))
#######################################################################
diff --git a/xtuner/configs/qwen/qwen1/qwen_7b/qwen_7b_qlora_oasst1_512_e3.py b/xtuner/configs/qwen/qwen1/qwen_7b/qwen_7b_qlora_oasst1_512_e3.py
index e6d252e8b..ec7704e6f 100644
--- a/xtuner/configs/qwen/qwen1/qwen_7b/qwen_7b_qlora_oasst1_512_e3.py
+++ b/xtuner/configs/qwen/qwen1/qwen_7b/qwen_7b_qlora_oasst1_512_e3.py
@@ -17,6 +17,7 @@
VarlenAttnArgsToMessageHubHook)
from xtuner.engine.runner import TrainLoop
from xtuner.model import SupervisedFinetune
+from xtuner.parallel.sequence import SequenceParallelSampler
from xtuner.utils import PROMPT_TEMPLATE
#######################################################################
@@ -32,9 +33,13 @@
max_length = 512
pack_to_max_length = False
+# parallel
+sequence_parallel_size = 1
+
# Scheduler & Optimizer
batch_size = 1 # per_device
accumulative_counts = 16
+accumulative_counts *= sequence_parallel_size
dataloader_num_workers = 0
max_epochs = 3
optim_type = AdamW
@@ -106,11 +111,14 @@
pack_to_max_length=pack_to_max_length,
use_varlen_attn=use_varlen_attn)
+sampler = SequenceParallelSampler \
+ if sequence_parallel_size > 1 else DefaultSampler
+
train_dataloader = dict(
batch_size=batch_size,
num_workers=dataloader_num_workers,
dataset=train_dataset,
- sampler=dict(type=DefaultSampler, shuffle=True),
+ sampler=dict(type=sampler, shuffle=True),
collate_fn=dict(type=default_collate_fn, use_varlen_attn=use_varlen_attn))
#######################################################################
diff --git a/xtuner/configs/qwen/qwen1/qwen_7b/qwen_7b_qlora_oasst1_e3.py b/xtuner/configs/qwen/qwen1/qwen_7b/qwen_7b_qlora_oasst1_e3.py
index c44eae661..080e4cfc9 100644
--- a/xtuner/configs/qwen/qwen1/qwen_7b/qwen_7b_qlora_oasst1_e3.py
+++ b/xtuner/configs/qwen/qwen1/qwen_7b/qwen_7b_qlora_oasst1_e3.py
@@ -17,6 +17,7 @@
VarlenAttnArgsToMessageHubHook)
from xtuner.engine.runner import TrainLoop
from xtuner.model import SupervisedFinetune
+from xtuner.parallel.sequence import SequenceParallelSampler
from xtuner.utils import PROMPT_TEMPLATE
#######################################################################
@@ -32,9 +33,13 @@
max_length = 2048
pack_to_max_length = True
+# parallel
+sequence_parallel_size = 1
+
# Scheduler & Optimizer
batch_size = 1 # per_device
accumulative_counts = 16
+accumulative_counts *= sequence_parallel_size
dataloader_num_workers = 0
max_epochs = 3
optim_type = AdamW
@@ -106,11 +111,14 @@
pack_to_max_length=pack_to_max_length,
use_varlen_attn=use_varlen_attn)
+sampler = SequenceParallelSampler \
+ if sequence_parallel_size > 1 else DefaultSampler
+
train_dataloader = dict(
batch_size=batch_size,
num_workers=dataloader_num_workers,
dataset=train_dataset,
- sampler=dict(type=DefaultSampler, shuffle=True),
+ sampler=dict(type=sampler, shuffle=True),
collate_fn=dict(type=default_collate_fn, use_varlen_attn=use_varlen_attn))
#######################################################################
diff --git a/xtuner/configs/qwen/qwen1/qwen_7b/qwen_7b_qlora_open_platypus_e3.py b/xtuner/configs/qwen/qwen1/qwen_7b/qwen_7b_qlora_open_platypus_e3.py
index 5d1a8f79f..bead03654 100644
--- a/xtuner/configs/qwen/qwen1/qwen_7b/qwen_7b_qlora_open_platypus_e3.py
+++ b/xtuner/configs/qwen/qwen1/qwen_7b/qwen_7b_qlora_open_platypus_e3.py
@@ -17,6 +17,7 @@
VarlenAttnArgsToMessageHubHook)
from xtuner.engine.runner import TrainLoop
from xtuner.model import SupervisedFinetune
+from xtuner.parallel.sequence import SequenceParallelSampler
from xtuner.utils import PROMPT_TEMPLATE, SYSTEM_TEMPLATE
#######################################################################
@@ -32,9 +33,13 @@
max_length = 2048
pack_to_max_length = True
+# parallel
+sequence_parallel_size = 1
+
# Scheduler & Optimizer
batch_size = 1 # per_device
accumulative_counts = 16
+accumulative_counts *= sequence_parallel_size
dataloader_num_workers = 0
max_epochs = 3
optim_type = AdamW
@@ -106,11 +111,14 @@
pack_to_max_length=pack_to_max_length,
use_varlen_attn=use_varlen_attn)
+sampler = SequenceParallelSampler \
+ if sequence_parallel_size > 1 else DefaultSampler
+
train_dataloader = dict(
batch_size=batch_size,
num_workers=dataloader_num_workers,
dataset=train_dataset,
- sampler=dict(type=DefaultSampler, shuffle=True),
+ sampler=dict(type=sampler, shuffle=True),
collate_fn=dict(type=default_collate_fn, use_varlen_attn=use_varlen_attn))
#######################################################################
diff --git a/xtuner/configs/qwen/qwen1/qwen_7b/qwen_7b_qlora_openorca_e1.py b/xtuner/configs/qwen/qwen1/qwen_7b/qwen_7b_qlora_openorca_e1.py
index 9f12be6a4..bbe3f18e0 100644
--- a/xtuner/configs/qwen/qwen1/qwen_7b/qwen_7b_qlora_openorca_e1.py
+++ b/xtuner/configs/qwen/qwen1/qwen_7b/qwen_7b_qlora_openorca_e1.py
@@ -17,6 +17,7 @@
VarlenAttnArgsToMessageHubHook)
from xtuner.engine.runner import TrainLoop
from xtuner.model import SupervisedFinetune
+from xtuner.parallel.sequence import SequenceParallelSampler
from xtuner.utils import PROMPT_TEMPLATE
#######################################################################
@@ -32,9 +33,13 @@
max_length = 2048
pack_to_max_length = True
+# parallel
+sequence_parallel_size = 1
+
# Scheduler & Optimizer
batch_size = 1 # per_device
accumulative_counts = 16
+accumulative_counts *= sequence_parallel_size
dataloader_num_workers = 0
max_epochs = 1
optim_type = AdamW
@@ -106,11 +111,14 @@
pack_to_max_length=pack_to_max_length,
use_varlen_attn=use_varlen_attn)
+sampler = SequenceParallelSampler \
+ if sequence_parallel_size > 1 else DefaultSampler
+
train_dataloader = dict(
batch_size=batch_size,
num_workers=dataloader_num_workers,
dataset=train_dataset,
- sampler=dict(type=DefaultSampler, shuffle=True),
+ sampler=dict(type=sampler, shuffle=True),
collate_fn=dict(type=default_collate_fn, use_varlen_attn=use_varlen_attn))
#######################################################################
diff --git a/xtuner/configs/qwen/qwen1/qwen_7b/qwen_7b_qlora_sql_e3.py b/xtuner/configs/qwen/qwen1/qwen_7b/qwen_7b_qlora_sql_e3.py
index 9ebdf176a..19de9c3c4 100644
--- a/xtuner/configs/qwen/qwen1/qwen_7b/qwen_7b_qlora_sql_e3.py
+++ b/xtuner/configs/qwen/qwen1/qwen_7b/qwen_7b_qlora_sql_e3.py
@@ -17,6 +17,7 @@
VarlenAttnArgsToMessageHubHook)
from xtuner.engine.runner import TrainLoop
from xtuner.model import SupervisedFinetune
+from xtuner.parallel.sequence import SequenceParallelSampler
from xtuner.utils import PROMPT_TEMPLATE, SYSTEM_TEMPLATE
#######################################################################
@@ -32,9 +33,13 @@
max_length = 2048
pack_to_max_length = True
+# parallel
+sequence_parallel_size = 1
+
# Scheduler & Optimizer
batch_size = 1 # per_device
accumulative_counts = 16
+accumulative_counts *= sequence_parallel_size
dataloader_num_workers = 0
max_epochs = 3
optim_type = AdamW
@@ -110,11 +115,14 @@
pack_to_max_length=pack_to_max_length,
use_varlen_attn=use_varlen_attn)
+sampler = SequenceParallelSampler \
+ if sequence_parallel_size > 1 else DefaultSampler
+
train_dataloader = dict(
batch_size=batch_size,
num_workers=dataloader_num_workers,
dataset=train_dataset,
- sampler=dict(type=DefaultSampler, shuffle=True),
+ sampler=dict(type=sampler, shuffle=True),
collate_fn=dict(type=default_collate_fn, use_varlen_attn=use_varlen_attn))
#######################################################################
diff --git a/xtuner/configs/qwen/qwen1/qwen_7b/qwen_7b_qlora_tiny_codes_e1.py b/xtuner/configs/qwen/qwen1/qwen_7b/qwen_7b_qlora_tiny_codes_e1.py
index 36b5c9093..c2391f8bc 100644
--- a/xtuner/configs/qwen/qwen1/qwen_7b/qwen_7b_qlora_tiny_codes_e1.py
+++ b/xtuner/configs/qwen/qwen1/qwen_7b/qwen_7b_qlora_tiny_codes_e1.py
@@ -17,6 +17,7 @@
VarlenAttnArgsToMessageHubHook)
from xtuner.engine.runner import TrainLoop
from xtuner.model import SupervisedFinetune
+from xtuner.parallel.sequence import SequenceParallelSampler
from xtuner.utils import PROMPT_TEMPLATE, SYSTEM_TEMPLATE
#######################################################################
@@ -32,9 +33,13 @@
max_length = 2048
pack_to_max_length = True
+# parallel
+sequence_parallel_size = 1
+
# Scheduler & Optimizer
batch_size = 1 # per_device
accumulative_counts = 16
+accumulative_counts *= sequence_parallel_size
dataloader_num_workers = 0
max_epochs = 1
optim_type = AdamW
@@ -110,11 +115,14 @@
pack_to_max_length=pack_to_max_length,
use_varlen_attn=use_varlen_attn)
+sampler = SequenceParallelSampler \
+ if sequence_parallel_size > 1 else DefaultSampler
+
train_dataloader = dict(
batch_size=batch_size,
num_workers=dataloader_num_workers,
dataset=train_dataset,
- sampler=dict(type=DefaultSampler, shuffle=True),
+ sampler=dict(type=sampler, shuffle=True),
collate_fn=dict(type=default_collate_fn, use_varlen_attn=use_varlen_attn))
#######################################################################
diff --git a/xtuner/configs/qwen/qwen1/qwen_7b_chat/qwen_7b_chat_qlora_alpaca_e3.py b/xtuner/configs/qwen/qwen1/qwen_7b_chat/qwen_7b_chat_qlora_alpaca_e3.py
index 504ca7920..eda0f5c9e 100644
--- a/xtuner/configs/qwen/qwen1/qwen_7b_chat/qwen_7b_chat_qlora_alpaca_e3.py
+++ b/xtuner/configs/qwen/qwen1/qwen_7b_chat/qwen_7b_chat_qlora_alpaca_e3.py
@@ -17,6 +17,7 @@
VarlenAttnArgsToMessageHubHook)
from xtuner.engine.runner import TrainLoop
from xtuner.model import SupervisedFinetune
+from xtuner.parallel.sequence import SequenceParallelSampler
from xtuner.utils import PROMPT_TEMPLATE, SYSTEM_TEMPLATE
#######################################################################
@@ -32,9 +33,13 @@
max_length = 2048
pack_to_max_length = True
+# parallel
+sequence_parallel_size = 1
+
# Scheduler & Optimizer
batch_size = 1 # per_device
accumulative_counts = 16
+accumulative_counts *= sequence_parallel_size
dataloader_num_workers = 0
max_epochs = 3
optim_type = AdamW
@@ -106,11 +111,14 @@
pack_to_max_length=pack_to_max_length,
use_varlen_attn=use_varlen_attn)
+sampler = SequenceParallelSampler \
+ if sequence_parallel_size > 1 else DefaultSampler
+
train_dataloader = dict(
batch_size=batch_size,
num_workers=dataloader_num_workers,
dataset=alpaca_en,
- sampler=dict(type=DefaultSampler, shuffle=True),
+ sampler=dict(type=sampler, shuffle=True),
collate_fn=dict(type=default_collate_fn, use_varlen_attn=use_varlen_attn))
#######################################################################
diff --git a/xtuner/configs/qwen/qwen1/qwen_7b_chat/qwen_7b_chat_qlora_alpaca_enzh_e3.py b/xtuner/configs/qwen/qwen1/qwen_7b_chat/qwen_7b_chat_qlora_alpaca_enzh_e3.py
index 43a257a28..e6d5c76e6 100644
--- a/xtuner/configs/qwen/qwen1/qwen_7b_chat/qwen_7b_chat_qlora_alpaca_enzh_e3.py
+++ b/xtuner/configs/qwen/qwen1/qwen_7b_chat/qwen_7b_chat_qlora_alpaca_enzh_e3.py
@@ -18,6 +18,7 @@
VarlenAttnArgsToMessageHubHook)
from xtuner.engine.runner import TrainLoop
from xtuner.model import SupervisedFinetune
+from xtuner.parallel.sequence import SequenceParallelSampler
from xtuner.utils import PROMPT_TEMPLATE, SYSTEM_TEMPLATE
#######################################################################
@@ -34,9 +35,13 @@
max_length = 2048
pack_to_max_length = True
+# parallel
+sequence_parallel_size = 1
+
# Scheduler & Optimizer
batch_size = 1 # per_device
accumulative_counts = 16
+accumulative_counts *= sequence_parallel_size
dataloader_num_workers = 0
max_epochs = 3
optim_type = AdamW
@@ -123,11 +128,14 @@
train_dataset = dict(type=ConcatDataset, datasets=[alpaca_en, alpaca_zh])
+sampler = SequenceParallelSampler \
+ if sequence_parallel_size > 1 else DefaultSampler
+
train_dataloader = dict(
batch_size=batch_size,
num_workers=dataloader_num_workers,
dataset=train_dataset,
- sampler=dict(type=DefaultSampler, shuffle=True),
+ sampler=dict(type=sampler, shuffle=True),
collate_fn=dict(type=default_collate_fn, use_varlen_attn=use_varlen_attn))
#######################################################################
diff --git a/xtuner/configs/qwen/qwen1/qwen_7b_chat/qwen_7b_chat_qlora_alpaca_enzh_oasst1_e3.py b/xtuner/configs/qwen/qwen1/qwen_7b_chat/qwen_7b_chat_qlora_alpaca_enzh_oasst1_e3.py
index cb1d9ee82..e9ee0420a 100644
--- a/xtuner/configs/qwen/qwen1/qwen_7b_chat/qwen_7b_chat_qlora_alpaca_enzh_oasst1_e3.py
+++ b/xtuner/configs/qwen/qwen1/qwen_7b_chat/qwen_7b_chat_qlora_alpaca_enzh_oasst1_e3.py
@@ -18,6 +18,7 @@
VarlenAttnArgsToMessageHubHook)
from xtuner.engine.runner import TrainLoop
from xtuner.model import SupervisedFinetune
+from xtuner.parallel.sequence import SequenceParallelSampler
from xtuner.utils import PROMPT_TEMPLATE, SYSTEM_TEMPLATE
#######################################################################
@@ -35,9 +36,13 @@
max_length = 2048
pack_to_max_length = True
+# parallel
+sequence_parallel_size = 1
+
# Scheduler & Optimizer
batch_size = 1 # per_device
accumulative_counts = 16
+accumulative_counts *= sequence_parallel_size
dataloader_num_workers = 0
max_epochs = 3
optim_type = AdamW
@@ -138,11 +143,14 @@
train_dataset = dict(
type=ConcatDataset, datasets=[alpaca_en, alpaca_zh, oasst1])
+sampler = SequenceParallelSampler \
+ if sequence_parallel_size > 1 else DefaultSampler
+
train_dataloader = dict(
batch_size=batch_size,
num_workers=dataloader_num_workers,
dataset=train_dataset,
- sampler=dict(type=DefaultSampler, shuffle=True),
+ sampler=dict(type=sampler, shuffle=True),
collate_fn=dict(type=default_collate_fn, use_varlen_attn=use_varlen_attn))
#######################################################################
diff --git a/xtuner/configs/qwen/qwen1/qwen_7b_chat/qwen_7b_chat_qlora_alpaca_zh_e3.py b/xtuner/configs/qwen/qwen1/qwen_7b_chat/qwen_7b_chat_qlora_alpaca_zh_e3.py
index eaf00df61..4aa6bac4f 100644
--- a/xtuner/configs/qwen/qwen1/qwen_7b_chat/qwen_7b_chat_qlora_alpaca_zh_e3.py
+++ b/xtuner/configs/qwen/qwen1/qwen_7b_chat/qwen_7b_chat_qlora_alpaca_zh_e3.py
@@ -17,6 +17,7 @@
VarlenAttnArgsToMessageHubHook)
from xtuner.engine.runner import TrainLoop
from xtuner.model import SupervisedFinetune
+from xtuner.parallel.sequence import SequenceParallelSampler
from xtuner.utils import PROMPT_TEMPLATE, SYSTEM_TEMPLATE
#######################################################################
@@ -32,9 +33,13 @@
max_length = 2048
pack_to_max_length = True
+# parallel
+sequence_parallel_size = 1
+
# Scheduler & Optimizer
batch_size = 1 # per_device
accumulative_counts = 16
+accumulative_counts *= sequence_parallel_size
dataloader_num_workers = 0
max_epochs = 3
optim_type = AdamW
@@ -106,11 +111,14 @@
pack_to_max_length=pack_to_max_length,
use_varlen_attn=use_varlen_attn)
+sampler = SequenceParallelSampler \
+ if sequence_parallel_size > 1 else DefaultSampler
+
train_dataloader = dict(
batch_size=batch_size,
num_workers=dataloader_num_workers,
dataset=alpaca_zh,
- sampler=dict(type=DefaultSampler, shuffle=True),
+ sampler=dict(type=sampler, shuffle=True),
collate_fn=dict(type=default_collate_fn, use_varlen_attn=use_varlen_attn))
#######################################################################
diff --git a/xtuner/configs/qwen/qwen1/qwen_7b_chat/qwen_7b_chat_qlora_arxiv_gentitle_e3.py b/xtuner/configs/qwen/qwen1/qwen_7b_chat/qwen_7b_chat_qlora_arxiv_gentitle_e3.py
index 3360a538e..be1b36849 100644
--- a/xtuner/configs/qwen/qwen1/qwen_7b_chat/qwen_7b_chat_qlora_arxiv_gentitle_e3.py
+++ b/xtuner/configs/qwen/qwen1/qwen_7b_chat/qwen_7b_chat_qlora_arxiv_gentitle_e3.py
@@ -17,6 +17,7 @@
VarlenAttnArgsToMessageHubHook)
from xtuner.engine.runner import TrainLoop
from xtuner.model import SupervisedFinetune
+from xtuner.parallel.sequence import SequenceParallelSampler
from xtuner.utils import PROMPT_TEMPLATE, SYSTEM_TEMPLATE
#######################################################################
@@ -34,9 +35,13 @@
max_length = 2048
pack_to_max_length = True
+# parallel
+sequence_parallel_size = 1
+
# Scheduler & Optimizer
batch_size = 1 # per_device
accumulative_counts = 16
+accumulative_counts *= sequence_parallel_size
dataloader_num_workers = 0
max_epochs = 3
optim_type = AdamW
@@ -141,11 +146,14 @@
pack_to_max_length=pack_to_max_length,
use_varlen_attn=use_varlen_attn)
+sampler = SequenceParallelSampler \
+ if sequence_parallel_size > 1 else DefaultSampler
+
train_dataloader = dict(
batch_size=batch_size,
num_workers=dataloader_num_workers,
dataset=train_dataset,
- sampler=dict(type=DefaultSampler, shuffle=True),
+ sampler=dict(type=sampler, shuffle=True),
collate_fn=dict(type=default_collate_fn, use_varlen_attn=use_varlen_attn))
#######################################################################
diff --git a/xtuner/configs/qwen/qwen1/qwen_7b_chat/qwen_7b_chat_qlora_code_alpaca_e3.py b/xtuner/configs/qwen/qwen1/qwen_7b_chat/qwen_7b_chat_qlora_code_alpaca_e3.py
index e7b1c591e..46ea7f28f 100644
--- a/xtuner/configs/qwen/qwen1/qwen_7b_chat/qwen_7b_chat_qlora_code_alpaca_e3.py
+++ b/xtuner/configs/qwen/qwen1/qwen_7b_chat/qwen_7b_chat_qlora_code_alpaca_e3.py
@@ -17,6 +17,7 @@
VarlenAttnArgsToMessageHubHook)
from xtuner.engine.runner import TrainLoop
from xtuner.model import SupervisedFinetune
+from xtuner.parallel.sequence import SequenceParallelSampler
from xtuner.utils import PROMPT_TEMPLATE, SYSTEM_TEMPLATE
#######################################################################
@@ -32,9 +33,13 @@
max_length = 2048
pack_to_max_length = True
+# parallel
+sequence_parallel_size = 1
+
# Scheduler & Optimizer
batch_size = 1 # per_device
accumulative_counts = 16
+accumulative_counts *= sequence_parallel_size
dataloader_num_workers = 0
max_epochs = 3
optim_type = AdamW
@@ -110,11 +115,14 @@
pack_to_max_length=pack_to_max_length,
use_varlen_attn=use_varlen_attn)
+sampler = SequenceParallelSampler \
+ if sequence_parallel_size > 1 else DefaultSampler
+
train_dataloader = dict(
batch_size=batch_size,
num_workers=dataloader_num_workers,
dataset=train_dataset,
- sampler=dict(type=DefaultSampler, shuffle=True),
+ sampler=dict(type=sampler, shuffle=True),
collate_fn=dict(type=default_collate_fn, use_varlen_attn=use_varlen_attn))
#######################################################################
diff --git a/xtuner/configs/qwen/qwen1/qwen_7b_chat/qwen_7b_chat_qlora_colorist_e5.py b/xtuner/configs/qwen/qwen1/qwen_7b_chat/qwen_7b_chat_qlora_colorist_e5.py
index 46aa0eba1..59eed5896 100644
--- a/xtuner/configs/qwen/qwen1/qwen_7b_chat/qwen_7b_chat_qlora_colorist_e5.py
+++ b/xtuner/configs/qwen/qwen1/qwen_7b_chat/qwen_7b_chat_qlora_colorist_e5.py
@@ -17,6 +17,7 @@
VarlenAttnArgsToMessageHubHook)
from xtuner.engine.runner import TrainLoop
from xtuner.model import SupervisedFinetune
+from xtuner.parallel.sequence import SequenceParallelSampler
from xtuner.utils import PROMPT_TEMPLATE, SYSTEM_TEMPLATE
#######################################################################
@@ -32,9 +33,13 @@
max_length = 2048
pack_to_max_length = True
+# parallel
+sequence_parallel_size = 1
+
# Scheduler & Optimizer
batch_size = 1 # per_device
accumulative_counts = 16
+accumulative_counts *= sequence_parallel_size
dataloader_num_workers = 0
max_epochs = 5
optim_type = AdamW
@@ -106,11 +111,14 @@
pack_to_max_length=pack_to_max_length,
use_varlen_attn=use_varlen_attn)
+sampler = SequenceParallelSampler \
+ if sequence_parallel_size > 1 else DefaultSampler
+
train_dataloader = dict(
batch_size=batch_size,
num_workers=dataloader_num_workers,
dataset=train_dataset,
- sampler=dict(type=DefaultSampler, shuffle=True),
+ sampler=dict(type=sampler, shuffle=True),
collate_fn=dict(type=default_collate_fn, use_varlen_attn=use_varlen_attn))
#######################################################################
diff --git a/xtuner/configs/qwen/qwen1/qwen_7b_chat/qwen_7b_chat_qlora_lawyer_e3.py b/xtuner/configs/qwen/qwen1/qwen_7b_chat/qwen_7b_chat_qlora_lawyer_e3.py
index 55d35f56b..b2cd75040 100644
--- a/xtuner/configs/qwen/qwen1/qwen_7b_chat/qwen_7b_chat_qlora_lawyer_e3.py
+++ b/xtuner/configs/qwen/qwen1/qwen_7b_chat/qwen_7b_chat_qlora_lawyer_e3.py
@@ -19,6 +19,7 @@
VarlenAttnArgsToMessageHubHook)
from xtuner.engine.runner import TrainLoop
from xtuner.model import SupervisedFinetune
+from xtuner.parallel.sequence import SequenceParallelSampler
from xtuner.utils import PROMPT_TEMPLATE, SYSTEM_TEMPLATE
#######################################################################
@@ -36,9 +37,13 @@
max_length = 2048
pack_to_max_length = True
+# parallel
+sequence_parallel_size = 1
+
# Scheduler & Optimizer
batch_size = 1 # per_device
accumulative_counts = 16
+accumulative_counts *= sequence_parallel_size
dataloader_num_workers = 0
max_epochs = 3
optim_type = AdamW
@@ -130,11 +135,14 @@
train_dataset = dict(
type=ConcatDataset, datasets=[crime_kg_assitant, law_reference_data])
+sampler = SequenceParallelSampler \
+ if sequence_parallel_size > 1 else DefaultSampler
+
train_dataloader = dict(
batch_size=batch_size,
num_workers=dataloader_num_workers,
dataset=train_dataset,
- sampler=dict(type=DefaultSampler, shuffle=True),
+ sampler=dict(type=sampler, shuffle=True),
collate_fn=dict(type=default_collate_fn, use_varlen_attn=use_varlen_attn))
#######################################################################
diff --git a/xtuner/configs/qwen/qwen1/qwen_7b_chat/qwen_7b_chat_qlora_medical_e1.py b/xtuner/configs/qwen/qwen1/qwen_7b_chat/qwen_7b_chat_qlora_medical_e1.py
index af29e0dfb..a3037d86f 100644
--- a/xtuner/configs/qwen/qwen1/qwen_7b_chat/qwen_7b_chat_qlora_medical_e1.py
+++ b/xtuner/configs/qwen/qwen1/qwen_7b_chat/qwen_7b_chat_qlora_medical_e1.py
@@ -17,6 +17,7 @@
VarlenAttnArgsToMessageHubHook)
from xtuner.engine.runner import TrainLoop
from xtuner.model import SupervisedFinetune
+from xtuner.parallel.sequence import SequenceParallelSampler
from xtuner.utils import PROMPT_TEMPLATE, SYSTEM_TEMPLATE
#######################################################################
@@ -33,9 +34,13 @@
max_length = 2048
pack_to_max_length = True
+# parallel
+sequence_parallel_size = 1
+
# Scheduler & Optimizer
batch_size = 1 # per_device
accumulative_counts = 16
+accumulative_counts *= sequence_parallel_size
dataloader_num_workers = 0
max_epochs = 1
optim_type = AdamW
@@ -108,11 +113,14 @@
pack_to_max_length=pack_to_max_length,
use_varlen_attn=use_varlen_attn)
+sampler = SequenceParallelSampler \
+ if sequence_parallel_size > 1 else DefaultSampler
+
train_dataloader = dict(
batch_size=batch_size,
num_workers=dataloader_num_workers,
dataset=train_dataset,
- sampler=dict(type=DefaultSampler, shuffle=True),
+ sampler=dict(type=sampler, shuffle=True),
collate_fn=dict(type=default_collate_fn, use_varlen_attn=use_varlen_attn))
#######################################################################
diff --git a/xtuner/configs/qwen/qwen1/qwen_7b_chat/qwen_7b_chat_qlora_oasst1_512_e3.py b/xtuner/configs/qwen/qwen1/qwen_7b_chat/qwen_7b_chat_qlora_oasst1_512_e3.py
index 8a54baf36..899939b24 100644
--- a/xtuner/configs/qwen/qwen1/qwen_7b_chat/qwen_7b_chat_qlora_oasst1_512_e3.py
+++ b/xtuner/configs/qwen/qwen1/qwen_7b_chat/qwen_7b_chat_qlora_oasst1_512_e3.py
@@ -17,6 +17,7 @@
VarlenAttnArgsToMessageHubHook)
from xtuner.engine.runner import TrainLoop
from xtuner.model import SupervisedFinetune
+from xtuner.parallel.sequence import SequenceParallelSampler
from xtuner.utils import PROMPT_TEMPLATE
#######################################################################
@@ -32,9 +33,13 @@
max_length = 512
pack_to_max_length = False
+# parallel
+sequence_parallel_size = 1
+
# Scheduler & Optimizer
batch_size = 1 # per_device
accumulative_counts = 16
+accumulative_counts *= sequence_parallel_size
dataloader_num_workers = 0
max_epochs = 3
optim_type = AdamW
@@ -106,11 +111,14 @@
pack_to_max_length=pack_to_max_length,
use_varlen_attn=use_varlen_attn)
+sampler = SequenceParallelSampler \
+ if sequence_parallel_size > 1 else DefaultSampler
+
train_dataloader = dict(
batch_size=batch_size,
num_workers=dataloader_num_workers,
dataset=train_dataset,
- sampler=dict(type=DefaultSampler, shuffle=True),
+ sampler=dict(type=sampler, shuffle=True),
collate_fn=dict(type=default_collate_fn, use_varlen_attn=use_varlen_attn))
#######################################################################
diff --git a/xtuner/configs/qwen/qwen1/qwen_7b_chat/qwen_7b_chat_qlora_oasst1_e3.py b/xtuner/configs/qwen/qwen1/qwen_7b_chat/qwen_7b_chat_qlora_oasst1_e3.py
index bbb5fddf4..20eb1f806 100644
--- a/xtuner/configs/qwen/qwen1/qwen_7b_chat/qwen_7b_chat_qlora_oasst1_e3.py
+++ b/xtuner/configs/qwen/qwen1/qwen_7b_chat/qwen_7b_chat_qlora_oasst1_e3.py
@@ -17,6 +17,7 @@
VarlenAttnArgsToMessageHubHook)
from xtuner.engine.runner import TrainLoop
from xtuner.model import SupervisedFinetune
+from xtuner.parallel.sequence import SequenceParallelSampler
from xtuner.utils import PROMPT_TEMPLATE
#######################################################################
@@ -32,9 +33,13 @@
max_length = 2048
pack_to_max_length = True
+# parallel
+sequence_parallel_size = 1
+
# Scheduler & Optimizer
batch_size = 1 # per_device
accumulative_counts = 16
+accumulative_counts *= sequence_parallel_size
dataloader_num_workers = 0
max_epochs = 3
optim_type = AdamW
@@ -106,11 +111,14 @@
pack_to_max_length=pack_to_max_length,
use_varlen_attn=use_varlen_attn)
+sampler = SequenceParallelSampler \
+ if sequence_parallel_size > 1 else DefaultSampler
+
train_dataloader = dict(
batch_size=batch_size,
num_workers=dataloader_num_workers,
dataset=train_dataset,
- sampler=dict(type=DefaultSampler, shuffle=True),
+ sampler=dict(type=sampler, shuffle=True),
collate_fn=dict(type=default_collate_fn, use_varlen_attn=use_varlen_attn))
#######################################################################
diff --git a/xtuner/configs/qwen/qwen1/qwen_7b_chat/qwen_7b_chat_qlora_open_platypus_e3.py b/xtuner/configs/qwen/qwen1/qwen_7b_chat/qwen_7b_chat_qlora_open_platypus_e3.py
index 9c57b6988..aa09ec408 100644
--- a/xtuner/configs/qwen/qwen1/qwen_7b_chat/qwen_7b_chat_qlora_open_platypus_e3.py
+++ b/xtuner/configs/qwen/qwen1/qwen_7b_chat/qwen_7b_chat_qlora_open_platypus_e3.py
@@ -17,6 +17,7 @@
VarlenAttnArgsToMessageHubHook)
from xtuner.engine.runner import TrainLoop
from xtuner.model import SupervisedFinetune
+from xtuner.parallel.sequence import SequenceParallelSampler
from xtuner.utils import PROMPT_TEMPLATE, SYSTEM_TEMPLATE
#######################################################################
@@ -32,9 +33,13 @@
max_length = 2048
pack_to_max_length = True
+# parallel
+sequence_parallel_size = 1
+
# Scheduler & Optimizer
batch_size = 1 # per_device
accumulative_counts = 16
+accumulative_counts *= sequence_parallel_size
dataloader_num_workers = 0
max_epochs = 3
optim_type = AdamW
@@ -106,11 +111,14 @@
pack_to_max_length=pack_to_max_length,
use_varlen_attn=use_varlen_attn)
+sampler = SequenceParallelSampler \
+ if sequence_parallel_size > 1 else DefaultSampler
+
train_dataloader = dict(
batch_size=batch_size,
num_workers=dataloader_num_workers,
dataset=train_dataset,
- sampler=dict(type=DefaultSampler, shuffle=True),
+ sampler=dict(type=sampler, shuffle=True),
collate_fn=dict(type=default_collate_fn, use_varlen_attn=use_varlen_attn))
#######################################################################
diff --git a/xtuner/configs/qwen/qwen1/qwen_7b_chat/qwen_7b_chat_qlora_openorca_e1.py b/xtuner/configs/qwen/qwen1/qwen_7b_chat/qwen_7b_chat_qlora_openorca_e1.py
index 20c14edea..1abd4ec50 100644
--- a/xtuner/configs/qwen/qwen1/qwen_7b_chat/qwen_7b_chat_qlora_openorca_e1.py
+++ b/xtuner/configs/qwen/qwen1/qwen_7b_chat/qwen_7b_chat_qlora_openorca_e1.py
@@ -17,6 +17,7 @@
VarlenAttnArgsToMessageHubHook)
from xtuner.engine.runner import TrainLoop
from xtuner.model import SupervisedFinetune
+from xtuner.parallel.sequence import SequenceParallelSampler
from xtuner.utils import PROMPT_TEMPLATE
#######################################################################
@@ -32,9 +33,13 @@
max_length = 2048
pack_to_max_length = True
+# parallel
+sequence_parallel_size = 1
+
# Scheduler & Optimizer
batch_size = 1 # per_device
accumulative_counts = 16
+accumulative_counts *= sequence_parallel_size
dataloader_num_workers = 0
max_epochs = 1
optim_type = AdamW
@@ -106,11 +111,14 @@
pack_to_max_length=pack_to_max_length,
use_varlen_attn=use_varlen_attn)
+sampler = SequenceParallelSampler \
+ if sequence_parallel_size > 1 else DefaultSampler
+
train_dataloader = dict(
batch_size=batch_size,
num_workers=dataloader_num_workers,
dataset=train_dataset,
- sampler=dict(type=DefaultSampler, shuffle=True),
+ sampler=dict(type=sampler, shuffle=True),
collate_fn=dict(type=default_collate_fn, use_varlen_attn=use_varlen_attn))
#######################################################################
diff --git a/xtuner/configs/qwen/qwen1/qwen_7b_chat/qwen_7b_chat_qlora_sql_e3.py b/xtuner/configs/qwen/qwen1/qwen_7b_chat/qwen_7b_chat_qlora_sql_e3.py
index cae844756..8f5a6fe4d 100644
--- a/xtuner/configs/qwen/qwen1/qwen_7b_chat/qwen_7b_chat_qlora_sql_e3.py
+++ b/xtuner/configs/qwen/qwen1/qwen_7b_chat/qwen_7b_chat_qlora_sql_e3.py
@@ -17,6 +17,7 @@
VarlenAttnArgsToMessageHubHook)
from xtuner.engine.runner import TrainLoop
from xtuner.model import SupervisedFinetune
+from xtuner.parallel.sequence import SequenceParallelSampler
from xtuner.utils import PROMPT_TEMPLATE, SYSTEM_TEMPLATE
#######################################################################
@@ -32,9 +33,13 @@
max_length = 2048
pack_to_max_length = True
+# parallel
+sequence_parallel_size = 1
+
# Scheduler & Optimizer
batch_size = 1 # per_device
accumulative_counts = 16
+accumulative_counts *= sequence_parallel_size
dataloader_num_workers = 0
max_epochs = 3
optim_type = AdamW
@@ -110,11 +115,14 @@
pack_to_max_length=pack_to_max_length,
use_varlen_attn=use_varlen_attn)
+sampler = SequenceParallelSampler \
+ if sequence_parallel_size > 1 else DefaultSampler
+
train_dataloader = dict(
batch_size=batch_size,
num_workers=dataloader_num_workers,
dataset=train_dataset,
- sampler=dict(type=DefaultSampler, shuffle=True),
+ sampler=dict(type=sampler, shuffle=True),
collate_fn=dict(type=default_collate_fn, use_varlen_attn=use_varlen_attn))
#######################################################################
diff --git a/xtuner/configs/qwen/qwen1/qwen_7b_chat/qwen_7b_chat_qlora_tiny_codes_e1.py b/xtuner/configs/qwen/qwen1/qwen_7b_chat/qwen_7b_chat_qlora_tiny_codes_e1.py
index ef4b2fea1..f0044f043 100644
--- a/xtuner/configs/qwen/qwen1/qwen_7b_chat/qwen_7b_chat_qlora_tiny_codes_e1.py
+++ b/xtuner/configs/qwen/qwen1/qwen_7b_chat/qwen_7b_chat_qlora_tiny_codes_e1.py
@@ -17,6 +17,7 @@
VarlenAttnArgsToMessageHubHook)
from xtuner.engine.runner import TrainLoop
from xtuner.model import SupervisedFinetune
+from xtuner.parallel.sequence import SequenceParallelSampler
from xtuner.utils import PROMPT_TEMPLATE, SYSTEM_TEMPLATE
#######################################################################
@@ -32,9 +33,13 @@
max_length = 2048
pack_to_max_length = True
+# parallel
+sequence_parallel_size = 1
+
# Scheduler & Optimizer
batch_size = 1 # per_device
accumulative_counts = 16
+accumulative_counts *= sequence_parallel_size
dataloader_num_workers = 0
max_epochs = 1
optim_type = AdamW
@@ -110,11 +115,14 @@
pack_to_max_length=pack_to_max_length,
use_varlen_attn=use_varlen_attn)
+sampler = SequenceParallelSampler \
+ if sequence_parallel_size > 1 else DefaultSampler
+
train_dataloader = dict(
batch_size=batch_size,
num_workers=dataloader_num_workers,
dataset=train_dataset,
- sampler=dict(type=DefaultSampler, shuffle=True),
+ sampler=dict(type=sampler, shuffle=True),
collate_fn=dict(type=default_collate_fn, use_varlen_attn=use_varlen_attn))
#######################################################################
diff --git a/xtuner/configs/qwen/qwen1_5/qwen1_5_0_5b/qwen1_5_0_5b_full_alpaca_e3.py b/xtuner/configs/qwen/qwen1_5/qwen1_5_0_5b/qwen1_5_0_5b_full_alpaca_e3.py
index c5f8d443f..dec0ed76e 100644
--- a/xtuner/configs/qwen/qwen1_5/qwen1_5_0_5b/qwen1_5_0_5b_full_alpaca_e3.py
+++ b/xtuner/configs/qwen/qwen1_5/qwen1_5_0_5b/qwen1_5_0_5b_full_alpaca_e3.py
@@ -14,6 +14,7 @@
VarlenAttnArgsToMessageHubHook)
from xtuner.engine.runner import TrainLoop
from xtuner.model import SupervisedFinetune
+from xtuner.parallel.sequence import SequenceParallelSampler
from xtuner.utils import PROMPT_TEMPLATE, SYSTEM_TEMPLATE
#######################################################################
@@ -29,9 +30,13 @@
max_length = 2048
pack_to_max_length = True
+# parallel
+sequence_parallel_size = 1
+
# Scheduler & Optimizer
batch_size = 1 # per_device
accumulative_counts = 16
+accumulative_counts *= sequence_parallel_size
dataloader_num_workers = 0
max_epochs = 3
optim_type = AdamW
@@ -85,11 +90,14 @@
pack_to_max_length=pack_to_max_length,
use_varlen_attn=use_varlen_attn)
+sampler = SequenceParallelSampler \
+ if sequence_parallel_size > 1 else DefaultSampler
+
train_dataloader = dict(
batch_size=batch_size,
num_workers=dataloader_num_workers,
dataset=alpaca_en,
- sampler=dict(type=DefaultSampler, shuffle=True),
+ sampler=dict(type=sampler, shuffle=True),
collate_fn=dict(type=default_collate_fn, use_varlen_attn=use_varlen_attn))
#######################################################################
diff --git a/xtuner/configs/qwen/qwen1_5/qwen1_5_0_5b/qwen1_5_0_5b_qlora_alpaca_e3.py b/xtuner/configs/qwen/qwen1_5/qwen1_5_0_5b/qwen1_5_0_5b_qlora_alpaca_e3.py
index 67cf05f20..341544eb9 100644
--- a/xtuner/configs/qwen/qwen1_5/qwen1_5_0_5b/qwen1_5_0_5b_qlora_alpaca_e3.py
+++ b/xtuner/configs/qwen/qwen1_5/qwen1_5_0_5b/qwen1_5_0_5b_qlora_alpaca_e3.py
@@ -17,6 +17,7 @@
VarlenAttnArgsToMessageHubHook)
from xtuner.engine.runner import TrainLoop
from xtuner.model import SupervisedFinetune
+from xtuner.parallel.sequence import SequenceParallelSampler
from xtuner.utils import PROMPT_TEMPLATE, SYSTEM_TEMPLATE
#######################################################################
@@ -32,9 +33,13 @@
max_length = 2048
pack_to_max_length = True
+# parallel
+sequence_parallel_size = 1
+
# Scheduler & Optimizer
batch_size = 1 # per_device
accumulative_counts = 16
+accumulative_counts *= sequence_parallel_size
dataloader_num_workers = 0
max_epochs = 3
optim_type = AdamW
@@ -105,11 +110,14 @@
pack_to_max_length=pack_to_max_length,
use_varlen_attn=use_varlen_attn)
+sampler = SequenceParallelSampler \
+ if sequence_parallel_size > 1 else DefaultSampler
+
train_dataloader = dict(
batch_size=batch_size,
num_workers=dataloader_num_workers,
dataset=alpaca_en,
- sampler=dict(type=DefaultSampler, shuffle=True),
+ sampler=dict(type=sampler, shuffle=True),
collate_fn=dict(type=default_collate_fn, use_varlen_attn=use_varlen_attn))
#######################################################################
diff --git a/xtuner/configs/qwen/qwen1_5/qwen1_5_0_5b_chat/qwen1_5_0_5b_chat_full_alpaca_e3.py b/xtuner/configs/qwen/qwen1_5/qwen1_5_0_5b_chat/qwen1_5_0_5b_chat_full_alpaca_e3.py
index 9baedcb9d..fcd9c24d2 100644
--- a/xtuner/configs/qwen/qwen1_5/qwen1_5_0_5b_chat/qwen1_5_0_5b_chat_full_alpaca_e3.py
+++ b/xtuner/configs/qwen/qwen1_5/qwen1_5_0_5b_chat/qwen1_5_0_5b_chat_full_alpaca_e3.py
@@ -14,6 +14,7 @@
VarlenAttnArgsToMessageHubHook)
from xtuner.engine.runner import TrainLoop
from xtuner.model import SupervisedFinetune
+from xtuner.parallel.sequence import SequenceParallelSampler
from xtuner.utils import PROMPT_TEMPLATE, SYSTEM_TEMPLATE
#######################################################################
@@ -29,9 +30,13 @@
max_length = 2048
pack_to_max_length = True
+# parallel
+sequence_parallel_size = 1
+
# Scheduler & Optimizer
batch_size = 1 # per_device
accumulative_counts = 16
+accumulative_counts *= sequence_parallel_size
dataloader_num_workers = 0
max_epochs = 3
optim_type = AdamW
@@ -85,11 +90,14 @@
pack_to_max_length=pack_to_max_length,
use_varlen_attn=use_varlen_attn)
+sampler = SequenceParallelSampler \
+ if sequence_parallel_size > 1 else DefaultSampler
+
train_dataloader = dict(
batch_size=batch_size,
num_workers=dataloader_num_workers,
dataset=alpaca_en,
- sampler=dict(type=DefaultSampler, shuffle=True),
+ sampler=dict(type=sampler, shuffle=True),
collate_fn=dict(type=default_collate_fn, use_varlen_attn=use_varlen_attn))
#######################################################################
diff --git a/xtuner/configs/qwen/qwen1_5/qwen1_5_0_5b_chat/qwen1_5_0_5b_chat_qlora_alpaca_e3.py b/xtuner/configs/qwen/qwen1_5/qwen1_5_0_5b_chat/qwen1_5_0_5b_chat_qlora_alpaca_e3.py
index e7113a53d..129b12752 100644
--- a/xtuner/configs/qwen/qwen1_5/qwen1_5_0_5b_chat/qwen1_5_0_5b_chat_qlora_alpaca_e3.py
+++ b/xtuner/configs/qwen/qwen1_5/qwen1_5_0_5b_chat/qwen1_5_0_5b_chat_qlora_alpaca_e3.py
@@ -17,6 +17,7 @@
VarlenAttnArgsToMessageHubHook)
from xtuner.engine.runner import TrainLoop
from xtuner.model import SupervisedFinetune
+from xtuner.parallel.sequence import SequenceParallelSampler
from xtuner.utils import PROMPT_TEMPLATE, SYSTEM_TEMPLATE
#######################################################################
@@ -32,9 +33,13 @@
max_length = 2048
pack_to_max_length = True
+# parallel
+sequence_parallel_size = 1
+
# Scheduler & Optimizer
batch_size = 1 # per_device
accumulative_counts = 16
+accumulative_counts *= sequence_parallel_size
dataloader_num_workers = 0
max_epochs = 3
optim_type = AdamW
@@ -105,11 +110,14 @@
pack_to_max_length=pack_to_max_length,
use_varlen_attn=use_varlen_attn)
+sampler = SequenceParallelSampler \
+ if sequence_parallel_size > 1 else DefaultSampler
+
train_dataloader = dict(
batch_size=batch_size,
num_workers=dataloader_num_workers,
dataset=alpaca_en,
- sampler=dict(type=DefaultSampler, shuffle=True),
+ sampler=dict(type=sampler, shuffle=True),
collate_fn=dict(type=default_collate_fn, use_varlen_attn=use_varlen_attn))
#######################################################################
diff --git a/xtuner/configs/qwen/qwen1_5/qwen1_5_110b/qwen1_5_110b_full_alpaca_e3.py b/xtuner/configs/qwen/qwen1_5/qwen1_5_110b/qwen1_5_110b_full_alpaca_e3.py
new file mode 100644
index 000000000..b16660ec0
--- /dev/null
+++ b/xtuner/configs/qwen/qwen1_5/qwen1_5_110b/qwen1_5_110b_full_alpaca_e3.py
@@ -0,0 +1,203 @@
+# Copyright (c) OpenMMLab. All rights reserved.
+from datasets import load_dataset
+from mmengine.dataset import DefaultSampler
+from mmengine.hooks import (CheckpointHook, DistSamplerSeedHook, IterTimerHook,
+ LoggerHook, ParamSchedulerHook)
+from mmengine.optim import AmpOptimWrapper, CosineAnnealingLR, LinearLR
+from torch.optim import AdamW
+from transformers import AutoModelForCausalLM, AutoTokenizer
+
+from xtuner.dataset import process_hf_dataset
+from xtuner.dataset.collate_fns import default_collate_fn
+from xtuner.dataset.map_fns import alpaca_map_fn, template_map_fn_factory
+from xtuner.engine.hooks import (DatasetInfoHook, EvaluateChatHook,
+ ThroughputHook,
+ VarlenAttnArgsToMessageHubHook)
+from xtuner.engine.runner import TrainLoop
+from xtuner.model import SupervisedFinetune
+from xtuner.parallel.sequence import SequenceParallelSampler
+from xtuner.utils import PROMPT_TEMPLATE, SYSTEM_TEMPLATE
+
+#######################################################################
+# PART 1 Settings #
+#######################################################################
+# Model
+pretrained_model_name_or_path = 'Qwen/Qwen1.5-110B'
+use_varlen_attn = False
+
+# Data
+alpaca_en_path = 'tatsu-lab/alpaca'
+prompt_template = PROMPT_TEMPLATE.default
+max_length = 2048
+pack_to_max_length = True
+
+# parallel
+sequence_parallel_size = 1
+
+# Scheduler & Optimizer
+batch_size = 1 # per_device
+# total batch = 32gpus * batch_size_per_device 1 * acc 1 = 32
+accumulative_counts = 1
+accumulative_counts *= sequence_parallel_size
+dataloader_num_workers = 4
+max_epochs = 3
+optim_type = AdamW
+lr = 1e-5
+betas = (0.9, 0.999)
+weight_decay = 0
+max_norm = 1 # grad clip
+warmup_ratio = 0.03
+
+# Save
+save_steps = 500
+save_total_limit = 2 # Maximum checkpoints to keep (-1 means unlimited)
+
+# Evaluate the generation performance during the training
+evaluation_freq = 500
+SYSTEM = SYSTEM_TEMPLATE.alpaca
+evaluation_inputs = [
+ '请给我介绍五个上海的景点', 'Please tell me five scenic spots in Shanghai'
+]
+
+#######################################################################
+# PART 2 Model & Tokenizer #
+#######################################################################
+tokenizer = dict(
+ type=AutoTokenizer.from_pretrained,
+ pretrained_model_name_or_path=pretrained_model_name_or_path,
+ trust_remote_code=True,
+ padding_side='right')
+
+model = dict(
+ type=SupervisedFinetune,
+ use_varlen_attn=use_varlen_attn,
+ llm=dict(
+ type=AutoModelForCausalLM.from_pretrained,
+ pretrained_model_name_or_path=pretrained_model_name_or_path,
+ trust_remote_code=True))
+
+#######################################################################
+# PART 3 Dataset & Dataloader #
+#######################################################################
+alpaca_en = dict(
+ type=process_hf_dataset,
+ dataset=dict(type=load_dataset, path=alpaca_en_path),
+ tokenizer=tokenizer,
+ max_length=max_length,
+ dataset_map_fn=alpaca_map_fn,
+ template_map_fn=dict(
+ type=template_map_fn_factory, template=prompt_template),
+ remove_unused_columns=True,
+ shuffle_before_pack=True,
+ pack_to_max_length=pack_to_max_length,
+ use_varlen_attn=use_varlen_attn)
+
+sampler = SequenceParallelSampler \
+ if sequence_parallel_size > 1 else DefaultSampler
+
+train_dataloader = dict(
+ batch_size=batch_size,
+ num_workers=dataloader_num_workers,
+ dataset=alpaca_en,
+ sampler=dict(type=sampler, shuffle=True),
+ collate_fn=dict(type=default_collate_fn, use_varlen_attn=use_varlen_attn))
+
+#######################################################################
+# PART 4 Scheduler & Optimizer #
+#######################################################################
+# optimizer
+optim_wrapper = dict(
+ type=AmpOptimWrapper,
+ optimizer=dict(
+ type=optim_type, lr=lr, betas=betas, weight_decay=weight_decay),
+ clip_grad=dict(max_norm=max_norm, error_if_nonfinite=False),
+ accumulative_counts=accumulative_counts,
+ loss_scale='dynamic',
+ dtype='float16')
+
+# learning policy
+# More information: https://github.com/open-mmlab/mmengine/blob/main/docs/en/tutorials/param_scheduler.md # noqa: E501
+param_scheduler = [
+ dict(
+ type=LinearLR,
+ start_factor=1e-5,
+ by_epoch=True,
+ begin=0,
+ end=warmup_ratio * max_epochs,
+ convert_to_iter_based=True),
+ dict(
+ type=CosineAnnealingLR,
+ eta_min=0.0,
+ by_epoch=True,
+ begin=warmup_ratio * max_epochs,
+ end=max_epochs,
+ convert_to_iter_based=True)
+]
+
+# train, val, test setting
+train_cfg = dict(type=TrainLoop, max_epochs=max_epochs)
+
+#######################################################################
+# PART 5 Runtime #
+#######################################################################
+# Log the dialogue periodically during the training process, optional
+custom_hooks = [
+ dict(type=DatasetInfoHook, tokenizer=tokenizer),
+ dict(
+ type=EvaluateChatHook,
+ tokenizer=tokenizer,
+ every_n_iters=evaluation_freq,
+ evaluation_inputs=evaluation_inputs,
+ system=SYSTEM,
+ prompt_template=prompt_template),
+ dict(type=ThroughputHook)
+]
+
+if use_varlen_attn:
+ custom_hooks += [dict(type=VarlenAttnArgsToMessageHubHook)]
+
+# configure default hooks
+default_hooks = dict(
+ # record the time of every iteration.
+ timer=dict(type=IterTimerHook),
+ # print log every 10 iterations.
+ logger=dict(type=LoggerHook, log_metric_by_epoch=False, interval=10),
+ # enable the parameter scheduler.
+ param_scheduler=dict(type=ParamSchedulerHook),
+ # save checkpoint per `save_steps`.
+ checkpoint=dict(
+ type=CheckpointHook,
+ by_epoch=False,
+ interval=save_steps,
+ max_keep_ckpts=save_total_limit),
+ # set sampler seed in distributed evrionment.
+ sampler_seed=dict(type=DistSamplerSeedHook),
+)
+
+# configure environment
+env_cfg = dict(
+ # whether to enable cudnn benchmark
+ cudnn_benchmark=False,
+ # set multi process parameters
+ mp_cfg=dict(mp_start_method='fork', opencv_num_threads=0),
+ # set distributed parameters
+ dist_cfg=dict(backend='nccl'),
+)
+
+# set visualizer
+visualizer = None
+
+# set log level
+log_level = 'INFO'
+
+# load from which checkpoint
+load_from = None
+
+# whether to resume training from the loaded checkpoint
+resume = False
+
+# Defaults to use random seed and disable `deterministic`
+randomness = dict(seed=None, deterministic=False)
+
+# set log processor
+log_processor = dict(by_epoch=False)
diff --git a/xtuner/configs/qwen/qwen1_5/qwen1_5_110b/qwen1_5_110b_qlora_alpaca_e3.py b/xtuner/configs/qwen/qwen1_5/qwen1_5_110b/qwen1_5_110b_qlora_alpaca_e3.py
new file mode 100644
index 000000000..747d0fe17
--- /dev/null
+++ b/xtuner/configs/qwen/qwen1_5/qwen1_5_110b/qwen1_5_110b_qlora_alpaca_e3.py
@@ -0,0 +1,223 @@
+# Copyright (c) OpenMMLab. All rights reserved.
+import torch
+from datasets import load_dataset
+from mmengine.dataset import DefaultSampler
+from mmengine.hooks import (CheckpointHook, DistSamplerSeedHook, IterTimerHook,
+ LoggerHook, ParamSchedulerHook)
+from mmengine.optim import AmpOptimWrapper, CosineAnnealingLR, LinearLR
+from peft import LoraConfig
+from torch.optim import AdamW
+from transformers import (AutoModelForCausalLM, AutoTokenizer,
+ BitsAndBytesConfig)
+
+from xtuner.dataset import process_hf_dataset
+from xtuner.dataset.collate_fns import default_collate_fn
+from xtuner.dataset.map_fns import alpaca_map_fn, template_map_fn_factory
+from xtuner.engine.hooks import (DatasetInfoHook, EvaluateChatHook,
+ ThroughputHook,
+ VarlenAttnArgsToMessageHubHook)
+from xtuner.engine.runner import TrainLoop
+from xtuner.model import SupervisedFinetune
+from xtuner.parallel.sequence import SequenceParallelSampler
+from xtuner.utils import PROMPT_TEMPLATE, SYSTEM_TEMPLATE
+
+#######################################################################
+# PART 1 Settings #
+#######################################################################
+# Model
+pretrained_model_name_or_path = 'Qwen/Qwen1.5-110B'
+use_varlen_attn = False
+
+# Data
+alpaca_en_path = 'tatsu-lab/alpaca'
+prompt_template = PROMPT_TEMPLATE.default
+max_length = 2048
+pack_to_max_length = True
+
+# parallel
+sequence_parallel_size = 1
+
+# Scheduler & Optimizer
+batch_size = 1 # per_device
+accumulative_counts = 1 # total bs = 1 bs_per_device * 8 gpus * 1 acc = 8
+accumulative_counts *= sequence_parallel_size
+dataloader_num_workers = 4
+max_epochs = 3
+optim_type = AdamW
+lr = 1e-4 # 110B model use smaller lr
+betas = (0.9, 0.999)
+weight_decay = 0
+max_norm = 1 # grad clip
+warmup_ratio = 0.03
+
+# Save
+save_steps = 500
+save_total_limit = 2 # Maximum checkpoints to keep (-1 means unlimited)
+
+# Evaluate the generation performance during the training
+evaluation_freq = 500
+SYSTEM = SYSTEM_TEMPLATE.alpaca
+evaluation_inputs = [
+ '请给我介绍五个上海的景点', 'Please tell me five scenic spots in Shanghai'
+]
+
+#######################################################################
+# PART 2 Model & Tokenizer #
+#######################################################################
+tokenizer = dict(
+ type=AutoTokenizer.from_pretrained,
+ pretrained_model_name_or_path=pretrained_model_name_or_path,
+ trust_remote_code=True,
+ padding_side='right')
+
+model = dict(
+ type=SupervisedFinetune,
+ use_varlen_attn=use_varlen_attn,
+ llm=dict(
+ type=AutoModelForCausalLM.from_pretrained,
+ pretrained_model_name_or_path=pretrained_model_name_or_path,
+ trust_remote_code=True,
+ torch_dtype=torch.float16,
+ quantization_config=dict(
+ type=BitsAndBytesConfig,
+ load_in_4bit=True,
+ load_in_8bit=False,
+ llm_int8_threshold=6.0,
+ llm_int8_has_fp16_weight=False,
+ bnb_4bit_compute_dtype=torch.float16,
+ bnb_4bit_use_double_quant=True,
+ bnb_4bit_quant_type='nf4',
+ bnb_4bit_quant_storage=torch.float16)),
+ lora=dict(
+ type=LoraConfig,
+ r=64,
+ lora_alpha=16,
+ lora_dropout=0.1,
+ bias='none',
+ task_type='CAUSAL_LM'))
+
+#######################################################################
+# PART 3 Dataset & Dataloader #
+#######################################################################
+alpaca_en = dict(
+ type=process_hf_dataset,
+ dataset=dict(type=load_dataset, path=alpaca_en_path),
+ tokenizer=tokenizer,
+ max_length=max_length,
+ dataset_map_fn=alpaca_map_fn,
+ template_map_fn=dict(
+ type=template_map_fn_factory, template=prompt_template),
+ remove_unused_columns=True,
+ shuffle_before_pack=True,
+ pack_to_max_length=pack_to_max_length,
+ use_varlen_attn=use_varlen_attn)
+
+sampler = SequenceParallelSampler \
+ if sequence_parallel_size > 1 else DefaultSampler
+
+train_dataloader = dict(
+ batch_size=batch_size,
+ num_workers=dataloader_num_workers,
+ dataset=alpaca_en,
+ sampler=dict(type=sampler, shuffle=True),
+ collate_fn=dict(type=default_collate_fn, use_varlen_attn=use_varlen_attn))
+
+#######################################################################
+# PART 4 Scheduler & Optimizer #
+#######################################################################
+# optimizer
+optim_wrapper = dict(
+ type=AmpOptimWrapper,
+ optimizer=dict(
+ type=optim_type, lr=lr, betas=betas, weight_decay=weight_decay),
+ clip_grad=dict(max_norm=max_norm, error_if_nonfinite=False),
+ accumulative_counts=accumulative_counts,
+ loss_scale='dynamic',
+ dtype='float16')
+
+# learning policy
+# More information: https://github.com/open-mmlab/mmengine/blob/main/docs/en/tutorials/param_scheduler.md # noqa: E501
+param_scheduler = [
+ dict(
+ type=LinearLR,
+ start_factor=1e-5,
+ by_epoch=True,
+ begin=0,
+ end=warmup_ratio * max_epochs,
+ convert_to_iter_based=True),
+ dict(
+ type=CosineAnnealingLR,
+ eta_min=0.0,
+ by_epoch=True,
+ begin=warmup_ratio * max_epochs,
+ end=max_epochs,
+ convert_to_iter_based=True)
+]
+
+# train, val, test setting
+train_cfg = dict(type=TrainLoop, max_epochs=max_epochs)
+
+#######################################################################
+# PART 5 Runtime #
+#######################################################################
+# Log the dialogue periodically during the training process, optional
+custom_hooks = [
+ dict(type=DatasetInfoHook, tokenizer=tokenizer),
+ dict(type=ThroughputHook),
+ dict(
+ type=EvaluateChatHook,
+ tokenizer=tokenizer,
+ every_n_iters=evaluation_freq,
+ evaluation_inputs=evaluation_inputs,
+ system=SYSTEM,
+ prompt_template=prompt_template)
+]
+
+if use_varlen_attn:
+ custom_hooks += [dict(type=VarlenAttnArgsToMessageHubHook)]
+
+# configure default hooks
+default_hooks = dict(
+ # record the time of every iteration.
+ timer=dict(type=IterTimerHook),
+ # print log every 10 iterations.
+ logger=dict(type=LoggerHook, log_metric_by_epoch=False, interval=10),
+ # enable the parameter scheduler.
+ param_scheduler=dict(type=ParamSchedulerHook),
+ # save checkpoint per `save_steps`.
+ checkpoint=dict(
+ type=CheckpointHook,
+ by_epoch=False,
+ interval=save_steps,
+ max_keep_ckpts=save_total_limit),
+ # set sampler seed in distributed evrionment.
+ sampler_seed=dict(type=DistSamplerSeedHook),
+)
+
+# configure environment
+env_cfg = dict(
+ # whether to enable cudnn benchmark
+ cudnn_benchmark=False,
+ # set multi process parameters
+ mp_cfg=dict(mp_start_method='fork', opencv_num_threads=0),
+ # set distributed parameters
+ dist_cfg=dict(backend='nccl'),
+)
+
+# set visualizer
+visualizer = None
+
+# set log level
+log_level = 'INFO'
+
+# load from which checkpoint
+load_from = None
+
+# whether to resume training from the loaded checkpoint
+resume = False
+
+# Defaults to use random seed and disable `deterministic`
+randomness = dict(seed=None, deterministic=False)
+
+# set log processor
+log_processor = dict(by_epoch=False)
diff --git a/xtuner/configs/qwen/qwen1_5/qwen1_5_110b_chat/README.md b/xtuner/configs/qwen/qwen1_5/qwen1_5_110b_chat/README.md
new file mode 100644
index 000000000..fc78ad510
--- /dev/null
+++ b/xtuner/configs/qwen/qwen1_5/qwen1_5_110b_chat/README.md
@@ -0,0 +1,26 @@
+# Qwen 110B
+
+## Install
+
+```bash
+# Install the latest xtuner
+pip install -U 'xtuner[deepspeed]'
+
+# We recommend installing flash_attn
+# pip install flash-attn
+
+# install the latest transformers
+pip install -U transformers
+```
+
+## QLoRA Fine-tune
+
+Training Qwen 110B with 32k context capability requires only 2 * A100 80G.
+
+```bash
+xtuner train xtuner/configs/qwen/qwen1_5/qwen1_5_110b_chat/qwen1_5_110b_chat_qlora_alpaca_e3_16k_2gpus.py --deepspeed deepspeed_zero3
+```
+
+
+

+
diff --git a/xtuner/configs/qwen/qwen1_5/qwen1_5_110b_chat/qwen1_5_110b_chat_full_alpaca_e3.py b/xtuner/configs/qwen/qwen1_5/qwen1_5_110b_chat/qwen1_5_110b_chat_full_alpaca_e3.py
new file mode 100644
index 000000000..9e16cc04d
--- /dev/null
+++ b/xtuner/configs/qwen/qwen1_5/qwen1_5_110b_chat/qwen1_5_110b_chat_full_alpaca_e3.py
@@ -0,0 +1,203 @@
+# Copyright (c) OpenMMLab. All rights reserved.
+from datasets import load_dataset
+from mmengine.dataset import DefaultSampler
+from mmengine.hooks import (CheckpointHook, DistSamplerSeedHook, IterTimerHook,
+ LoggerHook, ParamSchedulerHook)
+from mmengine.optim import AmpOptimWrapper, CosineAnnealingLR, LinearLR
+from torch.optim import AdamW
+from transformers import AutoModelForCausalLM, AutoTokenizer
+
+from xtuner.dataset import process_hf_dataset
+from xtuner.dataset.collate_fns import default_collate_fn
+from xtuner.dataset.map_fns import alpaca_map_fn, template_map_fn_factory
+from xtuner.engine.hooks import (DatasetInfoHook, EvaluateChatHook,
+ ThroughputHook,
+ VarlenAttnArgsToMessageHubHook)
+from xtuner.engine.runner import TrainLoop
+from xtuner.model import SupervisedFinetune
+from xtuner.parallel.sequence import SequenceParallelSampler
+from xtuner.utils import PROMPT_TEMPLATE, SYSTEM_TEMPLATE
+
+#######################################################################
+# PART 1 Settings #
+#######################################################################
+# Model
+pretrained_model_name_or_path = 'Qwen/Qwen1.5-110B-Chat'
+use_varlen_attn = False
+
+# Data
+alpaca_en_path = 'tatsu-lab/alpaca'
+prompt_template = PROMPT_TEMPLATE.qwen_chat
+max_length = 2048
+pack_to_max_length = True
+
+# parallel
+sequence_parallel_size = 1
+
+# Scheduler & Optimizer
+batch_size = 1 # per_device
+# total batch = 32gpus * batch_size_per_device 1 * acc 1 = 32
+accumulative_counts = 1
+accumulative_counts *= sequence_parallel_size
+dataloader_num_workers = 4
+max_epochs = 3
+optim_type = AdamW
+lr = 1e-5
+betas = (0.9, 0.999)
+weight_decay = 0
+max_norm = 1 # grad clip
+warmup_ratio = 0.03
+
+# Save
+save_steps = 500
+save_total_limit = 2 # Maximum checkpoints to keep (-1 means unlimited)
+
+# Evaluate the generation performance during the training
+evaluation_freq = 500
+SYSTEM = SYSTEM_TEMPLATE.alpaca
+evaluation_inputs = [
+ '请给我介绍五个上海的景点', 'Please tell me five scenic spots in Shanghai'
+]
+
+#######################################################################
+# PART 2 Model & Tokenizer #
+#######################################################################
+tokenizer = dict(
+ type=AutoTokenizer.from_pretrained,
+ pretrained_model_name_or_path=pretrained_model_name_or_path,
+ trust_remote_code=True,
+ padding_side='right')
+
+model = dict(
+ type=SupervisedFinetune,
+ use_varlen_attn=use_varlen_attn,
+ llm=dict(
+ type=AutoModelForCausalLM.from_pretrained,
+ pretrained_model_name_or_path=pretrained_model_name_or_path,
+ trust_remote_code=True))
+
+#######################################################################
+# PART 3 Dataset & Dataloader #
+#######################################################################
+alpaca_en = dict(
+ type=process_hf_dataset,
+ dataset=dict(type=load_dataset, path=alpaca_en_path),
+ tokenizer=tokenizer,
+ max_length=max_length,
+ dataset_map_fn=alpaca_map_fn,
+ template_map_fn=dict(
+ type=template_map_fn_factory, template=prompt_template),
+ remove_unused_columns=True,
+ shuffle_before_pack=True,
+ pack_to_max_length=pack_to_max_length,
+ use_varlen_attn=use_varlen_attn)
+
+sampler = SequenceParallelSampler \
+ if sequence_parallel_size > 1 else DefaultSampler
+
+train_dataloader = dict(
+ batch_size=batch_size,
+ num_workers=dataloader_num_workers,
+ dataset=alpaca_en,
+ sampler=dict(type=sampler, shuffle=True),
+ collate_fn=dict(type=default_collate_fn, use_varlen_attn=use_varlen_attn))
+
+#######################################################################
+# PART 4 Scheduler & Optimizer #
+#######################################################################
+# optimizer
+optim_wrapper = dict(
+ type=AmpOptimWrapper,
+ optimizer=dict(
+ type=optim_type, lr=lr, betas=betas, weight_decay=weight_decay),
+ clip_grad=dict(max_norm=max_norm, error_if_nonfinite=False),
+ accumulative_counts=accumulative_counts,
+ loss_scale='dynamic',
+ dtype='float16')
+
+# learning policy
+# More information: https://github.com/open-mmlab/mmengine/blob/main/docs/en/tutorials/param_scheduler.md # noqa: E501
+param_scheduler = [
+ dict(
+ type=LinearLR,
+ start_factor=1e-5,
+ by_epoch=True,
+ begin=0,
+ end=warmup_ratio * max_epochs,
+ convert_to_iter_based=True),
+ dict(
+ type=CosineAnnealingLR,
+ eta_min=0.0,
+ by_epoch=True,
+ begin=warmup_ratio * max_epochs,
+ end=max_epochs,
+ convert_to_iter_based=True)
+]
+
+# train, val, test setting
+train_cfg = dict(type=TrainLoop, max_epochs=max_epochs)
+
+#######################################################################
+# PART 5 Runtime #
+#######################################################################
+# Log the dialogue periodically during the training process, optional
+custom_hooks = [
+ dict(type=DatasetInfoHook, tokenizer=tokenizer),
+ dict(
+ type=EvaluateChatHook,
+ tokenizer=tokenizer,
+ every_n_iters=evaluation_freq,
+ evaluation_inputs=evaluation_inputs,
+ system=SYSTEM,
+ prompt_template=prompt_template),
+ dict(type=ThroughputHook)
+]
+
+if use_varlen_attn:
+ custom_hooks += [dict(type=VarlenAttnArgsToMessageHubHook)]
+
+# configure default hooks
+default_hooks = dict(
+ # record the time of every iteration.
+ timer=dict(type=IterTimerHook),
+ # print log every 10 iterations.
+ logger=dict(type=LoggerHook, log_metric_by_epoch=False, interval=10),
+ # enable the parameter scheduler.
+ param_scheduler=dict(type=ParamSchedulerHook),
+ # save checkpoint per `save_steps`.
+ checkpoint=dict(
+ type=CheckpointHook,
+ by_epoch=False,
+ interval=save_steps,
+ max_keep_ckpts=save_total_limit),
+ # set sampler seed in distributed evrionment.
+ sampler_seed=dict(type=DistSamplerSeedHook),
+)
+
+# configure environment
+env_cfg = dict(
+ # whether to enable cudnn benchmark
+ cudnn_benchmark=False,
+ # set multi process parameters
+ mp_cfg=dict(mp_start_method='fork', opencv_num_threads=0),
+ # set distributed parameters
+ dist_cfg=dict(backend='nccl'),
+)
+
+# set visualizer
+visualizer = None
+
+# set log level
+log_level = 'INFO'
+
+# load from which checkpoint
+load_from = None
+
+# whether to resume training from the loaded checkpoint
+resume = False
+
+# Defaults to use random seed and disable `deterministic`
+randomness = dict(seed=None, deterministic=False)
+
+# set log processor
+log_processor = dict(by_epoch=False)
diff --git a/xtuner/configs/qwen/qwen1_5/qwen1_5_110b_chat/qwen1_5_110b_chat_qlora_alpaca_e3.py b/xtuner/configs/qwen/qwen1_5/qwen1_5_110b_chat/qwen1_5_110b_chat_qlora_alpaca_e3.py
new file mode 100644
index 000000000..2abcf1d72
--- /dev/null
+++ b/xtuner/configs/qwen/qwen1_5/qwen1_5_110b_chat/qwen1_5_110b_chat_qlora_alpaca_e3.py
@@ -0,0 +1,223 @@
+# Copyright (c) OpenMMLab. All rights reserved.
+import torch
+from datasets import load_dataset
+from mmengine.dataset import DefaultSampler
+from mmengine.hooks import (CheckpointHook, DistSamplerSeedHook, IterTimerHook,
+ LoggerHook, ParamSchedulerHook)
+from mmengine.optim import AmpOptimWrapper, CosineAnnealingLR, LinearLR
+from peft import LoraConfig
+from torch.optim import AdamW
+from transformers import (AutoModelForCausalLM, AutoTokenizer,
+ BitsAndBytesConfig)
+
+from xtuner.dataset import process_hf_dataset
+from xtuner.dataset.collate_fns import default_collate_fn
+from xtuner.dataset.map_fns import alpaca_map_fn, template_map_fn_factory
+from xtuner.engine.hooks import (DatasetInfoHook, EvaluateChatHook,
+ ThroughputHook,
+ VarlenAttnArgsToMessageHubHook)
+from xtuner.engine.runner import TrainLoop
+from xtuner.model import SupervisedFinetune
+from xtuner.parallel.sequence import SequenceParallelSampler
+from xtuner.utils import PROMPT_TEMPLATE, SYSTEM_TEMPLATE
+
+#######################################################################
+# PART 1 Settings #
+#######################################################################
+# Model
+pretrained_model_name_or_path = 'Qwen/Qwen1.5-110B-Chat'
+use_varlen_attn = False
+
+# Data
+alpaca_en_path = 'tatsu-lab/alpaca'
+prompt_template = PROMPT_TEMPLATE.qwen_chat
+max_length = 2048
+pack_to_max_length = True
+
+# parallel
+sequence_parallel_size = 1
+
+# Scheduler & Optimizer
+batch_size = 1 # per_device
+accumulative_counts = 1 # total bs = 1 bs_per_device * 8 gpus * 1 acc = 8
+accumulative_counts *= sequence_parallel_size
+dataloader_num_workers = 0
+max_epochs = 3
+optim_type = AdamW
+lr = 1e-4 # 110B model use smaller lr
+betas = (0.9, 0.999)
+weight_decay = 0
+max_norm = 1 # grad clip
+warmup_ratio = 0.03
+
+# Save
+save_steps = 500
+save_total_limit = 2 # Maximum checkpoints to keep (-1 means unlimited)
+
+# Evaluate the generation performance during the training
+evaluation_freq = 500
+SYSTEM = SYSTEM_TEMPLATE.alpaca
+evaluation_inputs = [
+ '请给我介绍五个上海的景点', 'Please tell me five scenic spots in Shanghai'
+]
+
+#######################################################################
+# PART 2 Model & Tokenizer #
+#######################################################################
+tokenizer = dict(
+ type=AutoTokenizer.from_pretrained,
+ pretrained_model_name_or_path=pretrained_model_name_or_path,
+ trust_remote_code=True,
+ padding_side='right')
+
+model = dict(
+ type=SupervisedFinetune,
+ use_varlen_attn=use_varlen_attn,
+ llm=dict(
+ type=AutoModelForCausalLM.from_pretrained,
+ pretrained_model_name_or_path=pretrained_model_name_or_path,
+ trust_remote_code=True,
+ torch_dtype=torch.float16,
+ quantization_config=dict(
+ type=BitsAndBytesConfig,
+ load_in_4bit=True,
+ load_in_8bit=False,
+ llm_int8_threshold=6.0,
+ llm_int8_has_fp16_weight=False,
+ bnb_4bit_compute_dtype=torch.float16,
+ bnb_4bit_use_double_quant=True,
+ bnb_4bit_quant_type='nf4',
+ bnb_4bit_quant_storage=torch.float16)),
+ lora=dict(
+ type=LoraConfig,
+ r=64,
+ lora_alpha=16,
+ lora_dropout=0.1,
+ bias='none',
+ task_type='CAUSAL_LM'))
+
+#######################################################################
+# PART 3 Dataset & Dataloader #
+#######################################################################
+alpaca_en = dict(
+ type=process_hf_dataset,
+ dataset=dict(type=load_dataset, path=alpaca_en_path),
+ tokenizer=tokenizer,
+ max_length=max_length,
+ dataset_map_fn=alpaca_map_fn,
+ template_map_fn=dict(
+ type=template_map_fn_factory, template=prompt_template),
+ remove_unused_columns=True,
+ shuffle_before_pack=True,
+ pack_to_max_length=pack_to_max_length,
+ use_varlen_attn=use_varlen_attn)
+
+sampler = SequenceParallelSampler \
+ if sequence_parallel_size > 1 else DefaultSampler
+
+train_dataloader = dict(
+ batch_size=batch_size,
+ num_workers=dataloader_num_workers,
+ dataset=alpaca_en,
+ sampler=dict(type=sampler, shuffle=True),
+ collate_fn=dict(type=default_collate_fn, use_varlen_attn=use_varlen_attn))
+
+#######################################################################
+# PART 4 Scheduler & Optimizer #
+#######################################################################
+# optimizer
+optim_wrapper = dict(
+ type=AmpOptimWrapper,
+ optimizer=dict(
+ type=optim_type, lr=lr, betas=betas, weight_decay=weight_decay),
+ clip_grad=dict(max_norm=max_norm, error_if_nonfinite=False),
+ accumulative_counts=accumulative_counts,
+ loss_scale='dynamic',
+ dtype='float16')
+
+# learning policy
+# More information: https://github.com/open-mmlab/mmengine/blob/main/docs/en/tutorials/param_scheduler.md # noqa: E501
+param_scheduler = [
+ dict(
+ type=LinearLR,
+ start_factor=1e-5,
+ by_epoch=True,
+ begin=0,
+ end=warmup_ratio * max_epochs,
+ convert_to_iter_based=True),
+ dict(
+ type=CosineAnnealingLR,
+ eta_min=0.0,
+ by_epoch=True,
+ begin=warmup_ratio * max_epochs,
+ end=max_epochs,
+ convert_to_iter_based=True)
+]
+
+# train, val, test setting
+train_cfg = dict(type=TrainLoop, max_epochs=max_epochs)
+
+#######################################################################
+# PART 5 Runtime #
+#######################################################################
+# Log the dialogue periodically during the training process, optional
+custom_hooks = [
+ dict(type=DatasetInfoHook, tokenizer=tokenizer),
+ dict(type=ThroughputHook),
+ dict(
+ type=EvaluateChatHook,
+ tokenizer=tokenizer,
+ every_n_iters=evaluation_freq,
+ evaluation_inputs=evaluation_inputs,
+ system=SYSTEM,
+ prompt_template=prompt_template)
+]
+
+if use_varlen_attn:
+ custom_hooks += [dict(type=VarlenAttnArgsToMessageHubHook)]
+
+# configure default hooks
+default_hooks = dict(
+ # record the time of every iteration.
+ timer=dict(type=IterTimerHook),
+ # print log every 10 iterations.
+ logger=dict(type=LoggerHook, log_metric_by_epoch=False, interval=10),
+ # enable the parameter scheduler.
+ param_scheduler=dict(type=ParamSchedulerHook),
+ # save checkpoint per `save_steps`.
+ checkpoint=dict(
+ type=CheckpointHook,
+ by_epoch=False,
+ interval=save_steps,
+ max_keep_ckpts=save_total_limit),
+ # set sampler seed in distributed evrionment.
+ sampler_seed=dict(type=DistSamplerSeedHook),
+)
+
+# configure environment
+env_cfg = dict(
+ # whether to enable cudnn benchmark
+ cudnn_benchmark=False,
+ # set multi process parameters
+ mp_cfg=dict(mp_start_method='fork', opencv_num_threads=0),
+ # set distributed parameters
+ dist_cfg=dict(backend='nccl'),
+)
+
+# set visualizer
+visualizer = None
+
+# set log level
+log_level = 'INFO'
+
+# load from which checkpoint
+load_from = None
+
+# whether to resume training from the loaded checkpoint
+resume = False
+
+# Defaults to use random seed and disable `deterministic`
+randomness = dict(seed=None, deterministic=False)
+
+# set log processor
+log_processor = dict(by_epoch=False)
diff --git a/xtuner/configs/qwen/qwen1_5/qwen1_5_110b_chat/qwen1_5_110b_chat_qlora_alpaca_e3_16k_2gpus.py b/xtuner/configs/qwen/qwen1_5/qwen1_5_110b_chat/qwen1_5_110b_chat_qlora_alpaca_e3_16k_2gpus.py
new file mode 100644
index 000000000..ef8c7b6e6
--- /dev/null
+++ b/xtuner/configs/qwen/qwen1_5/qwen1_5_110b_chat/qwen1_5_110b_chat_qlora_alpaca_e3_16k_2gpus.py
@@ -0,0 +1,223 @@
+# Copyright (c) OpenMMLab. All rights reserved.
+import torch
+from datasets import load_dataset
+from mmengine.dataset import DefaultSampler
+from mmengine.hooks import (CheckpointHook, DistSamplerSeedHook, IterTimerHook,
+ LoggerHook, ParamSchedulerHook)
+from mmengine.optim import AmpOptimWrapper, CosineAnnealingLR, LinearLR
+from peft import LoraConfig
+from torch.optim import AdamW
+from transformers import (AutoModelForCausalLM, AutoTokenizer,
+ BitsAndBytesConfig)
+
+from xtuner.dataset import process_hf_dataset
+from xtuner.dataset.collate_fns import default_collate_fn
+from xtuner.dataset.map_fns import alpaca_map_fn, template_map_fn_factory
+from xtuner.engine.hooks import (DatasetInfoHook, EvaluateChatHook,
+ ThroughputHook,
+ VarlenAttnArgsToMessageHubHook)
+from xtuner.engine.runner import TrainLoop
+from xtuner.model import SupervisedFinetune
+from xtuner.parallel.sequence import SequenceParallelSampler
+from xtuner.utils import PROMPT_TEMPLATE, SYSTEM_TEMPLATE
+
+#######################################################################
+# PART 1 Settings #
+#######################################################################
+# Model
+pretrained_model_name_or_path = 'Qwen/Qwen1.5-110B-Chat'
+use_varlen_attn = False
+
+# Data
+alpaca_en_path = 'tatsu-lab/alpaca'
+prompt_template = PROMPT_TEMPLATE.qwen_chat
+max_length = 16384
+pack_to_max_length = True
+
+# parallel
+sequence_parallel_size = 2
+
+# Scheduler & Optimizer
+batch_size = 1 # per_device
+accumulative_counts = 1 # total bs = 1 bs_per_device * 2 gpus * 1 acc = 2
+accumulative_counts *= sequence_parallel_size
+dataloader_num_workers = 0
+max_epochs = 3
+optim_type = AdamW
+lr = 1e-4 # 110B model use smaller lr
+betas = (0.9, 0.999)
+weight_decay = 0
+max_norm = 1 # grad clip
+warmup_ratio = 0.03
+
+# Save
+save_steps = 500
+save_total_limit = 2 # Maximum checkpoints to keep (-1 means unlimited)
+
+# Evaluate the generation performance during the training
+evaluation_freq = 50
+SYSTEM = SYSTEM_TEMPLATE.alpaca
+evaluation_inputs = [
+ '请给我介绍五个上海的景点', 'Please tell me five scenic spots in Shanghai'
+]
+
+#######################################################################
+# PART 2 Model & Tokenizer #
+#######################################################################
+tokenizer = dict(
+ type=AutoTokenizer.from_pretrained,
+ pretrained_model_name_or_path=pretrained_model_name_or_path,
+ trust_remote_code=True,
+ padding_side='right')
+
+model = dict(
+ type=SupervisedFinetune,
+ use_varlen_attn=use_varlen_attn,
+ llm=dict(
+ type=AutoModelForCausalLM.from_pretrained,
+ pretrained_model_name_or_path=pretrained_model_name_or_path,
+ trust_remote_code=True,
+ torch_dtype=torch.float16,
+ quantization_config=dict(
+ type=BitsAndBytesConfig,
+ load_in_4bit=True,
+ load_in_8bit=False,
+ llm_int8_threshold=6.0,
+ llm_int8_has_fp16_weight=False,
+ bnb_4bit_compute_dtype=torch.float16,
+ bnb_4bit_use_double_quant=True,
+ bnb_4bit_quant_type='nf4',
+ bnb_4bit_quant_storage=torch.float16)),
+ lora=dict(
+ type=LoraConfig,
+ r=64,
+ lora_alpha=16,
+ lora_dropout=0.1,
+ bias='none',
+ task_type='CAUSAL_LM'))
+
+#######################################################################
+# PART 3 Dataset & Dataloader #
+#######################################################################
+alpaca_en = dict(
+ type=process_hf_dataset,
+ dataset=dict(type=load_dataset, path=alpaca_en_path),
+ tokenizer=tokenizer,
+ max_length=max_length,
+ dataset_map_fn=alpaca_map_fn,
+ template_map_fn=dict(
+ type=template_map_fn_factory, template=prompt_template),
+ remove_unused_columns=True,
+ shuffle_before_pack=True,
+ pack_to_max_length=pack_to_max_length,
+ use_varlen_attn=use_varlen_attn)
+
+sampler = SequenceParallelSampler \
+ if sequence_parallel_size > 1 else DefaultSampler
+
+train_dataloader = dict(
+ batch_size=batch_size,
+ num_workers=dataloader_num_workers,
+ dataset=alpaca_en,
+ sampler=dict(type=sampler, shuffle=True),
+ collate_fn=dict(type=default_collate_fn, use_varlen_attn=use_varlen_attn))
+
+#######################################################################
+# PART 4 Scheduler & Optimizer #
+#######################################################################
+# optimizer
+optim_wrapper = dict(
+ type=AmpOptimWrapper,
+ optimizer=dict(
+ type=optim_type, lr=lr, betas=betas, weight_decay=weight_decay),
+ clip_grad=dict(max_norm=max_norm, error_if_nonfinite=False),
+ accumulative_counts=accumulative_counts,
+ loss_scale='dynamic',
+ dtype='float16')
+
+# learning policy
+# More information: https://github.com/open-mmlab/mmengine/blob/main/docs/en/tutorials/param_scheduler.md # noqa: E501
+param_scheduler = [
+ dict(
+ type=LinearLR,
+ start_factor=1e-5,
+ by_epoch=True,
+ begin=0,
+ end=warmup_ratio * max_epochs,
+ convert_to_iter_based=True),
+ dict(
+ type=CosineAnnealingLR,
+ eta_min=0.0,
+ by_epoch=True,
+ begin=warmup_ratio * max_epochs,
+ end=max_epochs,
+ convert_to_iter_based=True)
+]
+
+# train, val, test setting
+train_cfg = dict(type=TrainLoop, max_epochs=max_epochs)
+
+#######################################################################
+# PART 5 Runtime #
+#######################################################################
+# Log the dialogue periodically during the training process, optional
+custom_hooks = [
+ dict(type=DatasetInfoHook, tokenizer=tokenizer),
+ dict(type=ThroughputHook),
+ dict(
+ type=EvaluateChatHook,
+ tokenizer=tokenizer,
+ every_n_iters=evaluation_freq,
+ evaluation_inputs=evaluation_inputs,
+ system=SYSTEM,
+ prompt_template=prompt_template)
+]
+
+if use_varlen_attn:
+ custom_hooks += [dict(type=VarlenAttnArgsToMessageHubHook)]
+
+# configure default hooks
+default_hooks = dict(
+ # record the time of every iteration.
+ timer=dict(type=IterTimerHook),
+ # print log every 10 iterations.
+ logger=dict(type=LoggerHook, log_metric_by_epoch=False, interval=1),
+ # enable the parameter scheduler.
+ param_scheduler=dict(type=ParamSchedulerHook),
+ # save checkpoint per `save_steps`.
+ checkpoint=dict(
+ type=CheckpointHook,
+ by_epoch=False,
+ interval=save_steps,
+ max_keep_ckpts=save_total_limit),
+ # set sampler seed in distributed evrionment.
+ sampler_seed=dict(type=DistSamplerSeedHook),
+)
+
+# configure environment
+env_cfg = dict(
+ # whether to enable cudnn benchmark
+ cudnn_benchmark=False,
+ # set multi process parameters
+ mp_cfg=dict(mp_start_method='fork', opencv_num_threads=0),
+ # set distributed parameters
+ dist_cfg=dict(backend='nccl'),
+)
+
+# set visualizer
+visualizer = None
+
+# set log level
+log_level = 'INFO'
+
+# load from which checkpoint
+load_from = None
+
+# whether to resume training from the loaded checkpoint
+resume = False
+
+# Defaults to use random seed and disable `deterministic`
+randomness = dict(seed=None, deterministic=False)
+
+# set log processor
+log_processor = dict(by_epoch=False, window_size=1)
diff --git a/xtuner/configs/qwen/qwen1_5/qwen1_5_14b/qwen1_5_14b_full_alpaca_e3.py b/xtuner/configs/qwen/qwen1_5/qwen1_5_14b/qwen1_5_14b_full_alpaca_e3.py
index ed03fa8d6..ff77e391f 100644
--- a/xtuner/configs/qwen/qwen1_5/qwen1_5_14b/qwen1_5_14b_full_alpaca_e3.py
+++ b/xtuner/configs/qwen/qwen1_5/qwen1_5_14b/qwen1_5_14b_full_alpaca_e3.py
@@ -14,6 +14,7 @@
VarlenAttnArgsToMessageHubHook)
from xtuner.engine.runner import TrainLoop
from xtuner.model import SupervisedFinetune
+from xtuner.parallel.sequence import SequenceParallelSampler
from xtuner.utils import PROMPT_TEMPLATE, SYSTEM_TEMPLATE
#######################################################################
@@ -29,9 +30,13 @@
max_length = 2048
pack_to_max_length = True
+# parallel
+sequence_parallel_size = 1
+
# Scheduler & Optimizer
batch_size = 1 # per_device
accumulative_counts = 16
+accumulative_counts *= sequence_parallel_size
dataloader_num_workers = 0
max_epochs = 3
optim_type = AdamW
@@ -85,11 +90,14 @@
pack_to_max_length=pack_to_max_length,
use_varlen_attn=use_varlen_attn)
+sampler = SequenceParallelSampler \
+ if sequence_parallel_size > 1 else DefaultSampler
+
train_dataloader = dict(
batch_size=batch_size,
num_workers=dataloader_num_workers,
dataset=alpaca_en,
- sampler=dict(type=DefaultSampler, shuffle=True),
+ sampler=dict(type=sampler, shuffle=True),
collate_fn=dict(type=default_collate_fn, use_varlen_attn=use_varlen_attn))
#######################################################################
diff --git a/xtuner/configs/qwen/qwen1_5/qwen1_5_14b/qwen1_5_14b_qlora_alpaca_e3.py b/xtuner/configs/qwen/qwen1_5/qwen1_5_14b/qwen1_5_14b_qlora_alpaca_e3.py
index b59a6f58c..dc2acd8b2 100644
--- a/xtuner/configs/qwen/qwen1_5/qwen1_5_14b/qwen1_5_14b_qlora_alpaca_e3.py
+++ b/xtuner/configs/qwen/qwen1_5/qwen1_5_14b/qwen1_5_14b_qlora_alpaca_e3.py
@@ -17,6 +17,7 @@
VarlenAttnArgsToMessageHubHook)
from xtuner.engine.runner import TrainLoop
from xtuner.model import SupervisedFinetune
+from xtuner.parallel.sequence import SequenceParallelSampler
from xtuner.utils import PROMPT_TEMPLATE, SYSTEM_TEMPLATE
#######################################################################
@@ -32,9 +33,13 @@
max_length = 2048
pack_to_max_length = True
+# parallel
+sequence_parallel_size = 1
+
# Scheduler & Optimizer
batch_size = 1 # per_device
accumulative_counts = 16
+accumulative_counts *= sequence_parallel_size
dataloader_num_workers = 0
max_epochs = 3
optim_type = AdamW
@@ -105,11 +110,14 @@
pack_to_max_length=pack_to_max_length,
use_varlen_attn=use_varlen_attn)
+sampler = SequenceParallelSampler \
+ if sequence_parallel_size > 1 else DefaultSampler
+
train_dataloader = dict(
batch_size=batch_size,
num_workers=dataloader_num_workers,
dataset=alpaca_en,
- sampler=dict(type=DefaultSampler, shuffle=True),
+ sampler=dict(type=sampler, shuffle=True),
collate_fn=dict(type=default_collate_fn, use_varlen_attn=use_varlen_attn))
#######################################################################
diff --git a/xtuner/configs/qwen/qwen1_5/qwen1_5_14b_chat/qwen1_5_14b_chat_full_alpaca_e3.py b/xtuner/configs/qwen/qwen1_5/qwen1_5_14b_chat/qwen1_5_14b_chat_full_alpaca_e3.py
index 424ec61b7..c217888b3 100644
--- a/xtuner/configs/qwen/qwen1_5/qwen1_5_14b_chat/qwen1_5_14b_chat_full_alpaca_e3.py
+++ b/xtuner/configs/qwen/qwen1_5/qwen1_5_14b_chat/qwen1_5_14b_chat_full_alpaca_e3.py
@@ -14,6 +14,7 @@
VarlenAttnArgsToMessageHubHook)
from xtuner.engine.runner import TrainLoop
from xtuner.model import SupervisedFinetune
+from xtuner.parallel.sequence import SequenceParallelSampler
from xtuner.utils import PROMPT_TEMPLATE, SYSTEM_TEMPLATE
#######################################################################
@@ -29,9 +30,13 @@
max_length = 2048
pack_to_max_length = True
+# parallel
+sequence_parallel_size = 1
+
# Scheduler & Optimizer
batch_size = 1 # per_device
accumulative_counts = 16
+accumulative_counts *= sequence_parallel_size
dataloader_num_workers = 0
max_epochs = 3
optim_type = AdamW
@@ -85,11 +90,14 @@
pack_to_max_length=pack_to_max_length,
use_varlen_attn=use_varlen_attn)
+sampler = SequenceParallelSampler \
+ if sequence_parallel_size > 1 else DefaultSampler
+
train_dataloader = dict(
batch_size=batch_size,
num_workers=dataloader_num_workers,
dataset=alpaca_en,
- sampler=dict(type=DefaultSampler, shuffle=True),
+ sampler=dict(type=sampler, shuffle=True),
collate_fn=dict(type=default_collate_fn, use_varlen_attn=use_varlen_attn))
#######################################################################
diff --git a/xtuner/configs/qwen/qwen1_5/qwen1_5_14b_chat/qwen1_5_14b_chat_qlora_alpaca_e3.py b/xtuner/configs/qwen/qwen1_5/qwen1_5_14b_chat/qwen1_5_14b_chat_qlora_alpaca_e3.py
index 037f8d662..36cff5aac 100644
--- a/xtuner/configs/qwen/qwen1_5/qwen1_5_14b_chat/qwen1_5_14b_chat_qlora_alpaca_e3.py
+++ b/xtuner/configs/qwen/qwen1_5/qwen1_5_14b_chat/qwen1_5_14b_chat_qlora_alpaca_e3.py
@@ -17,6 +17,7 @@
VarlenAttnArgsToMessageHubHook)
from xtuner.engine.runner import TrainLoop
from xtuner.model import SupervisedFinetune
+from xtuner.parallel.sequence import SequenceParallelSampler
from xtuner.utils import PROMPT_TEMPLATE, SYSTEM_TEMPLATE
#######################################################################
@@ -32,9 +33,13 @@
max_length = 2048
pack_to_max_length = True
+# parallel
+sequence_parallel_size = 1
+
# Scheduler & Optimizer
batch_size = 1 # per_device
accumulative_counts = 16
+accumulative_counts *= sequence_parallel_size
dataloader_num_workers = 0
max_epochs = 3
optim_type = AdamW
@@ -105,11 +110,14 @@
pack_to_max_length=pack_to_max_length,
use_varlen_attn=use_varlen_attn)
+sampler = SequenceParallelSampler \
+ if sequence_parallel_size > 1 else DefaultSampler
+
train_dataloader = dict(
batch_size=batch_size,
num_workers=dataloader_num_workers,
dataset=alpaca_en,
- sampler=dict(type=DefaultSampler, shuffle=True),
+ sampler=dict(type=sampler, shuffle=True),
collate_fn=dict(type=default_collate_fn, use_varlen_attn=use_varlen_attn))
#######################################################################
diff --git a/xtuner/configs/qwen/qwen1_5/qwen1_5_1_8b/qwen1_5_1_8b_full_alpaca_e3.py b/xtuner/configs/qwen/qwen1_5/qwen1_5_1_8b/qwen1_5_1_8b_full_alpaca_e3.py
index 522d6e0a2..4afdc0a75 100644
--- a/xtuner/configs/qwen/qwen1_5/qwen1_5_1_8b/qwen1_5_1_8b_full_alpaca_e3.py
+++ b/xtuner/configs/qwen/qwen1_5/qwen1_5_1_8b/qwen1_5_1_8b_full_alpaca_e3.py
@@ -14,6 +14,7 @@
VarlenAttnArgsToMessageHubHook)
from xtuner.engine.runner import TrainLoop
from xtuner.model import SupervisedFinetune
+from xtuner.parallel.sequence import SequenceParallelSampler
from xtuner.utils import PROMPT_TEMPLATE, SYSTEM_TEMPLATE
#######################################################################
@@ -29,9 +30,13 @@
max_length = 2048
pack_to_max_length = True
+# parallel
+sequence_parallel_size = 1
+
# Scheduler & Optimizer
batch_size = 1 # per_device
accumulative_counts = 16
+accumulative_counts *= sequence_parallel_size
dataloader_num_workers = 0
max_epochs = 3
optim_type = AdamW
@@ -85,11 +90,14 @@
pack_to_max_length=pack_to_max_length,
use_varlen_attn=use_varlen_attn)
+sampler = SequenceParallelSampler \
+ if sequence_parallel_size > 1 else DefaultSampler
+
train_dataloader = dict(
batch_size=batch_size,
num_workers=dataloader_num_workers,
dataset=alpaca_en,
- sampler=dict(type=DefaultSampler, shuffle=True),
+ sampler=dict(type=sampler, shuffle=True),
collate_fn=dict(type=default_collate_fn, use_varlen_attn=use_varlen_attn))
#######################################################################
diff --git a/xtuner/configs/qwen/qwen1_5/qwen1_5_1_8b/qwen1_5_1_8b_qlora_alpaca_e3.py b/xtuner/configs/qwen/qwen1_5/qwen1_5_1_8b/qwen1_5_1_8b_qlora_alpaca_e3.py
index f5ec81e85..a4687d7ae 100644
--- a/xtuner/configs/qwen/qwen1_5/qwen1_5_1_8b/qwen1_5_1_8b_qlora_alpaca_e3.py
+++ b/xtuner/configs/qwen/qwen1_5/qwen1_5_1_8b/qwen1_5_1_8b_qlora_alpaca_e3.py
@@ -17,6 +17,7 @@
VarlenAttnArgsToMessageHubHook)
from xtuner.engine.runner import TrainLoop
from xtuner.model import SupervisedFinetune
+from xtuner.parallel.sequence import SequenceParallelSampler
from xtuner.utils import PROMPT_TEMPLATE, SYSTEM_TEMPLATE
#######################################################################
@@ -32,9 +33,13 @@
max_length = 2048
pack_to_max_length = True
+# parallel
+sequence_parallel_size = 1
+
# Scheduler & Optimizer
batch_size = 1 # per_device
accumulative_counts = 16
+accumulative_counts *= sequence_parallel_size
dataloader_num_workers = 0
max_epochs = 3
optim_type = AdamW
@@ -105,11 +110,14 @@
pack_to_max_length=pack_to_max_length,
use_varlen_attn=use_varlen_attn)
+sampler = SequenceParallelSampler \
+ if sequence_parallel_size > 1 else DefaultSampler
+
train_dataloader = dict(
batch_size=batch_size,
num_workers=dataloader_num_workers,
dataset=alpaca_en,
- sampler=dict(type=DefaultSampler, shuffle=True),
+ sampler=dict(type=sampler, shuffle=True),
collate_fn=dict(type=default_collate_fn, use_varlen_attn=use_varlen_attn))
#######################################################################
diff --git a/xtuner/configs/qwen/qwen1_5/qwen1_5_1_8b_chat/qwen1_5_1_8b_chat_full_alpaca_e3.py b/xtuner/configs/qwen/qwen1_5/qwen1_5_1_8b_chat/qwen1_5_1_8b_chat_full_alpaca_e3.py
index 9d9c31b72..2ef12cb79 100644
--- a/xtuner/configs/qwen/qwen1_5/qwen1_5_1_8b_chat/qwen1_5_1_8b_chat_full_alpaca_e3.py
+++ b/xtuner/configs/qwen/qwen1_5/qwen1_5_1_8b_chat/qwen1_5_1_8b_chat_full_alpaca_e3.py
@@ -14,6 +14,7 @@
VarlenAttnArgsToMessageHubHook)
from xtuner.engine.runner import TrainLoop
from xtuner.model import SupervisedFinetune
+from xtuner.parallel.sequence import SequenceParallelSampler
from xtuner.utils import PROMPT_TEMPLATE, SYSTEM_TEMPLATE
#######################################################################
@@ -29,9 +30,13 @@
max_length = 2048
pack_to_max_length = True
+# parallel
+sequence_parallel_size = 1
+
# Scheduler & Optimizer
batch_size = 1 # per_device
accumulative_counts = 16
+accumulative_counts *= sequence_parallel_size
dataloader_num_workers = 0
max_epochs = 3
optim_type = AdamW
@@ -85,11 +90,14 @@
pack_to_max_length=pack_to_max_length,
use_varlen_attn=use_varlen_attn)
+sampler = SequenceParallelSampler \
+ if sequence_parallel_size > 1 else DefaultSampler
+
train_dataloader = dict(
batch_size=batch_size,
num_workers=dataloader_num_workers,
dataset=alpaca_en,
- sampler=dict(type=DefaultSampler, shuffle=True),
+ sampler=dict(type=sampler, shuffle=True),
collate_fn=dict(type=default_collate_fn, use_varlen_attn=use_varlen_attn))
#######################################################################
diff --git a/xtuner/configs/qwen/qwen1_5/qwen1_5_1_8b_chat/qwen1_5_1_8b_chat_qlora_alpaca_e3.py b/xtuner/configs/qwen/qwen1_5/qwen1_5_1_8b_chat/qwen1_5_1_8b_chat_qlora_alpaca_e3.py
index a464ad1ab..804bbbf96 100644
--- a/xtuner/configs/qwen/qwen1_5/qwen1_5_1_8b_chat/qwen1_5_1_8b_chat_qlora_alpaca_e3.py
+++ b/xtuner/configs/qwen/qwen1_5/qwen1_5_1_8b_chat/qwen1_5_1_8b_chat_qlora_alpaca_e3.py
@@ -17,6 +17,7 @@
VarlenAttnArgsToMessageHubHook)
from xtuner.engine.runner import TrainLoop
from xtuner.model import SupervisedFinetune
+from xtuner.parallel.sequence import SequenceParallelSampler
from xtuner.utils import PROMPT_TEMPLATE, SYSTEM_TEMPLATE
#######################################################################
@@ -32,9 +33,13 @@
max_length = 2048
pack_to_max_length = True
+# parallel
+sequence_parallel_size = 1
+
# Scheduler & Optimizer
batch_size = 1 # per_device
accumulative_counts = 16
+accumulative_counts *= sequence_parallel_size
dataloader_num_workers = 0
max_epochs = 3
optim_type = AdamW
@@ -105,11 +110,14 @@
pack_to_max_length=pack_to_max_length,
use_varlen_attn=use_varlen_attn)
+sampler = SequenceParallelSampler \
+ if sequence_parallel_size > 1 else DefaultSampler
+
train_dataloader = dict(
batch_size=batch_size,
num_workers=dataloader_num_workers,
dataset=alpaca_en,
- sampler=dict(type=DefaultSampler, shuffle=True),
+ sampler=dict(type=sampler, shuffle=True),
collate_fn=dict(type=default_collate_fn, use_varlen_attn=use_varlen_attn))
#######################################################################
diff --git a/xtuner/configs/qwen/qwen1_5/qwen1_5_4b/qwen1_5_4b_full_alpaca_e3.py b/xtuner/configs/qwen/qwen1_5/qwen1_5_4b/qwen1_5_4b_full_alpaca_e3.py
index d8d5e0366..32dea90dd 100644
--- a/xtuner/configs/qwen/qwen1_5/qwen1_5_4b/qwen1_5_4b_full_alpaca_e3.py
+++ b/xtuner/configs/qwen/qwen1_5/qwen1_5_4b/qwen1_5_4b_full_alpaca_e3.py
@@ -14,6 +14,7 @@
VarlenAttnArgsToMessageHubHook)
from xtuner.engine.runner import TrainLoop
from xtuner.model import SupervisedFinetune
+from xtuner.parallel.sequence import SequenceParallelSampler
from xtuner.utils import PROMPT_TEMPLATE, SYSTEM_TEMPLATE
#######################################################################
@@ -29,9 +30,13 @@
max_length = 2048
pack_to_max_length = True
+# parallel
+sequence_parallel_size = 1
+
# Scheduler & Optimizer
batch_size = 1 # per_device
accumulative_counts = 16
+accumulative_counts *= sequence_parallel_size
dataloader_num_workers = 0
max_epochs = 3
optim_type = AdamW
@@ -85,11 +90,14 @@
pack_to_max_length=pack_to_max_length,
use_varlen_attn=use_varlen_attn)
+sampler = SequenceParallelSampler \
+ if sequence_parallel_size > 1 else DefaultSampler
+
train_dataloader = dict(
batch_size=batch_size,
num_workers=dataloader_num_workers,
dataset=alpaca_en,
- sampler=dict(type=DefaultSampler, shuffle=True),
+ sampler=dict(type=sampler, shuffle=True),
collate_fn=dict(type=default_collate_fn, use_varlen_attn=use_varlen_attn))
#######################################################################
diff --git a/xtuner/configs/qwen/qwen1_5/qwen1_5_4b/qwen1_5_4b_qlora_alpaca_e3.py b/xtuner/configs/qwen/qwen1_5/qwen1_5_4b/qwen1_5_4b_qlora_alpaca_e3.py
index 16c1514c8..8f8b90229 100644
--- a/xtuner/configs/qwen/qwen1_5/qwen1_5_4b/qwen1_5_4b_qlora_alpaca_e3.py
+++ b/xtuner/configs/qwen/qwen1_5/qwen1_5_4b/qwen1_5_4b_qlora_alpaca_e3.py
@@ -17,6 +17,7 @@
VarlenAttnArgsToMessageHubHook)
from xtuner.engine.runner import TrainLoop
from xtuner.model import SupervisedFinetune
+from xtuner.parallel.sequence import SequenceParallelSampler
from xtuner.utils import PROMPT_TEMPLATE, SYSTEM_TEMPLATE
#######################################################################
@@ -32,9 +33,13 @@
max_length = 2048
pack_to_max_length = True
+# parallel
+sequence_parallel_size = 1
+
# Scheduler & Optimizer
batch_size = 1 # per_device
accumulative_counts = 16
+accumulative_counts *= sequence_parallel_size
dataloader_num_workers = 0
max_epochs = 3
optim_type = AdamW
@@ -105,11 +110,14 @@
pack_to_max_length=pack_to_max_length,
use_varlen_attn=use_varlen_attn)
+sampler = SequenceParallelSampler \
+ if sequence_parallel_size > 1 else DefaultSampler
+
train_dataloader = dict(
batch_size=batch_size,
num_workers=dataloader_num_workers,
dataset=alpaca_en,
- sampler=dict(type=DefaultSampler, shuffle=True),
+ sampler=dict(type=sampler, shuffle=True),
collate_fn=dict(type=default_collate_fn, use_varlen_attn=use_varlen_attn))
#######################################################################
diff --git a/xtuner/configs/qwen/qwen1_5/qwen1_5_4b/qwen1_5_4b_qlora_alpaca_e3_openmind.py b/xtuner/configs/qwen/qwen1_5/qwen1_5_4b/qwen1_5_4b_qlora_alpaca_e3_openmind.py
new file mode 100644
index 000000000..b1446eb48
--- /dev/null
+++ b/xtuner/configs/qwen/qwen1_5/qwen1_5_4b/qwen1_5_4b_qlora_alpaca_e3_openmind.py
@@ -0,0 +1,230 @@
+# Copyright (c) OpenMMLab. All rights reserved.
+import torch
+from datasets import load_dataset
+from mmengine.dataset import DefaultSampler
+from mmengine.hooks import (CheckpointHook, DistSamplerSeedHook, IterTimerHook,
+ LoggerHook, ParamSchedulerHook)
+from mmengine.optim import AmpOptimWrapper, CosineAnnealingLR, LinearLR
+from peft import LoraConfig
+from torch.optim import AdamW
+from transformers import (AutoModelForCausalLM, AutoTokenizer,
+ BitsAndBytesConfig)
+
+from xtuner.dataset import process_hf_dataset
+from xtuner.dataset.collate_fns import default_collate_fn
+from xtuner.dataset.map_fns import alpaca_map_fn, template_map_fn_factory
+from xtuner.engine.hooks import (DatasetInfoHook, EvaluateChatHook,
+ VarlenAttnArgsToMessageHubHook)
+from xtuner.engine.runner import TrainLoop
+from xtuner.model import SupervisedFinetune
+from xtuner.parallel.sequence import SequenceParallelSampler
+from xtuner.utils import PROMPT_TEMPLATE, SYSTEM_TEMPLATE
+from openmind_hub import snapshot_download
+from openmind import OmDataset
+
+#######################################################################
+# PART 1 Settings #
+#######################################################################
+# Model
+pretrained_model_name_or_path = 'Tianjin_Ascend/Qwen1.5-4B'
+model_resource = {
+ "fn": snapshot_download,
+ "args":{
+ # "token":"xxxxxxxxxx"
+ }
+}
+use_varlen_attn = False
+
+# Data
+alpaca_en_path = 'AI_Connect/alpaca'
+prompt_template = PROMPT_TEMPLATE.default
+max_length = 2048
+pack_to_max_length = True
+
+# parallel
+sequence_parallel_size = 1
+
+# Scheduler & Optimizer
+batch_size = 1 # per_device
+accumulative_counts = 16
+accumulative_counts *= sequence_parallel_size
+dataloader_num_workers = 0
+max_epochs = 3
+optim_type = AdamW
+lr = 2e-4
+betas = (0.9, 0.999)
+weight_decay = 0
+max_norm = 1 # grad clip
+warmup_ratio = 0.03
+
+# Save
+save_steps = 500
+save_total_limit = 2 # Maximum checkpoints to keep (-1 means unlimited)
+
+# Evaluate the generation performance during the training
+evaluation_freq = 500
+SYSTEM = SYSTEM_TEMPLATE.alpaca
+evaluation_inputs = [
+ '请给我介绍五个上海的景点', 'Please tell me five scenic spots in Shanghai'
+]
+
+#######################################################################
+# PART 2 Model & Tokenizer #
+#######################################################################
+tokenizer = dict(
+ type=AutoTokenizer.from_pretrained,
+ pretrained_model_name_or_path=pretrained_model_name_or_path,
+ trust_remote_code=True,
+ padding_side='right')
+
+model = dict(
+ type=SupervisedFinetune,
+ use_varlen_attn=use_varlen_attn,
+ llm=dict(
+ type=AutoModelForCausalLM.from_pretrained,
+ pretrained_model_name_or_path=pretrained_model_name_or_path,
+ trust_remote_code=True,
+ torch_dtype=torch.float16,
+ # NPU does not support quantization
+ # quantization_config=dict(
+ # type=BitsAndBytesConfig,
+ # load_in_4bit=True,
+ # load_in_8bit=False,
+ # llm_int8_threshold=6.0,
+ # llm_int8_has_fp16_weight=False,
+ # bnb_4bit_compute_dtype=torch.float16,
+ # bnb_4bit_use_double_quant=True,
+ # bnb_4bit_quant_type='nf4')
+ ),
+ lora=dict(
+ type=LoraConfig,
+ r=64,
+ lora_alpha=16,
+ lora_dropout=0.1,
+ bias='none',
+ task_type='CAUSAL_LM'))
+
+#######################################################################
+# PART 3 Dataset & Dataloader #
+#######################################################################
+alpaca_en = dict(
+ type=process_hf_dataset,
+ dataset=dict(type=OmDataset.load_dataset, path=alpaca_en_path),
+ tokenizer=tokenizer,
+ max_length=max_length,
+ dataset_map_fn=alpaca_map_fn,
+ template_map_fn=dict(
+ type=template_map_fn_factory, template=prompt_template),
+ remove_unused_columns=True,
+ shuffle_before_pack=True,
+ pack_to_max_length=pack_to_max_length,
+ use_varlen_attn=use_varlen_attn)
+
+sampler = SequenceParallelSampler \
+ if sequence_parallel_size > 1 else DefaultSampler
+
+train_dataloader = dict(
+ batch_size=batch_size,
+ num_workers=dataloader_num_workers,
+ dataset=alpaca_en,
+ sampler=dict(type=sampler, shuffle=True),
+ collate_fn=dict(type=default_collate_fn, use_varlen_attn=use_varlen_attn))
+
+#######################################################################
+# PART 4 Scheduler & Optimizer #
+#######################################################################
+# optimizer
+optim_wrapper = dict(
+ type=AmpOptimWrapper,
+ optimizer=dict(
+ type=optim_type, lr=lr, betas=betas, weight_decay=weight_decay),
+ clip_grad=dict(max_norm=max_norm, error_if_nonfinite=False),
+ accumulative_counts=accumulative_counts,
+ loss_scale='dynamic',
+ dtype='float16')
+
+# learning policy
+# More information: https://github.com/open-mmlab/mmengine/blob/main/docs/en/tutorials/param_scheduler.md # noqa: E501
+param_scheduler = [
+ dict(
+ type=LinearLR,
+ start_factor=1e-5,
+ by_epoch=True,
+ begin=0,
+ end=warmup_ratio * max_epochs,
+ convert_to_iter_based=True),
+ dict(
+ type=CosineAnnealingLR,
+ eta_min=0.0,
+ by_epoch=True,
+ begin=warmup_ratio * max_epochs,
+ end=max_epochs,
+ convert_to_iter_based=True)
+]
+
+# train, val, test setting
+train_cfg = dict(type=TrainLoop, max_epochs=max_epochs)
+
+#######################################################################
+# PART 5 Runtime #
+#######################################################################
+# Log the dialogue periodically during the training process, optional
+custom_hooks = [
+ dict(type=DatasetInfoHook, tokenizer=tokenizer),
+ dict(
+ type=EvaluateChatHook,
+ tokenizer=tokenizer,
+ every_n_iters=evaluation_freq,
+ evaluation_inputs=evaluation_inputs,
+ system=SYSTEM,
+ prompt_template=prompt_template)
+]
+
+if use_varlen_attn:
+ custom_hooks += [dict(type=VarlenAttnArgsToMessageHubHook)]
+
+# configure default hooks
+default_hooks = dict(
+ # record the time of every iteration.
+ timer=dict(type=IterTimerHook),
+ # print log every 10 iterations.
+ logger=dict(type=LoggerHook, log_metric_by_epoch=False, interval=10),
+ # enable the parameter scheduler.
+ param_scheduler=dict(type=ParamSchedulerHook),
+ # save checkpoint per `save_steps`.
+ checkpoint=dict(
+ type=CheckpointHook,
+ by_epoch=False,
+ interval=save_steps,
+ max_keep_ckpts=save_total_limit),
+ # set sampler seed in distributed evrionment.
+ sampler_seed=dict(type=DistSamplerSeedHook),
+)
+
+# configure environment
+env_cfg = dict(
+ # whether to enable cudnn benchmark
+ cudnn_benchmark=False,
+ # set multi process parameters
+ mp_cfg=dict(mp_start_method='fork', opencv_num_threads=0),
+ # set distributed parameters
+ dist_cfg=dict(backend='nccl'),
+)
+
+# set visualizer
+visualizer = None
+
+# set log level
+log_level = 'INFO'
+
+# load from which checkpoint
+load_from = None
+
+# whether to resume training from the loaded checkpoint
+resume = False
+
+# Defaults to use random seed and disable `deterministic`
+randomness = dict(seed=None, deterministic=False)
+
+# set log processor
+log_processor = dict(by_epoch=False)
diff --git a/xtuner/configs/qwen/qwen1_5/qwen1_5_4b_chat/qwen1_5_4b_chat_full_alpaca_e3.py b/xtuner/configs/qwen/qwen1_5/qwen1_5_4b_chat/qwen1_5_4b_chat_full_alpaca_e3.py
index 8a486619f..b959a1cd9 100644
--- a/xtuner/configs/qwen/qwen1_5/qwen1_5_4b_chat/qwen1_5_4b_chat_full_alpaca_e3.py
+++ b/xtuner/configs/qwen/qwen1_5/qwen1_5_4b_chat/qwen1_5_4b_chat_full_alpaca_e3.py
@@ -14,6 +14,7 @@
VarlenAttnArgsToMessageHubHook)
from xtuner.engine.runner import TrainLoop
from xtuner.model import SupervisedFinetune
+from xtuner.parallel.sequence import SequenceParallelSampler
from xtuner.utils import PROMPT_TEMPLATE, SYSTEM_TEMPLATE
#######################################################################
@@ -29,9 +30,13 @@
max_length = 2048
pack_to_max_length = True
+# parallel
+sequence_parallel_size = 1
+
# Scheduler & Optimizer
batch_size = 1 # per_device
accumulative_counts = 16
+accumulative_counts *= sequence_parallel_size
dataloader_num_workers = 0
max_epochs = 3
optim_type = AdamW
@@ -85,11 +90,14 @@
pack_to_max_length=pack_to_max_length,
use_varlen_attn=use_varlen_attn)
+sampler = SequenceParallelSampler \
+ if sequence_parallel_size > 1 else DefaultSampler
+
train_dataloader = dict(
batch_size=batch_size,
num_workers=dataloader_num_workers,
dataset=alpaca_en,
- sampler=dict(type=DefaultSampler, shuffle=True),
+ sampler=dict(type=sampler, shuffle=True),
collate_fn=dict(type=default_collate_fn, use_varlen_attn=use_varlen_attn))
#######################################################################
diff --git a/xtuner/configs/qwen/qwen1_5/qwen1_5_4b_chat/qwen1_5_4b_chat_qlora_alpaca_e3.py b/xtuner/configs/qwen/qwen1_5/qwen1_5_4b_chat/qwen1_5_4b_chat_qlora_alpaca_e3.py
index d2f97cfdb..5fb502e35 100644
--- a/xtuner/configs/qwen/qwen1_5/qwen1_5_4b_chat/qwen1_5_4b_chat_qlora_alpaca_e3.py
+++ b/xtuner/configs/qwen/qwen1_5/qwen1_5_4b_chat/qwen1_5_4b_chat_qlora_alpaca_e3.py
@@ -17,6 +17,7 @@
VarlenAttnArgsToMessageHubHook)
from xtuner.engine.runner import TrainLoop
from xtuner.model import SupervisedFinetune
+from xtuner.parallel.sequence import SequenceParallelSampler
from xtuner.utils import PROMPT_TEMPLATE, SYSTEM_TEMPLATE
#######################################################################
@@ -32,9 +33,13 @@
max_length = 2048
pack_to_max_length = True
+# parallel
+sequence_parallel_size = 1
+
# Scheduler & Optimizer
batch_size = 1 # per_device
accumulative_counts = 16
+accumulative_counts *= sequence_parallel_size
dataloader_num_workers = 0
max_epochs = 3
optim_type = AdamW
@@ -105,11 +110,14 @@
pack_to_max_length=pack_to_max_length,
use_varlen_attn=use_varlen_attn)
+sampler = SequenceParallelSampler \
+ if sequence_parallel_size > 1 else DefaultSampler
+
train_dataloader = dict(
batch_size=batch_size,
num_workers=dataloader_num_workers,
dataset=alpaca_en,
- sampler=dict(type=DefaultSampler, shuffle=True),
+ sampler=dict(type=sampler, shuffle=True),
collate_fn=dict(type=default_collate_fn, use_varlen_attn=use_varlen_attn))
#######################################################################
diff --git a/xtuner/configs/qwen/qwen1_5/qwen1_5_72b/qwen1_5_72b_full_alpaca_e3.py b/xtuner/configs/qwen/qwen1_5/qwen1_5_72b/qwen1_5_72b_full_alpaca_e3.py
index 534b27889..84235486e 100644
--- a/xtuner/configs/qwen/qwen1_5/qwen1_5_72b/qwen1_5_72b_full_alpaca_e3.py
+++ b/xtuner/configs/qwen/qwen1_5/qwen1_5_72b/qwen1_5_72b_full_alpaca_e3.py
@@ -14,6 +14,7 @@
VarlenAttnArgsToMessageHubHook)
from xtuner.engine.runner import TrainLoop
from xtuner.model import SupervisedFinetune
+from xtuner.parallel.sequence import SequenceParallelSampler
from xtuner.utils import PROMPT_TEMPLATE, SYSTEM_TEMPLATE
#######################################################################
@@ -29,9 +30,13 @@
max_length = 2048
pack_to_max_length = True
+# parallel
+sequence_parallel_size = 1
+
# Scheduler & Optimizer
batch_size = 1 # per_device
accumulative_counts = 16
+accumulative_counts *= sequence_parallel_size
dataloader_num_workers = 0
max_epochs = 3
optim_type = AdamW
@@ -85,11 +90,14 @@
pack_to_max_length=pack_to_max_length,
use_varlen_attn=use_varlen_attn)
+sampler = SequenceParallelSampler \
+ if sequence_parallel_size > 1 else DefaultSampler
+
train_dataloader = dict(
batch_size=batch_size,
num_workers=dataloader_num_workers,
dataset=alpaca_en,
- sampler=dict(type=DefaultSampler, shuffle=True),
+ sampler=dict(type=sampler, shuffle=True),
collate_fn=dict(type=default_collate_fn, use_varlen_attn=use_varlen_attn))
#######################################################################
diff --git a/xtuner/configs/qwen/qwen1_5/qwen1_5_72b/qwen1_5_72b_qlora_alpaca_e3.py b/xtuner/configs/qwen/qwen1_5/qwen1_5_72b/qwen1_5_72b_qlora_alpaca_e3.py
index 4055a1b89..373db5187 100644
--- a/xtuner/configs/qwen/qwen1_5/qwen1_5_72b/qwen1_5_72b_qlora_alpaca_e3.py
+++ b/xtuner/configs/qwen/qwen1_5/qwen1_5_72b/qwen1_5_72b_qlora_alpaca_e3.py
@@ -17,6 +17,7 @@
VarlenAttnArgsToMessageHubHook)
from xtuner.engine.runner import TrainLoop
from xtuner.model import SupervisedFinetune
+from xtuner.parallel.sequence import SequenceParallelSampler
from xtuner.utils import PROMPT_TEMPLATE, SYSTEM_TEMPLATE
#######################################################################
@@ -32,9 +33,13 @@
max_length = 2048
pack_to_max_length = True
+# parallel
+sequence_parallel_size = 1
+
# Scheduler & Optimizer
batch_size = 1 # per_device
accumulative_counts = 16
+accumulative_counts *= sequence_parallel_size
dataloader_num_workers = 0
max_epochs = 3
optim_type = AdamW
@@ -105,11 +110,14 @@
pack_to_max_length=pack_to_max_length,
use_varlen_attn=use_varlen_attn)
+sampler = SequenceParallelSampler \
+ if sequence_parallel_size > 1 else DefaultSampler
+
train_dataloader = dict(
batch_size=batch_size,
num_workers=dataloader_num_workers,
dataset=alpaca_en,
- sampler=dict(type=DefaultSampler, shuffle=True),
+ sampler=dict(type=sampler, shuffle=True),
collate_fn=dict(type=default_collate_fn, use_varlen_attn=use_varlen_attn))
#######################################################################
diff --git a/xtuner/configs/qwen/qwen1_5/qwen1_5_72b_chat/qwen1_5_72b_chat_full_alpaca_e3.py b/xtuner/configs/qwen/qwen1_5/qwen1_5_72b_chat/qwen1_5_72b_chat_full_alpaca_e3.py
index e40e78f5b..1de7c92b4 100644
--- a/xtuner/configs/qwen/qwen1_5/qwen1_5_72b_chat/qwen1_5_72b_chat_full_alpaca_e3.py
+++ b/xtuner/configs/qwen/qwen1_5/qwen1_5_72b_chat/qwen1_5_72b_chat_full_alpaca_e3.py
@@ -14,6 +14,7 @@
VarlenAttnArgsToMessageHubHook)
from xtuner.engine.runner import TrainLoop
from xtuner.model import SupervisedFinetune
+from xtuner.parallel.sequence import SequenceParallelSampler
from xtuner.utils import PROMPT_TEMPLATE, SYSTEM_TEMPLATE
#######################################################################
@@ -29,9 +30,13 @@
max_length = 2048
pack_to_max_length = True
+# parallel
+sequence_parallel_size = 1
+
# Scheduler & Optimizer
batch_size = 1 # per_device
accumulative_counts = 16
+accumulative_counts *= sequence_parallel_size
dataloader_num_workers = 0
max_epochs = 3
optim_type = AdamW
@@ -85,11 +90,14 @@
pack_to_max_length=pack_to_max_length,
use_varlen_attn=use_varlen_attn)
+sampler = SequenceParallelSampler \
+ if sequence_parallel_size > 1 else DefaultSampler
+
train_dataloader = dict(
batch_size=batch_size,
num_workers=dataloader_num_workers,
dataset=alpaca_en,
- sampler=dict(type=DefaultSampler, shuffle=True),
+ sampler=dict(type=sampler, shuffle=True),
collate_fn=dict(type=default_collate_fn, use_varlen_attn=use_varlen_attn))
#######################################################################
diff --git a/xtuner/configs/qwen/qwen1_5/qwen1_5_72b_chat/qwen1_5_72b_chat_qlora_alpaca_e3.py b/xtuner/configs/qwen/qwen1_5/qwen1_5_72b_chat/qwen1_5_72b_chat_qlora_alpaca_e3.py
index cf7934860..94786106d 100644
--- a/xtuner/configs/qwen/qwen1_5/qwen1_5_72b_chat/qwen1_5_72b_chat_qlora_alpaca_e3.py
+++ b/xtuner/configs/qwen/qwen1_5/qwen1_5_72b_chat/qwen1_5_72b_chat_qlora_alpaca_e3.py
@@ -17,6 +17,7 @@
VarlenAttnArgsToMessageHubHook)
from xtuner.engine.runner import TrainLoop
from xtuner.model import SupervisedFinetune
+from xtuner.parallel.sequence import SequenceParallelSampler
from xtuner.utils import PROMPT_TEMPLATE, SYSTEM_TEMPLATE
#######################################################################
@@ -32,9 +33,13 @@
max_length = 2048
pack_to_max_length = True
+# parallel
+sequence_parallel_size = 1
+
# Scheduler & Optimizer
batch_size = 1 # per_device
accumulative_counts = 16
+accumulative_counts *= sequence_parallel_size
dataloader_num_workers = 0
max_epochs = 3
optim_type = AdamW
@@ -105,11 +110,14 @@
pack_to_max_length=pack_to_max_length,
use_varlen_attn=use_varlen_attn)
+sampler = SequenceParallelSampler \
+ if sequence_parallel_size > 1 else DefaultSampler
+
train_dataloader = dict(
batch_size=batch_size,
num_workers=dataloader_num_workers,
dataset=alpaca_en,
- sampler=dict(type=DefaultSampler, shuffle=True),
+ sampler=dict(type=sampler, shuffle=True),
collate_fn=dict(type=default_collate_fn, use_varlen_attn=use_varlen_attn))
#######################################################################
diff --git a/xtuner/configs/qwen/qwen1_5/qwen1_5_7b/qwen1_5_7b_full_alpaca_e3.py b/xtuner/configs/qwen/qwen1_5/qwen1_5_7b/qwen1_5_7b_full_alpaca_e3.py
index 2dca84070..f4c7b1be3 100644
--- a/xtuner/configs/qwen/qwen1_5/qwen1_5_7b/qwen1_5_7b_full_alpaca_e3.py
+++ b/xtuner/configs/qwen/qwen1_5/qwen1_5_7b/qwen1_5_7b_full_alpaca_e3.py
@@ -14,6 +14,7 @@
VarlenAttnArgsToMessageHubHook)
from xtuner.engine.runner import TrainLoop
from xtuner.model import SupervisedFinetune
+from xtuner.parallel.sequence import SequenceParallelSampler
from xtuner.utils import PROMPT_TEMPLATE, SYSTEM_TEMPLATE
#######################################################################
@@ -29,9 +30,13 @@
max_length = 2048
pack_to_max_length = True
+# parallel
+sequence_parallel_size = 1
+
# Scheduler & Optimizer
batch_size = 1 # per_device
accumulative_counts = 16
+accumulative_counts *= sequence_parallel_size
dataloader_num_workers = 0
max_epochs = 3
optim_type = AdamW
@@ -85,11 +90,14 @@
pack_to_max_length=pack_to_max_length,
use_varlen_attn=use_varlen_attn)
+sampler = SequenceParallelSampler \
+ if sequence_parallel_size > 1 else DefaultSampler
+
train_dataloader = dict(
batch_size=batch_size,
num_workers=dataloader_num_workers,
dataset=alpaca_en,
- sampler=dict(type=DefaultSampler, shuffle=True),
+ sampler=dict(type=sampler, shuffle=True),
collate_fn=dict(type=default_collate_fn, use_varlen_attn=use_varlen_attn))
#######################################################################
diff --git a/xtuner/configs/qwen/qwen1_5/qwen1_5_7b/qwen1_5_7b_qlora_alpaca_e3.py b/xtuner/configs/qwen/qwen1_5/qwen1_5_7b/qwen1_5_7b_qlora_alpaca_e3.py
index 18a9a96f4..03cd6f6cb 100644
--- a/xtuner/configs/qwen/qwen1_5/qwen1_5_7b/qwen1_5_7b_qlora_alpaca_e3.py
+++ b/xtuner/configs/qwen/qwen1_5/qwen1_5_7b/qwen1_5_7b_qlora_alpaca_e3.py
@@ -17,6 +17,7 @@
VarlenAttnArgsToMessageHubHook)
from xtuner.engine.runner import TrainLoop
from xtuner.model import SupervisedFinetune
+from xtuner.parallel.sequence import SequenceParallelSampler
from xtuner.utils import PROMPT_TEMPLATE, SYSTEM_TEMPLATE
#######################################################################
@@ -32,9 +33,13 @@
max_length = 2048
pack_to_max_length = True
+# parallel
+sequence_parallel_size = 1
+
# Scheduler & Optimizer
batch_size = 1 # per_device
accumulative_counts = 16
+accumulative_counts *= sequence_parallel_size
dataloader_num_workers = 0
max_epochs = 3
optim_type = AdamW
@@ -105,11 +110,14 @@
pack_to_max_length=pack_to_max_length,
use_varlen_attn=use_varlen_attn)
+sampler = SequenceParallelSampler \
+ if sequence_parallel_size > 1 else DefaultSampler
+
train_dataloader = dict(
batch_size=batch_size,
num_workers=dataloader_num_workers,
dataset=alpaca_en,
- sampler=dict(type=DefaultSampler, shuffle=True),
+ sampler=dict(type=sampler, shuffle=True),
collate_fn=dict(type=default_collate_fn, use_varlen_attn=use_varlen_attn))
#######################################################################
diff --git a/xtuner/configs/qwen/qwen1_5/qwen1_5_7b_chat/qwen1_5_7b_chat_full_alpaca_e3.py b/xtuner/configs/qwen/qwen1_5/qwen1_5_7b_chat/qwen1_5_7b_chat_full_alpaca_e3.py
index 3e7d87d62..62bf9ed31 100644
--- a/xtuner/configs/qwen/qwen1_5/qwen1_5_7b_chat/qwen1_5_7b_chat_full_alpaca_e3.py
+++ b/xtuner/configs/qwen/qwen1_5/qwen1_5_7b_chat/qwen1_5_7b_chat_full_alpaca_e3.py
@@ -14,6 +14,7 @@
VarlenAttnArgsToMessageHubHook)
from xtuner.engine.runner import TrainLoop
from xtuner.model import SupervisedFinetune
+from xtuner.parallel.sequence import SequenceParallelSampler
from xtuner.utils import PROMPT_TEMPLATE, SYSTEM_TEMPLATE
#######################################################################
@@ -29,9 +30,13 @@
max_length = 2048
pack_to_max_length = True
+# parallel
+sequence_parallel_size = 1
+
# Scheduler & Optimizer
batch_size = 1 # per_device
accumulative_counts = 16
+accumulative_counts *= sequence_parallel_size
dataloader_num_workers = 0
max_epochs = 3
optim_type = AdamW
@@ -85,11 +90,14 @@
pack_to_max_length=pack_to_max_length,
use_varlen_attn=use_varlen_attn)
+sampler = SequenceParallelSampler \
+ if sequence_parallel_size > 1 else DefaultSampler
+
train_dataloader = dict(
batch_size=batch_size,
num_workers=dataloader_num_workers,
dataset=alpaca_en,
- sampler=dict(type=DefaultSampler, shuffle=True),
+ sampler=dict(type=sampler, shuffle=True),
collate_fn=dict(type=default_collate_fn, use_varlen_attn=use_varlen_attn))
#######################################################################
diff --git a/xtuner/configs/qwen/qwen1_5/qwen1_5_7b_chat/qwen1_5_7b_chat_qlora_alpaca_e3.py b/xtuner/configs/qwen/qwen1_5/qwen1_5_7b_chat/qwen1_5_7b_chat_qlora_alpaca_e3.py
index 0d8c8d4ad..5b42c8d70 100644
--- a/xtuner/configs/qwen/qwen1_5/qwen1_5_7b_chat/qwen1_5_7b_chat_qlora_alpaca_e3.py
+++ b/xtuner/configs/qwen/qwen1_5/qwen1_5_7b_chat/qwen1_5_7b_chat_qlora_alpaca_e3.py
@@ -17,6 +17,7 @@
VarlenAttnArgsToMessageHubHook)
from xtuner.engine.runner import TrainLoop
from xtuner.model import SupervisedFinetune
+from xtuner.parallel.sequence import SequenceParallelSampler
from xtuner.utils import PROMPT_TEMPLATE, SYSTEM_TEMPLATE
#######################################################################
@@ -32,9 +33,13 @@
max_length = 2048
pack_to_max_length = True
+# parallel
+sequence_parallel_size = 1
+
# Scheduler & Optimizer
batch_size = 1 # per_device
accumulative_counts = 16
+accumulative_counts *= sequence_parallel_size
dataloader_num_workers = 0
max_epochs = 3
optim_type = AdamW
@@ -105,11 +110,14 @@
pack_to_max_length=pack_to_max_length,
use_varlen_attn=use_varlen_attn)
+sampler = SequenceParallelSampler \
+ if sequence_parallel_size > 1 else DefaultSampler
+
train_dataloader = dict(
batch_size=batch_size,
num_workers=dataloader_num_workers,
dataset=alpaca_en,
- sampler=dict(type=DefaultSampler, shuffle=True),
+ sampler=dict(type=sampler, shuffle=True),
collate_fn=dict(type=default_collate_fn, use_varlen_attn=use_varlen_attn))
#######################################################################
diff --git a/xtuner/configs/qwen_moe/qwen1_5/qwen1_5_moe_a2_7_b_chat/qwen1_5_moe_a2_7_b_chat_full_alpaca_e3.py b/xtuner/configs/qwen_moe/qwen1_5/qwen1_5_moe_a2_7_b_chat/qwen1_5_moe_a2_7_b_chat_full_alpaca_e3.py
new file mode 100644
index 000000000..6e8c2fb00
--- /dev/null
+++ b/xtuner/configs/qwen_moe/qwen1_5/qwen1_5_moe_a2_7_b_chat/qwen1_5_moe_a2_7_b_chat_full_alpaca_e3.py
@@ -0,0 +1,219 @@
+# Copyright (c) OpenMMLab. All rights reserved.
+from datasets import load_dataset
+from mmengine.dataset import DefaultSampler
+from mmengine.hooks import (CheckpointHook, DistSamplerSeedHook, IterTimerHook,
+ LoggerHook, ParamSchedulerHook)
+from mmengine.optim import AmpOptimWrapper, CosineAnnealingLR, LinearLR
+from torch.optim import AdamW
+from transformers import AutoModelForCausalLM, AutoTokenizer
+
+from xtuner.dataset import ConcatDataset, process_hf_dataset
+from xtuner.dataset.collate_fns import default_collate_fn
+from xtuner.dataset.map_fns import (alpaca_map_fn, alpaca_zh_map_fn,
+ template_map_fn_factory)
+from xtuner.engine.hooks import (DatasetInfoHook, EvaluateChatHook,
+ ThroughputHook,
+ VarlenAttnArgsToMessageHubHook)
+from xtuner.engine.runner import TrainLoop
+from xtuner.model import SupervisedFinetune
+from xtuner.parallel.sequence import SequenceParallelSampler
+from xtuner.utils import PROMPT_TEMPLATE, SYSTEM_TEMPLATE
+
+#######################################################################
+# PART 1 Settings #
+#######################################################################
+# Model
+pretrained_model_name_or_path = 'Qwen/Qwen1.5-MoE-A2.7B-Chat'
+use_varlen_attn = False
+
+# Data
+alpaca_zh_path = 'silk-road/alpaca-data-gpt4-chinese'
+alpaca_en_path = 'tatsu-lab/alpaca'
+prompt_template = PROMPT_TEMPLATE.qwen_chat
+max_length = 32768
+pack_to_max_length = True
+
+# parallel
+sequence_parallel_size = 1
+
+# Scheduler & Optimizer
+batch_size = 1 # per_device
+accumulative_counts = 1
+accumulative_counts *= sequence_parallel_size
+dataloader_num_workers = 0
+max_epochs = 3
+optim_type = AdamW
+lr = 2e-5
+betas = (0.9, 0.999)
+weight_decay = 0
+max_norm = 1 # grad clip
+warmup_ratio = 0.03
+
+# Save
+save_steps = 500
+save_total_limit = 2 # Maximum checkpoints to keep (-1 means unlimited)
+
+# Evaluate the generation performance during the training
+evaluation_freq = 50
+SYSTEM = SYSTEM_TEMPLATE.alpaca
+evaluation_inputs = [
+ '请给我介绍五个上海的景点', 'Please tell me five scenic spots in Shanghai'
+]
+
+#######################################################################
+# PART 2 Model & Tokenizer #
+#######################################################################
+tokenizer = dict(
+ type=AutoTokenizer.from_pretrained,
+ pretrained_model_name_or_path=pretrained_model_name_or_path,
+ trust_remote_code=True,
+ padding_side='right')
+
+model = dict(
+ type=SupervisedFinetune,
+ use_varlen_attn=use_varlen_attn,
+ llm=dict(
+ type=AutoModelForCausalLM.from_pretrained,
+ pretrained_model_name_or_path=pretrained_model_name_or_path,
+ trust_remote_code=True))
+
+#######################################################################
+# PART 3 Dataset & Dataloader #
+#######################################################################
+alpaca_en = dict(
+ type=process_hf_dataset,
+ dataset=dict(type=load_dataset, path=alpaca_en_path),
+ tokenizer=tokenizer,
+ max_length=max_length,
+ dataset_map_fn=alpaca_map_fn,
+ template_map_fn=dict(
+ type=template_map_fn_factory, template=prompt_template),
+ remove_unused_columns=True,
+ shuffle_before_pack=False,
+ pack_to_max_length=pack_to_max_length,
+ use_varlen_attn=use_varlen_attn)
+
+alpaca_zh = dict(
+ type=process_hf_dataset,
+ dataset=dict(type=load_dataset, path=alpaca_zh_path),
+ tokenizer=tokenizer,
+ max_length=max_length,
+ dataset_map_fn=alpaca_zh_map_fn,
+ template_map_fn=dict(
+ type=template_map_fn_factory, template=prompt_template),
+ remove_unused_columns=True,
+ shuffle_before_pack=False,
+ pack_to_max_length=pack_to_max_length,
+ use_varlen_attn=use_varlen_attn)
+
+train_dataset = dict(type=ConcatDataset, datasets=[alpaca_en, alpaca_zh])
+
+sampler = SequenceParallelSampler \
+ if sequence_parallel_size > 1 else DefaultSampler
+
+train_dataloader = dict(
+ batch_size=batch_size,
+ num_workers=dataloader_num_workers,
+ dataset=train_dataset,
+ sampler=dict(type=sampler, shuffle=True),
+ collate_fn=dict(type=default_collate_fn, use_varlen_attn=use_varlen_attn))
+
+#######################################################################
+# PART 4 Scheduler & Optimizer #
+#######################################################################
+# optimizer
+optim_wrapper = dict(
+ type=AmpOptimWrapper,
+ optimizer=dict(
+ type=optim_type, lr=lr, betas=betas, weight_decay=weight_decay),
+ clip_grad=dict(max_norm=max_norm, error_if_nonfinite=False),
+ accumulative_counts=accumulative_counts,
+ loss_scale='dynamic',
+ dtype='float16')
+
+# learning policy
+# More information: https://github.com/open-mmlab/mmengine/blob/main/docs/en/tutorials/param_scheduler.md # noqa: E501
+param_scheduler = [
+ dict(
+ type=LinearLR,
+ start_factor=1e-5,
+ by_epoch=True,
+ begin=0,
+ end=warmup_ratio * max_epochs,
+ convert_to_iter_based=True),
+ dict(
+ type=CosineAnnealingLR,
+ eta_min=0.0,
+ by_epoch=True,
+ begin=warmup_ratio * max_epochs,
+ end=max_epochs,
+ convert_to_iter_based=True)
+]
+
+# train, val, test setting
+train_cfg = dict(type=TrainLoop, max_epochs=max_epochs)
+
+#######################################################################
+# PART 5 Runtime #
+#######################################################################
+# Log the dialogue periodically during the training process, optional
+custom_hooks = [
+ dict(type=DatasetInfoHook, tokenizer=tokenizer),
+ dict(
+ type=EvaluateChatHook,
+ tokenizer=tokenizer,
+ every_n_iters=evaluation_freq,
+ evaluation_inputs=evaluation_inputs,
+ system=SYSTEM,
+ prompt_template=prompt_template),
+ dict(type=ThroughputHook),
+]
+
+if use_varlen_attn:
+ custom_hooks += [dict(type=VarlenAttnArgsToMessageHubHook)]
+
+# configure default hooks
+default_hooks = dict(
+ # record the time of every iteration.
+ timer=dict(type=IterTimerHook),
+ # print log every 10 iterations.
+ logger=dict(type=LoggerHook, log_metric_by_epoch=False, interval=1),
+ # enable the parameter scheduler.
+ param_scheduler=dict(type=ParamSchedulerHook),
+ # save checkpoint per `save_steps`.
+ checkpoint=dict(
+ type=CheckpointHook,
+ by_epoch=False,
+ interval=save_steps,
+ max_keep_ckpts=save_total_limit),
+ # set sampler seed in distributed evrionment.
+ sampler_seed=dict(type=DistSamplerSeedHook),
+)
+
+# configure environment
+env_cfg = dict(
+ # whether to enable cudnn benchmark
+ cudnn_benchmark=False,
+ # set multi process parameters
+ mp_cfg=dict(mp_start_method='fork', opencv_num_threads=0),
+ # set distributed parameters
+ dist_cfg=dict(backend='nccl'),
+)
+
+# set visualizer
+visualizer = None
+
+# set log level
+log_level = 'INFO'
+
+# load from which checkpoint
+load_from = None
+
+# whether to resume training from the loaded checkpoint
+resume = False
+
+# Defaults to use random seed and disable `deterministic`
+randomness = dict(seed=None, deterministic=False)
+
+# set log processor
+log_processor = dict(by_epoch=False, window_size=1)
diff --git a/xtuner/configs/reward_model/internlm/internlm2_chat_1_8b_reward_full_ultrafeedback.py b/xtuner/configs/reward_model/internlm/internlm2_chat_1_8b_reward_full_ultrafeedback.py
new file mode 100644
index 000000000..ce48f5cda
--- /dev/null
+++ b/xtuner/configs/reward_model/internlm/internlm2_chat_1_8b_reward_full_ultrafeedback.py
@@ -0,0 +1,184 @@
+# Copyright (c) OpenMMLab. All rights reserved.
+from datasets import load_dataset
+from mmengine.dataset import DefaultSampler
+from mmengine.hooks import (CheckpointHook, DistSamplerSeedHook, IterTimerHook,
+ LoggerHook, ParamSchedulerHook)
+from mmengine.optim import AmpOptimWrapper, CosineAnnealingLR, LinearLR
+from torch.optim import AdamW
+from transformers import AutoModelForCausalLM, AutoTokenizer
+
+from xtuner.dataset.collate_fns.preference_collate_fn import \
+ preference_collate_fn
+from xtuner.dataset.preference_dataset import (build_preference_dataset,
+ orpo_dpo_mix_40k_map_fn)
+from xtuner.engine.hooks import VarlenAttnArgsToMessageHubHook
+from xtuner.engine.runner import TrainLoop
+from xtuner.model.reward import RewardModel
+
+#######################################################################
+# PART 1 Settings #
+#######################################################################
+# Model
+pretrained_model_name_or_path = 'internlm/internlm2-chat-1_8b-sft'
+use_varlen_attn = False
+reward_token_id = 92527 # use [UNUSED_TOKEN_130] as reward token
+loss_type = 'focal'
+penalty_type = 'log_barrier'
+
+# Data
+max_length = 2048
+
+# Scheduler & Optimizer
+batch_size = 4 # per_device
+accumulative_counts = 16
+dataloader_num_workers = 0
+max_epochs = 1 # reward model should not be trained for more than 1 epoch to avoid overfitting # noqa: E501
+optim_type = AdamW
+lr = 2e-5
+betas = (0.9, 0.999)
+weight_decay = 0
+max_norm = 1 # grad clip
+warmup_ratio = 0.03
+
+# Save
+save_steps = 500
+save_total_limit = 2 # Maximum checkpoints to keep (-1 means unlimited)
+
+# Evaluate the generation performance during the training
+evaluation_freq = 500
+
+#######################################################################
+# PART 2 Model & Tokenizer #
+#######################################################################
+tokenizer = dict(
+ type=AutoTokenizer.from_pretrained,
+ pretrained_model_name_or_path=pretrained_model_name_or_path,
+ trust_remote_code=True,
+ padding_side='right')
+
+model = dict(
+ type=RewardModel,
+ use_varlen_attn=use_varlen_attn,
+ loss_type=loss_type,
+ penalty_type=penalty_type,
+ llm=dict(
+ type=AutoModelForCausalLM.from_pretrained,
+ pretrained_model_name_or_path=pretrained_model_name_or_path,
+ trust_remote_code=True))
+
+#######################################################################
+# PART 3 Dataset & Dataloader #
+#######################################################################
+train_dataset = dict(
+ type=build_preference_dataset,
+ dataset=dict(
+ type=load_dataset,
+ path='argilla/ultrafeedback-binarized-preferences-cleaned'),
+ tokenizer=tokenizer,
+ max_length=max_length,
+ dataset_map_fn=orpo_dpo_mix_40k_map_fn,
+ is_dpo=False,
+ is_reward=True,
+ reward_token_id=reward_token_id,
+ num_proc=32,
+ use_varlen_attn=use_varlen_attn,
+ shuffle_before_pack=True,
+)
+
+train_dataloader = dict(
+ batch_size=batch_size,
+ num_workers=dataloader_num_workers,
+ dataset=train_dataset,
+ sampler=dict(type=DefaultSampler, shuffle=True),
+ collate_fn=dict(
+ type=preference_collate_fn, use_varlen_attn=use_varlen_attn))
+
+#######################################################################
+# PART 4 Scheduler & Optimizer #
+#######################################################################
+# optimizer
+optim_wrapper = dict(
+ type=AmpOptimWrapper,
+ optimizer=dict(
+ type=optim_type, lr=lr, betas=betas, weight_decay=weight_decay),
+ clip_grad=dict(max_norm=max_norm, error_if_nonfinite=False),
+ accumulative_counts=accumulative_counts,
+ loss_scale='dynamic',
+ dtype='float16')
+
+# learning policy
+# More information: https://github.com/open-mmlab/mmengine/blob/main/docs/en/tutorials/param_scheduler.md # noqa: E501
+param_scheduler = [
+ dict(
+ type=LinearLR,
+ start_factor=1e-5,
+ by_epoch=True,
+ begin=0,
+ end=warmup_ratio * max_epochs,
+ convert_to_iter_based=True),
+ dict(
+ type=CosineAnnealingLR,
+ eta_min=0.0,
+ by_epoch=True,
+ begin=warmup_ratio * max_epochs,
+ end=max_epochs,
+ convert_to_iter_based=True)
+]
+
+# train, val, test setting
+train_cfg = dict(type=TrainLoop, max_epochs=max_epochs)
+
+#######################################################################
+# PART 5 Runtime #
+#######################################################################
+# Log the dialogue periodically during the training process, optional
+custom_hooks = []
+
+if use_varlen_attn:
+ custom_hooks += [dict(type=VarlenAttnArgsToMessageHubHook)]
+
+# configure default hooks
+default_hooks = dict(
+ # record the time of every iteration.
+ timer=dict(type=IterTimerHook),
+ # print log every 10 iterations.
+ logger=dict(type=LoggerHook, log_metric_by_epoch=False, interval=10),
+ # enable the parameter scheduler.
+ param_scheduler=dict(type=ParamSchedulerHook),
+ # save checkpoint per `save_steps`.
+ checkpoint=dict(
+ type=CheckpointHook,
+ by_epoch=False,
+ interval=save_steps,
+ max_keep_ckpts=save_total_limit),
+ # set sampler seed in distributed evrionment.
+ sampler_seed=dict(type=DistSamplerSeedHook),
+)
+
+# configure environment
+env_cfg = dict(
+ # whether to enable cudnn benchmark
+ cudnn_benchmark=False,
+ # set multi process parameters
+ mp_cfg=dict(mp_start_method='fork', opencv_num_threads=0),
+ # set distributed parameters
+ dist_cfg=dict(backend='nccl'),
+)
+
+# set visualizer
+visualizer = None
+
+# set log level
+log_level = 'INFO'
+
+# load from which checkpoint
+load_from = None
+
+# whether to resume training from the loaded checkpoint
+resume = False
+
+# Defaults to use random seed and disable `deterministic`
+randomness = dict(seed=None, deterministic=False)
+
+# set log processor
+log_processor = dict(by_epoch=False)
diff --git a/xtuner/configs/reward_model/internlm/internlm2_chat_1_8b_reward_full_varlenattn_jsonl_dataset.py b/xtuner/configs/reward_model/internlm/internlm2_chat_1_8b_reward_full_varlenattn_jsonl_dataset.py
new file mode 100644
index 000000000..fc10c3189
--- /dev/null
+++ b/xtuner/configs/reward_model/internlm/internlm2_chat_1_8b_reward_full_varlenattn_jsonl_dataset.py
@@ -0,0 +1,197 @@
+# Copyright (c) OpenMMLab. All rights reserved.
+from mmengine.dataset import DefaultSampler
+from mmengine.hooks import (CheckpointHook, DistSamplerSeedHook, IterTimerHook,
+ LoggerHook, ParamSchedulerHook)
+from mmengine.optim import AmpOptimWrapper, CosineAnnealingLR, LinearLR
+from torch.optim import AdamW
+from transformers import AutoModelForCausalLM, AutoTokenizer
+
+from xtuner.dataset.collate_fns.preference_collate_fn import \
+ preference_collate_fn
+from xtuner.dataset.preference_dataset import (build_preference_dataset,
+ load_jsonl_dataset)
+from xtuner.engine.hooks import VarlenAttnArgsToMessageHubHook
+from xtuner.engine.runner import TrainLoop
+from xtuner.model.reward import RewardModel
+from xtuner.parallel.sequence import SequenceParallelSampler
+
+#######################################################################
+# PART 1 Settings #
+#######################################################################
+# Model
+pretrained_model_name_or_path = 'internlm/internlm2-chat-1_8b-sft'
+use_varlen_attn = True
+reward_token_id = 92527 # use [UNUSED_TOKEN_130] as reward token
+loss_type = 'focal'
+penalty_type = 'log_barrier'
+
+# Data
+max_length = 2048
+max_packed_length = max_length * 2
+
+# parallel
+sequence_parallel_size = 1
+
+# Scheduler & Optimizer
+batch_size = 1 # per_device
+accumulative_counts = 16
+accumulative_counts *= sequence_parallel_size
+dataloader_num_workers = 0
+max_epochs = 1 # reward model should not be trained for more than 1 epoch to avoid overfitting # noqa: E501
+optim_type = AdamW
+lr = 2e-5
+betas = (0.9, 0.999)
+weight_decay = 0
+max_norm = 1 # grad clip
+warmup_ratio = 0.03
+
+# Save
+save_steps = 500
+save_total_limit = 2 # Maximum checkpoints to keep (-1 means unlimited)
+
+# Evaluate the generation performance during the training
+# TODO: eval
+# evaluation_freq = 500
+
+#######################################################################
+# PART 2 Model & Tokenizer #
+#######################################################################
+tokenizer = dict(
+ type=AutoTokenizer.from_pretrained,
+ pretrained_model_name_or_path=pretrained_model_name_or_path,
+ trust_remote_code=True,
+ padding_side='right')
+
+model = dict(
+ type=RewardModel,
+ use_varlen_attn=use_varlen_attn,
+ loss_type=loss_type,
+ penalty_type=penalty_type,
+ llm=dict(
+ type=AutoModelForCausalLM.from_pretrained,
+ pretrained_model_name_or_path=pretrained_model_name_or_path,
+ trust_remote_code=True))
+
+#######################################################################
+# PART 3 Dataset & Dataloader #
+#######################################################################
+sampler = SequenceParallelSampler \
+ if sequence_parallel_size > 1 else DefaultSampler
+
+train_dataset = dict(
+ type=build_preference_dataset,
+ dataset=dict(
+ type=load_jsonl_dataset,
+ data_files=[
+ '/your/jsonl/path/here.jsonl',
+ '/your/another/jsonl/path/here.jsonl'
+ ]),
+ tokenizer=tokenizer,
+ max_length=max_length,
+ dataset_map_fn=None,
+ is_dpo=False,
+ is_reward=True,
+ reward_token_id=reward_token_id,
+ num_proc=32,
+ use_varlen_attn=use_varlen_attn,
+ max_packed_length=max_packed_length,
+ shuffle_before_pack=True,
+)
+
+train_dataloader = dict(
+ batch_size=batch_size,
+ num_workers=dataloader_num_workers,
+ dataset=train_dataset,
+ sampler=dict(type=sampler, shuffle=True),
+ collate_fn=dict(
+ type=preference_collate_fn, use_varlen_attn=use_varlen_attn))
+
+#######################################################################
+# PART 4 Scheduler & Optimizer #
+#######################################################################
+# optimizer
+optim_wrapper = dict(
+ type=AmpOptimWrapper,
+ optimizer=dict(
+ type=optim_type, lr=lr, betas=betas, weight_decay=weight_decay),
+ clip_grad=dict(max_norm=max_norm, error_if_nonfinite=False),
+ accumulative_counts=accumulative_counts,
+ loss_scale='dynamic',
+ dtype='float16')
+
+# learning policy
+# More information: https://github.com/open-mmlab/mmengine/blob/main/docs/en/tutorials/param_scheduler.md # noqa: E501
+param_scheduler = [
+ dict(
+ type=LinearLR,
+ start_factor=1e-5,
+ by_epoch=True,
+ begin=0,
+ end=warmup_ratio * max_epochs,
+ convert_to_iter_based=True),
+ dict(
+ type=CosineAnnealingLR,
+ eta_min=0.0,
+ by_epoch=True,
+ begin=warmup_ratio * max_epochs,
+ end=max_epochs,
+ convert_to_iter_based=True)
+]
+
+# train, val, test setting
+train_cfg = dict(type=TrainLoop, max_epochs=max_epochs)
+
+#######################################################################
+# PART 5 Runtime #
+#######################################################################
+# Log the dialogue periodically during the training process, optional
+custom_hooks = []
+
+if use_varlen_attn:
+ custom_hooks += [dict(type=VarlenAttnArgsToMessageHubHook)]
+
+# configure default hooks
+default_hooks = dict(
+ # record the time of every iteration.
+ timer=dict(type=IterTimerHook),
+ # print log every 10 iterations.
+ logger=dict(type=LoggerHook, log_metric_by_epoch=False, interval=10),
+ # enable the parameter scheduler.
+ param_scheduler=dict(type=ParamSchedulerHook),
+ # save checkpoint per `save_steps`.
+ checkpoint=dict(
+ type=CheckpointHook,
+ by_epoch=False,
+ interval=save_steps,
+ max_keep_ckpts=save_total_limit),
+ # set sampler seed in distributed evrionment.
+ sampler_seed=dict(type=DistSamplerSeedHook),
+)
+
+# configure environment
+env_cfg = dict(
+ # whether to enable cudnn benchmark
+ cudnn_benchmark=False,
+ # set multi process parameters
+ mp_cfg=dict(mp_start_method='fork', opencv_num_threads=0),
+ # set distributed parameters
+ dist_cfg=dict(backend='nccl'),
+)
+
+# set visualizer
+visualizer = None
+
+# set log level
+log_level = 'INFO'
+
+# load from which checkpoint
+load_from = None
+
+# whether to resume training from the loaded checkpoint
+resume = False
+
+# Defaults to use random seed and disable `deterministic`
+randomness = dict(seed=None, deterministic=False)
+
+# set log processor
+log_processor = dict(by_epoch=False)
diff --git a/xtuner/configs/reward_model/internlm/internlm2_chat_1_8b_reward_full_varlenattn_ultrafeedback.py b/xtuner/configs/reward_model/internlm/internlm2_chat_1_8b_reward_full_varlenattn_ultrafeedback.py
new file mode 100644
index 000000000..b2c7ebed7
--- /dev/null
+++ b/xtuner/configs/reward_model/internlm/internlm2_chat_1_8b_reward_full_varlenattn_ultrafeedback.py
@@ -0,0 +1,195 @@
+# Copyright (c) OpenMMLab. All rights reserved.
+from datasets import load_dataset
+from mmengine.dataset import DefaultSampler
+from mmengine.hooks import (CheckpointHook, DistSamplerSeedHook, IterTimerHook,
+ LoggerHook, ParamSchedulerHook)
+from mmengine.optim import AmpOptimWrapper, CosineAnnealingLR, LinearLR
+from torch.optim import AdamW
+from transformers import AutoModelForCausalLM, AutoTokenizer
+
+from xtuner.dataset.collate_fns.preference_collate_fn import \
+ preference_collate_fn
+from xtuner.dataset.preference_dataset import (build_preference_dataset,
+ orpo_dpo_mix_40k_map_fn)
+from xtuner.engine.hooks import VarlenAttnArgsToMessageHubHook
+from xtuner.engine.runner import TrainLoop
+from xtuner.model.reward import RewardModel
+from xtuner.parallel.sequence import SequenceParallelSampler
+
+#######################################################################
+# PART 1 Settings #
+#######################################################################
+# Model
+pretrained_model_name_or_path = 'internlm/internlm2-chat-1_8b-sft'
+use_varlen_attn = True
+reward_token_id = 92527 # use [UNUSED_TOKEN_130] as reward token
+loss_type = 'focal'
+penalty_type = 'log_barrier'
+
+# Data
+max_length = 2048
+max_packed_length = max_length * 2
+
+# parallel
+sequence_parallel_size = 1
+
+# Scheduler & Optimizer
+batch_size = 1 # per_device
+accumulative_counts = 16
+accumulative_counts *= sequence_parallel_size
+dataloader_num_workers = 0
+max_epochs = 1 # reward model should not be trained for more than 1 epoch to avoid overfitting # noqa: E501
+optim_type = AdamW
+lr = 2e-5
+betas = (0.9, 0.999)
+weight_decay = 0
+max_norm = 1 # grad clip
+warmup_ratio = 0.03
+
+# Save
+save_steps = 500
+save_total_limit = 2 # Maximum checkpoints to keep (-1 means unlimited)
+
+# Evaluate the generation performance during the training
+# TODO: eval
+# evaluation_freq = 500
+
+#######################################################################
+# PART 2 Model & Tokenizer #
+#######################################################################
+tokenizer = dict(
+ type=AutoTokenizer.from_pretrained,
+ pretrained_model_name_or_path=pretrained_model_name_or_path,
+ trust_remote_code=True,
+ padding_side='right')
+
+model = dict(
+ type=RewardModel,
+ use_varlen_attn=use_varlen_attn,
+ loss_type=loss_type,
+ penalty_type=penalty_type,
+ llm=dict(
+ type=AutoModelForCausalLM.from_pretrained,
+ pretrained_model_name_or_path=pretrained_model_name_or_path,
+ trust_remote_code=True))
+
+#######################################################################
+# PART 3 Dataset & Dataloader #
+#######################################################################
+sampler = SequenceParallelSampler \
+ if sequence_parallel_size > 1 else DefaultSampler
+
+train_dataset = dict(
+ type=build_preference_dataset,
+ dataset=dict(
+ type=load_dataset,
+ path='argilla/ultrafeedback-binarized-preferences-cleaned'),
+ tokenizer=tokenizer,
+ max_length=max_length,
+ dataset_map_fn=orpo_dpo_mix_40k_map_fn,
+ is_dpo=False,
+ is_reward=True,
+ reward_token_id=reward_token_id,
+ num_proc=32,
+ use_varlen_attn=use_varlen_attn,
+ max_packed_length=max_packed_length,
+ shuffle_before_pack=True,
+)
+
+train_dataloader = dict(
+ batch_size=batch_size,
+ num_workers=dataloader_num_workers,
+ dataset=train_dataset,
+ sampler=dict(type=sampler, shuffle=True),
+ collate_fn=dict(
+ type=preference_collate_fn, use_varlen_attn=use_varlen_attn))
+
+#######################################################################
+# PART 4 Scheduler & Optimizer #
+#######################################################################
+# optimizer
+optim_wrapper = dict(
+ type=AmpOptimWrapper,
+ optimizer=dict(
+ type=optim_type, lr=lr, betas=betas, weight_decay=weight_decay),
+ clip_grad=dict(max_norm=max_norm, error_if_nonfinite=False),
+ accumulative_counts=accumulative_counts,
+ loss_scale='dynamic',
+ dtype='float16')
+
+# learning policy
+# More information: https://github.com/open-mmlab/mmengine/blob/main/docs/en/tutorials/param_scheduler.md # noqa: E501
+param_scheduler = [
+ dict(
+ type=LinearLR,
+ start_factor=1e-5,
+ by_epoch=True,
+ begin=0,
+ end=warmup_ratio * max_epochs,
+ convert_to_iter_based=True),
+ dict(
+ type=CosineAnnealingLR,
+ eta_min=0.0,
+ by_epoch=True,
+ begin=warmup_ratio * max_epochs,
+ end=max_epochs,
+ convert_to_iter_based=True)
+]
+
+# train, val, test setting
+train_cfg = dict(type=TrainLoop, max_epochs=max_epochs)
+
+#######################################################################
+# PART 5 Runtime #
+#######################################################################
+# Log the dialogue periodically during the training process, optional
+custom_hooks = []
+
+if use_varlen_attn:
+ custom_hooks += [dict(type=VarlenAttnArgsToMessageHubHook)]
+
+# configure default hooks
+default_hooks = dict(
+ # record the time of every iteration.
+ timer=dict(type=IterTimerHook),
+ # print log every 10 iterations.
+ logger=dict(type=LoggerHook, log_metric_by_epoch=False, interval=10),
+ # enable the parameter scheduler.
+ param_scheduler=dict(type=ParamSchedulerHook),
+ # save checkpoint per `save_steps`.
+ checkpoint=dict(
+ type=CheckpointHook,
+ by_epoch=False,
+ interval=save_steps,
+ max_keep_ckpts=save_total_limit),
+ # set sampler seed in distributed evrionment.
+ sampler_seed=dict(type=DistSamplerSeedHook),
+)
+
+# configure environment
+env_cfg = dict(
+ # whether to enable cudnn benchmark
+ cudnn_benchmark=False,
+ # set multi process parameters
+ mp_cfg=dict(mp_start_method='fork', opencv_num_threads=0),
+ # set distributed parameters
+ dist_cfg=dict(backend='nccl'),
+)
+
+# set visualizer
+visualizer = None
+
+# set log level
+log_level = 'INFO'
+
+# load from which checkpoint
+load_from = None
+
+# whether to resume training from the loaded checkpoint
+resume = False
+
+# Defaults to use random seed and disable `deterministic`
+randomness = dict(seed=None, deterministic=False)
+
+# set log processor
+log_processor = dict(by_epoch=False)
diff --git a/xtuner/configs/reward_model/internlm/internlm2_chat_1_8b_reward_qlora_varlenattn_ultrafeedback.py b/xtuner/configs/reward_model/internlm/internlm2_chat_1_8b_reward_qlora_varlenattn_ultrafeedback.py
new file mode 100644
index 000000000..ffcf30cef
--- /dev/null
+++ b/xtuner/configs/reward_model/internlm/internlm2_chat_1_8b_reward_qlora_varlenattn_ultrafeedback.py
@@ -0,0 +1,215 @@
+# Copyright (c) OpenMMLab. All rights reserved.
+import torch
+from datasets import load_dataset
+from mmengine.dataset import DefaultSampler
+from mmengine.hooks import (CheckpointHook, DistSamplerSeedHook, IterTimerHook,
+ LoggerHook, ParamSchedulerHook)
+from mmengine.optim import AmpOptimWrapper, CosineAnnealingLR, LinearLR
+from peft import LoraConfig
+from torch.optim import AdamW
+from transformers import (AutoModelForCausalLM, AutoTokenizer,
+ BitsAndBytesConfig)
+
+from xtuner.dataset.collate_fns.preference_collate_fn import \
+ preference_collate_fn
+from xtuner.dataset.preference_dataset import (build_preference_dataset,
+ orpo_dpo_mix_40k_map_fn)
+from xtuner.engine.hooks import VarlenAttnArgsToMessageHubHook
+from xtuner.engine.runner import TrainLoop
+from xtuner.model.reward import RewardModel
+from xtuner.parallel.sequence import SequenceParallelSampler
+
+#######################################################################
+# PART 1 Settings #
+#######################################################################
+# Model
+pretrained_model_name_or_path = 'internlm/internlm2-chat-1_8b-sft'
+use_varlen_attn = True
+reward_token_id = 92527 # use [UNUSED_TOKEN_130] as reward token
+loss_type = 'focal'
+penalty_type = 'log_barrier'
+
+# Data
+max_length = 2048
+max_packed_length = max_length * 2
+
+# parallel
+sequence_parallel_size = 1
+
+# Scheduler & Optimizer
+batch_size = 1 # per_device
+accumulative_counts = 16
+accumulative_counts *= sequence_parallel_size
+dataloader_num_workers = 0
+max_epochs = 1 # reward model should not be trained for more than 1 epoch to avoid overfitting # noqa: E501
+optim_type = AdamW
+lr = 1e-4
+betas = (0.9, 0.999)
+weight_decay = 0
+max_norm = 1 # grad clip
+warmup_ratio = 0.03
+
+# Save
+save_steps = 500
+save_total_limit = 2 # Maximum checkpoints to keep (-1 means unlimited)
+
+# Evaluate the generation performance during the training
+# TODO: eval
+# evaluation_freq = 500
+
+#######################################################################
+# PART 2 Model & Tokenizer #
+#######################################################################
+tokenizer = dict(
+ type=AutoTokenizer.from_pretrained,
+ pretrained_model_name_or_path=pretrained_model_name_or_path,
+ trust_remote_code=True,
+ padding_side='right')
+
+model = dict(
+ type=RewardModel,
+ use_varlen_attn=use_varlen_attn,
+ loss_type=loss_type,
+ penalty_type=penalty_type,
+ llm=dict(
+ type=AutoModelForCausalLM.from_pretrained,
+ pretrained_model_name_or_path=pretrained_model_name_or_path,
+ trust_remote_code=True,
+ torch_dtype=torch.float16,
+ quantization_config=dict(
+ type=BitsAndBytesConfig,
+ load_in_4bit=True,
+ load_in_8bit=False,
+ llm_int8_threshold=6.0,
+ llm_int8_has_fp16_weight=False,
+ bnb_4bit_compute_dtype=torch.float16,
+ bnb_4bit_use_double_quant=True,
+ bnb_4bit_quant_type='nf4')),
+ lora=dict(
+ type=LoraConfig,
+ r=64,
+ lora_alpha=16,
+ lora_dropout=0.1,
+ bias='none',
+ task_type='FEATURE_EXTRACTION')) # this setting is important
+
+#######################################################################
+# PART 3 Dataset & Dataloader #
+#######################################################################
+sampler = SequenceParallelSampler \
+ if sequence_parallel_size > 1 else DefaultSampler
+
+train_dataset = dict(
+ type=build_preference_dataset,
+ dataset=dict(
+ type=load_dataset,
+ path='argilla/ultrafeedback-binarized-preferences-cleaned'),
+ tokenizer=tokenizer,
+ max_length=max_length,
+ dataset_map_fn=orpo_dpo_mix_40k_map_fn,
+ is_dpo=False,
+ is_reward=True,
+ reward_token_id=reward_token_id,
+ num_proc=32,
+ use_varlen_attn=use_varlen_attn,
+ max_packed_length=max_packed_length,
+ shuffle_before_pack=True,
+)
+
+train_dataloader = dict(
+ batch_size=batch_size,
+ num_workers=dataloader_num_workers,
+ dataset=train_dataset,
+ sampler=dict(type=sampler, shuffle=True),
+ collate_fn=dict(
+ type=preference_collate_fn, use_varlen_attn=use_varlen_attn))
+
+#######################################################################
+# PART 4 Scheduler & Optimizer #
+#######################################################################
+# optimizer
+optim_wrapper = dict(
+ type=AmpOptimWrapper,
+ optimizer=dict(
+ type=optim_type, lr=lr, betas=betas, weight_decay=weight_decay),
+ clip_grad=dict(max_norm=max_norm, error_if_nonfinite=False),
+ accumulative_counts=accumulative_counts,
+ loss_scale='dynamic',
+ dtype='float16')
+
+# learning policy
+# More information: https://github.com/open-mmlab/mmengine/blob/main/docs/en/tutorials/param_scheduler.md # noqa: E501
+param_scheduler = [
+ dict(
+ type=LinearLR,
+ start_factor=1e-5,
+ by_epoch=True,
+ begin=0,
+ end=warmup_ratio * max_epochs,
+ convert_to_iter_based=True),
+ dict(
+ type=CosineAnnealingLR,
+ eta_min=0.0,
+ by_epoch=True,
+ begin=warmup_ratio * max_epochs,
+ end=max_epochs,
+ convert_to_iter_based=True)
+]
+
+# train, val, test setting
+train_cfg = dict(type=TrainLoop, max_epochs=max_epochs)
+
+#######################################################################
+# PART 5 Runtime #
+#######################################################################
+# Log the dialogue periodically during the training process, optional
+custom_hooks = []
+
+if use_varlen_attn:
+ custom_hooks += [dict(type=VarlenAttnArgsToMessageHubHook)]
+
+# configure default hooks
+default_hooks = dict(
+ # record the time of every iteration.
+ timer=dict(type=IterTimerHook),
+ # print log every 10 iterations.
+ logger=dict(type=LoggerHook, log_metric_by_epoch=False, interval=10),
+ # enable the parameter scheduler.
+ param_scheduler=dict(type=ParamSchedulerHook),
+ # save checkpoint per `save_steps`.
+ checkpoint=dict(
+ type=CheckpointHook,
+ by_epoch=False,
+ interval=save_steps,
+ max_keep_ckpts=save_total_limit),
+ # set sampler seed in distributed evrionment.
+ sampler_seed=dict(type=DistSamplerSeedHook),
+)
+
+# configure environment
+env_cfg = dict(
+ # whether to enable cudnn benchmark
+ cudnn_benchmark=False,
+ # set multi process parameters
+ mp_cfg=dict(mp_start_method='fork', opencv_num_threads=0),
+ # set distributed parameters
+ dist_cfg=dict(backend='nccl'),
+)
+
+# set visualizer
+visualizer = None
+
+# set log level
+log_level = 'INFO'
+
+# load from which checkpoint
+load_from = None
+
+# whether to resume training from the loaded checkpoint
+resume = False
+
+# Defaults to use random seed and disable `deterministic`
+randomness = dict(seed=None, deterministic=False)
+
+# set log processor
+log_processor = dict(by_epoch=False)
diff --git a/xtuner/configs/reward_model/llama/llama3_8b_instruct_reward_full_varlenattn_ultrafeedback.py b/xtuner/configs/reward_model/llama/llama3_8b_instruct_reward_full_varlenattn_ultrafeedback.py
new file mode 100644
index 000000000..57d822a05
--- /dev/null
+++ b/xtuner/configs/reward_model/llama/llama3_8b_instruct_reward_full_varlenattn_ultrafeedback.py
@@ -0,0 +1,195 @@
+# Copyright (c) OpenMMLab. All rights reserved.
+from datasets import load_dataset
+from mmengine.dataset import DefaultSampler
+from mmengine.hooks import (CheckpointHook, DistSamplerSeedHook, IterTimerHook,
+ LoggerHook, ParamSchedulerHook)
+from mmengine.optim import AmpOptimWrapper, CosineAnnealingLR, LinearLR
+from torch.optim import AdamW
+from transformers import AutoModelForCausalLM, AutoTokenizer
+
+from xtuner.dataset.collate_fns.preference_collate_fn import \
+ preference_collate_fn
+from xtuner.dataset.preference_dataset import (build_preference_dataset,
+ orpo_dpo_mix_40k_map_fn)
+from xtuner.engine.hooks import VarlenAttnArgsToMessageHubHook
+from xtuner.engine.runner import TrainLoop
+from xtuner.model.reward import RewardModel
+from xtuner.parallel.sequence import SequenceParallelSampler
+
+#######################################################################
+# PART 1 Settings #
+#######################################################################
+# Model
+pretrained_model_name_or_path = 'meta-llama/Meta-Llama-3-8B-Instruct'
+use_varlen_attn = True
+reward_token_id = 128002 # use <|reserved_special_token_0|> as reward token
+loss_type = 'focal'
+penalty_type = 'log_barrier'
+
+# Data
+max_length = 2048
+max_packed_length = max_length * 2
+
+# parallel
+sequence_parallel_size = 1
+
+# Scheduler & Optimizer
+batch_size = 1 # per_device
+accumulative_counts = 16
+accumulative_counts *= sequence_parallel_size
+dataloader_num_workers = 0
+max_epochs = 1 # reward model should not be trained for more than 1 epoch to avoid overfitting # noqa: E501
+optim_type = AdamW
+lr = 2e-5
+betas = (0.9, 0.999)
+weight_decay = 0
+max_norm = 1 # grad clip
+warmup_ratio = 0.03
+
+# Save
+save_steps = 500
+save_total_limit = 2 # Maximum checkpoints to keep (-1 means unlimited)
+
+# Evaluate the generation performance during the training
+# TODO: eval
+# evaluation_freq = 500
+
+#######################################################################
+# PART 2 Model & Tokenizer #
+#######################################################################
+tokenizer = dict(
+ type=AutoTokenizer.from_pretrained,
+ pretrained_model_name_or_path=pretrained_model_name_or_path,
+ trust_remote_code=True,
+ padding_side='right')
+
+model = dict(
+ type=RewardModel,
+ use_varlen_attn=use_varlen_attn,
+ loss_type=loss_type,
+ penalty_type=penalty_type,
+ llm=dict(
+ type=AutoModelForCausalLM.from_pretrained,
+ pretrained_model_name_or_path=pretrained_model_name_or_path,
+ trust_remote_code=True))
+
+#######################################################################
+# PART 3 Dataset & Dataloader #
+#######################################################################
+sampler = SequenceParallelSampler \
+ if sequence_parallel_size > 1 else DefaultSampler
+
+train_dataset = dict(
+ type=build_preference_dataset,
+ dataset=dict(
+ type=load_dataset,
+ path='argilla/ultrafeedback-binarized-preferences-cleaned'),
+ tokenizer=tokenizer,
+ max_length=max_length,
+ dataset_map_fn=orpo_dpo_mix_40k_map_fn,
+ is_dpo=False,
+ is_reward=True,
+ reward_token_id=reward_token_id,
+ num_proc=32,
+ use_varlen_attn=use_varlen_attn,
+ max_packed_length=max_packed_length,
+ shuffle_before_pack=True,
+)
+
+train_dataloader = dict(
+ batch_size=batch_size,
+ num_workers=dataloader_num_workers,
+ dataset=train_dataset,
+ sampler=dict(type=sampler, shuffle=True),
+ collate_fn=dict(
+ type=preference_collate_fn, use_varlen_attn=use_varlen_attn))
+
+#######################################################################
+# PART 4 Scheduler & Optimizer #
+#######################################################################
+# optimizer
+optim_wrapper = dict(
+ type=AmpOptimWrapper,
+ optimizer=dict(
+ type=optim_type, lr=lr, betas=betas, weight_decay=weight_decay),
+ clip_grad=dict(max_norm=max_norm, error_if_nonfinite=False),
+ accumulative_counts=accumulative_counts,
+ loss_scale='dynamic',
+ dtype='float16')
+
+# learning policy
+# More information: https://github.com/open-mmlab/mmengine/blob/main/docs/en/tutorials/param_scheduler.md # noqa: E501
+param_scheduler = [
+ dict(
+ type=LinearLR,
+ start_factor=1e-5,
+ by_epoch=True,
+ begin=0,
+ end=warmup_ratio * max_epochs,
+ convert_to_iter_based=True),
+ dict(
+ type=CosineAnnealingLR,
+ eta_min=0.0,
+ by_epoch=True,
+ begin=warmup_ratio * max_epochs,
+ end=max_epochs,
+ convert_to_iter_based=True)
+]
+
+# train, val, test setting
+train_cfg = dict(type=TrainLoop, max_epochs=max_epochs)
+
+#######################################################################
+# PART 5 Runtime #
+#######################################################################
+# Log the dialogue periodically during the training process, optional
+custom_hooks = []
+
+if use_varlen_attn:
+ custom_hooks += [dict(type=VarlenAttnArgsToMessageHubHook)]
+
+# configure default hooks
+default_hooks = dict(
+ # record the time of every iteration.
+ timer=dict(type=IterTimerHook),
+ # print log every 10 iterations.
+ logger=dict(type=LoggerHook, log_metric_by_epoch=False, interval=10),
+ # enable the parameter scheduler.
+ param_scheduler=dict(type=ParamSchedulerHook),
+ # save checkpoint per `save_steps`.
+ checkpoint=dict(
+ type=CheckpointHook,
+ by_epoch=False,
+ interval=save_steps,
+ max_keep_ckpts=save_total_limit),
+ # set sampler seed in distributed evrionment.
+ sampler_seed=dict(type=DistSamplerSeedHook),
+)
+
+# configure environment
+env_cfg = dict(
+ # whether to enable cudnn benchmark
+ cudnn_benchmark=False,
+ # set multi process parameters
+ mp_cfg=dict(mp_start_method='fork', opencv_num_threads=0),
+ # set distributed parameters
+ dist_cfg=dict(backend='nccl'),
+)
+
+# set visualizer
+visualizer = None
+
+# set log level
+log_level = 'INFO'
+
+# load from which checkpoint
+load_from = None
+
+# whether to resume training from the loaded checkpoint
+resume = False
+
+# Defaults to use random seed and disable `deterministic`
+randomness = dict(seed=None, deterministic=False)
+
+# set log processor
+log_processor = dict(by_epoch=False)
diff --git a/xtuner/dataset/__init__.py b/xtuner/dataset/__init__.py
index 19ef58b9d..8f679a8cd 100644
--- a/xtuner/dataset/__init__.py
+++ b/xtuner/dataset/__init__.py
@@ -6,6 +6,8 @@
from .intern_repo import (build_packed_dataset,
load_intern_repo_tokenized_dataset,
load_intern_repo_untokenized_dataset)
+from .internvl_dataset import InternVL_V1_5_Dataset
+from .json_dataset import load_json_file
from .llava import LLaVADataset
from .modelscope import process_ms_dataset
from .moss_sft import MOSSSFTDataset
@@ -17,19 +19,11 @@
warnings.simplefilter(action='ignore', category=FutureWarning)
__all__ = [
- 'process_hf_dataset',
- 'ConcatDataset',
- 'MOSSSFTDataset',
- 'process_ms_dataset',
- 'LLaVADataset',
- 'expand2square',
- 'decode_base64_to_image',
- 'load_image',
- 'process_ms_dataset',
+ 'process_hf_dataset', 'ConcatDataset', 'MOSSSFTDataset',
+ 'process_ms_dataset', 'LLaVADataset', 'expand2square',
+ 'decode_base64_to_image', 'load_image',
'load_intern_repo_tokenized_dataset',
- 'load_intern_repo_untokenized_dataset',
- 'build_packed_dataset',
- 'RefCOCOJsonDataset',
- 'RefCOCOJsonEvalDataset',
- 'InvRefCOCOJsonDataset',
+ 'load_intern_repo_untokenized_dataset', 'build_packed_dataset',
+ 'RefCOCOJsonDataset', 'RefCOCOJsonEvalDataset', 'InvRefCOCOJsonDataset',
+ 'load_json_file', 'InternVL_V1_5_Dataset'
]
diff --git a/xtuner/dataset/collate_fns/__init__.py b/xtuner/dataset/collate_fns/__init__.py
index 0d2d1febe..96652b259 100644
--- a/xtuner/dataset/collate_fns/__init__.py
+++ b/xtuner/dataset/collate_fns/__init__.py
@@ -1,5 +1,5 @@
# Copyright (c) OpenMMLab. All rights reserved.
-from .defalut_collate_fn import default_collate_fn
+from .default_collate_fn import default_collate_fn
from .mmlu_collate_fn import mmlu_collate_fn
__all__ = ['default_collate_fn', 'mmlu_collate_fn']
diff --git a/xtuner/dataset/collate_fns/defalut_collate_fn.py b/xtuner/dataset/collate_fns/default_collate_fn.py
similarity index 73%
rename from xtuner/dataset/collate_fns/defalut_collate_fn.py
rename to xtuner/dataset/collate_fns/default_collate_fn.py
index f644df9cf..3d9fe18fb 100644
--- a/xtuner/dataset/collate_fns/defalut_collate_fn.py
+++ b/xtuner/dataset/collate_fns/default_collate_fn.py
@@ -5,8 +5,7 @@
from torch.nn.utils.rnn import pad_sequence
from xtuner.parallel.sequence import (get_sequence_parallel_world_size,
- pad_for_sequence_parallel,
- split_for_sequence_parallel)
+ pad_for_sequence_parallel)
from xtuner.utils import DEFAULT_PAD_TOKEN_INDEX, IGNORE_INDEX
@@ -39,6 +38,7 @@ def default_collate_fn(instances: Sequence[Dict],
if has_image:
pixel_values.append(example['pixel_values'])
+ ori_length = [len(ids) for ids in input_ids]
if len(instances) > 1:
input_ids = pad_sequence(
input_ids, batch_first=True, padding_value=pad_index)
@@ -53,16 +53,21 @@ def default_collate_fn(instances: Sequence[Dict],
attention_mask = None
position_ids = torch.stack(position_ids, dim=0)
else:
- attention_mask = input_ids.ne(pad_index)
- position_ids = attention_mask.long().cumsum(-1) - 1
+ # Some tokenizers have the same eos token and pad token, so input_ids
+ # cannot be masked directly based on the pad token id.
+ attention_mask = torch.zeros_like(input_ids).bool()
+ for i, length in enumerate(ori_length):
+ attention_mask[i, :length] = True
- input_ids, labels, position_ids, attention_mask = \
- pad_for_sequence_parallel(input_ids, labels, position_ids,
- attention_mask)
+ bs, seq_len = input_ids.shape
+ position_ids = torch.arange(seq_len).unsqueeze(0).long().repeat(bs, 1)
- # attention mask should not be split
- input_ids, labels, position_ids = split_for_sequence_parallel(
- input_ids, labels, position_ids)
+ if seq_parallel_world_size > 1:
+ input_ids = pad_for_sequence_parallel(input_ids, pad_index)
+ labels = pad_for_sequence_parallel(labels, IGNORE_INDEX)
+ position_ids = pad_for_sequence_parallel(position_ids, 0)
+ if attention_mask is not None:
+ attention_mask = pad_for_sequence_parallel(attention_mask, 0)
if use_varlen_attn:
max_seqlen = (
@@ -84,7 +89,8 @@ def default_collate_fn(instances: Sequence[Dict],
}
if has_image:
- pixel_values = torch.stack(pixel_values)
+ if all(x.shape == pixel_values[0].shape for x in pixel_values):
+ pixel_values = torch.stack(pixel_values, dim=0)
data_dict['pixel_values'] = pixel_values
if return_hf_format:
diff --git a/xtuner/dataset/collate_fns/preference_collate_fn.py b/xtuner/dataset/collate_fns/preference_collate_fn.py
new file mode 100644
index 000000000..4b6a7f5c3
--- /dev/null
+++ b/xtuner/dataset/collate_fns/preference_collate_fn.py
@@ -0,0 +1,109 @@
+# Copyright (c) OpenMMLab. All rights reserved.
+from typing import Dict, Sequence
+
+import torch
+from torch.nn.utils.rnn import pad_sequence
+
+from xtuner.parallel.sequence import (get_sequence_parallel_world_size,
+ pad_cumulative_len_for_sequence_parallel,
+ pad_for_sequence_parallel)
+from xtuner.utils import DEFAULT_PAD_TOKEN_INDEX, IGNORE_INDEX
+
+
+def preference_collate_fn(instances: Sequence[Dict],
+ pad_index: int = DEFAULT_PAD_TOKEN_INDEX,
+ return_hf_format: bool = False,
+ use_varlen_attn: bool = False):
+ seq_parallel_world_size = get_sequence_parallel_world_size()
+ ds_names = []
+ if not use_varlen_attn:
+ # split chosen and rejected into two instances
+ splited_instances = []
+ for d in instances:
+ splited_instances.append({
+ 'input_ids': d['chosen_ids'],
+ 'labels': d['chosen_labels']
+ })
+ splited_instances.append({
+ 'input_ids': d['rejected_ids'],
+ 'labels': d['rejected_labels']
+ })
+ ds_names.append(d.get('ds_name', None))
+ instances = splited_instances
+
+ input_ids, labels = [], []
+ if use_varlen_attn:
+ position_ids, cumulative_len = [], []
+ assert len(instances) == 1, (
+ f'If utilizing varlen attention, the batch size should be'
+ f' set to 1, but got {len(instances)}')
+
+ for example in instances:
+ input_ids.append(torch.LongTensor(example['input_ids']))
+ labels.append(torch.LongTensor(example['labels']))
+ if use_varlen_attn:
+ cumulative_len.append(torch.IntTensor(example['cumulative_len']))
+ position_ids.append(torch.LongTensor(example['position_ids']))
+ num_samples = (len(example['cumulative_len']) - 1) // 2
+ ds_names.extend(example.get('ds_names', [None] * num_samples))
+
+ ori_length = [len(ids) for ids in input_ids]
+ if len(instances) > 1:
+ input_ids = pad_sequence(
+ input_ids, batch_first=True, padding_value=pad_index)
+ labels = pad_sequence(
+ labels, batch_first=True, padding_value=IGNORE_INDEX)
+ else:
+ input_ids = torch.stack(input_ids)
+ labels = torch.stack(labels)
+
+ if use_varlen_attn:
+ attention_mask = None
+ position_ids = torch.stack(position_ids, dim=0)
+ else:
+ # Some tokenizers have the same eos token and pad token, so input_ids
+ # cannot be masked directly based on the pad token id.
+ attention_mask = torch.zeros_like(input_ids).bool()
+ for i, length in enumerate(ori_length):
+ attention_mask[i, :length] = True
+
+ bs, seq_len = input_ids.shape
+ position_ids = torch.arange(seq_len).unsqueeze(0).long().repeat(bs, 1)
+
+ if seq_parallel_world_size > 1:
+ input_ids = pad_for_sequence_parallel(input_ids, pad_index)
+ labels = pad_for_sequence_parallel(labels, IGNORE_INDEX)
+ position_ids = pad_for_sequence_parallel(position_ids, 0)
+ if attention_mask is not None:
+ attention_mask = pad_for_sequence_parallel(attention_mask, 0)
+ if use_varlen_attn:
+ # We use attention_mask to distinguish `input_ids` from
+ # (sequence parallel) pad tokens in `get_var_len_atten_logps`
+ # method of class `DPO` and `ORPO`
+ (cumulative_len, attention_mask
+ ) = pad_cumulative_len_for_sequence_parallel(cumulative_len)
+
+ if use_varlen_attn:
+ max_seqlen = (
+ cumulative_len[0][1:] - # noqa: W504
+ cumulative_len[0][:-1]).max().item()
+ data_dict = {
+ 'input_ids': input_ids,
+ 'attention_mask': attention_mask,
+ 'cumulative_len': cumulative_len,
+ 'position_ids': position_ids,
+ 'labels': labels,
+ 'max_seqlen': max_seqlen
+ }
+ else:
+ data_dict = {
+ 'input_ids': input_ids,
+ 'attention_mask': attention_mask,
+ 'position_ids': position_ids,
+ 'labels': labels
+ }
+
+ if return_hf_format:
+ return data_dict
+ else:
+ return {'data': data_dict, 'data_samples': {'ds_names': ds_names}}
diff --git a/xtuner/dataset/huggingface.py b/xtuner/dataset/huggingface.py
index 30f6bc394..c44e88688 100644
--- a/xtuner/dataset/huggingface.py
+++ b/xtuner/dataset/huggingface.py
@@ -298,7 +298,7 @@ def process_hf_dataset(dataset,
return process(**kwargs)
xtuner_dataset_timeout = timedelta(
- minutes=int(os.getenv('XTUNER_DATASET_TIMEOUT', default=30)))
+ minutes=int(os.getenv('XTUNER_DATASET_TIMEOUT', default=60)))
print_log(
f'xtuner_dataset_timeout = {xtuner_dataset_timeout}', logger='current')
# monitored barrier requires gloo process group to perform host-side sync.
diff --git a/xtuner/dataset/internvl_dataset.py b/xtuner/dataset/internvl_dataset.py
new file mode 100644
index 000000000..82904ae87
--- /dev/null
+++ b/xtuner/dataset/internvl_dataset.py
@@ -0,0 +1,409 @@
+import copy
+import io
+import json
+import os
+import random
+import warnings
+
+import numpy as np
+import torch
+import torchvision.transforms as T
+from mmengine import print_log
+from mmengine.fileio import get
+from PIL import Image
+from torch.utils.data import Dataset
+from torchvision.transforms.functional import InterpolationMode
+from transformers import AutoConfig, AutoTokenizer
+
+from xtuner.utils import IGNORE_INDEX
+
+
+# Referenced from InternVL
+def find_closest_aspect_ratio(aspect_ratio, target_ratios, width, height,
+ image_size):
+ best_ratio_diff = float('inf')
+ best_ratio = (1, 1)
+ area = width * height
+ for ratio in target_ratios:
+ target_aspect_ratio = ratio[0] / ratio[1]
+ ratio_diff = abs(aspect_ratio - target_aspect_ratio)
+ if ratio_diff < best_ratio_diff:
+ best_ratio_diff = ratio_diff
+ best_ratio = ratio
+ elif ratio_diff == best_ratio_diff:
+ if area > 0.5 * image_size * image_size * ratio[0] * ratio[1]:
+ best_ratio = ratio
+ return best_ratio
+
+
+def dynamic_preprocess(image,
+ min_num=1,
+ max_num=6,
+ image_size=448,
+ use_thumbnail=False):
+ orig_width, orig_height = image.size
+ aspect_ratio = orig_width / orig_height
+
+ # calculate the existing image aspect ratio
+ target_ratios = {(i, j)
+ for n in range(min_num, max_num + 1)
+ for i in range(1, n + 1) for j in range(1, n + 1)
+ if i * j <= max_num and i * j >= min_num}
+ target_ratios = sorted(target_ratios, key=lambda x: x[0] * x[1])
+
+ # find the closest aspect ratio to the target
+ target_aspect_ratio = find_closest_aspect_ratio(aspect_ratio,
+ target_ratios, orig_width,
+ orig_height, image_size)
+
+ # calculate the target width and height
+ target_width = image_size * target_aspect_ratio[0]
+ target_height = image_size * target_aspect_ratio[1]
+ blocks = target_aspect_ratio[0] * target_aspect_ratio[1]
+
+ # resize the image
+ resized_img = image.resize((target_width, target_height))
+ processed_images = []
+ for i in range(blocks):
+ box = ((i % (target_width // image_size)) * image_size,
+ (i // (target_width // image_size)) * image_size,
+ ((i % (target_width // image_size)) + 1) * image_size,
+ ((i // (target_width // image_size)) + 1) * image_size)
+ # split the image
+ split_img = resized_img.crop(box)
+ processed_images.append(split_img)
+ assert len(processed_images) == blocks
+ if use_thumbnail and len(processed_images) != 1:
+ thumbnail_img = image.resize((image_size, image_size))
+ processed_images.append(thumbnail_img)
+ return processed_images
+
+
+def total_image_token(orig_size,
+ min_num=1,
+ max_num=12,
+ image_size=448,
+ use_thumbnail=True):
+ orig_width, orig_height = orig_size
+
+ aspect_ratio = orig_width / orig_height
+
+ # calculate the existing image aspect ratio
+ target_ratios = {(i, j)
+ for n in range(min_num, max_num + 1)
+ for i in range(1, n + 1) for j in range(1, n + 1)
+ if max_num >= i * j >= min_num}
+ target_ratios = sorted(target_ratios, key=lambda x: x[0] * x[1])
+
+ # find the closest aspect ratio to the target
+ target_aspect_ratio = find_closest_aspect_ratio(aspect_ratio,
+ target_ratios, orig_width,
+ orig_height, image_size)
+ blocks = target_aspect_ratio[0] * target_aspect_ratio[1]
+
+ if use_thumbnail:
+ blocks += 1
+
+ return blocks
+
+
+def load_json_or_jsonl(json_path):
+ if json_path.endswith('.json'):
+ with open(json_path) as f:
+ data = json.load(f)
+ elif json_path.endswith('.jsonl'):
+ with open(json_path) as f:
+ data = [json.loads(line) for line in f]
+ else:
+ raise ValueError(f'Unsupported file format: {json_path}, '
+ f'only support .json and .jsonl.')
+ return data
+
+
+class InternVL_V1_5_Dataset(Dataset):
+ os.environ['TOKENIZERS_PARALLELISM'] = 'true'
+ IMG_CONTEXT_TOKEN = ''
+ IMG_START_TOKEN = '
'
+ IMG_END_TOKEN = ''
+
+ IMAGENET_MEAN = (0.485, 0.456, 0.406)
+ IMAGENET_STD = (0.229, 0.224, 0.225)
+
+ def __init__(self,
+ model_path,
+ template,
+ data_paths,
+ image_folders=None,
+ repeat_times=1,
+ max_length=8192):
+ self.template = template
+ self.max_length = max_length
+
+ self.cfg = AutoConfig.from_pretrained(
+ model_path, trust_remote_code=True)
+
+ # The following modifications are only to ensure full
+ # consistency with the official template,
+ # without investigating the impact on performance.
+ if self.cfg.llm_config.architectures[0] == 'Phi3ForCausalLM':
+ self._system = 'You are an AI assistant whose name is Phi-3.'
+ self.template[
+ 'INSTRUCTION'] = '<|user|>\n{input}<|end|><|assistant|>\n'
+ elif self.cfg.llm_config.architectures[0] == 'InternLM2ForCausalLM':
+ self._system = 'You are an AI assistant whose name ' \
+ 'is InternLM (书生·浦语).'
+ self.template['SYSTEM'] = '<|im_start|>system\n{system}<|im_end|>'
+ self.template[
+ 'INSTRUCTION'] = '<|im_start|>user\n{input}' \
+ '<|im_end|><|im_start|>assistant\n'
+ else:
+ raise NotImplementedError
+
+ self.min_dynamic_patch = self.cfg.min_dynamic_patch
+ self.max_dynamic_patch = self.cfg.max_dynamic_patch
+ self.downsample_ratio = self.cfg.downsample_ratio
+ self.image_size = self.cfg.force_image_size
+ self.use_thumbnail = self.cfg.use_thumbnail
+ patch_size = self.cfg.vision_config.patch_size
+ self.patch_token = int(
+ (self.image_size // patch_size)**2 * (self.downsample_ratio**2))
+ self.tokenizer = AutoTokenizer.from_pretrained(
+ model_path, trust_remote_code=True)
+ self.transformer = T.Compose([
+ T.Lambda(lambda img: img.convert('RGB')
+ if img.mode != 'RGB' else img),
+ T.Resize((self.image_size, self.image_size),
+ interpolation=InterpolationMode.BICUBIC),
+ T.ToTensor(),
+ T.Normalize(mean=self.IMAGENET_MEAN, std=self.IMAGENET_STD)
+ ])
+
+ if not isinstance(data_paths, (list, tuple)):
+ data_paths = [data_paths]
+ if not isinstance(image_folders, (list, tuple)):
+ image_folders = [image_folders]
+ if not isinstance(repeat_times, (list, tuple)):
+ repeat_times = [repeat_times]
+ assert len(data_paths) == len(image_folders) == len(repeat_times)
+
+ print_log('Starting to loading data and calc length', logger='current')
+ self.data = []
+ self.image_folder = []
+ self.group_length = []
+ self.conv2length_text = {
+ } # using dict to speedup the calculation of token length
+
+ for data_file, image_folder, repeat_time in zip(
+ data_paths, image_folders, repeat_times):
+ print_log(
+ f'=======Starting to process {data_file} =======',
+ logger='current')
+ assert repeat_time > 0
+ json_data = load_json_or_jsonl(data_file)
+ if repeat_time < 1:
+ json_data = random.sample(json_data,
+ int(len(json_data) * repeat_time))
+ elif repeat_time > 1:
+ int_repeat_time = int(repeat_time)
+ remaining_repeat_time = repeat_time - repeat_time
+ if remaining_repeat_time > 0:
+ remaining_json_data = random.sample(
+ json_data, int(len(json_data) * remaining_repeat_time))
+ json_data = json_data * int_repeat_time
+ json_data.extend(remaining_json_data)
+ else:
+ json_data = json_data * int_repeat_time
+
+ self.data.extend(json_data)
+ self.image_folder.extend([image_folder] * len(json_data))
+
+ # TODO: multi process
+ for data_item in json_data:
+ if 'length' in data_item:
+ token_length = data_item['length'] # include image token
+ else:
+ conversations = '\n'.join(
+ [temp['value'] for temp in data_item['conversations']])
+ str_length = len(conversations)
+
+ if str_length not in self.conv2length_text:
+ token_length = self.tokenizer(
+ conversations,
+ return_tensors='pt',
+ padding=False,
+ truncation=False,
+ ).input_ids.size(1)
+ self.conv2length_text[str_length] = token_length
+ else:
+ token_length = self.conv2length_text[str_length]
+
+ if 'image' in data_item and data_item['image'] is not None:
+ if 'image_wh' in data_item and data_item[
+ 'image_wh'] is not None:
+ # more accurate calculation of image token
+ image_wh = data_item['image_wh']
+ if isinstance(image_wh[0], list):
+ image_wh = image_wh[0]
+ image_token = total_image_token(
+ image_wh, self.min_dynamic_patch,
+ self.max_dynamic_patch, self.image_size,
+ self.use_thumbnail)
+ image_token = self.patch_token * image_token
+ else:
+ # max_dynamic_patch + use_thumbnail
+ image_token = self.patch_token * (
+ self.max_dynamic_patch + self.use_thumbnail)
+
+ token_length = token_length + image_token
+ else:
+ token_length = -token_length
+
+ self.group_length.append(token_length)
+ print_log(
+ f'=======total {len(json_data)} samples of {data_file}=======',
+ logger='current')
+
+ assert len(self.group_length) == len(self.data)
+ print_log('end loading data and calc length', logger='current')
+ print_log(
+ f'=======total {len(self.data)} samples=======', logger='current')
+ self._max_refetch = 1000
+
+ def __getitem__(self, index):
+ for _ in range(self._max_refetch + 1):
+ data = self.prepare_data(index)
+ # Broken images may cause the returned data to be None
+ if data is None:
+ index = self._rand_another()
+ continue
+ return data
+
+ def __len__(self):
+ return len(self.data)
+
+ @property
+ def modality_length(self):
+ return self.group_length
+
+ @property
+ def length(self):
+ group_length = np.array(self.group_length)
+ group_length = np.abs(group_length).tolist()
+ return group_length
+
+ def prepare_data(self, index):
+ data_dict: dict = self.data[index]
+ image_folder = self.image_folder[index]
+
+ out_data_dict = {}
+ if data_dict.get('image', None) is not None:
+ image_file = data_dict['image']
+ if isinstance(image_file, (list, tuple)):
+ assert len(image_file) == 1
+ image_file = image_file[0]
+
+ try:
+ image = self.get_image(os.path.join(image_folder, image_file))
+ except Exception as e:
+ print(f'Error: {e}', flush=True)
+ print_log(f'Error: {e}', logger='current')
+ return None
+
+ images = dynamic_preprocess(image, self.min_dynamic_patch,
+ self.max_dynamic_patch,
+ self.image_size, self.use_thumbnail)
+ pixel_values = [self.transformer(image) for image in images]
+ pixel_values = torch.stack(pixel_values)
+ out_data_dict['pixel_values'] = pixel_values
+
+ num_image_tokens = pixel_values.shape[0] * self.patch_token
+ image_token_str = f'{self.IMG_START_TOKEN}' \
+ f'{self.IMG_CONTEXT_TOKEN * num_image_tokens}' \
+ f'{self.IMG_END_TOKEN}'
+ token_dict = self.get_inputid_labels(data_dict['conversations'],
+ image_token_str)
+ out_data_dict.update(token_dict)
+ else:
+ token_dict = self.get_inputid_labels(data_dict['conversations'],
+ None)
+ out_data_dict.update(token_dict)
+ out_data_dict['pixel_values'] = torch.zeros(
+ 1, 3, self.image_size, self.image_size)
+ return out_data_dict
+
+ def _rand_another(self) -> int:
+ return np.random.randint(0, len(self.data))
+
+ def get_image(self, path):
+ if 's3://' in path:
+ img_bytes = get(path)
+ with io.BytesIO(img_bytes) as buff:
+ img = Image.open(buff).convert('RGB')
+ return img
+ else:
+ return Image.open(path).convert('RGB')
+
+ def get_inputid_labels(self, conversations, image_token_str) -> dict:
+ input = ''
+ out_conversation = []
+ while conversations and conversations[0]['from'] == 'gpt':
+ # Skip the first one if it is from gpt
+ conversations = conversations[1:]
+ for msg in conversations:
+ if msg['from'] == 'human':
+ if image_token_str is None and '' in msg['value']:
+ warnings.warn(
+ f'The current data << {msg["value"]} >> is '
+ f'in plain text mode, but '
+ 'there are tags present in the data. '
+ 'We need to remove the tags.')
+ msg['value'] = msg['value'].replace('', '')
+ if '' in msg['value']:
+ msg['value'] = msg['value'].replace('', '').strip()
+ msg['value'] = image_token_str + '\n' + msg['value']
+ msg['value'] = msg['value'].strip()
+ input += msg['value'].strip()
+ elif msg['from'] == 'gpt':
+ out_conversation.append({
+ 'input': input,
+ 'output': msg['value'].strip()
+ })
+ input = ''
+ else:
+ raise NotImplementedError
+
+ input_ids, labels = [], []
+ for i, single_turn_conversation in enumerate(out_conversation):
+ input = single_turn_conversation.get('input', '')
+ if input is None:
+ input = ''
+ input_text = self.template.INSTRUCTION.format(
+ input=input, round=i + 1)
+
+ if i == 0:
+ system = self.template.SYSTEM.format(system=self._system)
+ input_text = system + input_text
+ input_encode = self.tokenizer.encode(
+ input_text, add_special_tokens=True)
+ else:
+ input_encode = self.tokenizer.encode(
+ input_text, add_special_tokens=False)
+ input_ids += input_encode
+ labels += [IGNORE_INDEX] * len(input_encode)
+
+ output_text = single_turn_conversation.get('output', '')
+ if self.template.get('SUFFIX', None):
+ output_text += self.template.SUFFIX
+ output_encode = self.tokenizer.encode(
+ output_text, add_special_tokens=False)
+ input_ids += output_encode
+ labels += copy.deepcopy(output_encode)
+
+ if len(input_ids) > self.max_length:
+ input_ids = input_ids[:self.max_length]
+ labels = labels[:self.max_length]
+ print_log(
+ f'Warning: input_ids length({len(input_ids)}) '
+ f'is longer than max_length, cut to {self.max_length}',
+ logger='current')
+ return {'input_ids': input_ids, 'labels': labels}
diff --git a/xtuner/dataset/json_dataset.py b/xtuner/dataset/json_dataset.py
new file mode 100644
index 000000000..1c7ca0163
--- /dev/null
+++ b/xtuner/dataset/json_dataset.py
@@ -0,0 +1,24 @@
+import json
+import os
+
+from datasets import Dataset, concatenate_datasets
+
+
+def load_json_file(data_files=None, data_dir=None, suffix=None):
+ assert (data_files is not None) != (data_dir is not None)
+ if data_dir is not None:
+ data_files = os.listdir(data_dir)
+ data_files = [os.path.join(data_dir, fn) for fn in data_files]
+ if suffix is not None:
+ data_files = [fp for fp in data_files if fp.endswith(suffix)]
+ elif isinstance(data_files, str):
+ data_files = [data_files]
+
+ dataset_list = []
+ for fp in data_files:
+ with open(fp, encoding='utf-8') as file:
+ data = json.load(file)
+ ds = Dataset.from_list(data)
+ dataset_list.append(ds)
+ dataset = concatenate_datasets(dataset_list)
+ return dataset
diff --git a/xtuner/dataset/llava.py b/xtuner/dataset/llava.py
index 1c337c877..0fab0258a 100644
--- a/xtuner/dataset/llava.py
+++ b/xtuner/dataset/llava.py
@@ -16,6 +16,15 @@
from .utils import expand2square
+def load_jsonl(json_file):
+ with open(json_file) as f:
+ lines = f.readlines()
+ data = []
+ for line in lines:
+ data.append(json.loads(line))
+ return data
+
+
class LLaVADataset(Dataset):
def __init__(self,
@@ -44,7 +53,13 @@ def __init__(self,
if offline_processed_text_folder is not None:
self.text_data = load_from_disk(offline_processed_text_folder)
else:
- json_data = json.load(open(data_path))
+ if data_path.endswith('.json'):
+ json_data = json.load(open(data_path))
+ elif data_path.endswith('.jsonl'):
+ json_data = load_jsonl(data_path)
+ else:
+ raise NotImplementedError
+
for idx in range(len(json_data)):
if isinstance(json_data[idx]['id'], int):
json_data[idx]['id'] = str(json_data[idx]['id'])
diff --git a/xtuner/dataset/map_fns/dataset_map_fns/openai_map_fn.py b/xtuner/dataset/map_fns/dataset_map_fns/openai_map_fn.py
index 64ed642f6..468e738f7 100644
--- a/xtuner/dataset/map_fns/dataset_map_fns/openai_map_fn.py
+++ b/xtuner/dataset/map_fns/dataset_map_fns/openai_map_fn.py
@@ -32,10 +32,14 @@ def openai_map_fn(example):
elif msg['role'] == 'user':
input += msg['content']
elif msg['role'] == 'assistant':
+ output_with_loss = msg.get('loss', 'True')
+ output_with_loss = str(output_with_loss)
+ output_with_loss = output_with_loss.lower() == 'true'
conversation.append({
'system': system,
'input': input,
- 'output': msg['content']
+ 'output': msg['content'],
+ 'output_with_loss': output_with_loss
})
system = ''
input = ''
diff --git a/xtuner/dataset/map_fns/dataset_map_fns/pretrain_map_fn.py b/xtuner/dataset/map_fns/dataset_map_fns/pretrain_map_fn.py
index b25dc2136..861302ba8 100644
--- a/xtuner/dataset/map_fns/dataset_map_fns/pretrain_map_fn.py
+++ b/xtuner/dataset/map_fns/dataset_map_fns/pretrain_map_fn.py
@@ -11,4 +11,10 @@ def pretrain_map_fn(example):
},
]
"""
- return {'conversation': [{'input': '', 'output': example['text'].strip()}]}
+ return {
+ 'conversation': [{
+ 'input': '',
+ 'output': example['text'].strip(),
+ 'need_eos_token': False
+ }]
+ }
diff --git a/xtuner/dataset/preference_dataset.py b/xtuner/dataset/preference_dataset.py
new file mode 100644
index 000000000..371ef8290
--- /dev/null
+++ b/xtuner/dataset/preference_dataset.py
@@ -0,0 +1,386 @@
+import copy
+import json
+import os
+from datetime import timedelta
+from functools import partial
+from multiprocessing import Process, Queue
+from typing import Callable, Dict, List
+
+import numpy as np
+import torch.distributed as dist
+import tqdm
+from datasets import Dataset as HFDataset
+from datasets import concatenate_datasets
+from mmengine.config import Config, ConfigDict
+from mmengine.logging import print_log
+from mmengine.utils.misc import get_object_from_string
+from torch.utils.data import Dataset
+from transformers import AutoTokenizer
+
+from xtuner.registry import BUILDER, MAP_FUNC
+from .huggingface import build_origin_dataset
+
+
+def _worker(
+ tokenize_fun: Callable,
+ data_queue: Queue,
+ out_queue: Queue,
+):
+ while True:
+ data_chunk = data_queue.get()
+
+ if data_chunk is None:
+ out_queue.put(None)
+ break
+ chunk_results = []
+ for idx, data in data_chunk:
+ chunk_results.append([idx, tokenize_fun(data)])
+ out_queue.put(chunk_results)
+
+
+def _chunk_data_to_queue(data_queue: Queue, data: List[Dict], chunk_size: int,
+ nproc):
+ data_iter = iter(data)
+ chunk_data = []
+ while True:
+ try:
+ item = next(data_iter)
+ except StopIteration:
+ break
+ chunk_data.append(item)
+ if len(chunk_data) == chunk_size:
+ data_queue.put(chunk_data)
+ chunk_data = []
+ if chunk_data:
+ data_queue.put(chunk_data)
+
+ for _ in range(nproc):
+ data_queue.put(None)
+
+
+def _multi_progress(tokenize_fun_p, dataset, nproc, task_num, chunksize,
+ description):
+ processes = []
+ data_queue = Queue()
+ output_queue = Queue()
+ bar = tqdm.tqdm(total=task_num, desc=description)
+ # task_id = bar.add_task(total=task_num, description=description)
+ dataset = enumerate(dataset)
+ _chunk_data_to_queue(data_queue, dataset, chunksize, nproc)
+ for _ in range(nproc):
+ process = Process(
+ target=_worker, args=(tokenize_fun_p, data_queue, output_queue))
+ process.start()
+ processes.append(process)
+
+ results = []
+ finished_process = 0
+ while finished_process < nproc:
+ chunk_results = output_queue.get()
+ if chunk_results is None:
+ finished_process += 1
+ continue
+ results.extend(chunk_results)
+ bar.update(len(chunk_results))
+ bar.refresh()
+ results = map(lambda x: x[1], sorted(results, key=lambda x: x[0]))
+ return results
+
+
+def load_jsonl_dataset(data_files=None, data_dir=None, suffix=None):
+ assert (data_files is not None) != (data_dir is not None)
+ if data_dir is not None:
+ data_files = os.listdir(data_dir)
+ data_files = [os.path.join(data_dir, fn) for fn in data_files]
+ if suffix is not None:
+ data_files = [fp for fp in data_files if fp.endswith(suffix)]
+ elif isinstance(data_files, str):
+ data_files = [data_files]
+
+ dataset_list = []
+ for fp in data_files:
+ with open(fp, encoding='utf-8') as file:
+ data = [json.loads(line) for line in file]
+ ds = HFDataset.from_list(data)
+ dataset_list.append(ds)
+ dataset = concatenate_datasets(dataset_list)
+ return dataset
+
+
+def tokenize(pair: str,
+ tokenizer: AutoTokenizer,
+ max_length: int,
+ is_reward: bool = False,
+ reward_token_id: int = -1):
+ prompt = tokenizer.apply_chat_template(
+ pair['prompt'], tokenize=False, add_generation_prompt=True)
+ chosen = tokenizer.apply_chat_template(
+ pair['prompt'] + pair['chosen'],
+ tokenize=False,
+ add_generation_prompt=False)
+ rejected = tokenizer.apply_chat_template(
+ pair['prompt'] + pair['rejected'],
+ tokenize=False,
+ add_generation_prompt=False)
+ prompt_ids = tokenizer.encode(prompt, add_special_tokens=False)
+ chosen_ids = tokenizer.encode(chosen, add_special_tokens=False)
+ rejected_ids = tokenizer.encode(rejected, add_special_tokens=False)
+
+ if len(chosen_ids) > max_length:
+ chosen_ids = chosen_ids[:max_length]
+ if len(rejected_ids) > max_length:
+ rejected_ids = rejected_ids[:max_length]
+
+ if is_reward:
+ # reward label
+ chosen_ids = chosen_ids + [reward_token_id]
+ rejected_ids = rejected_ids + [reward_token_id]
+ chosen_labels = [-100] * len(chosen_ids[:-1]) + [0]
+ rejected_labels = [-100] * len(rejected_ids[:-1]) + [1]
+ else:
+ # dpo label
+ prompt_len = min(len(prompt_ids), max_length)
+ chosen_labels = [-100] * prompt_len + copy.deepcopy(
+ chosen_ids[prompt_len:])
+ rejected_labels = [-100] * prompt_len + copy.deepcopy(
+ rejected_ids[prompt_len:])
+
+ return {
+ 'chosen_ids': chosen_ids,
+ 'rejected_ids': rejected_ids,
+ 'chosen_labels': chosen_labels,
+ 'rejected_labels': rejected_labels,
+ }
+
+
+class PreferenceDataset(Dataset):
+
+ def __init__(
+ self,
+ dataset: HFDataset,
+ tokenizer: AutoTokenizer,
+ max_length: int,
+ is_dpo: bool = True,
+ is_reward: bool = False,
+ reward_token_id: int = -1,
+ num_proc: int = 32,
+ ) -> None:
+ self.max_length = max_length
+ assert is_dpo != is_reward, \
+ 'Only one of is_dpo and is_reward can be True'
+ if is_reward:
+ assert reward_token_id != -1, \
+ 'reward_token_id should be set if is_reward is True'
+
+ self.is_dpo = is_dpo
+ self.is_reward = is_reward
+ self.reward_token_id = reward_token_id
+ self.tokenized_pairs = []
+
+ for tokenized_pair in _multi_progress(
+ partial(
+ tokenize,
+ tokenizer=tokenizer,
+ max_length=max_length,
+ is_reward=is_reward,
+ reward_token_id=reward_token_id),
+ dataset,
+ nproc=num_proc,
+ task_num=len(dataset),
+ chunksize=num_proc,
+ description='Tokenizing dataset'):
+ self.tokenized_pairs.append(tokenized_pair)
+
+ def __len__(self):
+ return len(self.tokenized_pairs)
+
+ def __getitem__(self, idx):
+ return self.tokenized_pairs[idx]
+
+
+class PackedDatasetWrapper(Dataset):
+
+ def __init__(self,
+ dataset,
+ max_packed_length=16384,
+ shuffle_before_pack=True) -> None:
+ super().__init__()
+ self.max_packed_length = max_packed_length
+ self.lengths = []
+ self.data = []
+
+ indices = np.arange(len(dataset))
+ if shuffle_before_pack:
+ np.random.shuffle(indices)
+
+ data_bin = []
+ bin_seq_len = 0
+ removed = 0
+ for idx in indices:
+ data = dataset[int(idx)]
+ cur_len = len(data['chosen_ids']) + len(data['rejected_ids'])
+ if cur_len > max_packed_length:
+ print_log(
+ f'sequence length {cur_len} is '
+ f'larger than max_packed_length {max_packed_length}',
+ logger='current')
+ removed += 1
+ continue
+ if (bin_seq_len +
+ cur_len) > max_packed_length and len(data_bin) > 0:
+ self.data.append(data_bin)
+ self.lengths.append(bin_seq_len)
+ data_bin = []
+ bin_seq_len = 0
+ data_bin.append(data)
+ bin_seq_len += cur_len
+
+ if len(data_bin) > 0:
+ self.data.append(data_bin)
+ self.lengths.append(bin_seq_len)
+ if removed > 0:
+ print_log(
+ f'removed {removed} samples because '
+ f'of length larger than {max_packed_length}',
+ logger='current')
+ print_log(
+ f'The batch numbers of dataset is changed '
+ f'from {len(dataset)} to {len(self)} after'
+ ' using var len attention.',
+ logger='current')
+
+ def __len__(self):
+ return len(self.data)
+
+ def __getitem__(self, index):
+ pairs = self.data[index]
+ input_ids, cu_seqlens, position_ids, labels = [], [0], [], []
+
+ for pair in pairs:
+ input_ids.extend(pair['chosen_ids'])
+ input_ids.extend(pair['rejected_ids'])
+
+ position_ids.extend(list(range(len(pair['chosen_ids']))))
+ position_ids.extend(list(range(len(pair['rejected_ids']))))
+
+ labels.extend(pair['chosen_labels'])
+ labels.extend(pair['rejected_labels'])
+
+ cu_seqlens.append(cu_seqlens[-1] + len(pair['chosen_ids']))
+ cu_seqlens.append(cu_seqlens[-1] + len(pair['rejected_ids']))
+
+ return {
+ 'input_ids': input_ids,
+ 'labels': labels,
+ 'position_ids': position_ids,
+ 'cumulative_len': cu_seqlens
+ }
+
+
+def unpack_seq(seq, cu_seqlens):
+ """Unpack a packed sequence to a list of sequences with different
+ lengths."""
+ seqlens = (cu_seqlens[1:] - cu_seqlens[:-1]).tolist()
+ subseqs = seq.split(seqlens)
+ return subseqs
+
+
+def broad_cast_dataset(dataset):
+ xtuner_dataset_timeout = timedelta(
+ minutes=int(os.getenv('XTUNER_DATASET_TIMEOUT', default=60)))
+ print_log(
+ f'xtuner_dataset_timeout = {xtuner_dataset_timeout}', logger='current')
+ using_dist = dist.is_available() and dist.is_initialized()
+ if using_dist:
+ # monitored barrier requires gloo process group to perform host-side sync. # noqa
+ group_gloo = dist.new_group(
+ backend='gloo', timeout=xtuner_dataset_timeout)
+ if not using_dist or dist.get_rank() == 0:
+ objects = [dataset]
+ else:
+ objects = [None]
+ if using_dist:
+ dist.monitored_barrier(
+ group=group_gloo, timeout=xtuner_dataset_timeout)
+ dist.broadcast_object_list(objects, src=0)
+ return objects[0]
+
+
+def map_dataset(dataset, dataset_map_fn, map_num_proc):
+ if isinstance(dataset_map_fn, str):
+ map_fn_obj = MAP_FUNC.get(dataset_map_fn) or get_object_from_string(
+ dataset_map_fn)
+ if map_fn_obj is not None:
+ dataset_map_fn = map_fn_obj
+ else:
+ raise TypeError('dataset_map_fn must be a function or a '
+ "registered function's string in MAP_FUNC, "
+ f"but got a string of '{dataset_map_fn}'")
+
+ dataset = dataset.map(dataset_map_fn, num_proc=map_num_proc)
+ return dataset
+
+
+def build_preference_dataset(
+ dataset: str,
+ tokenizer: AutoTokenizer,
+ max_length: int,
+ dataset_map_fn: Callable = None,
+ is_dpo: bool = True,
+ is_reward: bool = False,
+ reward_token_id: int = -1,
+ num_proc: int = 32,
+ use_varlen_attn: bool = False,
+ max_packed_length: int = 16384,
+ shuffle_before_pack: bool = True,
+) -> Dataset:
+ using_dist = dist.is_available() and dist.is_initialized()
+ tokenized_ds = None
+ if not using_dist or dist.get_rank() == 0:
+ if isinstance(tokenizer, dict) or isinstance(
+ tokenizer, Config) or isinstance(tokenizer, ConfigDict):
+ tokenizer = BUILDER.build(tokenizer)
+
+ dataset = build_origin_dataset(dataset, split='train')
+ if dataset_map_fn is not None:
+ dataset = map_dataset(
+ dataset, dataset_map_fn, map_num_proc=num_proc)
+
+ tokenized_ds = PreferenceDataset(
+ dataset=dataset,
+ tokenizer=tokenizer,
+ max_length=max_length,
+ is_dpo=is_dpo,
+ is_reward=is_reward,
+ reward_token_id=reward_token_id,
+ num_proc=num_proc,
+ )
+ if use_varlen_attn:
+ tokenized_ds = PackedDatasetWrapper(
+ dataset=tokenized_ds,
+ max_packed_length=max_packed_length,
+ shuffle_before_pack=shuffle_before_pack,
+ )
+ tokenized_ds = broad_cast_dataset(tokenized_ds)
+ return tokenized_ds
+
+
+def intel_orca_dpo_map_fn(example):
+ prompt = [{
+ 'role': 'system',
+ 'content': example['system']
+ }, {
+ 'role': 'user',
+ 'content': example['question']
+ }]
+ chosen = [{'role': 'assistant', 'content': example['chosen']}]
+ rejected = [{'role': 'assistant', 'content': example['rejected']}]
+ return {'prompt': prompt, 'chosen': chosen, 'rejected': rejected}
+
+
+def orpo_dpo_mix_40k_map_fn(example):
+ assert len(example['chosen']) == len(example['rejected'])
+ prompt = example['chosen'][:-1]
+ chosen = example['chosen'][-1:]
+ rejected = example['rejected'][-1:]
+ return {'prompt': prompt, 'chosen': chosen, 'rejected': rejected}
diff --git a/xtuner/dataset/samplers/length_grouped.py b/xtuner/dataset/samplers/length_grouped.py
index ad37957f2..184827837 100644
--- a/xtuner/dataset/samplers/length_grouped.py
+++ b/xtuner/dataset/samplers/length_grouped.py
@@ -4,6 +4,7 @@
import torch
from mmengine.dist import get_dist_info, sync_random_seed
+from mmengine.logging import print_log
from torch.utils.data import ConcatDataset as TorchConcatDataset
from torch.utils.data import Sampler
@@ -78,6 +79,7 @@ def __init__(self,
mega_batch_mult: Optional[int] = None,
seed: Optional[int] = None,
round_up: bool = True) -> None:
+ print_log('LengthGroupedSampler is used.', logger='current')
rank, world_size = get_dist_info()
self.rank = rank
self.world_size = world_size
@@ -120,6 +122,10 @@ def __init__(self,
assert isinstance(self.length, (list, tuple))
self.total_batch_size = total_batch_size
+ print_log(
+ f'LengthGroupedSampler construction is complete, '
+ f'and the selected attribute is {length_property}',
+ logger='current')
def __iter__(self) -> Iterator[int]:
"""Iterate the indices."""
diff --git a/xtuner/engine/_strategy/deepspeed.py b/xtuner/engine/_strategy/deepspeed.py
index 42b7f5590..665396a1b 100644
--- a/xtuner/engine/_strategy/deepspeed.py
+++ b/xtuner/engine/_strategy/deepspeed.py
@@ -6,7 +6,7 @@
from xtuner import DS_CEPH_DIR
from xtuner.parallel.sequence import init_sequence_parallel
from xtuner.utils.fileio import patch_fileio
-
+from xtuner.utils.device import get_device
class DeepSpeedStrategy(MMEngineDeepSpeedStrategy):
@@ -27,7 +27,7 @@ def _wrap_model(self, model):
# When utilizing Zero3, the model isn't allocated to CUDA within the
# `deepspeed.initialize` process.
assert hasattr(wrapper.model, 'data_preprocessor')
- wrapper.model.data_preprocessor.cuda()
+ wrapper.model.data_preprocessor.to(get_device())
return wrapper
def save_checkpoint(self, *args, **kwargs) -> None:
diff --git a/xtuner/engine/hooks/__init__.py b/xtuner/engine/hooks/__init__.py
index 667f681ec..90262425d 100644
--- a/xtuner/engine/hooks/__init__.py
+++ b/xtuner/engine/hooks/__init__.py
@@ -1,10 +1,11 @@
# Copyright (c) OpenMMLab. All rights reserved.
from .dataset_info_hook import DatasetInfoHook
from .evaluate_chat_hook import EvaluateChatHook
+from .hf_checkpoint_hook import HFCheckpointHook
from .throughput_hook import ThroughputHook
from .varlen_attn_args_to_messagehub_hook import VarlenAttnArgsToMessageHubHook
__all__ = [
'EvaluateChatHook', 'DatasetInfoHook', 'ThroughputHook',
- 'VarlenAttnArgsToMessageHubHook'
+ 'VarlenAttnArgsToMessageHubHook', 'HFCheckpointHook'
]
diff --git a/xtuner/engine/hooks/dataset_info_hook.py b/xtuner/engine/hooks/dataset_info_hook.py
index d835311dc..84dc9498a 100644
--- a/xtuner/engine/hooks/dataset_info_hook.py
+++ b/xtuner/engine/hooks/dataset_info_hook.py
@@ -9,7 +9,7 @@ def split_list(lst, value):
res = []
tmp_res = []
for i in lst:
- if tmp_res and i == value:
+ if i == value:
res.append(tmp_res)
tmp_res = []
else:
@@ -25,33 +25,36 @@ def __init__(self, tokenizer, is_intern_repo_dataset=False):
self.is_intern_repo_dataset = is_intern_repo_dataset
def log(self, runner, dataset, mode='train'):
+
+ def _log(input_ids, log_prefix=''):
+ if self.is_intern_repo_dataset:
+ input_ids = [abs(x) for x in input_ids]
+ # Try to split list to be compatible with IMAGE token
+ input_ids = split_list(input_ids, IMAGE_TOKEN_INDEX)
+ text = log_prefix
+ for idx, ids in enumerate(input_ids):
+ text += self.tokenizer.decode(ids)
+ if idx != len(input_ids) - 1:
+ text += DEFAULT_IMAGE_TOKEN
+ runner.logger.info(text)
+
runner.logger.info(f'Num {mode} samples {len(dataset)}')
runner.logger.info(f'{mode} example:')
- input_ids = dataset[0]['input_ids']
- if self.is_intern_repo_dataset:
- input_ids = [abs(x) for x in input_ids]
- # Try to split list to be compatible with IMAGE token
- input_ids = split_list(input_ids, IMAGE_TOKEN_INDEX)
- text = ''
- for idx, ids in enumerate(input_ids):
- text += self.tokenizer.decode(ids)
- if idx != len(input_ids) - 1:
- text += DEFAULT_IMAGE_TOKEN
- runner.logger.info(text)
+ if 'chosen_ids' in dataset[0]:
+ _log(dataset[0]['chosen_ids'], log_prefix='chosen: ')
+ _log(dataset[0]['rejected_ids'], log_prefix='rejected: ')
+ else:
+ _log(dataset[0]['input_ids'])
def before_train(self, runner) -> None:
do_train = runner.train_loop is not None
do_eval = runner.val_loop is not None
- do_test = runner.test_loop is not None
if do_train:
train_dataset = runner.train_dataloader.dataset
self.log(runner, train_dataset, mode='train')
if do_eval:
eval_dataset = runner.val_dataloader.dataset
self.log(runner, eval_dataset, mode='eval')
- if do_test:
- test_dataset = runner.test_dataloader.dataset
- self.log(runner, test_dataset, mode='test')
def before_val(self, runner) -> None:
eval_dataset = runner.val_dataloader.dataset
diff --git a/xtuner/engine/hooks/evaluate_chat_hook.py b/xtuner/engine/hooks/evaluate_chat_hook.py
index 8e6a86822..05d508e4c 100644
--- a/xtuner/engine/hooks/evaluate_chat_hook.py
+++ b/xtuner/engine/hooks/evaluate_chat_hook.py
@@ -3,8 +3,10 @@
import warnings
import torch
+from mmengine.dist import master_only
from mmengine.hooks import Hook
from mmengine.model import is_model_wrapper
+from mmengine.utils import mkdir_or_exist
from mmengine.utils.misc import get_object_from_string
from transformers import GenerationConfig, StoppingCriteriaList
@@ -90,9 +92,13 @@ def __init__(self,
self.stop_criteria.append(
StopWordStoppingCriteria(self.tokenizer, word))
+ self.is_first_run = True
+
+ @master_only
def _save_eval_output(self, runner, eval_outputs):
save_path = os.path.join(runner.log_dir, 'vis_data',
f'eval_outputs_iter_{runner.iter}.txt')
+ mkdir_or_exist(os.path.dirname(save_path))
with open(save_path, 'w', encoding='utf-8') as f:
for i, output in enumerate(eval_outputs):
f.write(f'Eval output {i + 1}:\n{output}\n\n')
@@ -196,6 +202,13 @@ def _generate_samples(self,
model = model.module
device = next(iter(model.parameters())).device
+
+ if self.is_first_run:
+ # hardcode for qlora DeepSpeed ZeRO3, put buffers and QuantState to
+ # device
+ model.to(device)
+ self.is_first_run = False
+
is_checkpointing = model.llm.is_gradient_checkpointing
use_cache = model.llm.config.use_cache
diff --git a/xtuner/engine/hooks/hf_checkpoint_hook.py b/xtuner/engine/hooks/hf_checkpoint_hook.py
new file mode 100644
index 000000000..142af4cdb
--- /dev/null
+++ b/xtuner/engine/hooks/hf_checkpoint_hook.py
@@ -0,0 +1,73 @@
+# Copyright (c) OpenMMLab. All rights reserved.
+import os.path as osp
+from pathlib import Path
+from typing import Optional, Union
+
+import torch.distributed as dist
+from mmengine import print_log
+from mmengine._strategy import DeepSpeedStrategy
+from mmengine.hooks import Hook
+from mmengine.model import is_model_wrapper
+from mmengine.runner import FlexibleRunner
+
+from xtuner.registry import BUILDER
+from xtuner.utils import get_origin_state_dict
+
+DATA_BATCH = Optional[Union[dict, tuple, list]]
+
+
+class HFCheckpointHook(Hook):
+
+ priority = 95 # lower than CheckpointHook in MMEngine
+
+ def __init__(self, out_dir: Optional[Union[str, Path]] = None) -> None:
+ self.out_dir = out_dir
+
+ @staticmethod
+ def _use_shard_moe(llm):
+ config = llm.config
+ moe_implementation = getattr(config, 'moe_implementation', 'origin')
+ return moe_implementation == 'shard'
+
+ def after_run(self, runner) -> None:
+ assert isinstance(runner,
+ FlexibleRunner), 'Runner should be `FlexibleRunner`'
+ assert isinstance(
+ runner.strategy,
+ DeepSpeedStrategy), 'Strategy should be `DeepSpeedStrategy`'
+
+ if self.out_dir is None:
+ self.out_dir = osp.join(runner.work_dir, 'hf_model')
+
+ wrapped_model = runner.strategy.model
+ if wrapped_model.zero_optimization_partition_weights():
+ assert wrapped_model.zero_gather_16bit_weights_on_model_save(), \
+ ('Please set `gather_16bit_weights_on_model_save=True` '
+ 'in your DeepSpeed config.')
+ state_dict = wrapped_model._zero3_consolidated_16bit_state_dict()
+ else:
+ state_dict = wrapped_model.module_state_dict(
+ exclude_frozen_parameters=runner.strategy.
+ exclude_frozen_parameters)
+
+ model = runner.model
+ if is_model_wrapper(model):
+ model = model.module
+ llm = model.llm
+ if (not dist.is_initialized()) or dist.get_rank() == 0:
+ # keys in state_dict are prefixed with 'llm.'
+ keys = list(state_dict.keys())
+ for k in keys:
+ val = state_dict.pop(k)
+ state_dict[k[4:]] = val
+
+ if self._use_shard_moe(llm):
+ print_log('recover the origin state_dict from merged one ...')
+ state_dict = get_origin_state_dict(state_dict, llm)
+
+ print_log(f'Saving LLM to {self.out_dir}')
+ llm.save_pretrained(self.out_dir, state_dict=state_dict)
+
+ print_log(f'Saving LLM tokenizer to {self.out_dir}')
+ tokenizer = BUILDER.build(runner.cfg.tokenizer)
+ tokenizer.save_pretrained(self.out_dir)
diff --git a/xtuner/engine/hooks/throughput_hook.py b/xtuner/engine/hooks/throughput_hook.py
index a07e216fe..e74c0a0ac 100644
--- a/xtuner/engine/hooks/throughput_hook.py
+++ b/xtuner/engine/hooks/throughput_hook.py
@@ -96,6 +96,7 @@ def after_train_iter(self,
batch_size, sequence_len = self._get_batch_size_and_sequence_len(
data_batch)
sequence_parallel_size = get_sequence_parallel_world_size()
+ sequence_len /= sequence_parallel_size
message_hub = runner.message_hub
iter_time = message_hub.get_scalar('train/time').current()
diff --git a/xtuner/engine/hooks/varlen_attn_args_to_messagehub_hook.py b/xtuner/engine/hooks/varlen_attn_args_to_messagehub_hook.py
index f7a95a09c..e4fd1ec76 100644
--- a/xtuner/engine/hooks/varlen_attn_args_to_messagehub_hook.py
+++ b/xtuner/engine/hooks/varlen_attn_args_to_messagehub_hook.py
@@ -1,10 +1,12 @@
# Copyright (c) OpenMMLab. All rights reserved.
from typing import Optional, Union
-import torch.distributed as dist
from mmengine import MessageHub
+from mmengine.dist import get_rank
from mmengine.hooks import Hook
+from xtuner.utils.device import get_device
+
DATA_BATCH = Optional[Union[dict, tuple, list]]
@@ -14,7 +16,7 @@ def before_train_iter(self,
runner,
batch_idx: int,
data_batch: dict = None) -> None:
- rank = dist.get_rank()
+ rank = get_rank()
message_hub = MessageHub.get_instance('varlen_attn_args')
assert 'data' in data_batch.keys()
@@ -22,7 +24,7 @@ def before_train_iter(self,
cumulative_len = data.pop('cumulative_len')
assert len(cumulative_len) == 1
- cumulative_len = cumulative_len[0].cuda()
+ cumulative_len = cumulative_len[0].to(get_device())
message_hub.update_info(f'cumulative_len_rank_{rank}', cumulative_len)
max_seqlen = data.pop('max_seqlen')
@@ -33,7 +35,53 @@ def after_train_iter(self,
batch_idx: int,
data_batch: DATA_BATCH = None,
outputs: Optional[dict] = None) -> None:
- rank = dist.get_rank()
+ rank = get_rank()
+ message_hub = MessageHub.get_instance('varlen_attn_args')
+ message_hub.update_info(f'cumulative_len_rank_{rank}', None)
+ message_hub.update_info(f'max_seqlen_rank_{rank}', None)
+
+ def before_val_iter(self,
+ runner,
+ batch_idx: int,
+ data_batch: DATA_BATCH = None) -> None:
+ """All subclasses should override this method, if they need any
+ operations before each validation iteration.
+
+ Args:
+ runner (Runner): The runner of the validation process.
+ batch_idx (int): The index of the current batch in the val loop.
+ data_batch (dict, optional): Data from dataloader.
+ Defaults to None.
+ """
+ rank = get_rank()
+ message_hub = MessageHub.get_instance('varlen_attn_args')
+
+ assert 'data' in data_batch.keys()
+ data = data_batch['data']
+
+ cumulative_len = data.pop('cumulative_len')
+ assert len(cumulative_len) == 1
+ cumulative_len = cumulative_len[0].to(get_device())
+ message_hub.update_info(f'cumulative_len_rank_{rank}', cumulative_len)
+
+ max_seqlen = data.pop('max_seqlen')
+ message_hub.update_info(f'max_seqlen_rank_{rank}', max_seqlen)
+
+ def after_val_iter(self,
+ runner,
+ batch_idx,
+ data_batch=None,
+ outputs=None) -> None:
+ """All subclasses should override this method, if they need any
+ operations after each validation iteration.
+
+ Args:
+ runner (Runner): The runner of the validation process.
+ batch_idx (int): The index of the current batch in the val loop.
+ data_batch (dict or tuple or list, optional): Data from dataloader.
+ outputs (Sequence, optional): Outputs from model.
+ """
+ rank = get_rank()
message_hub = MessageHub.get_instance('varlen_attn_args')
message_hub.update_info(f'cumulative_len_rank_{rank}', None)
message_hub.update_info(f'max_seqlen_rank_{rank}', None)
diff --git a/xtuner/entry_point.py b/xtuner/entry_point.py
index a185da9d2..2af774fd3 100644
--- a/xtuner/entry_point.py
+++ b/xtuner/entry_point.py
@@ -265,9 +265,15 @@ def cli():
if fn in HELP_FUNCS:
fn()
else:
- nnodes = os.environ.get('NNODES', 1)
- nproc_per_node = os.environ.get('NPROC_PER_NODE', 1)
- if nnodes == 1 and nproc_per_node == 1:
+ slurm_launcher = False
+ for i in range(n_arg + 1, len(args)):
+ if args[i] == '--launcher':
+ if i + 1 < len(args) and args[i + 1] == 'slurm':
+ slurm_launcher = True
+ break
+ nnodes = int(os.environ.get('NNODES', 1))
+ nproc_per_node = int(os.environ.get('NPROC_PER_NODE', 1))
+ if slurm_launcher or (nnodes == 1 and nproc_per_node == 1):
subprocess.run(['python', fn()] + args[n_arg + 1:])
else:
port = os.environ.get('PORT', None)
diff --git a/xtuner/evaluation/metrics/reward_metric.py b/xtuner/evaluation/metrics/reward_metric.py
new file mode 100644
index 000000000..c5d019978
--- /dev/null
+++ b/xtuner/evaluation/metrics/reward_metric.py
@@ -0,0 +1,102 @@
+import itertools
+from collections import defaultdict
+from typing import List, Optional, Sequence
+
+import torch
+from mmengine.evaluator import BaseMetric
+from mmengine.logging import print_log
+from rich.console import Console
+from rich.table import Table
+
+
+class RewardMetric(BaseMetric):
+ r"""Reward model evaluation metric.
+ """
+ default_prefix: Optional[str] = ''
+
+ def __init__(self,
+ collect_device: str = 'cpu',
+ prefix: Optional[str] = None) -> None:
+ super().__init__(collect_device=collect_device, prefix=prefix)
+
+ def process(self, data_batch, data_samples: Sequence[dict]):
+ """Process one batch of data samples.
+
+ The processed results should be stored in ``self.results``, which will
+ be used to computed the metrics when all batches have been processed.
+
+ Args:
+ data_batch: A batch of data from the dataloader.
+ data_samples (Sequence[dict]): A batch of outputs from the model.
+ """
+ logits = torch.cat(
+ [sample['logits'].unsqueeze(0) for sample in data_samples], dim=0)
+ labels = data_batch['data']['labels']
+ ds_names = data_batch['data_samples']['ds_names']
+ chosen_idx = torch.where(labels == 0)
+ rejected_idx = torch.where(labels == 1)
+ chosen_logits = logits[chosen_idx].cpu()
+ rejected_logits = logits[rejected_idx].cpu()
+
+ correct = (chosen_logits > rejected_logits).cpu()
+ self.results.append({
+ 'chosen_logits': chosen_logits,
+ 'rejected_logits': rejected_logits,
+ 'correct': correct,
+ 'ds_names': ds_names
+ })
+
+ def compute_metrics(self, results: List):
+ """Compute the metrics from processed results.
+
+ Args:
+ results (dict): The processed results of each batch.
+
+ Returns:
+ Dict: The computed metrics. The keys are the names of the metrics,
+ and the values are corresponding results.
+ """
+ # NOTICE: don't access `self.results` from the method.
+ metrics = {}
+
+ correct = torch.cat([res['correct'] for res in results])
+ chosen_logits = torch.cat([res['chosen_logits'] for res in results])
+ rejected_logits = torch.cat(
+ [res['rejected_logits'] for res in results])
+ ds_names = list(itertools.chain(*[res['ds_names'] for res in results]))
+
+ # group by ds_names
+ grouped_correct = defaultdict(list)
+ grouped_chosen_logits = defaultdict(list)
+ grouped_rejected_logits = defaultdict(list)
+ for i, ds_name in enumerate(ds_names):
+ grouped_correct[ds_name].append(correct[i])
+ grouped_chosen_logits[ds_name].append(chosen_logits[i])
+ grouped_rejected_logits[ds_name].append(rejected_logits[i])
+
+ # print metrics in a rich table
+ table = Table(title='Reward Metrics')
+ table.add_column('Dataset Name')
+ table.add_column('Accuracy')
+ table.add_column('Chosen Score')
+ table.add_column('Rejected Score')
+
+ for ds_name in grouped_correct.keys():
+ correct = torch.stack(grouped_correct[ds_name])
+ chosen_logits = torch.stack(grouped_chosen_logits[ds_name])
+ rejected_logits = torch.stack(grouped_rejected_logits[ds_name])
+
+ acc = correct.float().mean()
+ metrics[f'accuracy/{ds_name}'] = acc.item()
+ metrics[f'chosen_score/{ds_name}'] = chosen_logits.mean().item()
+ metrics[f'rejected_score{ds_name}'] = rejected_logits.mean().item()
+
+ table.add_row(ds_name, f'{acc:.4f}', f'{chosen_logits.mean():.4f}',
+ f'{rejected_logits.mean():.4f}')
+
+ console = Console()
+ with console.capture() as capture:
+ console.print(table, end='')
+ print_log('\n' + capture.get(), 'current')
+
+ return metrics
diff --git a/xtuner/model/__init__.py b/xtuner/model/__init__.py
index 39547b2d7..1b3a501d4 100644
--- a/xtuner/model/__init__.py
+++ b/xtuner/model/__init__.py
@@ -1,5 +1,6 @@
# Copyright (c) OpenMMLab. All rights reserved.
+from .internvl import InternVL_V1_5
from .llava import LLaVAModel
from .sft import SupervisedFinetune
-__all__ = ['SupervisedFinetune', 'LLaVAModel']
+__all__ = ['SupervisedFinetune', 'LLaVAModel', 'InternVL_V1_5']
diff --git a/xtuner/model/dpo.py b/xtuner/model/dpo.py
new file mode 100644
index 000000000..faaa43402
--- /dev/null
+++ b/xtuner/model/dpo.py
@@ -0,0 +1,286 @@
+# DPO Authors: Rafael Rafailov, Archit Sharma, Eric Mitchell, Stefano Ermon, Christopher D. Manning, and Chelsea Finn 2023 # noqa
+# Copyright 2023 The HuggingFace Team. All rights reserved.
+# Copyright (c) OpenMMLab. All rights reserved.
+from copy import deepcopy
+
+import torch
+import torch.distributed as dist
+import torch.nn.functional as F
+from mmengine import MessageHub
+from transformers.integrations import is_deepspeed_zero3_enabled
+
+from xtuner.parallel.sequence import (gather_forward_split_backward,
+ get_sequence_parallel_group,
+ get_sequence_parallel_world_size,
+ split_for_sequence_parallel)
+from .sft import SupervisedFinetune
+
+
+def disable_grad(model):
+ # freeze parameters
+ parameter_names = [n for n, _ in model.named_parameters()]
+ for param_name in parameter_names:
+ param = model.get_parameter(param_name)
+ param.requires_grad = False
+ return model.eval()
+
+
+def create_reference_model(model):
+ if is_deepspeed_zero3_enabled():
+ raise ValueError('DeepSpeed ZeRO-3 is enabled and is not compatible '
+ 'with `create_reference_model()`. Please instantiate '
+ 'your reference model directly with '
+ '`AutoCausalLM.from_pretrained()`.')
+ ref_model = deepcopy(model)
+ ref_model = disable_grad(ref_model)
+ return ref_model
+
+
+class DPO(SupervisedFinetune):
+ """A general class of DPO and its variants."""
+
+ def __init__(self,
+ llm,
+ ref_llm=None,
+ beta=0.1,
+ loss_type='sigmoid',
+ label_smoothing=0.0,
+ **kwargs):
+ super().__init__(llm, **kwargs)
+ self.loss_type = loss_type
+ self.label_smoothing = label_smoothing
+ self.beta = beta
+
+ if ref_llm is not None:
+ ref_llm = self.build_llm_from_cfg(
+ ref_llm, kwargs.get('use_varlen_attn', False),
+ kwargs.get('max_position_embeddings', None))
+ self.ref_llm = disable_grad(ref_llm)
+ else:
+ self.ref_llm = None if self.use_lora else create_reference_model(
+ self.llm)
+
+ def _gather_masked_logits(self, logits, labels, mask):
+ logits = torch.gather(
+ logits.log_softmax(-1), dim=2,
+ index=labels.unsqueeze(2)).squeeze(2)
+ return logits * mask
+
+ def get_logps(
+ self,
+ policy_logps, # bs, seqlen,vocab_size
+ ref_logps, # bs, seqlen,vocab_size
+ loss_mask, # bs, seqlen
+ ):
+ policy_logps = policy_logps[:, :-1].sum(-1)
+ ref_logps = ref_logps[:, :-1].sum(-1)
+ loss_mask = loss_mask[:, :-1]
+
+ if self.loss_type == 'ipo': # average_log_prob
+ policy_logps = policy_logps / loss_mask.sum(-1)
+ ref_logps = ref_logps / loss_mask.sum(-1)
+
+ policy_chosen_logps = policy_logps[::2]
+ policy_rejected_logps = policy_logps[1::2]
+ reference_chosen_logps = ref_logps[::2]
+ reference_rejected_logps = ref_logps[1::2]
+ return (policy_chosen_logps, policy_rejected_logps,
+ reference_chosen_logps, reference_rejected_logps)
+
+ def get_var_len_atten_logps(self, policy_logps, ref_logps, loss_mask,
+ cu_seqlens, attention_mask):
+ seqlens = (cu_seqlens[1:] - cu_seqlens[:-1]).tolist()
+ # unpack sequence
+ unpacked_policy_logps = torch.split(policy_logps, seqlens, dim=1)
+ unpacked_ref_logps = torch.split(ref_logps, seqlens, dim=1)
+ unpacked_loss_mask = torch.split(loss_mask, seqlens, dim=1)
+ if attention_mask is not None:
+ # It indicate that we pad the original sequence, labels,
+ # position_ids and cumulative_len for sequence parallel if the
+ # attention_mask is not None.
+ # We then need to remove the padded segments.
+ assert False in attention_mask
+ unpacked_policy_logps = unpacked_policy_logps[:-1]
+ unpacked_ref_logps = unpacked_ref_logps[:-1]
+ unpacked_loss_mask = unpacked_loss_mask[:-1]
+ assert len(unpacked_policy_logps) % 2 == 0
+
+ def compute_logps(_logps, _mask):
+ _logps = _logps[:, :-1].sum(-1)
+ _mask = _mask[:, :-1]
+ if self.loss_type == 'ipo':
+ _logps /= _mask.sum(-1)
+ return _logps
+
+ (policy_chosen_logps, policy_rejected_logps, reference_chosen_logps,
+ reference_rejected_logps) = [], [], [], []
+ for i in range(len(unpacked_policy_logps) // 2):
+ chosen = unpacked_policy_logps[2 * i]
+ rejected = unpacked_policy_logps[2 * i + 1]
+ chosen_ref = unpacked_ref_logps[2 * i]
+ rejected_ref = unpacked_ref_logps[2 * i + 1]
+ chosen_mask = unpacked_loss_mask[2 * i]
+ rejected_mask = unpacked_loss_mask[2 * i + 1]
+ policy_chosen_logps.append(compute_logps(chosen, chosen_mask))
+ policy_rejected_logps.append(
+ compute_logps(rejected, rejected_mask))
+ reference_chosen_logps.append(
+ compute_logps(chosen_ref, chosen_mask))
+ reference_rejected_logps.append(
+ compute_logps(rejected_ref, rejected_mask))
+
+ return (torch.stack(policy_chosen_logps),
+ torch.stack(policy_rejected_logps),
+ torch.stack(reference_chosen_logps),
+ torch.stack(reference_rejected_logps))
+
+ @staticmethod
+ def _split_for_sequence_parallel(data):
+ # attention mask should not be split
+ ARGS_NEED_TO_SPLIT = ('input_ids', 'position_ids', 'labels')
+ sp_group = get_sequence_parallel_group()
+ for key in ARGS_NEED_TO_SPLIT:
+ val = data.get(key, None)
+ if val is not None:
+ # `dim` is 1 as the shape of tensor is (bs, seq_len, ...)
+ data[key] = split_for_sequence_parallel(
+ val, dim=1, sp_group=sp_group)
+ return data
+
+ def compute_loss(self, data, data_samples=None):
+ # modified from https://github.com/huggingface/trl/blob/main/trl/trainer/dpo_trainer.py # noqa
+ # shift labels first and add a dummy label at the end, to support sequence parallel # noqa
+ data['labels'] = torch.cat(
+ (data['labels'][:, 1:], torch.zeros_like(data['labels'][:, :1])),
+ dim=1)
+ tmp_label = data['labels'].clone()
+ tmp_label[tmp_label == 0] = -100
+ all_loss_mask = data[
+ 'labels'] != -100 # loss mask of all tokens in all sp ranks # noqa
+
+ if get_sequence_parallel_world_size() > 1:
+ data = self._split_for_sequence_parallel(data)
+
+ all_logits = self.llm(**data).logits
+ with torch.no_grad():
+ if self.ref_llm is None:
+ with self.llm.disable_adapter():
+ all_ref_logits = self.llm(**data).logits
+ else:
+ all_ref_logits = self.ref_llm(**data).logits
+
+ labels = data['labels']
+ labels[labels == -100] = 0
+ loss_mask = labels != 0 # loss mask in a single sp rank
+ policy_logps = self._gather_masked_logits(all_logits, labels,
+ loss_mask)
+ ref_logps = self._gather_masked_logits(all_ref_logits, labels,
+ loss_mask)
+
+ if get_sequence_parallel_world_size() > 1:
+ policy_logps = gather_forward_split_backward(
+ policy_logps,
+ dim=1,
+ sp_group=get_sequence_parallel_group(),
+ grad_scale='up')
+ ref_logps = gather_forward_split_backward(
+ ref_logps,
+ dim=1,
+ sp_group=get_sequence_parallel_group(),
+ grad_scale='up')
+
+ if not self.use_varlen_attn:
+ (policy_chosen_logps, policy_rejected_logps,
+ reference_chosen_logps,
+ reference_rejected_logps) = self.get_logps(
+ policy_logps, ref_logps, all_loss_mask)
+ else:
+ message_hub = MessageHub.get_instance('varlen_attn_args')
+ rank = dist.get_rank()
+ cu_seqlens = message_hub.get_info(f'cumulative_len_rank_{rank}')
+ (policy_chosen_logps, policy_rejected_logps,
+ reference_chosen_logps,
+ reference_rejected_logps) = self.get_var_len_atten_logps(
+ policy_logps, ref_logps, all_loss_mask, cu_seqlens,
+ data['attention_mask'])
+
+ pi_logratios = policy_chosen_logps - policy_rejected_logps
+ ref_logratios = reference_chosen_logps - reference_rejected_logps
+
+ logits = pi_logratios - ref_logratios
+ if self.loss_type == 'sigmoid':
+ loss = (-F.logsigmoid(self.beta * logits) *
+ (1 - self.label_smoothing) -
+ F.logsigmoid(-self.beta * logits) * self.label_smoothing)
+ elif self.loss_type == 'robust':
+ loss = (-F.logsigmoid(self.beta * logits) *
+ (1 - self.label_smoothing) +
+ F.logsigmoid(-self.beta * logits) *
+ self.label_smoothing) / (1 - 2 * self.label_smoothing)
+ elif self.loss_type == 'hinge':
+ loss = torch.relu(1 - self.beta * logits)
+ elif self.loss_type == 'ipo':
+ # eqn (17) of the paper where beta is the regularization
+ # parameter for the IPO loss, denoted by tau in the paper. # noqa
+ loss = (logits - 1 / (2 * self.beta))**2
+ elif self.loss_type == 'kto_pair':
+ # eqn (7) of the HALOs paper
+ chosen_KL = (policy_chosen_logps -
+ reference_chosen_logps).mean().clamp(min=0)
+ rejected_KL = (policy_rejected_logps -
+ reference_rejected_logps).mean().clamp(min=0)
+
+ chosen_logratios = policy_chosen_logps - reference_chosen_logps
+ rejected_logratios = \
+ policy_rejected_logps - reference_rejected_logps
+ # As described in the KTO report, the KL term for chosen (rejected)
+ # is estimated using the rejected (chosen) half. # noqa
+ loss = torch.cat(
+ (
+ 1 - F.sigmoid(self.beta *
+ (chosen_logratios - rejected_KL)),
+ 1 - F.sigmoid(self.beta *
+ (chosen_KL - rejected_logratios)),
+ ),
+ 0,
+ )
+ elif self.loss_type == 'sppo_hard':
+ # In the paper (https://arxiv.org/pdf/2405.00675),
+ # SPPO employs a soft probability approach,
+ # estimated using the PairRM score. The probability calculation
+ # is conducted outside of the trainer class.
+ # The version described here is the hard probability version,
+ # where P in Equation (4.7) of Algorithm 1 is set to 1 for
+ # the winner and 0 for the loser.
+ a = policy_chosen_logps - reference_chosen_logps
+ b = policy_rejected_logps - reference_rejected_logps
+
+ loss = (a - 0.5 / self.beta)**2 + (b + 0.5 / self.beta)**2
+ elif self.loss_type == 'nca_pair':
+ chosen_rewards = (policy_chosen_logps -
+ reference_chosen_logps) * self.beta
+ rejected_rewards = (policy_rejected_logps -
+ reference_rejected_logps) * self.beta
+ loss = (-F.logsigmoid(chosen_rewards) -
+ 0.5 * F.logsigmoid(-chosen_rewards) -
+ 0.5 * F.logsigmoid(-rejected_rewards))
+ else:
+ raise ValueError(
+ f'Unknown loss type: {self.loss_type}. Should be one of '
+ "['sigmoid', 'hinge', 'ipo', 'kto_pair', "
+ "'sppo_hard', 'nca_pair', 'robust']")
+ # for logging
+ chosen_rewards = self.beta * (
+ policy_chosen_logps - reference_chosen_logps)
+ rejected_rewards = self.beta * (
+ policy_rejected_logps - reference_rejected_logps)
+ reward_acc = (chosen_rewards > rejected_rewards).float().mean()
+
+ loss_dict = {
+ 'loss': loss,
+ 'chosen_rewards': chosen_rewards.mean(),
+ 'rejected_rewards': rejected_rewards.mean(),
+ 'reward_acc': reward_acc,
+ 'reward_margin': (chosen_rewards - rejected_rewards).mean(),
+ }
+ return loss_dict
diff --git a/xtuner/model/internvl.py b/xtuner/model/internvl.py
new file mode 100644
index 000000000..0358266a9
--- /dev/null
+++ b/xtuner/model/internvl.py
@@ -0,0 +1,320 @@
+# Copyright (c) OpenMMLab. All rights reserved.
+from collections import OrderedDict
+from typing import List, Optional, Tuple, Union
+
+import torch
+from mmengine import print_log
+from mmengine.config import Config, ConfigDict
+from mmengine.model import BaseModel
+from peft import get_peft_model, prepare_model_for_kbit_training
+from torch.nn import CrossEntropyLoss
+from transformers import (AutoConfig, AutoModel, AutoTokenizer,
+ BitsAndBytesConfig)
+from transformers.modeling_outputs import CausalLMOutputWithPast
+
+from xtuner.registry import BUILDER
+from .utils import (find_all_linear_names, get_peft_model_state_dict,
+ guess_load_checkpoint, make_inputs_require_grad)
+
+
+class InternVL_V1_5(BaseModel):
+
+ def __init__(self,
+ model_path,
+ freeze_llm=False,
+ freeze_visual_encoder=False,
+ llm_lora=None,
+ visual_encoder_lora=None,
+ quantization_vit=False,
+ quantization_llm=False,
+ pretrained_pth=None):
+ print_log('Start to load InternVL_V1_5 model.', logger='current')
+ super().__init__()
+ self.freeze_llm = freeze_llm
+ self.freeze_visual_encoder = freeze_visual_encoder
+ self.use_llm_lora = llm_lora is not None
+ self.use_visual_encoder_lora = visual_encoder_lora is not None
+ self.quantization_vit = quantization_vit
+ self.quantization_llm = quantization_llm
+ if quantization_vit:
+ assert visual_encoder_lora is not None
+ if quantization_llm:
+ assert quantization_llm and llm_lora is not None
+
+ config = AutoConfig.from_pretrained(model_path, trust_remote_code=True)
+ if config.llm_config.model_type == 'internlm2':
+ config.llm_config.attn_implementation = 'flash_attention_2'
+ else:
+ config.llm_config._attn_implementation = 'flash_attention_2'
+
+ if quantization_vit is False and quantization_llm is False:
+ quantization = None
+ else:
+ llm_int8_skip_modules = ['mlp1']
+ if quantization_llm and not quantization_vit:
+ llm_int8_skip_modules.append('vision_model')
+
+ if quantization_vit and not quantization_llm:
+ llm_int8_skip_modules.append('language_model')
+
+ quantization_config = dict(
+ type=BitsAndBytesConfig,
+ llm_int8_skip_modules=llm_int8_skip_modules,
+ load_in_4bit=True,
+ load_in_8bit=False,
+ llm_int8_threshold=6.0,
+ llm_int8_has_fp16_weight=False,
+ bnb_4bit_compute_dtype=torch.float16,
+ bnb_4bit_use_double_quant=True,
+ bnb_4bit_quant_type='nf4')
+ quantization_clazz = quantization_config.pop('type')
+ quantization = quantization_clazz(**quantization_config)
+
+ self.model = AutoModel.from_pretrained(
+ model_path,
+ torch_dtype=torch.bfloat16,
+ quantization_config=quantization,
+ config=config,
+ trust_remote_code=True)
+
+ tokenizer = AutoTokenizer.from_pretrained(
+ model_path, trust_remote_code=True)
+ img_context_token_id = tokenizer.convert_tokens_to_ids('')
+ self.model.img_context_token_id = img_context_token_id
+
+ if self.freeze_llm:
+ self.model.language_model.requires_grad_(False)
+ if self.freeze_visual_encoder:
+ self.model.vision_model.requires_grad_(False)
+
+ if hasattr(self.model.language_model, 'enable_input_require_grads'):
+ self.model.language_model.enable_input_require_grads()
+ else:
+ self.model.language_model.get_input_embeddings(
+ ).register_forward_hook(make_inputs_require_grad)
+
+ self.gradient_checkpointing_enable()
+
+ if self.use_llm_lora:
+ self._prepare_llm_for_lora(llm_lora)
+
+ if self.use_visual_encoder_lora:
+ self._prepare_visual_encoder_for_lora(visual_encoder_lora)
+
+ if pretrained_pth is not None:
+ pretrained_state_dict = guess_load_checkpoint(pretrained_pth)
+
+ self.load_state_dict(pretrained_state_dict, strict=False)
+ print(f'Load pretrained weight from {pretrained_pth}')
+
+ self._count = 0
+ print_log(self, logger='current')
+ print_log('InternVL_V1_5 construction is complete', logger='current')
+
+ def _parse_lora_config(self, lora_config):
+ if isinstance(lora_config, dict) or isinstance(
+ lora_config, Config) or isinstance(lora_config, ConfigDict):
+ lora_config = BUILDER.build(lora_config)
+ return lora_config
+
+ def _prepare_llm_for_lora(self,
+ lora_config,
+ use_activation_checkpointing=True):
+ lora_config = self._parse_lora_config(lora_config)
+ self.model.language_model = prepare_model_for_kbit_training(
+ self.model.language_model, use_activation_checkpointing)
+ if lora_config.target_modules is None:
+ modules = find_all_linear_names(self.model.language_model)
+ lora_config.target_modules = modules
+ self.model.language_model = get_peft_model(self.model.language_model,
+ lora_config)
+
+ def _prepare_visual_encoder_for_lora(self, lora_config):
+ lora_config = self._parse_lora_config(lora_config)
+ if lora_config.target_modules is None:
+ modules = find_all_linear_names(self.model.vision_model)
+ lora_config.target_modules = modules
+ self.model.vision_model = get_peft_model(self.model.vision_model,
+ lora_config)
+
+ def gradient_checkpointing_enable(self):
+ self.activation_checkpointing_enable()
+
+ def activation_checkpointing_enable(self):
+ self.model.language_model.gradient_checkpointing_enable()
+
+ def gradient_checkpointing_disable(self):
+ self.activation_checkpointing_disable()
+
+ def activation_checkpointing_disable(self):
+ self.model.language_model.gradient_checkpointing_disable()
+
+ def state_dict(self, *args, **kwargs):
+ state_dict = super().state_dict(*args, **kwargs)
+ to_return = OrderedDict()
+ # Step 1. visual_encoder
+ if self.use_visual_encoder_lora:
+ to_return.update(
+ get_peft_model_state_dict(
+ self.model.vision_model, state_dict=state_dict))
+ elif not self.freeze_visual_encoder:
+ to_return.update({
+ k: v
+ for k, v in state_dict.items() if 'model.vision_model.' in k
+ })
+ # Step 2. LLM
+ if self.use_llm_lora:
+ to_return.update(
+ get_peft_model_state_dict(
+ self.model.language_model, state_dict=state_dict))
+ elif not self.freeze_llm:
+ to_return.update({
+ k: v
+ for k, v in state_dict.items() if 'model.language_model.' in k
+ })
+ # Step 3. Projector
+ to_return.update(
+ {k: v
+ for k, v in state_dict.items() if 'model.mlp1.' in k})
+ return to_return
+
+ def init_weights(self):
+ pass
+
+ def forward(self, data, data_samples=None, mode='loss'):
+ pixel_values = data['pixel_values']
+
+ if type(pixel_values) is list or pixel_values.ndim == 5:
+ if type(pixel_values) is list:
+ pixel_values = [
+ x.unsqueeze(0) if x.ndim == 3 else x for x in pixel_values
+ ]
+ # b*n, c, h, w
+ concat_images = torch.cat([
+ image.to(self.model.vision_model.dtype)
+ for image in pixel_values
+ ],
+ dim=0)
+ else:
+ raise NotImplementedError()
+
+ input_ids = data['input_ids']
+ position_ids = data['position_ids']
+ attention_mask = data['attention_mask']
+ # sum is 0 are text
+ image_flags = torch.sum(concat_images, dim=(1, 2, 3)) != 0
+ image_flags = image_flags.long()
+
+ labels = data['labels']
+ use_cache = False
+
+ # Directly calling this code in LORA fine-tuning
+ # will result in an error,so we must rewrite it.
+ # TODO: Once the official is fixed, we can remove it.
+ # outputs = self.model(input_ids=input_ids,
+ # position_ids=position_ids,
+ # attention_mask=attention_mask,
+ # image_flags=image_flags,
+ # pixel_values=concat_images,
+ # labels=labels,
+ # use_cache=use_cache)
+ outputs = self._llm_forward(
+ input_ids=input_ids,
+ position_ids=position_ids,
+ attention_mask=attention_mask,
+ image_flags=image_flags,
+ pixel_values=concat_images,
+ labels=labels,
+ use_cache=use_cache)
+ loss_dict = {'loss': outputs.loss}
+ return loss_dict
+
+ def _llm_forward(
+ self,
+ pixel_values: torch.FloatTensor,
+ input_ids: torch.LongTensor = None,
+ attention_mask: Optional[torch.Tensor] = None,
+ position_ids: Optional[torch.LongTensor] = None,
+ image_flags: Optional[torch.LongTensor] = None,
+ past_key_values: Optional[List[torch.FloatTensor]] = None,
+ labels: Optional[torch.LongTensor] = None,
+ use_cache: Optional[bool] = None,
+ output_attentions: Optional[bool] = None,
+ output_hidden_states: Optional[bool] = None,
+ return_dict: Optional[bool] = None,
+ ) -> Union[Tuple, CausalLMOutputWithPast]:
+ return_dict = return_dict if return_dict is not None \
+ else self.model.config.use_return_dict
+
+ image_flags = image_flags.squeeze(-1)
+ # We only added the clone code here to avoid the error.
+ input_embeds = self.model.language_model.get_input_embeddings()(
+ input_ids).clone()
+
+ vit_embeds = self.model.extract_feature(pixel_values)
+ vit_embeds = vit_embeds[image_flags == 1]
+ vit_batch_size = pixel_values.shape[0]
+
+ B, N, C = input_embeds.shape
+ input_embeds = input_embeds.reshape(B * N, C)
+
+ if torch.distributed.get_rank() == 0 and self._count % 100 == 0:
+ print(f'dynamic ViT batch size: {vit_batch_size}, '
+ f'images per sample: {vit_batch_size / B}, '
+ f'dynamic token length: {N}')
+ self._count += 1
+
+ input_ids = input_ids.reshape(B * N)
+ selected = (input_ids == self.model.img_context_token_id)
+ try:
+ input_embeds[
+ selected] = input_embeds[selected] * 0.0 + vit_embeds.reshape(
+ -1, C)
+ except Exception as e:
+ vit_embeds = vit_embeds.reshape(-1, C)
+ print(f'warning: {e}, input_embeds[selected].shape='
+ f'{input_embeds[selected].shape}, '
+ f'vit_embeds.shape={vit_embeds.shape}')
+ n_token = selected.sum()
+ input_embeds[
+ selected] = input_embeds[selected] * 0.0 + vit_embeds[:n_token]
+
+ input_embeds = input_embeds.reshape(B, N, C)
+
+ outputs = self.model.language_model(
+ inputs_embeds=input_embeds,
+ attention_mask=attention_mask,
+ position_ids=position_ids,
+ past_key_values=past_key_values,
+ use_cache=use_cache,
+ output_attentions=output_attentions,
+ output_hidden_states=output_hidden_states,
+ return_dict=return_dict,
+ )
+ logits = outputs.logits
+
+ loss = None
+ if labels is not None:
+ # Shift so that tokens < n predict n
+ shift_logits = logits[..., :-1, :].contiguous()
+ shift_labels = labels[..., 1:].contiguous()
+ # Flatten the tokens
+ loss_fct = CrossEntropyLoss()
+ shift_logits = shift_logits.view(
+ -1, self.model.language_model.config.vocab_size)
+ shift_labels = shift_labels.view(-1)
+ # Enable model parallelism
+ shift_labels = shift_labels.to(shift_logits.device)
+ loss = loss_fct(shift_logits, shift_labels)
+
+ if not return_dict:
+ output = (logits, ) + outputs[1:]
+ return (loss, ) + output if loss is not None else output
+
+ return CausalLMOutputWithPast(
+ loss=loss,
+ logits=logits,
+ past_key_values=outputs.past_key_values,
+ hidden_states=outputs.hidden_states,
+ attentions=outputs.attentions,
+ )
diff --git a/xtuner/model/llava.py b/xtuner/model/llava.py
index 19b427a75..2040e6845 100644
--- a/xtuner/model/llava.py
+++ b/xtuner/model/llava.py
@@ -1,15 +1,25 @@
# Copyright (c) OpenMMLab. All rights reserved.
import math
+import os.path as osp
+import warnings
from collections import OrderedDict
import torch
import torch.nn as nn
+from accelerate import init_empty_weights
+from mmengine import print_log
from mmengine.config import Config, ConfigDict
from mmengine.model import BaseModel
from peft import get_peft_model, prepare_model_for_kbit_training
-from transformers import AutoConfig
+from transformers import (AddedToken, AutoConfig, CLIPImageProcessor,
+ CLIPVisionModel, LlamaForCausalLM,
+ LlamaTokenizerFast, LlavaConfig,
+ LlavaForConditionalGeneration, LlavaProcessor)
+from transformers.integrations import is_deepspeed_zero3_enabled
from xtuner.registry import BUILDER
+from xtuner.utils import DEFAULT_IMAGE_TOKEN
+from xtuner.utils.device import get_torch_device
from .modules import ProjectorConfig, ProjectorModel, dispatch_modules
from .modules.dispatch import SUPPORT_FLASH1, SUPPORT_FLASH2
from .utils import (LoadWoInit, find_all_linear_names,
@@ -17,6 +27,17 @@
make_inputs_require_grad,
prepare_inputs_labels_for_multimodal, traverse_dict)
+def convert_state_dict_to_hf(state_dict, mapping):
+ new_state_dict = {}
+ for key, value in state_dict.items():
+ if key.endswith('.inv_freq'):
+ continue
+ for key_to_modify, new_key in mapping.items():
+ if key_to_modify in key:
+ key = key.replace(key_to_modify, new_key)
+ new_state_dict[key] = value
+ return new_state_dict
+
class LLaVAModel(BaseModel):
@@ -45,10 +66,11 @@ def __init__(self,
self.llm.config.use_cache = False
dispatch_modules(self.llm)
+ self.projector_depth = projector_depth
projector_config = ProjectorConfig(
visual_hidden_size=self.visual_encoder.config.hidden_size,
llm_hidden_size=self.llm.config.hidden_size,
- depth=projector_depth)
+ depth=self.projector_depth)
self.projector = ProjectorModel(projector_config).to(
self.visual_encoder.dtype)
@@ -87,12 +109,15 @@ def __init__(self,
pretrained_state_dict = guess_load_checkpoint(pretrained_pth)
self.load_state_dict(pretrained_state_dict, strict=False)
- print(f'Load pretrained weight from {pretrained_pth}')
+ print_log(f'Load pretrained weight from {pretrained_pth}',
+ 'current')
self.visual_select_layer = visual_select_layer
self._is_init = True
+ self.is_first_iter = True
+
def _parse_lora_config(self, lora_config):
if isinstance(lora_config, dict) or isinstance(
lora_config, Config) or isinstance(lora_config, ConfigDict):
@@ -196,22 +221,50 @@ def _prepare_for_long_context_training(cfg, llm_cfg,
def _prepare_for_flash_attn(cfg, llm_cfg):
cls_name = type(llm_cfg).__name__
SUPPORT_SDPA_ATTN = ('LlamaConfig', 'GemmaConfig', 'MistralConfig',
- 'MixtralConfig', 'Qwen2Config',
- 'Starcoder2Config', 'Starcoder2Config')
+ 'MixtralConfig', 'Qwen2Config', 'Qwen2MoeConfig',
+ 'Starcoder2Config', 'Starcoder2Config',
+ 'Phi3Config')
SUPPORT_FLASH_ATTN2 = ('InternLM2Config', 'LlamaConfig', 'GemmaConfig',
'MistralConfig', 'MixtralConfig', 'Qwen2Config',
- 'Starcoder2Config', 'Starcoder2Config')
-
- if SUPPORT_FLASH2 and cls_name in SUPPORT_FLASH_ATTN2:
- cfg.torch_dtype = torch.bfloat16 \
- if torch.cuda.is_bf16_supported() else torch.float16
+ 'Qwen2MoeConfig', 'Starcoder2Config',
+ 'Starcoder2Config', 'Phi3Config')
+
+ torch_dtype = torch.bfloat16 if (
+ get_torch_device().is_available() and get_torch_device().is_bf16_supported()) \
+ else torch.float16
+
+ if getattr(cfg, 'attn_implementation', None) is not None:
+ # Flash Attention 2.0 only supports torch.float16 and
+ # torch.bfloat16 dtypes
+ if cfg.attn_implementation == 'flash_attention_2':
+ cfg.torch_dtype = torch_dtype
+ elif SUPPORT_FLASH2 and cls_name in SUPPORT_FLASH_ATTN2:
+ cfg.torch_dtype = torch_dtype
cfg.attn_implementation = 'flash_attention_2'
elif SUPPORT_FLASH1 and cls_name in SUPPORT_SDPA_ATTN:
cfg.attn_implementation = 'sdpa'
return cfg, llm_cfg
+ @staticmethod
+ def _prepare_for_qlora_zero3(cfg):
+ if (not is_deepspeed_zero3_enabled()) or (not hasattr(
+ cfg, 'quantization_config')):
+ return cfg
+
+ torch_dtype = torch.bfloat16 if (
+ get_torch_device().is_available() and get_torch_device().is_bf16_supported()) \
+ else torch.float16
+
+ cfg.torch_dtype = torch_dtype
+ quantization_config = cfg.quantization_config
+ quantization_config.bnb_4bit_compute_dtype = torch_dtype
+ quantization_config.bnb_4bit_quant_storage = torch_dtype
+
+ return cfg
+
def _dispatch_lm_model_cfg(self, cfg, max_position_embeddings=None):
+ cfg = self._prepare_for_qlora_zero3(cfg)
pretrained_model_name_or_path = cfg.pretrained_model_name_or_path
llm_cfg = AutoConfig.from_pretrained(
pretrained_model_name_or_path, trust_remote_code=True)
@@ -231,6 +284,14 @@ def _build_from_cfg_or_module(self, cfg_or_mod):
raise NotImplementedError
def forward(self, data, data_samples=None, mode='loss'):
+ if self.is_first_iter:
+ # hardcode for qlora DeepSpeed ZeRO3, put buffers and QuantState to
+ # device
+ # Only required in `LLaVAModel` .
+ # We do not need this in `SupervisedFinetune` .
+ self.to(data['input_ids'].device)
+ self.is_first_iter = False
+
if 'pixel_values' in data:
visual_outputs = self.visual_encoder(
data['pixel_values'].to(self.visual_encoder.dtype),
@@ -270,3 +331,305 @@ def __getattr__(self, name: str):
return super().__getattr__(name)
except AttributeError:
return getattr(self.llm, name)
+
+ def to_hf(self,
+ cfg,
+ save_dir,
+ fp32=False,
+ save_pretrained_kwargs={},
+ save_format='xtuner',
+ **kwargs):
+ if save_format == 'xtuner':
+ self.to_xtuner_llava(cfg, save_dir, fp32, save_pretrained_kwargs)
+ elif save_format == 'huggingface':
+ self.to_huggingface_llava(cfg, save_dir, fp32,
+ save_pretrained_kwargs)
+ elif save_format == 'official':
+ self.to_official_llava(cfg, save_dir, fp32, save_pretrained_kwargs)
+ else:
+ raise NotImplementedError
+
+ def to_xtuner_llava(self,
+ cfg,
+ save_dir,
+ fp32=False,
+ save_pretrained_kwargs={}):
+ # LLM
+ self.llm.config.use_cache = True
+ if not fp32:
+ print_log('Convert LLM to float16', 'current')
+ self.llm.half()
+ if self.use_llm_lora:
+ llm_path = osp.join(save_dir, 'llm_adapter')
+ print_log(f'Saving LLM adapter to {llm_path}', 'current')
+ self.llm.save_pretrained(llm_path, **save_pretrained_kwargs)
+ elif not self.freeze_llm:
+ llm_path = save_dir
+ print_log(f'Saving LLM tokenizer to {llm_path}', 'current')
+ tokenizer = BUILDER.build(cfg.tokenizer)
+ tokenizer.save_pretrained(llm_path, **save_pretrained_kwargs)
+ print_log(f'Saving LLM to {llm_path}', 'current')
+ self.llm.save_pretrained(llm_path, **save_pretrained_kwargs)
+ self.llm.config.use_cache = False
+
+ # Visual Encoder
+ if self.use_visual_encoder_lora:
+ visual_encoder_path = osp.join(save_dir, 'visual_encoder_adapter')
+ print_log(
+ f'Saving visual_encoder adapter to {visual_encoder_path}',
+ 'current')
+ self.visual_encoder.save_pretrained(visual_encoder_path,
+ **save_pretrained_kwargs)
+ elif not self.freeze_visual_encoder:
+ visual_encoder_path = osp.join(save_dir, 'visual_encoder')
+ print_log(
+ 'Saving visual_encoder image_processor to'
+ f'{visual_encoder_path}', 'current')
+ image_processor = BUILDER.build(cfg.image_processor)
+ image_processor.save_pretrained(visual_encoder_path,
+ **save_pretrained_kwargs)
+ print_log(f'Saving visual_encoder to {visual_encoder_path}',
+ 'current')
+ self.visual_encoder.save_pretrained(visual_encoder_path,
+ **save_pretrained_kwargs)
+
+ # Projector
+ projector_path = osp.join(save_dir, 'projector')
+ print_log(f'Saving projector to {projector_path}', 'current')
+ self.projector.save_pretrained(projector_path,
+ **save_pretrained_kwargs)
+
+ def to_huggingface_llava(self,
+ cfg,
+ save_dir,
+ fp32=False,
+ save_pretrained_kwargs={}):
+
+ LLM_MAPPING = {
+ 'model': 'language_model.model',
+ 'lm_head': 'language_model.lm_head',
+ }
+ VIT_MAPPING = {
+ 'vision_model': 'vision_tower.vision_model',
+ }
+ PROJECTOR_MAPPING = {
+ 'model.0': 'multi_modal_projector.linear_1',
+ 'model.2': 'multi_modal_projector.linear_2',
+ }
+
+ assert getattr(self.llm, 'hf_quantizer', None) is None, \
+ 'This conversion format does not support quantized LLM.'
+
+ # get state_dict
+ llm = self.llm
+ if self.use_llm_lora:
+ llm = self.llm.merge_and_unload()
+ llm.config.use_cache = True
+ if not fp32:
+ print_log('Convert LLM to float16', 'current')
+ llm.half()
+
+ assert isinstance(llm, LlamaForCausalLM), \
+ 'This conversion format only supports LlamaForCausalLM.'
+ llm_state_dict = llm.state_dict()
+ llm_state_dict = convert_state_dict_to_hf(llm_state_dict, LLM_MAPPING)
+
+ need_visual_encoder = (not self.freeze_visual_encoder
+ or self.use_visual_encoder_lora)
+ visual_encoder = self.visual_encoder
+ if self.use_visual_encoder_lora:
+ visual_encoder = self.visual_encoder.merge_and_unload()
+ assert isinstance(visual_encoder, CLIPVisionModel),\
+ 'This conversion format only supports CLIPVisionModel.'
+ if need_visual_encoder:
+ visual_encoder_state_dict = visual_encoder.state_dict()
+ visual_encoder_state_dict = convert_state_dict_to_hf(
+ visual_encoder_state_dict, VIT_MAPPING)
+ else:
+ visual_encoder_state_dict = {}
+
+ projector_state_dict = self.projector.state_dict()
+ projector_state_dict = convert_state_dict_to_hf(
+ projector_state_dict, PROJECTOR_MAPPING)
+
+ state_dict = {
+ **projector_state_dict,
+ **llm_state_dict,
+ **visual_encoder_state_dict
+ }
+
+ # init model
+ text_config = llm.config
+ vision_config = visual_encoder.config
+ config = LlavaConfig(
+ text_config=text_config,
+ vision_config=vision_config,
+ attn_implementation='eager')
+
+ with init_empty_weights():
+ with warnings.catch_warnings():
+ warnings.filterwarnings(
+ 'ignore', message='.*non-meta.*', category=UserWarning)
+ model = LlavaForConditionalGeneration(config)
+ model.load_state_dict(state_dict, strict=True, assign=True)
+
+ # processor
+ cfg.tokenizer.type = LlamaTokenizerFast.from_pretrained
+ tokenizer = BUILDER.build(cfg.tokenizer)
+
+ tokenizer.add_tokens(
+ AddedToken(DEFAULT_IMAGE_TOKEN, special=True, normalized=False),
+ special_tokens=True)
+ tokenizer.add_special_tokens({'pad_token': ''})
+
+ image_processor = BUILDER.build(cfg.image_processor)
+ assert isinstance(image_processor, CLIPImageProcessor),\
+ 'This conversion format only supports CLIPImageProcessor.'
+
+ processor = LlavaProcessor(
+ tokenizer=tokenizer, image_processor=image_processor)
+
+ # Pad to 64 for performance reasons
+ pad_shape = 64
+
+ pre_expansion_embeddings = \
+ model.language_model.model.embed_tokens.weight.data
+ mu = torch.mean(pre_expansion_embeddings, dim=0).float()
+ n = pre_expansion_embeddings.size()[0]
+ sigma = ((pre_expansion_embeddings - mu).T
+ @ (pre_expansion_embeddings - mu)) / n
+ dist = torch.distributions.multivariate_normal.MultivariateNormal(
+ mu, covariance_matrix=1e-5 * sigma)
+
+ # We add an image token so we need to resize the model
+ ori_vocab_size = config.text_config.vocab_size
+ tokenizer_vocab_size = tokenizer.encode('')[-1]
+ added_token = tokenizer_vocab_size - ori_vocab_size
+
+ if added_token > 0:
+ model.resize_token_embeddings(ori_vocab_size + added_token,
+ pad_shape)
+ model.language_model.model.embed_tokens.weight.data[
+ ori_vocab_size:] = torch.stack(
+ tuple(
+ dist.sample()
+ for _ in range(model.language_model.model.embed_tokens.
+ weight.data[ori_vocab_size:].shape[0])),
+ dim=0,
+ )
+ model.language_model.lm_head.weight.data[
+ ori_vocab_size:] = torch.stack(
+ tuple(dist.sample()
+ for _ in range(model.language_model.lm_head.weight.
+ data[ori_vocab_size:].shape[0])),
+ dim=0,
+ )
+ model.config.image_token_index = tokenizer.encode(
+ DEFAULT_IMAGE_TOKEN)[-1]
+ model.config.pad_token_id = tokenizer.encode('')[-1]
+
+ # save
+ print_log(f'Saving to {save_dir}', 'current')
+ model.save_pretrained(save_dir, **save_pretrained_kwargs)
+ processor.save_pretrained(save_dir, **save_pretrained_kwargs)
+
+ def to_official_llava(self,
+ cfg,
+ save_dir,
+ fp32=False,
+ save_pretrained_kwargs={}):
+
+ VIT_MAPPING = {
+ 'vision_model': 'model.vision_tower.vision_tower.vision_model',
+ }
+ PROJECTOR_MAPPING = {
+ 'model.0': 'model.mm_projector.0',
+ 'model.2': 'model.mm_projector.2',
+ }
+
+ try:
+ from llava.model import LlavaConfig, LlavaLlamaForCausalLM
+ except ImportError:
+ raise ImportError(
+ 'Please install llava with '
+ '`pip install git+https://github.com/haotian-liu/LLaVA.git '
+ '--no-deps`.')
+
+ assert getattr(self.llm, 'hf_quantizer', None) is None, \
+ 'This conversion format does not support quantized LLM.'
+
+ # get state_dict
+ llm = self.llm
+ if self.use_llm_lora:
+ llm = self.llm.merge_and_unload()
+ llm.config.use_cache = True
+ if not fp32:
+ print_log('Convert LLM to float16', 'current')
+ llm.half()
+
+ assert isinstance(llm, LlamaForCausalLM), \
+ 'This conversion format only supports LlamaForCausalLM.'
+ llm_state_dict = llm.state_dict()
+
+ need_visual_encoder = (not self.freeze_visual_encoder
+ or self.use_visual_encoder_lora)
+ visual_encoder = self.visual_encoder
+ if self.use_visual_encoder_lora:
+ visual_encoder = self.visual_encoder.merge_and_unload()
+ assert isinstance(visual_encoder, CLIPVisionModel),\
+ 'This conversion format only supports CLIPVisionModel.'
+ if need_visual_encoder:
+ visual_encoder_state_dict = visual_encoder.state_dict()
+ visual_encoder_state_dict = convert_state_dict_to_hf(
+ visual_encoder_state_dict, VIT_MAPPING)
+ else:
+ visual_encoder_state_dict = {}
+
+ projector_state_dict = self.projector.state_dict()
+ projector_state_dict = convert_state_dict_to_hf(
+ projector_state_dict, PROJECTOR_MAPPING)
+
+ state_dict = {
+ **projector_state_dict,
+ **llm_state_dict,
+ **visual_encoder_state_dict
+ }
+
+ # init model
+ tokenizer = BUILDER.build(cfg.tokenizer)
+ image_processor = BUILDER.build(cfg.image_processor)
+ assert isinstance(image_processor, CLIPImageProcessor),\
+ 'This conversion format only supports CLIPImageProcessor.'
+
+ llava_config_dict = llm.config.__dict__.copy()
+ llava_config_dict.update(
+ dict(
+ image_aspect_ratio='pad',
+ mm_hidden_size=visual_encoder.config.hidden_size,
+ mm_projector_type=f'mlp{self.projector_depth}x_gelu',
+ mm_use_im_patch_token=False,
+ mm_use_im_start_end=False,
+ mm_vision_select_feature='patch',
+ mm_vision_select_layer=self.visual_select_layer,
+ mm_vision_tower=visual_encoder.config.name_or_path,
+ unfreeze_mm_vision_tower=need_visual_encoder,
+ model_type='llava',
+ use_cache=True,
+ use_mm_proj=True))
+
+ llava_config = LlavaConfig(**llava_config_dict)
+
+ with init_empty_weights():
+ with warnings.catch_warnings():
+ warnings.filterwarnings(
+ 'ignore', message='.*non-meta.*', category=UserWarning)
+ model = LlavaLlamaForCausalLM(llava_config)
+
+ model.load_state_dict(state_dict, strict=True, assign=True)
+
+ # save
+ print_log(f'Saving to {save_dir}', 'current')
+
+ model.save_pretrained(save_dir, **save_pretrained_kwargs)
+ image_processor.save_pretrained(save_dir, **save_pretrained_kwargs)
+ tokenizer.save_pretrained(save_dir, **save_pretrained_kwargs)
diff --git a/xtuner/model/modules/dispatch/__init__.py b/xtuner/model/modules/dispatch/__init__.py
index 7da62ac0e..b40063bdf 100644
--- a/xtuner/model/modules/dispatch/__init__.py
+++ b/xtuner/model/modules/dispatch/__init__.py
@@ -1,29 +1,19 @@
# Copyright (c) OpenMMLab. All rights reserved.
-import logging
import os
import types
import torch
import transformers
-from mmengine import print_log
+from mmengine.config.lazy import LazyObject
from mmengine.utils import digit_version
-
-from .baichuan import (baichuan2_norm_head_forward, baichuan_7b_attn_forward,
- baichuan_13b_attn_forward)
-from .yi import yi_attn_forward
-
-IS_LOW_VERSION_TRANSFORMERS = digit_version(
- transformers.__version__) < digit_version('4.38')
-SUPPORT_FLASH1 = digit_version(torch.__version__) >= digit_version('2.0.0')
-SUPPORT_FLASH2 = False
-
-try:
- from flash_attn import flash_attn_func # pre-check # noqa: F401
-
- SUPPORT_FLASH2 = True
-except ImportError:
- pass
-
+from transformers.utils.import_utils import is_flash_attn_2_available
+
+TRANSFORMERS_VERSION = digit_version(transformers.__version__)
+IS_LOW_VERSION_TRANSFORMERS = TRANSFORMERS_VERSION < digit_version('4.38')
+# Transformers requires torch version >= 2.1.1 when using Torch SDPA.
+# Refer to https://github.com/huggingface/transformers/blob/caa5c65db1f4db617cdac2ad667ba62edf94dd98/src/transformers/modeling_utils.py#L1611 # noqa: E501
+SUPPORT_FLASH1 = digit_version(torch.__version__) >= digit_version('2.1.1')
+SUPPORT_FLASH2 = is_flash_attn_2_available()
SUPPORT_FLASH = SUPPORT_FLASH1 or SUPPORT_FLASH2
USE_TRITON_KERNEL = bool(os.getenv('USE_TRITON_KERNEL', default=0))
@@ -43,162 +33,200 @@
'even when the `output_attentions` flag is set to True, it is not '
'possible to return the `attn_weights`.')
-
-def dispatch_llama_attn_forward(model, use_varlen_attn):
- if use_varlen_attn:
- assert SUPPORT_FLASH2 and SUPPORT_TRITON, \
- 'flash_attn and triton is required if you want to use varlen_attn.'
- elif not SUPPORT_FLASH2:
+LOWEST_TRANSFORMERS_VERSION = dict(
+ InternLM3ForCausalLM=digit_version('4.48'),
+ InternLM2ForCausalLM=digit_version('4.36'),
+ InternLMForCausalLM=digit_version('4.36'),
+ LlamaForCausalLM=digit_version('4.48'),
+ Phi3ForCausalLM=digit_version('4.39'),
+ MistralForCausalLM=digit_version('4.48'),
+ # Training mixtral with lower version may lead to nccl timeout
+ # Refer to https://github.com/microsoft/DeepSpeed/issues/5066
+ MixtralForCausalLM=digit_version('4.48'),
+ CohereForCausalLM=digit_version('4.40'),
+ Qwen2ForCausalLM=digit_version('4.48'),
+ Qwen2MoeForCausalLM=digit_version('4.48'),
+ DeepseekV2ForCausalLM=digit_version('4.40'),
+)
+
+ATTN_DISPATCH_MAPPING = dict(
+ InternLM3Attention=LazyObject('xtuner.model.modules.dispatch.internlm3',
+ 'internlm3_attn_forward'),
+ InternLM2FlashAttention2=LazyObject(
+ 'xtuner.model.modules.dispatch.internlm2', 'internlm2_attn_forward'),
+ InternLMAttention=LazyObject('xtuner.model.modules.dispatch.internlm',
+ 'internlm_attn_forward'),
+ LlamaAttention=LazyObject('xtuner.model.modules.dispatch.llama',
+ 'llama_attn_forward'),
+ Phi3FlashAttention2=LazyObject('xtuner.model.modules.dispatch.phi3',
+ 'phi3_attn_forward'),
+ MistralAttention=LazyObject('xtuner.model.modules.dispatch.mistral',
+ 'mistral_attn_forward'),
+ MixtralAttention=LazyObject('xtuner.model.modules.dispatch.mistral',
+ 'mistral_attn_forward'),
+ CohereFlashAttention2=LazyObject('xtuner.model.modules.dispatch.cohere',
+ 'cohere_attn_forward'),
+ Qwen2Attention=LazyObject('xtuner.model.modules.dispatch.qwen2',
+ 'qwen2_attn_forward'),
+ Qwen2MoeAttention=LazyObject('xtuner.model.modules.dispatch.qwen2',
+ 'qwen2_attn_forward'),
+ DeepseekV2FlashAttention2=LazyObject(
+ 'xtuner.model.modules.dispatch.deepseek_v2', 'deepseek_attn_forward'),
+)
+
+VARLEN_ATTN_DISPATCH_MAPPING = dict(
+ InternLM3Attention=LazyObject('xtuner.model.modules.dispatch.internlm3',
+ 'internlm3_attn_forward'),
+ InternLM2FlashAttention2=LazyObject(
+ 'xtuner.model.modules.dispatch.internlm2',
+ 'internlm2_varlen_attn_forward'),
+ InternLMAttention=LazyObject('xtuner.model.modules.dispatch.internlm',
+ 'internlm_varlen_attn_forward'),
+ LlamaAttention=LazyObject('xtuner.model.modules.dispatch.llama',
+ 'llama_attn_forward'),
+ Phi3FlashAttention2=LazyObject('xtuner.model.modules.dispatch.phi3',
+ 'phi3_varlen_attn_forward'),
+ MistralAttention=LazyObject('xtuner.model.modules.dispatch.mistral',
+ 'mistral_attn_forward'),
+ MixtralAttention=LazyObject('xtuner.model.modules.dispatch.mistral',
+ 'mistral_attn_forward'),
+ CohereFlashAttention2=None,
+ Qwen2Attention=LazyObject('xtuner.model.modules.dispatch.qwen2',
+ 'qwen2_attn_forward'),
+ Qwen2MoeAttention=LazyObject('xtuner.model.modules.dispatch.qwen2',
+ 'qwen2_attn_forward'),
+ DeepseekV2FlashAttention2=LazyObject(
+ 'xtuner.model.modules.dispatch.deepseek_v2',
+ 'deepseek_varlen_attn_forward'),
+ InternLM3FlashCrossAttention2=LazyObject(
+ 'xtuner.model.modules.dispatch.internlm3',
+ 'internlm3_cross_attn_varlen_forward'),
+ InternLM3FlashSelfAttention2=LazyObject(
+ 'xtuner.model.modules.dispatch.internlm3',
+ 'internlm3_self_attn_varlen_forward')
+)
+
+RMS_DISPATCH_MAPPING = dict(
+ InternLM3RMSNorm=LazyObject('xtuner.model.modules.dispatch.triton_kernels',
+ 'rms_norm_forward'),
+ InternLM2RMSNorm=LazyObject('xtuner.model.modules.dispatch.triton_kernels',
+ 'rms_norm_forward'),
+ InternLMRMSNorm=LazyObject('xtuner.model.modules.dispatch.triton_kernels',
+ 'rms_norm_forward'),
+ LlamaRMSNorm=LazyObject('xtuner.model.modules.dispatch.triton_kernels',
+ 'rms_norm_forward'),
+ Phi3RMSNorm=LazyObject('xtuner.model.modules.dispatch.triton_kernels',
+ 'rms_norm_forward'),
+ MistralRMSNorm=LazyObject('xtuner.model.modules.dispatch.triton_kernels',
+ 'rms_norm_forward'),
+ MixtralRMSNorm=LazyObject('xtuner.model.modules.dispatch.triton_kernels',
+ 'rms_norm_forward'),
+ CohereLayerNorm=LazyObject('xtuner.model.modules.dispatch.triton_kernels',
+ 'layer_norm_forward'),
+ Qwen2RMSNorm=LazyObject('xtuner.model.modules.dispatch.triton_kernels',
+ 'rms_norm_forward'),
+ Qwen2MoeRMSNorm=LazyObject('xtuner.model.modules.dispatch.triton_kernels',
+ 'rms_norm_forward'),
+)
+
+ROTE_DISPATCH_MAPPING = dict(
+ InternLMRotaryEmbedding=LazyObject(
+ 'xtuner.model.modules.dispatch.internlm', 'InternLMRotaryEmbedding'), )
+
+
+def log_once(func):
+ logged = False
+
+ def wrapper(*args, **kwargs):
+ nonlocal logged
+ if not logged:
+ logged = True
+ func(*args, **kwargs)
return
- from .llama import (llama_attn_forward, llama_attn_forward_legacy,
- llama_varlen_attn_forward,
- llama_varlen_attn_forward_legacy)
+ return wrapper
- print_log(NO_ATTN_WEIGHTS_MSG, 'current', logging.WARNING)
- for module in model.modules():
- # Do not need to dispatch if
- # type(module).__name__ == 'LlamaSdpaAttention', as flash_attn is
- # required when using sequence parallel
- if type(module).__name__ in ('LlamaAttention', 'LlamaFlashAttention2'):
- if use_varlen_attn:
- print_log('dispatch llama varlen attn forward', 'current')
- if IS_LOW_VERSION_TRANSFORMERS:
- module.forward = types.MethodType(
- llama_varlen_attn_forward_legacy, module)
- else:
- module.forward = types.MethodType(
- llama_varlen_attn_forward, module)
- else:
- print_log('dispatch llama attn forward', 'current')
- if IS_LOW_VERSION_TRANSFORMERS:
- module.forward = types.MethodType(
- llama_attn_forward_legacy, module)
- else:
- module.forward = types.MethodType(llama_attn_forward,
- module)
+def dispatch_attn_forward(model):
-def dispatch_llama_rmsnorm_forward(model):
- if not SUPPORT_TRITON:
+ if not SUPPORT_FLASH2:
return
- from .triton_kernels import rms_norm_forward
+ from mmengine import print_log
+ print_log = log_once(print_log)
+ attn_forward = None
for module in model.modules():
- if type(module).__name__ == 'LlamaRMSNorm':
- print_log('dispatch llama rmsnorm forward', 'current')
- module.forward = types.MethodType(rms_norm_forward, module)
+ name = type(module).__name__
+ if name in ATTN_DISPATCH_MAPPING:
+ if attn_forward is None:
+ attn_forward = ATTN_DISPATCH_MAPPING[name]
+ attn_forward = attn_forward.build()
+ print_log(f'Dispatch {name} forward. {NO_ATTN_WEIGHTS_MSG}',
+ 'current')
+ module.forward = types.MethodType(attn_forward, module)
-def dispatch_internlm_attn_forward(model, use_varlen_attn):
- if use_varlen_attn:
- assert SUPPORT_FLASH2 and SUPPORT_TRITON, \
- 'flash_attn and triton is required if you want to use varlen_attn.'
- elif not SUPPORT_FLASH:
- return
+def dispatch_varlen_attn_forward(model):
- from .internlm import internlm_attn_forward, internlm_varlen_attn_forward
-
- print_log(NO_ATTN_WEIGHTS_MSG, 'current', logging.WARNING)
- for module in model.modules():
- if type(module).__name__ == 'InternLMAttention':
- if use_varlen_attn:
- print_log('dispatch internlm varlen attn forward', 'current')
- module.forward = types.MethodType(internlm_varlen_attn_forward,
- module)
- else:
- print_log('dispatch internlm attn forward', 'current')
- module.forward = types.MethodType(internlm_attn_forward,
- module)
-
-
-def dispatch_internlm2_attn_forward(model, use_varlen_attn):
- if use_varlen_attn:
- assert SUPPORT_FLASH2 and SUPPORT_TRITON, \
- 'flash_attn and triton is required if you want to use varlen_attn.'
- elif not SUPPORT_FLASH:
+ if not SUPPORT_FLASH2:
return
- from .internlm2 import (internlm2_attn_forward,
- internlm2_varlen_attn_forward)
+ from mmengine import print_log
+ # print_log = log_once(print_log)
- print_log(NO_ATTN_WEIGHTS_MSG, 'current', logging.WARNING)
+
for module in model.modules():
- if type(module).__name__ in ('InternLM2Attention',
- 'InternLM2FlashAttention2'):
- if use_varlen_attn:
- print_log('dispatch internlm2 varlen attn forward', 'current')
- module.forward = types.MethodType(
- internlm2_varlen_attn_forward, module)
- else:
- print_log('dispatch internlm2 attn forward', 'current')
- module.forward = types.MethodType(internlm2_attn_forward,
- module)
-
-
-def dispatch_internlm_rmsnorm_forward(model):
- if not SUPPORT_TRITON:
- return
-
- from .triton_kernels import rms_norm_forward
+ name = type(module).__name__
+ if name in VARLEN_ATTN_DISPATCH_MAPPING:
+ if varlen_attn_forward is None:
+ varlen_attn_forward = VARLEN_ATTN_DISPATCH_MAPPING[name]
+ varlen_attn_forward = varlen_attn_forward.build()
+ print_log(f'Dispatch {name} varlen forward. {NO_ATTN_WEIGHTS_MSG}',
+ 'current')
+ module.forward = types.MethodType(varlen_attn_forward, module)
- for module in model.modules():
- if type(module).__name__ == 'InternLMRMSNorm':
- print_log('dispatch internlm rmsnorm forward', 'current')
- module.forward = types.MethodType(rms_norm_forward, module)
+def dispatch_rmsnorm_forward(model):
-def dispatch_internlm2_rmsnorm_forward(model):
- if not SUPPORT_TRITON:
+ if (not SUPPORT_TRITON) or (not USE_TRITON_KERNEL):
return
- from .triton_kernels import rms_norm_forward
+ from mmengine import print_log
+ print_log = log_once(print_log)
+ rms_forward = None
for module in model.modules():
- if type(module).__name__ == 'InternLM2RMSNorm':
- print_log('dispatch internlm2 rmsnorm forward', 'current')
- module.forward = types.MethodType(rms_norm_forward, module)
+ name = type(module).__name__
+ if name in RMS_DISPATCH_MAPPING:
+ if rms_forward is None:
+ rms_forward = RMS_DISPATCH_MAPPING[name]
+ rms_forward = rms_forward.build()
+ print_log(f'Dispatch {name} forward.', 'current')
+ module.forward = types.MethodType(rms_forward, module)
-def replace_internlm_rote(model):
- from .internlm import InternLMRotaryEmbedding
-
- def traverse(module):
- for name, child in module.named_children():
- if type(child).__name__ in (
- 'InternLMRotaryEmbedding',
- 'InternLMDynamicNTKScalingRotaryEmbedding'):
- print_log('replace internlm rope', 'current')
- dim_model = child.inv_freq.shape[0] * 2
- child_new = InternLMRotaryEmbedding(
- dim_model, child.max_seq_len_cached).to(
- device=child.inv_freq.device,
- dtype=child.inv_freq.dtype)
- setattr(module, name, child_new)
- else:
- traverse(child)
-
- traverse(model)
+def replace_rote(model):
-
-def replace_internlm2_rote(model):
- from .internlm2 import InternLM2RotaryEmbedding
-
- rotary_base = model.config.rope_theta
+ from mmengine import print_log
+ print_log = log_once(print_log)
def traverse(module):
for name, child in module.named_children():
- if type(child).__name__ in (
- 'InternLM2RotaryEmbedding',
- 'InternLM2LinearScalingRotaryEmbedding',
- 'InternLM2DynamicNTKScalingRotaryEmbedding'):
- print_log('replace internlm2 rope', 'current')
+ cls_name = type(child).__name__
+ if cls_name in ROTE_DISPATCH_MAPPING:
+ assert hasattr(model.config, 'rope_theta'), \
+ '`rope_theta` should be in the model config.'
+ rope_theta = model.config.rope_theta
+
+ rote = ROTE_DISPATCH_MAPPING[cls_name]
+ rote = rote.build()
+ print_log(f'replace {cls_name}', 'current')
dim_model = child.inv_freq.shape[0] * 2
- child_new = InternLM2RotaryEmbedding(
- dim_model, child.max_position_embeddings, rotary_base).to(
- device=child.inv_freq.device,
- dtype=child.inv_freq.dtype)
+ child_new = rote(dim_model, child.max_seq_len_cached,
+ rope_theta).to(
+ device=child.inv_freq.device,
+ dtype=child.inv_freq.dtype)
setattr(module, name, child_new)
else:
traverse(child)
@@ -206,126 +234,26 @@ def traverse(module):
traverse(model)
-def dispath_baichuan2_norm_head_forward(model):
- print_log('dispatch baichuan2 NormHead forward', 'current')
- for module in model.modules():
- if type(module).__name__ == 'NormHead':
- module.forward = types.MethodType(baichuan2_norm_head_forward,
- module)
-
-
-def dispath_baichuan_7b_attn_forward(model):
- if digit_version(torch.__version__) < digit_version('2.0.0'):
- # flash attention is only supported after pytorch2.0
- return
- print_log('dispatch baichuan2-7B attn forward', 'current')
- print_log(NO_ATTN_WEIGHTS_MSG, 'current', logging.WARNING)
- for module in model.modules():
- if type(module).__name__ == 'Attention':
- module.forward = types.MethodType(baichuan_7b_attn_forward, module)
-
-
-def dispath_baichuan_13b_attn_forward(model):
- if digit_version(torch.__version__) < digit_version('2.0.0'):
- # flash attention is only supported after pytorch2.0
- return
- print_log('dispatch baichuan2-13B attn forward', 'current')
- print_log(NO_ATTN_WEIGHTS_MSG, 'current', logging.WARNING)
- for module in model.modules():
- if type(module).__name__ == 'BaichuanAttention':
- module.forward = types.MethodType(baichuan_13b_attn_forward,
- module)
-
-
-def dispatch_yi_attn_forward(model):
- if digit_version(torch.__version__) < digit_version('2.0.0'):
- # flash attention is only supported after pytorch2.0
- return
- print_log('dispatch yi attn forward', 'current')
- print_log(NO_ATTN_WEIGHTS_MSG, 'current', logging.WARNING)
- for module in model.modules():
- if type(module).__name__ == 'YiAttention':
- module.forward = types.MethodType(yi_attn_forward, module)
-
+def dispatch_modules(model, use_varlen_attn=False):
-def dispatch_mistral_attn_forward(model, use_varlen_attn):
- if (not SUPPORT_FLASH) or (not use_varlen_attn):
- return
+ def check(model_name):
+ if 'ForCausalLM' not in model_name and model_name.endswith('Model'):
+ # a walkaround for reward model
+ model_name = model_name[:-5] + 'ForCausalLM'
+ msg = '{} requires transformers version at least {}, but got {}'
+ if model_name in LOWEST_TRANSFORMERS_VERSION:
+ assert TRANSFORMERS_VERSION >= LOWEST_TRANSFORMERS_VERSION[
+ model_name], msg.format(
+ model_name, LOWEST_TRANSFORMERS_VERSION[model_name],
+ TRANSFORMERS_VERSION)
+
+ check(type(model).__name__)
if use_varlen_attn:
- assert SUPPORT_FLASH2 and SUPPORT_TRITON, \
- 'flash_attn and triton is required if you want to use varlen_attn.'
-
- from .mistral import mistral_varlen_attn_forward
-
- print_log(NO_ATTN_WEIGHTS_MSG, 'current', logging.WARNING)
- for module in model.modules():
- if type(module).__name__ in ('MistralAttention',
- 'MistralFlashAttention2'):
- print_log('dispatch mistral varlen attn forward', 'current')
- module.forward = types.MethodType(mistral_varlen_attn_forward,
- module)
-
-
-def dispatch_mistral_rmsnorm_forward(model):
- if not SUPPORT_TRITON:
- return
-
- from .triton_kernels import rms_norm_forward
-
- for module in model.modules():
- if type(module).__name__ == 'MistralRMSNorm':
- print_log('dispatch mistral rmsnorm forward', 'current')
- module.forward = types.MethodType(rms_norm_forward, module)
-
-
-def replace_mistral_rote(model):
- from .mistral import MistralRotaryEmbedding
-
- rotary_base = model.config.rope_theta
-
- def traverse(module):
- for name, child in module.named_children():
- if type(child).__name__ == 'MistralRotaryEmbedding':
- print_log('replace mistral rope', 'current')
- dim_model = child.inv_freq.shape[0] * 2
- child_new = MistralRotaryEmbedding(
- dim_model, child.max_seq_len_cached, rotary_base).to(
- device=child.inv_freq.device,
- dtype=child.inv_freq.dtype)
- setattr(module, name, child_new)
- else:
- traverse(child)
-
- traverse(model)
-
-
-def dispatch_modules(model, use_varlen_attn=False):
- model_name = model.__class__.__name__.lower()
- if 'internlm2' in model_name:
- dispatch_internlm2_attn_forward(model, use_varlen_attn)
- if USE_TRITON_KERNEL:
- dispatch_internlm2_rmsnorm_forward(model)
- replace_internlm2_rote(model)
- elif 'internlm' in model_name:
- dispatch_internlm_attn_forward(model, use_varlen_attn)
- if USE_TRITON_KERNEL:
- dispatch_internlm_rmsnorm_forward(model)
- replace_internlm_rote(model)
- elif 'llama' in model_name:
- dispatch_llama_attn_forward(model, use_varlen_attn)
- if USE_TRITON_KERNEL:
- dispatch_llama_rmsnorm_forward(model)
- elif 'baichuan' in model_name:
- dispath_baichuan2_norm_head_forward(model)
- dispath_baichuan_7b_attn_forward(model)
- dispath_baichuan_13b_attn_forward(model)
- elif 'yi' in model_name:
- dispatch_yi_attn_forward(model)
- elif 'mistral' in model_name:
- dispatch_mistral_attn_forward(model, use_varlen_attn)
- if USE_TRITON_KERNEL:
- dispatch_mistral_rmsnorm_forward(model)
- replace_mistral_rote(model)
+ dispatch_varlen_attn_forward(model)
+ else:
+ dispatch_attn_forward(model)
+ dispatch_rmsnorm_forward(model)
+ replace_rote(model)
__all__ = ['dispatch_modules']
diff --git a/xtuner/model/modules/dispatch/attention.py b/xtuner/model/modules/dispatch/attention.py
new file mode 100644
index 000000000..e89bb511c
--- /dev/null
+++ b/xtuner/model/modules/dispatch/attention.py
@@ -0,0 +1,97 @@
+from xtuner.parallel.sequence import sequence_parallel_wrapper
+from .utils import upad_qkv
+
+SUPPORT_FLASH2 = False
+
+try:
+ from flash_attn import flash_attn_func, flash_attn_varlen_func
+ from flash_attn.bert_padding import pad_input
+ SUPPORT_FLASH2 = True
+except ImportError:
+ pass
+
+
+@sequence_parallel_wrapper
+def flash_attn_wo_mask(
+ query_states,
+ key_states,
+ value_states,
+ dropout_p=0.0,
+ softmax_scale=None,
+ causal=True,
+ window_size=(-1, -1), # -1 means infinite context window
+):
+ attn_output = flash_attn_func(
+ query_states,
+ key_states,
+ value_states,
+ dropout_p=dropout_p,
+ softmax_scale=softmax_scale,
+ causal=causal,
+ window_size=window_size)
+ return attn_output
+
+
+@sequence_parallel_wrapper
+def flash_attn_w_mask(
+ query_states, # bs, q_len, nhead, h_dim
+ key_states,
+ value_states,
+ attention_mask,
+ softmax_scale=None,
+ causal=True,
+ dropout_p=0.0,
+ window_size=(-1, -1), # -1 means infinite context window
+):
+ batch_size, q_len = query_states.shape[:2]
+ query_states, key_states, value_states, indices_q, \
+ cu_seq_lens, max_seq_lens = upad_qkv(
+ query_states, key_states, value_states, attention_mask, q_len)
+
+ cu_seqlens_q, cu_seqlens_k = cu_seq_lens
+ max_seqlen_in_batch_q, max_seqlen_in_batch_k = max_seq_lens
+ attn_output_unpad = flash_attn_varlen_func(
+ query_states,
+ key_states,
+ value_states,
+ cu_seqlens_q=cu_seqlens_q,
+ cu_seqlens_k=cu_seqlens_k,
+ max_seqlen_q=max_seqlen_in_batch_q,
+ max_seqlen_k=max_seqlen_in_batch_k,
+ softmax_scale=softmax_scale,
+ dropout_p=dropout_p,
+ causal=causal,
+ window_size=window_size)
+ attn_output = pad_input(attn_output_unpad, indices_q, batch_size, q_len)
+ return attn_output
+
+
+@sequence_parallel_wrapper
+def varlen_flash_attn(
+ query_states,
+ key_states,
+ value_states,
+ cumulative_len,
+ max_seqlen,
+ softmax_scale=None,
+ dropout_p=0.,
+ causal=True,
+ window_size=(-1, -1), # -1 means infinite context window
+):
+ q_unpad, k_unpad, v_unpad = query_states.flatten(0, 1), key_states.flatten(
+ 0, 1), value_states.flatten(0, 1)
+ attn_output = flash_attn_varlen_func(
+ q_unpad,
+ k_unpad,
+ v_unpad,
+ cumulative_len,
+ cumulative_len,
+ max_seqlen,
+ max_seqlen,
+ softmax_scale=softmax_scale,
+ dropout_p=dropout_p,
+ return_attn_probs=False,
+ causal=causal,
+ window_size=window_size)
+ attn_output = attn_output.unsqueeze(0)
+ return attn_output
diff --git a/xtuner/model/modules/dispatch/cohere.py b/xtuner/model/modules/dispatch/cohere.py
new file mode 100644
index 000000000..8acf06747
--- /dev/null
+++ b/xtuner/model/modules/dispatch/cohere.py
@@ -0,0 +1,153 @@
+# Copyright (c) OpenMMLab. All rights reserved.
+from typing import Optional
+
+import torch
+import torch.distributed as dist
+import transformers
+from mmengine.utils import digit_version
+from transformers.models.cohere.modeling_cohere import apply_rotary_pos_emb
+
+from xtuner.parallel.sequence import get_sequence_parallel_world_size
+from xtuner.parallel.sequence.attention import (
+ post_process_for_sequence_parallel_attn,
+ pre_process_for_sequence_parallel_attn)
+
+try:
+ from transformers.cache_utils import Cache
+except ImportError:
+
+ class Cache:
+ pass
+
+
+TRANSFORMERS_VERSION = digit_version(transformers.__version__)
+IS_LOW_VERSION_TRANSFORMERS = TRANSFORMERS_VERSION < digit_version('4.43')
+
+if not IS_LOW_VERSION_TRANSFORMERS:
+ from transformers.modeling_flash_attention_utils import \
+ _flash_attention_forward
+
+
+def cohere_attn_forward(
+ self,
+ hidden_states: torch.Tensor,
+ attention_mask: Optional[torch.LongTensor] = None,
+ position_ids: Optional[torch.LongTensor] = None,
+ past_key_value: Optional[Cache] = None,
+ output_attentions: bool = False,
+ use_cache: bool = False,
+ cache_position: Optional[torch.LongTensor] = None,
+ **kwargs,
+):
+ output_attentions = False
+
+ bsz, q_len, _ = hidden_states.size()
+
+ query_states = self.q_proj(hidden_states)
+ key_states = self.k_proj(hidden_states)
+ value_states = self.v_proj(hidden_states)
+
+ query_states = query_states.view(bsz, q_len, self.num_heads, self.head_dim)
+ key_states = key_states.view(bsz, q_len, self.num_key_value_heads,
+ self.head_dim)
+ if self.use_qk_norm:
+ query_states = self.q_norm(query_states)
+ key_states = self.k_norm(key_states)
+
+ query_states = query_states.transpose(1, 2)
+ key_states = key_states.transpose(1, 2)
+ value_states = value_states.view(bsz, q_len, self.num_key_value_heads,
+ self.head_dim).transpose(1, 2)
+
+ cos, sin = self.rotary_emb(value_states, position_ids)
+ query_states, key_states = apply_rotary_pos_emb(query_states, key_states,
+ cos, sin)
+
+ past_key_value = getattr(self, 'past_key_value', past_key_value)
+
+ if past_key_value is not None:
+ # sin and cos are specific to RoPE models; position_ids needed for
+ # the static cache
+ cache_kwargs = {
+ 'sin': sin,
+ 'cos': cos,
+ 'cache_position': cache_position
+ }
+ key_states, value_states = past_key_value.update(
+ key_states, value_states, self.layer_idx, cache_kwargs)
+
+ # TODO: These transpose are quite inefficient but Flash Attention requires
+ # the layout [batch_size, sequence_length, num_heads, head_dim].
+ # We would need to refactor the KV cache to be able to avoid many of
+ # these transpose/reshape/view.
+ query_states = query_states.transpose(1, 2)
+ key_states = key_states.transpose(1, 2)
+ value_states = value_states.transpose(1, 2)
+
+ dropout_rate = self.attention_dropout if self.training else 0.0
+
+ # Ignore copy
+ # In PEFT, usually we cast the layer norms in float32 for training
+ # stability reasons therefore the input hidden states gets silently
+ # casted in float32. Hence, we need cast them back in the correct dtype
+ # just to be sure everything works as expected.
+ # This might slowdown training & inference so it is recommended to not
+ # cast the LayerNorms in fp32. (LlamaRMSNorm handles it correctly)
+
+ input_dtype = query_states.dtype
+ if input_dtype == torch.float32:
+ if torch.is_autocast_enabled():
+ target_dtype = torch.get_autocast_gpu_dtype()
+ # Handle the case where the model is quantized
+ elif hasattr(self.config, '_pre_quantization_dtype'):
+ target_dtype = self.config._pre_quantization_dtype
+ else:
+ target_dtype = self.q_proj.weight.dtype
+
+ query_states = query_states.to(target_dtype)
+ key_states = key_states.to(target_dtype)
+ value_states = value_states.to(target_dtype)
+
+ enable_sequence_parallel = (
+ dist.is_initialized() and get_sequence_parallel_world_size() > 1
+ and self.training)
+ if enable_sequence_parallel:
+ query_states, key_states, value_states = \
+ pre_process_for_sequence_parallel_attn(
+ query_states, key_states, value_states)
+ # self.num_heads is used in self._upad_input method
+ # num_heads has been changed because of sequence parallel
+ ori_num_head = self.num_heads
+ self.num_heads = query_states.shape[-2]
+
+ if IS_LOW_VERSION_TRANSFORMERS:
+ attn_output = self._flash_attention_forward(
+ query_states,
+ key_states,
+ value_states,
+ attention_mask,
+ query_states.shape[1],
+ dropout=dropout_rate)
+ else:
+ attn_output = _flash_attention_forward(
+ query_states,
+ key_states,
+ value_states,
+ attention_mask,
+ query_states.shape[1],
+ dropout=dropout_rate,
+ use_top_left_mask=self._flash_attn_uses_top_left_mask,
+ is_causal=self.is_causal,
+ )
+
+ if enable_sequence_parallel:
+ attn_output = post_process_for_sequence_parallel_attn(attn_output)
+ self.num_heads = ori_num_head
+
+ attn_output = attn_output.reshape(bsz, q_len, self.hidden_size)
+ attn_output = self.o_proj(attn_output)
+
+ if not output_attentions:
+ attn_weights = None
+
+ return attn_output, attn_weights, past_key_value
diff --git a/xtuner/model/modules/dispatch/deepseek_v2.py b/xtuner/model/modules/dispatch/deepseek_v2.py
new file mode 100644
index 000000000..bfa3ebb6d
--- /dev/null
+++ b/xtuner/model/modules/dispatch/deepseek_v2.py
@@ -0,0 +1,308 @@
+# Copyright (c) OpenMMLab. All rights reserved.
+import warnings
+from typing import Optional
+
+import torch
+import torch.distributed as dist
+import torch.nn.functional as F
+from mmengine import MessageHub
+from transformers.cache_utils import Cache
+
+from xtuner.model.transformers_models.deepseek_v2.modeling_deepseek import \
+ apply_rotary_pos_emb
+from xtuner.parallel.sequence import (get_sequence_parallel_world_size,
+ post_process_for_sequence_parallel_attn,
+ pre_process_for_sequence_parallel_attn)
+from .attention import flash_attn_wo_mask, varlen_flash_attn
+
+
+def deepseek_attn_forward(
+ self,
+ hidden_states: torch.Tensor,
+ attention_mask: Optional[torch.LongTensor] = None,
+ position_ids: Optional[torch.LongTensor] = None,
+ past_key_value: Optional[Cache] = None,
+ output_attentions: bool = False,
+ use_cache: bool = False,
+ **kwargs,
+):
+ # DeepseekV2FlashAttention2 attention does not support output_attentions
+ if 'padding_mask' in kwargs:
+ warnings.warn(
+ 'Passing `padding_mask` is deprecated and will be removed in '
+ 'v4.37. Please make sure use `attention_mask` instead.`')
+
+ # overwrite attention_mask with padding_mask
+ attention_mask = kwargs.pop('padding_mask')
+
+ output_attentions = False
+
+ bsz, q_len, _ = hidden_states.size()
+
+ if self.q_lora_rank is None:
+ q = self.q_proj(hidden_states)
+ else:
+ q = self.q_b_proj(self.q_a_layernorm(self.q_a_proj(hidden_states)))
+ q = q.view(bsz, q_len, self.num_heads, self.q_head_dim).transpose(1, 2)
+ q_nope, q_pe = torch.split(
+ q, [self.qk_nope_head_dim, self.qk_rope_head_dim], dim=-1)
+
+ # Flash attention requires the input to have the shape
+ # batch_size x seq_length x head_dim x hidden_dim
+ # therefore we just need to keep the original shape
+ compressed_kv = self.kv_a_proj_with_mqa(hidden_states)
+ compressed_kv, k_pe = torch.split(
+ compressed_kv, [self.kv_lora_rank, self.qk_rope_head_dim], dim=-1)
+ k_pe = k_pe.view(bsz, q_len, 1, self.qk_rope_head_dim).transpose(1, 2)
+ kv = (
+ self.kv_b_proj(self.kv_a_layernorm(compressed_kv)).view(
+ bsz, q_len, self.num_heads,
+ self.qk_nope_head_dim + self.v_head_dim).transpose(1, 2))
+
+ k_nope, value_states = torch.split(
+ kv, [self.qk_nope_head_dim, self.v_head_dim], dim=-1)
+ kv_seq_len = value_states.shape[-2]
+
+ kv_seq_len = value_states.shape[-2]
+ if past_key_value is not None:
+ kv_seq_len += past_key_value.get_usable_length(kv_seq_len,
+ self.layer_idx)
+
+ assert position_ids is not None, '`position_ids` should not be None.'
+ if self.training:
+ cos, sin = self.rotary_emb(
+ value_states, seq_len=position_ids.max() + 1)
+ else:
+ cos, sin = self.rotary_emb(value_states, seq_len=kv_seq_len)
+ q_pe, k_pe = apply_rotary_pos_emb(q_pe, k_pe, cos, sin, position_ids)
+
+ query_states = k_pe.new_empty(bsz, self.num_heads, q_len, self.q_head_dim)
+ query_states[:, :, :, :self.qk_nope_head_dim] = q_nope
+ query_states[:, :, :, self.qk_nope_head_dim:] = q_pe
+
+ key_states = k_pe.new_empty(bsz, self.num_heads, q_len, self.q_head_dim)
+ key_states[:, :, :, :self.qk_nope_head_dim] = k_nope
+ key_states[:, :, :, self.qk_nope_head_dim:] = k_pe
+
+ if self.q_head_dim != self.v_head_dim:
+ value_states = F.pad(value_states,
+ [0, self.q_head_dim - self.v_head_dim])
+
+ if past_key_value is not None:
+ cache_kwargs = {'sin': sin, 'cos': cos} # Specific to RoPE models
+ key_states, value_states = past_key_value.update(
+ key_states, value_states, self.layer_idx, cache_kwargs)
+
+ # Reashape to the expected shape for Flash Attention
+ query_states = query_states.transpose(1, 2)
+ key_states = key_states.transpose(1, 2)
+ value_states = value_states.transpose(1, 2)
+
+ dropout_rate = self.attention_dropout if self.training else 0.0
+
+ # In PEFT, usually we cast the layer norms in float32 for training
+ # stability reasons therefore the input hidden states gets silently
+ # casted in float32. Hence, we need cast them back in the correct dtype
+ # just to be sure everything works as expected.
+ # This might slowdown training & inference so it is recommended to not
+ # cast the LayerNorms in fp32. (DeepseekV2RMSNorm handles it correctly)
+
+ input_dtype = query_states.dtype
+ if input_dtype == torch.float32:
+ # Handle the case where the model is quantized
+ if hasattr(self.config, '_pre_quantization_dtype'):
+ target_dtype = self.config._pre_quantization_dtype
+ elif torch.is_autocast_enabled():
+ target_dtype = torch.get_autocast_gpu_dtype()
+ else:
+ target_dtype = self.q_a_proj.weight.dtype
+
+ query_states = query_states.to(target_dtype)
+ key_states = key_states.to(target_dtype)
+ value_states = value_states.to(target_dtype)
+
+ enable_sequence_parallel = (
+ dist.is_initialized() and get_sequence_parallel_world_size() > 1
+ and self.training)
+ if enable_sequence_parallel:
+ query_states, key_states, value_states = \
+ pre_process_for_sequence_parallel_attn(
+ query_states, key_states, value_states)
+ # self.num_heads is used in self._upad_input method
+ # num_heads has been changed because of sequence parallel
+ ori_num_head = self.num_heads
+ self.num_heads = query_states.shape[-2]
+
+ attn_output = self._flash_attention_forward(
+ query_states,
+ key_states,
+ value_states,
+ attention_mask,
+ query_states.shape[1],
+ dropout=dropout_rate,
+ softmax_scale=self.softmax_scale,
+ )
+
+ if enable_sequence_parallel:
+ attn_output = post_process_for_sequence_parallel_attn(attn_output)
+ self.num_heads = ori_num_head
+
+ if self.q_head_dim != self.v_head_dim:
+ attn_output = attn_output[:, :, :, :self.v_head_dim]
+
+ attn_output = attn_output.reshape(bsz, q_len, self.num_heads *
+ self.v_head_dim).contiguous()
+ attn_output = self.o_proj(attn_output)
+
+ if not output_attentions:
+ attn_weights = None
+
+ return attn_output, attn_weights, past_key_value
+
+
+def deepseek_varlen_attn_forward(
+ self,
+ hidden_states: torch.Tensor,
+ attention_mask: Optional[torch.LongTensor] = None,
+ position_ids: Optional[torch.LongTensor] = None,
+ past_key_value: Optional[Cache] = None,
+ output_attentions: bool = False,
+ use_cache: bool = False,
+ **kwargs,
+):
+ is_training = self.training
+
+ message_hub = MessageHub.get_instance('varlen_attn_args')
+ rank = dist.get_rank()
+ cumulative_len = message_hub.get_info(f'cumulative_len_rank_{rank}')
+ max_seqlen = message_hub.get_info(f'max_seqlen_rank_{rank}')
+
+ assert is_training == (cumulative_len is not None) == (
+ past_key_value is None)
+
+ output_attentions = False
+
+ bsz, q_len, _ = hidden_states.size()
+
+ if self.q_lora_rank is None:
+ q = self.q_proj(hidden_states)
+ else:
+ q = self.q_b_proj(self.q_a_layernorm(self.q_a_proj(hidden_states)))
+ q = q.view(bsz, q_len, self.num_heads, self.q_head_dim).transpose(1, 2)
+ q_nope, q_pe = torch.split(
+ q, [self.qk_nope_head_dim, self.qk_rope_head_dim], dim=-1)
+
+ # Flash attention requires the input to have the shape
+ # batch_size x seq_length x head_dim x hidden_dim
+ # therefore we just need to keep the original shape
+ compressed_kv = self.kv_a_proj_with_mqa(hidden_states)
+ compressed_kv, k_pe = torch.split(
+ compressed_kv, [self.kv_lora_rank, self.qk_rope_head_dim], dim=-1)
+ k_pe = k_pe.view(bsz, q_len, 1, self.qk_rope_head_dim).transpose(1, 2)
+ kv = (
+ self.kv_b_proj(self.kv_a_layernorm(compressed_kv)).view(
+ bsz, q_len, self.num_heads,
+ self.qk_nope_head_dim + self.v_head_dim).transpose(1, 2))
+
+ k_nope, value_states = torch.split(
+ kv, [self.qk_nope_head_dim, self.v_head_dim], dim=-1)
+ kv_seq_len = value_states.shape[-2]
+
+ kv_seq_len = value_states.shape[-2]
+ if past_key_value is not None:
+ kv_seq_len += past_key_value.get_usable_length(kv_seq_len,
+ self.layer_idx)
+
+ assert position_ids is not None, '`position_ids` should not be None.'
+ if self.training:
+ cos, sin = self.rotary_emb(
+ value_states, seq_len=position_ids.max() + 1)
+ else:
+ cos, sin = self.rotary_emb(value_states, seq_len=kv_seq_len)
+ q_pe, k_pe = apply_rotary_pos_emb(q_pe, k_pe, cos, sin, position_ids)
+
+ query_states = k_pe.new_empty(bsz, self.num_heads, q_len, self.q_head_dim)
+ query_states[:, :, :, :self.qk_nope_head_dim] = q_nope
+ query_states[:, :, :, self.qk_nope_head_dim:] = q_pe
+
+ key_states = k_pe.new_empty(bsz, self.num_heads, q_len, self.q_head_dim)
+ key_states[:, :, :, :self.qk_nope_head_dim] = k_nope
+ key_states[:, :, :, self.qk_nope_head_dim:] = k_pe
+
+ if self.q_head_dim != self.v_head_dim:
+ value_states = F.pad(value_states,
+ [0, self.q_head_dim - self.v_head_dim])
+
+ if past_key_value is not None:
+ cache_kwargs = {'sin': sin, 'cos': cos} # Specific to RoPE models
+ key_states, value_states = past_key_value.update(
+ key_states, value_states, self.layer_idx, cache_kwargs)
+
+ # In PEFT, usually we cast the layer norms in float32 for training
+ # stability reasons therefore the input hidden states gets silently
+ # casted in float32. Hence, we need cast them back in the correct dtype
+ # just to be sure everything works as expected.
+ # This might slowdown training & inference so it is recommended to not
+ # cast the LayerNorms in fp32. (DeepseekV2RMSNorm handles it correctly)
+
+ input_dtype = query_states.dtype
+ if input_dtype == torch.float32:
+ # Handle the case where the model is quantized
+ if hasattr(self.config, '_pre_quantization_dtype'):
+ target_dtype = self.config._pre_quantization_dtype
+ elif torch.is_autocast_enabled():
+ target_dtype = torch.get_autocast_gpu_dtype()
+ else:
+ target_dtype = self.q_a_proj.weight.dtype
+
+ query_states = query_states.to(target_dtype)
+ key_states = key_states.to(target_dtype)
+ value_states = value_states.to(target_dtype)
+
+ # Reashape to the expected shape for Flash Attention
+ query_states = query_states.transpose(1, 2)
+ key_states = key_states.transpose(1, 2)
+ value_states = value_states.transpose(1, 2)
+
+ # ----------------- varlen flash attention forward ----------------------#
+ dropout_rate = self.attention_dropout if self.training else 0.0
+
+ if not self._flash_attn_uses_top_left_mask:
+ causal = self.is_causal
+ else:
+ causal = self.is_causal and q_len != 1
+
+ if is_training:
+ attn_output = varlen_flash_attn(
+ query_states,
+ key_states,
+ value_states,
+ cumulative_len,
+ max_seqlen,
+ softmax_scale=self.softmax_scale,
+ causal=causal,
+ dropout_p=dropout_rate,
+ training=True)
+ else:
+ attn_output = flash_attn_wo_mask(
+ query_states,
+ key_states,
+ value_states,
+ softmax_scale=self.softmax_scale,
+ causal=causal,
+ dropout_p=dropout_rate,
+ training=False)
+
+ # ---------------- varlen flash attention forward end ------------------ #
+
+ if self.q_head_dim != self.v_head_dim:
+ attn_output = attn_output[:, :, :, :self.v_head_dim]
+
+ attn_output = attn_output.reshape(bsz, q_len,
+ self.num_heads * self.v_head_dim)
+ attn_output = self.o_proj(attn_output)
+
+ if not output_attentions:
+ attn_weights = None
+
+ return attn_output, attn_weights, past_key_value
diff --git a/xtuner/model/modules/dispatch/internlm.py b/xtuner/model/modules/dispatch/internlm.py
index fd06def33..37ca9ad31 100644
--- a/xtuner/model/modules/dispatch/internlm.py
+++ b/xtuner/model/modules/dispatch/internlm.py
@@ -149,14 +149,12 @@ def internlm_varlen_attn_forward(
Optional[Tuple[torch.Tensor]]]:
# Modified from https://huggingface.co/internlm/internlm-7b/blob/939a68c0dc1bd5f35b63c87d44af05ce33379061/modeling_internlm.py#L161 # noqa:E501
- is_training = self.training
-
message_hub = MessageHub.get_instance('varlen_attn_args')
rank = dist.get_rank()
cumulative_len = message_hub.get_info(f'cumulative_len_rank_{rank}')
# position_ids = message_hub.get_info(f'position_ids_rank_{rank}')
max_seqlen = message_hub.get_info(f'max_seqlen_rank_{rank}')
- assert is_training == (cumulative_len is not None)
+ use_varlen_atten = (cumulative_len is not None)
bsz, q_len, _ = hidden_states.size()
assert bsz == 1, (f'If utilizing local attention, the batch size should be'
@@ -173,7 +171,7 @@ def internlm_varlen_attn_forward(
if past_key_value is not None:
kv_seq_len += past_key_value[0].shape[-2]
- if is_training:
+ if use_varlen_atten:
cos, sin = self.rotary_emb(value_states, max_seqlen)
query_states = apply_rotary_emb(query_states,
cos[position_ids].squeeze(0),
@@ -199,7 +197,7 @@ def internlm_varlen_attn_forward(
value_states = value_states.transpose(1, 2)
assert SUPPORT_FLASH2
- if is_training:
+ if use_varlen_atten:
q_unpad, k_unpad, v_unpad = query_states.flatten(
0, 1), key_states.flatten(0, 1), value_states.flatten(0, 1)
cumulative_len = torch.cat(cumulative_len, dim=0)
diff --git a/xtuner/model/modules/dispatch/internlm2.py b/xtuner/model/modules/dispatch/internlm2.py
index 54e12e16f..7c601f0dc 100644
--- a/xtuner/model/modules/dispatch/internlm2.py
+++ b/xtuner/model/modules/dispatch/internlm2.py
@@ -1,71 +1,16 @@
# Copyright (c) OpenMMLab. All rights reserved.
-import warnings
from typing import Optional, Tuple
import torch
import torch.distributed as dist
-import torch.nn.functional as F
from einops import rearrange
from mmengine import MessageHub
+from transformers.cache_utils import Cache, StaticCache
-from xtuner.parallel.sequence import sequence_parallel_wrapper
-from .triton_kernels import apply_rotary_emb
-from .utils import upad_qkv
-
-SUPPORT_FLASH2 = False
-
-try:
- from flash_attn import flash_attn_func, flash_attn_varlen_func
- from flash_attn.bert_padding import pad_input
- SUPPORT_FLASH2 = True
-except ImportError:
- pass
-
-
-class InternLM2RotaryEmbedding(torch.nn.Module):
-
- def __init__(self,
- dim,
- max_position_embeddings=2048,
- base=1000000,
- device=None):
- super().__init__()
- self.dim = dim
- self.max_position_embeddings = max_position_embeddings
- self.base = base
- self.inv_freq = 1.0 / (
- base**(torch.arange(0, dim, 2).float().to(device) / dim))
-
- # Build here to make `torch.jit.trace` work.
- self.max_seq_len_cached = max_position_embeddings
- t = torch.arange(
- self.max_seq_len_cached,
- device=self.inv_freq.device,
- dtype=self.inv_freq.dtype)
- freqs = torch.einsum('i,j->ij', t, self.inv_freq)
- emb = torch.cat((freqs, freqs), dim=-1)
- self.cos_cached = emb.cos()
- self.sin_cached = emb.sin()
-
- def forward(self, x, seq_len):
- # x: [bs, num_attention_heads, seq_len, head_size]
- if (seq_len > self.max_seq_len_cached
- or self.cos_cached.device != x.device
- or self.cos_cached.dtype != x.dtype):
- self.max_seq_len_cached = seq_len
- assert self.inv_freq.dtype == torch.float32
- t = torch.arange(
- self.max_seq_len_cached,
- device=x.device,
- dtype=self.inv_freq.dtype)
- freqs = torch.einsum('i,j->ij', t, self.inv_freq.to(t.device))
- emb = torch.cat((freqs, freqs), dim=-1).to(x.device)
- self.cos_cached = emb.cos().to(x.dtype)
- self.sin_cached = emb.sin().to(x.dtype)
- return (
- self.cos_cached[:seq_len, ...],
- self.sin_cached[:seq_len, ...],
- )
+from xtuner.parallel.sequence import (get_sequence_parallel_world_size,
+ post_process_for_sequence_parallel_attn,
+ pre_process_for_sequence_parallel_attn)
+from .attention import SUPPORT_FLASH2, flash_attn_wo_mask, varlen_flash_attn
def rotate_half(x):
@@ -75,9 +20,9 @@ def rotate_half(x):
return torch.cat((-x2, x1), dim=-1)
-def apply_rotary_pos_emb(q, k, cos, sin, position_ids, unsqueeze_dim=1):
- cos = cos[position_ids].unsqueeze(unsqueeze_dim)
- sin = sin[position_ids].unsqueeze(unsqueeze_dim)
+def apply_rotary_pos_emb(q, k, cos, sin, position_ids=None, unsqueeze_dim=1):
+ cos = cos.unsqueeze(unsqueeze_dim)
+ sin = sin.unsqueeze(unsqueeze_dim)
q_embed = (q * cos) + (rotate_half(q) * sin)
k_embed = (k * cos) + (rotate_half(k) * sin)
return q_embed, k_embed
@@ -115,85 +60,22 @@ def repeat_kv_bshd(hidden_states: torch.Tensor, n_rep: int) -> torch.Tensor:
head_dim)
-@sequence_parallel_wrapper
-def flash_attn_wo_mask(query_states,
- key_states,
- value_states,
- causal,
- dropout_rate=0.0):
- attn_output = flash_attn_func(
- query_states, key_states, value_states, dropout_rate, causal=causal)
- return attn_output
-
-
-@sequence_parallel_wrapper
-def flash_attn_w_mask(
- query_states, # bs, q_len, nhead, h_dim
- key_states,
- value_states,
- attention_mask,
- causal,
- dropout_rate=0.0):
- batch_size, q_len = query_states.shape[:2]
- query_states, key_states, value_states, indices_q, \
- cu_seq_lens, max_seq_lens = upad_qkv(
- query_states, key_states, value_states, attention_mask, q_len)
-
- cu_seqlens_q, cu_seqlens_k = cu_seq_lens
- max_seqlen_in_batch_q, max_seqlen_in_batch_k = max_seq_lens
- attn_output_unpad = flash_attn_varlen_func(
- query_states,
- key_states,
- value_states,
- cu_seqlens_q=cu_seqlens_q,
- cu_seqlens_k=cu_seqlens_k,
- max_seqlen_q=max_seqlen_in_batch_q,
- max_seqlen_k=max_seqlen_in_batch_k,
- dropout_p=dropout_rate,
- causal=causal,
- )
- attn_output = pad_input(attn_output_unpad, indices_q, batch_size, q_len)
- return attn_output
-
-
-@sequence_parallel_wrapper
-def varlen_flash_attn(query_states, key_states, value_states, cumulative_len,
- max_seqlen):
- q_unpad, k_unpad, v_unpad = query_states.flatten(0, 1), key_states.flatten(
- 0, 1), value_states.flatten(0, 1)
- attn_output = flash_attn_varlen_func(
- q_unpad,
- k_unpad,
- v_unpad,
- cumulative_len,
- cumulative_len,
- max_seqlen,
- max_seqlen,
- 0,
- return_attn_probs=False,
- causal=True,
- )
- attn_output = attn_output.unsqueeze(0)
- return attn_output
-
-
def internlm2_attn_forward(
self,
hidden_states: torch.Tensor,
attention_mask: Optional[torch.LongTensor] = None,
position_ids: Optional[torch.LongTensor] = None,
- past_key_value: Optional[Tuple[torch.Tensor]] = None,
+ past_key_value: Optional[Cache] = None,
output_attentions: bool = False,
use_cache: bool = False,
- **kwargs,
+ cache_position: Optional[torch.LongTensor] = None,
):
- if 'padding_mask' in kwargs:
- warnings.warn(
- 'Passing `padding_mask` is deprecated and will be removed in v4.37'
- 'Please make sure use `attention_mask` instead.`')
-
- # overwrite attention_mask with padding_mask
- attention_mask = kwargs.pop('padding_mask')
+ if isinstance(past_key_value, StaticCache):
+ raise ValueError(
+ '`static` cache implementation is not compatible with '
+ '`attn_implementation==flash_attention_2` make sure to use `sdpa` '
+ 'in the mean time, and open an issue at '
+ 'https://github.com/huggingface/transformers')
output_attentions = False
@@ -217,64 +99,73 @@ def internlm2_attn_forward(
key_states = key_states.transpose(1, 2)
value_states = value_states.transpose(1, 2)
- kv_seq_len = key_states.shape[-2]
- if past_key_value is not None:
- kv_seq_len += past_key_value[0].shape[-2]
-
- # This modification is necessary for sequential parallel
- assert position_ids is not None and (position_ids.max() + 1) >= kv_seq_len
- cos, sin = self.rotary_emb(value_states, seq_len=position_ids.max() + 1)
+ cos, sin = self.rotary_emb(value_states, position_ids)
query_states, key_states = apply_rotary_pos_emb(query_states, key_states,
- cos, sin, position_ids)
+ cos, sin)
if past_key_value is not None:
- # reuse k, v, self_attention
- key_states = torch.cat([past_key_value[0], key_states], dim=2)
- value_states = torch.cat([past_key_value[1], value_states], dim=2)
+ # sin and cos are specific to RoPE models;
+ # cache_position needed for the static cache
+ cache_kwargs = {
+ 'sin': sin,
+ 'cos': cos,
+ 'cache_position': cache_position
+ }
+ key_states, value_states = past_key_value.update(
+ key_states, value_states, self.layer_idx, cache_kwargs)
- past_key_value = (key_states, value_states) if use_cache else None
-
- # repeat kv for sequence parallel
key_states = repeat_kv(key_states, self.num_key_value_groups)
value_states = repeat_kv(value_states, self.num_key_value_groups)
- if SUPPORT_FLASH2:
- # the shape of attention_mask used by flash_attn and
- # F.scaled_dot_product_attention are different
- assert attention_mask is None or attention_mask.ndim == 2, \
- ('When using flash_attn, attention_mask.ndim should equal to 2.'
- f'But got attention_mask.shape = {attention_mask.shape}.'
- 'We can pass the `attn_implementation="flash_attention_2"` flag '
- 'to `.from_pretrained` method when instantiating a Internlm2 '
- 'model.')
- # flash attn 2 need (bs, seq_len, nhead, h_dim)
- query_states = query_states.transpose(1, 2)
- key_states = key_states.transpose(1, 2)
- value_states = value_states.transpose(1, 2)
-
- causal = self.is_causal and q_len != 1
-
- if attention_mask is not None:
- attn_output = flash_attn_w_mask(
- query_states,
- key_states,
- value_states,
- attention_mask,
- causal,
- training=self.training)
+ query_states = query_states.transpose(1, 2)
+ key_states = key_states.transpose(1, 2)
+ value_states = value_states.transpose(1, 2)
+
+ # In PEFT, usually we cast the layer norms in float32 for training
+ # stability reasons therefore the input hidden states gets silently
+ # casted in float32. Hence, we need cast them back in the correct dtype
+ # just to be sure everything works as expected.
+ # This might slowdown training & inference so it is recommended to not
+ # cast the LayerNorms in fp32. (InternLM2RMSNorm handles it correctly)
+
+ input_dtype = query_states.dtype
+ if input_dtype == torch.float32:
+ if torch.is_autocast_enabled():
+ target_dtype = torch.get_autocast_gpu_dtype()
+ # Handle the case where the model is quantized
+ elif hasattr(self.config, '_pre_quantization_dtype'):
+ target_dtype = self.config._pre_quantization_dtype
else:
- attn_output = flash_attn_wo_mask(
- query_states,
- key_states,
- value_states,
- causal,
- training=self.training)
- else:
- # use flash attention implemented by pytorch
- # do not support sequence parallel
- attn_output = F.scaled_dot_product_attention(
- query_states, key_states, value_states, attn_mask=attention_mask)
- attn_output = attn_output.transpose(1, 2)
+ target_dtype = self.wqkv.weight.dtype
+
+ query_states = query_states.to(target_dtype)
+ key_states = key_states.to(target_dtype)
+ value_states = value_states.to(target_dtype)
+
+ enable_sequence_parallel = (
+ dist.is_initialized() and get_sequence_parallel_world_size() > 1
+ and self.training)
+ if enable_sequence_parallel:
+ query_states, key_states, value_states = \
+ pre_process_for_sequence_parallel_attn(
+ query_states, key_states, value_states)
+ # self.num_heads is used in self._upad_input method
+ # num_heads has been changed because of sequence parallel
+ ori_num_head = self.num_heads
+ self.num_heads = query_states.shape[-2]
+
+ dropout_rate = 0.0
+ attn_output = self._flash_attention_forward(
+ query_states,
+ key_states,
+ value_states,
+ attention_mask,
+ query_states.shape[1],
+ dropout=dropout_rate)
+
+ if enable_sequence_parallel:
+ attn_output = post_process_for_sequence_parallel_attn(attn_output)
+ self.num_heads = ori_num_head
attn_output = attn_output.reshape(bsz, q_len, self.hidden_size)
attn_output = self.wo(attn_output)
@@ -288,22 +179,27 @@ def internlm2_attn_forward(
def internlm2_varlen_attn_forward(
self,
hidden_states: torch.Tensor,
- attention_mask: Optional[torch.Tensor] = None,
+ attention_mask: Optional[torch.LongTensor] = None,
position_ids: Optional[torch.LongTensor] = None,
- past_key_value: Optional[Tuple[torch.Tensor]] = None,
+ past_key_value: Optional[Cache] = None,
output_attentions: bool = False,
use_cache: bool = False,
+ cache_position: Optional[torch.LongTensor] = None,
) -> Tuple[torch.Tensor, Optional[torch.Tensor],
Optional[Tuple[torch.Tensor]]]:
- # Modified from https://huggingface.co/internlm/internlm-7b/blob/939a68c0dc1bd5f35b63c87d44af05ce33379061/modeling_internlm.py#L161 # noqa:E501
- is_training = self.training
+ if isinstance(past_key_value, StaticCache):
+ raise ValueError(
+ '`static` cache implementation is not compatible with '
+ '`attn_implementation==flash_attention_2` make sure to use `sdpa` '
+ 'in the mean time, and open an issue at '
+ 'https://github.com/huggingface/transformers')
message_hub = MessageHub.get_instance('varlen_attn_args')
rank = dist.get_rank()
cumulative_len = message_hub.get_info(f'cumulative_len_rank_{rank}')
max_seqlen = message_hub.get_info(f'max_seqlen_rank_{rank}')
- assert is_training == (cumulative_len is not None)
+ use_varlen_atten = (cumulative_len is not None)
bsz, q_len, _ = hidden_states.size()
@@ -311,6 +207,7 @@ def internlm2_varlen_attn_forward(
f' set to 1, but got {bsz}')
qkv_states = self.wqkv(hidden_states)
+
qkv_states = rearrange(
qkv_states,
'b q (h gs d) -> b q h gs d',
@@ -323,50 +220,81 @@ def internlm2_varlen_attn_forward(
key_states = qkv_states[..., -2, :]
value_states = qkv_states[..., -1, :]
- kv_seq_len = key_states.shape[-3]
+ query_states = query_states.transpose(1, 2)
+ key_states = key_states.transpose(1, 2)
+ value_states = value_states.transpose(1, 2)
+
+ try:
+ cos, sin = self.rotary_emb(value_states, position_ids)
+ except RuntimeError:
+ raise RuntimeError(
+ 'You are using the old version of InternLM2 model. The '
+ '`modeling_internlm2.py` is outdated. Please update the InternLM2 '
+ 'model.')
+ query_states, key_states = apply_rotary_pos_emb(query_states, key_states,
+ cos, sin)
+
if past_key_value is not None:
- kv_seq_len += past_key_value[0].shape[-2]
-
- if is_training:
- cos, sin = self.rotary_emb(value_states, max_seqlen)
- query_states = apply_rotary_emb(query_states,
- cos[position_ids].squeeze(0),
- sin[position_ids].squeeze(0))
- key_states = apply_rotary_emb(key_states, cos[position_ids].squeeze(0),
- sin[position_ids].squeeze(0))
- else:
- query_states = query_states.transpose(1, 2)
- key_states = key_states.transpose(1, 2)
- value_states = value_states.transpose(1, 2)
- cos, sin = self.rotary_emb(value_states, kv_seq_len)
- query_states, key_states = apply_rotary_pos_emb(
- query_states, key_states, cos, sin, position_ids)
-
- if past_key_value is not None:
- # reuse k, v, self_attention
- key_states = torch.cat([past_key_value[0], key_states], dim=2)
- value_states = torch.cat([past_key_value[1], value_states], dim=2)
-
- past_key_value = (key_states, value_states) if use_cache else None
- query_states = query_states.transpose(1, 2)
- key_states = key_states.transpose(1, 2)
- value_states = value_states.transpose(1, 2)
+ # sin and cos are specific to RoPE models;
+ # cache_position needed for the static cache
+ cache_kwargs = {
+ 'sin': sin,
+ 'cos': cos,
+ 'cache_position': cache_position
+ }
+ key_states, value_states = past_key_value.update(
+ key_states, value_states, self.layer_idx, cache_kwargs)
+
+ query_states = query_states.transpose(1, 2)
+ key_states = key_states.transpose(1, 2)
+ value_states = value_states.transpose(1, 2)
+
+ # In PEFT, usually we cast the layer norms in float32 for training
+ # stability reasons therefore the input hidden states gets silently
+ # casted in float32. Hence, we need cast them back in the correct dtype
+ # just to be sure everything works as expected.
+ # This might slowdown training & inference so it is recommended to not
+ # cast the LayerNorms in fp32. (InternLM2RMSNorm handles it correctly)
+
+ input_dtype = query_states.dtype
+ if input_dtype == torch.float32:
+ if torch.is_autocast_enabled():
+ target_dtype = torch.get_autocast_gpu_dtype()
+ # Handle the case where the model is quantized
+ elif hasattr(self.config, '_pre_quantization_dtype'):
+ target_dtype = self.config._pre_quantization_dtype
+ else:
+ target_dtype = self.wqkv.weight.dtype
+
+ query_states = query_states.to(target_dtype)
+ key_states = key_states.to(target_dtype)
+ value_states = value_states.to(target_dtype)
# repeat kv for sequence parallel
key_states = repeat_kv_bshd(key_states, self.num_key_value_groups)
value_states = repeat_kv_bshd(value_states, self.num_key_value_groups)
assert SUPPORT_FLASH2
- if is_training:
- attn_output = varlen_flash_attn(query_states, key_states, value_states,
- cumulative_len, max_seqlen)
+
+ dropout_rate = 0.0
+ if use_varlen_atten:
+ attn_output = varlen_flash_attn(
+ query_states,
+ key_states,
+ value_states,
+ cumulative_len,
+ max_seqlen,
+ causal=True,
+ dropout_p=dropout_rate,
+ training=self.training)
else:
attn_output = flash_attn_wo_mask(
query_states,
key_states,
value_states,
causal=True,
- training=False)
+ dropout_p=dropout_rate,
+ training=self.training)
attn_output = attn_output.reshape(bsz, q_len, self.hidden_size)
diff --git a/xtuner/model/modules/dispatch/internlm3.py b/xtuner/model/modules/dispatch/internlm3.py
new file mode 100644
index 000000000..0532bb0ae
--- /dev/null
+++ b/xtuner/model/modules/dispatch/internlm3.py
@@ -0,0 +1,132 @@
+# Copyright (c) OpenMMLab. All rights reserved.
+import warnings
+from typing import Callable, Optional, Tuple
+
+import torch
+import torch.distributed as dist
+from mmengine import MessageHub
+from transformers.cache_utils import Cache
+from transformers.modeling_flash_attention_utils import FlashAttentionKwargs
+from transformers.modeling_utils import ALL_ATTENTION_FUNCTIONS
+from transformers.models.llama.modeling_llama import (apply_rotary_pos_emb,
+ eager_attention_forward,
+ repeat_kv)
+from transformers.processing_utils import Unpack
+
+from xtuner.parallel.sequence import get_sequence_parallel_world_size
+from xtuner.parallel.sequence.attention import (
+ post_process_for_sequence_parallel_attn,
+ pre_process_for_sequence_parallel_attn)
+
+
+def internlm3_attn_forward(
+ self,
+ hidden_states: torch.Tensor,
+ position_embeddings: Tuple[torch.Tensor, torch.Tensor],
+ attention_mask: Optional[torch.Tensor],
+ past_key_value: Optional[Cache] = None,
+ cache_position: Optional[torch.LongTensor] = None,
+ **kwargs: Unpack[FlashAttentionKwargs],
+):
+ input_shape = hidden_states.shape[:-1]
+ hidden_shape = (*input_shape, -1, self.head_dim)
+
+ query_states = self.q_proj(hidden_states).view(hidden_shape).transpose(
+ 1, 2)
+ key_states = self.k_proj(hidden_states).view(hidden_shape).transpose(1, 2)
+ value_states = self.v_proj(hidden_states).view(hidden_shape).transpose(
+ 1, 2)
+
+ cos, sin = position_embeddings
+ query_states, key_states = apply_rotary_pos_emb(query_states, key_states,
+ cos, sin)
+
+ if past_key_value is not None:
+ # sin and cos are specific to RoPE models; cache_position needed
+ # for the static cache
+ cache_kwargs = {
+ 'sin': sin,
+ 'cos': cos,
+ 'cache_position': cache_position
+ }
+ key_states, value_states = past_key_value.update(
+ key_states, value_states, self.layer_idx, cache_kwargs)
+
+ # different from LlamaAttention.forward
+ # repeat k/v heads if n_kv_heads < n_heads for sequence parallel
+ key_states = repeat_kv(key_states, self.num_key_value_groups)
+ value_states = repeat_kv(value_states, self.num_key_value_groups)
+
+ enable_sequence_parallel = (
+ dist.is_initialized() and get_sequence_parallel_world_size() > 1
+ and self.training)
+ if enable_sequence_parallel:
+ # Reashape for `pre_process_for_sequence_parallel_attn`
+ query_states = query_states.transpose(1, 2)
+ key_states = key_states.transpose(1, 2)
+ value_states = value_states.transpose(1, 2)
+ query_states, key_states, value_states = \
+ pre_process_for_sequence_parallel_attn(
+ query_states, key_states, value_states)
+ query_states = query_states.transpose(1, 2)
+ key_states = key_states.transpose(1, 2)
+ value_states = value_states.transpose(1, 2)
+ # different places end
+
+ attention_interface: Callable = eager_attention_forward
+ if self.config._attn_implementation != 'eager':
+ if self.config._attn_implementation == 'sdpa' and kwargs.get(
+ 'output_attentions', False):
+ warnings.warn(
+ '`torch.nn.functional.scaled_dot_product_attention` does not '
+ 'support `output_attentions=True`. Falling back to eager '
+ 'attention. This warning can be removed using the argument'
+ ' `attn_implementation="eager"` when loading the model.')
+ else:
+ attention_interface = ALL_ATTENTION_FUNCTIONS[
+ self.config._attn_implementation]
+
+ message_hub = MessageHub.get_instance('varlen_attn_args')
+ rank = dist.get_rank()
+ cumulative_len = message_hub.get_info(f'cumulative_len_rank_{rank}')
+ use_varlen_atten = (cumulative_len is not None)
+ if use_varlen_atten:
+ # When gradient_checkpointing is enabled, the flash_attn_kwargs
+ # parameter is not automatically passed to the model. In such
+ # cases, parameters like cu_seq_lens_q and max_length_q are
+ # computed based on position_ids. However, when sequence
+ # parallel is enabled, position_ids is split along the
+ # sequence length, leading to incorrect calculations of these
+ # parameters.
+ # To address this issue, it is necessary to manually provide
+ # the flash_attn_kwargs parameters.
+ max_seqlen = message_hub.get_info(f'max_seqlen_rank_{rank}')
+ kwargs['cu_seq_lens_q'] = cumulative_len
+ kwargs['cu_seq_lens_k'] = cumulative_len
+ kwargs['max_length_q'] = max_seqlen
+ kwargs['max_length_k'] = max_seqlen
+ kwargs.pop('position_ids', None)
+
+ # Hacky: `sdpa_attention_forward` does repeat_kv based on
+ # module.num_key_value_groups but it is done before
+ num_key_value_groups = self.num_key_value_groups
+ self.num_key_value_groups = 1
+ attn_output, attn_weights = attention_interface(
+ self,
+ query_states,
+ key_states,
+ value_states,
+ attention_mask,
+ dropout=0.0 if not self.training else self.attention_dropout,
+ scaling=self.scaling,
+ **kwargs,
+ )
+ self.num_key_value_groups = num_key_value_groups
+
+ # different from LlamaAttention.forward
+ if enable_sequence_parallel:
+ attn_output = post_process_for_sequence_parallel_attn(attn_output)
+
+ attn_output = attn_output.reshape(*input_shape, -1).contiguous()
+ attn_output = self.o_proj(attn_output)
+ return attn_output, attn_weights
diff --git a/xtuner/model/modules/dispatch/llama.py b/xtuner/model/modules/dispatch/llama.py
index df10159d1..a81dff790 100644
--- a/xtuner/model/modules/dispatch/llama.py
+++ b/xtuner/model/modules/dispatch/llama.py
@@ -1,154 +1,51 @@
# Copyright (c) OpenMMLab. All rights reserved.
import warnings
-from typing import Optional, Tuple
+from typing import Callable, Optional, Tuple
import torch
import torch.distributed as dist
from mmengine import MessageHub
+from transformers.cache_utils import Cache
+from transformers.modeling_flash_attention_utils import FlashAttentionKwargs
+from transformers.modeling_utils import ALL_ATTENTION_FUNCTIONS
from transformers.models.llama.modeling_llama import (apply_rotary_pos_emb,
+ eager_attention_forward,
repeat_kv)
-from transformers.utils import is_flash_attn_greater_or_equal_2_10
+from transformers.processing_utils import Unpack
-from xtuner.parallel.sequence import sequence_parallel_wrapper
-from .triton_kernels import apply_rotary_emb
-from .utils import upad_qkv
-
-SUPPORT_FLASH2 = False
-
-try:
- from flash_attn import flash_attn_func, flash_attn_varlen_func
- from flash_attn.bert_padding import pad_input
- SUPPORT_FLASH2 = True
-except ImportError:
- pass
-
-try:
- from transformers.cache_utils import Cache
-except ImportError:
-
- class Cache:
- pass
-
-
-def repeat_kv_bshd(hidden_states: torch.Tensor, n_rep: int) -> torch.Tensor:
- """The hidden states go from (batch, seqlen, num_key_value_heads, head_dim)
- to (batch, seqlen, num_attention_heads, head_dim)"""
- batch, slen, num_key_value_heads, head_dim = hidden_states.shape
- if n_rep == 1:
- return hidden_states
- hidden_states = hidden_states[:, :, :,
- None, :].expand(batch, slen,
- num_key_value_heads, n_rep,
- head_dim)
- return hidden_states.reshape(batch, slen, num_key_value_heads * n_rep,
- head_dim)
-
-
-@sequence_parallel_wrapper
-def flash_attn_wo_mask(query_states,
- key_states,
- value_states,
- causal,
- dropout_rate=0.0):
- attn_output = flash_attn_func(
- query_states, key_states, value_states, dropout_rate, causal=causal)
- return attn_output
-
-
-@sequence_parallel_wrapper
-def flash_attn_w_mask(
- query_states, # bs, q_len, nhead, h_dim
- key_states,
- value_states,
- attention_mask,
- causal,
- dropout_rate=0.0):
- batch_size, q_len = query_states.shape[:2]
- query_states, key_states, value_states, indices_q, \
- cu_seq_lens, max_seq_lens = upad_qkv(
- query_states, key_states, value_states, attention_mask, q_len)
-
- cu_seqlens_q, cu_seqlens_k = cu_seq_lens
- max_seqlen_in_batch_q, max_seqlen_in_batch_k = max_seq_lens
- attn_output_unpad = flash_attn_varlen_func(
- query_states,
- key_states,
- value_states,
- cu_seqlens_q=cu_seqlens_q,
- cu_seqlens_k=cu_seqlens_k,
- max_seqlen_q=max_seqlen_in_batch_q,
- max_seqlen_k=max_seqlen_in_batch_k,
- dropout_p=dropout_rate,
- causal=causal,
- )
- attn_output = pad_input(attn_output_unpad, indices_q, batch_size, q_len)
- return attn_output
-
-
-@sequence_parallel_wrapper
-def varlen_flash_attn(query_states,
- key_states,
- value_states,
- cumulative_len,
- max_seqlen,
- dropout_rate=0.):
- q_unpad, k_unpad, v_unpad = query_states.flatten(0, 1), key_states.flatten(
- 0, 1), value_states.flatten(0, 1)
- attn_output = flash_attn_varlen_func(
- q_unpad,
- k_unpad,
- v_unpad,
- cumulative_len,
- cumulative_len,
- max_seqlen,
- max_seqlen,
- dropout_p=dropout_rate,
- return_attn_probs=False,
- causal=True,
- )
- attn_output = attn_output.unsqueeze(0)
- return attn_output
+from xtuner.parallel.sequence import get_sequence_parallel_world_size
+from xtuner.parallel.sequence.attention import (
+ post_process_for_sequence_parallel_attn,
+ pre_process_for_sequence_parallel_attn)
+# modified from transformers.model.llama.modeling_llama.LlamaAttention.forward
+# and support sequence parallel
def llama_attn_forward(
self,
hidden_states: torch.Tensor,
- attention_mask: Optional[torch.LongTensor] = None,
- position_ids: Optional[torch.LongTensor] = None,
+ position_embeddings: Tuple[torch.Tensor, torch.Tensor],
+ attention_mask: Optional[torch.Tensor],
past_key_value: Optional[Cache] = None,
- output_attentions: bool = False,
- use_cache: bool = False,
cache_position: Optional[torch.LongTensor] = None,
- **kwargs,
+ **kwargs: Unpack[FlashAttentionKwargs],
):
- # Modified from https://github.com/huggingface/transformers/blob/66ce9593fdb8e340df546ddd0774eb444f17a12c/src/transformers/models/llama/modeling_llama.py#L422 # noqa:E501
- output_attentions = False
+ input_shape = hidden_states.shape[:-1]
+ hidden_shape = (*input_shape, -1, self.head_dim)
- bsz, q_len, _ = hidden_states.size()
+ query_states = self.q_proj(hidden_states).view(hidden_shape).transpose(
+ 1, 2)
+ key_states = self.k_proj(hidden_states).view(hidden_shape).transpose(1, 2)
+ value_states = self.v_proj(hidden_states).view(hidden_shape).transpose(
+ 1, 2)
- query_states = self.q_proj(hidden_states)
- key_states = self.k_proj(hidden_states)
- value_states = self.v_proj(hidden_states)
-
- # Flash attention requires the input to have the shape
- # batch_size x seq_length x head_dim x hidden_dim
- # therefore we just need to keep the original shape
- query_states = query_states.view(bsz, q_len, self.num_heads,
- self.head_dim).transpose(1, 2)
- key_states = key_states.view(bsz, q_len, self.num_key_value_heads,
- self.head_dim).transpose(1, 2)
- value_states = value_states.view(bsz, q_len, self.num_key_value_heads,
- self.head_dim).transpose(1, 2)
-
- cos, sin = self.rotary_emb(value_states, position_ids)
+ cos, sin = position_embeddings
query_states, key_states = apply_rotary_pos_emb(query_states, key_states,
cos, sin)
- past_key_value = getattr(self, 'past_key_value', past_key_value)
-
if past_key_value is not None:
- # sin and cos are specific to RoPE models;
- # cache_position needed for the static cache
+ # sin and cos are specific to RoPE models; cache_position needed
+ # for the static cache
cache_kwargs = {
'sin': sin,
'cos': cos,
@@ -157,437 +54,80 @@ def llama_attn_forward(
key_states, value_states = past_key_value.update(
key_states, value_states, self.layer_idx, cache_kwargs)
+ # different from LlamaAttention.forward
+ # repeat k/v heads if n_kv_heads < n_heads for sequence parallel
key_states = repeat_kv(key_states, self.num_key_value_groups)
value_states = repeat_kv(value_states, self.num_key_value_groups)
- assert SUPPORT_FLASH2
- query_states = query_states.transpose(1, 2)
- key_states = key_states.transpose(1, 2)
- value_states = value_states.transpose(1, 2)
-
- # In PEFT, usually we cast the layer norms in float32 for training
- # stability reasons therefore the input hidden states gets silently
- # casted in float32. Hence, we need cast them back in the correct dtype
- # just to be sure everything works as expected.
- # This might slowdown training & inference so it is recommended to not
- # cast the LayerNorms in fp32. (LlamaRMSNorm handles it correctly)
-
- input_dtype = query_states.dtype
- if input_dtype == torch.float32:
- if torch.is_autocast_enabled():
- target_dtype = torch.get_autocast_gpu_dtype()
- # Handle the case where the model is quantized
- elif hasattr(self.config, '_pre_quantization_dtype'):
- target_dtype = self.config._pre_quantization_dtype
- else:
- target_dtype = self.q_proj.weight.dtype
-
- query_states = query_states.to(target_dtype)
- key_states = key_states.to(target_dtype)
- value_states = value_states.to(target_dtype)
-
- dropout_rate = self.attention_dropout if self.training else 0.0
-
- if is_flash_attn_greater_or_equal_2_10():
- causal = self.is_causal
- else:
- # TODO: Remove the `q_len != 1` check once Flash Attention for RoCm
- # is bumped to 2.1. For details, please see the comment in
- # LlamaFlashAttention2 __init__.
- causal = self.is_causal and q_len != 1
-
- # the shape of attention_mask used by flash_attn and
- # F.scaled_dot_product_attention are different
- assert attention_mask is None or attention_mask.ndim == 2, \
- ('When using flash_attn, attention_mask.ndim should equal to 2.'
- f'But got attention_mask.shape = {attention_mask.shape}.'
- 'We can pass the `attn_implementation="flash_attention_2"` flag '
- 'to `.from_pretrained` method when instantiating a Internlm2 '
- 'model.')
-
- if attention_mask is not None:
- attn_output = flash_attn_w_mask(
- query_states,
- key_states,
- value_states,
- attention_mask,
- causal,
- dropout_rate,
- training=self.training)
- else:
- attn_output = flash_attn_wo_mask(
- query_states,
- key_states,
- value_states,
- causal,
- dropout_rate,
- training=self.training)
-
- attn_output = attn_output.reshape(bsz, q_len, self.hidden_size)
- attn_output = self.o_proj(attn_output)
-
- if not output_attentions:
- attn_weights = None
-
- return attn_output, attn_weights, past_key_value
-
-
-def llama_attn_forward_legacy(
- self,
- hidden_states: torch.Tensor,
- attention_mask: Optional[torch.Tensor] = None,
- position_ids: Optional[torch.LongTensor] = None,
- past_key_value: Optional[Cache] = None,
- output_attentions: bool = False,
- use_cache: bool = False,
- **kwargs,
-) -> Tuple[torch.Tensor, Optional[torch.Tensor],
- Optional[Tuple[torch.Tensor]]]:
- # Modified from https://github.com/huggingface/transformers/blob/ced9fd86f55ebb6b656c273f6e23f8ba50652f83/src/transformers/models/llama/modeling_llama.py#L331 # noqa:E501
- if 'padding_mask' in kwargs:
- warnings.warn(
- 'Passing `padding_mask` is deprecated and will be removed in '
- 'v4.37. Please make sure use `attention_mask` instead.`')
-
- bsz, q_len, _ = hidden_states.size()
-
- query_states = self.q_proj(hidden_states)
- key_states = self.k_proj(hidden_states)
- value_states = self.v_proj(hidden_states)
-
- query_states = query_states.view(bsz, q_len, self.num_heads,
- self.head_dim).transpose(1, 2)
- key_states = key_states.view(bsz, q_len, self.num_key_value_heads,
- self.head_dim).transpose(1, 2)
- value_states = value_states.view(bsz, q_len, self.num_key_value_heads,
- self.head_dim).transpose(1, 2)
-
- kv_seq_len = key_states.shape[-2]
- if past_key_value is not None:
- if self.layer_idx is None:
- raise ValueError(
- 'The cache structure has changed since version v4.36. '
- f'If you are using {self.__class__.__name__} '
- 'for auto-regressive decoding with k/v caching, '
- 'please make sure to initialize the attention class '
- 'with a layer index.')
- kv_seq_len += past_key_value.get_usable_length(kv_seq_len,
- self.layer_idx)
- assert position_ids is not None
- if self.training:
- cos, sin = self.rotary_emb(
- value_states, seq_len=position_ids.max() + 1)
- else:
- cos, sin = self.rotary_emb(value_states, seq_len=kv_seq_len)
- query_states, key_states = apply_rotary_pos_emb(query_states, key_states,
- cos, sin, position_ids)
-
- if past_key_value is not None:
- cache_kwargs = {'sin': sin, 'cos': cos} # Specific to RoPE models
- key_states, value_states = past_key_value.update(
- key_states, value_states, self.layer_idx, cache_kwargs)
-
- key_states = repeat_kv(key_states, self.num_key_value_groups)
- value_states = repeat_kv(value_states, self.num_key_value_groups)
-
- assert SUPPORT_FLASH2
- query_states = query_states.transpose(1, 2)
- key_states = key_states.transpose(1, 2)
- value_states = value_states.transpose(1, 2)
-
- # In PEFT, usually we cast the layer norms in float32 for training
- # stability reasons therefore the input hidden states gets silently
- # casted in float32. Hence, we need cast them back in the correct dtype
- # just to be sure everything works as expected.
- # This might slowdown training & inference so it is recommended to not
- # cast the LayerNorms in fp32. (LlamaRMSNorm handles it correctly)
-
- input_dtype = query_states.dtype
- if input_dtype == torch.float32:
- if torch.is_autocast_enabled():
- target_dtype = torch.get_autocast_gpu_dtype()
- # Handle the case where the model is quantized
- elif hasattr(self.config, '_pre_quantization_dtype'):
- target_dtype = self.config._pre_quantization_dtype
- else:
- target_dtype = self.q_proj.weight.dtype
-
- query_states = query_states.to(target_dtype)
- key_states = key_states.to(target_dtype)
- value_states = value_states.to(target_dtype)
-
- dropout_rate = self.attention_dropout if self.training else 0.0
-
- if is_flash_attn_greater_or_equal_2_10():
- causal = self.is_causal
- else:
- # TODO: Remove the `q_len != 1` check once Flash Attention for RoCm
- # is bumped to 2.1. For details, please see the comment in
- # LlamaFlashAttention2 __init__.
- causal = self.is_causal and q_len != 1
-
- # the shape of attention_mask used by flash_attn and
- # F.scaled_dot_product_attention are different
- assert attention_mask is None or attention_mask.ndim == 2, \
- ('When using flash_attn, attention_mask.ndim should equal to 2.'
- f'But got attention_mask.shape = {attention_mask.shape}.'
- 'We can pass the `attn_implementation="flash_attention_2"` flag '
- 'to `.from_pretrained` method when instantiating a Internlm2 '
- 'model.')
-
- if attention_mask is not None:
- attn_output = flash_attn_w_mask(
- query_states,
- key_states,
- value_states,
- attention_mask,
- causal,
- dropout_rate,
- training=self.training)
- else:
- attn_output = flash_attn_wo_mask(
- query_states,
- key_states,
- value_states,
- causal,
- dropout_rate,
- training=self.training)
-
- attn_output = attn_output.reshape(bsz, q_len, self.hidden_size)
- attn_output = self.o_proj(attn_output)
-
- # Due to the implementation of the PyTorch version of flash attention,
- # even when the output_attentions flag is set to True, it is not possible
- # to return the attn_weights.
- return attn_output, None, past_key_value
-
-
-def llama_varlen_attn_forward(
- self,
- hidden_states: torch.Tensor,
- attention_mask: Optional[torch.Tensor] = None,
- position_ids: Optional[torch.LongTensor] = None,
- past_key_value: Optional[Cache] = None,
- output_attentions: bool = False,
- use_cache: bool = False,
- cache_position: Optional[torch.LongTensor] = None,
- **kwargs,
-) -> Tuple[torch.Tensor, Optional[torch.Tensor],
- Optional[Tuple[torch.Tensor]]]:
- is_training = self.training
-
- message_hub = MessageHub.get_instance('varlen_attn_args')
- rank = dist.get_rank()
- cumulative_len = message_hub.get_info(f'cumulative_len_rank_{rank}')
- max_seqlen = message_hub.get_info(f'max_seqlen_rank_{rank}')
- assert is_training == (cumulative_len is not None)
-
- if 'padding_mask' in kwargs:
- warnings.warn('Passing `padding_mask` is deprecated and will be '
- 'removed in v4.37. Please make sure use '
- '`attention_mask` instead.`')
- bsz, q_len, _ = hidden_states.size()
-
- query_states = self.q_proj(hidden_states)
- key_states = self.k_proj(hidden_states)
- value_states = self.v_proj(hidden_states)
-
- query_states = query_states.view(bsz, q_len, self.num_heads,
- self.head_dim).transpose(1, 2)
- key_states = key_states.view(bsz, q_len, self.num_key_value_heads,
- self.head_dim).transpose(1, 2)
- value_states = value_states.view(bsz, q_len, self.num_key_value_heads,
- self.head_dim).transpose(1, 2)
-
- cos, sin = self.rotary_emb(value_states, position_ids)
- query_states, key_states = apply_rotary_pos_emb(query_states, key_states,
- cos, sin)
-
- past_key_value = getattr(self, 'past_key_value', past_key_value)
-
- if past_key_value is not None:
- # sin and cos are specific to RoPE models;
- # cache_position needed for the static cache
- cache_kwargs = {
- 'sin': sin,
- 'cos': cos,
- 'cache_position': cache_position
- }
- key_states, value_states = past_key_value.update(
- key_states, value_states, self.layer_idx, cache_kwargs)
-
- query_states = query_states.transpose(1, 2)
- key_states = key_states.transpose(1, 2)
- value_states = value_states.transpose(1, 2)
-
- dropout_rate = self.attention_dropout if self.training else 0.0
-
- # In PEFT, usually we cast the layer norms in float32 for training
- # stability reasons therefore the input hidden states gets silently casted
- # in float32. Hence, we need cast them back in the correct dtype
- # just to be sure everything works as expected.
- # This might slowdown training & inference so it is recommended to not
- # cast the LayerNorms in fp32. (LlamaRMSNorm handles it correctly)
-
- input_dtype = query_states.dtype
- if input_dtype == torch.float32:
- if torch.is_autocast_enabled():
- target_dtype = torch.get_autocast_gpu_dtype()
- # Handle the case where the model is quantized
- elif hasattr(self.config, '_pre_quantization_dtype'):
- target_dtype = self.config._pre_quantization_dtype
- else:
- target_dtype = self.q_proj.weight.dtype
-
- query_states = query_states.to(target_dtype)
- key_states = key_states.to(target_dtype)
- value_states = value_states.to(target_dtype)
-
- assert SUPPORT_FLASH2
- if is_training:
- attn_output = varlen_flash_attn(
- query_states,
- key_states,
- value_states,
- cumulative_len,
- max_seqlen,
- dropout_rate=dropout_rate)
- else:
- attn_output = flash_attn_wo_mask(
- query_states,
- key_states,
- value_states,
- causal=True,
- training=False)
-
- attn_output = attn_output.reshape(bsz, q_len, self.hidden_size)
- attn_output = self.o_proj(attn_output)
-
- return attn_output, None, past_key_value
-
-
-def llama_varlen_attn_forward_legacy(
- self,
- hidden_states: torch.Tensor,
- attention_mask: Optional[torch.Tensor] = None,
- position_ids: Optional[torch.LongTensor] = None,
- past_key_value: Optional[Cache] = None,
- output_attentions: bool = False,
- use_cache: bool = False,
- **kwargs,
-) -> Tuple[torch.Tensor, Optional[torch.Tensor],
- Optional[Tuple[torch.Tensor]]]:
- is_training = self.training
-
- message_hub = MessageHub.get_instance('varlen_attn_args')
- rank = dist.get_rank()
- cumulative_len = message_hub.get_info(f'cumulative_len_rank_{rank}')
- max_seqlen = message_hub.get_info(f'max_seqlen_rank_{rank}')
- assert is_training == (cumulative_len is not None)
-
- if 'padding_mask' in kwargs:
- warnings.warn('Passing `padding_mask` is deprecated and will be '
- 'removed in v4.37. Please make sure use '
- '`attention_mask` instead.`')
- bsz, q_len, _ = hidden_states.size()
-
- query_states = self.q_proj(hidden_states)
- key_states = self.k_proj(hidden_states)
- value_states = self.v_proj(hidden_states)
-
- query_states = query_states.view(bsz, q_len, self.num_heads, self.head_dim)
- key_states = key_states.view(bsz, q_len, self.num_key_value_heads,
- self.head_dim)
- value_states = value_states.view(bsz, q_len, self.num_key_value_heads,
- self.head_dim)
-
- kv_seq_len = key_states.shape[-3]
- if past_key_value is not None:
- if self.layer_idx is None:
- raise ValueError(
- 'The cache structure has changed since version v4.36. '
- f'If you are using {self.__class__.__name__} '
- 'for auto-regressive decoding with k/v caching, '
- 'please make sure to initialize the attention class '
- 'with a layer index.')
- kv_seq_len += past_key_value.get_usable_length(kv_seq_len,
- self.layer_idx)
-
- if is_training:
- cos, sin = self.rotary_emb(value_states, max_seqlen)
- # position_ids (1, seq_len)
- # cos, sin (1, seq_len, dim) -> (seq_len, dim)
- cos = cos[position_ids].squeeze(0)
- sin = sin[position_ids].squeeze(0)
- query_states = apply_rotary_emb(query_states, cos, sin)
- key_states = apply_rotary_emb(key_states, cos, sin)
- else:
+ enable_sequence_parallel = (
+ dist.is_initialized() and get_sequence_parallel_world_size() > 1
+ and self.training)
+ if enable_sequence_parallel:
+ # Reashape for `pre_process_for_sequence_parallel_attn`
query_states = query_states.transpose(1, 2)
key_states = key_states.transpose(1, 2)
value_states = value_states.transpose(1, 2)
- cos, sin = self.rotary_emb(value_states, kv_seq_len)
- query_states, key_states = apply_rotary_pos_emb(
- query_states, key_states, cos, sin, position_ids)
-
- if past_key_value is not None:
- cache_kwargs = {'sin': sin, 'cos': cos} # Specific to RoPE models
- key_states, value_states = past_key_value.update(
- key_states, value_states, self.layer_idx, cache_kwargs)
-
+ query_states, key_states, value_states = \
+ pre_process_for_sequence_parallel_attn(
+ query_states, key_states, value_states)
query_states = query_states.transpose(1, 2)
key_states = key_states.transpose(1, 2)
value_states = value_states.transpose(1, 2)
- # repeat kv for sequence parallel
- key_states = repeat_kv_bshd(key_states, self.num_key_value_groups)
- value_states = repeat_kv_bshd(value_states, self.num_key_value_groups)
-
- dropout_rate = self.attention_dropout if self.training else 0.0
-
- # In PEFT, usually we cast the layer norms in float32 for training
- # stability reasons therefore the input hidden states gets silently casted
- # in float32. Hence, we need cast them back in the correct dtype
- # just to be sure everything works as expected.
- # This might slowdown training & inference so it is recommended to not
- # cast the LayerNorms in fp32. (LlamaRMSNorm handles it correctly)
-
- input_dtype = query_states.dtype
- if input_dtype == torch.float32:
- if torch.is_autocast_enabled():
- target_dtype = torch.get_autocast_gpu_dtype()
- # Handle the case where the model is quantized
- elif hasattr(self.config, '_pre_quantization_dtype'):
- target_dtype = self.config._pre_quantization_dtype
+ attention_interface: Callable = eager_attention_forward
+ if self.config._attn_implementation != 'eager':
+ if self.config._attn_implementation == 'sdpa' and kwargs.get(
+ 'output_attentions', False):
+ warnings.warn(
+ '`torch.nn.functional.scaled_dot_product_attention` does not '
+ 'support `output_attentions=True`. Falling back to eager '
+ 'attention. This warning can be removed using the argument'
+ ' `attn_implementation="eager"` when loading the model.')
else:
- target_dtype = self.q_proj.weight.dtype
+ attention_interface = ALL_ATTENTION_FUNCTIONS[
+ self.config._attn_implementation]
- query_states = query_states.to(target_dtype)
- key_states = key_states.to(target_dtype)
- value_states = value_states.to(target_dtype)
-
- assert SUPPORT_FLASH2
- if is_training:
- attn_output = varlen_flash_attn(
- query_states,
- key_states,
- value_states,
- cumulative_len,
- max_seqlen,
- dropout_rate=dropout_rate)
- else:
- attn_output = flash_attn_wo_mask(
- query_states,
- key_states,
- value_states,
- causal=True,
- dropout_rate=dropout_rate,
- training=False)
+ message_hub = MessageHub.get_instance('varlen_attn_args')
+ rank = dist.get_rank()
+ cumulative_len = message_hub.get_info(f'cumulative_len_rank_{rank}')
+ use_varlen_atten = (cumulative_len is not None)
+ if use_varlen_atten:
+ # When gradient_checkpointing is enabled, the flash_attn_kwargs
+ # parameter is not automatically passed to the model. In such
+ # cases, parameters like cu_seq_lens_q and max_length_q are
+ # computed based on position_ids. However, when sequence
+ # parallel is enabled, position_ids is split along the
+ # sequence length, leading to incorrect calculations of these
+ # parameters.
+ # To address this issue, it is necessary to manually provide
+ # the flash_attn_kwargs parameters.
+ max_seqlen = message_hub.get_info(f'max_seqlen_rank_{rank}')
+ kwargs['cu_seq_lens_q'] = cumulative_len
+ kwargs['cu_seq_lens_k'] = cumulative_len
+ kwargs['max_length_q'] = max_seqlen
+ kwargs['max_length_k'] = max_seqlen
+ kwargs.pop('position_ids', None)
+
+ # Hacky: `sdpa_attention_forward` does repeat_kv based on
+ # module.num_key_value_groups but it is done before
+ num_key_value_groups = self.num_key_value_groups
+ self.num_key_value_groups = 1
+ attn_output, attn_weights = attention_interface(
+ self,
+ query_states,
+ key_states,
+ value_states,
+ attention_mask,
+ dropout=0.0 if not self.training else self.attention_dropout,
+ scaling=self.scaling,
+ **kwargs,
+ )
+ self.num_key_value_groups = num_key_value_groups
- attn_output = attn_output.reshape(bsz, q_len, self.hidden_size)
+ # different from LlamaAttention.forward
+ if enable_sequence_parallel:
+ attn_output = post_process_for_sequence_parallel_attn(attn_output)
+ attn_output = attn_output.reshape(*input_shape, -1).contiguous()
attn_output = self.o_proj(attn_output)
-
- # Due to the implementation of the PyTorch version of flash attention,
- # even when the output_attentions flag is set to True, it is not possible
- # to return the attn_weights.
- return attn_output, None, past_key_value
+ return attn_output, attn_weights
diff --git a/xtuner/model/modules/dispatch/mistral.py b/xtuner/model/modules/dispatch/mistral.py
index 92245230c..da87ac189 100644
--- a/xtuner/model/modules/dispatch/mistral.py
+++ b/xtuner/model/modules/dispatch/mistral.py
@@ -1,258 +1,134 @@
# Copyright (c) OpenMMLab. All rights reserved.
-import inspect
import warnings
-from typing import Optional
+from typing import Callable, Optional, Tuple
import torch
import torch.distributed as dist
-import torch.nn as nn
from mmengine import MessageHub
from transformers.cache_utils import Cache
-from transformers.models.mistral.modeling_mistral import apply_rotary_pos_emb
+from transformers.modeling_flash_attention_utils import FlashAttentionKwargs
+from transformers.modeling_utils import ALL_ATTENTION_FUNCTIONS
+from transformers.models.mistral.modeling_mistral import (
+ apply_rotary_pos_emb, eager_attention_forward, repeat_kv)
+from transformers.processing_utils import Unpack
-from .triton_kernels import apply_rotary_emb
+from xtuner.parallel.sequence import get_sequence_parallel_world_size
+from xtuner.parallel.sequence.attention import (
+ post_process_for_sequence_parallel_attn,
+ pre_process_for_sequence_parallel_attn)
-SUPPORT_FLASH2 = False
-try:
- from flash_attn import flash_attn_func, flash_attn_varlen_func
- _flash_supports_window_size = 'window_size' in list(
- inspect.signature(flash_attn_func).parameters)
- SUPPORT_FLASH2 = True
-except ImportError:
- pass
-
-
-class MistralRotaryEmbedding(nn.Module):
-
- def __init__(self,
- dim,
- max_position_embeddings=2048,
- base=10000,
- device=None):
- super().__init__()
-
- self.dim = dim
- self.max_position_embeddings = max_position_embeddings
- self.base = base
- self.inv_freq = 1.0 / (
- base**(torch.arange(0, self.dim, 2).float().to(device) / self.dim))
-
- # Build here to make `torch.jit.trace` work.
- self._set_cos_sin_cache(
- seq_len=max_position_embeddings,
- device=self.inv_freq.device,
- dtype=torch.get_default_dtype())
-
- def _set_cos_sin_cache(self, seq_len, device, dtype):
- self.max_seq_len_cached = seq_len
- t = torch.arange(
- self.max_seq_len_cached, device=device, dtype=self.inv_freq.dtype)
- freqs = torch.einsum('i,j->ij', t, self.inv_freq.to(device))
- # Different from paper, but it uses a different permutation
- # in order to obtain the same calculation
- emb = torch.cat((freqs, freqs), dim=-1).to(device)
- self.cos_cached = emb.cos().to(dtype)
- self.sin_cached = emb.sin().to(dtype)
-
- def forward(self, x, seq_len=None):
- # x: [bs, num_attention_heads, seq_len, head_size]
- if (seq_len > self.max_seq_len_cached
- or self.cos_cached.device != x.device # noqa: W503
- or self.cos_cached.dtype != x.dtype): # noqa: W503
- self._set_cos_sin_cache(
- seq_len=seq_len, device=x.device, dtype=x.dtype)
-
- return (
- self.cos_cached[:seq_len].to(dtype=x.dtype),
- self.sin_cached[:seq_len].to(dtype=x.dtype),
- )
-
-
-def mistral_varlen_attn_forward(
+# modified from transformers.model.mistral.modeling_mistral.MistralAttention.forward and # noqa: E501
+# support sequence parallel
+def mistral_attn_forward(
self,
hidden_states: torch.Tensor,
- attention_mask: Optional[torch.Tensor] = None,
- position_ids: Optional[torch.LongTensor] = None,
+ position_embeddings: Tuple[torch.Tensor, torch.Tensor],
+ attention_mask: Optional[torch.Tensor],
past_key_value: Optional[Cache] = None,
- output_attentions: bool = False,
- use_cache: bool = False,
- **kwargs,
+ cache_position: Optional[torch.LongTensor] = None,
+ **kwargs: Unpack[FlashAttentionKwargs],
):
- is_training = self.training
+ input_shape = hidden_states.shape[:-1]
+ hidden_shape = (*input_shape, -1, self.head_dim)
- message_hub = MessageHub.get_instance('varlen_attn_args')
- rank = dist.get_rank()
- cumulative_len = message_hub.get_info(f'cumulative_len_rank_{rank}')
- # position_ids = message_hub.get_info(f'position_ids_rank_{rank}')
- max_seqlen = message_hub.get_info(f'max_seqlen_rank_{rank}')
-
- assert is_training == (cumulative_len is not None) == (
- past_key_value is None)
-
- if 'padding_mask' in kwargs:
- warnings.warn(
- 'Passing `padding_mask` is deprecated and will be removed in v4.37'
- ' Please make sure use `attention_mask` instead.`')
+ query_states = self.q_proj(hidden_states).view(hidden_shape).transpose(
+ 1, 2)
+ key_states = self.k_proj(hidden_states).view(hidden_shape).transpose(1, 2)
+ value_states = self.v_proj(hidden_states).view(hidden_shape).transpose(
+ 1, 2)
- # overwrite attention_mask with padding_mask
- attention_mask = kwargs.pop('padding_mask')
- bsz, q_len, _ = hidden_states.size()
- assert bsz == 1, (f'If utilizing local attention, the batch size should be'
- f' set to 1, but got {bsz}')
- # attention_mask is set to None if no padding token in input_ids
- assert attention_mask is None
+ cos, sin = position_embeddings
+ query_states, key_states = apply_rotary_pos_emb(query_states, key_states,
+ cos, sin)
- query_states = self.q_proj(hidden_states)
- key_states = self.k_proj(hidden_states)
- value_states = self.v_proj(hidden_states)
-
- query_states = query_states.view(bsz, q_len, self.num_heads, self.head_dim)
- key_states = key_states.view(bsz, q_len, self.num_key_value_heads,
- self.head_dim)
- value_states = value_states.view(bsz, q_len, self.num_key_value_heads,
- self.head_dim)
-
- assert _flash_supports_window_size, \
- ('The current flash attention version does not support sliding window '
- 'attention, for a more memory efficient implementation make sure '
- 'to upgrade flash-attn library.')
-
- kv_seq_len = key_states.shape[-3]
if past_key_value is not None:
- if self.layer_idx is None:
- raise ValueError(
- 'The cache structure has changed since version v4.36. '
- f'If you are using {self.__class__.__name__} '
- 'for auto-regressive decoding with k/v caching, '
- 'please make sure to initialize the attention class '
- 'with a layer index.')
- kv_seq_len += past_key_value.get_usable_length(kv_seq_len,
- self.layer_idx)
+ # sin and cos are specific to RoPE models; cache_position needed
+ # for the static cache
+ cache_kwargs = {
+ 'sin': sin,
+ 'cos': cos,
+ 'cache_position': cache_position
+ }
+ key_states, value_states = past_key_value.update(
+ key_states, value_states, self.layer_idx, cache_kwargs)
- if is_training:
- cos, sin = self.rotary_emb(value_states, max_seqlen)
- query_states = apply_rotary_emb(query_states,
- cos[position_ids].squeeze(0),
- sin[position_ids].squeeze(0))
- key_states = apply_rotary_emb(key_states, cos[position_ids].squeeze(0),
- sin[position_ids].squeeze(0))
- else:
+ # different from MistralAttention.forward
+ # repeat k/v heads if n_kv_heads < n_heads for sequence parallel
+ key_states = repeat_kv(key_states, self.num_key_value_groups)
+ value_states = repeat_kv(value_states, self.num_key_value_groups)
+
+ enable_sequence_parallel = (
+ dist.is_initialized() and get_sequence_parallel_world_size() > 1
+ and self.training)
+ if enable_sequence_parallel:
+ # Reashape for `pre_process_for_sequence_parallel_attn`
query_states = query_states.transpose(1, 2)
key_states = key_states.transpose(1, 2)
value_states = value_states.transpose(1, 2)
- # Because the input can be padded, the absolute sequence length
- # depends on the max position id.
- rotary_seq_len = max(kv_seq_len, position_ids[:, -1].max().item() + 1)
- cos, sin = self.rotary_emb(value_states, seq_len=rotary_seq_len)
- query_states, key_states = apply_rotary_pos_emb(
- query_states, key_states, cos, sin, position_ids)
-
- # Activate slicing cache only if the config has a value
- # `sliding_windows` attribute
- cache_has_contents = past_key_value.get_seq_length(self.layer_idx) > 0
- if (getattr(self.config, 'sliding_window', None) is not None
- and kv_seq_len > self.config.sliding_window # noqa: W503
- and cache_has_contents): # noqa: W503
- slicing_tokens = 1 - self.config.sliding_window
-
- past_key = past_key_value[self.layer_idx][0]
- past_value = past_key_value[self.layer_idx][1]
-
- past_key = past_key[:, :, slicing_tokens:, :].contiguous()
- past_value = past_value[:, :, slicing_tokens:, :].contiguous()
-
- if past_key.shape[-2] != self.config.sliding_window - 1:
- raise ValueError(
- 'past key must have a shape of (`batch_size, num_heads, '
- 'self.config.sliding_window-1, head_dim`), got'
- f' {past_key.shape}')
-
- if attention_mask is not None:
- attention_mask = attention_mask[:, slicing_tokens:]
- attention_mask = torch.cat(
- [attention_mask,
- torch.ones_like(attention_mask[:, -1:])],
- dim=-1)
-
- cache_kwargs = {'sin': sin, 'cos': cos} # Specific to RoPE models
- key_states, value_states = past_key_value.update(
- key_states, value_states, self.layer_idx, cache_kwargs)
+ query_states, key_states, value_states = \
+ pre_process_for_sequence_parallel_attn(
+ query_states, key_states, value_states)
query_states = query_states.transpose(1, 2)
key_states = key_states.transpose(1, 2)
value_states = value_states.transpose(1, 2)
- # repeat_kv is Done in flash_attn
- # key_states = repeat_kv(key_states, self.num_key_value_groups)
- # value_states = repeat_kv(value_states, self.num_key_value_groups)
- dropout_rate = 0.0 if not self.training else self.attention_dropout
-
- # In PEFT, usually we cast the layer norms in float32 for
- # training stability reasons, therefore the input hidden states gets
- # silently casted in float32. Hence, we need
- # cast them back in float16 just to be sure everything works as expected.
- input_dtype = query_states.dtype
- if input_dtype == torch.float32:
- if torch.is_autocast_enabled():
- target_dtype = torch.get_autocast_gpu_dtype()
- # Handle the case where the model is quantized
- elif hasattr(self.config, '_pre_quantization_dtype'):
- target_dtype = self.config._pre_quantization_dtype
+ attention_interface: Callable = eager_attention_forward
+ if self.config._attn_implementation != 'eager':
+ if self.config._attn_implementation == 'sdpa' and kwargs.get(
+ 'output_attentions', False):
+ warnings.warn(
+ '`torch.nn.functional.scaled_dot_product_attention` does not '
+ 'support `output_attentions=True`. Falling back to eager '
+ 'attention. This warning can be removed using the argument'
+ ' `attn_implementation="eager"` when loading the model.')
else:
- target_dtype = self.q_proj.weight.dtype
+ attention_interface = ALL_ATTENTION_FUNCTIONS[
+ self.config._attn_implementation]
- query_states = query_states.to(target_dtype)
- key_states = key_states.to(target_dtype)
- value_states = value_states.to(target_dtype)
-
- # ----------------- flash attention forward ------------------------#
- if not self._flash_attn_uses_top_left_mask:
- causal = self.is_causal
- else:
- causal = self.is_causal and q_len != 1
-
- use_sliding_windows = (
- _flash_supports_window_size and # noqa: W504
- getattr(self.config, 'sliding_window', None) is not None # noqa: W503
- and kv_seq_len > self.config.sliding_window) # noqa: W503
- window_size = (self.config.sliding_window,
- self.config.sliding_window) if use_sliding_windows else (-1,
- -1)
- if is_training:
- q_unpad, k_unpad, v_unpad = query_states.flatten(
- 0, 1), key_states.flatten(0, 1), value_states.flatten(0, 1)
- cumulative_len = torch.cat(cumulative_len, dim=0)
- attn_output = flash_attn_varlen_func(
- q_unpad,
- k_unpad,
- v_unpad,
- cumulative_len,
- cumulative_len,
- max_seqlen,
- max_seqlen,
- dropout_rate,
- return_attn_probs=False,
- causal=True,
- window_size=window_size,
- )
- else:
- attn_output = flash_attn_func(
- query_states,
- key_states,
- value_states,
- 0,
- softmax_scale=None,
- causal=causal,
- window_size=window_size,
- )
-
- # ---------------- flash attention forward end ------------------- #
-
- attn_output = attn_output.reshape(bsz, q_len,
- self.hidden_size).contiguous()
+ message_hub = MessageHub.get_instance('varlen_attn_args')
+ rank = dist.get_rank()
+ cumulative_len = message_hub.get_info(f'cumulative_len_rank_{rank}')
+ use_varlen_atten = (cumulative_len is not None)
+ if use_varlen_atten:
+ # When gradient_checkpointing is enabled, the flash_attn_kwargs
+ # parameter is not automatically passed to the model. In such
+ # cases, parameters like cu_seq_lens_q and max_length_q are
+ # computed based on position_ids. However, when sequence
+ # parallel is enabled, position_ids is split along the
+ # sequence length, leading to incorrect calculations of these
+ # parameters.
+ # To address this issue, it is necessary to manually provide
+ # the flash_attn_kwargs parameters.
+ max_seqlen = message_hub.get_info(f'max_seqlen_rank_{rank}')
+ kwargs['cu_seq_lens_q'] = cumulative_len
+ kwargs['cu_seq_lens_k'] = cumulative_len
+ kwargs['max_length_q'] = max_seqlen
+ kwargs['max_length_k'] = max_seqlen
+ kwargs.pop('position_ids', None)
+
+ # Hacky: `sdpa_attention_forward` does repeat_kv based on
+ # module.num_key_value_groups but it is done before
+ num_key_value_groups = self.num_key_value_groups
+ self.num_key_value_groups = 1
+ attn_output, attn_weights = attention_interface(
+ self,
+ query_states,
+ key_states,
+ value_states,
+ attention_mask,
+ dropout=0.0 if not self.training else self.attention_dropout,
+ scaling=self.scaling,
+ sliding_window=getattr(self.config, 'sliding_window',
+ None), # main diff with Llama
+ **kwargs,
+ )
+ self.num_key_value_groups = num_key_value_groups
+
+ # different from MistralAttention.forward
+ if enable_sequence_parallel:
+ attn_output = post_process_for_sequence_parallel_attn(attn_output)
+
+ attn_output = attn_output.reshape(*input_shape, -1).contiguous()
attn_output = self.o_proj(attn_output)
-
- if not output_attentions:
- attn_weights = None
-
- return attn_output, attn_weights, past_key_value
+ return attn_output, attn_weights
diff --git a/xtuner/model/modules/dispatch/phi3.py b/xtuner/model/modules/dispatch/phi3.py
new file mode 100644
index 000000000..10f60f939
--- /dev/null
+++ b/xtuner/model/modules/dispatch/phi3.py
@@ -0,0 +1,480 @@
+# Copyright (c) OpenMMLab. All rights reserved.
+import inspect
+import warnings
+from typing import Optional, Tuple
+
+import torch
+import torch.distributed as dist
+import transformers
+from mmengine import MessageHub
+from mmengine.utils import digit_version
+
+from xtuner.parallel.sequence import (get_sequence_parallel_world_size,
+ post_process_for_sequence_parallel_attn,
+ pre_process_for_sequence_parallel_attn)
+from .attention import flash_attn_wo_mask, varlen_flash_attn
+
+try:
+ from transformers.cache_utils import Cache
+except ImportError:
+
+ class Cache:
+ pass
+
+
+TRANSFORMERS_VERSION = digit_version(transformers.__version__)
+IS_LOW_VERSION_TRANSFORMERS = TRANSFORMERS_VERSION < digit_version('4.43')
+
+if not IS_LOW_VERSION_TRANSFORMERS:
+ from transformers.modeling_flash_attention_utils import \
+ _flash_attention_forward
+
+_flash_supports_window_size = False
+try:
+ from flash_attn import flash_attn_func
+
+ _flash_supports_window_size = 'window_size' in list(
+ inspect.signature(flash_attn_func).parameters)
+
+ if not _flash_supports_window_size:
+ raise ValueError(
+ 'Please update flash-attention to support window size.')
+# else:
+except ImportError:
+ pass
+
+
+# Copied from https://huggingface.co/microsoft/Phi-3-mini-4k-instruct/blob/3a811845d89f3c1b3f41b341d0f9f05104769f35/modeling_phi3.py#L302 # noqa:E501
+def repeat_kv(hidden_states: torch.Tensor, n_rep: int) -> torch.Tensor:
+ """This is the equivalent of torch.repeat_interleave(x, dim=1,
+ repeats=n_rep).
+
+ The hidden states go from (batch, num_key_value_heads, seqlen, head_dim) to
+ (batch, num_attention_heads, seqlen, head_dim)
+ """
+ batch, num_key_value_heads, slen, head_dim = hidden_states.shape
+ if n_rep == 1:
+ return hidden_states
+ hidden_states = hidden_states[:, :,
+ None, :, :].expand(batch,
+ num_key_value_heads,
+ n_rep, slen, head_dim)
+ return hidden_states.reshape(batch, num_key_value_heads * n_rep, slen,
+ head_dim)
+
+
+# https://huggingface.co/microsoft/Phi-3-mini-4k-instruct/blob/3a811845d89f3c1b3f41b341d0f9f05104769f35/modeling_phi3.py#L247 # noqa:E501
+def rotate_half(x):
+ """Rotates half the hidden dims of the input."""
+ x1 = x[..., :x.shape[-1] // 2]
+ x2 = x[..., x.shape[-1] // 2:]
+ return torch.cat((-x2, x1), dim=-1)
+
+
+# Copied from https://huggingface.co/microsoft/Phi-3-mini-4k-instruct/blob/3a811845d89f3c1b3f41b341d0f9f05104769f35/modeling_phi3.py#L255 # noqa:E501
+def apply_rotary_pos_emb(q, k, cos, sin, position_ids=None, unsqueeze_dim=1):
+ """Applies Rotary Position Embedding to the query and key tensors.
+
+ Args:
+ q (`torch.Tensor`): The query tensor.
+ k (`torch.Tensor`): The key tensor.
+ cos (`torch.Tensor`): The cosine part of the rotary embedding.
+ sin (`torch.Tensor`): The sine part of the rotary embedding.
+ position_ids (`torch.Tensor`, *optional*):
+ Deprecated and unused.
+ unsqueeze_dim (`int`, *optional*, defaults to 1):
+ The 'unsqueeze_dim' argument specifies the dimension along which to unsqueeze cos[position_ids] and
+ sin[position_ids] so that they can be properly broadcasted to the dimensions of q and k. For example, note
+ that cos[position_ids] and sin[position_ids] have the shape [batch_size, seq_len, head_dim]. Then, if q and
+ k have the shape [batch_size, heads, seq_len, head_dim], then setting unsqueeze_dim=1 makes
+ cos[position_ids] and sin[position_ids] broadcastable to the shapes of q and k. Similarly, if q and k have
+ the shape [batch_size, seq_len, heads, head_dim], then set unsqueeze_dim=2.
+ Returns:
+ `tuple(torch.Tensor)` comprising of the query and key tensors rotated using the Rotary Position Embedding.
+ """ # noqa:E501
+ cos = cos.unsqueeze(unsqueeze_dim)
+ sin = sin.unsqueeze(unsqueeze_dim)
+ q_embed = (q * cos) + (rotate_half(q) * sin)
+ k_embed = (k * cos) + (rotate_half(k) * sin)
+ return q_embed, k_embed
+
+
+def phi3_attn_forward(
+ self,
+ hidden_states: torch.Tensor,
+ attention_mask: Optional[torch.LongTensor] = None,
+ position_ids: Optional[torch.LongTensor] = None,
+ past_key_value: Optional[Cache] = None,
+ output_attentions: bool = False,
+ use_cache: bool = False,
+ cache_position: Optional[torch.LongTensor] = None,
+ **kwargs,
+):
+ if not _flash_supports_window_size:
+ raise ValueError(
+ 'The current flash attention version does not support '
+ 'sliding window attention.')
+
+ output_attentions = False
+
+ if 'padding_mask' in kwargs:
+ warnings.warn(
+ 'Passing `padding_mask` is deprecated and will be removed in '
+ 'v4.37. Please make sure use `attention_mask` instead.`')
+
+ # overwrite attention_mask with padding_mask
+ attention_mask = kwargs.pop('padding_mask')
+
+ bsz, q_len, _ = hidden_states.size()
+
+ qkv = self.qkv_proj(hidden_states)
+ query_pos = self.num_heads * self.head_dim
+ query_states = qkv[..., :query_pos]
+ key_states = qkv[..., query_pos:query_pos +
+ self.num_key_value_heads * self.head_dim]
+ value_states = qkv[...,
+ query_pos + self.num_key_value_heads * self.head_dim:]
+
+ # Flash attention requires the input to have the shape
+ # batch_size x seq_length x head_dim x hidden_dim
+ # therefore we just need to keep the original shape
+ query_states = query_states.view(bsz, q_len, self.num_heads,
+ self.head_dim).transpose(1, 2)
+ key_states = key_states.view(bsz, q_len, self.num_key_value_heads,
+ self.head_dim).transpose(1, 2)
+ value_states = value_states.view(bsz, q_len, self.num_key_value_heads,
+ self.head_dim).transpose(1, 2)
+
+ kv_seq_len = key_states.shape[-2]
+ if past_key_value is not None:
+ if self.layer_idx is None:
+ raise ValueError(
+ 'The cache structure has changed since version v4.36. '
+ f'If you are using {self.__class__.__name__} '
+ 'for auto-regressive decoding with k/v caching, '
+ 'please make sure to initialize the attention class '
+ 'with a layer index.')
+ kv_seq_len += past_key_value.get_usable_length(kv_seq_len,
+ self.layer_idx)
+
+ rotary_seq_len = max(kv_seq_len, position_ids.max().item() + 1)
+ cos, sin = self.rotary_emb(
+ value_states, position_ids, seq_len=rotary_seq_len)
+
+ query_states, key_states = apply_rotary_pos_emb(query_states, key_states,
+ cos, sin, position_ids)
+
+ use_sliding_windows = (
+ _flash_supports_window_size
+ and getattr(self.config, 'sliding_window', None) is not None
+ and kv_seq_len > self.config.sliding_window)
+
+ if past_key_value is not None:
+ # Activate slicing cache only if the config has a value
+ # `sliding_windows` attribute
+ cache_has_contents = past_key_value.get_seq_length(self.layer_idx) > 0
+ if (getattr(self.config, 'sliding_window', None) is not None
+ and kv_seq_len > self.config.sliding_window
+ and cache_has_contents):
+ slicing_tokens = 1 - self.config.sliding_window
+
+ past_key = past_key_value[self.layer_idx][0]
+ past_value = past_key_value[self.layer_idx][1]
+
+ past_key = past_key[:, :, slicing_tokens:, :].contiguous()
+ past_value = past_value[:, :, slicing_tokens:, :].contiguous()
+
+ if past_key.shape[-2] != self.config.sliding_window - 1:
+ raise ValueError(
+ 'past key must have a shape of (`batch_size, num_heads, '
+ 'self.config.sliding_window-1, head_dim`), got'
+ f' {past_key.shape}')
+
+ if attention_mask is not None:
+ attention_mask = attention_mask[:, slicing_tokens:]
+ attention_mask = torch.cat(
+ [attention_mask,
+ torch.ones_like(attention_mask[:, -1:])],
+ dim=-1)
+
+ cache_kwargs = {'sin': sin, 'cos': cos} # Specific to RoPE models
+ key_states, value_states = past_key_value.update(
+ key_states, value_states, self.layer_idx, cache_kwargs)
+
+ # repeat k/v heads if n_kv_heads < n_heads
+ key_states = repeat_kv(key_states, self.num_key_value_groups)
+ value_states = repeat_kv(value_states, self.num_key_value_groups)
+
+ attn_dropout = self.attention_dropout if self.training else 0.0
+
+ # In PEFT, usually we cast the layer norms in float32 for training
+ # stability reasons therefore the input hidden states gets silently
+ # casted in float32. Hence, we need cast them back in the correct dtype
+ # just to be sure everything works as expected.
+ # This might slowdown training & inference so it is recommended to not
+ # cast the LayerNorms in fp32.
+
+ if query_states.dtype == torch.float32:
+ if torch.is_autocast_enabled():
+ target_dtype = torch.get_autocast_gpu_dtype()
+ # Handle the case where the model is quantized
+ elif hasattr(self.config, '_pre_quantization_dtype'):
+ target_dtype = self.config._pre_quantization_dtype
+ else:
+ target_dtype = self.qkv_proj.weight.dtype
+
+ query_states = query_states.to(target_dtype)
+ key_states = key_states.to(target_dtype)
+ value_states = value_states.to(target_dtype)
+
+ # Reashape to the expected shape for Flash Attention
+ query_states = query_states.transpose(1, 2)
+ key_states = key_states.transpose(1, 2)
+ value_states = value_states.transpose(1, 2)
+
+ enable_sequence_parallel = (
+ dist.is_initialized() and get_sequence_parallel_world_size() > 1
+ and self.training)
+ if enable_sequence_parallel:
+ # (b, s // sp_world_size, nd, dim) -> (b, s, nd // sp_world_size, dim)
+ query_states, key_states, value_states = \
+ pre_process_for_sequence_parallel_attn(
+ query_states, key_states, value_states,
+ scatter_dim=2, gather_dim=1)
+ # num_heads has been changed because of sequence parallel
+ # `self.num_heads`` is not used in self._flash_attention_forward
+ # in mistral/mixtral, we are doing this to avoid some unnecessary risk
+ ori_num_head = self.num_heads
+ self.num_heads = query_states.shape[-2]
+
+ if IS_LOW_VERSION_TRANSFORMERS:
+ attn_output = self._flash_attention_forward(
+ query_states,
+ key_states,
+ value_states,
+ attention_mask,
+ query_states.shape[1],
+ dropout=attn_dropout,
+ use_sliding_windows=use_sliding_windows,
+ )
+ else:
+ attn_output = _flash_attention_forward(
+ query_states,
+ key_states,
+ value_states,
+ attention_mask,
+ query_states.shape[1],
+ dropout=attn_dropout,
+ sliding_window=getattr(self.config, 'sliding_window', None),
+ use_top_left_mask=self._flash_attn_uses_top_left_mask,
+ is_causal=self.is_causal,
+ )
+
+ if enable_sequence_parallel:
+ # (b, s, nd // sp_world_size, dim) -> (b, s // sp_world_size, nd, dim)
+ attn_output = post_process_for_sequence_parallel_attn(
+ attn_output, scatter_dim=1, gather_dim=2)
+ self.num_heads = ori_num_head
+
+ attn_output = attn_output.reshape(bsz, q_len, self.hidden_size)
+ attn_output = self.o_proj(attn_output)
+
+ if not output_attentions:
+ attn_weights = None
+
+ return attn_output, attn_weights, past_key_value
+
+
+def phi3_varlen_attn_forward(
+ self,
+ hidden_states: torch.Tensor,
+ attention_mask: Optional[torch.Tensor] = None,
+ position_ids: Optional[torch.LongTensor] = None,
+ past_key_value: Optional[Cache] = None,
+ output_attentions: bool = False,
+ use_cache: bool = False,
+ cache_position: Optional[torch.LongTensor] = None,
+ **kwargs,
+) -> Tuple[torch.Tensor, Optional[torch.Tensor],
+ Optional[Tuple[torch.Tensor]]]:
+ if not _flash_supports_window_size:
+ raise ValueError(
+ 'The current flash attention version does not support '
+ 'sliding window attention.')
+
+ output_attentions = False
+
+ is_training = self.training
+
+ message_hub = MessageHub.get_instance('varlen_attn_args')
+ rank = dist.get_rank()
+ cumulative_len = message_hub.get_info(f'cumulative_len_rank_{rank}')
+ max_seqlen = message_hub.get_info(f'max_seqlen_rank_{rank}')
+
+ assert is_training == (past_key_value is None)
+ use_varlen_atten = (cumulative_len is not None)
+
+ if 'padding_mask' in kwargs:
+ warnings.warn(
+ 'Passing `padding_mask` is deprecated and will be removed in v4.37'
+ ' Please make sure use `attention_mask` instead.`')
+
+ # overwrite attention_mask with padding_mask
+ attention_mask = kwargs.pop('padding_mask')
+
+ bsz, q_len, _ = hidden_states.size()
+ assert bsz == 1, (f'If utilizing local attention, the batch size should be'
+ f' set to 1, but got {bsz}')
+ # attention_mask is set to None if no padding token in input_ids
+ # varlen attn need data packing so no padding tokens in input_ids
+ assert attention_mask is None
+
+ qkv = self.qkv_proj(hidden_states)
+ query_pos = self.num_heads * self.head_dim
+ query_states = qkv[..., :query_pos]
+ key_states = qkv[..., query_pos:query_pos +
+ self.num_key_value_heads * self.head_dim]
+ value_states = qkv[...,
+ query_pos + self.num_key_value_heads * self.head_dim:]
+
+ # Flash attention requires the input to have the shape
+ # batch_size x seq_length x head_dim x hidden_dim
+ # therefore we just need to keep the original shape
+ query_states = query_states.view(bsz, q_len, self.num_heads,
+ self.head_dim).transpose(1, 2)
+ key_states = key_states.view(bsz, q_len, self.num_key_value_heads,
+ self.head_dim).transpose(1, 2)
+ value_states = value_states.view(bsz, q_len, self.num_key_value_heads,
+ self.head_dim).transpose(1, 2)
+
+ kv_seq_len = key_states.shape[-2]
+ if past_key_value is not None:
+ if self.layer_idx is None:
+ raise ValueError(
+ 'The cache structure has changed since version v4.36. '
+ f'If you are using {self.__class__.__name__} '
+ 'for auto-regressive decoding with k/v caching, '
+ 'please make sure to initialize the attention class '
+ 'with a layer index.')
+ kv_seq_len += past_key_value.get_usable_length(kv_seq_len,
+ self.layer_idx)
+
+ assert position_ids is not None
+ rotary_seq_len = max(kv_seq_len, position_ids.max().item() + 1)
+ cos, sin = self.rotary_emb(
+ value_states, position_ids, seq_len=rotary_seq_len)
+
+ query_states, key_states = apply_rotary_pos_emb(query_states, key_states,
+ cos, sin, position_ids)
+
+ use_sliding_windows = (
+ _flash_supports_window_size
+ and getattr(self.config, 'sliding_window', None) is not None
+ and kv_seq_len > self.config.sliding_window)
+
+ if past_key_value is not None:
+ # Activate slicing cache only if the config has a value
+ # `sliding_windows` attribute
+ cache_has_contents = past_key_value.get_seq_length(self.layer_idx) > 0
+ if (getattr(self.config, 'sliding_window', None) is not None
+ and kv_seq_len > self.config.sliding_window
+ and cache_has_contents):
+ slicing_tokens = 1 - self.config.sliding_window
+
+ past_key = past_key_value[self.layer_idx][0]
+ past_value = past_key_value[self.layer_idx][1]
+
+ past_key = past_key[:, :, slicing_tokens:, :].contiguous()
+ past_value = past_value[:, :, slicing_tokens:, :].contiguous()
+
+ if past_key.shape[-2] != self.config.sliding_window - 1:
+ raise ValueError(
+ 'past key must have a shape of (`batch_size, num_heads, '
+ 'self.config.sliding_window-1, head_dim`), got'
+ f' {past_key.shape}')
+
+ if attention_mask is not None:
+ attention_mask = attention_mask[:, slicing_tokens:]
+ attention_mask = torch.cat(
+ [attention_mask,
+ torch.ones_like(attention_mask[:, -1:])],
+ dim=-1)
+
+ cache_kwargs = {'sin': sin, 'cos': cos} # Specific to RoPE models
+ key_states, value_states = past_key_value.update(
+ key_states, value_states, self.layer_idx, cache_kwargs)
+
+ # repeat k/v heads if n_kv_heads < n_heads
+ key_states = repeat_kv(key_states, self.num_key_value_groups)
+ value_states = repeat_kv(value_states, self.num_key_value_groups)
+
+ # In PEFT, usually we cast the layer norms in float32 for
+ # training stability reasons, therefore the input hidden states gets
+ # silently casted in float32. Hence, we need
+ # cast them back in float16 just to be sure everything works as expected.
+
+ if query_states.dtype == torch.float32:
+ if torch.is_autocast_enabled():
+ target_dtype = torch.get_autocast_gpu_dtype()
+ # Handle the case where the model is quantized
+ elif hasattr(self.config, '_pre_quantization_dtype'):
+ target_dtype = self.config._pre_quantization_dtype
+ else:
+ target_dtype = self.qkv_proj.weight.dtype
+
+ query_states = query_states.to(target_dtype)
+ key_states = key_states.to(target_dtype)
+ value_states = value_states.to(target_dtype)
+
+ # Reashape to the expected shape for Flash Attention
+ query_states = query_states.transpose(1, 2)
+ key_states = key_states.transpose(1, 2)
+ value_states = value_states.transpose(1, 2)
+
+ # ----------------- flash attention forward ------------------------#
+
+ if not self._flash_attn_uses_top_left_mask:
+ causal = self.is_causal
+ else:
+ causal = self.is_causal and q_len != 1
+
+ use_sliding_windows = (
+ _flash_supports_window_size
+ and getattr(self.config, 'sliding_window', None) is not None
+ and kv_seq_len > self.config.sliding_window)
+
+ window_size = (self.config.sliding_window,
+ self.config.sliding_window) if use_sliding_windows else (-1,
+ -1)
+ attn_dropout = self.attention_dropout if self.training else 0.0
+
+ if use_varlen_atten:
+ attn_output = varlen_flash_attn(
+ query_states,
+ key_states,
+ value_states,
+ cumulative_len,
+ max_seqlen,
+ causal=causal,
+ dropout_p=attn_dropout,
+ window_size=window_size,
+ training=self.training)
+ else:
+ attn_output = flash_attn_wo_mask(
+ query_states,
+ key_states,
+ value_states,
+ causal=causal,
+ dropout_p=attn_dropout,
+ window_size=window_size,
+ training=self.training)
+
+ # ---------------- flash attention forward end ------------------- #
+
+ attn_output = attn_output.reshape(bsz, q_len, self.hidden_size)
+ attn_output = self.o_proj(attn_output)
+
+ if not output_attentions:
+ attn_weights = None
+
+ return attn_output, attn_weights, past_key_value
diff --git a/xtuner/model/modules/dispatch/qwen2.py b/xtuner/model/modules/dispatch/qwen2.py
new file mode 100644
index 000000000..179a3aba4
--- /dev/null
+++ b/xtuner/model/modules/dispatch/qwen2.py
@@ -0,0 +1,140 @@
+# Copyright (c) OpenMMLab. All rights reserved.
+import warnings
+from typing import Callable, Optional, Tuple
+
+import torch
+import torch.distributed as dist
+from mmengine import MessageHub
+from transformers.cache_utils import Cache
+from transformers.modeling_flash_attention_utils import FlashAttentionKwargs
+from transformers.modeling_utils import ALL_ATTENTION_FUNCTIONS
+from transformers.models.qwen2.modeling_qwen2 import (apply_rotary_pos_emb,
+ eager_attention_forward,
+ repeat_kv)
+from transformers.processing_utils import Unpack
+
+from xtuner.parallel.sequence import get_sequence_parallel_world_size
+from xtuner.parallel.sequence.attention import (
+ post_process_for_sequence_parallel_attn,
+ pre_process_for_sequence_parallel_attn)
+
+
+# modified from transformers.model.qwen2.modeling_qwen2.Qwen2Attention.forward and # noqa: E501
+# support sequence parallel
+def qwen2_attn_forward(
+ self,
+ hidden_states: torch.Tensor,
+ position_embeddings: Tuple[torch.Tensor, torch.Tensor],
+ attention_mask: Optional[torch.Tensor],
+ past_key_value: Optional[Cache] = None,
+ cache_position: Optional[torch.LongTensor] = None,
+ **kwargs: Unpack[FlashAttentionKwargs],
+):
+ input_shape = hidden_states.shape[:-1]
+ hidden_shape = (*input_shape, -1, self.head_dim)
+
+ query_states = self.q_proj(hidden_states).view(hidden_shape).transpose(
+ 1, 2)
+ key_states = self.k_proj(hidden_states).view(hidden_shape).transpose(1, 2)
+ value_states = self.v_proj(hidden_states).view(hidden_shape).transpose(
+ 1, 2)
+
+ cos, sin = position_embeddings
+ query_states, key_states = apply_rotary_pos_emb(query_states, key_states,
+ cos, sin)
+
+ if past_key_value is not None:
+ # sin and cos are specific to RoPE models; cache_position needed
+ # for the static cache
+ cache_kwargs = {
+ 'sin': sin,
+ 'cos': cos,
+ 'cache_position': cache_position
+ }
+ key_states, value_states = past_key_value.update(
+ key_states, value_states, self.layer_idx, cache_kwargs)
+
+ # different from Qwen2Attention.forward
+ # repeat k/v heads if n_kv_heads < n_heads for sequence parallel
+ key_states = repeat_kv(key_states, self.num_key_value_groups)
+ value_states = repeat_kv(value_states, self.num_key_value_groups)
+
+ enable_sequence_parallel = (
+ dist.is_initialized() and get_sequence_parallel_world_size() > 1
+ and self.training)
+ if enable_sequence_parallel:
+ # Reashape for `pre_process_for_sequence_parallel_attn`
+ query_states = query_states.transpose(1, 2)
+ key_states = key_states.transpose(1, 2)
+ value_states = value_states.transpose(1, 2)
+ query_states, key_states, value_states = \
+ pre_process_for_sequence_parallel_attn(
+ query_states, key_states, value_states)
+ query_states = query_states.transpose(1, 2)
+ key_states = key_states.transpose(1, 2)
+ value_states = value_states.transpose(1, 2)
+
+ sliding_window = None
+ if (self.config.use_sliding_window
+ and getattr(self.config, 'sliding_window', None) is not None
+ and self.layer_idx >= self.config.max_window_layers):
+ sliding_window = self.config.sliding_window
+
+ attention_interface: Callable = eager_attention_forward
+ if self.config._attn_implementation != 'eager':
+ if self.config._attn_implementation == 'sdpa' and kwargs.get(
+ 'output_attentions', False):
+ warnings.warn(
+ '`torch.nn.functional.scaled_dot_product_attention` does not '
+ 'support `output_attentions=True`. Falling back to eager '
+ 'attention. This warning can be removed using the argument'
+ ' `attn_implementation="eager"` when loading the model.')
+ else:
+ attention_interface = ALL_ATTENTION_FUNCTIONS[
+ self.config._attn_implementation]
+
+ message_hub = MessageHub.get_instance('varlen_attn_args')
+ rank = dist.get_rank()
+ cumulative_len = message_hub.get_info(f'cumulative_len_rank_{rank}')
+ use_varlen_atten = (cumulative_len is not None)
+ if use_varlen_atten:
+ # When gradient_checkpointing is enabled, the flash_attn_kwargs
+ # parameter is not automatically passed to the model. In such
+ # cases, parameters like cu_seq_lens_q and max_length_q are
+ # computed based on position_ids. However, when sequence
+ # parallel is enabled, position_ids is split along the
+ # sequence length, leading to incorrect calculations of these
+ # parameters.
+ # To address this issue, it is necessary to manually provide
+ # the flash_attn_kwargs parameters.
+ max_seqlen = message_hub.get_info(f'max_seqlen_rank_{rank}')
+ kwargs['cu_seq_lens_q'] = cumulative_len
+ kwargs['cu_seq_lens_k'] = cumulative_len
+ kwargs['max_length_q'] = max_seqlen
+ kwargs['max_length_k'] = max_seqlen
+ kwargs.pop('position_ids', None)
+
+ # Hacky: `sdpa_attention_forward` does repeat_kv based on
+ # module.num_key_value_groups but it is done before
+ num_key_value_groups = self.num_key_value_groups
+ self.num_key_value_groups = 1
+ attn_output, attn_weights = attention_interface(
+ self,
+ query_states,
+ key_states,
+ value_states,
+ attention_mask,
+ dropout=0.0 if not self.training else self.attention_dropout,
+ scaling=self.scaling,
+ sliding_window=sliding_window, # main diff with Llama
+ **kwargs,
+ )
+ self.num_key_value_groups = num_key_value_groups
+
+ # different from Qwen2Attention.forward
+ if enable_sequence_parallel:
+ attn_output = post_process_for_sequence_parallel_attn(attn_output)
+
+ attn_output = attn_output.reshape(*input_shape, -1).contiguous()
+ attn_output = self.o_proj(attn_output)
+ return attn_output, attn_weights
diff --git a/xtuner/model/modules/dispatch/triton_kernels/__init__.py b/xtuner/model/modules/dispatch/triton_kernels/__init__.py
index 6f9da4a4c..ed29f409f 100644
--- a/xtuner/model/modules/dispatch/triton_kernels/__init__.py
+++ b/xtuner/model/modules/dispatch/triton_kernels/__init__.py
@@ -1,5 +1,6 @@
# Copyright (c) OpenMMLab. All rights reserved.
+from .layer_norm import layer_norm_forward
from .rms_norm import rms_norm_forward
from .rotary import apply_rotary_emb
-__all__ = ['rms_norm_forward', 'apply_rotary_emb']
+__all__ = ['rms_norm_forward', 'layer_norm_forward', 'apply_rotary_emb']
diff --git a/xtuner/model/modules/dispatch/triton_kernels/layer_norm.py b/xtuner/model/modules/dispatch/triton_kernels/layer_norm.py
new file mode 100644
index 000000000..f808d6ad1
--- /dev/null
+++ b/xtuner/model/modules/dispatch/triton_kernels/layer_norm.py
@@ -0,0 +1,12 @@
+# Copyright (c) OpenMMLab. All rights reserved.
+import torch
+import torch.nn.functional as F
+
+
+def layer_norm_forward(self, hidden_states):
+ input_dtype = hidden_states.dtype
+ hidden_states = hidden_states.to(torch.float32)
+ hidden_states = F.layer_norm(
+ hidden_states, (hidden_states.shape[-1], ), eps=self.variance_epsilon)
+ hidden_states = self.weight.to(torch.float32) * hidden_states
+ return hidden_states.to(input_dtype)
diff --git a/xtuner/model/modules/dispatch/triton_kernels/rms_norm.py b/xtuner/model/modules/dispatch/triton_kernels/rms_norm.py
index 6191d55ba..a6c9069ab 100644
--- a/xtuner/model/modules/dispatch/triton_kernels/rms_norm.py
+++ b/xtuner/model/modules/dispatch/triton_kernels/rms_norm.py
@@ -3,6 +3,7 @@
import triton
import triton.language as tl
+from xtuner.utils.device import get_device_name
@triton.jit
def _rms_norm_fwd_fused(
@@ -128,7 +129,7 @@ def forward(ctx, x, weight, eps):
# reshape input data into 2D tensor
x_arg = x.reshape(-1, x.shape[-1])
M, N = x_arg.shape
- rstd = torch.empty((M, ), dtype=torch.float32, device='cuda')
+ rstd = torch.empty((M, ), dtype=torch.float32, device=get_device_name())
# Less than 64KB per feature: enqueue fused kernel
MAX_FUSED_SIZE = 65536 // x.element_size()
BLOCK_SIZE = min(MAX_FUSED_SIZE, triton.next_power_of_2(N))
@@ -168,7 +169,7 @@ def backward(ctx, dy):
if N <= 1024:
GROUP_SIZE_M = 256
# allocate output
- locks = torch.zeros(2 * GROUP_SIZE_M, dtype=torch.int32, device='cuda')
+ locks = torch.zeros(2 * GROUP_SIZE_M, dtype=torch.int32, device=get_device_name())
_dw = torch.empty((GROUP_SIZE_M, w.shape[0]),
dtype=x.dtype,
device=w.device)
diff --git a/xtuner/model/modules/dispatch/triton_kernels/rotary.py b/xtuner/model/modules/dispatch/triton_kernels/rotary.py
index 1e09c1662..82b3ea38e 100644
--- a/xtuner/model/modules/dispatch/triton_kernels/rotary.py
+++ b/xtuner/model/modules/dispatch/triton_kernels/rotary.py
@@ -6,6 +6,7 @@
import triton
import triton.language as tl
+from xtuner.utils.device import get_torch_device
@triton.jit
def rotary_kernel(
@@ -231,7 +232,7 @@ def grid(META):
# Need this, otherwise Triton tries to launch from cuda:0 and we get
# ValueError: Pointer argument (at 0) cannot be accessed from Triton
# (cpu tensor?)
- with torch.cuda.device(x.device.index):
+ with get_torch_device().device(x.device.index):
rotary_kernel[grid](
output, # data ptrs
x,
diff --git a/xtuner/model/orpo.py b/xtuner/model/orpo.py
new file mode 100644
index 000000000..37264088a
--- /dev/null
+++ b/xtuner/model/orpo.py
@@ -0,0 +1,212 @@
+# ORPO Authors: Jiwoo Hong, Noah Lee, and James Thorne
+# Official code: https://github.com/xfactlab/orpo
+# Copyright (c) OpenMMLab. All rights reserved.
+import torch
+import torch.distributed as dist
+import torch.nn.functional as F
+from mmengine import MessageHub
+from torch import nn
+
+from xtuner.parallel.sequence import (gather_forward_split_backward,
+ get_sequence_parallel_group,
+ get_sequence_parallel_world_size,
+ split_for_sequence_parallel)
+from .sft import SupervisedFinetune
+
+
+class ORPO(SupervisedFinetune):
+ """ORPO: Monolithic Preference Optimization without Reference Model
+ https://arxiv.org/abs/2403.07691
+
+ Args:
+ beta (float): Weight of the odds_ratio_loss. Defaults to 0.1.
+ """
+
+ def __init__(self, *args, beta=0.1, **kwargs):
+ super().__init__(*args, **kwargs)
+ self.beta = beta
+
+ def _gather_masked_logits(self, logits, labels, mask):
+ logits = torch.gather(
+ logits.log_softmax(-1), dim=2,
+ index=labels.unsqueeze(2)).squeeze(2)
+ return logits * mask
+
+ def get_logps(
+ self,
+ all_logps, # bs, seqlen
+ average_log_prob,
+ loss_mask, # bs, seqlen
+ ):
+ all_logps = all_logps[:, :-1].sum(-1)
+ loss_mask = loss_mask[:, :-1]
+
+ if average_log_prob: # average_log_prob
+ all_logps = all_logps / loss_mask.sum(-1)
+
+ chosen_logps = all_logps[::2]
+ rejected_logps = all_logps[1::2]
+ return chosen_logps, rejected_logps
+
+ def get_var_len_atten_logps(self, all_logps, average_log_prob, loss_mask,
+ cu_seqlens, attention_mask):
+ seqlens = (cu_seqlens[1:] - cu_seqlens[:-1]).tolist()
+ # unpack sequence
+ unpacked_logps = torch.split(all_logps, seqlens, dim=1)
+ unpacked_loss_mask = torch.split(loss_mask, seqlens, dim=1)
+ if attention_mask is not None:
+ # It indicate that we pad the original sequence, labels,
+ # position_ids and cumulative_len for sequence parallel if the
+ # attention_mask is not None.
+ # We then need to remove the padded segments.
+ assert False in attention_mask
+ unpacked_logps = unpacked_logps[:-1]
+ unpacked_loss_mask = unpacked_loss_mask[:-1]
+ assert len(unpacked_logps) % 2 == 0
+
+ def compute_logps(_logps, _mask):
+ _logps = _logps[:, :-1].sum(-1)
+ _mask = _mask[:, :-1]
+ if average_log_prob:
+ _logps /= _mask.sum(-1)
+ return _logps
+
+ chosen_logps, rejected_logps = [], []
+ for i in range(len(unpacked_logps) // 2):
+ chosen = unpacked_logps[2 * i]
+ rejected = unpacked_logps[2 * i + 1]
+ chosen_mask = unpacked_loss_mask[2 * i]
+ rejected_mask = unpacked_loss_mask[2 * i + 1]
+ chosen_logps.append(compute_logps(chosen, chosen_mask))
+ rejected_logps.append(compute_logps(rejected, rejected_mask))
+
+ return (torch.stack(chosen_logps), torch.stack(rejected_logps))
+
+ def cross_entropy_loss(self, logits, labels):
+ logits = logits[..., :-1, :].contiguous()
+ # labels are already shifted, now we need to remove the last dummy label # noqa
+ labels = labels[..., :-1].contiguous()
+ # Flatten the tokens
+ loss_fct = nn.CrossEntropyLoss()
+ logits = logits.view(-1, logits.shape[-1])
+ labels = labels.view(-1)
+ # Enable model parallelism
+ labels = labels.to(logits.device)
+ loss = loss_fct(logits, labels)
+ return loss
+
+ def odds_ratio_loss(
+ self,
+ chosen_logps: torch.FloatTensor,
+ rejected_logps: torch.FloatTensor,
+ ):
+ # modified from https://github.com/huggingface/trl/blob/b031adfdb8708f1f295eab6c3f2cb910e8fe0c23/trl/trainer/orpo_trainer.py#L597 # noqa
+ # Derived from Eqs. (4) and (7) from https://arxiv.org/abs/2403.07691 by using log identities and exp(log(P(y|x)) = P(y|x) # noqa
+ log_odds = (chosen_logps - rejected_logps) - (
+ torch.log1p(-torch.exp(chosen_logps)) -
+ torch.log1p(-torch.exp(rejected_logps)))
+ ratio = F.logsigmoid(log_odds)
+ ratio = ratio[~torch.isnan(ratio)] # select valid loss
+ losses = self.beta * ratio
+
+ chosen_rewards = self.beta * chosen_logps
+ rejected_rewards = self.beta * rejected_logps
+
+ return losses, chosen_rewards, rejected_rewards, torch.mean(
+ ratio), torch.mean(log_odds)
+
+ @staticmethod
+ def _split_for_sequence_parallel(data):
+ # attention mask should not be split
+ ARGS_NEED_TO_SPLIT = ('input_ids', 'position_ids', 'labels',
+ 'chosen_rejected_tag')
+ sp_group = get_sequence_parallel_group()
+ for key in ARGS_NEED_TO_SPLIT:
+ val = data.get(key, None)
+ if val is not None:
+ # `dim` is 1 as the shape of tensor is (bs, seq_len, ...)
+ data[key] = split_for_sequence_parallel(
+ val, dim=1, sp_group=sp_group)
+ return data
+
+ def compute_loss(self, data, data_samples=None):
+ # shift labels first and add a dummy label at the end, to support sequence parallel # noqa
+ data['labels'] = torch.cat(
+ (data['labels'][:, 1:], torch.zeros_like(data['labels'][:, :1])),
+ dim=1)
+ tmp_label = data['labels'].clone()
+ tmp_label[tmp_label == 0] = -100
+ # loss mask of all tokens in all sp ranks
+ all_loss_mask = data['labels'] != -100
+
+ if self.use_varlen_attn:
+ # create a chosen rejected tag for varlen_attn ce loss
+ message_hub = MessageHub.get_instance('varlen_attn_args')
+ rank = dist.get_rank()
+ cu_seqlens = message_hub.get_info(f'cumulative_len_rank_{rank}')
+ seqlens = (cu_seqlens[1:] - cu_seqlens[:-1]).tolist()
+
+ chosen_rejected_tag = torch.ones_like(data['labels'])
+ unpacked_tag = list(
+ torch.split(chosen_rejected_tag, seqlens, dim=1))
+ # import pdb; pdb.set_trace()
+ for i in range(len(unpacked_tag) // 2):
+ # import pdb; pdb.set_trace()
+ unpacked_tag[2 * i + 1] *= 0
+ chosen_rejected_tag = torch.cat(unpacked_tag, dim=1)
+ data['chosen_rejected_tag'] = chosen_rejected_tag
+
+ if get_sequence_parallel_world_size() > 1:
+ data = self._split_for_sequence_parallel(data)
+ chosen_rejected_tag = data.pop('chosen_rejected_tag', None)
+ all_logits = self.llm(**data).logits
+
+ labels = data['labels'].clone()
+ labels[labels == -100] = 0
+ loss_mask = labels != 0 # loss mask in a single sp rank
+ all_logps = self._gather_masked_logits(all_logits, labels, loss_mask)
+ if get_sequence_parallel_world_size() > 1:
+ all_logps = gather_forward_split_backward(
+ all_logps,
+ dim=1,
+ sp_group=get_sequence_parallel_group(),
+ grad_scale='up')
+
+ if not self.use_varlen_attn:
+ chosen_nll_loss = self.cross_entropy_loss(all_logits[::2],
+ data['labels'][::2])
+ chosen_logps, rejected_logps = self.get_logps(
+ all_logps, True, all_loss_mask)
+ else:
+ chosen_idxs = chosen_rejected_tag == 1
+ chosen_logits = all_logits[chosen_idxs]
+ chosen_labels = data['labels'][chosen_idxs]
+ chosen_nll_loss = self.cross_entropy_loss(chosen_logits,
+ chosen_labels)
+
+ chosen_logps, rejected_logps = self.get_var_len_atten_logps(
+ all_logps, True, all_loss_mask, cu_seqlens,
+ data['attention_mask'])
+ (losses, chosen_rewards, rejected_rewards, log_odds_ratio,
+ log_odds_chosen) = self.odds_ratio_loss(chosen_logps, rejected_logps)
+ losses = losses.mean()
+ # skip nan loss
+ if torch.isnan(chosen_nll_loss):
+ chosen_nll_loss = all_logits.mean() * 0
+ if torch.isnan(losses):
+ losses = all_logits.mean() * 0
+ loss = chosen_nll_loss - losses
+
+ reward_acc = (chosen_rewards > rejected_rewards).float().mean()
+
+ loss_dict = {
+ 'loss': loss,
+ 'chosen_rewards': chosen_rewards.mean(),
+ 'rejected_rewards': rejected_rewards.mean(),
+ 'reward_acc': reward_acc,
+ 'reward_margin': (chosen_rewards - rejected_rewards).mean(),
+ 'log_odds_ratio': log_odds_ratio,
+ 'log_odds_chosen': log_odds_chosen,
+ 'nll_loss': chosen_nll_loss.detach().mean()
+ }
+ return loss_dict
diff --git a/xtuner/model/reward.py b/xtuner/model/reward.py
new file mode 100644
index 000000000..6e4628050
--- /dev/null
+++ b/xtuner/model/reward.py
@@ -0,0 +1,491 @@
+# Copyright (c) OpenMMLab. All rights reserved.
+import json
+import math
+import os
+import warnings
+from collections import OrderedDict
+from contextlib import nullcontext
+
+import torch
+import torch.distributed as dist
+from mmengine import print_log
+from mmengine.config import Config, ConfigDict
+from mmengine.model import BaseModel
+from mmengine.runner import load_checkpoint
+from peft import get_peft_model, prepare_model_for_kbit_training
+from torch import nn
+from transformers import (AutoConfig, AutoModelForSequenceClassification,
+ PreTrainedModel, PreTrainedTokenizer)
+from transformers.dynamic_module_utils import get_class_from_dynamic_module
+from transformers.integrations import is_deepspeed_zero3_enabled
+from transformers.modeling_utils import no_init_weights
+
+from xtuner.parallel.sequence import (gather_forward_split_backward,
+ get_sequence_parallel_group,
+ get_sequence_parallel_world_size,
+ split_for_sequence_parallel)
+from xtuner.registry import BUILDER
+from xtuner.utils.device import get_torch_device
+from .modules import dispatch_modules
+from .modules.dispatch import SUPPORT_FLASH1, SUPPORT_FLASH2
+from .utils import (LoadWoInit, find_all_linear_names,
+ get_peft_model_state_dict, make_inputs_require_grad,
+ traverse_dict)
+
+
+def reduce_mean(tensor):
+ """"Obtain the mean of tensor on different GPUs."""
+ if not (dist.is_available() and dist.is_initialized()):
+ return tensor
+ tensor = tensor.clone()
+ dist.all_reduce(tensor.div_(dist.get_world_size()), op=dist.ReduceOp.SUM)
+ return tensor
+
+
+def smart_tokenizer_and_embedding_resize(
+ tokenizer: PreTrainedTokenizer,
+ model: PreTrainedModel,
+):
+ """Resize embedding."""
+ if is_deepspeed_zero3_enabled():
+ import deepspeed
+
+ params = [model.get_input_embeddings().weight]
+ if model.get_output_embeddings(
+ ) is not None and not model.config.tie_word_embeddings:
+ params.append(model.get_output_embeddings().weight)
+
+ context_maybe_zero3 = deepspeed.zero.GatheredParameters(
+ params, modifier_rank=0)
+ else:
+ context_maybe_zero3 = nullcontext()
+
+ with context_maybe_zero3:
+ current_embedding_size = model.get_input_embeddings().weight.size(0)
+
+ if len(tokenizer) > current_embedding_size:
+ assert isinstance(model.get_output_embeddings(), nn.Linear)
+
+ model.resize_token_embeddings(len(tokenizer), pad_to_multiple_of=64)
+ with context_maybe_zero3:
+ num_new_tokens = len(tokenizer) - current_embedding_size
+ input_embeddings = model.get_input_embeddings().weight.data
+ output_embeddings = model.get_output_embeddings().weight.data
+
+ input_embeddings_avg = input_embeddings[:-num_new_tokens].mean(
+ dim=0, keepdim=True)
+ output_embeddings_avg = output_embeddings[:-num_new_tokens].mean(
+ dim=0, keepdim=True)
+
+ input_embeddings[-num_new_tokens:] = input_embeddings_avg
+ output_embeddings[-num_new_tokens:] = output_embeddings_avg
+
+ print_log(
+ f'Resized token embeddings from {current_embedding_size} to '
+ f'{len(tokenizer)}.', 'current')
+
+
+class RewardModel(BaseModel):
+
+ def __init__(
+ self,
+ llm,
+ lora=None,
+ peft_model=None,
+ use_activation_checkpointing=True,
+ use_varlen_attn=False,
+ tokenizer=None,
+ max_position_embeddings=None,
+ reward_token_id=None,
+ loss_type='ranking',
+ penalty_type='log_barrier',
+ penalty_weight=0.01,
+ ):
+ super().__init__()
+ with LoadWoInit():
+ if isinstance(llm, dict):
+ llm = self._dispatch_lm_model_cfg(llm, max_position_embeddings)
+ self.llm = self._build_from_cfg_or_module(llm).model
+ self.v_head = nn.Linear(self.llm.config.hidden_size, 1, bias=False)
+ # zero init
+ self.v_head.weight.data.zero_()
+
+ self.reward_token_id = reward_token_id
+ assert loss_type in ('ranking',
+ 'focal'), f'Unsupported loss type {loss_type}'
+ self.loss_type = loss_type
+ assert penalty_type in (
+ 'log_barrier', 'L2',
+ 'none'), f'Unsupported penalty type {penalty_type}'
+ self.penalty_type = penalty_type
+ self.penalty_weight = penalty_weight
+
+ if tokenizer is not None:
+ if isinstance(tokenizer, dict):
+ tokenizer = BUILDER.build(tokenizer)
+ smart_tokenizer_and_embedding_resize(tokenizer, self.llm)
+
+ self.llm.config.use_cache = False
+ dispatch_modules(self.llm, use_varlen_attn=use_varlen_attn)
+
+ if use_activation_checkpointing:
+ # For backward compatibility
+ if hasattr(self.llm, 'enable_input_require_grads'):
+ self.llm.enable_input_require_grads()
+ else:
+ self.llm.get_input_embeddings().register_forward_hook(
+ make_inputs_require_grad)
+
+ # enable gradient checkpointing for memory efficiency
+ self.gradient_checkpointing_enable()
+
+ if isinstance(lora, dict) or isinstance(lora, Config) or isinstance(
+ lora, ConfigDict):
+ self.lora = BUILDER.build(lora)
+ else:
+ self.lora = lora
+ self.peft_model = peft_model
+ self.use_lora = lora is not None
+ if self.use_lora:
+ self._prepare_for_lora(peft_model, use_activation_checkpointing)
+
+ self._is_init = True
+ # Determines whether to calculate attention based on the
+ # seq_len dimension (use_varlen_attn = False) or the actual length of
+ # the sequence.
+ self.use_varlen_attn = use_varlen_attn
+
+ def gradient_checkpointing_enable(self):
+ self.activation_checkpointing_enable()
+
+ def activation_checkpointing_enable(self):
+ self.llm.gradient_checkpointing_enable()
+
+ def gradient_checkpointing_disable(self):
+ self.activation_checkpointing_disable()
+
+ def activation_checkpointing_disable(self):
+ self.llm.gradient_checkpointing_disable()
+
+ def _prepare_for_lora(self,
+ peft_model=None,
+ use_activation_checkpointing=True):
+ self.llm = prepare_model_for_kbit_training(
+ self.llm, use_activation_checkpointing)
+ if self.lora.target_modules is None:
+ modules = find_all_linear_names(self.llm)
+ self.lora.target_modules = modules
+
+ self.llm = get_peft_model(self.llm, self.lora)
+ if peft_model is not None:
+ _ = load_checkpoint(self, peft_model)
+
+ def init_weights(self):
+ pass
+
+ @staticmethod
+ def _prepare_for_long_context_training(cfg, llm_cfg,
+ max_position_embeddings):
+ if not hasattr(llm_cfg, 'rope_scaling'):
+ print_log('Current model does not support RoPE scaling.',
+ 'current')
+ return
+
+ current_max_length = getattr(llm_cfg, 'max_position_embeddings', None)
+ if current_max_length and max_position_embeddings > current_max_length:
+ print_log(
+ f'Enlarge max model length from {current_max_length} '
+ f'to {max_position_embeddings}.', 'current')
+ scaling_factor = float(
+ math.ceil(max_position_embeddings / current_max_length))
+ else:
+ print_log(
+ 'The input `max_position_embeddings` is smaller than '
+ 'origin max length. Consider increase input length.',
+ 'current')
+ scaling_factor = 1.0
+ cfg.rope_scaling = {'type': 'linear', 'factor': scaling_factor}
+
+ return cfg
+
+ @staticmethod
+ def _prepare_for_flash_attn(cfg, llm_cfg):
+ cls_name = type(llm_cfg).__name__
+ SUPPORT_SDPA_ATTN = ('LlamaConfig', 'GemmaConfig', 'MistralConfig',
+ 'MixtralConfig', 'Qwen2Config', 'Qwen2MoeConfig',
+ 'Starcoder2Config', 'Starcoder2Config',
+ 'Phi3Config')
+ SUPPORT_FLASH_ATTN2 = ('InternLM2Config', 'LlamaConfig', 'GemmaConfig',
+ 'MistralConfig', 'MixtralConfig', 'Qwen2Config',
+ 'Qwen2MoeConfig', 'Starcoder2Config',
+ 'Starcoder2Config', 'Phi3Config')
+
+ torch_dtype = torch.bfloat16 if (
+ get_torch_device().is_available() and get_torch_device().is_bf16_supported()) \
+ else torch.float16
+
+ if getattr(cfg, 'attn_implementation', None) is not None:
+ # Flash Attention 2.0 only supports torch.float16 and
+ # torch.bfloat16 dtypes
+ if cfg.attn_implementation == 'flash_attention_2':
+ cfg.torch_dtype = torch_dtype
+ elif SUPPORT_FLASH2 and cls_name in SUPPORT_FLASH_ATTN2:
+ cfg.torch_dtype = torch_dtype
+ cfg.attn_implementation = 'flash_attention_2'
+ elif SUPPORT_FLASH1 and cls_name in SUPPORT_SDPA_ATTN:
+ cfg.attn_implementation = 'sdpa'
+
+ return cfg
+
+ @staticmethod
+ def _prepare_for_qlora_zero3(cfg):
+ if (not is_deepspeed_zero3_enabled()) or (not hasattr(
+ cfg, 'quantization_config')):
+ return cfg
+
+ torch_dtype = torch.bfloat16 if (
+ get_torch_device().is_available() and get_torch_device().is_bf16_supported()) \
+ else torch.float16
+
+ cfg.torch_dtype = torch_dtype
+ quantization_config = cfg.quantization_config
+ quantization_config.bnb_4bit_compute_dtype = torch_dtype
+ quantization_config.bnb_4bit_quant_storage = torch_dtype
+
+ return cfg
+
+ def _dispatch_lm_model_cfg(self, cfg, max_position_embeddings=None):
+ cfg = self._prepare_for_qlora_zero3(cfg)
+ pretrained_model_name_or_path = cfg.pretrained_model_name_or_path
+ llm_cfg = AutoConfig.from_pretrained(
+ pretrained_model_name_or_path, trust_remote_code=True)
+ cfg = self._prepare_for_flash_attn(cfg, llm_cfg)
+ if max_position_embeddings is not None:
+ cfg = self._prepare_for_long_context_training(
+ cfg, llm_cfg, max_position_embeddings)
+ return cfg
+
+ def _build_from_cfg_or_module(self, cfg_or_mod):
+ if isinstance(cfg_or_mod, nn.Module):
+ return cfg_or_mod
+ elif isinstance(cfg_or_mod, dict):
+ traverse_dict(cfg_or_mod)
+ return BUILDER.build(cfg_or_mod)
+ else:
+ raise NotImplementedError
+
+ def forward(self, data, data_samples=None, mode='loss'):
+ labels = data.pop('labels', None)
+ if mode == 'loss':
+ return self.compute_loss(data, labels)
+ elif mode == 'predict':
+ return self.predict(data, data_samples)
+ elif mode == 'tensor':
+ return self._forward(data, data_samples)
+ else:
+ raise NotImplementedError
+
+ def _forward(self, data, data_samples=None):
+ hidden_states = self.llm(**data)[0]
+ logits = self.v_head(hidden_states)
+ return logits
+
+ def predict(self, data, data_samples=None):
+ hidden_states = self.llm(**data)[0]
+ logits = self.v_head(hidden_states)
+ logits_dict = [{'logits': log} for log in logits]
+ return logits_dict
+
+ @staticmethod
+ def _split_for_sequence_parallel(data):
+ # attention mask should not be split
+ ARGS_NEED_TO_SPLIT = ('input_ids', 'position_ids')
+ sp_group = get_sequence_parallel_group()
+ for key in ARGS_NEED_TO_SPLIT:
+ val = data.get(key, None)
+ if val is not None:
+ # `dim` is 1 as the shape of tensor is (bs, seq_len, ...)
+ data[key] = split_for_sequence_parallel(
+ val, dim=1, sp_group=sp_group)
+ return data
+
+ def compute_loss(self, data, labels=None):
+ if get_sequence_parallel_world_size() > 1:
+ data = self._split_for_sequence_parallel(data)
+
+ hidden_states = self.llm(**data)[0]
+ logits = self.v_head(hidden_states)
+
+ if get_sequence_parallel_world_size() > 1:
+ logits = gather_forward_split_backward(
+ logits,
+ dim=1,
+ sp_group=get_sequence_parallel_group(),
+ grad_scale='up')
+
+ chosen_idx = torch.where(labels == 0)
+ rejected_idx = torch.where(labels == 1)
+ chosen_logits = logits[chosen_idx]
+ rejected_logits = logits[rejected_idx]
+
+ num_samples = torch.tensor(len(chosen_logits)).float().to(
+ hidden_states.device)
+ avg_factor = 1.0 / num_samples
+ avg_factor = reduce_mean(avg_factor).to(hidden_states.device)
+
+ chosen_mean = reduce_mean(chosen_logits.mean().detach())
+ rejected_mean = reduce_mean(rejected_logits.mean().detach())
+ acc = reduce_mean(
+ (chosen_logits > rejected_logits).sum() / num_samples).detach()
+ num_tokens = torch.tensor(labels.shape[1]).float()
+
+ # ranking loss
+ if self.loss_type == 'ranking':
+ rank_loss = self.ranking_loss(
+ chosen_logits, rejected_logits, avg_factor=avg_factor)
+ elif self.loss_type == 'focal':
+ rank_loss = self.focal_loss(
+ chosen_logits, rejected_logits, avg_factor=avg_factor)
+ else:
+ raise NotImplementedError(
+ f'Unsupported loss type {self.loss_type}')
+
+ # penalty loss
+ if self.penalty_type == 'log_barrier':
+ penalty = self.log_barrier_penalty(
+ torch.cat([chosen_logits, rejected_logits]),
+ lower_bound=-5,
+ upper_bound=5,
+ avg_factor=avg_factor)
+ elif self.penalty_type == 'L2':
+ penalty = self.l2_penalty(
+ torch.cat([chosen_logits, rejected_logits]),
+ avg_factor=avg_factor)
+ elif self.penalty_type == 'none':
+ penalty = 0
+ else:
+ raise NotImplementedError(
+ f'Unsupported penalty type {self.penalty_type}')
+
+ loss = rank_loss + self.penalty_weight * penalty
+ loss_dict = {
+ 'loss': loss,
+ 'acc': acc,
+ 'chosen_score_mean': chosen_mean,
+ 'rejected_score_mean': rejected_mean,
+ 'num_samples': num_samples,
+ 'num_tokens': num_tokens,
+ }
+
+ return loss_dict
+
+ def ranking_loss(self, chosen_logits, rejected_logits, avg_factor):
+ rank_loss = -nn.functional.logsigmoid(chosen_logits - rejected_logits)
+ return rank_loss.sum() * avg_factor
+
+ def focal_loss(self, chosen_logits, rejected_logits, avg_factor):
+ # focal ranking loss from InternLM2 paper https://arxiv.org/abs/2403.17297 # noqa
+ rank_loss = -nn.functional.logsigmoid(chosen_logits - rejected_logits)
+ p_ij = torch.sigmoid(chosen_logits - rejected_logits)
+ p = 2 * torch.relu(p_ij - 0.5)
+ gamma = 2
+ focal_loss = ((1 - p)**gamma) * rank_loss
+ return focal_loss.sum() * avg_factor
+
+ def log_barrier_penalty(self,
+ logits,
+ lower_bound,
+ upper_bound,
+ epsilon=1e-3,
+ avg_factor=1):
+ # log barrier penalty from InternLM2 paper https://arxiv.org/abs/2403.17297 # noqa
+ logits_fp32 = logits.float()
+ logits_clamped = torch.clamp(logits_fp32, lower_bound + epsilon,
+ upper_bound - epsilon)
+ penalty = -torch.log(upper_bound - logits_clamped) - torch.log(
+ logits_clamped - lower_bound)
+ return penalty.sum() * avg_factor
+
+ def l2_penalty(self, logits, avg_factor=1):
+ return (logits**2).sum() * avg_factor
+
+ def state_dict(self, *args, **kwargs):
+ state_dict = super().state_dict(*args, **kwargs)
+ if not self.use_lora:
+ return state_dict
+ to_return = get_peft_model_state_dict(self.llm, state_dict=state_dict)
+ return OrderedDict(to_return)
+
+ def __getattr__(self, name: str):
+ try:
+ return super().__getattr__(name)
+ except AttributeError:
+ return getattr(self.llm, name)
+
+ def to_hf(self,
+ cfg,
+ save_dir,
+ fp32=False,
+ save_pretrained_kwargs={},
+ **kwargs):
+ print(f'Saving LLM tokenizer to {save_dir}')
+ tokenizer = BUILDER.build(cfg.tokenizer)
+ tokenizer.save_pretrained(save_dir)
+
+ if 'PeftModel' in self.llm.__class__.__name__:
+ # merge adapter
+ self.llm = self.llm.merge_and_unload()
+ if 'InternLM2' in self.llm.__class__.__name__:
+ from xtuner.tools.model_converters.modeling_internlm2_reward.modeling_internlm2 import \
+ InternLM2ForRewardModel # noqa
+ print(f'Saving Reward Model to {save_dir}')
+ hf_cfg = self.llm.config
+ hf_cfg.reward_token_id = self.reward_token_id if \
+ self.reward_token_id is not None else cfg.reward_token_id
+ if not fp32:
+ dtype = torch.float16
+ else:
+ dtype = torch.float32
+ with no_init_weights():
+ reward_model = InternLM2ForRewardModel._from_config(
+ hf_cfg, torch_dtype=dtype)
+ reward_model.model.load_state_dict(self.llm.state_dict())
+ reward_model.v_head.load_state_dict(self.v_head.state_dict())
+ reward_model.save_pretrained(save_dir, **save_pretrained_kwargs)
+ # fix auto_map in config
+ with open(os.path.join(save_dir, 'config.json')) as fp:
+ config_dict = json.load(fp)
+ config_dict['auto_map'][
+ 'AutoModel'] = 'modeling_internlm2.InternLM2ForRewardModel'
+ config_dict['auto_map'].pop('AutoModelForCausalLM', None)
+ with open(os.path.join(save_dir, 'config.json'), 'w') as fp:
+ json.dump(config_dict, fp, indent=2)
+ else:
+ warnings.warn(
+ f'The pretrained model type: {self.llm.__class__.__name__} '
+ 'has no reward model class defined. Use '
+ 'the SequenceClassification class instead.'
+ 'You can refer to `xtuner/tools/model_converters/modeling_internlm2_reward` ' # noqa
+ 'to implement the reward model class.')
+
+ hf_cfg = self.llm.config
+ hf_cfg.num_labels = 1 # set the output dim to 1
+ try:
+ with no_init_weights():
+ reward_model = \
+ AutoModelForSequenceClassification.from_config(hf_cfg)
+ except Exception as e:
+ warnings.warn(f'Cannot find SequenceClassification class '
+ f'from transformers: {e}, \n'
+ 'try to find it in the dynamic module.')
+ module_file, causal_model_name = hf_cfg.auto_map[
+ 'AutoModelForCausalLM'].split('.')
+ seqcls_model_name = causal_model_name.split(
+ 'For')[0] + 'ForSequenceClassification'
+ seqcls_class = get_class_from_dynamic_module(
+ f'{module_file}.{seqcls_model_name}', hf_cfg._name_or_path)
+ with no_init_weights():
+ reward_model = seqcls_class(hf_cfg)
+ reward_model.model.load_state_dict(self.llm.state_dict())
+ reward_model.score.load_state_dict(self.v_head.state_dict())
+ reward_model.save_pretrained(save_dir, **save_pretrained_kwargs)
diff --git a/xtuner/model/sft.py b/xtuner/model/sft.py
index e1a29ab8a..f58e17c43 100644
--- a/xtuner/model/sft.py
+++ b/xtuner/model/sft.py
@@ -13,9 +13,12 @@
from transformers import AutoConfig, PreTrainedModel, PreTrainedTokenizer
from transformers.integrations import is_deepspeed_zero3_enabled
-from xtuner.parallel.sequence import (get_sequence_parallel_world_size,
- reduce_sequence_parallel_loss)
+from xtuner.parallel.sequence import (get_sequence_parallel_group,
+ get_sequence_parallel_world_size,
+ reduce_sequence_parallel_loss,
+ split_for_sequence_parallel)
from xtuner.registry import BUILDER
+from xtuner.utils.device import get_torch_device
from .modules import dispatch_modules
from .modules.dispatch import SUPPORT_FLASH1, SUPPORT_FLASH2
from .utils import (LoadWoInit, find_all_linear_names,
@@ -77,10 +80,9 @@ def __init__(self,
tokenizer=None,
max_position_embeddings=None):
super().__init__()
- with LoadWoInit():
- if isinstance(llm, dict):
- llm = self._dispatch_lm_model_cfg(llm, max_position_embeddings)
- self.llm = self._build_from_cfg_or_module(llm)
+
+ self.llm = self.build_llm_from_cfg(llm, use_varlen_attn,
+ max_position_embeddings)
if tokenizer is not None:
if isinstance(tokenizer, dict):
@@ -88,8 +90,6 @@ def __init__(self,
smart_tokenizer_and_embedding_resize(tokenizer, self.llm)
self.llm.config.use_cache = False
- dispatch_modules(self.llm, use_varlen_attn=use_varlen_attn)
-
if use_activation_checkpointing:
# For backward compatibility
if hasattr(self.llm, 'enable_input_require_grads'):
@@ -117,6 +117,19 @@ def __init__(self,
# the sequence.
self.use_varlen_attn = use_varlen_attn
+ def build_llm_from_cfg(self, llm_cfg, use_varlen_attn,
+ max_position_embeddings):
+ # For forward
+ with LoadWoInit():
+ if isinstance(llm_cfg, dict):
+ llm = self._dispatch_lm_model_cfg(llm_cfg,
+ max_position_embeddings)
+ llm = self._build_from_cfg_or_module(llm)
+
+ llm.config.use_cache = False
+ dispatch_modules(llm, use_varlen_attn=use_varlen_attn)
+ return llm
+
def gradient_checkpointing_enable(self):
self.activation_checkpointing_enable()
@@ -148,56 +161,84 @@ def init_weights(self):
@staticmethod
def _prepare_for_long_context_training(cfg, llm_cfg,
max_position_embeddings):
+ if not hasattr(llm_cfg, 'rope_scaling'):
+ print_log('Current model does not support RoPE scaling.',
+ 'current')
+ return
+
+ current_max_length = getattr(llm_cfg, 'max_position_embeddings', None)
+ if current_max_length and max_position_embeddings > current_max_length:
+ print_log(
+ f'Enlarge max model length from {current_max_length} '
+ f'to {max_position_embeddings}.', 'current')
+ scaling_factor = float(
+ math.ceil(max_position_embeddings / current_max_length))
+ else:
+ print_log(
+ 'The input `max_position_embeddings` is smaller than '
+ 'origin max length. Consider increase input length.',
+ 'current')
+ scaling_factor = 1.0
+ cfg.rope_scaling = {'type': 'linear', 'factor': scaling_factor}
- orig_rope_scaling = getattr(llm_cfg, 'rope_scaling', None)
- if orig_rope_scaling is None:
- orig_rope_scaling = {'factor': 1}
-
- orig_rope_scaling_factor = orig_rope_scaling[
- 'factor'] if 'factor' in orig_rope_scaling.keys() else 1
- orig_ctx_len = getattr(llm_cfg, 'max_position_embeddings', None)
- if orig_ctx_len:
- orig_ctx_len *= orig_rope_scaling_factor
- if max_position_embeddings > orig_ctx_len:
- scaling_factor = float(
- math.ceil(max_position_embeddings / orig_ctx_len))
- llm_cfg.rope_scaling = {
- 'type': 'linear',
- 'factor': scaling_factor
- }
-
- # hardcode for internlm2
- llm_cfg.attn_implementation = 'flash_attention_2'
- cfg.config = llm_cfg
-
- return cfg, llm_cfg
+ return cfg
@staticmethod
def _prepare_for_flash_attn(cfg, llm_cfg):
cls_name = type(llm_cfg).__name__
- SUPPORT_SDPA_ATTN = ('LlamaConfig', 'GemmaConfig', 'MistralConfig',
- 'MixtralConfig', 'Qwen2Config',
- 'Starcoder2Config', 'Starcoder2Config')
- SUPPORT_FLASH_ATTN2 = ('InternLM2Config', 'LlamaConfig', 'GemmaConfig',
- 'MistralConfig', 'MixtralConfig', 'Qwen2Config',
- 'Starcoder2Config', 'Starcoder2Config')
-
- if SUPPORT_FLASH2 and cls_name in SUPPORT_FLASH_ATTN2:
- cfg.torch_dtype = torch.bfloat16 \
- if torch.cuda.is_bf16_supported() else torch.float16
+ SUPPORT_SDPA_ATTN = ('InternLM3Config', 'LlamaConfig', 'GemmaConfig',
+ 'MistralConfig', 'MixtralConfig', 'Qwen2Config',
+ 'Qwen2MoeConfig', 'Starcoder2Config',
+ 'Starcoder2Config', 'Phi3Config')
+ SUPPORT_FLASH_ATTN2 = ('InternLM3Config', 'InternLM2Config',
+ 'LlamaConfig', 'GemmaConfig', 'MistralConfig',
+ 'MixtralConfig', 'Qwen2Config',
+ 'Qwen2MoeConfig', 'Starcoder2Config',
+ 'Starcoder2Config', 'Phi3Config',
+ 'DeepseekV2Config')
+
+ torch_dtype = torch.bfloat16 if (
+ get_torch_device().is_available() and get_torch_device().is_bf16_supported()) \
+ else torch.float16
+
+ if getattr(cfg, 'attn_implementation', None) is not None:
+ # Flash Attention 2.0 only supports torch.float16 and
+ # torch.bfloat16 dtypes
+ if cfg.attn_implementation == 'flash_attention_2':
+ cfg.torch_dtype = torch_dtype
+ elif SUPPORT_FLASH2 and cls_name in SUPPORT_FLASH_ATTN2:
+ cfg.torch_dtype = torch_dtype
cfg.attn_implementation = 'flash_attention_2'
elif SUPPORT_FLASH1 and cls_name in SUPPORT_SDPA_ATTN:
cfg.attn_implementation = 'sdpa'
- return cfg, llm_cfg
+ return cfg
+
+ @staticmethod
+ def _prepare_for_qlora_zero3(cfg):
+ if (not is_deepspeed_zero3_enabled()) or (not hasattr(
+ cfg, 'quantization_config')):
+ return cfg
+
+ torch_dtype = torch.bfloat16 if (
+ get_torch_device().is_available() and get_torch_device().is_bf16_supported()) \
+ else torch.float16
+
+ cfg.torch_dtype = torch_dtype
+ quantization_config = cfg.quantization_config
+ quantization_config.bnb_4bit_compute_dtype = torch_dtype
+ quantization_config.bnb_4bit_quant_storage = torch_dtype
+
+ return cfg
def _dispatch_lm_model_cfg(self, cfg, max_position_embeddings=None):
+ cfg = self._prepare_for_qlora_zero3(cfg)
pretrained_model_name_or_path = cfg.pretrained_model_name_or_path
llm_cfg = AutoConfig.from_pretrained(
pretrained_model_name_or_path, trust_remote_code=True)
- cfg, llm_cfg = self._prepare_for_flash_attn(cfg, llm_cfg)
+ cfg = self._prepare_for_flash_attn(cfg, llm_cfg)
if max_position_embeddings is not None:
- cfg, llm_cfg = self._prepare_for_long_context_training(
+ cfg = self._prepare_for_long_context_training(
cfg, llm_cfg, max_position_embeddings)
return cfg
@@ -232,16 +273,32 @@ def predict(self, data, data_samples=None):
logits_dict = [{'logits': logits} for logits in outputs.logits]
return logits_dict
- def compute_sequence_parallel_loss(self, data):
+ @staticmethod
+ def _split_for_sequence_parallel(data):
+ # attention mask should not be split
+ ARGS_NEED_TO_SPLIT = ('input_ids', 'labels', 'position_ids')
+ sp_group = get_sequence_parallel_group()
+ for key in ARGS_NEED_TO_SPLIT:
+ val = data.get(key, None)
+ if val is not None:
+ # `dim` is 1 as the shape of tensor is (bs, seq_len, ...)
+ data[key] = split_for_sequence_parallel(
+ val, dim=1, sp_group=sp_group)
+ return data
+
+ def _compute_sequence_parallel_loss(self, data):
+ data = self._split_for_sequence_parallel(data)
outputs = self.llm(**data)
labels = data['labels']
num_tokens = (labels != -100).sum()
- loss = reduce_sequence_parallel_loss(outputs.loss, num_tokens)
+ sp_group = get_sequence_parallel_group()
+ loss = reduce_sequence_parallel_loss(outputs.loss, num_tokens,
+ sp_group)
return {'loss': loss}
def compute_loss(self, data, data_samples=None):
if get_sequence_parallel_world_size() > 1:
- return self.compute_sequence_parallel_loss(data)
+ return self._compute_sequence_parallel_loss(data)
else:
outputs = self.llm(**data)
loss_dict = {'loss': outputs.loss}
@@ -259,3 +316,23 @@ def __getattr__(self, name: str):
return super().__getattr__(name)
except AttributeError:
return getattr(self.llm, name)
+
+ def to_hf(self,
+ cfg,
+ save_dir,
+ fp32=False,
+ save_pretrained_kwargs={},
+ **kwargs):
+ self.llm.config.use_cache = True
+ if not fp32:
+ print_log('Convert LLM to float16', 'current')
+ self.llm.half()
+ if self.use_lora:
+ print_log(f'Saving adapter to {save_dir}', 'current')
+ else:
+ print_log(f'Saving LLM tokenizer to {save_dir}', 'current')
+ tokenizer = BUILDER.build(cfg.tokenizer)
+ tokenizer.save_pretrained(save_dir)
+ print_log(f'Saving LLM to {save_dir}', 'current')
+ self.llm.save_pretrained(save_dir, **save_pretrained_kwargs)
+ self.llm.config.use_cache = False
diff --git a/xtuner/model/transformers_models/__init__.py b/xtuner/model/transformers_models/__init__.py
new file mode 100644
index 000000000..71f7ea1d4
--- /dev/null
+++ b/xtuner/model/transformers_models/__init__.py
@@ -0,0 +1,8 @@
+from .deepseek_v2 import (DeepseekTokenizerFast, DeepseekV2Config,
+ DeepseekV2ForCausalLM, DeepseekV2Model)
+from .mixtral import MixtralConfig, MixtralForCausalLM, MixtralModel
+
+__all__ = [
+ 'DeepseekTokenizerFast', 'DeepseekV2Config', 'DeepseekV2ForCausalLM',
+ 'DeepseekV2Model', 'MixtralConfig', 'MixtralForCausalLM', 'MixtralModel'
+]
diff --git a/xtuner/model/transformers_models/deepseek_v2/__init__.py b/xtuner/model/transformers_models/deepseek_v2/__init__.py
new file mode 100644
index 000000000..6a74b483c
--- /dev/null
+++ b/xtuner/model/transformers_models/deepseek_v2/__init__.py
@@ -0,0 +1,8 @@
+from .configuration_deepseek import DeepseekV2Config
+from .modeling_deepseek import DeepseekV2ForCausalLM, DeepseekV2Model
+from .tokenization_deepseek_fast import DeepseekTokenizerFast
+
+__all__ = [
+ 'DeepseekV2ForCausalLM', 'DeepseekV2Model', 'DeepseekV2Config',
+ 'DeepseekTokenizerFast'
+]
diff --git a/xtuner/model/transformers_models/deepseek_v2/configuration_deepseek.py b/xtuner/model/transformers_models/deepseek_v2/configuration_deepseek.py
new file mode 100644
index 000000000..daaddcf49
--- /dev/null
+++ b/xtuner/model/transformers_models/deepseek_v2/configuration_deepseek.py
@@ -0,0 +1,219 @@
+from transformers.configuration_utils import PretrainedConfig
+from transformers.utils import logging
+
+logger = logging.get_logger(__name__)
+
+DEEPSEEK_PRETRAINED_CONFIG_ARCHIVE_MAP = {}
+
+
+# Compared to the original version, two parameters, `moe_implementation` and
+# `expert_in_one_shard`, have been added.
+class DeepseekV2Config(PretrainedConfig):
+ r"""
+ This is the configuration class to store the configuration of a [`DeepseekV2Model`]. It is used to instantiate an DeepSeek
+ model according to the specified arguments, defining the model architecture. Instantiating a configuration with the
+ defaults will yield a similar configuration to that of the DeepSeek-V2.
+
+ Configuration objects inherit from [`PretrainedConfig`] and can be used to control the model outputs. Read the
+ documentation from [`PretrainedConfig`] for more information.
+
+
+ Args:
+ vocab_size (`int`, *optional*, defaults to 102400):
+ Vocabulary size of the Deep model. Defines the number of different tokens that can be represented by the
+ `inputs_ids` passed when calling [`DeepseekV2Model`]
+ hidden_size (`int`, *optional*, defaults to 4096):
+ Dimension of the hidden representations.
+ intermediate_size (`int`, *optional*, defaults to 11008):
+ Dimension of the MLP representations.
+ moe_intermediate_size (`int`, *optional*, defaults to 1407):
+ Dimension of the MoE representations.
+ num_hidden_layers (`int`, *optional*, defaults to 32):
+ Number of hidden layers in the Transformer decoder.
+ num_attention_heads (`int`, *optional*, defaults to 32):
+ Number of attention heads for each attention layer in the Transformer decoder.
+ n_shared_experts (`int`, *optional*, defaults to None):
+ Number of shared experts, None means dense model.
+ n_routed_experts (`int`, *optional*, defaults to None):
+ Number of routed experts, None means dense model.
+ routed_scaling_factor (`float`, *optional*, defaults to 1.0):
+ Scaling factor or routed experts.
+ topk_method (`str`, *optional*, defaults to `gready`):
+ Topk method used in routed gate.
+ n_group (`int`, *optional*, defaults to None):
+ Number of groups for routed experts.
+ topk_group (`int`, *optional*, defaults to None):
+ Number of selected groups for each token(for each token, ensuring the selected experts is only within `topk_group` groups).
+ num_experts_per_tok (`int`, *optional*, defaults to None):
+ Number of selected experts, None means dense model.
+ moe_layer_freq (`int`, *optional*, defaults to 1):
+ The frequency of the MoE layer: one expert layer for every `moe_layer_freq - 1` dense layers.
+ first_k_dense_replace (`int`, *optional*, defaults to 0):
+ Number of dense layers in shallow layers(embed->dense->dense->...->dense->moe->moe...->lm_head).
+ \--k dense layers--/
+ norm_topk_prob (`bool`, *optional*, defaults to False):
+ Whether to normalize the weights of the routed experts.
+ scoring_func (`str`, *optional*, defaults to 'softmax'):
+ Method of computing expert weights.
+ aux_loss_alpha (`float`, *optional*, defaults to 0.001):
+ Auxiliary loss weight coefficient.
+ seq_aux = (`bool`, *optional*, defaults to True):
+ Whether to compute the auxiliary loss for each individual sample.
+ num_key_value_heads (`int`, *optional*):
+ This is the number of key_value heads that should be used to implement Grouped Query Attention. If
+ `num_key_value_heads=num_attention_heads`, the model will use Multi Head Attention (MHA), if
+ `num_key_value_heads=1 the model will use Multi Query Attention (MQA) otherwise GQA is used. When
+ converting a multi-head checkpoint to a GQA checkpoint, each group key and value head should be constructed
+ by meanpooling all the original heads within that group. For more details checkout [this
+ paper](https://arxiv.org/pdf/2305.13245.pdf). If it is not specified, will default to
+ `num_attention_heads`.
+ hidden_act (`str` or `function`, *optional*, defaults to `"silu"`):
+ The non-linear activation function (function or string) in the decoder.
+ max_position_embeddings (`int`, *optional*, defaults to 2048):
+ The maximum sequence length that this model might ever be used with.
+ initializer_range (`float`, *optional*, defaults to 0.02):
+ The standard deviation of the truncated_normal_initializer for initializing all weight matrices.
+ rms_norm_eps (`float`, *optional*, defaults to 1e-06):
+ The epsilon used by the rms normalization layers.
+ use_cache (`bool`, *optional*, defaults to `True`):
+ Whether or not the model should return the last key/values attentions (not used by all models). Only
+ relevant if `config.is_decoder=True`.
+ pad_token_id (`int`, *optional*):
+ Padding token id.
+ bos_token_id (`int`, *optional*, defaults to 1):
+ Beginning of stream token id.
+ eos_token_id (`int`, *optional*, defaults to 2):
+ End of stream token id.
+ pretraining_tp (`int`, *optional*, defaults to 1):
+ Experimental feature. Tensor parallelism rank used during pretraining. Please refer to [this
+ document](https://huggingface.co/docs/transformers/parallelism) to understand more about it. This value is
+ necessary to ensure exact reproducibility of the pretraining results. Please refer to [this
+ issue](https://github.com/pytorch/pytorch/issues/76232).
+ tie_word_embeddings (`bool`, *optional*, defaults to `False`):
+ Whether to tie weight embeddings
+ rope_theta (`float`, *optional*, defaults to 10000.0):
+ The base period of the RoPE embeddings.
+ rope_scaling (`Dict`, *optional*):
+ Dictionary containing the scaling configuration for the RoPE embeddings. Currently supports two scaling
+ strategies: linear and dynamic. Their scaling factor must be a float greater than 1. The expected format is
+ `{"type": strategy name, "factor": scaling factor}`. When using this flag, don't update
+ `max_position_embeddings` to the expected new maximum.
+ attention_bias (`bool`, defaults to `False`, *optional*, defaults to `False`):
+ Whether to use a bias in the query, key, value and output projection layers during self-attention.
+ attention_dropout (`float`, *optional*, defaults to 0.0):
+ The dropout ratio for the attention probabilities.
+ moe_implementation (`str`, *optional*, defaults to 'origin'):
+ The implementation of the moe blocks. 'origin' or 'shard'.
+ expert_in_one_shard (`int`, *optional*, defaults to None):
+ How many expert models are integrated into a shard. It is used only
+ when `moe_implementation` == 'shard'
+
+ ```python
+ >>> from transformers import DeepseekV2Model, DeepseekV2Config
+
+ >>> # Initializing a Deepseek-V2 style configuration
+ >>> configuration = DeepseekV2Config()
+
+ >>> # Accessing the model configuration
+ >>> configuration = model.config
+ ```"""
+
+ model_type = 'deepseek_v2'
+ keys_to_ignore_at_inference = ['past_key_values']
+
+ def __init__(
+ self,
+ vocab_size=102400,
+ hidden_size=4096,
+ intermediate_size=11008,
+ moe_intermediate_size=1407,
+ num_hidden_layers=30,
+ num_attention_heads=32,
+ num_key_value_heads=32,
+ n_shared_experts=None,
+ n_routed_experts=None,
+ ep_size=1,
+ routed_scaling_factor=1.0,
+ kv_lora_rank=512,
+ q_lora_rank=1536,
+ qk_rope_head_dim=64,
+ v_head_dim=128,
+ qk_nope_head_dim=128,
+ topk_method='gready',
+ n_group=None,
+ topk_group=None,
+ num_experts_per_tok=None,
+ moe_layer_freq=1,
+ first_k_dense_replace=0,
+ norm_topk_prob=False,
+ scoring_func='softmax',
+ aux_loss_alpha=0.001,
+ seq_aux=True,
+ hidden_act='silu',
+ max_position_embeddings=2048,
+ initializer_range=0.02,
+ rms_norm_eps=1e-6,
+ use_cache=True,
+ pad_token_id=None,
+ bos_token_id=100000,
+ eos_token_id=100001,
+ pretraining_tp=1,
+ tie_word_embeddings=False,
+ rope_theta=10000.0,
+ rope_scaling=None,
+ attention_bias=False,
+ attention_dropout=0.0,
+ moe_implementation='origin',
+ expert_in_one_shard=None,
+ **kwargs,
+ ):
+ self.vocab_size = vocab_size
+ self.max_position_embeddings = max_position_embeddings
+ self.hidden_size = hidden_size
+ self.intermediate_size = intermediate_size
+ self.moe_intermediate_size = moe_intermediate_size
+ self.num_hidden_layers = num_hidden_layers
+ self.num_attention_heads = num_attention_heads
+ self.n_shared_experts = n_shared_experts
+ self.n_routed_experts = n_routed_experts
+ self.ep_size = ep_size
+ self.routed_scaling_factor = routed_scaling_factor
+ self.kv_lora_rank = kv_lora_rank
+ self.q_lora_rank = q_lora_rank
+ self.qk_rope_head_dim = qk_rope_head_dim
+ self.v_head_dim = v_head_dim
+ self.qk_nope_head_dim = qk_nope_head_dim
+ self.topk_method = topk_method
+ self.n_group = n_group
+ self.topk_group = topk_group
+ self.num_experts_per_tok = num_experts_per_tok
+ self.moe_layer_freq = moe_layer_freq
+ self.first_k_dense_replace = first_k_dense_replace
+ self.norm_topk_prob = norm_topk_prob
+ self.scoring_func = scoring_func
+ self.aux_loss_alpha = aux_loss_alpha
+ self.seq_aux = seq_aux
+ # for backward compatibility
+ if num_key_value_heads is None:
+ num_key_value_heads = num_attention_heads
+
+ self.num_key_value_heads = num_key_value_heads
+ self.hidden_act = hidden_act
+ self.initializer_range = initializer_range
+ self.rms_norm_eps = rms_norm_eps
+ self.pretraining_tp = pretraining_tp
+ self.use_cache = use_cache
+ self.rope_theta = rope_theta
+ self.rope_scaling = rope_scaling
+ self.attention_bias = attention_bias
+ self.attention_dropout = attention_dropout
+ self.moe_implementation = moe_implementation
+ self.expert_in_one_shard = expert_in_one_shard
+
+ super().__init__(
+ pad_token_id=pad_token_id,
+ bos_token_id=bos_token_id,
+ eos_token_id=eos_token_id,
+ tie_word_embeddings=tie_word_embeddings,
+ **kwargs,
+ )
diff --git a/xtuner/model/transformers_models/deepseek_v2/modeling_deepseek.py b/xtuner/model/transformers_models/deepseek_v2/modeling_deepseek.py
new file mode 100644
index 000000000..f58dd466f
--- /dev/null
+++ b/xtuner/model/transformers_models/deepseek_v2/modeling_deepseek.py
@@ -0,0 +1,2037 @@
+# Copyright 2023 DeepSeek-AI and The HuggingFace Inc. team. All rights reserved.
+#
+# This code is based on EleutherAI's GPT-NeoX library and the GPT-NeoX
+# and OPT implementations in this library. It has been modified from its
+# original forms to accommodate minor architectural differences compared
+# to GPT-NeoX and OPT used by the Meta AI team that trained the model.
+#
+# Licensed under the Apache License, Version 2.0 (the "License");
+# you may not use this file except in compliance with the License.
+# You may obtain a copy of the License at
+#
+# http://www.apache.org/licenses/LICENSE-2.0
+#
+# Unless required by applicable law or agreed to in writing, software
+# distributed under the License is distributed on an "AS IS" BASIS,
+# WITHOUT WARRANTIES OR CONDITIONS OF ANY KIND, either express or implied.
+# See the License for the specific language governing permissions and
+# limitations under the License.
+"""PyTorch DeepSeek model."""
+import copy
+import math
+import os
+import types
+import warnings
+from typing import List, Optional, Tuple, Union
+
+import numpy as np
+import torch
+import torch.distributed as dist
+import torch.nn.functional as F
+import torch.utils.checkpoint
+from torch import nn
+from torch.nn import BCEWithLogitsLoss, CrossEntropyLoss, MSELoss
+from transformers.activations import ACT2FN
+from transformers.cache_utils import Cache, DynamicCache
+from transformers.configuration_utils import PretrainedConfig
+from transformers.modeling_attn_mask_utils import (
+ AttentionMaskConverter, _prepare_4d_attention_mask,
+ _prepare_4d_causal_attention_mask,
+ _prepare_4d_causal_attention_mask_for_sdpa)
+from transformers.modeling_outputs import (BaseModelOutputWithPast,
+ CausalLMOutputWithPast,
+ SequenceClassifierOutputWithPast)
+from transformers.modeling_utils import PreTrainedModel
+from transformers.pytorch_utils import (ALL_LAYERNORM_LAYERS,
+ is_torch_greater_or_equal_than_1_13)
+from transformers.utils import (add_start_docstrings,
+ add_start_docstrings_to_model_forward,
+ is_flash_attn_2_available,
+ is_flash_attn_greater_or_equal_2_10, logging,
+ replace_return_docstrings)
+from transformers.utils.import_utils import is_torch_fx_available
+
+from xtuner.utils import load_state_dict_into_model
+from .configuration_deepseek import DeepseekV2Config
+
+if is_flash_attn_2_available():
+ from flash_attn import flash_attn_func, flash_attn_varlen_func
+ from flash_attn.bert_padding import pad_input # noqa
+ from flash_attn.bert_padding import index_first_axis, unpad_input
+
+# This makes `_prepare_4d_causal_attention_mask` a leaf function in the FX graph.
+# It means that the function will not be traced through and simply appear as a node in the graph.
+if is_torch_fx_available():
+ if not is_torch_greater_or_equal_than_1_13:
+ import torch.fx
+
+ _prepare_4d_causal_attention_mask = torch.fx.wrap(
+ _prepare_4d_causal_attention_mask)
+
+logger = logging.get_logger(__name__)
+
+_CONFIG_FOR_DOC = 'DeepseekV2Config'
+
+
+def _get_unpad_data(attention_mask):
+ seqlens_in_batch = attention_mask.sum(dim=-1, dtype=torch.int32)
+ indices = torch.nonzero(attention_mask.flatten(), as_tuple=False).flatten()
+ max_seqlen_in_batch = seqlens_in_batch.max().item()
+ cu_seqlens = F.pad(
+ torch.cumsum(seqlens_in_batch, dim=0, dtype=torch.torch.int32), (1, 0))
+ return (
+ indices,
+ cu_seqlens,
+ max_seqlen_in_batch,
+ )
+
+
+class DeepseekV2RMSNorm(nn.Module):
+
+ def __init__(self, hidden_size, eps=1e-6):
+ """DeepseekV2RMSNorm is equivalent to T5LayerNorm."""
+ super().__init__()
+ self.weight = nn.Parameter(torch.ones(hidden_size))
+ self.variance_epsilon = eps
+
+ def forward(self, hidden_states):
+ input_dtype = hidden_states.dtype
+ hidden_states = hidden_states.to(torch.float32)
+ variance = hidden_states.pow(2).mean(-1, keepdim=True)
+ hidden_states = hidden_states * torch.rsqrt(variance +
+ self.variance_epsilon)
+ return self.weight * hidden_states.to(input_dtype)
+
+
+ALL_LAYERNORM_LAYERS.append(DeepseekV2RMSNorm)
+
+
+class DeepseekV2RotaryEmbedding(nn.Module):
+
+ def __init__(self,
+ dim,
+ max_position_embeddings=2048,
+ base=10000,
+ device=None):
+ super().__init__()
+
+ self.dim = dim
+ self.max_position_embeddings = max_position_embeddings
+ self.base = base
+ inv_freq = 1.0 / (
+ self.base
+ **(torch.arange(0, self.dim, 2).float().to(device) / self.dim))
+ self.register_buffer('inv_freq', inv_freq, persistent=False)
+
+ # Build here to make `torch.jit.trace` work.
+ self._set_cos_sin_cache(
+ seq_len=max_position_embeddings,
+ device=self.inv_freq.device,
+ dtype=torch.get_default_dtype(),
+ )
+ self.max_seq_len_cached = None
+
+ def _set_cos_sin_cache(self, seq_len, device, dtype):
+ self.max_seq_len_cached = seq_len
+ t = torch.arange(
+ self.max_seq_len_cached, device=device, dtype=self.inv_freq.dtype)
+
+ freqs = torch.outer(t, self.inv_freq.to(t.device))
+ # Different from paper, but it uses a different permutation in order to obtain the same calculation
+ emb = torch.cat((freqs, freqs), dim=-1)
+ self.register_buffer(
+ 'cos_cached', emb.cos().to(dtype), persistent=False)
+ self.register_buffer(
+ 'sin_cached', emb.sin().to(dtype), persistent=False)
+
+ def forward(self, x, seq_len=None):
+ # x: [bs, num_attention_heads, seq_len, head_size]
+ if self.max_seq_len_cached is None or seq_len > self.max_seq_len_cached:
+ self._set_cos_sin_cache(
+ seq_len=seq_len, device=x.device, dtype=x.dtype)
+
+ return (
+ self.cos_cached[:seq_len].to(dtype=x.dtype),
+ self.sin_cached[:seq_len].to(dtype=x.dtype),
+ )
+
+
+# Copied from transformers.models.llama.modeling_llama.LlamaLinearScalingRotaryEmbedding with Llama->DeepseekV2
+class DeepseekV2LinearScalingRotaryEmbedding(DeepseekV2RotaryEmbedding):
+ """DeepseekV2RotaryEmbedding extended with linear scaling.
+
+ Credits to the Reddit user /u/kaiokendev
+ """
+
+ def __init__(
+ self,
+ dim,
+ max_position_embeddings=2048,
+ base=10000,
+ device=None,
+ scaling_factor=1.0,
+ ):
+ self.scaling_factor = scaling_factor
+ super().__init__(dim, max_position_embeddings, base, device)
+
+ def _set_cos_sin_cache(self, seq_len, device, dtype):
+ self.max_seq_len_cached = seq_len
+ t = torch.arange(
+ self.max_seq_len_cached, device=device, dtype=self.inv_freq.dtype)
+ t = t / self.scaling_factor
+
+ freqs = torch.outer(t, self.inv_freq)
+ # Different from paper, but it uses a different permutation in order to obtain the same calculation
+ emb = torch.cat((freqs, freqs), dim=-1)
+ self.register_buffer(
+ 'cos_cached', emb.cos().to(dtype), persistent=False)
+ self.register_buffer(
+ 'sin_cached', emb.sin().to(dtype), persistent=False)
+
+
+# Copied from transformers.models.llama.modeling_llama.LlamaDynamicNTKScalingRotaryEmbedding with Llama->DeepseekV2
+class DeepseekV2DynamicNTKScalingRotaryEmbedding(DeepseekV2RotaryEmbedding):
+ """DeepseekV2RotaryEmbedding extended with Dynamic NTK scaling.
+
+ Credits to the Reddit users /u/bloc97 and /u/emozilla
+ """
+
+ def __init__(
+ self,
+ dim,
+ max_position_embeddings=2048,
+ base=10000,
+ device=None,
+ scaling_factor=1.0,
+ ):
+ self.scaling_factor = scaling_factor
+ super().__init__(dim, max_position_embeddings, base, device)
+
+ def _set_cos_sin_cache(self, seq_len, device, dtype):
+ self.max_seq_len_cached = seq_len
+
+ if seq_len > self.max_position_embeddings:
+ base = self.base * ((self.scaling_factor * seq_len /
+ self.max_position_embeddings) -
+ (self.scaling_factor - 1))**(
+ self.dim / (self.dim - 2))
+ inv_freq = 1.0 / (
+ base
+ **(torch.arange(0, self.dim, 2).float().to(device) / self.dim))
+ self.register_buffer('inv_freq', inv_freq, persistent=False)
+
+ t = torch.arange(
+ self.max_seq_len_cached, device=device, dtype=self.inv_freq.dtype)
+
+ freqs = torch.outer(t, self.inv_freq)
+ # Different from paper, but it uses a different permutation in order to obtain the same calculation
+ emb = torch.cat((freqs, freqs), dim=-1)
+ self.register_buffer(
+ 'cos_cached', emb.cos().to(dtype), persistent=False)
+ self.register_buffer(
+ 'sin_cached', emb.sin().to(dtype), persistent=False)
+
+
+# Inverse dim formula to find dim based on number of rotations
+def yarn_find_correction_dim(num_rotations,
+ dim,
+ base=10000,
+ max_position_embeddings=2048):
+ return (dim * math.log(max_position_embeddings /
+ (num_rotations * 2 * math.pi))) / (2 *
+ math.log(base))
+
+
+# Find dim range bounds based on rotations
+def yarn_find_correction_range(low_rot,
+ high_rot,
+ dim,
+ base=10000,
+ max_position_embeddings=2048):
+ low = math.floor(
+ yarn_find_correction_dim(low_rot, dim, base, max_position_embeddings))
+ high = math.ceil(
+ yarn_find_correction_dim(high_rot, dim, base, max_position_embeddings))
+ return max(low, 0), min(high, dim - 1) # Clamp values just in case
+
+
+def yarn_get_mscale(scale=1, mscale=1):
+ if scale <= 1:
+ return 1.0
+ return 0.1 * mscale * math.log(scale) + 1.0
+
+
+def yarn_linear_ramp_mask(min, max, dim):
+ if min == max:
+ max += 0.001 # Prevent singularity
+
+ linear_func = (torch.arange(dim, dtype=torch.float32) - min) / (max - min)
+ ramp_func = torch.clamp(linear_func, 0, 1)
+ return ramp_func
+
+
+class DeepseekV2YarnRotaryEmbedding(DeepseekV2RotaryEmbedding):
+
+ def __init__(
+ self,
+ dim,
+ max_position_embeddings=2048,
+ base=10000,
+ device=None,
+ scaling_factor=1.0,
+ original_max_position_embeddings=4096,
+ beta_fast=32,
+ beta_slow=1,
+ mscale=1,
+ mscale_all_dim=0,
+ ):
+ self.scaling_factor = scaling_factor
+ self.original_max_position_embeddings = original_max_position_embeddings
+ self.beta_fast = beta_fast
+ self.beta_slow = beta_slow
+ self.mscale = mscale
+ self.mscale_all_dim = mscale_all_dim
+ super().__init__(dim, max_position_embeddings, base, device)
+
+ def _set_cos_sin_cache(self, seq_len, device, dtype):
+ self.max_seq_len_cached = seq_len
+ dim = self.dim
+
+ freq_extra = 1.0 / (
+ self.base**(torch.arange(
+ 0, dim, 2, dtype=torch.float32, device=device) / dim))
+ freq_inter = 1.0 / (
+ self.scaling_factor * self.base**(torch.arange(
+ 0, dim, 2, dtype=torch.float32, device=device) / dim))
+
+ low, high = yarn_find_correction_range(
+ self.beta_fast,
+ self.beta_slow,
+ dim,
+ self.base,
+ self.original_max_position_embeddings,
+ )
+ inv_freq_mask = 1.0 - yarn_linear_ramp_mask(low, high, dim // 2).to(
+ device=device, dtype=torch.float32)
+ inv_freq = freq_inter * (1 -
+ inv_freq_mask) + freq_extra * inv_freq_mask
+ self.register_buffer('inv_freq', inv_freq, persistent=False)
+
+ t = torch.arange(seq_len, device=device, dtype=torch.float32)
+
+ freqs = torch.outer(t, inv_freq)
+
+ _mscale = float(
+ yarn_get_mscale(self.scaling_factor, self.mscale) /
+ yarn_get_mscale(self.scaling_factor, self.mscale_all_dim))
+
+ emb = torch.cat((freqs, freqs), dim=-1)
+ self.register_buffer(
+ 'cos_cached', (emb.cos() * _mscale).to(dtype), persistent=False)
+ self.register_buffer(
+ 'sin_cached', (emb.sin() * _mscale).to(dtype), persistent=False)
+
+
+# Copied from transformers.models.llama.modeling_llama.rotate_half
+def rotate_half(x):
+ """Rotates half the hidden dims of the input."""
+ x1 = x[..., :x.shape[-1] // 2]
+ x2 = x[..., x.shape[-1] // 2:]
+ return torch.cat((-x2, x1), dim=-1)
+
+
+# Copied from transformers.models.llama.modeling_llama.apply_rotary_pos_emb
+def apply_rotary_pos_emb(q, k, cos, sin, position_ids, unsqueeze_dim=1):
+ """Applies Rotary Position Embedding to the query and key tensors.
+
+ Args:
+ q (`torch.Tensor`): The query tensor.
+ k (`torch.Tensor`): The key tensor.
+ cos (`torch.Tensor`): The cosine part of the rotary embedding.
+ sin (`torch.Tensor`): The sine part of the rotary embedding.
+ position_ids (`torch.Tensor`):
+ The position indices of the tokens corresponding to the query and key tensors. For example, this can be
+ used to pass offsetted position ids when working with a KV-cache.
+ unsqueeze_dim (`int`, *optional*, defaults to 1):
+ The 'unsqueeze_dim' argument specifies the dimension along which to unsqueeze cos[position_ids] and
+ sin[position_ids] so that they can be properly broadcasted to the dimensions of q and k. For example, note
+ that cos[position_ids] and sin[position_ids] have the shape [batch_size, seq_len, head_dim]. Then, if q and
+ k have the shape [batch_size, heads, seq_len, head_dim], then setting unsqueeze_dim=1 makes
+ cos[position_ids] and sin[position_ids] broadcastable to the shapes of q and k. Similarly, if q and k have
+ the shape [batch_size, seq_len, heads, head_dim], then set unsqueeze_dim=2.
+ Returns:
+ `tuple(torch.Tensor)` comprising of the query and key tensors rotated using the Rotary Position Embedding.
+ """
+ cos = cos[position_ids].unsqueeze(unsqueeze_dim)
+ sin = sin[position_ids].unsqueeze(unsqueeze_dim)
+
+ b, h, s, d = q.shape
+ q = q.view(b, h, s, d // 2, 2).transpose(4, 3).reshape(b, h, s, d)
+
+ b, h, s, d = k.shape
+ k = k.view(b, h, s, d // 2, 2).transpose(4, 3).reshape(b, h, s, d)
+
+ q_embed = (q * cos) + (rotate_half(q) * sin)
+ k_embed = (k * cos) + (rotate_half(k) * sin)
+ return q_embed, k_embed
+
+
+class DeepseekV2MLP(nn.Module):
+
+ def __init__(self, config, hidden_size=None, intermediate_size=None):
+ super().__init__()
+ self.config = config
+ self.hidden_size = config.hidden_size if hidden_size is None else hidden_size
+ self.intermediate_size = (
+ config.intermediate_size
+ if intermediate_size is None else intermediate_size)
+
+ self.gate_proj = nn.Linear(
+ self.hidden_size, self.intermediate_size, bias=False)
+ self.up_proj = nn.Linear(
+ self.hidden_size, self.intermediate_size, bias=False)
+ self.down_proj = nn.Linear(
+ self.intermediate_size, self.hidden_size, bias=False)
+ self.act_fn = ACT2FN[config.hidden_act]
+
+ def forward(self, x):
+ down_proj = self.down_proj(
+ self.act_fn(self.gate_proj(x)) * self.up_proj(x))
+ return down_proj
+
+
+class MoEGate(nn.Module):
+
+ def __init__(self, config):
+ super().__init__()
+ self.config = config
+ self.top_k = config.num_experts_per_tok
+ self.n_routed_experts = config.n_routed_experts
+ self.routed_scaling_factor = config.routed_scaling_factor
+ self.scoring_func = config.scoring_func
+ self.alpha = config.aux_loss_alpha
+ self.seq_aux = config.seq_aux
+ self.topk_method = config.topk_method
+ self.n_group = config.n_group
+ self.topk_group = config.topk_group
+
+ # topk selection algorithm
+ self.norm_topk_prob = config.norm_topk_prob
+ self.gating_dim = config.hidden_size
+ self.weight = nn.Parameter(
+ torch.empty((self.n_routed_experts, self.gating_dim)))
+ self.reset_parameters()
+
+ def reset_parameters(self) -> None:
+ import torch.nn.init as init
+
+ init.kaiming_uniform_(self.weight, a=math.sqrt(5))
+
+ def forward(self, hidden_states):
+ bsz, seq_len, h = hidden_states.shape
+ ### compute gating score
+ hidden_states = hidden_states.view(-1, h)
+ logits = F.linear(
+ hidden_states.type(torch.float32), self.weight.type(torch.float32),
+ None)
+ if self.scoring_func == 'softmax':
+ scores = logits.softmax(dim=-1, dtype=torch.float32)
+ else:
+ raise NotImplementedError(
+ f'insupportable scoring function for MoE gating: {self.scoring_func}'
+ )
+
+ ### select top-k experts
+ # fix official typos
+ if self.topk_method in ('gready', 'greedy'):
+ topk_weight, topk_idx = torch.topk(
+ scores, k=self.top_k, dim=-1, sorted=False)
+ elif self.topk_method == 'group_limited_greedy':
+ group_scores = (scores.view(bsz * seq_len, self.n_group,
+ -1).max(dim=-1).values) # [n, n_group]
+ group_idx = torch.topk(
+ group_scores, k=self.topk_group, dim=-1,
+ sorted=False)[1] # [n, top_k_group]
+ group_mask = torch.zeros_like(group_scores) # [n, n_group]
+ group_mask.scatter_(1, group_idx, 1) # [n, n_group]
+ score_mask = (group_mask.unsqueeze(-1).expand(
+ bsz * seq_len, self.n_group,
+ self.n_routed_experts // self.n_group).reshape(
+ bsz * seq_len, -1)) # [n, e]
+ tmp_scores = scores.masked_fill(~score_mask.bool(), 0.0) # [n, e]
+ topk_weight, topk_idx = torch.topk(
+ tmp_scores, k=self.top_k, dim=-1, sorted=False)
+
+ ### norm gate to sum 1
+ if self.top_k > 1 and self.norm_topk_prob:
+ denominator = topk_weight.sum(dim=-1, keepdim=True) + 1e-20
+ topk_weight = topk_weight / denominator
+ else:
+ topk_weight = topk_weight * self.routed_scaling_factor
+ ### expert-level computation auxiliary loss
+ if self.training and self.alpha > 0.0:
+ scores_for_aux = scores
+ aux_topk = self.top_k
+ # always compute aux loss based on the naive greedy topk method
+ topk_idx_for_aux_loss = topk_idx.view(bsz, -1)
+ if self.seq_aux:
+ scores_for_seq_aux = scores_for_aux.view(bsz, seq_len, -1)
+ ce = torch.zeros(
+ bsz, self.n_routed_experts, device=hidden_states.device)
+ ce.scatter_add_(
+ 1,
+ topk_idx_for_aux_loss,
+ torch.ones(
+ bsz, seq_len * aux_topk, device=hidden_states.device),
+ ).div_(seq_len * aux_topk / self.n_routed_experts)
+ aux_loss = (ce * scores_for_seq_aux.mean(dim=1)).sum(
+ dim=1).mean() * self.alpha
+ else:
+ mask_ce = F.one_hot(
+ topk_idx_for_aux_loss.view(-1),
+ num_classes=self.n_routed_experts)
+ ce = mask_ce.float().mean(0)
+ Pi = scores_for_aux.mean(0)
+ fi = ce * self.n_routed_experts
+ aux_loss = (Pi * fi).sum() * self.alpha
+ else:
+ aux_loss = None
+ return topk_idx, topk_weight, aux_loss
+
+
+class AddAuxiliaryLoss(torch.autograd.Function):
+ """The trick function of adding auxiliary (aux) loss, which includes the
+ gradient of the aux loss during backpropagation."""
+
+ @staticmethod
+ def forward(ctx, x, loss):
+ assert loss.numel() == 1
+ ctx.dtype = loss.dtype
+ ctx.required_aux_loss = loss.requires_grad
+ return x
+
+ @staticmethod
+ def backward(ctx, grad_output):
+ grad_loss = None
+ if ctx.required_aux_loss:
+ grad_loss = torch.ones(
+ 1, dtype=ctx.dtype, device=grad_output.device)
+ return grad_output, grad_loss
+
+
+class ExpertShard(nn.Module):
+
+ def __init__(self, config, shard_idx, expert_in_one_shard=10):
+ super().__init__()
+ hidden_dim = config.hidden_size
+ ffn_dim = config.moe_intermediate_size
+ self.w1w3 = nn.Parameter(
+ torch.empty(expert_in_one_shard, ffn_dim * 2, hidden_dim))
+ self.w2 = nn.Parameter(
+ torch.empty(expert_in_one_shard, hidden_dim, ffn_dim))
+
+ self.act = nn.SiLU()
+ self.expert_in_one_shard = expert_in_one_shard
+ self.shard_idx = shard_idx
+
+ self.reset_parameters()
+
+ def reset_parameters(self) -> None:
+ # Different from nn.Linear module, weights of self.w1w3 and self.w2
+ # can not be initialized by DeepseekV2PreTrainedModel._init_weights method
+ self.w1w3.data.normal_(0, 0.02)
+ self.w2.data.normal_(0, 0.02)
+
+ def expert_forward(self, current_state, expert_idx):
+ w1w3 = self.w1w3[expert_idx]
+ w2 = self.w2[expert_idx]
+ gate_up_out = torch.matmul(current_state, w1w3.T)
+ gate_out, up_out = gate_up_out.chunk(2, dim=-1)
+ gate_out = self.act(gate_out)
+ out = gate_out * up_out
+ out = torch.matmul(out, w2.T)
+ return out
+
+ def forward(self, hidden_states, flat_topk_idx, y):
+ for i in range(self.expert_in_one_shard):
+ expert_idx = i + self.expert_in_one_shard * self.shard_idx
+ y[flat_topk_idx == expert_idx] = self.expert_forward(
+ hidden_states[flat_topk_idx == expert_idx], i)
+ return y
+
+
+class DeepseekV2MoEShard(nn.Module):
+ """A mixed expert module containing shared experts."""
+
+ def __init__(self, config):
+ super().__init__()
+ self.config = config
+ self.num_experts_per_tok = config.num_experts_per_tok
+
+ if hasattr(config, 'ep_size') and config.ep_size > 1:
+ raise NotImplementedError
+ else:
+ self.ep_size = 1
+ self.experts_per_rank = config.n_routed_experts
+ self.ep_rank = 0
+ self.n_routed_experts = config.n_routed_experts
+
+ expert_in_one_shard = config.expert_in_one_shard
+ assert config.n_routed_experts % expert_in_one_shard == 0, \
+ ('n_routed_experts should be divisible by expert_in_one_shard, but got '
+ f'n_routed_experts = {config.n_routed_experts} and expert_in_one_shard = {expert_in_one_shard}')
+
+ self.shard_num = config.n_routed_experts // expert_in_one_shard
+ self.expert_in_one_shard = expert_in_one_shard
+ self.experts = nn.ModuleList([
+ ExpertShard(config, i, self.expert_in_one_shard)
+ for i in range(self.shard_num)
+ ])
+
+ self.gate = MoEGate(config)
+ if config.n_shared_experts is not None:
+ intermediate_size = config.moe_intermediate_size * config.n_shared_experts
+ self.shared_experts = DeepseekV2MLP(
+ config=config, intermediate_size=intermediate_size)
+
+ def forward(self, hidden_states):
+ if not self.training:
+ raise NotImplementedError
+
+ identity = hidden_states
+ orig_shape = hidden_states.shape
+ topk_idx, topk_weight, aux_loss = self.gate(hidden_states)
+ hidden_states = hidden_states.view(-1, hidden_states.shape[-1])
+ flat_topk_idx = topk_idx.view(-1)
+
+ hidden_states = hidden_states.repeat_interleave(
+ self.num_experts_per_tok, dim=0)
+ y = torch.empty_like(hidden_states)
+ y_dtype = y.dtype
+ for shard_index in range(self.shard_num):
+ y = self.experts[shard_index](hidden_states, flat_topk_idx, y)
+ y = ((y.view(*topk_weight.shape, -1) *
+ topk_weight.unsqueeze(-1)).sum(dim=1)).type(y_dtype)
+ y = y.view(*orig_shape)
+ y = AddAuxiliaryLoss.apply(y, aux_loss)
+
+ if self.config.n_shared_experts is not None:
+ y = y + self.shared_experts(identity)
+ return y
+
+
+class DeepseekV2MoE(nn.Module):
+ """A mixed expert module containing shared experts."""
+
+ def __init__(self, config):
+ super().__init__()
+ self.config = config
+ self.num_experts_per_tok = config.num_experts_per_tok
+
+ if hasattr(config, 'ep_size') and config.ep_size > 1:
+ assert config.ep_size == dist.get_world_size()
+ self.ep_size = config.ep_size
+ self.experts_per_rank = config.n_routed_experts // config.ep_size
+ self.ep_rank = dist.get_rank()
+ self.experts = nn.ModuleList([
+ (DeepseekV2MLP(
+ config, intermediate_size=config.moe_intermediate_size)
+ if i >= self.ep_rank * self.experts_per_rank and i <
+ (self.ep_rank + 1) * self.experts_per_rank else None)
+ for i in range(config.n_routed_experts)
+ ])
+ else:
+ self.ep_size = 1
+ self.experts_per_rank = config.n_routed_experts
+ self.ep_rank = 0
+ self.experts = nn.ModuleList([
+ DeepseekV2MLP(
+ config, intermediate_size=config.moe_intermediate_size)
+ for i in range(config.n_routed_experts)
+ ])
+ self.gate = MoEGate(config)
+ if config.n_shared_experts is not None:
+ intermediate_size = config.moe_intermediate_size * config.n_shared_experts
+ self.shared_experts = DeepseekV2MLP(
+ config=config, intermediate_size=intermediate_size)
+
+ def forward(self, hidden_states):
+ identity = hidden_states
+ orig_shape = hidden_states.shape
+ topk_idx, topk_weight, aux_loss = self.gate(hidden_states)
+ hidden_states = hidden_states.view(-1, hidden_states.shape[-1])
+ flat_topk_idx = topk_idx.view(-1)
+ if self.training:
+ hidden_states = hidden_states.repeat_interleave(
+ self.num_experts_per_tok, dim=0)
+ y = torch.empty_like(hidden_states)
+ y_dtype = y.dtype
+ for i, expert in enumerate(self.experts):
+ y[flat_topk_idx == i] = expert(
+ hidden_states[flat_topk_idx == i])
+ y = ((y.view(*topk_weight.shape, -1) *
+ topk_weight.unsqueeze(-1)).sum(dim=1)).type(y_dtype)
+ y = y.view(*orig_shape)
+ y = AddAuxiliaryLoss.apply(y, aux_loss)
+ else:
+ y = self.moe_infer(hidden_states, topk_idx,
+ topk_weight).view(*orig_shape)
+ if self.config.n_shared_experts is not None:
+ y = y + self.shared_experts(identity)
+ return y
+
+ @torch.no_grad()
+ def moe_infer(self, x, topk_ids, topk_weight):
+ cnts = topk_ids.new_zeros((topk_ids.shape[0], len(self.experts)))
+ cnts.scatter_(1, topk_ids, 1)
+ tokens_per_expert = cnts.sum(dim=0)
+ idxs = topk_ids.view(-1).argsort()
+ sorted_tokens = x[idxs // topk_ids.shape[1]]
+ sorted_tokens_shape = sorted_tokens.shape
+ if self.ep_size > 1:
+ tokens_per_ep_rank = tokens_per_expert.view(self.ep_size,
+ -1).sum(dim=1)
+ tokens_per_expert_group = tokens_per_expert.new_empty(
+ tokens_per_expert.shape[0])
+ dist.all_to_all_single(tokens_per_expert_group, tokens_per_expert)
+ output_splits = (
+ tokens_per_expert_group.view(self.ep_size,
+ -1).sum(1).cpu().numpy().tolist())
+ gathered_tokens = sorted_tokens.new_empty(
+ tokens_per_expert_group.sum(dim=0).cpu().item(),
+ sorted_tokens.shape[1])
+ input_split_sizes = tokens_per_ep_rank.cpu().numpy().tolist()
+ dist.all_to_all(
+ list(gathered_tokens.split(output_splits)),
+ list(sorted_tokens.split(input_split_sizes)),
+ )
+ tokens_per_expert_post_gather = tokens_per_expert_group.view(
+ self.ep_size, self.experts_per_rank).sum(dim=0)
+ gatherd_idxs = np.zeros(
+ shape=(gathered_tokens.shape[0], ), dtype=np.int32)
+ s = 0
+ for i, k in enumerate(tokens_per_expert_group.cpu().numpy()):
+ gatherd_idxs[s:s + k] = i % self.experts_per_rank
+ s += k
+ gatherd_idxs = gatherd_idxs.argsort()
+ sorted_tokens = gathered_tokens[gatherd_idxs]
+ tokens_per_expert = tokens_per_expert_post_gather
+ tokens_per_expert = tokens_per_expert.cpu().numpy()
+
+ outputs = []
+ start_idx = 0
+ for i, num_tokens in enumerate(tokens_per_expert):
+ end_idx = start_idx + num_tokens
+ if num_tokens == 0:
+ continue
+ expert = self.experts[i + self.ep_rank * self.experts_per_rank]
+ tokens_for_this_expert = sorted_tokens[start_idx:end_idx]
+ expert_out = expert(tokens_for_this_expert)
+ outputs.append(expert_out)
+ start_idx = end_idx
+
+ outs = torch.cat(
+ outputs, dim=0) if len(outputs) else sorted_tokens.new_empty(0)
+ if self.ep_size > 1:
+ new_x = torch.empty_like(outs)
+ new_x[gatherd_idxs] = outs
+ gathered_tokens = new_x.new_empty(*sorted_tokens_shape)
+ dist.all_to_all(
+ list(gathered_tokens.split(input_split_sizes)),
+ list(new_x.split(output_splits)),
+ )
+ outs = gathered_tokens
+
+ new_x = torch.empty_like(outs)
+ new_x[idxs] = outs
+ final_out = (
+ new_x.view(*topk_ids.shape, -1).type(topk_weight.dtype).mul_(
+ topk_weight.unsqueeze(dim=-1)).sum(dim=1).type(new_x.dtype))
+ return final_out
+
+
+# Copied from transformers.models.llama.modeling_llama.repeat_kv
+def repeat_kv(hidden_states: torch.Tensor, n_rep: int) -> torch.Tensor:
+ """This is the equivalent of torch.repeat_interleave(x, dim=1,
+ repeats=n_rep).
+
+ The hidden states go from (batch, num_key_value_heads, seqlen, head_dim) to
+ (batch, num_attention_heads, seqlen, head_dim)
+ """
+ batch, num_key_value_heads, slen, head_dim = hidden_states.shape
+ if n_rep == 1:
+ return hidden_states
+ hidden_states = hidden_states[:, :,
+ None, :, :].expand(batch,
+ num_key_value_heads,
+ n_rep, slen, head_dim)
+ return hidden_states.reshape(batch, num_key_value_heads * n_rep, slen,
+ head_dim)
+
+
+# Copied from transformers.models.llama.modeling_llama.LlamaAttention with Llama->DeepseekV2
+class DeepseekV2Attention(nn.Module):
+ """Multi-headed attention from 'Attention Is All You Need' paper."""
+
+ def __init__(self,
+ config: DeepseekV2Config,
+ layer_idx: Optional[int] = None):
+ super().__init__()
+ self.config = config
+ self.layer_idx = layer_idx
+ if layer_idx is None:
+ logger.warning_once(
+ f'Instantiating {self.__class__.__name__} without passing `layer_idx` is not recommended and will '
+ 'to errors during the forward call, if caching is used. Please make sure to provide a `layer_idx` '
+ 'when creating this class.')
+
+ self.attention_dropout = config.attention_dropout
+ self.hidden_size = config.hidden_size
+ self.num_heads = config.num_attention_heads
+
+ self.max_position_embeddings = config.max_position_embeddings
+ self.rope_theta = config.rope_theta
+ self.q_lora_rank = config.q_lora_rank
+ self.qk_rope_head_dim = config.qk_rope_head_dim
+ self.kv_lora_rank = config.kv_lora_rank
+ self.v_head_dim = config.v_head_dim
+ self.qk_nope_head_dim = config.qk_nope_head_dim
+ self.q_head_dim = config.qk_nope_head_dim + config.qk_rope_head_dim
+
+ self.is_causal = True
+
+ if self.q_lora_rank is None:
+ self.q_proj = nn.Linear(
+ self.hidden_size, self.num_heads * self.q_head_dim, bias=False)
+ else:
+ self.q_a_proj = nn.Linear(
+ self.hidden_size,
+ config.q_lora_rank,
+ bias=config.attention_bias)
+ self.q_a_layernorm = DeepseekV2RMSNorm(config.q_lora_rank)
+ self.q_b_proj = nn.Linear(
+ config.q_lora_rank,
+ self.num_heads * self.q_head_dim,
+ bias=False)
+
+ self.kv_a_proj_with_mqa = nn.Linear(
+ self.hidden_size,
+ config.kv_lora_rank + config.qk_rope_head_dim,
+ bias=config.attention_bias,
+ )
+ self.kv_a_layernorm = DeepseekV2RMSNorm(config.kv_lora_rank)
+ self.kv_b_proj = nn.Linear(
+ config.kv_lora_rank,
+ self.num_heads *
+ (self.q_head_dim - self.qk_rope_head_dim + self.v_head_dim),
+ bias=False,
+ )
+
+ self.o_proj = nn.Linear(
+ self.num_heads * self.v_head_dim,
+ self.hidden_size,
+ bias=config.attention_bias,
+ )
+ self._init_rope()
+
+ self.softmax_scale = self.q_head_dim**(-0.5)
+ if self.config.rope_scaling is not None:
+ mscale_all_dim = self.config.rope_scaling.get('mscale_all_dim', 0)
+ scaling_factor = self.config.rope_scaling['factor']
+ if mscale_all_dim:
+ mscale = yarn_get_mscale(scaling_factor, mscale_all_dim)
+ self.softmax_scale = self.softmax_scale * mscale * mscale
+
+ def _init_rope(self):
+ if self.config.rope_scaling is None:
+ self.rotary_emb = DeepseekV2RotaryEmbedding(
+ self.qk_rope_head_dim,
+ max_position_embeddings=self.max_position_embeddings,
+ base=self.rope_theta,
+ )
+ else:
+ scaling_type = self.config.rope_scaling['type']
+ scaling_factor = self.config.rope_scaling['factor']
+ if scaling_type == 'linear':
+ self.rotary_emb = DeepseekV2LinearScalingRotaryEmbedding(
+ self.qk_rope_head_dim,
+ max_position_embeddings=self.max_position_embeddings,
+ scaling_factor=scaling_factor,
+ base=self.rope_theta,
+ )
+ elif scaling_type == 'dynamic':
+ self.rotary_emb = DeepseekV2DynamicNTKScalingRotaryEmbedding(
+ self.qk_rope_head_dim,
+ max_position_embeddings=self.max_position_embeddings,
+ scaling_factor=scaling_factor,
+ base=self.rope_theta,
+ )
+ elif scaling_type == 'yarn':
+ kwargs = {
+ key: self.config.rope_scaling[key]
+ for key in [
+ 'original_max_position_embeddings',
+ 'beta_fast',
+ 'beta_slow',
+ 'mscale',
+ 'mscale_all_dim',
+ ] if key in self.config.rope_scaling
+ }
+ self.rotary_emb = DeepseekV2YarnRotaryEmbedding(
+ self.qk_rope_head_dim,
+ max_position_embeddings=self.max_position_embeddings,
+ scaling_factor=scaling_factor,
+ base=self.rope_theta,
+ **kwargs,
+ )
+ else:
+ raise ValueError(f'Unknown RoPE scaling type {scaling_type}')
+
+ def _shape(self, tensor: torch.Tensor, seq_len: int, bsz: int):
+ return (tensor.view(bsz, seq_len, self.num_heads,
+ self.v_head_dim).transpose(1, 2).contiguous())
+
+ def forward(
+ self,
+ hidden_states: torch.Tensor,
+ attention_mask: Optional[torch.Tensor] = None,
+ position_ids: Optional[torch.LongTensor] = None,
+ past_key_value: Optional[Cache] = None,
+ output_attentions: bool = False,
+ use_cache: bool = False,
+ **kwargs,
+ ) -> Tuple[torch.Tensor, Optional[torch.Tensor],
+ Optional[Tuple[torch.Tensor]]]:
+ if 'padding_mask' in kwargs:
+ warnings.warn(
+ 'Passing `padding_mask` is deprecated and will be removed in v4.37. Please make sure use `attention_mask` instead.`'
+ )
+ bsz, q_len, _ = hidden_states.size()
+
+ if self.q_lora_rank is None:
+ q = self.q_proj(hidden_states)
+ else:
+ q = self.q_b_proj(self.q_a_layernorm(self.q_a_proj(hidden_states)))
+ q = q.view(bsz, q_len, self.num_heads, self.q_head_dim).transpose(1, 2)
+ q_nope, q_pe = torch.split(
+ q, [self.qk_nope_head_dim, self.qk_rope_head_dim], dim=-1)
+
+ compressed_kv = self.kv_a_proj_with_mqa(hidden_states)
+ compressed_kv, k_pe = torch.split(
+ compressed_kv, [self.kv_lora_rank, self.qk_rope_head_dim], dim=-1)
+ k_pe = k_pe.view(bsz, q_len, 1, self.qk_rope_head_dim).transpose(1, 2)
+ kv = (
+ self.kv_b_proj(self.kv_a_layernorm(compressed_kv)).view(
+ bsz, q_len, self.num_heads,
+ self.qk_nope_head_dim + self.v_head_dim).transpose(1, 2))
+
+ k_nope, value_states = torch.split(
+ kv, [self.qk_nope_head_dim, self.v_head_dim], dim=-1)
+ kv_seq_len = value_states.shape[-2]
+ if past_key_value is not None:
+ if self.layer_idx is None:
+ raise ValueError(
+ f'The cache structure has changed since version v4.36. If you are using {self.__class__.__name__} '
+ 'for auto-regressive decoding with k/v caching, please make sure to initialize the attention class '
+ 'with a layer index.')
+ kv_seq_len += past_key_value.get_usable_length(
+ kv_seq_len, self.layer_idx)
+ cos, sin = self.rotary_emb(value_states, seq_len=kv_seq_len)
+
+ q_pe, k_pe = apply_rotary_pos_emb(q_pe, k_pe, cos, sin, position_ids)
+
+ query_states = k_pe.new_empty(bsz, self.num_heads, q_len,
+ self.q_head_dim)
+ query_states[:, :, :, :self.qk_nope_head_dim] = q_nope
+ query_states[:, :, :, self.qk_nope_head_dim:] = q_pe
+
+ key_states = k_pe.new_empty(bsz, self.num_heads, q_len,
+ self.q_head_dim)
+ key_states[:, :, :, :self.qk_nope_head_dim] = k_nope
+ key_states[:, :, :, self.qk_nope_head_dim:] = k_pe
+ if past_key_value is not None:
+ cache_kwargs = {'sin': sin, 'cos': cos} # Specific to RoPE models
+ key_states, value_states = past_key_value.update(
+ key_states, value_states, self.layer_idx, cache_kwargs)
+
+ attn_weights = (
+ torch.matmul(query_states, key_states.transpose(2, 3)) *
+ self.softmax_scale)
+
+ if attn_weights.size() != (bsz, self.num_heads, q_len, kv_seq_len):
+ raise ValueError(
+ f'Attention weights should be of size {(bsz, self.num_heads, q_len, kv_seq_len)}, but is'
+ f' {attn_weights.size()}')
+ assert attention_mask is not None
+ if attention_mask is not None:
+ if attention_mask.size() != (bsz, 1, q_len, kv_seq_len):
+ raise ValueError(
+ f'Attention mask should be of size {(bsz, 1, q_len, kv_seq_len)}, but is {attention_mask.size()}'
+ )
+ attn_weights = attn_weights + attention_mask
+
+ # upcast attention to fp32
+ attn_weights = nn.functional.softmax(
+ attn_weights, dim=-1, dtype=torch.float32).to(query_states.dtype)
+ attn_weights = nn.functional.dropout(
+ attn_weights, p=self.attention_dropout, training=self.training)
+ attn_output = torch.matmul(attn_weights, value_states)
+
+ if attn_output.size() != (bsz, self.num_heads, q_len, self.v_head_dim):
+ raise ValueError(
+ f'`attn_output` should be of size {(bsz, self.num_heads, q_len, self.v_head_dim)}, but is'
+ f' {attn_output.size()}')
+
+ attn_output = attn_output.transpose(1, 2).contiguous()
+
+ attn_output = attn_output.reshape(bsz, q_len,
+ self.num_heads * self.v_head_dim)
+
+ attn_output = self.o_proj(attn_output)
+
+ if not output_attentions:
+ attn_weights = None
+
+ return attn_output, attn_weights, past_key_value
+
+
+# Copied from transformers.models.llama.modeling_llama.LlamaFlashAttention2 with Llama->DeepseekV2
+class DeepseekV2FlashAttention2(DeepseekV2Attention):
+ """DeepseekV2 flash attention module.
+
+ This module inherits from `DeepseekV2Attention` as the weights of the
+ module stays untouched. The only required change would be on the forward
+ pass where it needs to correctly call the public API of flash attention and
+ deal with padding tokens in case the input contains any of them.
+ """
+
+ def __init__(self, *args, **kwargs):
+ super().__init__(*args, **kwargs)
+
+ # TODO: Should be removed once Flash Attention for RoCm is bumped to 2.1.
+ # flash_attn<2.1 generates top-left aligned causal mask, while what is needed here is bottom-right alignment, that was made default for flash_attn>=2.1. This attribute is used to handle this difference. Reference: https://github.com/Dao-AILab/flash-attention/releases/tag/v2.1.0.
+ # Beware that with flash_attn<2.1, using q_seqlen != k_seqlen (except for the case q_seqlen == 1) produces a wrong mask (top-left).
+ self._flash_attn_uses_top_left_mask = not is_flash_attn_greater_or_equal_2_10(
+ )
+
+ def forward(
+ self,
+ hidden_states: torch.Tensor,
+ attention_mask: Optional[torch.LongTensor] = None,
+ position_ids: Optional[torch.LongTensor] = None,
+ past_key_value: Optional[Cache] = None,
+ output_attentions: bool = False,
+ use_cache: bool = False,
+ **kwargs,
+ ) -> Tuple[torch.Tensor, Optional[torch.Tensor],
+ Optional[Tuple[torch.Tensor]]]:
+ # DeepseekV2FlashAttention2 attention does not support output_attentions
+ if 'padding_mask' in kwargs:
+ warnings.warn(
+ 'Passing `padding_mask` is deprecated and will be removed in v4.37. Please make sure use `attention_mask` instead.`'
+ )
+
+ # overwrite attention_mask with padding_mask
+ attention_mask = kwargs.pop('padding_mask')
+
+ output_attentions = False
+
+ bsz, q_len, _ = hidden_states.size()
+
+ if self.q_lora_rank is None:
+ q = self.q_proj(hidden_states)
+ else:
+ q = self.q_b_proj(self.q_a_layernorm(self.q_a_proj(hidden_states)))
+ q = q.view(bsz, q_len, self.num_heads, self.q_head_dim).transpose(1, 2)
+ q_nope, q_pe = torch.split(
+ q, [self.qk_nope_head_dim, self.qk_rope_head_dim], dim=-1)
+
+ # Flash attention requires the input to have the shape
+ # batch_size x seq_length x head_dim x hidden_dim
+ # therefore we just need to keep the original shape
+ compressed_kv = self.kv_a_proj_with_mqa(hidden_states)
+ compressed_kv, k_pe = torch.split(
+ compressed_kv, [self.kv_lora_rank, self.qk_rope_head_dim], dim=-1)
+ k_pe = k_pe.view(bsz, q_len, 1, self.qk_rope_head_dim).transpose(1, 2)
+ kv = (
+ self.kv_b_proj(self.kv_a_layernorm(compressed_kv)).view(
+ bsz, q_len, self.num_heads,
+ self.qk_nope_head_dim + self.v_head_dim).transpose(1, 2))
+
+ k_nope, value_states = torch.split(
+ kv, [self.qk_nope_head_dim, self.v_head_dim], dim=-1)
+ kv_seq_len = value_states.shape[-2]
+
+ kv_seq_len = value_states.shape[-2]
+ if past_key_value is not None:
+ kv_seq_len += past_key_value.get_usable_length(
+ kv_seq_len, self.layer_idx)
+
+ cos, sin = self.rotary_emb(value_states, seq_len=kv_seq_len)
+ q_pe, k_pe = apply_rotary_pos_emb(q_pe, k_pe, cos, sin, position_ids)
+
+ query_states = k_pe.new_empty(bsz, self.num_heads, q_len,
+ self.q_head_dim)
+ query_states[:, :, :, :self.qk_nope_head_dim] = q_nope
+ query_states[:, :, :, self.qk_nope_head_dim:] = q_pe
+
+ key_states = k_pe.new_empty(bsz, self.num_heads, q_len,
+ self.q_head_dim)
+ key_states[:, :, :, :self.qk_nope_head_dim] = k_nope
+ key_states[:, :, :, self.qk_nope_head_dim:] = k_pe
+
+ if self.q_head_dim != self.v_head_dim:
+ value_states = F.pad(value_states,
+ [0, self.q_head_dim - self.v_head_dim])
+
+ if past_key_value is not None:
+ cache_kwargs = {'sin': sin, 'cos': cos} # Specific to RoPE models
+ key_states, value_states = past_key_value.update(
+ key_states, value_states, self.layer_idx, cache_kwargs)
+
+ # TODO: These transpose are quite inefficient but Flash Attention requires the layout [batch_size, sequence_length, num_heads, head_dim]. We would need to refactor the KV cache
+ # to be able to avoid many of these transpose/reshape/view.
+ query_states = query_states.transpose(1, 2)
+ key_states = key_states.transpose(1, 2)
+ value_states = value_states.transpose(1, 2)
+
+ dropout_rate = self.attention_dropout if self.training else 0.0
+
+ # In PEFT, usually we cast the layer norms in float32 for training stability reasons
+ # therefore the input hidden states gets silently casted in float32. Hence, we need
+ # cast them back in the correct dtype just to be sure everything works as expected.
+ # This might slowdown training & inference so it is recommended to not cast the LayerNorms
+ # in fp32. (DeepseekV2RMSNorm handles it correctly)
+
+ input_dtype = query_states.dtype
+ if input_dtype == torch.float32:
+ # Handle the case where the model is quantized
+ if hasattr(self.config, '_pre_quantization_dtype'):
+ target_dtype = self.config._pre_quantization_dtype
+ elif torch.is_autocast_enabled():
+ target_dtype = torch.get_autocast_gpu_dtype()
+ else:
+ target_dtype = self.q_proj.weight.dtype if self.q_lora_rank is None else self.q_a_proj.weight.dtype
+
+ logger.warning_once(
+ f'The input hidden states seems to be silently casted in float32, this might be related to'
+ f' the fact you have upcasted embedding or layer norm layers in float32. We will cast back the input in'
+ f' {target_dtype}.')
+
+ query_states = query_states.to(target_dtype)
+ key_states = key_states.to(target_dtype)
+ value_states = value_states.to(target_dtype)
+
+ attn_output = self._flash_attention_forward(
+ query_states,
+ key_states,
+ value_states,
+ attention_mask,
+ q_len,
+ dropout=dropout_rate,
+ softmax_scale=self.softmax_scale,
+ )
+ if self.q_head_dim != self.v_head_dim:
+ attn_output = attn_output[:, :, :, :self.v_head_dim]
+
+ attn_output = attn_output.reshape(bsz, q_len, self.num_heads *
+ self.v_head_dim).contiguous()
+ attn_output = self.o_proj(attn_output)
+
+ if not output_attentions:
+ attn_weights = None
+
+ return attn_output, attn_weights, past_key_value
+
+ def _flash_attention_forward(
+ self,
+ query_states,
+ key_states,
+ value_states,
+ attention_mask,
+ query_length,
+ dropout=0.0,
+ softmax_scale=None,
+ ):
+ """
+ Calls the forward method of Flash Attention - if the input hidden states contain at least one padding token
+ first unpad the input, then computes the attention scores and pad the final attention scores.
+
+ Args:
+ query_states (`torch.Tensor`):
+ Input query states to be passed to Flash Attention API
+ key_states (`torch.Tensor`):
+ Input key states to be passed to Flash Attention API
+ value_states (`torch.Tensor`):
+ Input value states to be passed to Flash Attention API
+ attention_mask (`torch.Tensor`):
+ The padding mask - corresponds to a tensor of size `(batch_size, seq_len)` where 0 stands for the
+ position of padding tokens and 1 for the position of non-padding tokens.
+ dropout (`int`, *optional*):
+ Attention dropout
+ softmax_scale (`float`, *optional*):
+ The scaling of QK^T before applying softmax. Default to 1 / sqrt(head_dim)
+ """
+ if not self._flash_attn_uses_top_left_mask:
+ causal = self.is_causal
+ else:
+ # TODO: Remove the `query_length != 1` check once Flash Attention for RoCm is bumped to 2.1. For details, please see the comment in DeepseekV2FlashAttention2 __init__.
+ causal = self.is_causal and query_length != 1
+
+ # Contains at least one padding token in the sequence
+ if attention_mask is not None:
+ batch_size = query_states.shape[0]
+ (
+ query_states,
+ key_states,
+ value_states,
+ indices_q,
+ cu_seq_lens,
+ max_seq_lens,
+ ) = self._upad_input(query_states, key_states, value_states,
+ attention_mask, query_length)
+
+ cu_seqlens_q, cu_seqlens_k = cu_seq_lens
+ max_seqlen_in_batch_q, max_seqlen_in_batch_k = max_seq_lens
+
+ attn_output_unpad = flash_attn_varlen_func(
+ query_states,
+ key_states,
+ value_states,
+ cu_seqlens_q=cu_seqlens_q,
+ cu_seqlens_k=cu_seqlens_k,
+ max_seqlen_q=max_seqlen_in_batch_q,
+ max_seqlen_k=max_seqlen_in_batch_k,
+ dropout_p=dropout,
+ softmax_scale=softmax_scale,
+ causal=causal,
+ )
+
+ attn_output = pad_input(attn_output_unpad, indices_q, batch_size,
+ query_length)
+ else:
+ attn_output = flash_attn_func(
+ query_states,
+ key_states,
+ value_states,
+ dropout,
+ softmax_scale=softmax_scale,
+ causal=causal,
+ )
+
+ return attn_output
+
+ def _upad_input(self, query_layer, key_layer, value_layer, attention_mask,
+ query_length):
+ indices_k, cu_seqlens_k, max_seqlen_in_batch_k = _get_unpad_data(
+ attention_mask)
+ batch_size, kv_seq_len, num_key_value_heads, head_dim = key_layer.shape
+
+ key_layer = index_first_axis(
+ key_layer.reshape(batch_size * kv_seq_len, num_key_value_heads,
+ head_dim),
+ indices_k,
+ )
+ value_layer = index_first_axis(
+ value_layer.reshape(batch_size * kv_seq_len, num_key_value_heads,
+ head_dim),
+ indices_k,
+ )
+ if query_length == kv_seq_len:
+ query_layer = index_first_axis(
+ query_layer.reshape(batch_size * kv_seq_len, self.num_heads,
+ head_dim),
+ indices_k,
+ )
+ cu_seqlens_q = cu_seqlens_k
+ max_seqlen_in_batch_q = max_seqlen_in_batch_k
+ indices_q = indices_k
+ elif query_length == 1:
+ max_seqlen_in_batch_q = 1
+ cu_seqlens_q = torch.arange(
+ batch_size + 1, dtype=torch.int32, device=query_layer.device
+ ) # There is a memcpy here, that is very bad.
+ indices_q = cu_seqlens_q[:-1]
+ query_layer = query_layer.squeeze(1)
+ else:
+ # The -q_len: slice assumes left padding.
+ attention_mask = attention_mask[:, -query_length:]
+ query_layer, indices_q, cu_seqlens_q, max_seqlen_in_batch_q = unpad_input(
+ query_layer, attention_mask)
+
+ return (
+ query_layer,
+ key_layer,
+ value_layer,
+ indices_q,
+ (cu_seqlens_q, cu_seqlens_k),
+ (max_seqlen_in_batch_q, max_seqlen_in_batch_k),
+ )
+
+
+ATTENTION_CLASSES = {
+ 'eager': DeepseekV2Attention,
+ 'flash_attention_2': DeepseekV2FlashAttention2,
+}
+
+
+class DeepseekV2DecoderLayer(nn.Module):
+
+ def __init__(self, config: DeepseekV2Config, layer_idx: int):
+ super().__init__()
+ self.hidden_size = config.hidden_size
+
+ self.self_attn = ATTENTION_CLASSES[config._attn_implementation](
+ config=config, layer_idx=layer_idx)
+
+ moe_implementation = config.moe_implementation
+ if moe_implementation == 'origin':
+ block = DeepseekV2MoE
+ elif moe_implementation == 'shard':
+ block = DeepseekV2MoEShard
+ else:
+ raise NotImplementedError
+
+ self.mlp = (
+ block(config) if
+ (config.n_routed_experts is not None
+ and layer_idx >= config.first_k_dense_replace and layer_idx %
+ config.moe_layer_freq == 0) else DeepseekV2MLP(config))
+ self.input_layernorm = DeepseekV2RMSNorm(
+ config.hidden_size, eps=config.rms_norm_eps)
+ self.post_attention_layernorm = DeepseekV2RMSNorm(
+ config.hidden_size, eps=config.rms_norm_eps)
+
+ def forward(
+ self,
+ hidden_states: torch.Tensor,
+ attention_mask: Optional[torch.Tensor] = None,
+ position_ids: Optional[torch.LongTensor] = None,
+ past_key_value: Optional[Tuple[torch.Tensor]] = None,
+ output_attentions: Optional[bool] = False,
+ use_cache: Optional[bool] = False,
+ **kwargs,
+ ) -> Tuple[torch.FloatTensor, Optional[Tuple[torch.FloatTensor,
+ torch.FloatTensor]]]:
+ """
+ Args:
+ hidden_states (`torch.FloatTensor`): input to the layer of shape `(batch, seq_len, embed_dim)`
+ attention_mask (`torch.FloatTensor`, *optional*):
+ attention mask of size `(batch_size, sequence_length)` if flash attention is used or `(batch_size, 1,
+ query_sequence_length, key_sequence_length)` if default attention is used.
+ output_attentions (`bool`, *optional*):
+ Whether or not to return the attentions tensors of all attention layers. See `attentions` under
+ returned tensors for more detail.
+ use_cache (`bool`, *optional*):
+ If set to `True`, `past_key_values` key value states are returned and can be used to speed up decoding
+ (see `past_key_values`).
+ past_key_value (`Tuple(torch.FloatTensor)`, *optional*): cached past key and value projection states
+ """
+ if 'padding_mask' in kwargs:
+ warnings.warn(
+ 'Passing `padding_mask` is deprecated and will be removed in v4.37. Please make sure use `attention_mask` instead.`'
+ )
+ residual = hidden_states
+
+ hidden_states = self.input_layernorm(hidden_states)
+
+ # Self Attention
+ hidden_states, self_attn_weights, present_key_value = self.self_attn(
+ hidden_states=hidden_states,
+ attention_mask=attention_mask,
+ position_ids=position_ids,
+ past_key_value=past_key_value,
+ output_attentions=output_attentions,
+ use_cache=use_cache,
+ **kwargs,
+ )
+ hidden_states = residual + hidden_states
+
+ # Fully Connected
+ residual = hidden_states
+ hidden_states = self.post_attention_layernorm(hidden_states)
+ hidden_states = self.mlp(hidden_states)
+ hidden_states = residual + hidden_states
+
+ outputs = (hidden_states, )
+
+ if output_attentions:
+ outputs += (self_attn_weights, )
+
+ if use_cache:
+ outputs += (present_key_value, )
+
+ return outputs
+
+
+def _load_pretrained_model(
+ cls,
+ model,
+ state_dict,
+ loaded_keys,
+ resolved_archive_file,
+ pretrained_model_name_or_path,
+ ignore_mismatched_sizes=False,
+ sharded_metadata=None,
+ _fast_init=True,
+ low_cpu_mem_usage=False,
+ device_map=None,
+ offload_folder=None,
+ offload_state_dict=None,
+ dtype=None,
+ hf_quantizer=None,
+ keep_in_fp32_modules=None,
+ gguf_path=None,
+):
+ if ((state_dict is not None) or (resolved_archive_file is None)
+ or (low_cpu_mem_usage) or (device_map is not None)
+ or (offload_folder is not None) or
+ (not (offload_state_dict is None or offload_state_dict is False))
+ or (hf_quantizer is not None) or
+ (keep_in_fp32_modules is not None and len(keep_in_fp32_modules) > 0)
+ or (gguf_path is not None)):
+ raise NotImplementedError
+
+ folder = os.path.sep.join(resolved_archive_file[0].split(os.path.sep)[:-1])
+ error_msgs = load_state_dict_into_model(model, folder)
+ return model, [], [], [], None, error_msgs
+
+
+DeepseekV2_START_DOCSTRING = r"""
+ This model inherits from [`PreTrainedModel`]. Check the superclass documentation for the generic methods the
+ library implements for all its model (such as downloading or saving, resizing the input embeddings, pruning heads
+ etc.)
+
+ This model is also a PyTorch [torch.nn.Module](https://pytorch.org/docs/stable/nn.html#torch.nn.Module) subclass.
+ Use it as a regular PyTorch Module and refer to the PyTorch documentation for all matter related to general usage
+ and behavior.
+
+ Parameters:
+ config ([`DeepseekV2Config`]):
+ Model configuration class with all the parameters of the model. Initializing with a config file does not
+ load the weights associated with the model, only the configuration. Check out the
+ [`~PreTrainedModel.from_pretrained`] method to load the model weights.
+"""
+
+
+@add_start_docstrings(
+ 'The bare DeepseekV2 Model outputting raw hidden-states without any specific head on top.',
+ DeepseekV2_START_DOCSTRING,
+)
+class DeepseekV2PreTrainedModel(PreTrainedModel):
+ config_class = DeepseekV2Config
+ base_model_prefix = 'model'
+ supports_gradient_checkpointing = True
+ _no_split_modules = ['DeepseekV2DecoderLayer']
+ _skip_keys_device_placement = 'past_key_values'
+ _supports_flash_attn_2 = True
+ _supports_sdpa = True
+ _supports_cache_class = True
+
+ def _init_weights(self, module):
+ std = self.config.initializer_range
+ if isinstance(module, nn.Linear):
+ module.weight.data.normal_(mean=0.0, std=std)
+ if module.bias is not None:
+ module.bias.data.zero_()
+ elif isinstance(module, nn.Embedding):
+ module.weight.data.normal_(mean=0.0, std=std)
+ if module.padding_idx is not None:
+ module.weight.data[module.padding_idx].zero_()
+
+ @classmethod
+ def from_pretrained(cls, pretrained_model_name_or_path, *args, **kwargs):
+ moe_implementation = kwargs.get('moe_implementation', 'origin')
+ if moe_implementation == 'origin':
+ return super().from_pretrained(pretrained_model_name_or_path,
+ *args, **kwargs)
+
+ cls._load_pretrained_model = types.MethodType(_load_pretrained_model,
+ cls)
+ return super().from_pretrained(pretrained_model_name_or_path, *args,
+ **kwargs)
+
+
+DeepseekV2_INPUTS_DOCSTRING = r"""
+ Args:
+ input_ids (`torch.LongTensor` of shape `(batch_size, sequence_length)`):
+ Indices of input sequence tokens in the vocabulary. Padding will be ignored by default should you provide
+ it.
+
+ Indices can be obtained using [`AutoTokenizer`]. See [`PreTrainedTokenizer.encode`] and
+ [`PreTrainedTokenizer.__call__`] for details.
+
+ [What are input IDs?](../glossary#input-ids)
+ attention_mask (`torch.Tensor` of shape `(batch_size, sequence_length)`, *optional*):
+ Mask to avoid performing attention on padding token indices. Mask values selected in `[0, 1]`:
+
+ - 1 for tokens that are **not masked**,
+ - 0 for tokens that are **masked**.
+
+ [What are attention masks?](../glossary#attention-mask)
+
+ Indices can be obtained using [`AutoTokenizer`]. See [`PreTrainedTokenizer.encode`] and
+ [`PreTrainedTokenizer.__call__`] for details.
+
+ If `past_key_values` is used, optionally only the last `input_ids` have to be input (see
+ `past_key_values`).
+
+ If you want to change padding behavior, you should read [`modeling_opt._prepare_decoder_attention_mask`]
+ and modify to your needs. See diagram 1 in [the paper](https://arxiv.org/abs/1910.13461) for more
+ information on the default strategy.
+
+ - 1 indicates the head is **not masked**,
+ - 0 indicates the head is **masked**.
+ position_ids (`torch.LongTensor` of shape `(batch_size, sequence_length)`, *optional*):
+ Indices of positions of each input sequence tokens in the position embeddings. Selected in the range `[0,
+ config.n_positions - 1]`.
+
+ [What are position IDs?](../glossary#position-ids)
+ past_key_values (`Cache` or `tuple(tuple(torch.FloatTensor))`, *optional*):
+ Pre-computed hidden-states (key and values in the self-attention blocks and in the cross-attention
+ blocks) that can be used to speed up sequential decoding. This typically consists in the `past_key_values`
+ returned by the model at a previous stage of decoding, when `use_cache=True` or `config.use_cache=True`.
+
+ Two formats are allowed:
+ - a [`~cache_utils.Cache`] instance;
+ - Tuple of `tuple(torch.FloatTensor)` of length `config.n_layers`, with each tuple having 2 tensors of
+ shape `(batch_size, num_heads, sequence_length, embed_size_per_head)`). This is also known as the legacy
+ cache format.
+
+ The model will output the same cache format that is fed as input. If no `past_key_values` are passed, the
+ legacy cache format will be returned.
+
+ If `past_key_values` are used, the user can optionally input only the last `input_ids` (those that don't
+ have their past key value states given to this model) of shape `(batch_size, 1)` instead of all `input_ids`
+ of shape `(batch_size, sequence_length)`.
+ inputs_embeds (`torch.FloatTensor` of shape `(batch_size, sequence_length, hidden_size)`, *optional*):
+ Optionally, instead of passing `input_ids` you can choose to directly pass an embedded representation. This
+ is useful if you want more control over how to convert `input_ids` indices into associated vectors than the
+ model's internal embedding lookup matrix.
+ use_cache (`bool`, *optional*):
+ If set to `True`, `past_key_values` key value states are returned and can be used to speed up decoding (see
+ `past_key_values`).
+ output_attentions (`bool`, *optional*):
+ Whether or not to return the attentions tensors of all attention layers. See `attentions` under returned
+ tensors for more detail.
+ output_hidden_states (`bool`, *optional*):
+ Whether or not to return the hidden states of all layers. See `hidden_states` under returned tensors for
+ more detail.
+ return_dict (`bool`, *optional*):
+ Whether or not to return a [`~utils.ModelOutput`] instead of a plain tuple.
+"""
+
+
+@add_start_docstrings(
+ 'The bare DeepseekV2 Model outputting raw hidden-states without any specific head on top.',
+ DeepseekV2_START_DOCSTRING,
+)
+class DeepseekV2Model(DeepseekV2PreTrainedModel):
+ """Transformer decoder consisting of *config.num_hidden_layers* layers.
+ Each layer is a [`DeepseekV2DecoderLayer`]
+
+ Args:
+ config: DeepseekV2Config
+ """
+
+ def __init__(self, config: DeepseekV2Config):
+ super().__init__(config)
+ self.padding_idx = config.pad_token_id
+ self.vocab_size = config.vocab_size
+
+ self.embed_tokens = nn.Embedding(config.vocab_size, config.hidden_size,
+ self.padding_idx)
+ self.layers = nn.ModuleList([
+ DeepseekV2DecoderLayer(config, layer_idx)
+ for layer_idx in range(config.num_hidden_layers)
+ ])
+ self._use_sdpa = config._attn_implementation == 'sdpa'
+ self._use_flash_attention_2 = config._attn_implementation == 'flash_attention_2'
+ self.norm = DeepseekV2RMSNorm(
+ config.hidden_size, eps=config.rms_norm_eps)
+
+ self.gradient_checkpointing = False
+ # Initialize weights and apply final processing
+ self.post_init()
+
+ def get_input_embeddings(self):
+ return self.embed_tokens
+
+ def set_input_embeddings(self, value):
+ self.embed_tokens = value
+
+ @add_start_docstrings_to_model_forward(DeepseekV2_INPUTS_DOCSTRING)
+ def forward(
+ self,
+ input_ids: torch.LongTensor = None,
+ attention_mask: Optional[torch.Tensor] = None,
+ position_ids: Optional[torch.LongTensor] = None,
+ past_key_values: Optional[List[torch.FloatTensor]] = None,
+ inputs_embeds: Optional[torch.FloatTensor] = None,
+ use_cache: Optional[bool] = None,
+ output_attentions: Optional[bool] = None,
+ output_hidden_states: Optional[bool] = None,
+ return_dict: Optional[bool] = None,
+ ) -> Union[Tuple, BaseModelOutputWithPast]:
+ output_attentions = (
+ output_attentions if output_attentions is not None else
+ self.config.output_attentions)
+ output_hidden_states = (
+ output_hidden_states if output_hidden_states is not None else
+ self.config.output_hidden_states)
+ use_cache = use_cache if use_cache is not None else self.config.use_cache
+
+ return_dict = (
+ return_dict
+ if return_dict is not None else self.config.use_return_dict)
+
+ # retrieve input_ids and inputs_embeds
+ if input_ids is not None and inputs_embeds is not None:
+ raise ValueError(
+ 'You cannot specify both input_ids and inputs_embeds at the same time'
+ )
+ elif input_ids is not None:
+ batch_size, seq_length = input_ids.shape[:2]
+ elif inputs_embeds is not None:
+ batch_size, seq_length = inputs_embeds.shape[:2]
+ else:
+ raise ValueError(
+ 'You have to specify either input_ids or inputs_embeds')
+
+ if self.gradient_checkpointing and self.training:
+ if use_cache:
+ logger.warning_once(
+ '`use_cache=True` is incompatible with gradient checkpointing. Setting `use_cache=False`transformers.'
+ )
+ use_cache = False
+
+ past_key_values_length = 0
+ if use_cache:
+ use_legacy_cache = not isinstance(past_key_values, Cache)
+ if use_legacy_cache:
+ past_key_values = DynamicCache.from_legacy_cache(
+ past_key_values)
+ past_key_values_length = past_key_values.get_usable_length(
+ seq_length)
+
+ if position_ids is None:
+ device = input_ids.device if input_ids is not None else inputs_embeds.device
+ position_ids = torch.arange(
+ past_key_values_length,
+ seq_length + past_key_values_length,
+ dtype=torch.long,
+ device=device,
+ )
+ position_ids = position_ids.unsqueeze(0)
+
+ if inputs_embeds is None:
+ inputs_embeds = self.embed_tokens(input_ids)
+
+ if self._use_flash_attention_2:
+ # 2d mask is passed through the layers
+ attention_mask = (
+ attention_mask if
+ (attention_mask is not None and 0 in attention_mask) else None)
+ elif self._use_sdpa and not output_attentions:
+ # output_attentions=True can not be supported when using SDPA, and we fall back on
+ # the manual implementation that requires a 4D causal mask in all cases.
+ attention_mask = _prepare_4d_causal_attention_mask_for_sdpa(
+ attention_mask,
+ (batch_size, seq_length),
+ inputs_embeds,
+ past_key_values_length,
+ )
+ else:
+ # 4d mask is passed through the layers
+ attention_mask = _prepare_4d_causal_attention_mask(
+ attention_mask,
+ (batch_size, seq_length),
+ inputs_embeds,
+ past_key_values_length,
+ )
+
+ # embed positions
+ hidden_states = inputs_embeds
+
+ # decoder layers
+ all_hidden_states = () if output_hidden_states else None
+ all_self_attns = () if output_attentions else None
+ next_decoder_cache = None
+
+ for decoder_layer in self.layers:
+ if output_hidden_states:
+ all_hidden_states += (hidden_states, )
+
+ if self.gradient_checkpointing and self.training:
+ layer_outputs = self._gradient_checkpointing_func(
+ decoder_layer.__call__,
+ hidden_states,
+ attention_mask,
+ position_ids,
+ past_key_values,
+ output_attentions,
+ use_cache,
+ )
+ else:
+ layer_outputs = decoder_layer(
+ hidden_states,
+ attention_mask=attention_mask,
+ position_ids=position_ids,
+ past_key_value=past_key_values,
+ output_attentions=output_attentions,
+ use_cache=use_cache,
+ )
+
+ hidden_states = layer_outputs[0]
+
+ if use_cache:
+ next_decoder_cache = layer_outputs[
+ 2 if output_attentions else 1]
+
+ if output_attentions:
+ all_self_attns += (layer_outputs[1], )
+
+ hidden_states = self.norm(hidden_states)
+
+ # add hidden states from the last decoder layer
+ if output_hidden_states:
+ all_hidden_states += (hidden_states, )
+
+ next_cache = None
+ if use_cache:
+ next_cache = (
+ next_decoder_cache.to_legacy_cache()
+ if use_legacy_cache else next_decoder_cache)
+ if not return_dict:
+ return tuple(
+ v for v in
+ [hidden_states, next_cache, all_hidden_states, all_self_attns]
+ if v is not None)
+ return BaseModelOutputWithPast(
+ last_hidden_state=hidden_states,
+ past_key_values=next_cache,
+ hidden_states=all_hidden_states,
+ attentions=all_self_attns,
+ )
+
+
+class DeepseekV2ForCausalLM(DeepseekV2PreTrainedModel):
+ _tied_weights_keys = ['lm_head.weight']
+
+ def __init__(self, config):
+ super().__init__(config)
+ self.model = DeepseekV2Model(config)
+ self.vocab_size = config.vocab_size
+ self.lm_head = nn.Linear(
+ config.hidden_size, config.vocab_size, bias=False)
+
+ # Initialize weights and apply final processing
+ self.post_init()
+
+ def get_input_embeddings(self):
+ return self.model.embed_tokens
+
+ def set_input_embeddings(self, value):
+ self.model.embed_tokens = value
+
+ def get_output_embeddings(self):
+ return self.lm_head
+
+ def set_output_embeddings(self, new_embeddings):
+ self.lm_head = new_embeddings
+
+ def set_decoder(self, decoder):
+ self.model = decoder
+
+ def get_decoder(self):
+ return self.model
+
+ @add_start_docstrings_to_model_forward(DeepseekV2_INPUTS_DOCSTRING)
+ @replace_return_docstrings(
+ output_type=CausalLMOutputWithPast, config_class=_CONFIG_FOR_DOC)
+ def forward(
+ self,
+ input_ids: torch.LongTensor = None,
+ attention_mask: Optional[torch.Tensor] = None,
+ position_ids: Optional[torch.LongTensor] = None,
+ past_key_values: Optional[List[torch.FloatTensor]] = None,
+ inputs_embeds: Optional[torch.FloatTensor] = None,
+ labels: Optional[torch.LongTensor] = None,
+ use_cache: Optional[bool] = None,
+ output_attentions: Optional[bool] = None,
+ output_hidden_states: Optional[bool] = None,
+ return_dict: Optional[bool] = None,
+ ) -> Union[Tuple, CausalLMOutputWithPast]:
+ r"""
+ Args:
+ labels (`torch.LongTensor` of shape `(batch_size, sequence_length)`, *optional*):
+ Labels for computing the masked language modeling loss. Indices should either be in `[0, transformers.,
+ config.vocab_size]` or -100 (see `input_ids` docstring). Tokens with indices set to `-100` are ignored
+ (masked), the loss is only computed for the tokens with labels in `[0, transformers., config.vocab_size]`.
+
+ Returns:
+
+ Example:
+
+ ```python
+ >>> from transformers import AutoTokenizer, DeepseekV2ForCausalLM
+
+ >>> model = DeepseekV2ForCausalLM.from_pretrained(PATH_TO_CONVERTED_WEIGHTS)
+ >>> tokenizer = AutoTokenizer.from_pretrained(PATH_TO_CONVERTED_TOKENIZER)
+
+ >>> prompt = "Hey, are you conscious? Can you talk to me?"
+ >>> inputs = tokenizer(prompt, return_tensors="pt")
+
+ >>> # Generate
+ >>> generate_ids = model.generate(inputs.input_ids, max_length=30)
+ >>> tokenizer.batch_decode(generate_ids, skip_special_tokens=True, clean_up_tokenization_spaces=False)[0]
+ "Hey, are you conscious? Can you talk to me?\nI'm not conscious, but I can talk to you."
+ ```"""
+ output_attentions = (
+ output_attentions if output_attentions is not None else
+ self.config.output_attentions)
+ output_hidden_states = (
+ output_hidden_states if output_hidden_states is not None else
+ self.config.output_hidden_states)
+ return_dict = (
+ return_dict
+ if return_dict is not None else self.config.use_return_dict)
+
+ # decoder outputs consists of (dec_features, layer_state, dec_hidden, dec_attn)
+ outputs = self.model(
+ input_ids=input_ids,
+ attention_mask=attention_mask,
+ position_ids=position_ids,
+ past_key_values=past_key_values,
+ inputs_embeds=inputs_embeds,
+ use_cache=use_cache,
+ output_attentions=output_attentions,
+ output_hidden_states=output_hidden_states,
+ return_dict=return_dict,
+ )
+
+ hidden_states = outputs[0]
+ logits = self.lm_head(hidden_states)
+ logits = logits.float()
+
+ loss = None
+ if labels is not None:
+ # Shift so that tokens < n predict n
+ shift_logits = logits[..., :-1, :].contiguous()
+ shift_labels = labels[..., 1:].contiguous()
+ # Flatten the tokens
+ loss_fct = CrossEntropyLoss()
+ shift_logits = shift_logits.view(-1, self.config.vocab_size)
+ shift_labels = shift_labels.view(-1)
+ # Enable model parallelism
+ shift_labels = shift_labels.to(shift_logits.device)
+ loss = loss_fct(shift_logits, shift_labels)
+
+ if not return_dict:
+ output = (logits, ) + outputs[1:]
+ return (loss, ) + output if loss is not None else output
+
+ return CausalLMOutputWithPast(
+ loss=loss,
+ logits=logits,
+ past_key_values=outputs.past_key_values,
+ hidden_states=outputs.hidden_states,
+ attentions=outputs.attentions,
+ )
+
+ def prepare_inputs_for_generation(
+ self,
+ input_ids,
+ past_key_values=None,
+ attention_mask=None,
+ inputs_embeds=None,
+ **kwargs,
+ ):
+ if past_key_values is not None:
+ if isinstance(past_key_values, Cache):
+ cache_length = past_key_values.get_seq_length()
+ past_length = past_key_values.seen_tokens
+ max_cache_length = past_key_values.get_max_length()
+ else:
+ cache_length = past_length = past_key_values[0][0].shape[2]
+ max_cache_length = None
+
+ # Keep only the unprocessed tokens:
+ # 1 - If the length of the attention_mask exceeds the length of input_ids, then we are in a setting where
+ # some of the inputs are exclusively passed as part of the cache (e.g. when passing input_embeds as
+ # input)
+ if (attention_mask is not None
+ and attention_mask.shape[1] > input_ids.shape[1]):
+ input_ids = input_ids[:, -(attention_mask.shape[1] -
+ past_length):]
+ # 2 - If the past_length is smaller than input_ids', then input_ids holds all input tokens. We can discard
+ # input_ids based on the past_length.
+ elif past_length < input_ids.shape[1]:
+ input_ids = input_ids[:, past_length:]
+ # 3 - Otherwise (past_length >= input_ids.shape[1]), let's assume input_ids only has unprocessed tokens.
+
+ # If we are about to go beyond the maximum cache length, we need to crop the input attention mask.
+ if (max_cache_length is not None and attention_mask is not None
+ and cache_length + input_ids.shape[1] > max_cache_length):
+ attention_mask = attention_mask[:, -max_cache_length:]
+
+ position_ids = kwargs.get('position_ids', None)
+ if attention_mask is not None and position_ids is None:
+ # create position_ids on the fly for batch generation
+ position_ids = attention_mask.long().cumsum(-1) - 1
+ position_ids.masked_fill_(attention_mask == 0, 1)
+ if past_key_values:
+ position_ids = position_ids[:, -input_ids.shape[1]:]
+
+ # if `inputs_embeds` are passed, we only want to use them in the 1st generation step
+ if inputs_embeds is not None and past_key_values is None:
+ model_inputs = {'inputs_embeds': inputs_embeds}
+ else:
+ model_inputs = {'input_ids': input_ids}
+
+ model_inputs.update({
+ 'position_ids': position_ids,
+ 'past_key_values': past_key_values,
+ 'use_cache': kwargs.get('use_cache'),
+ 'attention_mask': attention_mask,
+ })
+ return model_inputs
+
+ @staticmethod
+ def _reorder_cache(past_key_values, beam_idx):
+ reordered_past = ()
+ for layer_past in past_key_values:
+ reordered_past += (tuple(
+ past_state.index_select(0, beam_idx.to(past_state.device))
+ for past_state in layer_past), )
+ return reordered_past
+
+
+@add_start_docstrings(
+ """
+ The DeepseekV2 Model transformer with a sequence classification head on top (linear layer).
+
+ [`DeepseekV2ForSequenceClassification`] uses the last token in order to do the classification, as other causal models
+ (e.g. GPT-2) do.
+
+ Since it does classification on the last token, it requires to know the position of the last token. If a
+ `pad_token_id` is defined in the configuration, it finds the last token that is not a padding token in each row. If
+ no `pad_token_id` is defined, it simply takes the last value in each row of the batch. Since it cannot guess the
+ padding tokens when `inputs_embeds` are passed instead of `input_ids`, it does the same (take the last value in
+ each row of the batch).
+ """,
+ DeepseekV2_START_DOCSTRING,
+)
+class DeepseekV2ForSequenceClassification(DeepseekV2PreTrainedModel):
+
+ def __init__(self, config):
+ super().__init__(config)
+ self.num_labels = config.num_labels
+ self.model = DeepseekV2Model(config)
+ self.score = nn.Linear(config.hidden_size, self.num_labels, bias=False)
+
+ # Initialize weights and apply final processing
+ self.post_init()
+
+ def get_input_embeddings(self):
+ return self.model.embed_tokens
+
+ def set_input_embeddings(self, value):
+ self.model.embed_tokens = value
+
+ @add_start_docstrings_to_model_forward(DeepseekV2_INPUTS_DOCSTRING)
+ def forward(
+ self,
+ input_ids: torch.LongTensor = None,
+ attention_mask: Optional[torch.Tensor] = None,
+ position_ids: Optional[torch.LongTensor] = None,
+ past_key_values: Optional[List[torch.FloatTensor]] = None,
+ inputs_embeds: Optional[torch.FloatTensor] = None,
+ labels: Optional[torch.LongTensor] = None,
+ use_cache: Optional[bool] = None,
+ output_attentions: Optional[bool] = None,
+ output_hidden_states: Optional[bool] = None,
+ return_dict: Optional[bool] = None,
+ ) -> Union[Tuple, SequenceClassifierOutputWithPast]:
+ r"""
+ labels (`torch.LongTensor` of shape `(batch_size,)`, *optional*):
+ Labels for computing the sequence classification/regression loss. Indices should be in `[0, transformers.,
+ config.num_labels - 1]`. If `config.num_labels == 1` a regression loss is computed (Mean-Square loss), If
+ `config.num_labels > 1` a classification loss is computed (Cross-Entropy).
+ """
+ return_dict = (
+ return_dict
+ if return_dict is not None else self.config.use_return_dict)
+
+ transformer_outputs = self.model(
+ input_ids,
+ attention_mask=attention_mask,
+ position_ids=position_ids,
+ past_key_values=past_key_values,
+ inputs_embeds=inputs_embeds,
+ use_cache=use_cache,
+ output_attentions=output_attentions,
+ output_hidden_states=output_hidden_states,
+ return_dict=return_dict,
+ )
+ hidden_states = transformer_outputs[0]
+ logits = self.score(hidden_states)
+
+ if input_ids is not None:
+ batch_size = input_ids.shape[0]
+ else:
+ batch_size = inputs_embeds.shape[0]
+
+ if self.config.pad_token_id is None and batch_size != 1:
+ raise ValueError(
+ 'Cannot handle batch sizes > 1 if no padding token is defined.'
+ )
+ if self.config.pad_token_id is None:
+ sequence_lengths = -1
+ else:
+ if input_ids is not None:
+ sequence_lengths = (torch.eq(
+ input_ids, self.config.pad_token_id).int().argmax(-1) -
+ 1).to(logits.device)
+ else:
+ sequence_lengths = -1
+
+ pooled_logits = logits[torch.arange(batch_size, device=logits.device),
+ sequence_lengths]
+
+ loss = None
+ if labels is not None:
+ labels = labels.to(logits.device)
+ if self.config.problem_type is None:
+ if self.num_labels == 1:
+ self.config.problem_type = 'regression'
+ elif self.num_labels > 1 and (labels.dtype == torch.long
+ or labels.dtype == torch.int):
+ self.config.problem_type = 'single_label_classification'
+ else:
+ self.config.problem_type = 'multi_label_classification'
+
+ if self.config.problem_type == 'regression':
+ loss_fct = MSELoss()
+ if self.num_labels == 1:
+ loss = loss_fct(pooled_logits.squeeze(), labels.squeeze())
+ else:
+ loss = loss_fct(pooled_logits, labels)
+ elif self.config.problem_type == 'single_label_classification':
+ loss_fct = CrossEntropyLoss()
+ loss = loss_fct(
+ pooled_logits.view(-1, self.num_labels), labels.view(-1))
+ elif self.config.problem_type == 'multi_label_classification':
+ loss_fct = BCEWithLogitsLoss()
+ loss = loss_fct(pooled_logits, labels)
+ if not return_dict:
+ output = (pooled_logits, ) + transformer_outputs[1:]
+ return ((loss, ) + output) if loss is not None else output
+
+ return SequenceClassifierOutputWithPast(
+ loss=loss,
+ logits=pooled_logits,
+ past_key_values=transformer_outputs.past_key_values,
+ hidden_states=transformer_outputs.hidden_states,
+ attentions=transformer_outputs.attentions,
+ )
diff --git a/xtuner/model/transformers_models/deepseek_v2/tokenization_deepseek_fast.py b/xtuner/model/transformers_models/deepseek_v2/tokenization_deepseek_fast.py
new file mode 100644
index 000000000..89e3cbb50
--- /dev/null
+++ b/xtuner/model/transformers_models/deepseek_v2/tokenization_deepseek_fast.py
@@ -0,0 +1,37 @@
+from typing import List, Optional, Union
+
+from transformers.models.llama import LlamaTokenizerFast
+
+
+class DeepseekTokenizerFast(LlamaTokenizerFast):
+
+ def convert_ids_to_tokens(
+ self,
+ ids: Union[int, List[int]],
+ skip_special_tokens: bool = False) -> Union[str, List[str]]:
+ """Converts a single index or a sequence of indices in a token or a
+ sequence of tokens, using the vocabulary and added tokens.
+
+ Args:
+ ids (`int` or `List[int]`):
+ The token id (or token ids) to convert to tokens.
+ skip_special_tokens (`bool`, *optional*, defaults to `False`):
+ Whether or not to remove special tokens in the decoding.
+
+ Returns:
+ `str` or `List[str]`: The decoded token(s).
+ """
+ if isinstance(ids, int):
+ return self._convert_id_to_token(ids)
+ tokens = []
+ for index in ids:
+ index = int(index)
+ if skip_special_tokens and index in self.all_special_ids:
+ continue
+ token = self._tokenizer.id_to_token(index)
+ tokens.append(token if token is not None else '')
+ return tokens
+
+ def _convert_id_to_token(self, index: int) -> Optional[str]:
+ token = self._tokenizer.id_to_token(int(index))
+ return token if token is not None else ''
diff --git a/xtuner/model/transformers_models/mixtral/__init__.py b/xtuner/model/transformers_models/mixtral/__init__.py
new file mode 100644
index 000000000..aabfd89db
--- /dev/null
+++ b/xtuner/model/transformers_models/mixtral/__init__.py
@@ -0,0 +1,4 @@
+from .configuration_mixtral import MixtralConfig
+from .modeling_mixtral import MixtralForCausalLM, MixtralModel
+
+__all__ = ['MixtralForCausalLM', 'MixtralModel', 'MixtralConfig']
diff --git a/xtuner/model/transformers_models/mixtral/configuration_mixtral.py b/xtuner/model/transformers_models/mixtral/configuration_mixtral.py
new file mode 100644
index 000000000..457aefd47
--- /dev/null
+++ b/xtuner/model/transformers_models/mixtral/configuration_mixtral.py
@@ -0,0 +1,178 @@
+# Copyright 2023 Mixtral AI and the HuggingFace Inc. team. All rights reserved.
+#
+# Licensed under the Apache License, Version 2.0 (the "License");
+# you may not use this file except in compliance with the License.
+# You may obtain a copy of the License at
+#
+# http://www.apache.org/licenses/LICENSE-2.0
+#
+# Unless required by applicable law or agreed to in writing, software
+# distributed under the License is distributed on an "AS IS" BASIS,
+# WITHOUT WARRANTIES OR CONDITIONS OF ANY KIND, either express or implied.
+# See the License for the specific language governing permissions and
+# limitations under the License.
+"""Mixtral model configuration."""
+
+from transformers.configuration_utils import PretrainedConfig
+from transformers.utils import logging
+
+logger = logging.get_logger(__name__)
+
+
+class MixtralConfig(PretrainedConfig):
+ r"""
+ This is the configuration class to store the configuration of a [`MixtralModel`]. It is used to instantiate an
+ Mixtral model according to the specified arguments, defining the model architecture. Instantiating a configuration
+ with the defaults will yield a similar configuration to that of the Mixtral-7B-v0.1 or Mixtral-7B-Instruct-v0.1.
+
+ [mixtralai/Mixtral-8x7B](https://huggingface.co/mixtralai/Mixtral-8x7B)
+ [mixtralai/Mixtral-7B-Instruct-v0.1](https://huggingface.co/mixtralai/Mixtral-7B-Instruct-v0.1)
+
+ Configuration objects inherit from [`PretrainedConfig`] and can be used to control the model outputs. Read the
+ documentation from [`PretrainedConfig`] for more information.
+
+
+ Args:
+ vocab_size (`int`, *optional*, defaults to 32000):
+ Vocabulary size of the Mixtral model. Defines the number of different tokens that can be represented by the
+ `inputs_ids` passed when calling [`MixtralModel`]
+ hidden_size (`int`, *optional*, defaults to 4096):
+ Dimension of the hidden representations.
+ intermediate_size (`int`, *optional*, defaults to 14336):
+ Dimension of the MLP representations.
+ num_hidden_layers (`int`, *optional*, defaults to 32):
+ Number of hidden layers in the Transformer encoder.
+ num_attention_heads (`int`, *optional*, defaults to 32):
+ Number of attention heads for each attention layer in the Transformer encoder.
+ num_key_value_heads (`int`, *optional*, defaults to 8):
+ This is the number of key_value heads that should be used to implement Grouped Query Attention. If
+ `num_key_value_heads=num_attention_heads`, the model will use Multi Head Attention (MHA), if
+ `num_key_value_heads=1 the model will use Multi Query Attention (MQA) otherwise GQA is used. When
+ converting a multi-head checkpoint to a GQA checkpoint, each group key and value head should be constructed
+ by meanpooling all the original heads within that group. For more details checkout [this
+ paper](https://arxiv.org/pdf/2305.13245.pdf). If it is not specified, will default to `8`.
+ hidden_act (`str` or `function`, *optional*, defaults to `"silu"`):
+ The non-linear activation function (function or string) in the decoder.
+ max_position_embeddings (`int`, *optional*, defaults to `4096*32`):
+ The maximum sequence length that this model might ever be used with. Mixtral's sliding window attention
+ allows sequence of up to 4096*32 tokens.
+ initializer_range (`float`, *optional*, defaults to 0.02):
+ The standard deviation of the truncated_normal_initializer for initializing all weight matrices.
+ rms_norm_eps (`float`, *optional*, defaults to 1e-05):
+ The epsilon used by the rms normalization layers.
+ use_cache (`bool`, *optional*, defaults to `True`):
+ Whether or not the model should return the last key/values attentions (not used by all models). Only
+ relevant if `config.is_decoder=True`.
+ pad_token_id (`int`, *optional*):
+ The id of the padding token.
+ bos_token_id (`int`, *optional*, defaults to 1):
+ The id of the "beginning-of-sequence" token.
+ eos_token_id (`int`, *optional*, defaults to 2):
+ The id of the "end-of-sequence" token.
+ tie_word_embeddings (`bool`, *optional*, defaults to `False`):
+ Whether the model's input and output word embeddings should be tied.
+ rope_theta (`float`, *optional*, defaults to 1000000.0):
+ The base period of the RoPE embeddings.
+ sliding_window (`int`, *optional*):
+ Sliding window attention window size. If not specified, will default to `4096`.
+ attention_dropout (`float`, *optional*, defaults to 0.0):
+ The dropout ratio for the attention probabilities.
+ num_experts_per_tok (`int`, *optional*, defaults to 2):
+ The number of experts to root per-token, can be also interpreted as the `top-p` routing
+ parameter
+ num_local_experts (`int`, *optional*, defaults to 8):
+ Number of experts per Sparse MLP layer.
+ output_router_logits (`bool`, *optional*, defaults to `False`):
+ Whether or not the router logits should be returned by the model. Enabling this will also
+ allow the model to output the auxiliary loss. See [here]() for more details
+ router_aux_loss_coef (`float`, *optional*, defaults to 0.001):
+ The aux loss factor for the total loss.
+ router_jitter_noise (`float`, *optional*, defaults to 0.0):
+ Amount of noise to add to the router.
+ moe_implementation (`str`, *optional*, defaults to 'origin'):
+ The implementation of the moe blocks. 'origin' or 'shard'.
+ expert_in_one_shard (`int`, *optional*, defaults to None):
+ How many expert models are integrated into a shard. It is used only
+ when `moe_implementation` == 'shard'.
+
+ ```python
+ >>> from transformers import MixtralModel, MixtralConfig
+
+ >>> # Initializing a Mixtral 7B style configuration
+ >>> configuration = MixtralConfig()
+
+ >>> # Initializing a model from the Mixtral 7B style configuration
+ >>> model = MixtralModel(configuration)
+
+ >>> # Accessing the model configuration
+ >>> configuration = model.config
+ ```"""
+
+ model_type = 'mixtral'
+ keys_to_ignore_at_inference = ['past_key_values']
+
+ def __init__(
+ self,
+ vocab_size=32000,
+ hidden_size=4096,
+ intermediate_size=14336,
+ num_hidden_layers=32,
+ num_attention_heads=32,
+ num_key_value_heads=8,
+ hidden_act='silu',
+ max_position_embeddings=4096 * 32,
+ initializer_range=0.02,
+ rms_norm_eps=1e-5,
+ use_cache=True,
+ pad_token_id=None,
+ bos_token_id=1,
+ eos_token_id=2,
+ tie_word_embeddings=False,
+ rope_theta=1e6,
+ sliding_window=None,
+ attention_dropout=0.0,
+ num_experts_per_tok=2,
+ num_local_experts=8,
+ output_router_logits=False,
+ router_aux_loss_coef=0.001,
+ router_jitter_noise=0.0,
+ moe_implementation='origin',
+ expert_in_one_shard=None,
+ **kwargs,
+ ):
+ self.vocab_size = vocab_size
+ self.max_position_embeddings = max_position_embeddings
+ self.hidden_size = hidden_size
+ self.intermediate_size = intermediate_size
+ self.num_hidden_layers = num_hidden_layers
+ self.num_attention_heads = num_attention_heads
+ self.sliding_window = sliding_window
+
+ # for backward compatibility
+ if num_key_value_heads is None:
+ num_key_value_heads = num_attention_heads
+
+ self.num_key_value_heads = num_key_value_heads
+ self.hidden_act = hidden_act
+ self.initializer_range = initializer_range
+ self.rms_norm_eps = rms_norm_eps
+ self.use_cache = use_cache
+ self.rope_theta = rope_theta
+ self.attention_dropout = attention_dropout
+
+ self.num_experts_per_tok = num_experts_per_tok
+ self.num_local_experts = num_local_experts
+ self.output_router_logits = output_router_logits
+ self.router_aux_loss_coef = router_aux_loss_coef
+ self.router_jitter_noise = router_jitter_noise
+
+ self.moe_implementation = moe_implementation
+ self.expert_in_one_shard = expert_in_one_shard
+
+ super().__init__(
+ pad_token_id=pad_token_id,
+ bos_token_id=bos_token_id,
+ eos_token_id=eos_token_id,
+ tie_word_embeddings=tie_word_embeddings,
+ **kwargs,
+ )
diff --git a/xtuner/model/transformers_models/mixtral/modeling_mixtral.py b/xtuner/model/transformers_models/mixtral/modeling_mixtral.py
new file mode 100644
index 000000000..9d275841e
--- /dev/null
+++ b/xtuner/model/transformers_models/mixtral/modeling_mixtral.py
@@ -0,0 +1,1821 @@
+# Modified from https://github.com/huggingface/transformers/blob/v4.41.0/src/transformers/models/mixtral/modeling_mixtral.py
+"""PyTorch Mixtral model."""
+import inspect
+import math
+import os
+import types
+from typing import List, Optional, Tuple, Union
+
+import torch
+import torch.nn.functional as F
+import torch.utils.checkpoint
+from torch import nn
+from torch.nn import BCEWithLogitsLoss, CrossEntropyLoss, MSELoss
+from transformers.activations import ACT2FN
+from transformers.cache_utils import Cache, DynamicCache
+from transformers.modeling_attn_mask_utils import (
+ _prepare_4d_causal_attention_mask,
+ _prepare_4d_causal_attention_mask_for_sdpa)
+from transformers.modeling_outputs import (MoeCausalLMOutputWithPast,
+ MoeModelOutputWithPast,
+ SequenceClassifierOutputWithPast)
+from transformers.modeling_utils import PreTrainedModel
+from transformers.pytorch_utils import is_torch_greater_or_equal_than_1_13
+from transformers.utils import (add_start_docstrings,
+ add_start_docstrings_to_model_forward,
+ is_flash_attn_2_available,
+ is_flash_attn_greater_or_equal_2_10, logging,
+ replace_return_docstrings)
+from transformers.utils.import_utils import is_torch_fx_available
+
+from xtuner.utils import load_state_dict_into_model
+from .configuration_mixtral import MixtralConfig
+
+if is_flash_attn_2_available():
+ from flash_attn import flash_attn_func, flash_attn_varlen_func
+ from flash_attn.bert_padding import pad_input # noqa
+ from flash_attn.bert_padding import index_first_axis, unpad_input
+
+ _flash_supports_window_size = 'window_size' in list(
+ inspect.signature(flash_attn_func).parameters)
+
+# This makes `_prepare_4d_causal_attention_mask` a leaf function in the FX graph.
+# It means that the function will not be traced through and simply appear as a node in the graph.
+if is_torch_fx_available():
+ if not is_torch_greater_or_equal_than_1_13:
+ import torch.fx
+
+ _prepare_4d_causal_attention_mask = torch.fx.wrap(
+ _prepare_4d_causal_attention_mask)
+
+logger = logging.get_logger(__name__)
+
+_CONFIG_FOR_DOC = 'MixtralConfig'
+
+
+def load_balancing_loss_func(
+ gate_logits: torch.Tensor,
+ num_experts: torch.Tensor = None,
+ top_k=2,
+ attention_mask: Optional[torch.Tensor] = None) -> float:
+ r"""
+ Computes auxiliary load balancing loss as in Switch Transformer - implemented in Pytorch.
+
+ See Switch Transformer (https://arxiv.org/abs/2101.03961) for more details. This function implements the loss
+ function presented in equations (4) - (6) of the paper. It aims at penalizing cases where the routing between
+ experts is too unbalanced.
+
+ Args:
+ gate_logits (Union[`torch.Tensor`, Tuple[torch.Tensor]):
+ Logits from the `gate`, should be a tuple of model.config.num_hidden_layers tensors of
+ shape [batch_size X sequence_length, num_experts].
+ attention_mask (`torch.Tensor`, None):
+ The attention_mask used in forward function
+ shape [batch_size X sequence_length] if not None.
+ num_experts (`int`, *optional*):
+ Number of experts
+
+ Returns:
+ The auxiliary loss.
+ """
+ if gate_logits is None or not isinstance(gate_logits, tuple):
+ return 0
+
+ if isinstance(gate_logits, tuple):
+ compute_device = gate_logits[0].device
+ concatenated_gate_logits = torch.cat(
+ [layer_gate.to(compute_device) for layer_gate in gate_logits],
+ dim=0)
+
+ routing_weights = torch.nn.functional.softmax(
+ concatenated_gate_logits, dim=-1)
+
+ _, selected_experts = torch.topk(routing_weights, top_k, dim=-1)
+
+ expert_mask = torch.nn.functional.one_hot(selected_experts, num_experts)
+
+ if attention_mask is None:
+ # Compute the percentage of tokens routed to each experts
+ tokens_per_expert = torch.mean(expert_mask.float(), dim=0)
+
+ # Compute the average probability of routing to these experts
+ router_prob_per_expert = torch.mean(routing_weights, dim=0)
+ else:
+ batch_size, sequence_length = attention_mask.shape
+ num_hidden_layers = concatenated_gate_logits.shape[0] // (
+ batch_size * sequence_length)
+
+ # Compute the mask that masks all padding tokens as 0 with the same shape of expert_mask
+ expert_attention_mask = (
+ attention_mask[None, :, :, None, None].expand(
+ (num_hidden_layers, batch_size, sequence_length, top_k,
+ num_experts)).reshape(-1, top_k,
+ num_experts).to(compute_device))
+
+ # Compute the percentage of tokens routed to each experts
+ tokens_per_expert = torch.sum(
+ expert_mask.float() * expert_attention_mask, dim=0) / torch.sum(
+ expert_attention_mask, dim=0)
+
+ # Compute the mask that masks all padding tokens as 0 with the same shape of tokens_per_expert
+ router_per_expert_attention_mask = (
+ attention_mask[None, :, :, None].expand(
+ (num_hidden_layers, batch_size, sequence_length,
+ num_experts)).reshape(-1, num_experts).to(compute_device))
+
+ # Compute the average probability of routing to these experts
+ router_prob_per_expert = torch.sum(
+ routing_weights * router_per_expert_attention_mask,
+ dim=0) / torch.sum(
+ router_per_expert_attention_mask, dim=0)
+
+ overall_loss = torch.sum(tokens_per_expert *
+ router_prob_per_expert.unsqueeze(0))
+ return overall_loss * num_experts
+
+
+# Copied from transformers.models.llama.modeling_llama._get_unpad_data
+def _get_unpad_data(attention_mask):
+ seqlens_in_batch = attention_mask.sum(dim=-1, dtype=torch.int32)
+ indices = torch.nonzero(attention_mask.flatten(), as_tuple=False).flatten()
+ max_seqlen_in_batch = seqlens_in_batch.max().item()
+ cu_seqlens = F.pad(
+ torch.cumsum(seqlens_in_batch, dim=0, dtype=torch.int32), (1, 0))
+ return (
+ indices,
+ cu_seqlens,
+ max_seqlen_in_batch,
+ )
+
+
+# Copied from transformers.models.llama.modeling_llama.LlamaRMSNorm with Llama->Mixtral
+class MixtralRMSNorm(nn.Module):
+
+ def __init__(self, hidden_size, eps=1e-6):
+ """MixtralRMSNorm is equivalent to T5LayerNorm."""
+ super().__init__()
+ self.weight = nn.Parameter(torch.ones(hidden_size))
+ self.variance_epsilon = eps
+
+ def forward(self, hidden_states):
+ input_dtype = hidden_states.dtype
+ hidden_states = hidden_states.to(torch.float32)
+ variance = hidden_states.pow(2).mean(-1, keepdim=True)
+ hidden_states = hidden_states * torch.rsqrt(variance +
+ self.variance_epsilon)
+ return self.weight * hidden_states.to(input_dtype)
+
+
+# Copied from transformers.models.mistral.modeling_mistral.MistralRotaryEmbedding with Mistral->Mixtral
+class MixtralRotaryEmbedding(nn.Module):
+
+ def __init__(self,
+ dim,
+ max_position_embeddings=2048,
+ base=10000,
+ device=None):
+ super().__init__()
+
+ self.dim = dim
+ self.max_position_embeddings = max_position_embeddings
+ self.base = base
+ inv_freq = 1.0 / (
+ self.base
+ **(torch.arange(0, self.dim, 2,
+ dtype=torch.int64).float().to(device) / self.dim))
+ self.register_buffer('inv_freq', inv_freq, persistent=False)
+
+ # Build here to make `torch.jit.trace` work.
+ self._set_cos_sin_cache(
+ seq_len=max_position_embeddings,
+ device=self.inv_freq.device,
+ dtype=torch.get_default_dtype())
+
+ def _set_cos_sin_cache(self, seq_len, device, dtype):
+ self.max_seq_len_cached = seq_len
+ t = torch.arange(
+ self.max_seq_len_cached, device=device,
+ dtype=torch.int64).type_as(self.inv_freq)
+
+ freqs = torch.outer(t, self.inv_freq)
+ # Different from paper, but it uses a different permutation in order to obtain the same calculation
+ emb = torch.cat((freqs, freqs), dim=-1)
+ self.register_buffer(
+ 'cos_cached', emb.cos().to(dtype), persistent=False)
+ self.register_buffer(
+ 'sin_cached', emb.sin().to(dtype), persistent=False)
+
+ def forward(self, x, seq_len=None):
+ # x: [bs, num_attention_heads, seq_len, head_size]
+ if seq_len > self.max_seq_len_cached:
+ self._set_cos_sin_cache(
+ seq_len=seq_len, device=x.device, dtype=x.dtype)
+
+ return (
+ self.cos_cached[:seq_len].to(dtype=x.dtype),
+ self.sin_cached[:seq_len].to(dtype=x.dtype),
+ )
+
+
+# Copied from transformers.models.llama.modeling_llama.rotate_half
+def rotate_half(x):
+ """Rotates half the hidden dims of the input."""
+ x1 = x[..., :x.shape[-1] // 2]
+ x2 = x[..., x.shape[-1] // 2:]
+ return torch.cat((-x2, x1), dim=-1)
+
+
+# Copied from transformers.models.mistral.modeling_mistral.apply_rotary_pos_emb
+def apply_rotary_pos_emb(q, k, cos, sin, position_ids, unsqueeze_dim=1):
+ """Applies Rotary Position Embedding to the query and key tensors.
+
+ Args:
+ q (`torch.Tensor`): The query tensor.
+ k (`torch.Tensor`): The key tensor.
+ cos (`torch.Tensor`): The cosine part of the rotary embedding.
+ sin (`torch.Tensor`): The sine part of the rotary embedding.
+ position_ids (`torch.Tensor`):
+ The position indices of the tokens corresponding to the query and key tensors. For example, this can be
+ used to pass offsetted position ids when working with a KV-cache.
+ unsqueeze_dim (`int`, *optional*, defaults to 1):
+ The 'unsqueeze_dim' argument specifies the dimension along which to unsqueeze cos[position_ids] and
+ sin[position_ids] so that they can be properly broadcasted to the dimensions of q and k. For example, note
+ that cos[position_ids] and sin[position_ids] have the shape [batch_size, seq_len, head_dim]. Then, if q and
+ k have the shape [batch_size, heads, seq_len, head_dim], then setting unsqueeze_dim=1 makes
+ cos[position_ids] and sin[position_ids] broadcastable to the shapes of q and k. Similarly, if q and k have
+ the shape [batch_size, seq_len, heads, head_dim], then set unsqueeze_dim=2.
+ Returns:
+ `tuple(torch.Tensor)` comprising of the query and key tensors rotated using the Rotary Position Embedding.
+ """
+ cos = cos[position_ids].unsqueeze(unsqueeze_dim)
+ sin = sin[position_ids].unsqueeze(unsqueeze_dim)
+ q_embed = (q * cos) + (rotate_half(q) * sin)
+ k_embed = (k * cos) + (rotate_half(k) * sin)
+ return q_embed, k_embed
+
+
+# Copied from transformers.models.llama.modeling_llama.repeat_kv
+def repeat_kv(hidden_states: torch.Tensor, n_rep: int) -> torch.Tensor:
+ """This is the equivalent of torch.repeat_interleave(x, dim=1,
+ repeats=n_rep).
+
+ The hidden states go from (batch, num_key_value_heads, seqlen, head_dim) to
+ (batch, num_attention_heads, seqlen, head_dim)
+ """
+ batch, num_key_value_heads, slen, head_dim = hidden_states.shape
+ if n_rep == 1:
+ return hidden_states
+ hidden_states = hidden_states[:, :,
+ None, :, :].expand(batch,
+ num_key_value_heads,
+ n_rep, slen, head_dim)
+ return hidden_states.reshape(batch, num_key_value_heads * n_rep, slen,
+ head_dim)
+
+
+# Copied from transformers.models.mistral.modeling_mistral.MistralAttention with Mistral->Mixtral
+class MixtralAttention(nn.Module):
+ """Multi-headed attention from 'Attention Is All You Need' paper.
+
+ Modified to use sliding window attention: Longformer and "Generating Long
+ Sequences with Sparse Transformers".
+ """
+
+ def __init__(self, config: MixtralConfig, layer_idx: Optional[int] = None):
+ super().__init__()
+ self.config = config
+ self.layer_idx = layer_idx
+ if layer_idx is None:
+ logger.warning_once(
+ f'Instantiating {self.__class__.__name__} without passing a `layer_idx` is not recommended and will '
+ 'lead to errors during the forward call if caching is used. Please make sure to provide a `layer_idx` '
+ 'when creating this class.')
+
+ self.hidden_size = config.hidden_size
+ self.num_heads = config.num_attention_heads
+ self.head_dim = self.hidden_size // self.num_heads
+ self.num_key_value_heads = config.num_key_value_heads
+ self.num_key_value_groups = self.num_heads // self.num_key_value_heads
+ self.max_position_embeddings = config.max_position_embeddings
+ self.rope_theta = config.rope_theta
+ self.is_causal = True
+ self.attention_dropout = config.attention_dropout
+
+ if (self.head_dim * self.num_heads) != self.hidden_size:
+ raise ValueError(
+ f'hidden_size must be divisible by num_heads (got `hidden_size`: {self.hidden_size}'
+ f' and `num_heads`: {self.num_heads}).')
+ self.q_proj = nn.Linear(
+ self.hidden_size, self.num_heads * self.head_dim, bias=False)
+ self.k_proj = nn.Linear(
+ self.hidden_size,
+ self.num_key_value_heads * self.head_dim,
+ bias=False)
+ self.v_proj = nn.Linear(
+ self.hidden_size,
+ self.num_key_value_heads * self.head_dim,
+ bias=False)
+ self.o_proj = nn.Linear(
+ self.num_heads * self.head_dim, self.hidden_size, bias=False)
+
+ self.rotary_emb = MixtralRotaryEmbedding(
+ self.head_dim,
+ max_position_embeddings=self.max_position_embeddings,
+ base=self.rope_theta,
+ )
+
+ def _shape(self, tensor: torch.Tensor, seq_len: int, bsz: int):
+ return tensor.view(bsz, seq_len, self.num_heads,
+ self.head_dim).transpose(1, 2).contiguous()
+
+ def forward(
+ self,
+ hidden_states: torch.Tensor,
+ attention_mask: Optional[torch.Tensor] = None,
+ position_ids: Optional[torch.LongTensor] = None,
+ past_key_value: Optional[Cache] = None,
+ output_attentions: bool = False,
+ use_cache: bool = False,
+ ) -> Tuple[torch.Tensor, Optional[torch.Tensor],
+ Optional[Tuple[torch.Tensor]]]:
+ bsz, q_len, _ = hidden_states.size()
+
+ query_states = self.q_proj(hidden_states)
+ key_states = self.k_proj(hidden_states)
+ value_states = self.v_proj(hidden_states)
+
+ query_states = query_states.view(bsz, q_len, self.num_heads,
+ self.head_dim).transpose(1, 2)
+ key_states = key_states.view(bsz, q_len, self.num_key_value_heads,
+ self.head_dim).transpose(1, 2)
+ value_states = value_states.view(bsz, q_len, self.num_key_value_heads,
+ self.head_dim).transpose(1, 2)
+
+ kv_seq_len = key_states.shape[-2]
+ if past_key_value is not None:
+ if self.layer_idx is None:
+ raise ValueError(
+ f'The cache structure has changed since version v4.36. If you are using {self.__class__.__name__} '
+ 'for auto-regressive decoding with k/v caching, please make sure to initialize the attention class '
+ 'with a layer index.')
+ kv_seq_len += past_key_value.get_usable_length(
+ kv_seq_len, self.layer_idx)
+ cos, sin = self.rotary_emb(value_states, seq_len=kv_seq_len)
+ query_states, key_states = apply_rotary_pos_emb(
+ query_states, key_states, cos, sin, position_ids)
+
+ if past_key_value is not None:
+ cache_kwargs = {'sin': sin, 'cos': cos} # Specific to RoPE models
+ key_states, value_states = past_key_value.update(
+ key_states, value_states, self.layer_idx, cache_kwargs)
+
+ # repeat k/v heads if n_kv_heads < n_heads
+ key_states = repeat_kv(key_states, self.num_key_value_groups)
+ value_states = repeat_kv(value_states, self.num_key_value_groups)
+
+ attn_weights = torch.matmul(query_states, key_states.transpose(
+ 2, 3)) / math.sqrt(self.head_dim)
+
+ if attn_weights.size() != (bsz, self.num_heads, q_len, kv_seq_len):
+ raise ValueError(
+ f'Attention weights should be of size {(bsz, self.num_heads, q_len, kv_seq_len)}, but is'
+ f' {attn_weights.size()}')
+
+ if attention_mask is not None:
+ if attention_mask.size() != (bsz, 1, q_len, kv_seq_len):
+ raise ValueError(
+ f'Attention mask should be of size {(bsz, 1, q_len, kv_seq_len)}, but is {attention_mask.size()}'
+ )
+
+ attn_weights = attn_weights + attention_mask
+
+ # upcast attention to fp32
+ attn_weights = nn.functional.softmax(
+ attn_weights, dim=-1, dtype=torch.float32).to(query_states.dtype)
+ attn_weights = nn.functional.dropout(
+ attn_weights, p=self.attention_dropout, training=self.training)
+ attn_output = torch.matmul(attn_weights, value_states)
+
+ if attn_output.size() != (bsz, self.num_heads, q_len, self.head_dim):
+ raise ValueError(
+ f'`attn_output` should be of size {(bsz, self.num_heads, q_len, self.head_dim)}, but is'
+ f' {attn_output.size()}')
+
+ attn_output = attn_output.transpose(1, 2).contiguous()
+ attn_output = attn_output.reshape(bsz, q_len, self.hidden_size)
+
+ attn_output = self.o_proj(attn_output)
+
+ if not output_attentions:
+ attn_weights = None
+
+ return attn_output, attn_weights, past_key_value
+
+
+# Copied from transformers.models.mistral.modeling_mistral.MistralFlashAttention2 with Mistral->Mixtral
+class MixtralFlashAttention2(MixtralAttention):
+ """Mixtral flash attention module.
+
+ This module inherits from `MixtralAttention` as the weights of the module
+ stays untouched. The only required change would be on the forward pass
+ where it needs to correctly call the public API of flash attention and deal
+ with padding tokens in case the input contains any of them.
+ """
+
+ # Copied from transformers.models.llama.modeling_llama.LlamaFlashAttention2.__init__
+ def __init__(self, *args, **kwargs):
+ super().__init__(*args, **kwargs)
+
+ # TODO: Should be removed once Flash Attention for RoCm is bumped to 2.1.
+ # flash_attn<2.1 generates top-left aligned causal mask, while what is needed here is bottom-right alignment, that was made default for flash_attn>=2.1. This attribute is used to handle this difference. Reference: https://github.com/Dao-AILab/flash-attention/releases/tag/v2.1.0.
+ # Beware that with flash_attn<2.1, using q_seqlen != k_seqlen (except for the case q_seqlen == 1) produces a wrong mask (top-left).
+ self._flash_attn_uses_top_left_mask = not is_flash_attn_greater_or_equal_2_10(
+ )
+
+ def forward(
+ self,
+ hidden_states: torch.Tensor,
+ attention_mask: Optional[torch.Tensor] = None,
+ position_ids: Optional[torch.LongTensor] = None,
+ past_key_value: Optional[Cache] = None,
+ output_attentions: bool = False,
+ use_cache: bool = False,
+ ):
+ bsz, q_len, _ = hidden_states.size()
+
+ query_states = self.q_proj(hidden_states)
+ key_states = self.k_proj(hidden_states)
+ value_states = self.v_proj(hidden_states)
+
+ query_states = query_states.view(bsz, q_len, self.num_heads,
+ self.head_dim).transpose(1, 2)
+ key_states = key_states.view(bsz, q_len, self.num_key_value_heads,
+ self.head_dim).transpose(1, 2)
+ value_states = value_states.view(bsz, q_len, self.num_key_value_heads,
+ self.head_dim).transpose(1, 2)
+
+ kv_seq_len = key_states.shape[-2]
+ if past_key_value is not None:
+ if self.layer_idx is None:
+ raise ValueError(
+ f'The cache structure has changed since version v4.36. If you are using {self.__class__.__name__} '
+ 'for auto-regressive decoding with k/v caching, please make sure to initialize the attention class '
+ 'with a layer index.')
+ kv_seq_len += past_key_value.get_usable_length(
+ kv_seq_len, self.layer_idx)
+
+ # Because the input can be padded, the absolute sequence length depends on the max position id.
+ rotary_seq_len = max(kv_seq_len, position_ids[:, -1].max().item()) + 1
+ cos, sin = self.rotary_emb(value_states, seq_len=rotary_seq_len)
+
+ query_states, key_states = apply_rotary_pos_emb(
+ query_states, key_states, cos, sin, position_ids)
+
+ use_sliding_windows = (
+ _flash_supports_window_size
+ and getattr(self.config, 'sliding_window', None) is not None
+ and kv_seq_len > self.config.sliding_window)
+
+ if not _flash_supports_window_size:
+ logger.warning_once(
+ 'The current flash attention version does not support sliding window attention, for a more memory efficient implementation'
+ ' make sure to upgrade flash-attn library.')
+
+ if past_key_value is not None:
+ # Activate slicing cache only if the config has a value `sliding_windows` attribute
+ cache_has_contents = past_key_value.get_seq_length(
+ self.layer_idx) > 0
+ if (getattr(self.config, 'sliding_window', None) is not None
+ and kv_seq_len > self.config.sliding_window
+ and cache_has_contents):
+ slicing_tokens = 1 - self.config.sliding_window
+
+ past_key = past_key_value[self.layer_idx][0]
+ past_value = past_key_value[self.layer_idx][1]
+
+ past_key = past_key[:, :, slicing_tokens:, :].contiguous()
+ past_value = past_value[:, :, slicing_tokens:, :].contiguous()
+
+ if past_key.shape[-2] != self.config.sliding_window - 1:
+ raise ValueError(
+ f'past key must have a shape of (`batch_size, num_heads, self.config.sliding_window-1, head_dim`), got'
+ f' {past_key.shape}')
+
+ if attention_mask is not None:
+ attention_mask = attention_mask[:, slicing_tokens:]
+ attention_mask = torch.cat([
+ attention_mask,
+ torch.ones_like(attention_mask[:, -1:])
+ ],
+ dim=-1)
+
+ cache_kwargs = {'sin': sin, 'cos': cos} # Specific to RoPE models
+ key_states, value_states = past_key_value.update(
+ key_states, value_states, self.layer_idx, cache_kwargs)
+
+ # repeat k/v heads if n_kv_heads < n_heads
+ key_states = repeat_kv(key_states, self.num_key_value_groups)
+ value_states = repeat_kv(value_states, self.num_key_value_groups)
+ dropout_rate = 0.0 if not self.training else self.attention_dropout
+
+ # In PEFT, usually we cast the layer norms in float32 for training stability reasons
+ # therefore the input hidden states gets silently casted in float32. Hence, we need
+ # cast them back in float16 just to be sure everything works as expected.
+ input_dtype = query_states.dtype
+ if input_dtype == torch.float32:
+ if torch.is_autocast_enabled():
+ target_dtype = torch.get_autocast_gpu_dtype()
+ # Handle the case where the model is quantized
+ elif hasattr(self.config, '_pre_quantization_dtype'):
+ target_dtype = self.config._pre_quantization_dtype
+ else:
+ target_dtype = self.q_proj.weight.dtype
+
+ logger.warning_once(
+ f'The input hidden states seems to be silently casted in float32, this might be related to'
+ f' the fact you have upcasted embedding or layer norm layers in float32. We will cast back the input in'
+ f' {target_dtype}.')
+
+ query_states = query_states.to(target_dtype)
+ key_states = key_states.to(target_dtype)
+ value_states = value_states.to(target_dtype)
+
+ # Reashape to the expected shape for Flash Attention
+ query_states = query_states.transpose(1, 2)
+ key_states = key_states.transpose(1, 2)
+ value_states = value_states.transpose(1, 2)
+
+ attn_output = self._flash_attention_forward(
+ query_states,
+ key_states,
+ value_states,
+ attention_mask,
+ q_len,
+ dropout=dropout_rate,
+ use_sliding_windows=use_sliding_windows,
+ )
+
+ attn_output = attn_output.reshape(bsz, q_len,
+ self.hidden_size).contiguous()
+ attn_output = self.o_proj(attn_output)
+
+ if not output_attentions:
+ attn_weights = None
+
+ return attn_output, attn_weights, past_key_value
+
+ def _flash_attention_forward(
+ self,
+ query_states,
+ key_states,
+ value_states,
+ attention_mask,
+ query_length,
+ dropout=0.0,
+ softmax_scale=None,
+ use_sliding_windows=False,
+ ):
+ """
+ Calls the forward method of Flash Attention - if the input hidden states contain at least one padding token
+ first unpad the input, then computes the attention scores and pad the final attention scores.
+
+ Args:
+ query_states (`torch.Tensor`):
+ Input query states to be passed to Flash Attention API
+ key_states (`torch.Tensor`):
+ Input key states to be passed to Flash Attention API
+ value_states (`torch.Tensor`):
+ Input value states to be passed to Flash Attention API
+ attention_mask (`torch.Tensor`):
+ The padding mask - corresponds to a tensor of size `(batch_size, seq_len)` where 0 stands for the
+ position of padding tokens and 1 for the position of non-padding tokens.
+ dropout (`float`):
+ Attention dropout
+ softmax_scale (`float`, *optional*):
+ The scaling of QK^T before applying softmax. Default to 1 / sqrt(head_dim)
+ use_sliding_windows (`bool`, *optional*):
+ Whether to activate sliding window attention.
+ """
+ if not self._flash_attn_uses_top_left_mask:
+ causal = self.is_causal
+ else:
+ # TODO: Remove the `query_length != 1` check once Flash Attention for RoCm is bumped to 2.1. For details, please see the comment in LlamaFlashAttention2 __init__.
+ causal = self.is_causal and query_length != 1
+
+ # Contains at least one padding token in the sequence
+ if attention_mask is not None:
+ batch_size = query_states.shape[0]
+ query_states, key_states, value_states, indices_q, cu_seq_lens, max_seq_lens = self._upad_input(
+ query_states, key_states, value_states, attention_mask,
+ query_length)
+
+ cu_seqlens_q, cu_seqlens_k = cu_seq_lens
+ max_seqlen_in_batch_q, max_seqlen_in_batch_k = max_seq_lens
+
+ if not use_sliding_windows:
+ attn_output_unpad = flash_attn_varlen_func(
+ query_states,
+ key_states,
+ value_states,
+ cu_seqlens_q=cu_seqlens_q,
+ cu_seqlens_k=cu_seqlens_k,
+ max_seqlen_q=max_seqlen_in_batch_q,
+ max_seqlen_k=max_seqlen_in_batch_k,
+ dropout_p=dropout,
+ softmax_scale=softmax_scale,
+ causal=causal,
+ )
+ else:
+ attn_output_unpad = flash_attn_varlen_func(
+ query_states,
+ key_states,
+ value_states,
+ cu_seqlens_q=cu_seqlens_q,
+ cu_seqlens_k=cu_seqlens_k,
+ max_seqlen_q=max_seqlen_in_batch_q,
+ max_seqlen_k=max_seqlen_in_batch_k,
+ dropout_p=dropout,
+ softmax_scale=softmax_scale,
+ causal=causal,
+ window_size=(self.config.sliding_window,
+ self.config.sliding_window),
+ )
+
+ attn_output = pad_input(attn_output_unpad, indices_q, batch_size,
+ query_length)
+ else:
+ if not use_sliding_windows:
+ attn_output = flash_attn_func(
+ query_states,
+ key_states,
+ value_states,
+ dropout,
+ softmax_scale=softmax_scale,
+ causal=causal,
+ )
+ else:
+ attn_output = flash_attn_func(
+ query_states,
+ key_states,
+ value_states,
+ dropout,
+ softmax_scale=softmax_scale,
+ causal=causal,
+ window_size=(self.config.sliding_window,
+ self.config.sliding_window),
+ )
+
+ return attn_output
+
+ def _upad_input(self, query_layer, key_layer, value_layer, attention_mask,
+ query_length):
+ batch_size, kv_seq_len, num_heads, head_dim = key_layer.shape
+
+ # On the first iteration we need to properly re-create the padding mask
+ # by slicing it on the proper place
+ if kv_seq_len != attention_mask.shape[-1]:
+ attention_mask_num_tokens = attention_mask.shape[-1]
+ attention_mask = attention_mask[:, attention_mask_num_tokens -
+ kv_seq_len:]
+
+ indices_k, cu_seqlens_k, max_seqlen_in_batch_k = _get_unpad_data(
+ attention_mask)
+
+ key_layer = index_first_axis(
+ key_layer.reshape(batch_size * kv_seq_len, num_heads, head_dim),
+ indices_k)
+ value_layer = index_first_axis(
+ value_layer.reshape(batch_size * kv_seq_len, num_heads, head_dim),
+ indices_k)
+
+ if query_length == kv_seq_len:
+ query_layer = index_first_axis(
+ query_layer.reshape(batch_size * kv_seq_len, num_heads,
+ head_dim), indices_k)
+ cu_seqlens_q = cu_seqlens_k
+ max_seqlen_in_batch_q = max_seqlen_in_batch_k
+ indices_q = indices_k
+ elif query_length == 1:
+ max_seqlen_in_batch_q = 1
+ cu_seqlens_q = torch.arange(
+ batch_size + 1, dtype=torch.int32, device=query_layer.device
+ ) # There is a memcpy here, that is very bad.
+ indices_q = cu_seqlens_q[:-1]
+ query_layer = query_layer.squeeze(1)
+ else:
+ # The -q_len: slice assumes left padding.
+ attention_mask = attention_mask[:, -query_length:]
+ query_layer, indices_q, cu_seqlens_q, max_seqlen_in_batch_q = unpad_input(
+ query_layer, attention_mask)
+
+ return (
+ query_layer,
+ key_layer,
+ value_layer,
+ indices_q,
+ (cu_seqlens_q, cu_seqlens_k),
+ (max_seqlen_in_batch_q, max_seqlen_in_batch_k),
+ )
+
+
+# Copied from transformers.models.mistral.modeling_mistral.MistralSdpaAttention with Mistral->Mixtral
+class MixtralSdpaAttention(MixtralAttention):
+ """Mixtral attention module using
+ torch.nn.functional.scaled_dot_product_attention.
+
+ This module inherits from `MixtralAttention` as the weights of the module
+ stays untouched. The only changes are on the forward pass to adapt to SDPA
+ API.
+ """
+
+ # Adapted from MixtralAttention.forward
+ def forward(
+ self,
+ hidden_states: torch.Tensor,
+ attention_mask: Optional[torch.Tensor] = None,
+ position_ids: Optional[torch.LongTensor] = None,
+ past_key_value: Optional[Cache] = None,
+ output_attentions: bool = False,
+ use_cache: bool = False,
+ ) -> Tuple[torch.Tensor, Optional[torch.Tensor],
+ Optional[Tuple[torch.Tensor]]]:
+ if output_attentions:
+ # TODO: Improve this warning with e.g. `model.config.attn_implementation = "manual"` once this is implemented.
+ logger.warning_once(
+ 'MixtralModel is using MixtralSdpaAttention, but `torch.nn.functional.scaled_dot_product_attention` does not support `output_attentions=True`. Falling back to the manual attention implementation, '
+ 'but specifying the manual implementation will be required from Transformers version v5.0.0 onwards. This warning can be removed using the argument `attn_implementation="eager"` when loading the model.'
+ )
+ return super().forward(
+ hidden_states=hidden_states,
+ attention_mask=attention_mask,
+ position_ids=position_ids,
+ past_key_value=past_key_value,
+ output_attentions=output_attentions,
+ use_cache=use_cache,
+ )
+
+ bsz, q_len, _ = hidden_states.size()
+
+ query_states = self.q_proj(hidden_states)
+ key_states = self.k_proj(hidden_states)
+ value_states = self.v_proj(hidden_states)
+
+ query_states = query_states.view(bsz, q_len, self.num_heads,
+ self.head_dim).transpose(1, 2)
+ key_states = key_states.view(bsz, q_len, self.num_key_value_heads,
+ self.head_dim).transpose(1, 2)
+ value_states = value_states.view(bsz, q_len, self.num_key_value_heads,
+ self.head_dim).transpose(1, 2)
+
+ kv_seq_len = key_states.shape[-2]
+ if past_key_value is not None:
+ kv_seq_len += past_key_value.get_usable_length(
+ kv_seq_len, self.layer_idx)
+ cos, sin = self.rotary_emb(value_states, seq_len=kv_seq_len)
+
+ query_states, key_states = apply_rotary_pos_emb(
+ query_states, key_states, cos, sin, position_ids)
+
+ if past_key_value is not None:
+ cache_kwargs = {'sin': sin, 'cos': cos} # Specific to RoPE models
+ key_states, value_states = past_key_value.update(
+ key_states, value_states, self.layer_idx, cache_kwargs)
+
+ key_states = repeat_kv(key_states, self.num_key_value_groups)
+ value_states = repeat_kv(value_states, self.num_key_value_groups)
+
+ if attention_mask is not None:
+ if attention_mask.size() != (bsz, 1, q_len, kv_seq_len):
+ raise ValueError(
+ f'Attention mask should be of size {(bsz, 1, q_len, kv_seq_len)}, but is {attention_mask.size()}'
+ )
+
+ # SDPA with memory-efficient backend is currently (torch==2.1.2) bugged with non-contiguous inputs with custom attn_mask,
+ # Reference: https://github.com/pytorch/pytorch/issues/112577.
+ if query_states.device.type != 'cpu' and attention_mask is not None:
+ query_states = query_states.contiguous()
+ key_states = key_states.contiguous()
+ value_states = value_states.contiguous()
+
+ attn_output = torch.nn.functional.scaled_dot_product_attention(
+ query_states,
+ key_states,
+ value_states,
+ attn_mask=attention_mask,
+ dropout_p=self.attention_dropout if self.training else 0.0,
+ # The q_len > 1 is necessary to match with AttentionMaskConverter.to_causal_4d that does not create a causal mask in case q_len == 1.
+ is_causal=self.is_causal and attention_mask is None and q_len > 1,
+ )
+
+ attn_output = attn_output.transpose(1, 2).contiguous()
+ attn_output = attn_output.view(bsz, q_len, self.hidden_size)
+
+ attn_output = self.o_proj(attn_output)
+
+ return attn_output, None, past_key_value
+
+
+MIXTRAL_ATTENTION_CLASSES = {
+ 'eager': MixtralAttention,
+ 'flash_attention_2': MixtralFlashAttention2,
+ 'sdpa': MixtralSdpaAttention,
+}
+
+
+class MixtralBlockSparseTop2MLP(nn.Module):
+
+ def __init__(self, config: MixtralConfig):
+ super().__init__()
+ self.ffn_dim = config.intermediate_size
+ self.hidden_dim = config.hidden_size
+
+ self.w1 = nn.Linear(self.hidden_dim, self.ffn_dim, bias=False)
+ self.w2 = nn.Linear(self.ffn_dim, self.hidden_dim, bias=False)
+ self.w3 = nn.Linear(self.hidden_dim, self.ffn_dim, bias=False)
+
+ self.act_fn = ACT2FN[config.hidden_act]
+
+ def forward(self, hidden_states):
+ current_hidden_states = self.act_fn(
+ self.w1(hidden_states)) * self.w3(hidden_states)
+ current_hidden_states = self.w2(current_hidden_states)
+ return current_hidden_states
+
+
+class MixtralSparseMoeBlock(nn.Module):
+ """This implementation is strictly equivalent to standard MoE with full
+ capacity (no dropped tokens).
+
+ It's faster since it formulates MoE operations in terms of block-sparse
+ operations to accommodate imbalanced assignments of tokens to experts,
+ whereas standard MoE either (1) drop tokens at the cost of reduced
+ performance or (2) set capacity factor to number of experts and thus waste
+ computation and memory on padding.
+ """
+
+ def __init__(self, config):
+ super().__init__()
+ self.hidden_dim = config.hidden_size
+ self.ffn_dim = config.intermediate_size
+ self.num_experts = config.num_local_experts
+ self.top_k = config.num_experts_per_tok
+
+ # gating
+ self.gate = nn.Linear(self.hidden_dim, self.num_experts, bias=False)
+
+ self.experts = nn.ModuleList([
+ MixtralBlockSparseTop2MLP(config) for _ in range(self.num_experts)
+ ])
+
+ # Jitter parameters
+ self.jitter_noise = config.router_jitter_noise
+
+ def forward(self, hidden_states: torch.Tensor) -> torch.Tensor:
+ """"""
+ batch_size, sequence_length, hidden_dim = hidden_states.shape
+ if self.training and self.jitter_noise > 0:
+ hidden_states *= torch.empty_like(hidden_states).uniform_(
+ 1.0 - self.jitter_noise, 1.0 + self.jitter_noise)
+ hidden_states = hidden_states.view(-1, hidden_dim)
+ # router_logits: (batch * sequence_length, n_experts)
+ router_logits = self.gate(hidden_states)
+
+ routing_weights = F.softmax(router_logits, dim=1, dtype=torch.float)
+ routing_weights, selected_experts = torch.topk(
+ routing_weights, self.top_k, dim=-1)
+ routing_weights /= routing_weights.sum(dim=-1, keepdim=True)
+ # we cast back to the input dtype
+ routing_weights = routing_weights.to(hidden_states.dtype)
+
+ final_hidden_states = torch.zeros(
+ (batch_size * sequence_length, hidden_dim),
+ dtype=hidden_states.dtype,
+ device=hidden_states.device)
+
+ # One hot encode the selected experts to create an expert mask
+ # this will be used to easily index which expert is going to be sollicitated
+ expert_mask = torch.nn.functional.one_hot(
+ selected_experts, num_classes=self.num_experts).permute(2, 1, 0)
+
+ # Loop over all available experts in the model and perform the computation on each expert
+ for expert_idx in range(self.num_experts):
+ expert_layer = self.experts[expert_idx]
+ idx, top_x = torch.where(expert_mask[expert_idx])
+
+ # Index the correct hidden states and compute the expert hidden state for
+ # the current expert. We need to make sure to multiply the output hidden
+ # states by `routing_weights` on the corresponding tokens (top-1 and top-2)
+ current_state = hidden_states[None, top_x].reshape(-1, hidden_dim)
+ current_hidden_states = expert_layer(
+ current_state) * routing_weights[top_x, idx, None]
+
+ # However `index_add_` only support torch tensors for indexing so we'll use
+ # the `top_x` tensor here.
+ final_hidden_states.index_add_(
+ 0, top_x, current_hidden_states.to(hidden_states.dtype))
+ final_hidden_states = final_hidden_states.reshape(
+ batch_size, sequence_length, hidden_dim)
+ return final_hidden_states, router_logits
+
+
+class ExpertShard(nn.Module):
+
+ def __init__(self, config, expert_in_one_shard=1):
+ super().__init__()
+ self.w1w3 = nn.Parameter(
+ torch.empty(expert_in_one_shard, config.intermediate_size * 2,
+ config.hidden_size))
+ self.w2 = nn.Parameter(
+ torch.empty(expert_in_one_shard, config.hidden_size,
+ config.intermediate_size))
+ self.act = ACT2FN[config.hidden_act]
+ self.expert_in_one_shard = expert_in_one_shard
+
+ def forward(self, hidden_states, expert_mask, routing_weights,
+ final_hidden_states):
+ hidden_dim = hidden_states.shape[-1]
+ for expert_idx in range(self.expert_in_one_shard):
+ idx, top_x = torch.where(expert_mask[expert_idx])
+ current_state = hidden_states[None, top_x].reshape(-1, hidden_dim)
+
+ w1w3 = self.w1w3[expert_idx]
+ w2 = self.w2[expert_idx]
+ gate_up_out = torch.matmul(current_state, w1w3.T)
+ gate_out, up_out = gate_up_out.chunk(2, dim=-1)
+ gate_out = self.act(gate_out)
+ out = gate_out * up_out
+ out = torch.matmul(out, w2.T)
+
+ current_hidden_states = out * routing_weights[top_x, idx, None]
+ final_hidden_states.index_add_(
+ 0, top_x, current_hidden_states.to(hidden_states.dtype))
+ return final_hidden_states
+
+
+class MixtralSparseShardMoeBlock(nn.Module):
+
+ def __init__(self, config):
+ super().__init__()
+ self.hidden_dim = config.hidden_size
+ self.ffn_dim = config.intermediate_size
+ self.num_experts = config.num_local_experts
+ self.top_k = config.num_experts_per_tok
+
+ # gating
+ self.gate = nn.Linear(self.hidden_dim, self.num_experts, bias=False)
+
+ expert_in_one_shard = config.expert_in_one_shard
+ assert config.num_local_experts % expert_in_one_shard == 0, \
+ ('num_local_experts should be divisible by expert_in_one_shard, but got '
+ f'num_local_experts = {config.num_local_experts} and expert_in_one_shard = {expert_in_one_shard}')
+ self.shard_num = config.num_local_experts // expert_in_one_shard
+ self.expert_in_one_shard = expert_in_one_shard
+ self.experts = nn.ModuleList([
+ ExpertShard(config, self.expert_in_one_shard)
+ for i in range(self.shard_num)
+ ])
+
+ # Jitter parameters
+ self.jitter_noise = config.router_jitter_noise
+
+ def forward(self, hidden_states: torch.Tensor) -> torch.Tensor:
+ """"""
+ batch_size, sequence_length, hidden_dim = hidden_states.shape
+ if self.training and self.jitter_noise > 0:
+ hidden_states *= torch.empty_like(hidden_states).uniform_(
+ 1.0 - self.jitter_noise, 1.0 + self.jitter_noise)
+ hidden_states = hidden_states.view(-1, hidden_dim)
+ # router_logits: (batch * sequence_length, n_experts)
+ router_logits = self.gate(hidden_states)
+
+ routing_weights = F.softmax(router_logits, dim=1, dtype=torch.float)
+ routing_weights, selected_experts = torch.topk(
+ routing_weights, self.top_k, dim=-1)
+ routing_weights /= routing_weights.sum(dim=-1, keepdim=True)
+ # we cast back to the input dtype
+ routing_weights = routing_weights.to(hidden_states.dtype)
+
+ final_hidden_states = torch.zeros(
+ (batch_size * sequence_length, hidden_dim),
+ dtype=hidden_states.dtype,
+ device=hidden_states.device)
+
+ # One hot encode the selected experts to create an expert mask
+ # this will be used to easily index which expert is going to be sollicitated
+ expert_mask = torch.nn.functional.one_hot(
+ selected_experts, num_classes=self.num_experts).permute(2, 1, 0)
+
+ # Loop over all available experts in the model and perform the computation on each expert
+ for shard_index in range(self.shard_num):
+ mask = expert_mask[shard_index *
+ self.expert_in_one_shard:(shard_index + 1) *
+ self.expert_in_one_shard]
+ final_hidden_states = self.experts[shard_index](
+ hidden_states, mask, routing_weights, final_hidden_states)
+
+ final_hidden_states = final_hidden_states.reshape(
+ batch_size, sequence_length, hidden_dim)
+ return final_hidden_states, router_logits
+
+
+class MixtralDecoderLayer(nn.Module):
+
+ def __init__(self, config: MixtralConfig, layer_idx: int):
+ super().__init__()
+ self.hidden_size = config.hidden_size
+
+ self.self_attn = MIXTRAL_ATTENTION_CLASSES[
+ config._attn_implementation](config, layer_idx)
+
+ moe_implementation = config.moe_implementation
+ if moe_implementation == 'origin':
+ block = MixtralSparseMoeBlock
+ elif moe_implementation == 'shard':
+ block = MixtralSparseShardMoeBlock
+ else:
+ raise NotImplementedError
+ self.block_sparse_moe = block(config)
+
+ self.input_layernorm = MixtralRMSNorm(
+ config.hidden_size, eps=config.rms_norm_eps)
+ self.post_attention_layernorm = MixtralRMSNorm(
+ config.hidden_size, eps=config.rms_norm_eps)
+
+ def forward(
+ self,
+ hidden_states: torch.Tensor,
+ attention_mask: Optional[torch.Tensor] = None,
+ position_ids: Optional[torch.LongTensor] = None,
+ past_key_value: Optional[Tuple[torch.Tensor]] = None,
+ output_attentions: Optional[bool] = False,
+ output_router_logits: Optional[bool] = False,
+ use_cache: Optional[bool] = False,
+ ) -> Tuple[torch.FloatTensor, Optional[Tuple[torch.FloatTensor,
+ torch.FloatTensor]]]:
+ """
+ Args:
+ hidden_states (`torch.FloatTensor`): input to the layer of shape `(batch, seq_len, embed_dim)`
+ attention_mask (`torch.FloatTensor`, *optional*): attention mask of size
+ `(batch, sequence_length)` where padding elements are indicated by 0.
+ past_key_value (`Tuple(torch.FloatTensor)`, *optional*): cached past key and value projection states
+ output_attentions (`bool`, *optional*):
+ Whether or not to return the attentions tensors of all attention layers. See `attentions` under
+ returned tensors for more detail.
+ output_router_logits (`bool`, *optional*):
+ Whether or not to return the logits of all the routers. They are useful for computing the router loss, and
+ should not be returned during inference.
+ use_cache (`bool`, *optional*):
+ If set to `True`, `past_key_values` key value states are returned and can be used to speed up decoding
+ (see `past_key_values`).
+ """
+
+ residual = hidden_states
+
+ hidden_states = self.input_layernorm(hidden_states)
+
+ # Self Attention
+ hidden_states, self_attn_weights, present_key_value = self.self_attn(
+ hidden_states=hidden_states,
+ attention_mask=attention_mask,
+ position_ids=position_ids,
+ past_key_value=past_key_value,
+ output_attentions=output_attentions,
+ use_cache=use_cache,
+ )
+ hidden_states = residual + hidden_states
+
+ # Fully Connected
+ residual = hidden_states
+ hidden_states = self.post_attention_layernorm(hidden_states)
+ hidden_states, router_logits = self.block_sparse_moe(hidden_states)
+ hidden_states = residual + hidden_states
+
+ outputs = (hidden_states, )
+
+ if output_attentions:
+ outputs += (self_attn_weights, )
+
+ if use_cache:
+ outputs += (present_key_value, )
+
+ if output_router_logits:
+ outputs += (router_logits, )
+
+ return outputs
+
+
+def _load_pretrained_model(
+ cls,
+ model,
+ state_dict,
+ loaded_keys,
+ resolved_archive_file,
+ pretrained_model_name_or_path,
+ ignore_mismatched_sizes=False,
+ sharded_metadata=None,
+ _fast_init=True,
+ low_cpu_mem_usage=False,
+ device_map=None,
+ offload_folder=None,
+ offload_state_dict=None,
+ dtype=None,
+ hf_quantizer=None,
+ keep_in_fp32_modules=None,
+ gguf_path=None,
+):
+ if ((state_dict is not None) or (resolved_archive_file is None)
+ or (low_cpu_mem_usage) or (device_map is not None)
+ or (offload_folder is not None) or
+ (not (offload_state_dict is None or offload_state_dict is False))
+ or (hf_quantizer is not None) or
+ (keep_in_fp32_modules is not None and len(keep_in_fp32_modules) > 0)
+ or (gguf_path is not None)):
+ raise NotImplementedError
+
+ folder = os.path.sep.join(resolved_archive_file[0].split(os.path.sep)[:-1])
+ error_msgs = load_state_dict_into_model(model, folder)
+ return model, [], [], [], None, error_msgs
+
+
+MIXTRAL_START_DOCSTRING = r"""
+ This model inherits from [`PreTrainedModel`]. Check the superclass documentation for the generic methods the
+ library implements for all its model (such as downloading or saving, resizing the input embeddings, pruning heads
+ etc.)
+
+ This model is also a PyTorch [torch.nn.Module](https://pytorch.org/docs/stable/nn.html#torch.nn.Module) subclass.
+ Use it as a regular PyTorch Module and refer to the PyTorch documentation for all matter related to general usage
+ and behavior.
+
+ Parameters:
+ config ([`MixtralConfig`]):
+ Model configuration class with all the parameters of the model. Initializing with a config file does not
+ load the weights associated with the model, only the configuration. Check out the
+ [`~PreTrainedModel.from_pretrained`] method to load the model weights.
+"""
+
+
+@add_start_docstrings(
+ 'The bare Mixtral Model outputting raw hidden-states without any specific head on top.',
+ MIXTRAL_START_DOCSTRING,
+)
+# Copied from transformers.models.mistral.modeling_mistral.MistralPreTrainedModel with Mistral->Mixtral
+class MixtralPreTrainedModel(PreTrainedModel):
+ config_class = MixtralConfig
+ base_model_prefix = 'model'
+ supports_gradient_checkpointing = True
+ _no_split_modules = ['MixtralDecoderLayer']
+ _skip_keys_device_placement = 'past_key_values'
+ _supports_flash_attn_2 = True
+ _supports_sdpa = True
+ _supports_cache_class = True
+
+ def _init_weights(self, module):
+ std = self.config.initializer_range
+ if isinstance(module, nn.Linear):
+ module.weight.data.normal_(mean=0.0, std=std)
+ if module.bias is not None:
+ module.bias.data.zero_()
+ elif isinstance(module, nn.Embedding):
+ module.weight.data.normal_(mean=0.0, std=std)
+ if module.padding_idx is not None:
+ module.weight.data[module.padding_idx].zero_()
+
+ @classmethod
+ def from_pretrained(cls, pretrained_model_name_or_path, *args, **kwargs):
+ moe_implementation = kwargs.get('moe_implementation', 'origin')
+ if moe_implementation == 'origin':
+ return super().from_pretrained(pretrained_model_name_or_path,
+ *args, **kwargs)
+
+ cls._load_pretrained_model = types.MethodType(_load_pretrained_model,
+ cls)
+ return super().from_pretrained(pretrained_model_name_or_path, *args,
+ **kwargs)
+
+
+MIXTRAL_INPUTS_DOCSTRING = r"""
+ Args:
+ input_ids (`torch.LongTensor` of shape `(batch_size, sequence_length)`):
+ Indices of input sequence tokens in the vocabulary. Padding will be ignored by default should you provide
+ it.
+
+ Indices can be obtained using [`AutoTokenizer`]. See [`PreTrainedTokenizer.encode`] and
+ [`PreTrainedTokenizer.__call__`] for details.
+
+ [What are input IDs?](../glossary#input-ids)
+ attention_mask (`torch.Tensor` of shape `(batch_size, sequence_length)`, *optional*):
+ Mask to avoid performing attention on padding token indices. Mask values selected in `[0, 1]`:
+
+ - 1 for tokens that are **not masked**,
+ - 0 for tokens that are **masked**.
+
+ [What are attention masks?](../glossary#attention-mask)
+
+ Indices can be obtained using [`AutoTokenizer`]. See [`PreTrainedTokenizer.encode`] and
+ [`PreTrainedTokenizer.__call__`] for details.
+
+ If `past_key_values` is used, optionally only the last `decoder_input_ids` have to be input (see
+ `past_key_values`).
+
+ If you want to change padding behavior, you should read [`modeling_opt._prepare_decoder_attention_mask`]
+ and modify to your needs. See diagram 1 in [the paper](https://arxiv.org/abs/1910.13461) for more
+ information on the default strategy.
+
+ - 1 indicates the head is **not masked**,
+ - 0 indicates the head is **masked**.
+ position_ids (`torch.LongTensor` of shape `(batch_size, sequence_length)`, *optional*):
+ Indices of positions of each input sequence tokens in the position embeddings. Selected in the range `[0,
+ config.n_positions - 1]`.
+
+ [What are position IDs?](../glossary#position-ids)
+ past_key_values (`tuple(tuple(torch.FloatTensor))`, *optional*, returned when `use_cache=True` is passed or when `config.use_cache=True`):
+ Tuple of `tuple(torch.FloatTensor)` of length `config.n_layers`, with each tuple having 2 tensors of shape
+ `(batch_size, num_heads, sequence_length, embed_size_per_head)`) and 2 additional tensors of shape
+ `(batch_size, num_heads, encoder_sequence_length, embed_size_per_head)`.
+
+ Contains pre-computed hidden-states (key and values in the self-attention blocks and in the cross-attention
+ blocks) that can be used (see `past_key_values` input) to speed up sequential decoding.
+
+ If `past_key_values` are used, the user can optionally input only the last `decoder_input_ids` (those that
+ don't have their past key value states given to this model) of shape `(batch_size, 1)` instead of all
+ `decoder_input_ids` of shape `(batch_size, sequence_length)`.
+ inputs_embeds (`torch.FloatTensor` of shape `(batch_size, sequence_length, hidden_size)`, *optional*):
+ Optionally, instead of passing `input_ids` you can choose to directly pass an embedded representation. This
+ is useful if you want more control over how to convert `input_ids` indices into associated vectors than the
+ model's internal embedding lookup matrix.
+ use_cache (`bool`, *optional*):
+ If set to `True`, `past_key_values` key value states are returned and can be used to speed up decoding (see
+ `past_key_values`).
+ output_attentions (`bool`, *optional*):
+ Whether or not to return the attentions tensors of all attention layers. See `attentions` under returned
+ tensors for more detail.
+ output_hidden_states (`bool`, *optional*):
+ Whether or not to return the hidden states of all layers. See `hidden_states` under returned tensors for
+ more detail.
+ output_router_logits (`bool`, *optional*):
+ Whether or not to return the logits of all the routers. They are useful for computing the router loss, and
+ should not be returned during inference.
+ return_dict (`bool`, *optional*):
+ Whether or not to return a [`~utils.ModelOutput`] instead of a plain tuple.
+"""
+
+
+@add_start_docstrings(
+ 'The bare Mixtral Model outputting raw hidden-states without any specific head on top.',
+ MIXTRAL_START_DOCSTRING,
+)
+# Copied from transformers.models.mistral.modeling_mistral.MistralModel with MISTRAL->MIXTRAL,Mistral->Mixtral
+class MixtralModel(MixtralPreTrainedModel):
+ """Transformer decoder consisting of *config.num_hidden_layers* layers.
+ Each layer is a [`MixtralDecoderLayer`]
+
+ Args:
+ config: MixtralConfig
+ """
+
+ def __init__(self, config: MixtralConfig):
+ super().__init__(config)
+ self.padding_idx = config.pad_token_id
+ self.vocab_size = config.vocab_size
+
+ self.embed_tokens = nn.Embedding(config.vocab_size, config.hidden_size,
+ self.padding_idx)
+ self.layers = nn.ModuleList([
+ MixtralDecoderLayer(config, layer_idx)
+ for layer_idx in range(config.num_hidden_layers)
+ ])
+ self._attn_implementation = config._attn_implementation
+ self.norm = MixtralRMSNorm(config.hidden_size, eps=config.rms_norm_eps)
+
+ self.gradient_checkpointing = False
+ # Initialize weights and apply final processing
+ self.post_init()
+
+ def get_input_embeddings(self):
+ return self.embed_tokens
+
+ def set_input_embeddings(self, value):
+ self.embed_tokens = value
+
+ # Ignore copy
+ @add_start_docstrings_to_model_forward(MIXTRAL_INPUTS_DOCSTRING)
+ def forward(
+ self,
+ input_ids: torch.LongTensor = None,
+ attention_mask: Optional[torch.Tensor] = None,
+ position_ids: Optional[torch.LongTensor] = None,
+ past_key_values: Optional[List[torch.FloatTensor]] = None,
+ inputs_embeds: Optional[torch.FloatTensor] = None,
+ use_cache: Optional[bool] = None,
+ output_attentions: Optional[bool] = None,
+ output_hidden_states: Optional[bool] = None,
+ output_router_logits: Optional[bool] = None,
+ return_dict: Optional[bool] = None,
+ ) -> Union[Tuple, MoeModelOutputWithPast]:
+ output_attentions = output_attentions if output_attentions is not None else self.config.output_attentions
+ output_router_logits = (
+ output_router_logits if output_router_logits is not None else
+ self.config.output_router_logits)
+ output_hidden_states = (
+ output_hidden_states if output_hidden_states is not None else
+ self.config.output_hidden_states)
+ use_cache = use_cache if use_cache is not None else self.config.use_cache
+
+ return_dict = return_dict if return_dict is not None else self.config.use_return_dict
+
+ # retrieve input_ids and inputs_embeds
+ if input_ids is not None and inputs_embeds is not None:
+ raise ValueError(
+ 'You cannot specify both decoder_input_ids and decoder_inputs_embeds at the same time'
+ )
+ elif input_ids is not None:
+ batch_size, seq_length = input_ids.shape
+ elif inputs_embeds is not None:
+ batch_size, seq_length, _ = inputs_embeds.shape
+ else:
+ raise ValueError(
+ 'You have to specify either decoder_input_ids or decoder_inputs_embeds'
+ )
+
+ past_key_values_length = 0
+
+ if self.gradient_checkpointing and self.training:
+ if use_cache:
+ logger.warning_once(
+ '`use_cache=True` is incompatible with gradient checkpointing. Setting `use_cache=False`...'
+ )
+ use_cache = False
+
+ if use_cache:
+ use_legacy_cache = not isinstance(past_key_values, Cache)
+ if use_legacy_cache:
+ past_key_values = DynamicCache.from_legacy_cache(
+ past_key_values)
+ past_key_values_length = past_key_values.get_usable_length(
+ seq_length)
+
+ if position_ids is None:
+ device = input_ids.device if input_ids is not None else inputs_embeds.device
+ position_ids = torch.arange(
+ past_key_values_length,
+ seq_length + past_key_values_length,
+ dtype=torch.long,
+ device=device)
+ position_ids = position_ids.unsqueeze(0).view(-1, seq_length)
+ else:
+ position_ids = position_ids.view(-1, seq_length).long()
+
+ if inputs_embeds is None:
+ inputs_embeds = self.embed_tokens(input_ids)
+
+ if attention_mask is not None and self._attn_implementation == 'flash_attention_2' and use_cache:
+ is_padding_right = attention_mask[:, -1].sum().item() != batch_size
+ if is_padding_right:
+ raise ValueError(
+ "You are attempting to perform batched generation with padding_side='right'"
+ ' this may lead to unexpected behaviour for Flash Attention version of Mixtral. Make sure to '
+ " call `tokenizer.padding_side = 'left'` before tokenizing the input. "
+ )
+
+ if self._attn_implementation == 'flash_attention_2':
+ # 2d mask is passed through the layers
+ attention_mask = attention_mask if (
+ attention_mask is not None and 0 in attention_mask) else None
+ elif self._attn_implementation == 'sdpa' and not output_attentions:
+ # output_attentions=True can not be supported when using SDPA, and we fall back on
+ # the manual implementation that requires a 4D causal mask in all cases.
+ attention_mask = _prepare_4d_causal_attention_mask_for_sdpa(
+ attention_mask,
+ (batch_size, seq_length),
+ inputs_embeds,
+ past_key_values_length,
+ sliding_window=self.config.sliding_window,
+ )
+ else:
+ # 4d mask is passed through the layers
+ attention_mask = _prepare_4d_causal_attention_mask(
+ attention_mask,
+ (batch_size, seq_length),
+ inputs_embeds,
+ past_key_values_length,
+ sliding_window=self.config.sliding_window,
+ )
+
+ hidden_states = inputs_embeds
+
+ # decoder layers
+ all_hidden_states = () if output_hidden_states else None
+ all_self_attns = () if output_attentions else None
+ all_router_logits = () if output_router_logits else None
+ next_decoder_cache = None
+
+ for decoder_layer in self.layers:
+ if output_hidden_states:
+ all_hidden_states += (hidden_states, )
+
+ if self.gradient_checkpointing and self.training:
+ layer_outputs = self._gradient_checkpointing_func(
+ decoder_layer.__call__,
+ hidden_states,
+ attention_mask,
+ position_ids,
+ past_key_values,
+ output_attentions,
+ output_router_logits,
+ use_cache,
+ )
+ else:
+ layer_outputs = decoder_layer(
+ hidden_states,
+ attention_mask=attention_mask,
+ position_ids=position_ids,
+ past_key_value=past_key_values,
+ output_attentions=output_attentions,
+ output_router_logits=output_router_logits,
+ use_cache=use_cache,
+ )
+
+ hidden_states = layer_outputs[0]
+
+ if use_cache:
+ next_decoder_cache = layer_outputs[
+ 2 if output_attentions else 1]
+
+ if output_attentions:
+ all_self_attns += (layer_outputs[1], )
+
+ if output_router_logits:
+ all_router_logits += (layer_outputs[-1], )
+
+ hidden_states = self.norm(hidden_states)
+
+ # add hidden states from the last decoder layer
+ if output_hidden_states:
+ all_hidden_states += (hidden_states, )
+
+ next_cache = None
+ if use_cache:
+ next_cache = next_decoder_cache.to_legacy_cache(
+ ) if use_legacy_cache else next_decoder_cache
+
+ if not return_dict:
+ return tuple(v for v in [
+ hidden_states, next_cache, all_hidden_states, all_self_attns,
+ all_router_logits
+ ] if v is not None)
+ return MoeModelOutputWithPast(
+ last_hidden_state=hidden_states,
+ past_key_values=next_cache,
+ hidden_states=all_hidden_states,
+ attentions=all_self_attns,
+ router_logits=all_router_logits,
+ )
+
+
+class MixtralForCausalLM(MixtralPreTrainedModel):
+ _tied_weights_keys = ['lm_head.weight']
+
+ def __init__(self, config):
+ super().__init__(config)
+ self.model = MixtralModel(config)
+ self.vocab_size = config.vocab_size
+ self.lm_head = nn.Linear(
+ config.hidden_size, config.vocab_size, bias=False)
+ self.router_aux_loss_coef = config.router_aux_loss_coef
+ self.num_experts = config.num_local_experts
+ self.num_experts_per_tok = config.num_experts_per_tok
+ # Initialize weights and apply final processing
+ self.post_init()
+
+ def get_input_embeddings(self):
+ return self.model.embed_tokens
+
+ def set_input_embeddings(self, value):
+ self.model.embed_tokens = value
+
+ def get_output_embeddings(self):
+ return self.lm_head
+
+ def set_output_embeddings(self, new_embeddings):
+ self.lm_head = new_embeddings
+
+ def set_decoder(self, decoder):
+ self.model = decoder
+
+ def get_decoder(self):
+ return self.model
+
+ @add_start_docstrings_to_model_forward(MIXTRAL_INPUTS_DOCSTRING)
+ @replace_return_docstrings(
+ output_type=MoeCausalLMOutputWithPast, config_class=_CONFIG_FOR_DOC)
+ # Ignore copy
+ def forward(
+ self,
+ input_ids: torch.LongTensor = None,
+ attention_mask: Optional[torch.Tensor] = None,
+ position_ids: Optional[torch.LongTensor] = None,
+ past_key_values: Optional[List[torch.FloatTensor]] = None,
+ inputs_embeds: Optional[torch.FloatTensor] = None,
+ labels: Optional[torch.LongTensor] = None,
+ use_cache: Optional[bool] = None,
+ output_attentions: Optional[bool] = None,
+ output_hidden_states: Optional[bool] = None,
+ output_router_logits: Optional[bool] = None,
+ return_dict: Optional[bool] = None,
+ ) -> Union[Tuple, MoeCausalLMOutputWithPast]:
+ r"""
+ Args:
+ labels (`torch.LongTensor` of shape `(batch_size, sequence_length)`, *optional*):
+ Labels for computing the masked language modeling loss. Indices should either be in `[0, ...,
+ config.vocab_size]` or -100 (see `input_ids` docstring). Tokens with indices set to `-100` are ignored
+ (masked), the loss is only computed for the tokens with labels in `[0, ..., config.vocab_size]`.
+
+ Returns:
+
+ Example:
+
+ ```python
+ >>> from transformers import AutoTokenizer, MixtralForCausalLM
+
+ >>> model = MixtralForCausalLM.from_pretrained("mistralai/Mixtral-8x7B-v0.1")
+ >>> tokenizer = AutoTokenizer.from_pretrained("mistralai/Mixtral-8x7B-v0.1")
+
+ >>> prompt = "Hey, are you conscious? Can you talk to me?"
+ >>> inputs = tokenizer(prompt, return_tensors="pt")
+
+ >>> # Generate
+ >>> generate_ids = model.generate(inputs.input_ids, max_length=30)
+ >>> tokenizer.batch_decode(generate_ids, skip_special_tokens=True, clean_up_tokenization_spaces=False)[0]
+ "Hey, are you conscious? Can you talk to me?\nI'm not conscious, but I can talk to you."
+ ```"""
+
+ output_attentions = output_attentions if output_attentions is not None else self.config.output_attentions
+ output_router_logits = (
+ output_router_logits if output_router_logits is not None else
+ self.config.output_router_logits)
+
+ output_hidden_states = (
+ output_hidden_states if output_hidden_states is not None else
+ self.config.output_hidden_states)
+ return_dict = return_dict if return_dict is not None else self.config.use_return_dict
+
+ # decoder outputs consists of (dec_features, layer_state, dec_hidden, dec_attn)
+ outputs = self.model(
+ input_ids=input_ids,
+ attention_mask=attention_mask,
+ position_ids=position_ids,
+ past_key_values=past_key_values,
+ inputs_embeds=inputs_embeds,
+ use_cache=use_cache,
+ output_attentions=output_attentions,
+ output_hidden_states=output_hidden_states,
+ output_router_logits=output_router_logits,
+ return_dict=return_dict,
+ )
+
+ hidden_states = outputs[0]
+ logits = self.lm_head(hidden_states)
+ logits = logits.float()
+
+ loss = None
+ if labels is not None:
+ # Shift so that tokens < n predict n
+ shift_logits = logits[..., :-1, :].contiguous()
+ shift_labels = labels[..., 1:].contiguous()
+ # Flatten the tokens
+ loss_fct = CrossEntropyLoss()
+ shift_logits = shift_logits.view(-1, self.config.vocab_size)
+ shift_labels = shift_labels.view(-1)
+ # Enable model parallelism
+ shift_labels = shift_labels.to(shift_logits.device)
+ loss = loss_fct(shift_logits, shift_labels)
+
+ aux_loss = None
+ if output_router_logits:
+ aux_loss = load_balancing_loss_func(
+ outputs.router_logits if return_dict else outputs[-1],
+ self.num_experts,
+ self.num_experts_per_tok,
+ attention_mask,
+ )
+ if labels is not None:
+ loss += self.router_aux_loss_coef * aux_loss.to(
+ loss.device) # make sure to reside in the same device
+
+ if not return_dict:
+ output = (logits, ) + outputs[1:]
+ if output_router_logits:
+ output = (aux_loss, ) + output
+ return (loss, ) + output if loss is not None else output
+
+ return MoeCausalLMOutputWithPast(
+ loss=loss,
+ aux_loss=aux_loss,
+ logits=logits,
+ past_key_values=outputs.past_key_values,
+ hidden_states=outputs.hidden_states,
+ attentions=outputs.attentions,
+ router_logits=outputs.router_logits,
+ )
+
+ def prepare_inputs_for_generation(
+ self,
+ input_ids,
+ past_key_values=None,
+ attention_mask=None,
+ inputs_embeds=None,
+ output_router_logits=False,
+ **kwargs,
+ ):
+ # Omit tokens covered by past_key_values
+ if past_key_values is not None:
+ if isinstance(past_key_values, Cache):
+ cache_length = past_key_values.get_seq_length()
+ past_length = past_key_values.seen_tokens
+ max_cache_length = past_key_values.get_max_length()
+ else:
+ cache_length = past_length = past_key_values[0][0].shape[2]
+ max_cache_length = None
+
+ # Keep only the unprocessed tokens:
+ # 1 - If the length of the attention_mask exceeds the length of input_ids, then we are in a setting where
+ # some of the inputs are exclusively passed as part of the cache (e.g. when passing input_embeds as
+ # input)
+ if attention_mask is not None and attention_mask.shape[
+ 1] > input_ids.shape[1]:
+ input_ids = input_ids[:, -(attention_mask.shape[1] -
+ past_length):]
+ # 2 - If the past_length is smaller than input_ids', then input_ids holds all input tokens. We can discard
+ # input_ids based on the past_length.
+ elif past_length < input_ids.shape[1]:
+ input_ids = input_ids[:, past_length:]
+ # 3 - Otherwise (past_length >= input_ids.shape[1]), let's assume input_ids only has unprocessed tokens.
+
+ # If we are about to go beyond the maximum cache length, we need to crop the input attention mask.
+ if (max_cache_length is not None and attention_mask is not None
+ and cache_length + input_ids.shape[1] > max_cache_length):
+ attention_mask = attention_mask[:, -max_cache_length:]
+
+ position_ids = kwargs.get('position_ids', None)
+ if attention_mask is not None and position_ids is None:
+ # create position_ids on the fly for batch generation
+ position_ids = attention_mask.long().cumsum(-1) - 1
+ position_ids.masked_fill_(attention_mask == 0, 1)
+ if past_key_values:
+ position_ids = position_ids[:, -input_ids.shape[1]:]
+
+ # if `inputs_embeds` are passed, we only want to use them in the 1st generation step
+ if inputs_embeds is not None and past_key_values is None:
+ model_inputs = {'inputs_embeds': inputs_embeds}
+ else:
+ model_inputs = {'input_ids': input_ids}
+
+ model_inputs.update({
+ 'position_ids': position_ids,
+ 'past_key_values': past_key_values,
+ 'use_cache': kwargs.get('use_cache'),
+ 'attention_mask': attention_mask,
+ 'output_router_logits': output_router_logits,
+ })
+ return model_inputs
+
+ @staticmethod
+ def _reorder_cache(past_key_values, beam_idx):
+ reordered_past = ()
+ for layer_past in past_key_values:
+ reordered_past += (tuple(
+ past_state.index_select(0, beam_idx.to(past_state.device))
+ for past_state in layer_past), )
+ return reordered_past
+
+
+@add_start_docstrings(
+ """
+ The Mixtral Model transformer with a sequence classification head on top (linear layer).
+
+ [`MixtralForSequenceClassification`] uses the last token in order to do the classification, as other causal models
+ (e.g. GPT-2) do.
+
+ Since it does classification on the last token, it requires to know the position of the last token. If a
+ `pad_token_id` is defined in the configuration, it finds the last token that is not a padding token in each row. If
+ no `pad_token_id` is defined, it simply takes the last value in each row of the batch. Since it cannot guess the
+ padding tokens when `inputs_embeds` are passed instead of `input_ids`, it does the same (take the last value in
+ each row of the batch).
+ """,
+ MIXTRAL_START_DOCSTRING,
+)
+# Copied from transformers.models.llama.modeling_llama.LlamaForSequenceClassification with Llama->Mixtral, LLAMA->MIXTRAL
+class MixtralForSequenceClassification(MixtralPreTrainedModel):
+
+ def __init__(self, config):
+ super().__init__(config)
+ self.num_labels = config.num_labels
+ self.model = MixtralModel(config)
+ self.score = nn.Linear(config.hidden_size, self.num_labels, bias=False)
+
+ # Initialize weights and apply final processing
+ self.post_init()
+
+ def get_input_embeddings(self):
+ return self.model.embed_tokens
+
+ def set_input_embeddings(self, value):
+ self.model.embed_tokens = value
+
+ @add_start_docstrings_to_model_forward(MIXTRAL_INPUTS_DOCSTRING)
+ def forward(
+ self,
+ input_ids: torch.LongTensor = None,
+ attention_mask: Optional[torch.Tensor] = None,
+ position_ids: Optional[torch.LongTensor] = None,
+ past_key_values: Optional[Union[Cache,
+ List[torch.FloatTensor]]] = None,
+ inputs_embeds: Optional[torch.FloatTensor] = None,
+ labels: Optional[torch.LongTensor] = None,
+ use_cache: Optional[bool] = None,
+ output_attentions: Optional[bool] = None,
+ output_hidden_states: Optional[bool] = None,
+ return_dict: Optional[bool] = None,
+ ) -> Union[Tuple, SequenceClassifierOutputWithPast]:
+ r"""
+ labels (`torch.LongTensor` of shape `(batch_size,)`, *optional*):
+ Labels for computing the sequence classification/regression loss. Indices should be in `[0, ...,
+ config.num_labels - 1]`. If `config.num_labels == 1` a regression loss is computed (Mean-Square loss), If
+ `config.num_labels > 1` a classification loss is computed (Cross-Entropy).
+ """
+ return_dict = return_dict if return_dict is not None else self.config.use_return_dict
+
+ transformer_outputs = self.model(
+ input_ids,
+ attention_mask=attention_mask,
+ position_ids=position_ids,
+ past_key_values=past_key_values,
+ inputs_embeds=inputs_embeds,
+ use_cache=use_cache,
+ output_attentions=output_attentions,
+ output_hidden_states=output_hidden_states,
+ return_dict=return_dict,
+ )
+ hidden_states = transformer_outputs[0]
+ logits = self.score(hidden_states)
+
+ if input_ids is not None:
+ batch_size = input_ids.shape[0]
+ else:
+ batch_size = inputs_embeds.shape[0]
+
+ if self.config.pad_token_id is None and batch_size != 1:
+ raise ValueError(
+ 'Cannot handle batch sizes > 1 if no padding token is defined.'
+ )
+ if self.config.pad_token_id is None:
+ sequence_lengths = -1
+ else:
+ if input_ids is not None:
+ # if no pad token found, use modulo instead of reverse indexing for ONNX compatibility
+ sequence_lengths = torch.eq(
+ input_ids, self.config.pad_token_id).int().argmax(-1) - 1
+ sequence_lengths = sequence_lengths % input_ids.shape[-1]
+ sequence_lengths = sequence_lengths.to(logits.device)
+ else:
+ sequence_lengths = -1
+
+ pooled_logits = logits[torch.arange(batch_size, device=logits.device),
+ sequence_lengths]
+
+ loss = None
+ if labels is not None:
+ labels = labels.to(logits.device)
+ if self.config.problem_type is None:
+ if self.num_labels == 1:
+ self.config.problem_type = 'regression'
+ elif self.num_labels > 1 and (labels.dtype == torch.long
+ or labels.dtype == torch.int):
+ self.config.problem_type = 'single_label_classification'
+ else:
+ self.config.problem_type = 'multi_label_classification'
+
+ if self.config.problem_type == 'regression':
+ loss_fct = MSELoss()
+ if self.num_labels == 1:
+ loss = loss_fct(pooled_logits.squeeze(), labels.squeeze())
+ else:
+ loss = loss_fct(pooled_logits, labels)
+ elif self.config.problem_type == 'single_label_classification':
+ loss_fct = CrossEntropyLoss()
+ loss = loss_fct(
+ pooled_logits.view(-1, self.num_labels), labels.view(-1))
+ elif self.config.problem_type == 'multi_label_classification':
+ loss_fct = BCEWithLogitsLoss()
+ loss = loss_fct(pooled_logits, labels)
+ if not return_dict:
+ output = (pooled_logits, ) + transformer_outputs[1:]
+ return ((loss, ) + output) if loss is not None else output
+
+ return SequenceClassifierOutputWithPast(
+ loss=loss,
+ logits=pooled_logits,
+ past_key_values=transformer_outputs.past_key_values,
+ hidden_states=transformer_outputs.hidden_states,
+ attentions=transformer_outputs.attentions,
+ )
diff --git a/xtuner/model/utils.py b/xtuner/model/utils.py
index dce86315d..a8bbf2944 100644
--- a/xtuner/model/utils.py
+++ b/xtuner/model/utils.py
@@ -3,7 +3,6 @@
from typing import List, Optional
import torch
-from mmengine import print_log
from mmengine.utils.misc import get_object_from_string
from peft import PeftType
from torch import nn
@@ -18,6 +17,19 @@ def set_obj_dtype(d):
d[key] = getattr(torch, value.split('.')[-1])
+def try_build_module(cfg):
+ builder = cfg['type']
+ if isinstance(builder, str):
+ builder = get_object_from_string(builder)
+ if builder is None:
+ # support handling cfg with key 'type' can not be built, such as
+ # {'rope_scaling': {'type': 'linear', 'factor': 2.0}}
+ return cfg
+ cfg.pop('type')
+ module_built = builder(**cfg)
+ return module_built
+
+
def traverse_dict(d):
if isinstance(d, dict):
set_obj_dtype(d)
@@ -25,12 +37,8 @@ def traverse_dict(d):
if isinstance(value, dict):
traverse_dict(value)
if 'type' in value:
- builder = value.pop('type')
- if isinstance(builder, str):
- builder = get_object_from_string(builder)
- new_value = builder(**value)
- d[key] = new_value
- print_log(f'{key} convert to {builder}')
+ module_built = try_build_module(value)
+ d[key] = module_built
elif isinstance(d, list):
for element in d:
traverse_dict(element)
@@ -293,8 +301,8 @@ def guess_load_checkpoint(pth_model):
state_dict = state_dict['state_dict']
elif osp.isdir(pth_model):
try:
- from deepspeed.utils.zero_to_fp32 import \
- get_fp32_state_dict_from_zero_checkpoint
+ from xtuner.utils.zero_to_any_dtype import \
+ get_state_dict_from_zero_checkpoint
except ImportError:
raise ImportError(
'The provided PTH model appears to be a DeepSpeed checkpoint. '
@@ -302,7 +310,7 @@ def guess_load_checkpoint(pth_model):
'environment. This suggests that DeepSpeed may not be '
'installed or is incorrectly configured. Please verify your '
'setup.')
- state_dict = get_fp32_state_dict_from_zero_checkpoint(
+ state_dict = get_state_dict_from_zero_checkpoint(
osp.dirname(pth_model), osp.basename(pth_model))
else:
raise FileNotFoundError(f'Cannot find {pth_model}')
diff --git a/xtuner/parallel/sequence/__init__.py b/xtuner/parallel/sequence/__init__.py
index a50921336..6e2992f78 100644
--- a/xtuner/parallel/sequence/__init__.py
+++ b/xtuner/parallel/sequence/__init__.py
@@ -1,24 +1,41 @@
# Copyright (c) OpenMMLab. All rights reserved.
from mmengine.dist import init_dist
-from .attention import sequence_parallel_wrapper
-from .data_collate import (pad_for_sequence_parallel,
- split_for_sequence_parallel)
+from .attention import (post_process_for_sequence_parallel_attn,
+ pre_process_for_sequence_parallel_attn,
+ sequence_parallel_wrapper)
+from .comm import (all_to_all, gather_for_sequence_parallel,
+ gather_forward_split_backward, split_for_sequence_parallel,
+ split_forward_gather_backward)
+from .data_collate import (pad_cumulative_len_for_sequence_parallel,
+ pad_for_sequence_parallel)
from .reduce_loss import reduce_sequence_parallel_loss
from .sampler import SequenceParallelSampler
from .setup_distributed import (get_data_parallel_group,
get_data_parallel_rank,
get_data_parallel_world_size,
+ get_inner_sequence_parallel_group,
+ get_inner_sequence_parallel_rank,
+ get_inner_sequence_parallel_world_size,
get_sequence_parallel_group,
get_sequence_parallel_rank,
get_sequence_parallel_world_size,
- init_sequence_parallel)
+ init_inner_sequence_parallel,
+ init_sequence_parallel,
+ is_inner_sequence_parallel_initialized)
__all__ = [
- 'sequence_parallel_wrapper', 'pad_for_sequence_parallel',
+ 'sequence_parallel_wrapper', 'pre_process_for_sequence_parallel_attn',
+ 'post_process_for_sequence_parallel_attn', 'pad_for_sequence_parallel',
'split_for_sequence_parallel', 'SequenceParallelSampler',
'init_sequence_parallel', 'get_sequence_parallel_group',
'get_sequence_parallel_world_size', 'get_sequence_parallel_rank',
'get_data_parallel_group', 'get_data_parallel_world_size',
- 'get_data_parallel_rank', 'reduce_sequence_parallel_loss', 'init_dist'
+ 'get_data_parallel_rank', 'reduce_sequence_parallel_loss', 'init_dist',
+ 'all_to_all', 'gather_for_sequence_parallel',
+ 'split_forward_gather_backward', 'gather_forward_split_backward',
+ 'get_inner_sequence_parallel_group', 'get_inner_sequence_parallel_rank',
+ 'get_inner_sequence_parallel_world_size', 'init_inner_sequence_parallel',
+ 'is_inner_sequence_parallel_initialized',
+ 'pad_cumulative_len_for_sequence_parallel'
]
diff --git a/xtuner/parallel/sequence/attention.py b/xtuner/parallel/sequence/attention.py
index b1b1ebcee..e8bb1adac 100644
--- a/xtuner/parallel/sequence/attention.py
+++ b/xtuner/parallel/sequence/attention.py
@@ -1,80 +1,129 @@
# Copyright (c) OpenMMLab. All rights reserved.
-from typing import Any, Tuple
+import math
-import torch
import torch.distributed as dist
-from torch import Tensor
-
-from .setup_distributed import (get_sequence_parallel_group,
- get_sequence_parallel_world_size)
-
-
-def all_to_all_scatter_nhead(input):
- # bs, seq, nhead, dim ==>
- # bs, seq * sp_world_size, nhead / sp_world_size, dim
- sp_world_size = get_sequence_parallel_world_size()
- sp_group = get_sequence_parallel_group()
- bs, seq, nhead, dim = input.shape
- input_t = input.reshape(bs, seq, sp_world_size, nhead // sp_world_size,
- dim)
- input_t = input_t.permute(2, 0, 1, 3, 4).contiguous()
- output = torch.empty_like(input_t)
- dist.all_to_all_single(output, input_t, group=sp_group)
- output = output.transpose(0, 1)
- return output.reshape(bs, seq * sp_world_size, nhead // sp_world_size, dim)
-
-
-def all_to_all_scatter_seq(input):
- # bs, seq * sp_world_size, nhead / sp_world_size, dim ==>
- # bs, seq, nhead, dim
- sp_world_size = get_sequence_parallel_world_size()
- sp_group = get_sequence_parallel_group()
- bs, seq, nhead, dim = input.shape
- input_t = input.reshape(bs, sp_world_size, seq // sp_world_size, nhead,
- dim)
- input_t = input_t.transpose(0, 1).contiguous()
- output = torch.empty_like(input_t)
- dist.all_to_all_single(output, input_t, group=sp_group)
- output = output.permute(1, 2, 0, 3, 4)
- return output.reshape(bs, seq // sp_world_size, nhead * sp_world_size, dim)
-
-
-class _SeqAllToAll(torch.autograd.Function):
-
- @staticmethod
- def forward(ctx: Any, input: Tensor, scatter_seq) -> Tensor:
- ctx.scatter_seq = scatter_seq
- ctx.input_shape = input.shape
- if scatter_seq:
- return all_to_all_scatter_seq(input)
- return all_to_all_scatter_nhead(input)
-
- @staticmethod
- def backward(ctx: Any, *grad_output: Tensor) -> Tuple[Tensor, None]:
- grad = _SeqAllToAll.apply(*grad_output, not ctx.scatter_seq)
- return (grad, None)
-
-
-def pre_process_for_sequence_parallel_attn(query_states, key_states,
- value_states):
- sequence_parallel_world_size = get_sequence_parallel_world_size()
- n_head = query_states.shape[2]
- assert n_head % sequence_parallel_world_size == 0, \
- ('The number of attention heads should be divisible by '
- f'sequence_parallel_world_size. But got n_head = {n_head} and '
- f'sequence_parallel_world_size = {sequence_parallel_world_size}.')
- # (b, s // sp_world_size, nd, dim) -> (b, s, nd // sp_world_size, dim)
- query_states = _SeqAllToAll.apply(query_states, False)
- key_states = _SeqAllToAll.apply(key_states, False)
- value_states = _SeqAllToAll.apply(value_states, False)
+from .comm import (all_to_all, gather_forward_split_backward,
+ split_forward_gather_backward)
+from .setup_distributed import (get_inner_sequence_parallel_group,
+ get_inner_sequence_parallel_world_size,
+ get_sequence_parallel_group,
+ get_sequence_parallel_world_size,
+ init_inner_sequence_parallel,
+ is_inner_sequence_parallel_initialized)
+
+
+def pre_process_for_sequence_parallel_attn(query_states,
+ key_states,
+ value_states,
+ scatter_dim=2,
+ gather_dim=1):
+ b, s_div_sp, h, d = query_states.shape
+ sp = get_sequence_parallel_world_size()
+
+ if not is_inner_sequence_parallel_initialized():
+ insp = sp // math.gcd(h, sp)
+ init_inner_sequence_parallel(insp)
+ else:
+ insp = get_inner_sequence_parallel_world_size()
+
+ def pre_process_for_inner_sp(q, k, v):
+ if scatter_dim != 2 and gather_dim != 1:
+ raise NotImplementedError(
+ 'Currently only `scatter_dim == 2` and `gather_dim == 1` '
+ f'is supported. But got scatter_dim = {scatter_dim} and '
+ f'gather_dim = {gather_dim}.')
+
+ # (b, s_div_sp, h, d) ->
+ # (b, s_div_sp, sp/insp, h*insp/sp, insp, d/insp) ->
+ # (b, s_div_sp, sp/insp, insp, h*insp/sp, d/insp) ->
+ # (b, s_div_sp, insp*h, d/insp)
+ q = q.view(b, s_div_sp, sp // insp, h * insp // sp, insp,
+ d // insp).transpose(3, 4).flatten(2, 4)
+ k = k.view(b, s_div_sp, sp // insp, h * insp // sp, insp,
+ d // insp).transpose(3, 4).flatten(2, 4)
+ v = v.view(b, s_div_sp, sp // insp, h * insp // sp, insp,
+ d // insp).transpose(3, 4).flatten(2, 4)
+
+ return q, k, v
+
+ def post_process_for_inner_sp(q, k, v):
+ # (b, s, insp*h/sp, d/insp) -> (b, s, insp*h/sp, d)
+ q = gather_forward_split_backward(q, -1,
+ get_inner_sequence_parallel_group())
+ k = gather_forward_split_backward(k, -1,
+ get_inner_sequence_parallel_group())
+ v = gather_forward_split_backward(v, -1,
+ get_inner_sequence_parallel_group())
+
+ return q, k, v
+
+ assert (h * insp) % sp == 0, \
+ ('The number of attention heads should be divisible by '
+ '(sequence_parallel_world_size // sequence_parallel_inner_world_size)'
+ f'. But got n_head = {h}, sequence_parallel_world_size = '
+ f'{sp} and sequence_parallel_inner_world_size = {insp}.')
+
+ if insp > 1:
+ query_states, key_states, value_states = pre_process_for_inner_sp(
+ query_states, key_states, value_states)
+
+ # (b, s_div_sp, insp*h, d/insp) -> (b, s, insp*h/sp, d/insp)
+ sequence_parallel_group = get_sequence_parallel_group()
+ query_states = all_to_all(
+ query_states,
+ sequence_parallel_group,
+ scatter_dim=scatter_dim,
+ gather_dim=gather_dim)
+ key_states = all_to_all(
+ key_states,
+ sequence_parallel_group,
+ scatter_dim=scatter_dim,
+ gather_dim=gather_dim)
+ value_states = all_to_all(
+ value_states,
+ sequence_parallel_group,
+ scatter_dim=scatter_dim,
+ gather_dim=gather_dim)
+
+ if insp > 1:
+ query_states, key_states, value_states = post_process_for_inner_sp(
+ query_states, key_states, value_states)
return query_states, key_states, value_states
-def post_process_for_sequence_parallel_attn(attn_output):
- # (b, s, nd // sp_world_size, dim) -> (b, s // sp_world_size, nd, dim)
- output = _SeqAllToAll.apply(attn_output, True)
+def post_process_for_sequence_parallel_attn(attn_output,
+ scatter_dim=1,
+ gather_dim=2):
+ sp = get_sequence_parallel_world_size()
+ insp = get_inner_sequence_parallel_world_size()
+ b, s, h_mul_insp_div_sp, d = attn_output.shape
+ h = h_mul_insp_div_sp * sp // insp
+ s_div_sp = s // sp
+
+ if insp > 1:
+ # (b, s, insp*h/sp, d) -> (b, s, insp*h/sp, d/insp)
+ attn_output = split_forward_gather_backward(
+ attn_output, -1, get_inner_sequence_parallel_group())
+
+ # (b, s, insp*h/sp, d/insp) -> (b, s_div_sp, insp*h, d/insp)
+ sequence_parallel_group = get_sequence_parallel_group()
+ output = all_to_all(
+ attn_output,
+ sequence_parallel_group,
+ scatter_dim=scatter_dim,
+ gather_dim=gather_dim)
+
+ if insp > 1:
+ # (b, s_div_sp, insp*h, d/insp) ->
+ # (b, s_div_sp, sp/insp, insp, h*insp/sp, d/insp) ->
+ # (b, s_div_sp, sp/insp, h*insp/sp, insp, d/insp) ->
+ # (b, s_div_sp, h, d)
+ output = output.view(b, s_div_sp, sp // insp, insp, h * insp // sp,
+ d // insp).transpose(3, 4).reshape(
+ b, s_div_sp, h, d)
+
return output
diff --git a/xtuner/parallel/sequence/comm.py b/xtuner/parallel/sequence/comm.py
new file mode 100644
index 000000000..22396ce11
--- /dev/null
+++ b/xtuner/parallel/sequence/comm.py
@@ -0,0 +1,269 @@
+# Copyright (c) OpenMMLab. All rights reserved.
+from typing import Any, Tuple
+
+import torch
+import torch.distributed as dist
+from torch import Tensor
+
+
+def _all_to_all(
+ input: Tensor,
+ world_size: int,
+ group: dist.ProcessGroup,
+ scatter_dim: int,
+ gather_dim: int,
+):
+ input_list = [
+ t.contiguous()
+ for t in torch.tensor_split(input, world_size, scatter_dim)
+ ]
+ output_list = [torch.empty_like(input_list[0]) for _ in range(world_size)]
+ dist.all_to_all(output_list, input_list, group=group)
+ return torch.cat(output_list, dim=gather_dim).contiguous()
+
+
+class _AllToAll(torch.autograd.Function):
+ """All-to-all communication.
+
+ Args:
+ input: Input tensor
+ sp_group: Sequence parallel process group
+ scatter_dim: Scatter dimension
+ gather_dim: Gather dimension
+ """
+
+ @staticmethod
+ def forward(ctx: Any, input: Tensor, sp_group: dist.ProcessGroup,
+ scatter_dim: int, gather_dim: int):
+ ctx.sp_group = sp_group
+ ctx.scatter_dim = scatter_dim
+ ctx.gather_dim = gather_dim
+ ctx.world_size = dist.get_world_size(sp_group)
+ output = _all_to_all(input, ctx.world_size, sp_group, scatter_dim,
+ gather_dim)
+ return output
+
+ @staticmethod
+ def backward(ctx: Any, grad_output: Tensor) -> Tuple:
+ grad_output = _all_to_all(
+ grad_output,
+ ctx.world_size,
+ ctx.sp_group,
+ ctx.gather_dim,
+ ctx.scatter_dim,
+ )
+ return (
+ grad_output,
+ None,
+ None,
+ None,
+ )
+
+
+def all_to_all(
+ input: Tensor,
+ sp_group: dist.ProcessGroup,
+ scatter_dim: int = 2,
+ gather_dim: int = 1,
+):
+ """Convenience function to apply the all-to-all operation with scatter and
+ gather dimensions.
+
+ Notes:
+ We have wrapped the `torch.distributed.all_to_all` function to
+ enable automatic differentiation of the all-to-all operation.
+
+ Args:
+ input: The input tensor for which all-to-all communication is performed
+ sp_group: The sequence parallel process group.
+ scatter_dim: The dimension along which the input tensor is scattered
+ (default: 2).
+ gather_dim: The dimension along which the output tensor is gathered
+ (default: 1).
+
+ Returns:
+ The output tensor after the all-to-all communication.
+ """
+ return _AllToAll.apply(input, sp_group, scatter_dim, gather_dim)
+
+
+def split_for_sequence_parallel(input, dim: int, sp_group: dist.ProcessGroup):
+ """Splits the input tensor along a given dimension for sequence parallel.
+
+ Args:
+ input: The input tensor to be split.
+ dim: The dimension along which the tensor should be split.
+ sp_group: The sequence parallel process group.
+
+ Returns:
+ The split tensor corresponding to the current rank's chunk.
+ """
+ world_size = dist.get_world_size(sp_group)
+ if world_size == 1:
+ return input
+
+ rank = dist.get_rank(sp_group)
+ dim_size = input.size(dim)
+ assert dim_size % world_size == 0, (
+ f'The dimension to split ({dim_size}) is not a multiple of '
+ f'world size ({world_size}), cannot split tensor evenly')
+
+ tensor_list = torch.split(input, dim_size // world_size, dim=dim)
+ output = tensor_list[rank].contiguous()
+
+ return output
+
+
+def gather_for_sequence_parallel(input, dim: int, sp_group: dist.ProcessGroup):
+ """Gathers the input tensor along a given dimension for sequence parallel.
+
+ Args:
+ input: The input tensor to be gathered.
+ dim: The dimension along which the tensor should be gathered.
+ sp_group: The sequence parallel process group.
+
+ Returns:
+ The gathered tensor concatenated along the specified dimension.
+ """
+ input = input.contiguous()
+ world_size = dist.get_world_size(sp_group)
+ dist.get_rank(sp_group)
+
+ if world_size == 1:
+ return input
+
+ tensor_list = [torch.empty_like(input) for _ in range(world_size)]
+ assert input.device.type != 'cpu'
+ dist.all_gather(tensor_list, input, group=sp_group)
+
+ output = torch.cat(tensor_list, dim=dim).contiguous()
+
+ return output
+
+
+class _GatherForwardSplitBackward(torch.autograd.Function):
+ """Gather the input during forward.
+
+ Scale and split the grad and keep only the corresponding chuck to the rank
+ during backward.
+ """
+
+ @staticmethod
+ def forward(ctx, input, dim, sp_group, grad_scale):
+ ctx.dim = dim
+ ctx.sp_group = sp_group
+ ctx.grad_scale = grad_scale
+ return gather_for_sequence_parallel(input, dim, sp_group)
+
+ @staticmethod
+ def backward(ctx, grad_output):
+ if ctx.grad_scale == 'up':
+ grad_output = grad_output * dist.get_world_size(ctx.sp_group)
+ elif ctx.grad_scale == 'down':
+ grad_output = grad_output / dist.get_world_size(ctx.sp_group)
+
+ return (split_for_sequence_parallel(grad_output, ctx.dim,
+ ctx.sp_group), None, None, None)
+
+
+class _SplitForwardGatherBackward(torch.autograd.Function):
+ """Split the input and keep only the corresponding chuck to the rank during
+ forward.
+
+ Scale and gather the grad during backward.
+ """
+
+ @staticmethod
+ def forward(ctx, input, dim, sp_group, grad_scale):
+ ctx.dim = dim
+ ctx.sp_group = sp_group
+ ctx.grad_scale = grad_scale
+ return split_for_sequence_parallel(input, dim, sp_group)
+
+ @staticmethod
+ def backward(ctx, grad_output):
+ if ctx.grad_scale == 'up':
+ grad_output = grad_output * dist.get_world_size(ctx.sp_group)
+ elif ctx.grad_scale == 'down':
+ grad_output = grad_output / dist.get_world_size(ctx.sp_group)
+ return (gather_for_sequence_parallel(grad_output, ctx.dim,
+ ctx.sp_group), None, None, None)
+
+
+def split_forward_gather_backward(input, dim, sp_group, grad_scale=None):
+ """Split tensors according to the sp rank during forward propagation and
+ gather the grad from the whole sp group during backward propagation.
+
+ 1. When do we need this? input.requires_grad = True
+
+ 2. Why we need grad scale?
+
+ We have to scale down the grads as `gather_forward_split_backward` scales
+ up the grads.
+ """
+ return _SplitForwardGatherBackward.apply(input, dim, sp_group, grad_scale)
+
+
+def gather_forward_split_backward(input, dim, sp_group, grad_scale=None):
+ """Gather tensors from the whole sp group during forward propagation and
+ split the grad according to the sp rank during backward propagation.
+
+ 1. When do we need this?
+
+ When sp is greater than 1, we need to slice the input `x` along
+ sequence length dimension before it is passed into the model and get
+ `sub_seq_x`. We then pass `sub_seq_x` into model and get output
+ `sub_seq_out`. If the loss calculation process needs to use the complete
+ output, we have to gather the `sub_seq_out` in all sp ranks during forward
+ propagation and split the grad during backward propagation.
+
+ 2. Why we need grad scale?
+ Here is a simple case.
+
+ -------- SP 1 -----------
+ Suppose here is a toy model with only one linear module
+ (in_features = 2, out_features = 1) and the input x has shape(2, 2).
+ Y = [[y1], = [[w11x11 + w21x12], = [[x11, x12], dot [[w11],
+ [y2]] [w11x21 + w21x22]] [x21, x22]] [w21]]
+ z = mean(Y) = (y1 + y2) / 2
+ Here is the partial derivative of z with respect to w11:
+ ∂z / ∂w11 = ∂z / ∂y1 * ∂y1 / ∂w11 + ∂z / ∂y2 * ∂y2 / ∂w11
+ = 1/2 * x11 + 1/2 * x21 = (x11 + x21) / 2
+
+ -------- SP 2 -----------
+ When sequence parallel world size is set to 2, we will split the input x
+ and scatter them to the two rank in the same sequence parallel group.
+ ```Step 1
+ Y_rank0 = [[y1]] = [[w11x11 + w21x12]] = [[x11, x12]] dot [[w11, w21]]^T
+ Y_rank1 = [[y2]] = [[w11x21 + w21x22]] = [[x21, x22]] dot [[w11, w21]]^T
+ ```
+
+ Then, we have to gather them:
+ ```Step 2
+ Y_rank0 = [[y1],
+ detach([y2])]
+ Y_rank1 = [detach([y1]),
+ [y2]]
+ ```
+ Note that y2 in Y_rank0 does not have grad, neither does y1 in Y_rank1.
+
+ Similarly, we calculate the loss in each rank:
+ ```Step 3
+ z_rank0 = mean(Y_rank0) = (y1 + detach(y2)) / 2
+ z_rank1 = mean(Y_rank1) = (detach(y1) + y2) / 2
+ ```
+ So the partial derivative of loss_rank0 with respect to w11:
+ ```∂z / ∂w11 = ∂z / ∂y1 * ∂y1 / ∂w11 = x11 / 2```
+ The same for rank1:
+ ```∂z / ∂w11 = ∂z / ∂y2 * ∂y2 / ∂w11 = x21 / 2```
+
+ Finally, we need to all_reduce them:
+ ```Step 4
+ In both rank:
+ ∂z / ∂w11 = (x11 / 2 + x21 / 2) / 2 = (x11 + x21) / 4
+ ```
+
+ In SP2, the gradient of each param is only half of that in SP1.
+ So we should scale up the grad during the backward process in Step 2.
+ """ # noqa: E501
+ return _GatherForwardSplitBackward.apply(input, dim, sp_group, grad_scale)
diff --git a/xtuner/parallel/sequence/data_collate.py b/xtuner/parallel/sequence/data_collate.py
index 15b242d73..048eaec10 100644
--- a/xtuner/parallel/sequence/data_collate.py
+++ b/xtuner/parallel/sequence/data_collate.py
@@ -1,78 +1,46 @@
# Copyright (c) OpenMMLab. All rights reserved.
import torch
-from xtuner.utils import DEFAULT_PAD_TOKEN_INDEX, IGNORE_INDEX
-from .setup_distributed import (get_sequence_parallel_rank,
- get_sequence_parallel_world_size)
+from .setup_distributed import get_sequence_parallel_world_size
-def pad_for_sequence_parallel(tokens,
- labels=None,
- position_ids=None,
- attention_mask=None,
- tokens_pad_index=DEFAULT_PAD_TOKEN_INDEX,
- labels_pad_index=IGNORE_INDEX,
- position_ids_pad_index=0,
- attention_mask_pad_index=0):
- if labels is not None:
- assert tokens.shape == labels.shape
- if position_ids is not None:
- assert tokens.shape == position_ids.shape
- if attention_mask is not None:
- assert tokens.shape == attention_mask.shape
-
- bs, seq_len = tokens.shape
+def pad_for_sequence_parallel(tensor, padding_value, dim=-1):
+ length = tensor.shape[dim]
seq_parallel_world_size = get_sequence_parallel_world_size()
- if seq_len % seq_parallel_world_size == 0:
- return tokens, labels, position_ids, attention_mask
-
- pad_num = seq_parallel_world_size - (seq_len % seq_parallel_world_size)
- pad = torch.full((bs, pad_num),
- tokens_pad_index,
- dtype=tokens.dtype,
- device=tokens.device)
- tokens = torch.cat([tokens, pad], dim=1)
-
- if labels is not None:
- pad = torch.full((bs, pad_num),
- labels_pad_index,
- dtype=labels.dtype,
- device=labels.device)
- labels = torch.cat([labels, pad], dim=1)
-
- if position_ids is not None:
- pad = torch.full((bs, pad_num),
- position_ids_pad_index,
- dtype=position_ids.dtype,
- device=position_ids.device)
- position_ids = torch.cat([position_ids, pad], dim=1)
-
- if attention_mask is not None:
- pad = torch.full((bs, pad_num),
- attention_mask_pad_index,
- dtype=attention_mask.dtype,
- device=attention_mask.device)
- attention_mask = torch.cat([attention_mask, pad], dim=1)
-
- return tokens, labels, position_ids, attention_mask
-
-
-def split_for_sequence_parallel(tokens, labels=None, position_ids=None):
+ if length % seq_parallel_world_size == 0:
+ return tensor
+
+ pad_num = seq_parallel_world_size - (length % seq_parallel_world_size)
+ pad_shape = (*tensor.shape[:dim], pad_num,
+ *tensor.shape[dim + 1:]) if dim != -1 else (
+ *tensor.shape[:dim], pad_num)
+ pad = torch.full(
+ pad_shape, padding_value, dtype=tensor.dtype, device=tensor.device)
+ tensor = torch.cat([tensor, pad], dim=dim)
+ return tensor
+
+
+# This function only meets the following two conditions:
+# 1. use_varlen_attn = True
+# 2. pack_to_max_length = True and the lengths of each sequence are different
+def pad_cumulative_len_for_sequence_parallel(cumulative_len):
+ assert len(cumulative_len) == 1
+ seqlen = cumulative_len[0][-1]
seq_parallel_world_size = get_sequence_parallel_world_size()
- if seq_parallel_world_size == 1:
- return tokens, labels, position_ids
-
- seq_parallel_world_rank = get_sequence_parallel_rank()
- seq_len = tokens.size(1)
- assert seq_len % seq_parallel_world_size == 0
- sub_seq_len = seq_len // seq_parallel_world_size
- sub_seq_start = seq_parallel_world_rank * sub_seq_len
- sub_seq_end = (seq_parallel_world_rank + 1) * sub_seq_len
-
- tokens = tokens[:, sub_seq_start:sub_seq_end]
- if labels is not None:
- labels = labels[:, sub_seq_start:sub_seq_end]
- if position_ids is not None:
- position_ids = position_ids[:, sub_seq_start:sub_seq_end]
-
- return tokens, labels, position_ids
+ if seqlen % seq_parallel_world_size == 0:
+ return cumulative_len, None
+
+ bs = len(cumulative_len)
+ pad_len = seq_parallel_world_size - (seqlen % seq_parallel_world_size)
+ seqlen_new = seqlen + pad_len
+ attention_mask = torch.zeros(
+ bs, seqlen_new, dtype=torch.bool, device=cumulative_len[0].device)
+ attention_mask[:, :seqlen] = True
+
+ for i, cu_len in enumerate(cumulative_len):
+ pad = torch.tensor([seqlen_new],
+ device=cu_len.device,
+ dtype=cu_len.dtype)
+ cumulative_len[i] = torch.cat([cu_len, pad], dim=0)
+
+ return cumulative_len, attention_mask
diff --git a/xtuner/parallel/sequence/reduce_loss.py b/xtuner/parallel/sequence/reduce_loss.py
index 56a8389f4..fb37242a3 100644
--- a/xtuner/parallel/sequence/reduce_loss.py
+++ b/xtuner/parallel/sequence/reduce_loss.py
@@ -4,14 +4,31 @@
from .setup_distributed import get_sequence_parallel_group
-def reduce_sequence_parallel_loss(mean_loss, num_tokens_for_loss):
- sequence_parallel_group = get_sequence_parallel_group()
- if num_tokens_for_loss == 0:
- # convert nan to 0 just for logging
- mean_loss = torch.nan_to_num(mean_loss)
- loss_sum = mean_loss * num_tokens_for_loss
- dist.all_reduce(loss_sum, group=sequence_parallel_group)
- dist.all_reduce(num_tokens_for_loss, group=sequence_parallel_group)
+class _ReduceLoss(torch.autograd.Function):
- loss = loss_sum / num_tokens_for_loss
- return loss
+ @staticmethod
+ def forward(ctx, mean_loss, loss_scale, process_group):
+ ctx.mode = process_group
+ if loss_scale == 0:
+ # convert nan to 0 just for logging
+ mean_loss = torch.nan_to_num(mean_loss)
+ loss_sum = mean_loss * loss_scale
+ dist.all_reduce(loss_sum, group=process_group)
+ dist.all_reduce(loss_scale, group=process_group)
+ loss = loss_sum / loss_scale
+ return loss
+
+ @staticmethod
+ def backward(ctx, grad_output):
+ return grad_output, None, None
+
+
+def reduce_sequence_parallel_loss(mean_loss,
+ loss_scale,
+ sp_group: dist.ProcessGroup = None):
+ if dist.get_world_size(sp_group) == 1:
+ return mean_loss
+ if sp_group is None:
+ # avoid bc breaking
+ sp_group = get_sequence_parallel_group()
+ return _ReduceLoss.apply(mean_loss, loss_scale, sp_group)
diff --git a/xtuner/parallel/sequence/setup_distributed.py b/xtuner/parallel/sequence/setup_distributed.py
index 9eb159e66..473993a33 100644
--- a/xtuner/parallel/sequence/setup_distributed.py
+++ b/xtuner/parallel/sequence/setup_distributed.py
@@ -5,6 +5,10 @@
_SEQUENCE_PARALLEL_WORLD_SIZE = None
_SEQUENCE_PARALLEL_RANK = None
+_INNER_SEQUENCE_PARALLEL_GROUP = None
+_INNER_SEQUENCE_PARALLEL_WORLD_SIZE = None
+_INNER_SEQUENCE_PARALLEL_RANK = None
+
_DATA_PARALLEL_GROUP = None
_DATA_PARALLEL_WORLD_SIZE = None
_DATA_PARALLEL_RANK = None
@@ -49,6 +53,64 @@ def init_sequence_parallel(sequence_parallel_size: int = 1):
_DATA_PARALLEL_GROUP = group
+def init_inner_sequence_parallel(inner_sequence_parallel_size: int = 1):
+ """Build the sequence parallel inner groups.
+
+ They are helpful when sp size is not evenly divided by the number of attn
+ heads.
+ """
+ assert _SEQUENCE_PARALLEL_GROUP is not None, \
+ ('Please call `init_inner_sequence_parallel` after calling '
+ '`init_sequence_parallel`.')
+
+ rank = dist.get_rank()
+ world_size: int = dist.get_world_size()
+
+ n_inner_group = world_size // inner_sequence_parallel_size
+
+ global _INNER_SEQUENCE_PARALLEL_GROUP
+ assert _INNER_SEQUENCE_PARALLEL_GROUP is None
+
+ for i in range(n_inner_group):
+ ranks = range(i * inner_sequence_parallel_size,
+ (i + 1) * inner_sequence_parallel_size)
+ group = dist.new_group(ranks)
+ if rank in ranks:
+ _INNER_SEQUENCE_PARALLEL_GROUP = group
+
+
+def is_inner_sequence_parallel_initialized():
+ return _INNER_SEQUENCE_PARALLEL_GROUP is not None
+
+
+def get_inner_sequence_parallel_group():
+ return _INNER_SEQUENCE_PARALLEL_GROUP
+
+
+def get_inner_sequence_parallel_world_size():
+ global _INNER_SEQUENCE_PARALLEL_WORLD_SIZE
+ if _INNER_SEQUENCE_PARALLEL_WORLD_SIZE is not None:
+ return _INNER_SEQUENCE_PARALLEL_WORLD_SIZE
+ if not dist.is_initialized() or (_INNER_SEQUENCE_PARALLEL_GROUP is None):
+ _INNER_SEQUENCE_PARALLEL_WORLD_SIZE = 1
+ else:
+ _INNER_SEQUENCE_PARALLEL_WORLD_SIZE = dist.get_world_size(
+ group=get_inner_sequence_parallel_group())
+ return _INNER_SEQUENCE_PARALLEL_WORLD_SIZE
+
+
+def get_inner_sequence_parallel_rank():
+ global _INNER_SEQUENCE_PARALLEL_RANK
+ if _INNER_SEQUENCE_PARALLEL_RANK is not None:
+ return _INNER_SEQUENCE_PARALLEL_RANK
+ if not dist.is_initialized() or (_INNER_SEQUENCE_PARALLEL_GROUP is None):
+ _INNER_SEQUENCE_PARALLEL_RANK = 0
+ else:
+ _INNER_SEQUENCE_PARALLEL_RANK = dist.get_rank(
+ group=get_inner_sequence_parallel_group())
+ return _INNER_SEQUENCE_PARALLEL_RANK
+
+
def get_sequence_parallel_group():
"""Get the sequence parallel group the caller rank belongs to."""
return _SEQUENCE_PARALLEL_GROUP
@@ -59,7 +121,7 @@ def get_sequence_parallel_world_size():
global _SEQUENCE_PARALLEL_WORLD_SIZE
if _SEQUENCE_PARALLEL_WORLD_SIZE is not None:
return _SEQUENCE_PARALLEL_WORLD_SIZE
- if not dist.is_initialized():
+ if not dist.is_initialized() or (_SEQUENCE_PARALLEL_GROUP is None):
_SEQUENCE_PARALLEL_WORLD_SIZE = 1
else:
_SEQUENCE_PARALLEL_WORLD_SIZE = dist.get_world_size(
@@ -72,7 +134,7 @@ def get_sequence_parallel_rank():
global _SEQUENCE_PARALLEL_RANK
if _SEQUENCE_PARALLEL_RANK is not None:
return _SEQUENCE_PARALLEL_RANK
- if not dist.is_initialized():
+ if not dist.is_initialized() or (_SEQUENCE_PARALLEL_GROUP is None):
_SEQUENCE_PARALLEL_RANK = 0
else:
_SEQUENCE_PARALLEL_RANK = dist.get_rank(
diff --git a/xtuner/tools/chat.py b/xtuner/tools/chat.py
index 3bddac52c..209676e3b 100644
--- a/xtuner/tools/chat.py
+++ b/xtuner/tools/chat.py
@@ -18,6 +18,7 @@
from xtuner.tools.utils import get_stop_criteria
from xtuner.utils import (DEFAULT_IMAGE_TOKEN, IMAGE_TOKEN_INDEX,
PROMPT_TEMPLATE, SYSTEM_TEMPLATE)
+from xtuner.utils.device import get_device
TORCH_DTYPE_MAP = dict(
fp16=torch.float16, bf16=torch.bfloat16, fp32=torch.float32, auto='auto')
@@ -293,9 +294,9 @@ def main():
trust_remote_code=True)
print(f'Load projector from {args.llava}')
- projector.cuda()
+ projector.to(get_device())
projector.eval()
- visual_encoder.cuda()
+ visual_encoder.to(get_device())
visual_encoder.eval()
llm.eval()
@@ -306,7 +307,7 @@ def main():
image, tuple(int(x * 255) for x in image_processor.image_mean))
image = image_processor.preprocess(
image, return_tensors='pt')['pixel_values'][0]
- image = image.cuda().unsqueeze(0).to(visual_encoder.dtype)
+ image = image.to(get_device()).unsqueeze(0).to(visual_encoder.dtype)
visual_outputs = visual_encoder(image, output_hidden_states=True)
pixel_values = projector(
visual_outputs.hidden_states[args.visual_select_layer][:, 1:])
@@ -399,7 +400,7 @@ def main():
if args.with_plugins is not None:
generate_output = llm.generate(
- inputs=ids.cuda(),
+ inputs=ids.to(get_device()),
generation_config=gen_config,
streamer=streamer,
stopping_criteria=stop_criteria).cpu()
@@ -426,7 +427,7 @@ def main():
dim=1)
generate_output = llm.generate(
- inputs=new_ids.cuda(),
+ inputs=new_ids.to(get_device()),
generation_config=gen_config,
streamer=streamer,
stopping_criteria=stop_criteria)
@@ -437,7 +438,7 @@ def main():
print(output_text, end=end)
else:
generate_output = llm.generate(
- inputs=ids.cuda(),
+ inputs=ids.to(get_device()),
generation_config=gen_config,
streamer=streamer,
stopping_criteria=stop_criteria)
@@ -462,7 +463,7 @@ def main():
ids.extend(cur_chunk_encode)
if idx != len(chunk_encode) - 1:
ids.append(IMAGE_TOKEN_INDEX)
- ids = torch.tensor(ids).cuda().unsqueeze(0)
+ ids = torch.tensor(ids).to(get_device()).unsqueeze(0)
mm_inputs = prepare_inputs_labels_for_multimodal(
llm=llm, input_ids=ids, pixel_values=pixel_values)
diff --git a/xtuner/tools/eval_refcoco.py b/xtuner/tools/eval_refcoco.py
index cbdc1bf6e..675e2958d 100644
--- a/xtuner/tools/eval_refcoco.py
+++ b/xtuner/tools/eval_refcoco.py
@@ -23,6 +23,7 @@
from xtuner.tools.utils import get_stop_criteria
from xtuner.utils import (DEFAULT_IMAGE_TOKEN, IMAGE_TOKEN_INDEX,
PROMPT_TEMPLATE)
+from xtuner.utils.device import get_device, get_torch_device
TORCH_DTYPE_MAP = dict(
fp16=torch.float16, bf16=torch.bfloat16, fp32=torch.float32, auto='auto')
@@ -220,10 +221,10 @@ def build_model(args):
projector_path, torch_dtype=TORCH_DTYPE_MAP[args.torch_dtype])
master_print(f'Load projector from {args.llava}')
- projector.cuda()
+ projector.to(get_device())
projector.eval()
- visual_encoder.cuda()
+ visual_encoder.to(get_device())
visual_encoder.eval()
llm.eval()
@@ -263,7 +264,7 @@ def generate(
ids.extend(cur_chunk_encode)
if idx != len(chunk_encode) - 1:
ids.append(IMAGE_TOKEN_INDEX)
- ids = torch.tensor(ids).cuda().unsqueeze(0)
+ ids = torch.tensor(ids).to(get_device()).unsqueeze(0)
visual_outputs = visual_encoder(
samples['pixel_values'].to(device), output_hidden_states=True)
@@ -304,7 +305,7 @@ def main():
init_dist(args.launcher)
rank, world_size = get_dist_info()
- torch.cuda.set_device(rank)
+ get_torch_device().set_device(rank)
else:
rank = 0
world_size = 1
diff --git a/xtuner/tools/mmbench.py b/xtuner/tools/mmbench.py
index 133355b73..d2cd65c75 100644
--- a/xtuner/tools/mmbench.py
+++ b/xtuner/tools/mmbench.py
@@ -30,6 +30,7 @@
from xtuner.tools.utils import get_stop_criteria, is_cn_string
from xtuner.utils import (DEFAULT_IMAGE_TOKEN, IMAGE_TOKEN_INDEX,
PROMPT_TEMPLATE)
+from xtuner.utils.device import get_device, get_torch_device
TORCH_DTYPE_MAP = dict(
fp16=torch.float16, bf16=torch.bfloat16, fp32=torch.float32, auto='auto')
@@ -278,7 +279,7 @@ def main():
init_dist(args.launcher)
rank, world_size = get_dist_info()
- torch.cuda.set_device(rank)
+ get_torch_device().set_device(rank)
else:
rank = 0
world_size = 1
@@ -359,10 +360,10 @@ def main():
projector_path, torch_dtype=TORCH_DTYPE_MAP[args.torch_dtype])
master_print(f'Load projector from {args.llava}')
- projector.cuda()
+ projector.to(get_device())
projector.eval()
- visual_encoder.cuda()
+ visual_encoder.to(get_device())
visual_encoder.eval()
llm.eval()
@@ -445,7 +446,7 @@ def main():
image, tuple(int(x * 255) for x in image_processor.image_mean))
image = image_processor.preprocess(
image, return_tensors='pt')['pixel_values'][0]
- image = image.cuda().unsqueeze(0).to(visual_encoder.dtype)
+ image = image.to(get_device()).unsqueeze(0).to(visual_encoder.dtype)
visual_outputs = visual_encoder(image, output_hidden_states=True)
pixel_values = projector(
visual_outputs.hidden_states[args.visual_select_layer][:, 1:])
@@ -458,12 +459,15 @@ def main():
cur_encode = tokenizer.encode(chunk, add_special_tokens=False)
chunk_encode.append(cur_encode)
assert len(chunk_encode) == 2
+
+ # TODO: Auto-detect whether to prepend a bos_token_id at the beginning.
ids = []
+
for idx, cur_chunk_encode in enumerate(chunk_encode):
ids.extend(cur_chunk_encode)
if idx != len(chunk_encode) - 1:
ids.append(IMAGE_TOKEN_INDEX)
- ids = torch.tensor(ids).cuda().unsqueeze(0)
+ ids = torch.tensor(ids).to(get_device()).unsqueeze(0)
mm_inputs = prepare_inputs_labels_for_multimodal(
llm=llm, input_ids=ids, pixel_values=pixel_values)
diff --git a/xtuner/tools/model_converters/merge.py b/xtuner/tools/model_converters/merge.py
index 5d6826cd2..df13c7841 100644
--- a/xtuner/tools/model_converters/merge.py
+++ b/xtuner/tools/model_converters/merge.py
@@ -7,7 +7,7 @@
CLIPImageProcessor, CLIPVisionModel)
from xtuner.model.utils import LoadWoInit
-
+from xtuner.utils.device import get_device_name
def parse_args():
parser = argparse.ArgumentParser(
@@ -26,10 +26,14 @@ def parse_args():
'--is-clip',
action='store_true',
help='Indicate if the model is a clip model')
+ parser.add_argument(
+ '--safe-serialization',
+ action='store_true',
+ help='Indicate if using `safe_serialization`')
parser.add_argument(
'--device',
- default='cuda',
- choices=('cuda', 'cpu', 'auto'),
+ default=get_device_name(),
+ choices=('cuda', 'cpu', 'npu', 'auto'),
help='Indicate the device')
args = parser.parse_args()
@@ -63,7 +67,7 @@ def main():
print(f'Saving to {args.save_dir}...')
model_merged.save_pretrained(
args.save_dir,
- safe_serialization=False,
+ safe_serialization=args.safe_serialization,
max_shard_size=args.max_shard_size)
processor.save_pretrained(args.save_dir)
print('All done!')
diff --git a/xtuner/tools/model_converters/modeling_internlm2_reward/__init__.py b/xtuner/tools/model_converters/modeling_internlm2_reward/__init__.py
new file mode 100644
index 000000000..e69de29bb
diff --git a/xtuner/tools/model_converters/modeling_internlm2_reward/configuration_internlm2.py b/xtuner/tools/model_converters/modeling_internlm2_reward/configuration_internlm2.py
new file mode 100644
index 000000000..12fdffe28
--- /dev/null
+++ b/xtuner/tools/model_converters/modeling_internlm2_reward/configuration_internlm2.py
@@ -0,0 +1,154 @@
+# coding=utf-8
+# Copyright (c) The InternLM team and The HuggingFace Inc. team. All rights reserved.
+#
+# This code is based on transformers/src/transformers/models/llama/configuration_llama.py
+#
+# Licensed under the Apache License, Version 2.0 (the "License");
+# you may not use this file except in compliance with the License.
+# You may obtain a copy of the License at
+#
+# http://www.apache.org/licenses/LICENSE-2.0
+#
+# Unless required by applicable law or agreed to in writing, software
+# distributed under the License is distributed on an "AS IS" BASIS,
+# WITHOUT WARRANTIES OR CONDITIONS OF ANY KIND, either express or implied.
+# See the License for the specific language governing permissions and
+# limitations under the License.
+""" InternLM2 model configuration"""
+
+from transformers.configuration_utils import PretrainedConfig
+from transformers.utils import logging
+
+logger = logging.get_logger(__name__)
+
+INTERNLM2_PRETRAINED_CONFIG_ARCHIVE_MAP = {}
+
+
+# Modified from transformers.model.llama.configuration_llama.LlamaConfig
+class InternLM2Config(PretrainedConfig):
+ r"""
+ This is the configuration class to store the configuration of a [`InternLM2Model`]. It is used to instantiate
+ an InternLM2 model according to the specified arguments, defining the model architecture. Instantiating a
+ configuration with the defaults will yield a similar configuration to that of the InternLM2-7B.
+
+ Configuration objects inherit from [`PretrainedConfig`] and can be used to control the model outputs. Read the
+ documentation from [`PretrainedConfig`] for more information.
+
+
+ Args:
+ vocab_size (`int`, *optional*, defaults to 32000):
+ Vocabulary size of the InternLM2 model. Defines the number of different tokens that can be represented by the
+ `inputs_ids` passed when calling [`InternLM2Model`]
+ hidden_size (`int`, *optional*, defaults to 4096):
+ Dimension of the hidden representations.
+ intermediate_size (`int`, *optional*, defaults to 11008):
+ Dimension of the MLP representations.
+ num_hidden_layers (`int`, *optional*, defaults to 32):
+ Number of hidden layers in the Transformer encoder.
+ num_attention_heads (`int`, *optional*, defaults to 32):
+ Number of attention heads for each attention layer in the Transformer encoder.
+ num_key_value_heads (`int`, *optional*):
+ This is the number of key_value heads that should be used to implement Grouped Query Attention. If
+ `num_key_value_heads=num_attention_heads`, the model will use Multi Head Attention (MHA), if
+ `num_key_value_heads=1 the model will use Multi Query Attention (MQA) otherwise GQA is used. When
+ converting a multi-head checkpoint to a GQA checkpoint, each group key and value head should be constructed
+ by meanpooling all the original heads within that group. For more details checkout [this
+ paper](https://arxiv.org/pdf/2305.13245.pdf). If it is not specified, will default to
+ `num_attention_heads`.
+ hidden_act (`str` or `function`, *optional*, defaults to `"silu"`):
+ The non-linear activation function (function or string) in the decoder.
+ max_position_embeddings (`int`, *optional*, defaults to 2048):
+ The maximum sequence length that this model might ever be used with. Typically set this to something large
+ just in case (e.g., 512 or 1024 or 2048).
+ initializer_range (`float`, *optional*, defaults to 0.02):
+ The standard deviation of the truncated_normal_initializer for initializing all weight matrices.
+ rms_norm_eps (`float`, *optional*, defaults to 1e-12):
+ The epsilon used by the rms normalization layers.
+ use_cache (`bool`, *optional*, defaults to `True`):
+ Whether or not the model should return the last key/values attentions (not used by all models). Only
+ relevant if `config.is_decoder=True`.
+ tie_word_embeddings(`bool`, *optional*, defaults to `False`):
+ Whether to tie weight embeddings
+ Example:
+
+ """
+ model_type = "internlm2"
+ _auto_class = "AutoConfig"
+
+ def __init__( # pylint: disable=W0102
+ self,
+ vocab_size=103168,
+ hidden_size=4096,
+ intermediate_size=11008,
+ num_hidden_layers=32,
+ num_attention_heads=32,
+ num_key_value_heads=None,
+ hidden_act="silu",
+ max_position_embeddings=2048,
+ initializer_range=0.02,
+ rms_norm_eps=1e-6,
+ use_cache=True,
+ pad_token_id=0,
+ bos_token_id=1,
+ eos_token_id=2,
+ reward_token_id=92527,
+ tie_word_embeddings=False,
+ bias=True,
+ rope_theta=10000,
+ rope_scaling=None,
+ attn_implementation="eager",
+ **kwargs,
+ ):
+ self.vocab_size = vocab_size
+ self.max_position_embeddings = max_position_embeddings
+ self.hidden_size = hidden_size
+ self.intermediate_size = intermediate_size
+ self.num_hidden_layers = num_hidden_layers
+ self.num_attention_heads = num_attention_heads
+ self.bias = bias
+
+ if num_key_value_heads is None:
+ num_key_value_heads = num_attention_heads
+ self.num_key_value_heads = num_key_value_heads
+
+ self.hidden_act = hidden_act
+ self.initializer_range = initializer_range
+ self.rms_norm_eps = rms_norm_eps
+ self.use_cache = use_cache
+ self.rope_theta = rope_theta
+ self.rope_scaling = rope_scaling
+ self._rope_scaling_validation()
+
+ self.attn_implementation = attn_implementation
+ if self.attn_implementation is None:
+ self.attn_implementation = "eager"
+
+ self.reward_token_id = reward_token_id
+ super().__init__(
+ pad_token_id=pad_token_id,
+ bos_token_id=bos_token_id,
+ eos_token_id=eos_token_id,
+ tie_word_embeddings=tie_word_embeddings,
+ **kwargs,
+ )
+
+ def _rope_scaling_validation(self):
+ """
+ Validate the `rope_scaling` configuration.
+ """
+ if self.rope_scaling is None:
+ return
+
+ if not isinstance(self.rope_scaling, dict) or len(self.rope_scaling) != 2:
+ raise ValueError(
+ "`rope_scaling` must be a dictionary with with two fields, `type` and `factor`, "
+ f"got {self.rope_scaling}"
+ )
+ rope_scaling_type = self.rope_scaling.get("type", None)
+ rope_scaling_factor = self.rope_scaling.get("factor", None)
+ if rope_scaling_type is None or rope_scaling_type not in ["linear", "dynamic"]:
+ raise ValueError(
+ f"`rope_scaling`'s type field must be one of ['linear', 'dynamic'], got {rope_scaling_type}"
+ )
+ if rope_scaling_factor is None or not isinstance(rope_scaling_factor, float) or rope_scaling_factor < 1.0:
+ raise ValueError(f"`rope_scaling`'s factor field must be a float >= 1, got {rope_scaling_factor}")
diff --git a/xtuner/tools/model_converters/modeling_internlm2_reward/modeling_internlm2.py b/xtuner/tools/model_converters/modeling_internlm2_reward/modeling_internlm2.py
new file mode 100644
index 000000000..59cba8456
--- /dev/null
+++ b/xtuner/tools/model_converters/modeling_internlm2_reward/modeling_internlm2.py
@@ -0,0 +1,1578 @@
+# Copyright (c) The InternLM team and The HuggingFace Inc. team. All rights reserved.
+#
+# This code is based on transformers/src/transformers/models/llama/modeling_llama.py
+#
+# Licensed under the Apache License, Version 2.0 (the "License");
+# you may not use this file except in compliance with the License.
+# You may obtain a copy of the License at
+#
+# http://www.apache.org/licenses/LICENSE-2.0
+#
+# Unless required by applicable law or agreed to in writing, software
+# distributed under the License is distributed on an "AS IS" BASIS,
+# WITHOUT WARRANTIES OR CONDITIONS OF ANY KIND, either express or implied.
+# See the License for the specific language governing permissions and
+# limitations under the License.
+""" PyTorch InternLM2 model."""
+import math
+import queue
+import threading
+import warnings
+from typing import List, Optional, Tuple, Union
+
+import torch
+import torch.nn.functional as F
+import torch.utils.checkpoint
+from einops import rearrange
+from torch import nn
+from torch.nn import BCEWithLogitsLoss, CrossEntropyLoss, MSELoss
+from transformers.activations import ACT2FN
+from transformers.modeling_outputs import (
+ BaseModelOutputWithPast,
+ CausalLMOutputWithPast,
+ SequenceClassifierOutputWithPast,
+)
+from transformers.modeling_utils import PreTrainedModel
+from transformers.utils import (
+ add_start_docstrings,
+ add_start_docstrings_to_model_forward,
+ logging,
+ replace_return_docstrings,
+)
+
+try:
+ from transformers.generation.streamers import BaseStreamer
+except: # noqa # pylint: disable=bare-except
+ BaseStreamer = None
+
+from .configuration_internlm2 import InternLM2Config
+
+logger = logging.get_logger(__name__)
+
+_CONFIG_FOR_DOC = "InternLM2Config"
+
+flash_attn_func, flash_attn_varlen_func = None, None
+pad_input, index_first_axis, unpad_input = None, None, None
+def _import_flash_attn():
+ global flash_attn_func, flash_attn_varlen_func
+ global pad_input, index_first_axis, unpad_input
+ try:
+ from flash_attn import flash_attn_func as _flash_attn_func, flash_attn_varlen_func as _flash_attn_varlen_func
+ from flash_attn.bert_padding import pad_input as _pad_input, index_first_axis as _index_first_axis, unpad_input as _unpad_input
+ flash_attn_func, flash_attn_varlen_func = _flash_attn_func, _flash_attn_varlen_func
+ pad_input, index_first_axis, unpad_input = _pad_input, _index_first_axis, _unpad_input
+ except ImportError:
+ raise ImportError("flash_attn is not installed.")
+
+# Copied from transformers.models.llama.modeling_llama._get_unpad_data
+def _get_unpad_data(attention_mask):
+ seqlens_in_batch = attention_mask.sum(dim=-1, dtype=torch.int32)
+ indices = torch.nonzero(attention_mask.flatten(), as_tuple=False).flatten()
+ max_seqlen_in_batch = seqlens_in_batch.max().item()
+ cu_seqlens = F.pad(torch.cumsum(seqlens_in_batch, dim=0, dtype=torch.torch.int32), (1, 0))
+ return (
+ indices,
+ cu_seqlens,
+ max_seqlen_in_batch,
+ )
+
+
+# Copied from transformers.models.bart.modeling_bart._make_causal_mask
+def _make_causal_mask(
+ input_ids_shape: torch.Size, dtype: torch.dtype, device: torch.device, past_key_values_length: int = 0
+):
+ """
+ Make causal mask used for bi-directional self-attention.
+ """
+ bsz, tgt_len = input_ids_shape
+ mask = torch.full((tgt_len, tgt_len), torch.tensor(torch.finfo(dtype).min, device=device), device=device)
+ mask_cond = torch.arange(mask.size(-1), device=device)
+ mask.masked_fill_(mask_cond < (mask_cond + 1).view(mask.size(-1), 1), 0)
+ mask = mask.to(dtype)
+
+ if past_key_values_length > 0:
+ mask = torch.cat([torch.zeros(tgt_len, past_key_values_length, dtype=dtype, device=device), mask], dim=-1)
+ return mask[None, None, :, :].expand(bsz, 1, tgt_len, tgt_len + past_key_values_length)
+
+
+# Copied from transformers.models.bart.modeling_bart._expand_mask
+def _expand_mask(mask: torch.Tensor, dtype: torch.dtype, tgt_len: Optional[int] = None):
+ """
+ Expands attention_mask from `[bsz, seq_len]` to `[bsz, 1, tgt_seq_len, src_seq_len]`.
+ """
+ bsz, src_len = mask.size()
+ tgt_len = tgt_len if tgt_len is not None else src_len
+
+ expanded_mask = mask[:, None, None, :].expand(bsz, 1, tgt_len, src_len).to(dtype)
+
+ inverted_mask = 1.0 - expanded_mask
+
+ return inverted_mask.masked_fill(inverted_mask.to(torch.bool), torch.finfo(dtype).min)
+
+
+# Copied from transformers.models.llama.modeling_llama.LlamaRMSNorm with Llama->InternLM2
+class InternLM2RMSNorm(nn.Module):
+ def __init__(self, hidden_size, eps=1e-6):
+ """
+ InternLM2RMSNorm is equivalent to T5LayerNorm
+ """
+ super().__init__()
+ self.weight = nn.Parameter(torch.ones(hidden_size))
+ self.variance_epsilon = eps
+
+ def forward(self, hidden_states):
+ input_dtype = hidden_states.dtype
+ hidden_states = hidden_states.to(torch.float32)
+ variance = hidden_states.pow(2).mean(-1, keepdim=True)
+ hidden_states = hidden_states * torch.rsqrt(variance + self.variance_epsilon)
+ return self.weight * hidden_states.to(input_dtype)
+
+
+# Copied from transformers.model.llama.modeling_llama.LlamaRotaryEmbedding with Llama->InternLM2
+class InternLM2RotaryEmbedding(nn.Module):
+ def __init__(self, dim, max_position_embeddings=2048, base=10000, device=None):
+ super().__init__()
+
+ self.dim = dim
+ self.max_position_embeddings = max_position_embeddings
+ self.base = base
+ inv_freq = 1.0 / (self.base ** (torch.arange(0, self.dim, 2).float().to(device) / self.dim))
+ self.register_buffer("inv_freq", inv_freq, persistent=False)
+
+ # Build here to make `torch.jit.trace` work.
+ self._set_cos_sin_cache(
+ seq_len=max_position_embeddings, device=self.inv_freq.device, dtype=torch.get_default_dtype()
+ )
+
+ def _set_cos_sin_cache(self, seq_len, device, dtype):
+ self.max_seq_len_cached = seq_len
+ t = torch.arange(self.max_seq_len_cached, device=device, dtype=self.inv_freq.dtype)
+
+ freqs = torch.einsum("i,j->ij", t, self.inv_freq)
+ # Different from paper, but it uses a different permutation in order to obtain the same calculation
+ emb = torch.cat((freqs, freqs), dim=-1)
+ self.register_buffer("cos_cached", emb.cos().to(dtype), persistent=False)
+ self.register_buffer("sin_cached", emb.sin().to(dtype), persistent=False)
+
+ def forward(self, x, seq_len=None):
+ # x: [bs, num_attention_heads, seq_len, head_size]
+ if seq_len > self.max_seq_len_cached:
+ self._set_cos_sin_cache(seq_len=seq_len, device=x.device, dtype=torch.float32)
+
+ return (
+ self.cos_cached[:seq_len].to(dtype=x.dtype),
+ self.sin_cached[:seq_len].to(dtype=x.dtype),
+ )
+
+
+# Copied from transformers.model.llama.modeling_llama.LlamaLinearScalingRotaryEmbedding with Llama->InternLM2
+class InternLM2LinearScalingRotaryEmbedding(InternLM2RotaryEmbedding):
+ """InternLM2RotaryEmbedding extended with linear scaling. Credits to the Reddit user /u/kaiokendev"""
+
+ def __init__(self, dim, max_position_embeddings=2048, base=10000, device=None, scaling_factor=1.0):
+ self.scaling_factor = scaling_factor
+ super().__init__(dim, max_position_embeddings, base, device)
+
+ def _set_cos_sin_cache(self, seq_len, device, dtype):
+ self.max_seq_len_cached = seq_len
+ t = torch.arange(self.max_seq_len_cached, device=device, dtype=self.inv_freq.dtype)
+ t = t / self.scaling_factor
+
+ freqs = torch.einsum("i,j->ij", t, self.inv_freq)
+ # Different from paper, but it uses a different permutation in order to obtain the same calculation
+ emb = torch.cat((freqs, freqs), dim=-1)
+ self.register_buffer("cos_cached", emb.cos().to(dtype), persistent=False)
+ self.register_buffer("sin_cached", emb.sin().to(dtype), persistent=False)
+
+
+# Copied from transformers.model.llama.modeling_llama.LlamaDynamicNTKScalingRotaryEmbedding with Llama->InternLM2
+class InternLM2DynamicNTKScalingRotaryEmbedding(InternLM2RotaryEmbedding):
+ """InternLM2RotaryEmbedding extended with Dynamic NTK scaling.
+ Credits to the Reddit users /u/bloc97 and /u/emozilla.
+ """
+
+ def __init__(self, dim, max_position_embeddings=2048, base=10000, device=None, scaling_factor=1.0):
+ self.scaling_factor = scaling_factor
+ super().__init__(dim, max_position_embeddings, base, device)
+
+ def _set_cos_sin_cache(self, seq_len, device, dtype):
+ self.max_seq_len_cached = seq_len
+
+ if seq_len > self.max_position_embeddings:
+ base = self.base * (
+ (self.scaling_factor * seq_len / self.max_position_embeddings) - (self.scaling_factor - 1)
+ ) ** (self.dim / (self.dim - 2))
+ inv_freq = 1.0 / (base ** (torch.arange(0, self.dim, 2).float().to(device) / self.dim))
+ self.register_buffer("inv_freq", inv_freq, persistent=False)
+
+ t = torch.arange(self.max_seq_len_cached, device=device, dtype=self.inv_freq.dtype)
+
+ freqs = torch.einsum("i,j->ij", t, self.inv_freq)
+ # Different from paper, but it uses a different permutation in order to obtain the same calculation
+ emb = torch.cat((freqs, freqs), dim=-1)
+ self.register_buffer("cos_cached", emb.cos().to(dtype), persistent=False)
+ self.register_buffer("sin_cached", emb.sin().to(dtype), persistent=False)
+
+
+# Copied from transformers.model.llama.modeling_llama.rotate_half
+def rotate_half(x):
+ """Rotates half the hidden dims of the input."""
+ x1 = x[..., : x.shape[-1] // 2]
+ x2 = x[..., x.shape[-1] // 2 :]
+ return torch.cat((-x2, x1), dim=-1)
+
+
+# Copied from transformers.model.llama.modeling_llama.apply_rotary_pos_emb
+def apply_rotary_pos_emb(q, k, cos, sin, position_ids, unsqueeze_dim=1):
+ """Applies Rotary Position Embedding to the query and key tensors."""
+ cos = cos[position_ids].unsqueeze(unsqueeze_dim)
+ sin = sin[position_ids].unsqueeze(unsqueeze_dim)
+ q_embed = (q * cos) + (rotate_half(q) * sin)
+ k_embed = (k * cos) + (rotate_half(k) * sin)
+ return q_embed, k_embed
+
+
+class InternLM2MLP(nn.Module):
+ def __init__(self, config):
+ super().__init__()
+ self.config = config
+ self.hidden_size = config.hidden_size
+ self.intermediate_size = config.intermediate_size
+ self.w1 = nn.Linear(self.hidden_size, self.intermediate_size, bias=False)
+ self.w3 = nn.Linear(self.hidden_size, self.intermediate_size, bias=False)
+ self.w2 = nn.Linear(self.intermediate_size, self.hidden_size, bias=False)
+ self.act_fn = ACT2FN[config.hidden_act]
+
+ def forward(self, x):
+ down_proj = self.w2(self.act_fn(self.w1(x)) * self.w3(x))
+
+ return down_proj
+
+
+# Copied from transformers.model.llama.modeling_llama.repeat_kv
+def repeat_kv(hidden_states: torch.Tensor, n_rep: int) -> torch.Tensor:
+ """
+ This is the equivalent of torch.repeat_interleave(x, dim=1, repeats=n_rep). The hidden states go from (batch,
+ num_key_value_heads, seqlen, head_dim) to (batch, num_attention_heads, seqlen, head_dim)
+ """
+ batch, num_key_value_heads, slen, head_dim = hidden_states.shape
+ if n_rep == 1:
+ return hidden_states
+ hidden_states = hidden_states[:, :, None, :, :].expand(batch, num_key_value_heads, n_rep, slen, head_dim)
+ return hidden_states.reshape(batch, num_key_value_heads * n_rep, slen, head_dim)
+
+
+# Modified from transformers.model.llama.modeling_llama.LlamaAttention
+class InternLM2Attention(nn.Module):
+ """Multi-headed attention from 'Attention Is All You Need' paper"""
+
+ def __init__(self, config: InternLM2Config):
+ super().__init__()
+ self.config = config
+ self.hidden_size = config.hidden_size
+ self.num_heads = config.num_attention_heads
+ self.head_dim = self.hidden_size // self.num_heads
+ self.num_key_value_heads = config.num_key_value_heads
+ self.num_key_value_groups = self.num_heads // self.num_key_value_heads
+ self.max_position_embeddings = config.max_position_embeddings
+ self.is_causal = True
+
+ if (self.head_dim * self.num_heads) != self.hidden_size:
+ raise ValueError(
+ f"hidden_size must be divisible by num_heads (got `hidden_size`: {self.hidden_size}"
+ f" and `num_heads`: {self.num_heads})."
+ )
+
+ self.wqkv = nn.Linear(
+ self.hidden_size,
+ (self.num_heads + 2 * self.num_key_value_heads) * self.head_dim,
+ bias=config.bias,
+ )
+
+ self.wo = nn.Linear(self.num_heads * self.head_dim, self.hidden_size, bias=config.bias)
+ self._init_rope()
+
+ def _init_rope(self):
+ if self.config.rope_scaling is None:
+ self.rotary_emb = InternLM2RotaryEmbedding(
+ self.head_dim,
+ max_position_embeddings=self.max_position_embeddings,
+ base=self.config.rope_theta,
+ )
+ else:
+ scaling_type = self.config.rope_scaling["type"]
+ scaling_factor = self.config.rope_scaling["factor"]
+ if scaling_type == "dynamic":
+ self.rotary_emb = InternLM2DynamicNTKScalingRotaryEmbedding(
+ self.head_dim,
+ max_position_embeddings=self.max_position_embeddings,
+ base=self.config.rope_theta,
+ scaling_factor=scaling_factor,
+ )
+ elif scaling_type == "linear":
+ self.rotary_emb = InternLM2LinearScalingRotaryEmbedding(
+ self.head_dim,
+ max_position_embeddings=self.max_position_embeddings,
+ base=self.config.rope_theta,
+ scaling_factor=scaling_factor,
+ )
+ else:
+ raise ValueError("Currently we only support rotary embedding's type being 'dynamic' or 'linear'.")
+ return self.rotary_emb
+
+ def _shape(self, tensor: torch.Tensor, seq_len: int, bsz: int):
+ return tensor.view(bsz, seq_len, self.num_heads, self.head_dim).transpose(1, 2).contiguous()
+
+ def forward(
+ self,
+ hidden_states: torch.Tensor,
+ attention_mask: Optional[torch.Tensor] = None,
+ position_ids: Optional[torch.LongTensor] = None,
+ past_key_value: Optional[Tuple[torch.Tensor]] = None,
+ output_attentions: bool = False,
+ use_cache: bool = False,
+ **kwargs,
+ ) -> Tuple[torch.Tensor, Optional[torch.Tensor], Optional[Tuple[torch.Tensor]]]:
+ if "padding_mask" in kwargs:
+ warnings.warn(
+ "Passing `padding_mask` is deprecated and will be removed in v4.37. "
+ "Please make sure use `attention_mask` instead.`"
+ )
+
+ bsz, q_len, _ = hidden_states.size()
+
+ qkv_states = self.wqkv(hidden_states)
+
+ qkv_states = rearrange(
+ qkv_states,
+ "b q (h gs d) -> b q h gs d",
+ gs=2 + self.num_key_value_groups,
+ d=self.head_dim,
+ )
+
+ query_states = qkv_states[..., : self.num_key_value_groups, :]
+ query_states = rearrange(query_states, "b q h gs d -> b q (h gs) d")
+ key_states = qkv_states[..., -2, :]
+ value_states = qkv_states[..., -1, :]
+
+ query_states = query_states.transpose(1, 2)
+ key_states = key_states.transpose(1, 2)
+ value_states = value_states.transpose(1, 2)
+
+ kv_seq_len = key_states.shape[-2]
+ if past_key_value is not None:
+ kv_seq_len += past_key_value[0].shape[-2]
+ cos, sin = self.rotary_emb(value_states, seq_len=kv_seq_len)
+ query_states, key_states = apply_rotary_pos_emb(query_states, key_states, cos, sin, position_ids)
+
+ if past_key_value is not None:
+ # reuse k, v, self_attention
+ key_states = torch.cat([past_key_value[0], key_states], dim=2)
+ value_states = torch.cat([past_key_value[1], value_states], dim=2)
+
+ past_key_value = (key_states, value_states) if use_cache else None
+
+ key_states = repeat_kv(key_states, self.num_key_value_groups)
+ value_states = repeat_kv(value_states, self.num_key_value_groups)
+
+ attn_weights = torch.matmul(query_states, key_states.transpose(2, 3)) / math.sqrt(self.head_dim)
+
+ if attn_weights.size() != (bsz, self.num_heads, q_len, kv_seq_len):
+ raise ValueError(
+ f"Attention weights should be of size {(bsz, self.num_heads, q_len, kv_seq_len)}, but is"
+ f" {attn_weights.size()}"
+ )
+
+ if attention_mask is not None:
+ if attention_mask.size() != (bsz, 1, q_len, kv_seq_len):
+ raise ValueError(
+ f"Attention mask should be of size {(bsz, 1, q_len, kv_seq_len)}, but is {attention_mask.size()}"
+ )
+ attn_weights = attn_weights + attention_mask
+
+ # upcast attention to fp32
+ attn_weights = nn.functional.softmax(attn_weights, dim=-1, dtype=torch.float32).to(query_states.dtype)
+ attn_output = torch.matmul(attn_weights, value_states)
+
+ if attn_output.size() != (bsz, self.num_heads, q_len, self.head_dim):
+ raise ValueError(
+ f"`attn_output` should be of size {(bsz, self.num_heads, q_len, self.head_dim)}, but is"
+ f" {attn_output.size()}"
+ )
+
+ attn_output = attn_output.transpose(1, 2).contiguous()
+ attn_output = attn_output.reshape(bsz, q_len, self.hidden_size)
+
+ attn_output = self.wo(attn_output)
+
+ if not output_attentions:
+ attn_weights = None
+
+ return attn_output, attn_weights, past_key_value
+
+
+# Modified from transformers.model.llama.modeling_llama.InternLM2FlashAttention2
+class InternLM2FlashAttention2(InternLM2Attention):
+ """
+ InternLM2 flash attention module. This module inherits from `InternLM2Attention` as the weights of the module stays
+ untouched. The only required change would be on the forward pass where it needs to correctly call the public API of
+ flash attention and deal with padding tokens in case the input contains any of them.
+ """
+
+ def forward(
+ self,
+ hidden_states: torch.Tensor,
+ attention_mask: Optional[torch.LongTensor] = None,
+ position_ids: Optional[torch.LongTensor] = None,
+ past_key_value: Optional[Tuple[torch.Tensor]] = None,
+ output_attentions: bool = False,
+ use_cache: bool = False,
+ **kwargs,
+ ) -> Tuple[torch.Tensor, Optional[torch.Tensor], Optional[Tuple[torch.Tensor]]]:
+ # InternLM2FlashAttention2 attention does not support output_attentions
+ if "padding_mask" in kwargs:
+ warnings.warn(
+ "Passing `padding_mask` is deprecated and will be removed in v4.37. "
+ "Please make sure use `attention_mask` instead.`"
+ )
+
+ # overwrite attention_mask with padding_mask
+ attention_mask = kwargs.pop("padding_mask")
+
+ output_attentions = False
+
+ bsz, q_len, _ = hidden_states.size()
+
+ qkv_states = self.wqkv(hidden_states)
+
+ qkv_states = rearrange(
+ qkv_states,
+ "b q (h gs d) -> b q h gs d",
+ gs=2 + self.num_key_value_groups,
+ d=self.head_dim,
+ )
+
+ query_states = qkv_states[..., : self.num_key_value_groups, :]
+ query_states = rearrange(query_states, "b q h gs d -> b q (h gs) d")
+ key_states = qkv_states[..., -2, :]
+ value_states = qkv_states[..., -1, :]
+
+ query_states = query_states.transpose(1, 2)
+ key_states = key_states.transpose(1, 2)
+ value_states = value_states.transpose(1, 2)
+
+ kv_seq_len = key_states.shape[-2]
+ if past_key_value is not None:
+ kv_seq_len += past_key_value[0].shape[-2]
+
+ cos, sin = self.rotary_emb(value_states, seq_len=kv_seq_len)
+
+ query_states, key_states = apply_rotary_pos_emb(query_states, key_states, cos, sin, position_ids)
+
+ if past_key_value is not None:
+ # reuse k, v, self_attention
+ key_states = torch.cat([past_key_value[0], key_states], dim=2)
+ value_states = torch.cat([past_key_value[1], value_states], dim=2)
+
+ past_key_value = (key_states, value_states) if use_cache else None
+
+ query_states = query_states.transpose(1, 2)
+ key_states = key_states.transpose(1, 2)
+ value_states = value_states.transpose(1, 2)
+
+ attn_output = self._flash_attention_forward(
+ query_states, key_states, value_states, attention_mask, q_len
+ )
+ attn_output = attn_output.reshape(bsz, q_len, self.hidden_size).contiguous()
+ attn_output = self.wo(attn_output)
+
+ if not output_attentions:
+ attn_weights = None
+
+ return attn_output, attn_weights, past_key_value
+
+ def _flash_attention_forward(
+ self, query_states, key_states, value_states, attention_mask, query_length, dropout=0.0, softmax_scale=None
+ ):
+ """
+ Calls the forward method of Flash Attention - if the input hidden states contain at least one padding token
+ first unpad the input, then computes the attention scores and pad the final attention scores.
+
+ Args:
+ query_states (`torch.Tensor`):
+ Input query states to be passed to Flash Attention API
+ key_states (`torch.Tensor`):
+ Input key states to be passed to Flash Attention API
+ value_states (`torch.Tensor`):
+ Input value states to be passed to Flash Attention API
+ attention_mask (`torch.Tensor`):
+ The padding mask - corresponds to a tensor of size `(batch_size, seq_len)` where 0 stands for the
+ position of padding tokens and 1 for the position of non-padding tokens.
+ dropout (`int`, *optional*):
+ Attention dropout
+ softmax_scale (`float`, *optional*):
+ The scaling of QK^T before applying softmax. Default to 1 / sqrt(head_dim)
+ """
+ # Contains at least one padding token in the sequence
+ causal = self.is_causal and query_length != 1
+ if attention_mask is not None:
+ batch_size = query_states.shape[0]
+ query_states, key_states, value_states, indices_q, cu_seq_lens, max_seq_lens = self._unpad_input(
+ query_states, key_states, value_states, attention_mask, query_length
+ )
+
+ cu_seqlens_q, cu_seqlens_k = cu_seq_lens
+ max_seqlen_in_batch_q, max_seqlen_in_batch_k = max_seq_lens
+
+ attn_output_unpad = flash_attn_varlen_func(
+ query_states,
+ key_states,
+ value_states,
+ cu_seqlens_q=cu_seqlens_q,
+ cu_seqlens_k=cu_seqlens_k,
+ max_seqlen_q=max_seqlen_in_batch_q,
+ max_seqlen_k=max_seqlen_in_batch_k,
+ dropout_p=dropout,
+ softmax_scale=softmax_scale,
+ causal=causal,
+ )
+
+ attn_output = pad_input(attn_output_unpad, indices_q, batch_size, query_length)
+ else:
+ attn_output = flash_attn_func(
+ query_states, key_states, value_states, dropout, softmax_scale=softmax_scale, causal=causal
+ )
+
+ return attn_output
+
+ def _unpad_input(self, query_layer, key_layer, value_layer, attention_mask, query_length):
+ indices_k, cu_seqlens_k, max_seqlen_in_batch_k = _get_unpad_data(attention_mask)
+ batch_size, kv_seq_len, num_key_value_heads, head_dim = key_layer.shape
+
+ key_layer = index_first_axis(
+ key_layer.reshape(batch_size * kv_seq_len, num_key_value_heads, head_dim), indices_k
+ )
+ value_layer = index_first_axis(
+ value_layer.reshape(batch_size * kv_seq_len, num_key_value_heads, head_dim), indices_k
+ )
+
+ if query_length == kv_seq_len:
+ query_layer = index_first_axis(
+ query_layer.reshape(batch_size * kv_seq_len, self.num_heads, head_dim), indices_k
+ )
+ cu_seqlens_q = cu_seqlens_k
+ max_seqlen_in_batch_q = max_seqlen_in_batch_k
+ indices_q = indices_k
+ elif query_length == 1:
+ max_seqlen_in_batch_q = 1
+ cu_seqlens_q = torch.arange(
+ batch_size + 1, dtype=torch.int32, device=query_layer.device
+ ) # There is a memcpy here, that is very bad.
+ indices_q = cu_seqlens_q[:-1]
+ query_layer = query_layer.squeeze(1)
+ else:
+ # The -q_len: slice assumes left padding.
+ attention_mask = attention_mask[:, -query_length:]
+ query_layer, indices_q, cu_seqlens_q, max_seqlen_in_batch_q = unpad_input(query_layer, attention_mask)
+
+ return (
+ query_layer,
+ key_layer,
+ value_layer,
+ indices_q.to(torch.int64),
+ (cu_seqlens_q, cu_seqlens_k),
+ (max_seqlen_in_batch_q, max_seqlen_in_batch_k),
+ )
+
+INTERNLM2_ATTENTION_CLASSES = {
+ "eager": InternLM2Attention,
+ "flash_attention_2": InternLM2FlashAttention2,
+}
+
+# Modified from transformers.model.llama.modeling_llama.LlamaDecoderLayer
+class InternLM2DecoderLayer(nn.Module):
+ def __init__(self, config: InternLM2Config):
+ super().__init__()
+ self.hidden_size = config.hidden_size
+
+ self.attention = INTERNLM2_ATTENTION_CLASSES[config.attn_implementation](config=config)
+
+ self.feed_forward = InternLM2MLP(config)
+ self.attention_norm = InternLM2RMSNorm(config.hidden_size, eps=config.rms_norm_eps)
+ self.ffn_norm = InternLM2RMSNorm(config.hidden_size, eps=config.rms_norm_eps)
+
+ def forward(
+ self,
+ hidden_states: torch.Tensor,
+ attention_mask: Optional[torch.Tensor] = None,
+ position_ids: Optional[torch.LongTensor] = None,
+ past_key_value: Optional[Tuple[torch.Tensor]] = None,
+ output_attentions: Optional[bool] = False,
+ use_cache: Optional[bool] = False,
+ **kwargs,
+ ) -> Tuple[torch.FloatTensor, Optional[Tuple[torch.FloatTensor, torch.FloatTensor]]]:
+ """
+ Args:
+ hidden_states (`torch.FloatTensor`): input to the layer of shape `(batch, seq_len, embed_dim)`
+ attention_mask (`torch.FloatTensor`, *optional*):
+ attention mask of size `(batch_size, sequence_length)` if flash attention is used or `(batch_size, 1,
+ query_sequence_length, key_sequence_length)` if default attention is used.
+ output_attentions (`bool`, *optional*):
+ Whether or not to return the attentions tensors of all attention layers. See `attentions` under
+ returned tensors for more detail.
+ use_cache (`bool`, *optional*):
+ If set to `True`, `past_key_values` key value states are returned and can be used to speed up decoding
+ (see `past_key_values`).
+ past_key_value (`Tuple(torch.FloatTensor)`, *optional*): cached past key and value projection states
+ """
+ if "padding_mask" in kwargs:
+ warnings.warn(
+ "Passing `padding_mask` is deprecated and will be removed in v4.37. "
+ "Please make sure use `attention_mask` instead.`"
+ )
+
+ residual = hidden_states
+
+ hidden_states = self.attention_norm(hidden_states)
+
+ # Self Attention
+ hidden_states, self_attn_weights, present_key_value = self.attention(
+ hidden_states=hidden_states,
+ attention_mask=attention_mask,
+ position_ids=position_ids,
+ past_key_value=past_key_value,
+ output_attentions=output_attentions,
+ use_cache=use_cache,
+ **kwargs,
+ )
+ hidden_states = residual + hidden_states
+
+ # Fully Connected
+ residual = hidden_states
+ hidden_states = self.ffn_norm(hidden_states)
+ hidden_states = self.feed_forward(hidden_states)
+ hidden_states = residual + hidden_states
+
+ outputs = (hidden_states,)
+
+ if output_attentions:
+ outputs += (self_attn_weights,)
+
+ if use_cache:
+ outputs += (present_key_value,)
+
+ return outputs
+
+
+InternLM2_START_DOCSTRING = r"""
+ This model inherits from [`PreTrainedModel`]. Check the superclass documentation for the generic methods the
+ library implements for all its model (such as downloading or saving, resizing the input embeddings, pruning heads
+ etc.)
+
+ This model is also a PyTorch [torch.nn.Module](https://pytorch.org/docs/stable/nn.html#torch.nn.Module) subclass.
+ Use it as a regular PyTorch Module and refer to the PyTorch documentation for all matter related to general usage
+ and behavior.
+
+ Parameters:
+ config ([`InternLM2Config`]):
+ Model configuration class with all the parameters of the model. Initializing with a config file does not
+ load the weights associated with the model, only the configuration. Check out the
+ [`~PreTrainedModel.from_pretrained`] method to load the model weights.
+"""
+
+
+# Copied from transformers.models.llama.modeling_llama.LlamaPreTrainedModel with Llama->InternLM2
+@add_start_docstrings(
+ "The bare InternLM2 Model outputting raw hidden-states without any specific head on top.",
+ InternLM2_START_DOCSTRING,
+)
+class InternLM2PreTrainedModel(PreTrainedModel):
+ config_class = InternLM2Config
+ base_model_prefix = "model"
+ supports_gradient_checkpointing = True
+ _no_split_modules = ["InternLM2DecoderLayer"]
+ _skip_keys_device_placement = "past_key_values"
+
+ def _init_weights(self, module):
+ std = self.config.initializer_range
+ if isinstance(module, nn.Linear):
+ module.weight.data.normal_(mean=0.0, std=std)
+ if module.bias is not None:
+ module.bias.data.zero_()
+ elif isinstance(module, nn.Embedding):
+ module.weight.data.normal_(mean=0.0, std=std)
+ if module.padding_idx is not None:
+ module.weight.data[module.padding_idx].zero_()
+
+
+InternLM2_INPUTS_DOCSTRING = r"""
+ Args:
+ input_ids (`torch.LongTensor` of shape `(batch_size, sequence_length)`):
+ Indices of input sequence tokens in the vocabulary. Padding will be ignored by default should you provide
+ it.
+
+ Indices can be obtained using [`AutoTokenizer`]. See [`PreTrainedTokenizer.encode`] and
+ [`PreTrainedTokenizer.__call__`] for details.
+
+ [What are input IDs?](../glossary#input-ids)
+ attention_mask (`torch.Tensor` of shape `(batch_size, sequence_length)`, *optional*):
+ Mask to avoid performing attention on padding token indices. Mask values selected in `[0, 1]`:
+
+ - 1 for tokens that are **not masked**,
+ - 0 for tokens that are **masked**.
+
+ [What are attention masks?](../glossary#attention-mask)
+
+ Indices can be obtained using [`AutoTokenizer`]. See [`PreTrainedTokenizer.encode`] and
+ [`PreTrainedTokenizer.__call__`] for details.
+
+ If `past_key_values` is used, optionally only the last `input_ids` have to be input (see
+ `past_key_values`).
+
+ If you want to change padding behavior, you should read [`modeling_opt._prepare_decoder_attention_mask`]
+ and modify to your needs. See diagram 1 in [the paper](https://arxiv.org/abs/1910.13461) for more
+ information on the default strategy.
+
+ - 1 indicates the head is **not masked**,
+ - 0 indicates the head is **masked**.
+ position_ids (`torch.LongTensor` of shape `(batch_size, sequence_length)`, *optional*):
+ Indices of positions of each input sequence tokens in the position embeddings. Selected in the range `[0,
+ config.n_positions - 1]`.
+
+ [What are position IDs?](../glossary#position-ids)
+ past_key_values (`tuple(tuple(torch.FloatTensor))`, *optional*, returned when `use_cache=True` is passed or
+ when `config.use_cache=True`):
+ Tuple of `tuple(torch.FloatTensor)` of length `config.n_layers`, with each tuple having 2 tensors of shape
+ `(batch_size, num_heads, sequence_length, embed_size_per_head)`) and 2 additional tensors of shape
+ `(batch_size, num_heads, decoder_sequence_length, embed_size_per_head)`.
+
+ Contains pre-computed hidden-states (key and values in the self-attention blocks and in the cross-attention
+ blocks) that can be used (see `past_key_values` input) to speed up sequential decoding.
+
+ If `past_key_values` are used, the user can optionally input only the last `input_ids` (those that don't
+ have their past key value states given to this model) of shape `(batch_size, 1)` instead of all `input_ids`
+ of shape `(batch_size, sequence_length)`.
+ inputs_embeds (`torch.FloatTensor` of shape `(batch_size, sequence_length, hidden_size)`, *optional*):
+ Optionally, instead of passing `input_ids` you can choose to directly pass an embedded representation. This
+ is useful if you want more control over how to convert `input_ids` indices into associated vectors than the
+ model's internal embedding lookup matrix.
+ use_cache (`bool`, *optional*):
+ If set to `True`, `past_key_values` key value states are returned and can be used to speed up decoding (see
+ `past_key_values`).
+ output_attentions (`bool`, *optional*):
+ Whether or not to return the attentions tensors of all attention layers. See `attentions` under returned
+ tensors for more detail.
+ output_hidden_states (`bool`, *optional*):
+ Whether or not to return the hidden states of all layers. See `hidden_states` under returned tensors for
+ more detail.
+ return_dict (`bool`, *optional*):
+ Whether or not to return a [`~utils.ModelOutput`] instead of a plain tuple.
+"""
+
+
+# Modified from transformers.model.llama.modeling_llama.LlamaModel
+@add_start_docstrings(
+ "The bare InternLM2 Model outputting raw hidden-states without any specific head on top.",
+ InternLM2_START_DOCSTRING,
+)
+class InternLM2Model(InternLM2PreTrainedModel):
+ """
+ Transformer decoder consisting of *config.num_hidden_layers* layers. Each layer is a [`InternLM2DecoderLayer`]
+
+ Args:
+ config: InternLM2Config
+ """
+
+ _auto_class = "AutoModel"
+
+ def __init__(self, config: InternLM2Config):
+ super().__init__(config)
+ self.padding_idx = config.pad_token_id
+ self.vocab_size = config.vocab_size
+ self.config = config
+
+ self.tok_embeddings = nn.Embedding(config.vocab_size, config.hidden_size, self.padding_idx)
+
+ self.layers = nn.ModuleList([InternLM2DecoderLayer(config) for _ in range(config.num_hidden_layers)])
+ self.norm = InternLM2RMSNorm(config.hidden_size, eps=config.rms_norm_eps)
+
+ self.gradient_checkpointing = False
+ # Initialize weights and apply final processing
+ self.post_init()
+
+ def get_input_embeddings(self):
+ return self.tok_embeddings
+
+ def set_input_embeddings(self, value):
+ self.tok_embeddings = value
+
+ def _prepare_decoder_attention_mask(self, attention_mask, input_shape, inputs_embeds, past_key_values_length):
+ # create causal mask
+ # [bsz, seq_len] -> [bsz, 1, tgt_seq_len, src_seq_len]
+ combined_attention_mask = None
+ if input_shape[-1] > 1:
+ combined_attention_mask = _make_causal_mask(
+ input_shape,
+ inputs_embeds.dtype,
+ device=inputs_embeds.device,
+ past_key_values_length=past_key_values_length,
+ )
+
+ if attention_mask is not None:
+ # [bsz, seq_len] -> [bsz, 1, tgt_seq_len, src_seq_len]
+ expanded_attn_mask = _expand_mask(attention_mask, inputs_embeds.dtype, tgt_len=input_shape[-1]).to(
+ inputs_embeds.device
+ )
+ combined_attention_mask = (
+ expanded_attn_mask if combined_attention_mask is None else expanded_attn_mask + combined_attention_mask
+ )
+
+ return combined_attention_mask
+
+ @add_start_docstrings_to_model_forward(InternLM2_INPUTS_DOCSTRING)
+ def forward(
+ self,
+ input_ids: torch.LongTensor = None,
+ attention_mask: Optional[torch.Tensor] = None,
+ position_ids: Optional[torch.LongTensor] = None,
+ past_key_values: Optional[List[torch.FloatTensor]] = None,
+ inputs_embeds: Optional[torch.FloatTensor] = None,
+ use_cache: Optional[bool] = None,
+ output_attentions: Optional[bool] = None,
+ output_hidden_states: Optional[bool] = None,
+ return_dict: Optional[bool] = None,
+ ) -> Union[Tuple, BaseModelOutputWithPast]:
+ output_attentions = output_attentions if output_attentions is not None else self.config.output_attentions
+ output_hidden_states = (
+ output_hidden_states if output_hidden_states is not None else self.config.output_hidden_states
+ )
+ use_cache = use_cache if use_cache is not None else self.config.use_cache
+
+ return_dict = return_dict if return_dict is not None else self.config.use_return_dict
+
+ if self.config.attn_implementation == "flash_attention_2":
+ _import_flash_attn()
+
+ # retrieve input_ids and inputs_embeds
+ if input_ids is not None and inputs_embeds is not None:
+ raise ValueError("You cannot specify both input_ids and inputs_embeds at the same time")
+ elif input_ids is not None:
+ batch_size, seq_length = input_ids.shape[:2]
+ elif inputs_embeds is not None:
+ batch_size, seq_length = inputs_embeds.shape[:2]
+ else:
+ raise ValueError("You have to specify either input_ids or inputs_embeds")
+
+ seq_length_with_past = seq_length
+ past_key_values_length = 0
+ if past_key_values is not None:
+ past_key_values_length = past_key_values[0][0].shape[2]
+ seq_length_with_past = seq_length_with_past + past_key_values_length
+
+ if position_ids is None:
+ device = input_ids.device if input_ids is not None else inputs_embeds.device
+ position_ids = torch.arange(
+ past_key_values_length, seq_length + past_key_values_length, dtype=torch.long, device=device
+ )
+ position_ids = position_ids.unsqueeze(0)
+
+ if inputs_embeds is None:
+ inputs_embeds = self.tok_embeddings(input_ids)
+
+ if self.config.attn_implementation == "flash_attention_2":
+ # 2d mask is passed through the layers
+ attention_mask = attention_mask if (attention_mask is not None and 0 in attention_mask) else None
+ else:
+ if attention_mask is None:
+ attention_mask = torch.ones(
+ (batch_size, seq_length_with_past), dtype=torch.bool, device=inputs_embeds.device
+ )
+ attention_mask = self._prepare_decoder_attention_mask(
+ attention_mask, (batch_size, seq_length), inputs_embeds, past_key_values_length
+ )
+
+ # embed positions
+ hidden_states = inputs_embeds
+
+ if self.gradient_checkpointing and self.training:
+ if use_cache:
+ logger.warning_once(
+ "`use_cache=True` is incompatible with gradient checkpointing. Setting `use_cache=False`..."
+ )
+ use_cache = False
+
+ # decoder layers
+ all_hidden_states = () if output_hidden_states else None
+ all_self_attns = () if output_attentions else None
+ next_decoder_cache = () if use_cache else None
+
+ for idx, decoder_layer in enumerate(self.layers):
+ if output_hidden_states:
+ all_hidden_states += (hidden_states,)
+
+ past_key_value = past_key_values[idx] if past_key_values is not None else None
+
+ if self.gradient_checkpointing and self.training:
+
+ def create_custom_forward(module):
+ def custom_forward(*inputs):
+ # None for past_key_value
+ return module(*inputs, output_attentions, None)
+
+ return custom_forward
+
+ layer_outputs = torch.utils.checkpoint.checkpoint(
+ create_custom_forward(decoder_layer),
+ hidden_states,
+ attention_mask,
+ position_ids,
+ None,
+ )
+ else:
+ layer_outputs = decoder_layer(
+ hidden_states,
+ attention_mask=attention_mask,
+ position_ids=position_ids,
+ past_key_value=past_key_value,
+ output_attentions=output_attentions,
+ use_cache=use_cache,
+ )
+
+ hidden_states = layer_outputs[0]
+
+ if use_cache:
+ next_decoder_cache += (layer_outputs[2 if output_attentions else 1],)
+
+ if output_attentions:
+ all_self_attns += (layer_outputs[1],)
+
+ hidden_states = self.norm(hidden_states)
+
+ # add hidden states from the last decoder layer
+ if output_hidden_states:
+ all_hidden_states += (hidden_states,)
+
+ next_cache = next_decoder_cache if use_cache else None
+ if not return_dict:
+ return tuple(v for v in [hidden_states, next_cache, all_hidden_states, all_self_attns] if v is not None)
+ return BaseModelOutputWithPast(
+ last_hidden_state=hidden_states,
+ past_key_values=next_cache,
+ hidden_states=all_hidden_states,
+ attentions=all_self_attns,
+ )
+
+
+# Modified from transformers.model.llama.modeling_llama.LlamaForCausalLM
+class InternLM2ForCausalLM(InternLM2PreTrainedModel):
+ _auto_class = "AutoModelForCausalLM"
+
+ _tied_weights_keys = ["output.weight"]
+
+ def __init__(self, config):
+ super().__init__(config)
+ self.model = InternLM2Model(config)
+ self.vocab_size = config.vocab_size
+ self.output = nn.Linear(config.hidden_size, config.vocab_size, bias=False)
+
+ # Initialize weights and apply final processing
+ self.post_init()
+
+ def get_input_embeddings(self):
+ return self.model.tok_embeddings
+
+ def set_input_embeddings(self, value):
+ self.model.tok_embeddings = value
+
+ def get_output_embeddings(self):
+ return self.output
+
+ def set_output_embeddings(self, new_embeddings):
+ self.output = new_embeddings
+
+ def set_decoder(self, decoder):
+ self.model = decoder
+
+ def get_decoder(self):
+ return self.model
+
+ @add_start_docstrings_to_model_forward(InternLM2_INPUTS_DOCSTRING)
+ @replace_return_docstrings(output_type=CausalLMOutputWithPast, config_class=_CONFIG_FOR_DOC)
+ def forward(
+ self,
+ input_ids: torch.LongTensor = None,
+ attention_mask: Optional[torch.Tensor] = None,
+ position_ids: Optional[torch.LongTensor] = None,
+ past_key_values: Optional[List[torch.FloatTensor]] = None,
+ inputs_embeds: Optional[torch.FloatTensor] = None,
+ labels: Optional[torch.LongTensor] = None,
+ use_cache: Optional[bool] = None,
+ output_attentions: Optional[bool] = None,
+ output_hidden_states: Optional[bool] = None,
+ return_dict: Optional[bool] = None,
+ ) -> Union[Tuple, CausalLMOutputWithPast]:
+ r"""
+ Args:
+ labels (`torch.LongTensor` of shape `(batch_size, sequence_length)`, *optional*):
+ Labels for computing the masked language modeling loss. Indices should either be in `[0, ...,
+ config.vocab_size]` or -100 (see `input_ids` docstring). Tokens with indices set to `-100` are ignored
+ (masked), the loss is only computed for the tokens with labels in `[0, ..., config.vocab_size]`.
+
+ Returns:
+
+ Example:
+
+ ```python
+ >>> from transformers import AutoTokenizer, InternLM2ForCausalLM
+
+ >>> model = InternLM2ForCausalLM.from_pretrained(PATH_TO_CONVERTED_WEIGHTS)
+ >>> tokenizer = AutoTokenizer.from_pretrained(PATH_TO_CONVERTED_TOKENIZER)
+
+ >>> prompt = "Hey, are you conscious? Can you talk to me?"
+ >>> inputs = tokenizer(prompt, return_tensors="pt")
+
+ >>> # Generate
+ >>> generate_ids = model.generate(inputs.input_ids, max_length=30)
+ >>> tokenizer.batch_decode(generate_ids, skip_special_tokens=True, clean_up_tokenization_spaces=False)[0]
+ "Hey, are you conscious? Can you talk to me?\nI'm not conscious, but I can talk to you."
+ ```"""
+
+ output_attentions = output_attentions if output_attentions is not None else self.config.output_attentions
+ output_hidden_states = (
+ output_hidden_states if output_hidden_states is not None else self.config.output_hidden_states
+ )
+ return_dict = return_dict if return_dict is not None else self.config.use_return_dict
+
+ # decoder outputs consists of (dec_features, layer_state, dec_hidden, dec_attn)
+ outputs = self.model(
+ input_ids=input_ids,
+ attention_mask=attention_mask,
+ position_ids=position_ids,
+ past_key_values=past_key_values,
+ inputs_embeds=inputs_embeds,
+ use_cache=use_cache,
+ output_attentions=output_attentions,
+ output_hidden_states=output_hidden_states,
+ return_dict=return_dict,
+ )
+
+ hidden_states = outputs[0]
+ logits = self.output(hidden_states)
+ logits = logits.float()
+
+ loss = None
+ if labels is not None:
+ # Shift so that tokens < n predict n
+ shift_logits = logits[..., :-1, :].contiguous()
+ shift_labels = labels[..., 1:].contiguous()
+ # Flatten the tokens
+ loss_fct = CrossEntropyLoss()
+ shift_logits = shift_logits.view(-1, self.config.vocab_size)
+ shift_labels = shift_labels.view(-1)
+ # Enable model parallelism
+ shift_labels = shift_labels.to(shift_logits.device)
+ loss = loss_fct(shift_logits, shift_labels)
+
+ if not return_dict:
+ output = (logits,) + outputs[1:]
+ return (loss,) + output if loss is not None else output
+
+ return CausalLMOutputWithPast(
+ loss=loss,
+ logits=logits,
+ past_key_values=outputs.past_key_values,
+ hidden_states=outputs.hidden_states,
+ attentions=outputs.attentions,
+ )
+
+ def prepare_inputs_for_generation(
+ self, input_ids, past_key_values=None, attention_mask=None, inputs_embeds=None, **kwargs
+ ):
+ if past_key_values is not None:
+ past_length = past_key_values[0][0].shape[2]
+
+ # Some generation methods already pass only the last input ID
+ if input_ids.shape[1] > past_length:
+ remove_prefix_length = past_length
+ else:
+ # Default to old behavior: keep only final ID
+ remove_prefix_length = input_ids.shape[1] - 1
+
+ input_ids = input_ids[:, remove_prefix_length:]
+
+ position_ids = kwargs.get("position_ids", None)
+ if attention_mask is not None and position_ids is None:
+ # create position_ids on the fly for batch generation
+ position_ids = attention_mask.long().cumsum(-1) - 1
+ position_ids.masked_fill_(attention_mask == 0, 1)
+ if past_key_values:
+ position_ids = position_ids[:, -input_ids.shape[1] :]
+
+ # if `inputs_embeds` are passed, we only want to use them in the 1st generation step
+ if inputs_embeds is not None and past_key_values is None:
+ model_inputs = {"inputs_embeds": inputs_embeds}
+ else:
+ model_inputs = {"input_ids": input_ids}
+
+ model_inputs.update(
+ {
+ "position_ids": position_ids,
+ "past_key_values": past_key_values,
+ "use_cache": kwargs.get("use_cache"),
+ "attention_mask": attention_mask,
+ }
+ )
+ return model_inputs
+
+ @staticmethod
+ def _reorder_cache(past_key_values, beam_idx):
+ reordered_past = ()
+ for layer_past in past_key_values:
+ reordered_past += (
+ tuple(past_state.index_select(0, beam_idx.to(past_state.device)) for past_state in layer_past),
+ )
+ return reordered_past
+
+ def build_inputs(self, tokenizer, query: str, history: List[Tuple[str, str]] = [], meta_instruction=""):
+ if tokenizer.add_bos_token:
+ prompt = ""
+ else:
+ prompt = tokenizer.bos_token
+ if meta_instruction:
+ prompt += f"""<|im_start|>system\n{meta_instruction}<|im_end|>\n"""
+ for record in history:
+ prompt += f"""<|im_start|>user\n{record[0]}<|im_end|>\n<|im_start|>assistant\n{record[1]}<|im_end|>\n"""
+ prompt += f"""<|im_start|>user\n{query}<|im_end|>\n<|im_start|>assistant\n"""
+ return tokenizer([prompt], return_tensors="pt")
+
+ @torch.no_grad()
+ def chat(
+ self,
+ tokenizer,
+ query: str,
+ history: List[Tuple[str, str]] = [],
+ streamer: Optional[BaseStreamer] = None,
+ max_new_tokens: int = 1024,
+ do_sample: bool = True,
+ temperature: float = 0.8,
+ top_p: float = 0.8,
+ meta_instruction: str = "You are an AI assistant whose name is InternLM (书生·浦语).\n"
+ "- InternLM (书生·浦语) is a conversational language model that is developed by Shanghai AI Laboratory (上海人工智能实验室). It is designed to be helpful, honest, and harmless.\n"
+ "- InternLM (书生·浦语) can understand and communicate fluently in the language chosen by the user such as English and 中文.",
+ **kwargs,
+ ):
+ inputs = self.build_inputs(tokenizer, query, history, meta_instruction)
+ inputs = {k: v.to(self.device) for k, v in inputs.items() if torch.is_tensor(v)}
+ # also add end-of-assistant token in eos token id to avoid unnecessary generation
+ eos_token_id = [tokenizer.eos_token_id, tokenizer.convert_tokens_to_ids(["<|im_end|>"])[0]]
+ outputs = self.generate(
+ **inputs,
+ streamer=streamer,
+ max_new_tokens=max_new_tokens,
+ do_sample=do_sample,
+ temperature=temperature,
+ top_p=top_p,
+ eos_token_id=eos_token_id,
+ **kwargs,
+ )
+ outputs = outputs[0].cpu().tolist()[len(inputs["input_ids"][0]) :]
+ response = tokenizer.decode(outputs, skip_special_tokens=True)
+ response = response.split("<|im_end|>")[0]
+ history = history + [(query, response)]
+ return response, history
+
+ @torch.no_grad()
+ def stream_chat(
+ self,
+ tokenizer,
+ query: str,
+ history: List[Tuple[str, str]] = [],
+ max_new_tokens: int = 1024,
+ do_sample: bool = True,
+ temperature: float = 0.8,
+ top_p: float = 0.8,
+ **kwargs,
+ ):
+ """
+ Return a generator in format: (response, history)
+ Eg.
+ ('你好,有什么可以帮助您的吗', [('你好', '你好,有什么可以帮助您的吗')])
+ ('你好,有什么可以帮助您的吗?', [('你好', '你好,有什么可以帮助您的吗?')])
+ """
+ if BaseStreamer is None:
+ raise ModuleNotFoundError(
+ "The version of `transformers` is too low. Please make sure "
+ "that you have installed `transformers>=4.28.0`."
+ )
+
+ response_queue = queue.Queue(maxsize=20)
+
+ class ChatStreamer(BaseStreamer):
+ def __init__(self, tokenizer) -> None:
+ super().__init__()
+ self.tokenizer = tokenizer
+ self.queue = response_queue
+ self.query = query
+ self.history = history
+ self.response = ""
+ self.cache = []
+ self.received_inputs = False
+ self.queue.put((self.response, history + [(self.query, self.response)]))
+
+ def put(self, value):
+ if len(value.shape) > 1 and value.shape[0] > 1:
+ raise ValueError("ChatStreamer only supports batch size 1")
+ elif len(value.shape) > 1:
+ value = value[0]
+
+ if not self.received_inputs:
+ # The first received value is input_ids, ignore here
+ self.received_inputs = True
+ return
+
+ self.cache.extend(value.tolist())
+ token = self.tokenizer.decode(self.cache, skip_special_tokens=True)
+ if token.strip() != "<|im_end|>":
+ self.response = self.response + token
+ history = self.history + [(self.query, self.response)]
+ self.queue.put((self.response, history))
+ self.cache = []
+ else:
+ self.end()
+
+ def end(self):
+ self.queue.put(None)
+
+ def stream_producer():
+ return self.chat(
+ tokenizer=tokenizer,
+ query=query,
+ streamer=ChatStreamer(tokenizer=tokenizer),
+ history=history,
+ max_new_tokens=max_new_tokens,
+ do_sample=do_sample,
+ temperature=temperature,
+ top_p=top_p,
+ **kwargs,
+ )
+
+ def consumer():
+ producer = threading.Thread(target=stream_producer)
+ producer.start()
+ while True:
+ res = response_queue.get()
+ if res is None:
+ return
+ yield res
+
+ return consumer()
+
+# Modified from transformers.model.llama.modeling_llama.LlamaForCausalLM
+class InternLM2ForRewardModel(InternLM2PreTrainedModel):
+
+ _auto_class = "AutoModel"
+ _tied_weights_keys = ["v_head.weight"]
+
+ def __init__(self, config):
+ super().__init__(config)
+ self.model = InternLM2Model(config)
+ self.vocab_size = config.vocab_size
+ self.v_head = nn.Linear(config.hidden_size, 1, bias=False)
+ self.reward_token_id = config.reward_token_id
+
+ # Initialize weights and apply final processing
+ self.post_init()
+
+ def get_input_embeddings(self):
+ return self.model.tok_embeddings
+
+ def set_input_embeddings(self, value):
+ self.model.tok_embeddings = value
+
+ def get_output_embeddings(self):
+ return self.v_head
+
+ def set_output_embeddings(self, new_embeddings):
+ self.v_head = new_embeddings
+
+ def set_decoder(self, decoder):
+ self.model = decoder
+
+ def get_decoder(self):
+ return self.model
+
+ @add_start_docstrings_to_model_forward(InternLM2_INPUTS_DOCSTRING)
+ @replace_return_docstrings(output_type=SequenceClassifierOutputWithPast, config_class=_CONFIG_FOR_DOC)
+ def forward(
+ self,
+ input_ids: torch.LongTensor = None,
+ attention_mask: Optional[torch.Tensor] = None,
+ position_ids: Optional[torch.LongTensor] = None,
+ past_key_values: Optional[List[torch.FloatTensor]] = None,
+ inputs_embeds: Optional[torch.FloatTensor] = None,
+ labels: Optional[torch.LongTensor] = None,
+ use_cache: Optional[bool] = None,
+ output_attentions: Optional[bool] = None,
+ output_hidden_states: Optional[bool] = None,
+ return_dict: Optional[bool] = None,
+ ) -> Union[Tuple, SequenceClassifierOutputWithPast]:
+ r"""
+ Args:
+ labels (`torch.LongTensor` of shape `(batch_size, sequence_length)`, *optional*):
+ Labels for computing the masked language modeling loss. Indices should either be in `[0, ...,
+ config.vocab_size]` or -100 (see `input_ids` docstring). Tokens with indices set to `-100` are ignored
+ (masked), the loss is only computed for the tokens with labels in `[0, ..., config.vocab_size]`.
+
+ Returns:
+
+ Example:
+
+ ```python
+ >>> from transformers import AutoTokenizer, InternLM2ForCausalLM
+
+ >>> model = InternLM2ForCausalLM.from_pretrained(PATH_TO_CONVERTED_WEIGHTS)
+ >>> tokenizer = AutoTokenizer.from_pretrained(PATH_TO_CONVERTED_TOKENIZER)
+
+ >>> prompt = "Hey, are you conscious? Can you talk to me?"
+ >>> inputs = tokenizer(prompt, return_tensors="pt")
+
+ >>> # Generate
+ >>> generate_ids = model.generate(inputs.input_ids, max_length=30)
+ >>> tokenizer.batch_decode(generate_ids, skip_special_tokens=True, clean_up_tokenization_spaces=False)[0]
+ "Hey, are you conscious? Can you talk to me?\nI'm not conscious, but I can talk to you."
+ ```"""
+
+ output_attentions = output_attentions if output_attentions is not None else self.config.output_attentions
+ output_hidden_states = (
+ output_hidden_states if output_hidden_states is not None else self.config.output_hidden_states
+ )
+ return_dict = return_dict if return_dict is not None else self.config.use_return_dict
+
+ # decoder outputs consists of (dec_features, layer_state, dec_hidden, dec_attn)
+ outputs = self.model(
+ input_ids=input_ids,
+ attention_mask=attention_mask,
+ position_ids=position_ids,
+ past_key_values=past_key_values,
+ inputs_embeds=inputs_embeds,
+ use_cache=use_cache,
+ output_attentions=output_attentions,
+ output_hidden_states=output_hidden_states,
+ return_dict=return_dict,
+ )
+
+ hidden_states = outputs[0]
+ hidden_states = self.v_head(hidden_states)
+ # get end reward token's score
+ ends = attention_mask.cumsum(dim=1).argmax(dim=1).view(-1,1)
+
+ reward_scores = torch.gather(hidden_states.squeeze(-1), 1, ends)
+
+ loss = None
+
+ if not return_dict:
+ output = (reward_scores,) + outputs[1:]
+ return (loss,) + output if loss is not None else output
+
+ return SequenceClassifierOutputWithPast(
+ loss=loss,
+ logits=reward_scores,
+ past_key_values=outputs.past_key_values,
+ hidden_states=outputs.hidden_states,
+ attentions=outputs.attentions,
+ )
+
+ @torch.no_grad()
+ def get_score(
+ self,
+ tokenizer,
+ conversation: List[dict],
+ **kwargs,
+ ):
+ conversation_str = tokenizer.apply_chat_template(conversation, tokenize=False, add_generation_prompt=False)
+ input_ids = tokenizer.encode(conversation_str, return_tensors="pt", add_special_tokens=False)
+ # add reward score token at the end of the input_ids
+ input_ids = torch.cat([input_ids, torch.tensor([[self.reward_token_id]], dtype=torch.long)], dim=1).to(self.device)
+ attention_mask = torch.ones_like(input_ids, dtype=torch.bool).to(self.device)
+
+ outputs = self.forward(input_ids=input_ids, attention_mask=attention_mask, **kwargs)
+ score = outputs[0].cpu().item()
+ return score
+
+ @torch.no_grad()
+ def get_scores(
+ self,
+ tokenizer,
+ conversations: List[List[dict]],
+ **kwargs,
+ ):
+ conversation_strs = [tokenizer.apply_chat_template(conversation, tokenize=False, add_generation_prompt=False) for conversation in conversations]
+ batch_input_ids = []
+ attention_masks = []
+
+ for conversation_str in conversation_strs:
+ input_ids = tokenizer.encode(conversation_str, return_tensors="pt", add_special_tokens=False)
+ input_ids = torch.cat([input_ids, torch.tensor([[self.reward_token_id]], dtype=torch.long)], dim=1).squeeze(0)
+ attention_mask = torch.ones(input_ids.shape, dtype=torch.bool)
+ batch_input_ids.append(input_ids)
+ attention_masks.append(attention_mask)
+
+ r_pad_batch_input_ids = torch.nn.utils.rnn.pad_sequence(batch_input_ids, batch_first=True, padding_value=tokenizer.pad_token_id)
+ r_pad_attention_masks = torch.nn.utils.rnn.pad_sequence(attention_masks, batch_first=True, padding_value=False)
+
+ outputs = self.forward(input_ids=r_pad_batch_input_ids.to(self.device), attention_mask=r_pad_attention_masks.to(self.device), **kwargs)
+ scores = outputs[0].cpu().tolist()
+ return scores
+
+ @torch.no_grad()
+ def compare(
+ self,
+ tokenizer,
+ conversation1: List[dict],
+ conversation2: List[dict],
+ return_logits: bool = False,
+ **kwargs,
+ ):
+ score1 = self.get_score(tokenizer, conversation1, **kwargs)
+ score2 = self.get_score(tokenizer, conversation2, **kwargs)
+ if return_logits:
+ return score1, score2
+ else:
+ return score1 > score2
+
+ @torch.no_grad()
+ def rank(
+ self,
+ tokenizer,
+ conversations: List[List[dict]],
+ return_logits: bool = False,
+ **kwargs,
+ ):
+ scores = self.get_scores(tokenizer, conversations, **kwargs)
+ if return_logits:
+ return scores
+ else:
+ return sorted(range(len(scores)), key=lambda i: scores[i], reverse=True)
+
+
+# Copied from transformers.model.llama.modeling_llama.LlamaForSequenceClassification with Llama->InternLM2
+@add_start_docstrings(
+ """
+ The InternLM2 Model transformer with a sequence classification head on top (linear layer).
+
+ [`InternLM2ForSequenceClassification`] uses the last token in order to do the classification,
+ as other causal models (e.g. GPT-2) do.
+
+ Since it does classification on the last token, it requires to know the position of the last token. If a
+ `pad_token_id` is defined in the configuration, it finds the last token that is not a padding token in each row. If
+ no `pad_token_id` is defined, it simply takes the last value in each row of the batch. Since it cannot guess the
+ padding tokens when `inputs_embeds` are passed instead of `input_ids`, it does the same (take the last value in
+ each row of the batch).
+ """,
+ InternLM2_START_DOCSTRING,
+)
+class InternLM2ForSequenceClassification(InternLM2PreTrainedModel):
+ def __init__(self, config):
+ super().__init__(config)
+ self.num_labels = config.num_labels
+ self.model = InternLM2Model(config)
+ self.score = nn.Linear(config.hidden_size, self.num_labels, bias=False)
+
+ # Initialize weights and apply final processing
+ self.post_init()
+
+ def get_input_embeddings(self):
+ return self.model.tok_embeddings
+
+ def set_input_embeddings(self, value):
+ self.model.tok_embeddings = value
+
+ @add_start_docstrings_to_model_forward(InternLM2_INPUTS_DOCSTRING)
+ def forward(
+ self,
+ input_ids: torch.LongTensor = None,
+ attention_mask: Optional[torch.Tensor] = None,
+ position_ids: Optional[torch.LongTensor] = None,
+ past_key_values: Optional[List[torch.FloatTensor]] = None,
+ inputs_embeds: Optional[torch.FloatTensor] = None,
+ labels: Optional[torch.LongTensor] = None,
+ use_cache: Optional[bool] = None,
+ output_attentions: Optional[bool] = None,
+ output_hidden_states: Optional[bool] = None,
+ return_dict: Optional[bool] = None,
+ ) -> Union[Tuple, SequenceClassifierOutputWithPast]:
+ r"""
+ labels (`torch.LongTensor` of shape `(batch_size,)`, *optional*):
+ Labels for computing the sequence classification/regression loss. Indices should be in `[0, ...,
+ config.num_labels - 1]`. If `config.num_labels == 1` a regression loss is computed (Mean-Square loss), If
+ `config.num_labels > 1` a classification loss is computed (Cross-Entropy).
+ """
+ return_dict = return_dict if return_dict is not None else self.config.use_return_dict
+
+ transformer_outputs = self.model(
+ input_ids,
+ attention_mask=attention_mask,
+ position_ids=position_ids,
+ past_key_values=past_key_values,
+ inputs_embeds=inputs_embeds,
+ use_cache=use_cache,
+ output_attentions=output_attentions,
+ output_hidden_states=output_hidden_states,
+ return_dict=return_dict,
+ )
+ hidden_states = transformer_outputs[0]
+ logits = self.score(hidden_states)
+
+ if input_ids is not None:
+ batch_size = input_ids.shape[0]
+ else:
+ batch_size = inputs_embeds.shape[0]
+
+ if self.config.pad_token_id is None and batch_size != 1:
+ raise ValueError("Cannot handle batch sizes > 1 if no padding token is defined.")
+ if self.config.pad_token_id is None:
+ sequence_lengths = -1
+ else:
+ if input_ids is not None:
+ sequence_lengths = (torch.eq(input_ids, self.config.pad_token_id).int().argmax(-1) - 1).to(
+ logits.device
+ )
+ else:
+ sequence_lengths = -1
+
+ pooled_logits = logits[torch.arange(batch_size, device=logits.device), sequence_lengths]
+
+ loss = None
+ if labels is not None:
+ labels = labels.to(logits.device)
+ if self.config.problem_type is None:
+ if self.num_labels == 1:
+ self.config.problem_type = "regression"
+ elif self.num_labels > 1 and (labels.dtype == torch.long or labels.dtype == torch.int):
+ self.config.problem_type = "single_label_classification"
+ else:
+ self.config.problem_type = "multi_label_classification"
+
+ if self.config.problem_type == "regression":
+ loss_fct = MSELoss()
+ if self.num_labels == 1:
+ loss = loss_fct(pooled_logits.squeeze(), labels.squeeze())
+ else:
+ loss = loss_fct(pooled_logits, labels)
+ elif self.config.problem_type == "single_label_classification":
+ loss_fct = CrossEntropyLoss()
+ loss = loss_fct(pooled_logits.view(-1, self.num_labels), labels.view(-1))
+ elif self.config.problem_type == "multi_label_classification":
+ loss_fct = BCEWithLogitsLoss()
+ loss = loss_fct(pooled_logits, labels)
+ if not return_dict:
+ output = (pooled_logits,) + transformer_outputs[1:]
+ return ((loss,) + output) if loss is not None else output
+
+ return SequenceClassifierOutputWithPast(
+ loss=loss,
+ logits=pooled_logits,
+ past_key_values=transformer_outputs.past_key_values,
+ hidden_states=transformer_outputs.hidden_states,
+ attentions=transformer_outputs.attentions,
+ )
diff --git a/xtuner/tools/model_converters/pth_to_hf.py b/xtuner/tools/model_converters/pth_to_hf.py
index a2763dbed..2a4b28883 100644
--- a/xtuner/tools/model_converters/pth_to_hf.py
+++ b/xtuner/tools/model_converters/pth_to_hf.py
@@ -2,9 +2,15 @@
import argparse
import os.path as osp
import shutil
+import warnings
+from accelerate import init_empty_weights
+from accelerate.utils import set_module_tensor_to_device
+from mmengine import print_log
from mmengine.config import Config, DictAction
from mmengine.fileio import PetrelBackend, get_file_backend
+from mmengine.utils import mkdir_or_exist
+from tqdm import tqdm
from xtuner.configs import cfgs_name_path
from xtuner.model.utils import guess_load_checkpoint
@@ -28,6 +34,15 @@ def parse_args():
default='2GB',
help='Only applicable for LLM. The maximum size for '
'each sharded checkpoint.')
+ parser.add_argument(
+ '--safe-serialization',
+ action='store_true',
+ help='Indicate if using `safe_serialization`')
+ parser.add_argument(
+ '--save-format',
+ default='xtuner',
+ choices=('xtuner', 'official', 'huggingface'),
+ help='Only applicable for LLaVAModel. Indicate the save format.')
parser.add_argument(
'--cfg-options',
nargs='+',
@@ -59,10 +74,37 @@ def main():
model_name = cfg.model.type if isinstance(cfg.model.type,
str) else cfg.model.type.__name__
+ use_meta_init = True
+
if 'LLaVAModel' in model_name:
cfg.model.pretrained_pth = None
-
- model = BUILDER.build(cfg.model)
+ if args.save_format != 'xtuner':
+ use_meta_init = False
+ if 'Reward' in model_name:
+ use_meta_init = False
+ cfg.model.llm.pop('quantization_config', None)
+ if hasattr(cfg.model.llm, 'quantization_config'):
+ # Can not build a qlora model on meta device
+ use_meta_init = False
+
+ if use_meta_init:
+ try:
+ # Initializing the model with meta-tensor can reduce unwanted
+ # memory usage.
+ with init_empty_weights():
+ with warnings.catch_warnings():
+ warnings.filterwarnings(
+ 'ignore', message='.*non-meta.*', category=UserWarning)
+ model = BUILDER.build(cfg.model)
+ except NotImplementedError as e:
+ # Cannot initialize the model with meta tensor if the model is
+ # quantized.
+ if 'Cannot copy out of meta tensor' in str(e):
+ model = BUILDER.build(cfg.model)
+ else:
+ raise e
+ else:
+ model = BUILDER.build(cfg.model)
backend = get_file_backend(args.pth_model)
if isinstance(backend, PetrelBackend):
@@ -72,69 +114,28 @@ def main():
else:
state_dict = guess_load_checkpoint(args.pth_model)
- model.load_state_dict(state_dict, strict=False)
- print(f'Load PTH model from {args.pth_model}')
+ for name, param in tqdm(state_dict.items(), desc='Load State Dict'):
+ set_module_tensor_to_device(model, name, 'cpu', param)
- if 'LLaVAModel' in model_name:
- if cfg.model.get('llm') and (not cfg.model.get('freeze_llm', False)
- or cfg.model.get('llm_lora')):
- if 'PeftModel' in model.llm.__class__.__name__:
- llm_path = osp.join(args.save_dir, 'llm_adapter')
- print(f'Saving LLM adapter to {llm_path}')
- else:
- llm_path = args.save_dir
- print(f'Saving LLM tokenizer to {llm_path}')
- tokenizer = BUILDER.build(cfg.tokenizer)
- tokenizer.save_pretrained(llm_path)
- print(f'Saving LLM to {llm_path}')
- if not args.fp32:
- print('Convert LLM to float16')
- model.llm.half()
- model.llm.save_pretrained(
- llm_path, max_shard_size=args.max_shard_size)
-
- if cfg.model.get('visual_encoder') and (
- not cfg.model.get('freeze_visual_encoder', False)
- or cfg.model.get('visual_encoder_lora')):
- if 'PeftModel' in model.visual_encoder.__class__.__name__:
- visual_encoder_path = osp.join(args.save_dir,
- 'visual_encoder_adapter')
- print(
- f'Saving visual_encoder adapter to {visual_encoder_path}')
- else:
- visual_encoder_path = osp.join(args.save_dir, 'visual_encoder')
- print('Saving visual_encoder image_processor to'
- f'{visual_encoder_path}')
- image_processor = BUILDER.build(cfg.image_processor)
- image_processor.save_pretrained(visual_encoder_path)
- print(f'Saving visual_encoder to {visual_encoder_path}')
- model.visual_encoder.save_pretrained(
- visual_encoder_path, max_shard_size=args.max_shard_size)
-
- if hasattr(model, 'projector'):
- projector_path = osp.join(args.save_dir, 'projector')
- print(f'Saving projector to {projector_path}')
- model.projector.save_pretrained(
- projector_path, max_shard_size=args.max_shard_size)
- else:
- llm_path = args.save_dir
- if 'PeftModel' in model.llm.__class__.__name__:
- print(f'Saving adapter to {llm_path}')
- else:
- print(f'Saving LLM tokenizer to {llm_path}')
- tokenizer = BUILDER.build(cfg.tokenizer)
- tokenizer.save_pretrained(llm_path)
- print(f'Saving LLM to {llm_path}')
- if not args.fp32:
- print('Convert LLM to float16')
- model.llm.half()
- model.llm.save_pretrained(
- llm_path,
- max_shard_size=args.max_shard_size,
- safe_serialization=False)
+ model.llm.config.use_cache = True
+
+ print_log(f'Load PTH model from {args.pth_model}', 'current')
+
+ mkdir_or_exist(args.save_dir)
+
+ save_pretrained_kwargs = {
+ 'max_shard_size': args.max_shard_size,
+ 'safe_serialization': args.safe_serialization
+ }
+ model.to_hf(
+ cfg=cfg,
+ save_dir=args.save_dir,
+ fp32=args.fp32,
+ save_pretrained_kwargs=save_pretrained_kwargs,
+ save_format=args.save_format)
shutil.copyfile(args.config, osp.join(args.save_dir, 'xtuner_config.py'))
- print('All done!')
+ print_log('All done!', 'current')
if __name__ == '__main__':
diff --git a/xtuner/tools/model_converters/split.py b/xtuner/tools/model_converters/split.py
index da0e4d7b7..3433451e6 100644
--- a/xtuner/tools/model_converters/split.py
+++ b/xtuner/tools/model_converters/split.py
@@ -9,6 +9,7 @@
import torch
from mmengine.utils import mkdir_or_exist
+from xtuner.utils.device import get_device_name, get_torch_device
def parse_args():
parser = argparse.ArgumentParser(
@@ -41,7 +42,7 @@ def main():
checkpoints = set(index['weight_map'].values())
for ckpt in checkpoints:
state_dict = torch.load(
- osp.join(args.src_dir, ckpt), map_location='cuda')
+ osp.join(args.src_dir, ckpt), map_location=get_device_name())
keys = sorted(list(state_dict.keys()))
for k in keys:
new_state_dict_name = 'pytorch_model-{:05d}-of-{:05d}.bin'.format(
@@ -52,7 +53,7 @@ def main():
osp.join(args.dst_dir, new_state_dict_name))
cnt += 1
del state_dict
- torch.cuda.empty_cache()
+ get_torch_device().empty_cache()
with open(osp.join(args.dst_dir, 'pytorch_model.bin.index.json'),
'w') as f:
json.dump(new_index, f)
diff --git a/xtuner/tools/tokenize_ftdp_datasets.py b/xtuner/tools/tokenize_ftdp_datasets.py
index 3d37b47ee..9327a91fe 100644
--- a/xtuner/tools/tokenize_ftdp_datasets.py
+++ b/xtuner/tools/tokenize_ftdp_datasets.py
@@ -203,7 +203,7 @@ def format_sub_role(messages: List[Dict], roles_cfg) -> List[Dict]:
]:
new_message.append(message)
continue
- role_cfg = getattr(roles_cfg, message['role'])
+ role_cfg = roles_cfg[message['role']]
begin = format_begin(role_cfg, message)
new_content = begin + message['content'] + role_cfg['end']
if role_cfg.get('fallback_role'):
diff --git a/xtuner/tools/train.py b/xtuner/tools/train.py
index 23e3d2a3f..29b5d5395 100644
--- a/xtuner/tools/train.py
+++ b/xtuner/tools/train.py
@@ -23,7 +23,7 @@
from xtuner.model.utils import LoadWoInit, find_all_linear_names, traverse_dict
from xtuner.registry import BUILDER, MAP_FUNC
from xtuner.tools.utils import (auto_dtype_of_deepspeed_config,
- get_seed_from_checkpoint)
+ get_seed_from_checkpoint, set_model_resource)
def parse_args():
@@ -77,20 +77,13 @@ def register_function(cfg_dict):
register_function(value)
-def check_cfg(cfg):
+def check_cfg(cfg, args):
if getattr(cfg, 'use_varlen_attn',
False) and cfg.train_dataloader.batch_size > 1:
raise NotImplementedError(
f'If utilizing varlen attention, the batch size should be'
f' set to 1, but got {cfg.train_dataloader.batch_size}')
- if getattr(cfg, 'use_varlen_attn', False) and (not getattr(
- cfg.train_dataloader.dataset, 'pack_to_max_length', True)):
- raise AssertionError(
- 'When using varlen attention, `pack_to_max_length`'
- 'should be set to True, but got use_varlen_attn = True and '
- 'pack_to_max_length = False.')
-
if getattr(cfg, 'use_varlen_attn', False):
sequence_parallel = getattr(cfg, 'sequence_parallel', 1)
max_length = getattr(cfg.train_dataloader.dataset, 'max_length', None)
@@ -104,6 +97,34 @@ def check_cfg(cfg):
if getattr(cfg, 'sequence_parallel_size', 1) > 1:
assert SUPPORT_FLASH2, ('`flash_attn` is required if you want to use '
'sequence parallel.')
+ attn_implementation = getattr(cfg.model.llm, 'attn_implementation',
+ None)
+ assert (attn_implementation is None or
+ attn_implementation == 'flash_attention_2'), \
+ ('If you want to use sequence parallel, please set '
+ 'attn_implementation to `flash_attention_2` or do not '
+ f'set this attribute. Got `{attn_implementation}` .')
+
+ if getattr(cfg, 'use_varlen_attn', False):
+ assert SUPPORT_FLASH2, ('`flash_attn` is required if you set '
+ '`use_varlen_attn` to True.')
+ attn_implementation = getattr(cfg.model.llm, 'attn_implementation',
+ None)
+ assert (attn_implementation is None or
+ attn_implementation == 'flash_attention_2'), \
+ ('If you want to set `use_varlen_attn` to True, please set'
+ ' attn_implementation to `flash_attention_2` or do not '
+ f'set this attribute. Got `{attn_implementation}` .')
+
+ if args.deepspeed is None:
+ assert getattr(cfg, 'sequence_parallel_size', 1) == 1, \
+ ('Sequence parallel training without DeepSpeed lacks validation.'
+ 'Please use DeepSpeed to optimize the training phase by '
+ '`--deepspeed deepspeed_zero1 (deepspeed_zero2 or '
+ 'deepspeed_zero3)`.')
+
+
+
def main():
@@ -118,6 +139,7 @@ def main():
# load config
cfg = Config.fromfile(args.config)
+ set_model_resource(cfg)
if args.cfg_options is not None:
cfg.merge_from_dict(args.cfg_options)
@@ -126,7 +148,7 @@ def main():
# change these FunctionType object to str
register_function(cfg._cfg_dict)
- check_cfg(cfg)
+ check_cfg(cfg, args)
if cfg.get('framework', 'mmengine').lower() == 'huggingface':
# set default training_args
diff --git a/xtuner/tools/utils.py b/xtuner/tools/utils.py
index f0324109d..ad9c72278 100644
--- a/xtuner/tools/utils.py
+++ b/xtuner/tools/utils.py
@@ -8,6 +8,7 @@
from transformers.generation.streamers import BaseStreamer
from xtuner.utils import StopWordStoppingCriteria
+from xtuner.utils.device import get_torch_device
def get_base_model(model):
@@ -37,6 +38,24 @@ def get_streamer(model):
else:
return DecodeOutputStreamer
+def set_model_resource(cfg):
+ if cfg.get("model_resource"):
+ fn = cfg["model_resource"].get("fn")
+ args = cfg["model_resource"].get("args", {})
+ local_path = fn(cfg["pretrained_model_name_or_path"], **args)
+ s = [(cfg._cfg_dict, k, v) for k, v in cfg._cfg_dict.items()]
+ while s:
+ current_d, current_k, current_v = s.pop()
+ if current_k == "pretrained_model_name_or_path":
+ current_d[current_k] = local_path
+
+ if isinstance(current_v, dict):
+ s.extend([(current_v, k, v) for k, v in current_v.items()])
+ elif isinstance(current_v, list):
+ for i in current_v:
+ if isinstance(i, dict):
+ s.extend((i, k, v) for k, v in i.items())
+
class DecodeOutputStreamer(BaseStreamer):
"""Default streamer for HuggingFace models."""
@@ -133,15 +152,15 @@ def get_stop_criteria(
def auto_dtype_of_deepspeed_config(ds_config):
if ds_config.get('fp16') and not ds_config.get('bf16'):
if ds_config.get('fp16').get('enabled') == 'auto':
- ds_config['fp16']['enabled'] = torch.cuda.is_available()
+ ds_config['fp16']['enabled'] = get_torch_device().is_available()
elif not ds_config.get('fp16') and ds_config.get('bf16'):
if ds_config.get('bf16').get('enabled') == 'auto':
- ds_config['bf16']['enabled'] = torch.cuda.is_bf16_supported()
+ ds_config['bf16']['enabled'] = get_torch_device().is_bf16_supported()
elif ds_config.get('fp16') and ds_config.get('bf16'):
if ds_config.get('fp16').get('enabled') == 'auto':
- ds_config['fp16']['enabled'] = torch.cuda.is_available()
+ ds_config['fp16']['enabled'] = get_torch_device().is_available()
if ds_config.get('bf16').get('enabled') == 'auto':
- ds_config['bf16']['enabled'] = torch.cuda.is_bf16_supported()
+ ds_config['bf16']['enabled'] = get_torch_device().is_bf16_supported()
if (ds_config['fp16']['enabled'] is True
and ds_config['bf16']['enabled'] is True):
ds_config['fp16']['enabled'] = False
diff --git a/xtuner/utils/__init__.py b/xtuner/utils/__init__.py
index 6bc9a1173..6663b3225 100644
--- a/xtuner/utils/__init__.py
+++ b/xtuner/utils/__init__.py
@@ -1,11 +1,14 @@
# Copyright (c) OpenMMLab. All rights reserved.
from .constants import (DEFAULT_IMAGE_TOKEN, DEFAULT_PAD_TOKEN_INDEX,
IGNORE_INDEX, IMAGE_TOKEN_INDEX)
+from .handle_moe_load_and_save import (SUPPORT_MODELS, get_origin_state_dict,
+ load_state_dict_into_model)
from .stop_criteria import StopWordStoppingCriteria
from .templates import PROMPT_TEMPLATE, SYSTEM_TEMPLATE
__all__ = [
'IGNORE_INDEX', 'DEFAULT_PAD_TOKEN_INDEX', 'PROMPT_TEMPLATE',
'DEFAULT_IMAGE_TOKEN', 'SYSTEM_TEMPLATE', 'StopWordStoppingCriteria',
- 'IMAGE_TOKEN_INDEX'
+ 'IMAGE_TOKEN_INDEX', 'load_state_dict_into_model', 'get_origin_state_dict',
+ 'SUPPORT_MODELS'
]
diff --git a/xtuner/utils/device.py b/xtuner/utils/device.py
new file mode 100644
index 000000000..162885e45
--- /dev/null
+++ b/xtuner/utils/device.py
@@ -0,0 +1,82 @@
+# This code is inspired by the torchtune.
+# https://github.com/pytorch/torchtune/blob/main/torchtune/utils/_device.py
+
+import os
+import logging
+from enum import Enum
+from typing import Optional
+
+import torch
+
+logger = logging.getLogger(__name__)
+
+
+def is_torch_npu_available() -> bool:
+ """Check the availability of NPU"""
+ try:
+ import torch_npu # noqa: F401
+
+ return torch.npu.is_available()
+ except ImportError:
+ return False
+
+
+is_cuda_available = torch.cuda.is_available()
+is_npu_available = is_torch_npu_available()
+
+
+def get_device_name() -> str:
+ """Function that gets the torch.device based on the current machine.
+
+ This currently only supports CPU, CUDA, NPU.
+
+ Returns:
+ device
+ """
+ if is_cuda_available:
+ device = "cuda"
+ elif is_npu_available:
+ device = "npu"
+ else:
+ device = "cpu"
+ return device
+
+
+def get_device(device_name: Optional[str] = None) -> torch.device:
+ """Function that takes an optional device string, verifies it's correct and available given the machine and
+ distributed settings, and returns a :func:`~torch.device`. If device string is not provided, this function will
+ infer the device based on the environment.
+
+ If CUDA-like is available and being used, this function also sets the CUDA-like device.
+
+ Args:
+ device (Optional[str]): The name of the device to use, e.g. "cuda" or "cpu" or "npu".
+
+ Example:
+ >>> device = get_device("cuda")
+ >>> device
+ device(type='cuda', index=0)
+
+ Returns:
+ torch.device: Device
+ """
+ if device_name is None:
+ device_name = get_device_name()
+ device = torch.device(device_name)
+ return device
+
+
+def get_torch_device() -> any:
+ """Return the corresponding torch attribute based on the device type string.
+
+ Returns:
+ module: The corresponding torch device namespace, or torch.cuda if not found.
+ """
+ device_name = get_device_name()
+ try:
+ return getattr(torch, device_name)
+ except AttributeError:
+ logger.warning(
+ f"Device namespace '{device_name}' not found in torch, try to load torch.cuda."
+ )
+ return torch.cuda
\ No newline at end of file
diff --git a/xtuner/utils/handle_moe_load_and_save.py b/xtuner/utils/handle_moe_load_and_save.py
new file mode 100644
index 000000000..18764a82d
--- /dev/null
+++ b/xtuner/utils/handle_moe_load_and_save.py
@@ -0,0 +1,232 @@
+import json
+import os
+import re
+from collections import OrderedDict
+
+import torch
+import torch.distributed as dist
+import torch.nn as nn
+from mmengine import print_log
+from transformers.integrations import is_deepspeed_zero3_enabled
+from transformers.modeling_utils import load_state_dict
+from transformers.utils import (SAFE_WEIGHTS_INDEX_NAME, WEIGHTS_INDEX_NAME,
+ is_safetensors_available)
+
+SUPPORT_MODELS = (
+ 'DeepseekV2ForCausalLM',
+ 'MixtralForCausalLM',
+)
+
+ORDER_MAPPING = dict(
+ DeepseekV2ForCausalLM=dict(down_proj=0, gate_proj=1, up_proj=2),
+ MixtralForCausalLM=dict(down_proj=1, gate_proj=0, up_proj=2),
+)
+
+PARAM_NAME_MAPPING = dict(
+ DeepseekV2ForCausalLM=dict(
+ gate_proj='gate_proj', up_proj='up_proj', down_proj='down_proj'),
+ MixtralForCausalLM=dict(gate_proj='w1', up_proj='w3', down_proj='w2'),
+)
+
+
+def print_on_rank0(info):
+ if dist.get_rank() == 0:
+ print_log(info, 'current')
+
+
+def get_expert_num_per_shard(model):
+ for module in model.modules():
+ if hasattr(module, 'expert_in_one_shard'):
+ return module.expert_in_one_shard
+
+
+def mix_sort(expert_name):
+ components = re.findall(r'(\D+|\d+)', expert_name)
+ out = [int(comp) if comp.isdigit() else comp for comp in components]
+ return tuple(out)
+
+
+def _get_merged_param_name(origin_param_name, expert_num_per_shard):
+ split_name = origin_param_name.split('.experts.')
+ expert_idx = re.findall(r'\d+', split_name[1])[0]
+ expert_idx = int(expert_idx)
+ assert expert_idx % expert_num_per_shard == 0
+ shard_idx = expert_idx // expert_num_per_shard
+ w1w3 = split_name[0] + f'.experts.{shard_idx}.w1w3'
+ w2 = split_name[0] + f'.experts.{shard_idx}.w2'
+ return w1w3, w2
+
+
+def _merge_experts_weight(state_dict, expert_num_per_shard, order_mapping):
+ experts_name = [key for key in state_dict.keys() if '.experts.' in key]
+ experts_name = sorted(experts_name, key=mix_sort)
+ linear_num_per_expert = 3
+ linear_num_per_shard = expert_num_per_shard * linear_num_per_expert
+ expert_shard_num = len(experts_name) // linear_num_per_shard
+ for shard_idx in range(expert_shard_num):
+ begin, end = shard_idx * linear_num_per_shard, (
+ shard_idx + 1) * linear_num_per_shard
+ experts_name_cur = experts_name[begin:end]
+
+ down_proj_weight = [
+ state_dict.pop(key)
+ for key in experts_name_cur[order_mapping['down_proj']::3]
+ ]
+ gate_proj_weight = [
+ state_dict.pop(key)
+ for key in experts_name_cur[order_mapping['gate_proj']::3]
+ ]
+ up_proj_weight = [
+ state_dict.pop(key)
+ for key in experts_name_cur[order_mapping['up_proj']::3]
+ ]
+ w1 = torch.stack(gate_proj_weight)
+ w3 = torch.stack(up_proj_weight)
+ w1w3 = torch.cat([w1, w3], dim=1)
+ assert w1w3.ndim == 3, w1w3.shape
+ w2 = torch.stack(down_proj_weight)
+ assert w2.ndim == 3, w2.shape
+ merged_key_w1w3, merged_key_w2 = _get_merged_param_name(
+ experts_name_cur[0], expert_num_per_shard)
+ print_on_rank0(f'merged key {merged_key_w1w3}')
+ state_dict[merged_key_w1w3] = w1w3
+ print_on_rank0(f'merged key {merged_key_w2}')
+ state_dict[merged_key_w2] = w2
+
+ return
+
+
+def load_state_dict_into_model(model_to_load, pretrained_model_path):
+
+ model_name = type(model_to_load).__name__
+ if model_name not in SUPPORT_MODELS:
+ raise RuntimeError(
+ f'Only models in {SUPPORT_MODELS} may need to load pretrained '
+ f'weights via `load_state_dict_into_model`, but got {model_name}.')
+ order_mapping = ORDER_MAPPING[model_name]
+
+ index_file = os.path.join(pretrained_model_path, WEIGHTS_INDEX_NAME)
+ safe_index_file = os.path.join(pretrained_model_path,
+ SAFE_WEIGHTS_INDEX_NAME)
+ index_present = os.path.isfile(index_file)
+ safe_index_present = os.path.isfile(safe_index_file)
+ assert index_present or (safe_index_present and is_safetensors_available())
+ if safe_index_present and is_safetensors_available():
+ load_index = safe_index_file
+ else:
+ load_index = index_file
+ with open(load_index, encoding='utf-8') as f:
+ index = json.load(f)
+ weight_map = index['weight_map']
+ unloaded_shard_files = list(set(weight_map.values()))
+ unloaded_shard_files.sort(reverse=True)
+
+ expert_num_per_shard = get_expert_num_per_shard(model_to_load)
+ error_msgs = []
+
+ def load(module: nn.Module, state_dict, unloaded_shard_files, prefix=''):
+ params_to_gather = []
+ param_names = []
+ for name, param in module.named_parameters(
+ prefix=prefix[:-1], recurse=False):
+ while name not in state_dict:
+ assert len(unloaded_shard_files) > 0
+ shard_file = unloaded_shard_files.pop()
+ shard_file = os.path.join(pretrained_model_path, shard_file)
+ print_on_rank0(
+ f'{name} not in state_dict, loading {shard_file}')
+ new_shard = load_state_dict(shard_file, is_quantized=False)
+ state_dict.update(new_shard)
+ _merge_experts_weight(state_dict, expert_num_per_shard,
+ order_mapping)
+ params_to_gather.append(param)
+ param_names.append(name)
+ if len(params_to_gather) > 0:
+ args = (state_dict, prefix, {}, True, [], [], error_msgs)
+ if is_deepspeed_zero3_enabled():
+ import deepspeed
+ with deepspeed.zero.GatheredParameters(
+ params_to_gather, modifier_rank=0):
+ if dist.get_rank() == 0:
+ module._load_from_state_dict(*args)
+ else:
+ module._load_from_state_dict(*args)
+
+ for name in param_names:
+ print_on_rank0(f'state_dict pop {name}')
+ state_dict.pop(name)
+
+ for name, child in module._modules.items():
+ if child is not None:
+ load(child, state_dict, unloaded_shard_files,
+ prefix + name + '.')
+
+ state_dict = OrderedDict()
+ load(model_to_load, state_dict, unloaded_shard_files, prefix='')
+ print_on_rank0(f'{state_dict.keys()}')
+ del state_dict
+
+ return error_msgs
+
+
+def _get_origin_param_name(merged_param_name, expert_num_per_shard, is_w1w3,
+ param_name_mapping):
+ split_name = merged_param_name.split('.experts.')
+ shard_idx = re.findall(r'\d+', split_name[1])[0]
+ shard_idx = int(shard_idx)
+ origin_param_names = [None] * (expert_num_per_shard * (1 + int(is_w1w3)))
+ expert_idx_begin = expert_num_per_shard * shard_idx
+ for i in range(expert_num_per_shard):
+ if is_w1w3:
+ gate_proj, up_proj = param_name_mapping[
+ 'gate_proj'], param_name_mapping['up_proj']
+ gate = split_name[
+ 0] + f'.experts.{expert_idx_begin + i}.{gate_proj}.weight'
+ up = split_name[
+ 0] + f'.experts.{expert_idx_begin + i}.{up_proj}.weight'
+ origin_param_names[i * 2] = gate
+ origin_param_names[i * 2 + 1] = up
+ else:
+ down_proj = param_name_mapping['down_proj']
+ down = split_name[
+ 0] + f'.experts.{expert_idx_begin + i}.{down_proj}.weight'
+ origin_param_names[i] = down
+ return origin_param_names
+
+
+def _split_param(merged_param, is_w1w3):
+ if is_w1w3:
+ expert_num, _, hidden_dim = merged_param.shape
+ merged_param = merged_param.view(expert_num * 2, -1, hidden_dim)
+ return torch.unbind(merged_param, dim=0)
+ else:
+ # (e, hidden_dim, ffn_dim)
+ return torch.unbind(merged_param, dim=0)
+
+
+def get_origin_state_dict(state_dict, model):
+
+ model_name = type(model).__name__
+ if model_name not in SUPPORT_MODELS:
+ raise RuntimeError(
+ f'Only models in {SUPPORT_MODELS} may need to convert state_dict '
+ f'via `get_origin_state_dict` interface, but got {model_name}.')
+ param_name_mapping = PARAM_NAME_MAPPING[model_name]
+
+ expert_num_per_shard = get_expert_num_per_shard(model)
+ experts_param_name = [
+ name for name in state_dict.keys() if '.experts.' in name
+ ]
+ for expert_param_name in experts_param_name:
+ print_on_rank0(f'processing {expert_param_name} ...')
+ is_w1w3 = expert_param_name.split('.')[-1] == 'w1w3'
+ origin_param_names = _get_origin_param_name(expert_param_name,
+ expert_num_per_shard,
+ is_w1w3,
+ param_name_mapping)
+ merged_param = state_dict.pop(expert_param_name)
+ origin_params = _split_param(merged_param, is_w1w3)
+ assert len(origin_param_names) == len(origin_params)
+ for name, param in zip(origin_param_names, origin_params):
+ state_dict[name] = param
+ return state_dict
diff --git a/xtuner/utils/templates.py b/xtuner/utils/templates.py
index 077c9cf14..0e5732a3e 100644
--- a/xtuner/utils/templates.py
+++ b/xtuner/utils/templates.py
@@ -116,6 +116,12 @@
SYSTEM=('[INST] {system} [/INST]\n'),
INSTRUCTION=('[INST] {input} [/INST]'),
SEP='\n'),
+ deepseek_v2=dict(
+ SYSTEM='{system}\n\n',
+ INSTRUCTION='User: {input}\n\nAssistant: ',
+ SUFFIX='<|end▁of▁sentence|>',
+ SUFFIX_AS_EOS=True,
+ STOP_WORDS=['<|end▁of▁sentence|>']),
mistral=dict(
SYSTEM=('[INST] {system} [/INST]\n'),
INSTRUCTION=('[INST] {input} [/INST]'),
@@ -124,6 +130,15 @@
SYSTEM=('[INST] {system} [/INST]\n'),
INSTRUCTION=('[INST] {input} [/INST]'),
SEP='\n'),
+ minicpm=dict(INSTRUCTION=('<用户> {input} '), SEP='\n'),
+ minicpm3=dict(
+ SYSTEM=('<|im_start|>system\n{system}<|im_end|>\n'),
+ INSTRUCTION=('<|im_start|>user\n{input}<|im_end|>\n'
+ '<|im_start|>assistant\n'),
+ SUFFIX='<|im_end|>',
+ SUFFIX_AS_EOS=True,
+ SEP='\n',
+ STOP_WORDS=['<|im_end|>', '<|endoftext|>']),
gemma=dict(
# `system` field is extended by xtuner
SYSTEM=('system\n{system}\n'),
@@ -133,6 +148,31 @@
SUFFIX_AS_EOS=False,
SEP='\n',
STOP_WORDS=['']),
+ cohere_chat=dict(
+ SYSTEM=('<|START_OF_TURN_TOKEN|><|SYSTEM_TOKEN|>{system}'
+ '<|END_OF_TURN_TOKEN|>'),
+ INSTRUCTION=(
+ '<|START_OF_TURN_TOKEN|><|USER_TOKEN|>{input}<|END_OF_TURN_TOKEN|>'
+ '<|START_OF_TURN_TOKEN|><|CHATBOT_TOKEN|>'),
+ SUFFIX='<|END_OF_TURN_TOKEN|>',
+ SUFFIX_AS_EOS=True,
+ STOP_WORDS=['<|END_OF_TURN_TOKEN|>']),
+ llama3_chat=dict(
+ SYSTEM=('<|start_header_id|>system<|end_header_id|>\n\n'
+ '{system}<|eot_id|>'),
+ INSTRUCTION=(
+ '<|start_header_id|>user<|end_header_id|>\n\n{input}<|eot_id|>'
+ '<|start_header_id|>assistant<|end_header_id|>\n\n'),
+ SUFFIX='<|eot_id|>',
+ SUFFIX_AS_EOS=True,
+ STOP_WORDS=['<|eot_id|>']),
+ phi3_chat=dict(
+ SYSTEM='<|system|>\n{system}<|end|>\n',
+ INSTRUCTION='<|user|>\n{input}<|end|>\n<|assistant|>\n',
+ SUFFIX='<|end|>',
+ SUFFIX_AS_EOS=True,
+ SEP='\n',
+ STOP_WORDS=['<|end|>']),
)
SYSTEM_TEMPLATE = ConfigDict(
diff --git a/xtuner/utils/zero_to_any_dtype.py b/xtuner/utils/zero_to_any_dtype.py
new file mode 100644
index 000000000..efe1fc0a1
--- /dev/null
+++ b/xtuner/utils/zero_to_any_dtype.py
@@ -0,0 +1,696 @@
+#!/usr/bin/env python
+
+# Copyright (c) Microsoft Corporation.
+# SPDX-License-Identifier: Apache-2.0
+
+# DeepSpeed Team
+
+# This script extracts consolidated weights from a zero 1, 2 and 3 DeepSpeed
+# checkpoints. It gets copied into the top level checkpoint dir, so the user
+# can easily do the conversion at any point in the future. Once extracted, the
+# weights don't require DeepSpeed and can be used in any application.
+#
+# example: python zero_to_any_dtype.py . pytorch_model.bin
+
+import argparse
+import glob
+import math
+import os
+import re
+from collections import OrderedDict
+from dataclasses import dataclass
+
+import torch
+# yapf: disable
+from deepspeed.checkpoint.constants import (BUFFER_NAMES, DS_VERSION,
+ FP32_FLAT_GROUPS,
+ FROZEN_PARAM_FRAGMENTS,
+ FROZEN_PARAM_SHAPES,
+ OPTIMIZER_STATE_DICT, PARAM_SHAPES,
+ PARTITION_COUNT,
+ SINGLE_PARTITION_OF_FP32_GROUPS,
+ ZERO_STAGE)
+# while this script doesn't use deepspeed to recover data, since the
+# checkpoints are pickled with DeepSpeed data structures it has to be
+# available in the current python environment.
+from deepspeed.utils import logger
+from tqdm import tqdm
+
+# yapf: enable
+
+
+@dataclass
+class zero_model_state:
+ buffers: dict()
+ param_shapes: dict()
+ shared_params: list
+ ds_version: int
+ frozen_param_shapes: dict()
+ frozen_param_fragments: dict()
+
+
+debug = 0
+
+# load to cpu
+device = torch.device('cpu')
+
+DEFAULT_DTYPE = torch.float16
+
+
+def atoi(text):
+ return int(text) if text.isdigit() else text
+
+
+def natural_keys(text):
+ """alist.sort(key=natural_keys) sorts in human order
+ http://nedbatchelder.com/blog/200712/human_sorting.html (See Toothy's
+ implementation in the comments)"""
+ return [atoi(c) for c in re.split(r'(\d+)', text)]
+
+
+def get_model_state_file(checkpoint_dir, zero_stage):
+ if not os.path.isdir(checkpoint_dir):
+ raise FileNotFoundError(f"Directory '{checkpoint_dir}' doesn't exist")
+
+ # there should be only one file
+ if zero_stage <= 2:
+ file = os.path.join(checkpoint_dir, 'mp_rank_00_model_states.pt')
+ elif zero_stage == 3:
+ file = os.path.join(checkpoint_dir,
+ 'zero_pp_rank_0_mp_rank_00_model_states.pt')
+
+ if not os.path.exists(file):
+ raise FileNotFoundError(f"can't find model states file at '{file}'")
+
+ return file
+
+
+def get_checkpoint_files(checkpoint_dir, glob_pattern):
+ # XXX: need to test that this simple glob rule works for multi-node
+ # setup too
+ ckpt_files = sorted(
+ glob.glob(os.path.join(checkpoint_dir, glob_pattern)),
+ key=natural_keys)
+
+ if len(ckpt_files) == 0:
+ raise FileNotFoundError(
+ f"can't find {glob_pattern} files in directory '{checkpoint_dir}'")
+
+ return ckpt_files
+
+
+def get_optim_files(checkpoint_dir):
+ return get_checkpoint_files(checkpoint_dir, '*_optim_states.pt')
+
+
+def get_model_state_files(checkpoint_dir):
+ return get_checkpoint_files(checkpoint_dir, '*_model_states.pt')
+
+
+def parse_model_states(files, dtype=DEFAULT_DTYPE):
+ zero_model_states = []
+ for file in files:
+ state_dict = torch.load(file, map_location=device)
+
+ if BUFFER_NAMES not in state_dict:
+ raise ValueError(f'{file} is not a model state checkpoint')
+ buffer_names = state_dict[BUFFER_NAMES]
+ if debug:
+ print('Found buffers:', buffer_names)
+
+ buffers = {
+ k: v.to(dtype)
+ for k, v in state_dict['module'].items() if k in buffer_names
+ }
+ param_shapes = state_dict[PARAM_SHAPES]
+
+ # collect parameters that are included in param_shapes
+ param_names = []
+ for s in param_shapes:
+ for name in s.keys():
+ param_names.append(name)
+
+ # update with frozen parameters
+ frozen_param_shapes = state_dict.get(FROZEN_PARAM_SHAPES, None)
+ if frozen_param_shapes is not None:
+ if debug:
+ print(f'Found frozen_param_shapes: {frozen_param_shapes}')
+ param_names += list(frozen_param_shapes.keys())
+
+ # handle shared params
+ shared_params = [[k, v]
+ for k, v in state_dict['shared_params'].items()]
+
+ ds_version = state_dict.get(DS_VERSION, None)
+
+ frozen_param_fragments = state_dict.get(FROZEN_PARAM_FRAGMENTS, None)
+
+ z_model_state = zero_model_state(
+ buffers=buffers,
+ param_shapes=param_shapes,
+ shared_params=shared_params,
+ ds_version=ds_version,
+ frozen_param_shapes=frozen_param_shapes,
+ frozen_param_fragments=frozen_param_fragments)
+ zero_model_states.append(z_model_state)
+
+ return zero_model_states
+
+
+@torch.no_grad()
+def parse_optim_states(files, ds_checkpoint_dir, dtype=DEFAULT_DTYPE):
+
+ zero_stage = None
+ world_size = None
+ total_files = len(files)
+ flat_groups = []
+ for f in tqdm(files, desc='Load Checkpoints'):
+ state_dict = torch.load(f, map_location=device)
+ if ZERO_STAGE not in state_dict[OPTIMIZER_STATE_DICT]:
+ raise ValueError(f'{f} is not a zero checkpoint')
+
+ zero_stage = state_dict[OPTIMIZER_STATE_DICT][ZERO_STAGE]
+ world_size = state_dict[OPTIMIZER_STATE_DICT][PARTITION_COUNT]
+
+ # the groups are named differently in each stage
+ if zero_stage <= 2:
+ fp32_groups_key = SINGLE_PARTITION_OF_FP32_GROUPS
+ elif zero_stage == 3:
+ fp32_groups_key = FP32_FLAT_GROUPS
+ else:
+ raise ValueError(f'unknown zero stage {zero_stage}')
+
+ # immediately discard the potentially huge 2 optimizer states as we
+ # only care for fp32 master weights and also handle the case where it
+ # was already removed by another helper script
+ state_dict['optimizer_state_dict'].pop('optimizer_state_dict', None)
+ fp32_groups = state_dict['optimizer_state_dict'].pop(fp32_groups_key)
+ if zero_stage <= 2:
+ flat_groups.append([param.to(dtype) for param in fp32_groups])
+ elif zero_stage == 3:
+ # if there is more than one param group, there will be multiple
+ # flattened tensors - one flattened tensor per group - for
+ # simplicity merge them into a single tensor
+
+ # XXX: could make the script more memory efficient for when there
+ # are multiple groups - it will require matching the sub-lists of
+ # param_shapes for each param group flattened tensor
+ flat_groups.append(torch.cat(fp32_groups, 0).to(dtype))
+
+ # For ZeRO-2 each param group can have different partition_count as data
+ # parallelism for expert parameters can be different from data parallelism
+ # for non-expert parameters. So we can just use the max of the
+ # partition_count to get the dp world_size.
+ if type(world_size) is list:
+ world_size = max(world_size)
+
+ if world_size != total_files:
+ raise ValueError(
+ f"Expected {world_size} of '*_optim_states.pt' under "
+ f"'{ds_checkpoint_dir}' but found {total_files} files. "
+ 'Possibly due to an overwrite of an old checkpoint, '
+ "or a checkpoint didn't get saved by one or more processes.")
+
+ return zero_stage, world_size, flat_groups
+
+
+def _get_state_dict_from_zero_checkpoint(ds_checkpoint_dir,
+ exclude_frozen_parameters,
+ dtype=DEFAULT_DTYPE):
+ """Returns state_dict reconstructed from ds checkpoint.
+
+ Args:
+ - ``ds_checkpoint_dir``: path to the deepspeed checkpoint folder
+ (where the optimizer files are)
+ """
+ print(f"Processing zero checkpoint '{ds_checkpoint_dir}'")
+
+ optim_files = get_optim_files(ds_checkpoint_dir)
+ zero_stage, world_size, flat_groups = parse_optim_states(
+ optim_files, ds_checkpoint_dir, dtype)
+ print(f'Detected checkpoint of type zero stage {zero_stage}, '
+ f'world_size: {world_size}')
+
+ model_files = get_model_state_files(ds_checkpoint_dir)
+
+ zero_model_states = parse_model_states(model_files)
+ print(f'Parsing checkpoint created by deepspeed=='
+ f'{zero_model_states[0].ds_version}')
+
+ if zero_stage <= 2:
+ return _get_state_dict_from_zero2_checkpoint(
+ world_size, flat_groups, zero_model_states,
+ exclude_frozen_parameters)
+ elif zero_stage == 3:
+ return _get_state_dict_from_zero3_checkpoint(
+ world_size, flat_groups, zero_model_states,
+ exclude_frozen_parameters)
+
+
+def _zero2_merge_frozen_params(state_dict, zero_model_states):
+ if zero_model_states[0].frozen_param_shapes is None or len(
+ zero_model_states[0].frozen_param_shapes) == 0:
+ return
+
+ frozen_param_shapes = zero_model_states[0].frozen_param_shapes
+ frozen_param_fragments = zero_model_states[0].frozen_param_fragments
+
+ if debug:
+ num_elem = sum(s.numel() for s in frozen_param_shapes.values())
+ print(f'rank 0: {FROZEN_PARAM_SHAPES}.numel = {num_elem}')
+
+ wanted_params = len(frozen_param_shapes)
+ wanted_numel = sum(s.numel() for s in frozen_param_shapes.values())
+ avail_numel = sum([p.numel() for p in frozen_param_fragments.values()])
+ print(f'Frozen params: Have {avail_numel} numels to process.')
+ print(f'Frozen params: Need {wanted_numel} numels in '
+ f'{wanted_params} params')
+
+ total_params = 0
+ total_numel = 0
+ for name, shape in frozen_param_shapes.items():
+ total_params += 1
+ unpartitioned_numel = shape.numel()
+ total_numel += unpartitioned_numel
+
+ state_dict[name] = frozen_param_fragments[name]
+
+ if debug:
+ print(f'{name} full shape: {shape} unpartitioned numel '
+ f'{unpartitioned_numel} ')
+
+ print(f'Reconstructed Frozen state dict with {total_params} params '
+ f'{total_numel} elements')
+
+
+def _has_callable(obj, fn):
+ attr = getattr(obj, fn, None)
+ return callable(attr)
+
+
+def _zero2_merge_trainable_params(state_dict, world_size, flat_groups,
+ zero_model_states):
+ param_shapes = zero_model_states[0].param_shapes
+
+ # Reconstruction protocol:
+ #
+ # XXX: document this
+
+ if debug:
+ for i in range(world_size):
+ for j in range(len(flat_groups[0])):
+ print(f'flat_groups[{i}][{j}].shape={flat_groups[i][j].shape}')
+
+ # XXX: memory usage doubles here (zero2)
+ num_param_groups = len(flat_groups[0])
+ merged_single_partition_of_groups = []
+ for i in range(num_param_groups):
+ merged_partitions = [sd[i] for sd in flat_groups]
+ full_single_vector = torch.cat(merged_partitions, 0)
+ merged_single_partition_of_groups.append(full_single_vector)
+ avail_numel = sum([
+ full_single_vector.numel()
+ for full_single_vector in merged_single_partition_of_groups
+ ])
+
+ if debug:
+ wanted_params = sum([len(shapes) for shapes in param_shapes])
+ wanted_numel = sum([
+ sum(shape.numel() for shape in shapes.values())
+ for shapes in param_shapes
+ ])
+ # not asserting if there is a mismatch due to possible padding
+ print(f'Have {avail_numel} numels to process.')
+ print(f'Need {wanted_numel} numels in {wanted_params} params.')
+
+ # params
+ # XXX: for huge models that can't fit into the host's RAM we will have to
+ # recode this to support out-of-core computing solution
+ total_numel = 0
+ total_params = 0
+ for shapes, full_single_vector in zip(param_shapes,
+ merged_single_partition_of_groups):
+ offset = 0
+ avail_numel = full_single_vector.numel()
+ for name, shape in shapes.items():
+
+ unpartitioned_numel = shape.numel() if _has_callable(
+ shape, 'numel') else math.prod(shape)
+ total_numel += unpartitioned_numel
+ total_params += 1
+
+ if debug:
+ print(f'{name} full shape: {shape} unpartitioned numel '
+ f'{unpartitioned_numel} ')
+ state_dict[name] = full_single_vector.narrow(
+ 0, offset, unpartitioned_numel).view(shape)
+ offset += unpartitioned_numel
+
+ # Z2 started to align to 2*world_size to improve nccl performance.
+ # Therefore both offset and avail_numel can differ by anywhere between
+ # 0..2*world_size. Due to two unrelated complex paddings performed in
+ # the code it's almost impossible to predict the exact numbers w/o the
+ # live optimizer object, so we are checking that the numbers are
+ # within the right range
+ align_to = 2 * world_size
+
+ def zero2_align(x):
+ return align_to * math.ceil(x / align_to)
+
+ if debug:
+ print(f'original offset={offset}, avail_numel={avail_numel}')
+
+ offset = zero2_align(offset)
+ avail_numel = zero2_align(avail_numel)
+
+ if debug:
+ print(f'aligned offset={offset}, avail_numel={avail_numel}')
+
+ # Sanity check
+ if offset != avail_numel:
+ raise ValueError(f'consumed {offset} numels out of {avail_numel} '
+ '- something is wrong')
+
+ print(f'Reconstructed state dict with {total_params} params '
+ f'{total_numel} elements')
+
+
+def _get_state_dict_from_zero2_checkpoint(world_size, flat_groups,
+ zero_model_states,
+ exclude_frozen_parameters):
+ state_dict = OrderedDict()
+
+ # buffers
+ buffers = zero_model_states[0].buffers
+ state_dict.update(buffers)
+ if debug:
+ print(f'added {len(buffers)} buffers')
+
+ if not exclude_frozen_parameters:
+ _zero2_merge_frozen_params(state_dict, zero_model_states)
+
+ _zero2_merge_trainable_params(state_dict, world_size, flat_groups,
+ zero_model_states)
+
+ # recover shared parameters
+ for pair in zero_model_states[0].shared_params:
+ if pair[1] in state_dict:
+ state_dict[pair[0]] = state_dict[pair[1]]
+
+ return state_dict
+
+
+def zero3_partitioned_param_info(unpartitioned_numel, world_size):
+ remainder = unpartitioned_numel % world_size
+ padding_numel = (world_size - remainder) if remainder else 0
+ partitioned_numel = math.ceil(unpartitioned_numel / world_size)
+ return partitioned_numel, padding_numel
+
+
+def _zero3_merge_frozen_params(state_dict, world_size, zero_model_states):
+ if zero_model_states[0].frozen_param_shapes is None or len(
+ zero_model_states[0].frozen_param_shapes) == 0:
+ return
+
+ if debug:
+ for i in range(world_size):
+ num_elem = sum(
+ s.numel()
+ for s in zero_model_states[i].frozen_param_fragments.values())
+ print(f'rank {i}: {FROZEN_PARAM_SHAPES}.numel = {num_elem}')
+
+ frozen_param_shapes = zero_model_states[0].frozen_param_shapes
+ wanted_params = len(frozen_param_shapes)
+ wanted_numel = sum(s.numel() for s in frozen_param_shapes.values())
+ avail_numel = sum([
+ p.numel()
+ for p in zero_model_states[0].frozen_param_fragments.values()
+ ]) * world_size
+ print(f'Frozen params: Have {avail_numel} numels to process.')
+ print(f'Frozen params: Need {wanted_numel} numels in '
+ f'{wanted_params} params')
+
+ total_params = 0
+ total_numel = 0
+ for name, shape in zero_model_states[0].frozen_param_shapes.items():
+ total_params += 1
+ unpartitioned_numel = shape.numel()
+ total_numel += unpartitioned_numel
+
+ param_frags = tuple(model_state.frozen_param_fragments[name]
+ for model_state in zero_model_states)
+ state_dict[name] = torch.cat(param_frags, 0).narrow(
+ 0, 0, unpartitioned_numel).view(shape) # noqa: E501
+
+ _partitioned = zero3_partitioned_param_info(unpartitioned_numel,
+ world_size)
+ partitioned_numel, partitioned_padding_numel = _partitioned
+ if debug:
+ print(f'Frozen params: {total_params} {name} full shape: {shape} '
+ f'partition0 numel={partitioned_numel} '
+ f'partitioned_padding_numel={partitioned_padding_numel}')
+
+ print(f'Reconstructed Frozen state dict with {total_params} params '
+ f'{total_numel} elements')
+
+
+def _zero3_merge_trainable_params(state_dict, world_size, flat_groups,
+ zero_model_states):
+ param_shapes = zero_model_states[0].param_shapes
+ avail_numel = flat_groups[0].numel() * world_size
+ # Reconstruction protocol: For zero3 we need to zip the partitions
+ # together at boundary of each param, re-consolidating each param, while
+ # dealing with padding if any
+
+ # merge list of dicts, preserving order
+ param_shapes = {k: v for d in param_shapes for k, v in d.items()}
+
+ if debug:
+ for i in range(world_size):
+ print(f'flat_groups[{i}].shape={flat_groups[i].shape}')
+
+ wanted_params = len(param_shapes)
+ wanted_numel = sum(shape.numel() for shape in param_shapes.values())
+ # not asserting if there is a mismatch due to possible padding
+ avail_numel = flat_groups[0].numel() * world_size
+ print(f'Trainable params: Have {avail_numel} numels to process.')
+ print(f'Trainable params: Need {wanted_numel} numels in '
+ f'{wanted_params} params.')
+
+ offset = 0
+ total_numel = 0
+ total_params = 0
+ partitioned_sizes = []
+ for name, shape in param_shapes.items():
+
+ unpartitioned_numel = shape.numel()
+ total_numel += unpartitioned_numel
+ total_params += 1
+
+ _info = zero3_partitioned_param_info(unpartitioned_numel, world_size)
+
+ partitioned_numel, partitioned_padding_numel = _info
+ partitioned_sizes.append(partitioned_numel)
+ if debug:
+ print(
+ f'Trainable params: {total_params} {name} full shape: {shape} '
+ f'partition0 numel={partitioned_numel} '
+ f'partitioned_padding_numel={partitioned_padding_numel}')
+
+ offset += partitioned_numel
+
+ offset *= world_size
+
+ # Sanity check
+ if offset != avail_numel:
+ raise ValueError(f'consumed {offset} numels out of {avail_numel} '
+ '- something is wrong')
+
+ mat_chunks = []
+ for rank in range(world_size):
+ rank_chunks = flat_groups.pop(0).split(partitioned_sizes)
+ rank_chunks = [tensor.clone() for tensor in rank_chunks]
+ mat_chunks.append(rank_chunks)
+
+ for name, shape in tqdm(
+ param_shapes.items(), desc='Gather Sharded Weights'):
+
+ pad_flat_param_chunks = []
+ for rank in range(world_size):
+ pad_flat_param_chunks.append(mat_chunks[rank].pop(0))
+
+ pad_flat_param = torch.cat(pad_flat_param_chunks, dim=0)
+
+ # Because pad_flat_param_chunks is a list, it is necessary to manually
+ # release the tensors in the list; Python will not automatically do so.
+ for rank in range(world_size):
+ pad_flat_param_chunks.pop()
+
+ param = pad_flat_param[:shape.numel()].view(shape)
+ state_dict[name] = param
+
+ print(f'Reconstructed Trainable state dict with {total_params} params '
+ f'{total_numel} elements')
+
+
+def _get_state_dict_from_zero3_checkpoint(world_size, flat_groups,
+ zero_model_states,
+ exclude_frozen_parameters):
+ state_dict = OrderedDict()
+
+ # buffers
+ buffers = zero_model_states[0].buffers
+ state_dict.update(buffers)
+ if debug:
+ print(f'added {len(buffers)} buffers')
+
+ if not exclude_frozen_parameters:
+ _zero3_merge_frozen_params(state_dict, world_size, zero_model_states)
+
+ _zero3_merge_trainable_params(state_dict, world_size, flat_groups,
+ zero_model_states)
+
+ # recover shared parameters
+ for pair in zero_model_states[0].shared_params:
+ if pair[1] in state_dict:
+ state_dict[pair[0]] = state_dict[pair[1]]
+
+ return state_dict
+
+
+def get_state_dict_from_zero_checkpoint(checkpoint_dir,
+ tag=None,
+ exclude_frozen_parameters=False,
+ dtype=DEFAULT_DTYPE):
+ # flake8: noqa
+ """Convert ZeRO 2 or 3 checkpoint into a single consolidated state_dict
+ that can be loaded with ``load_state_dict()`` and used for training without
+ DeepSpeed or shared with others, for example via a model hub.
+
+ Args:
+ - ``checkpoint_dir``: path to the desired checkpoint folder
+ - ``tag``: checkpoint tag used as a unique identifier for checkpoint.
+ If not provided will attempt to load tag in 'latest' file.
+ e.g., ``global_step14``
+ - ``exclude_frozen_parameters``: exclude frozen parameters
+
+ Returns:
+ - pytorch ``state_dict``
+
+ Note: this approach may not work if your application doesn't have
+ sufficient free CPU memory and you may need to use the offline approach
+ using the ``zero_to_any_dtype.py`` script that is saved with the
+ checkpoint.
+
+ A typical usage might be ::
+
+ from xtuner.utils.zero_to_any_dtype import get_state_dict_from_zero_checkpoint
+ # do the training and checkpoint saving
+ state_dict = get_state_dict_from_zero_checkpoint(checkpoint_dir, dtype=torch.float16) # already on cpu
+ model = model.cpu() # move to cpu
+ model.load_state_dict(state_dict)
+ # submit to model hub or save the model to share with others
+
+ In this example the ``model`` will no longer be usable in the deepspeed
+ context of the same application. i.e. you will need to re-initialize the
+ deepspeed engine, since ``model.load_state_dict(state_dict)`` will remove
+ all the deepspeed magic from it.
+
+ If you want it all done for you, use
+ ``load_state_dict_from_zero_checkpoint`` instead.
+ """
+ # flake8: noqa
+ if tag is None:
+ latest_path = os.path.join(checkpoint_dir, 'latest')
+ if os.path.isfile(latest_path):
+ with open(latest_path) as fd:
+ tag = fd.read().strip()
+ else:
+ raise ValueError(f"Unable to find 'latest' file at {latest_path}")
+
+ ds_checkpoint_dir = os.path.join(checkpoint_dir, tag)
+
+ if not os.path.isdir(ds_checkpoint_dir):
+ raise FileNotFoundError(
+ f"Directory '{ds_checkpoint_dir}' doesn't exist")
+
+ return _get_state_dict_from_zero_checkpoint(ds_checkpoint_dir,
+ exclude_frozen_parameters,
+ dtype)
+
+
+def convert_zero_checkpoint_to_state_dict(checkpoint_dir,
+ output_file,
+ tag=None,
+ exclude_frozen_parameters=False,
+ dtype=DEFAULT_DTYPE):
+ """Convert ZeRO 2 or 3 checkpoint into a single consolidated ``state_dict``
+ file that can be loaded with ``torch.load(file)`` + ``load_state_dict()``
+ and used for training without DeepSpeed.
+
+ Args:
+ - ``checkpoint_dir``: path to the desired checkpoint folder.
+ (one that contains the tag-folder, like ``global_step14``)
+ - ``output_file``: path to the pytorch state_dict output file
+ (e.g. path/pytorch_model.bin)
+ - ``tag``: checkpoint tag used as a unique identifier for checkpoint.
+ If not provided will attempt to load tag in the file named
+ ``latest`` in the checkpoint folder, e.g., ``global_step14``
+ - ``exclude_frozen_parameters``: exclude frozen parameters
+ """
+
+ state_dict = get_state_dict_from_zero_checkpoint(
+ checkpoint_dir, tag, exclude_frozen_parameters, dtype)
+ print(f'Saving {dtype} state dict to {output_file}')
+ torch.save(state_dict, output_file)
+
+
+def load_state_dict_from_zero_checkpoint(model,
+ checkpoint_dir,
+ tag=None,
+ dtype=DEFAULT_DTYPE):
+
+ # flake8: noqa
+ """
+ 1. Put the provided model to cpu
+ 2. Convert ZeRO 2 or 3 checkpoint into a single consolidated ``state_dict``
+ 3. Load it into the provided model
+
+ Args:
+ - ``model``: the model object to update
+ - ``checkpoint_dir``: path to the desired checkpoint folder. (one that
+ contains the tag-folder, like ``global_step14``)
+ - ``tag``: checkpoint tag used as a unique identifier for checkpoint.
+ If not provided will attempt to load tag in the file named
+ ``latest`` in the checkpoint folder, e.g., ``global_step14``
+
+ Returns:
+ - ``model`: modified model
+
+ Make sure you have plenty of CPU memory available before you call this
+ function. If you don't have enough use the ``zero_to_any_dtype.py``
+ utility to do the conversion. You will find it conveniently placed for you
+ in the checkpoint folder.
+
+ A typical usage might be ::
+
+ from xtuner.utils.zero_to_any_dtype import load_state_dict_from_zero_checkpoint
+ model = load_state_dict_from_zero_checkpoint(trainer.model, checkpoint_dir, dtype=torch.float16)
+ # submit to model hub or save the model to share with others
+
+ Note, that once this was run, the ``model`` will no longer be usable in
+ the deepspeed context of the same application. i.e. you will need to
+ re-initialize the deepspeed engine, since
+ ``model.load_state_dict(state_dict)`` will remove all the deepspeed magic
+ from it.
+ """
+ # flake8: noqa
+ logger.info(f'Extracting {dtype} weights')
+ state_dict = get_state_dict_from_zero_checkpoint(
+ checkpoint_dir, tag, dtype=dtype)
+
+ logger.info(f'Overwriting model with {dtype} weights')
+ model = model.cpu()
+ model.load_state_dict(state_dict, strict=False)
+
+ return model
diff --git a/xtuner/version.py b/xtuner/version.py
index fd9b131a9..e4669c188 100644
--- a/xtuner/version.py
+++ b/xtuner/version.py
@@ -1,5 +1,5 @@
# Copyright (c) OpenMMLab. All rights reserved.
-__version__ = '0.1.17'
+__version__ = '0.1.23'
short_version = __version__