forked from PaddlePaddle/PaddleOCR
-
Notifications
You must be signed in to change notification settings - Fork 0
Commit
This commit does not belong to any branch on this repository, and may belong to a fork outside of the repository.
add hetong notebook (PaddlePaddle#7706)
- Loading branch information
Showing
1 changed file
with
284 additions
and
0 deletions.
There are no files selected for viewing
This file contains bidirectional Unicode text that may be interpreted or compiled differently than what appears below. To review, open the file in an editor that reveals hidden Unicode characters.
Learn more about bidirectional Unicode characters
Original file line number | Diff line number | Diff line change |
---|---|---|
@@ -0,0 +1,284 @@ | ||
# 金融智能核验:扫描合同关键信息抽取 | ||
|
||
本案例将使用OCR技术和通用信息抽取技术,实现合同关键信息审核和比对。通过本章的学习,你可以快速掌握: | ||
|
||
1. 使用PaddleOCR提取扫描文本内容 | ||
2. 使用PaddleNLP抽取自定义信息 | ||
|
||
点击进入 [AI Studio 项目](https://aistudio.baidu.com/aistudio/projectdetail/4545772) | ||
|
||
## 1. 项目背景 | ||
合同审核广泛应用于大中型企业、上市公司、证券、基金公司中,是规避风险的重要任务。 | ||
- 合同内容对比:合同审核场景中,快速找出不同版本合同修改区域、版本差异;如合同盖章归档场景中有效识别实际签署的纸质合同、电子版合同差异。 | ||
|
||
- 合规性检查:法务人员进行合同审核,如合同完备性检查、大小写金额检查、签约主体一致性检查、双方权利和义务对等性分析等。 | ||
|
||
- 风险点识别:通过合同审核可识别事实倾向型风险点和数值计算型风险点等,例如交付地点约定不明、合同总价款不一致、重要条款缺失等风险点。 | ||
|
||
|
||
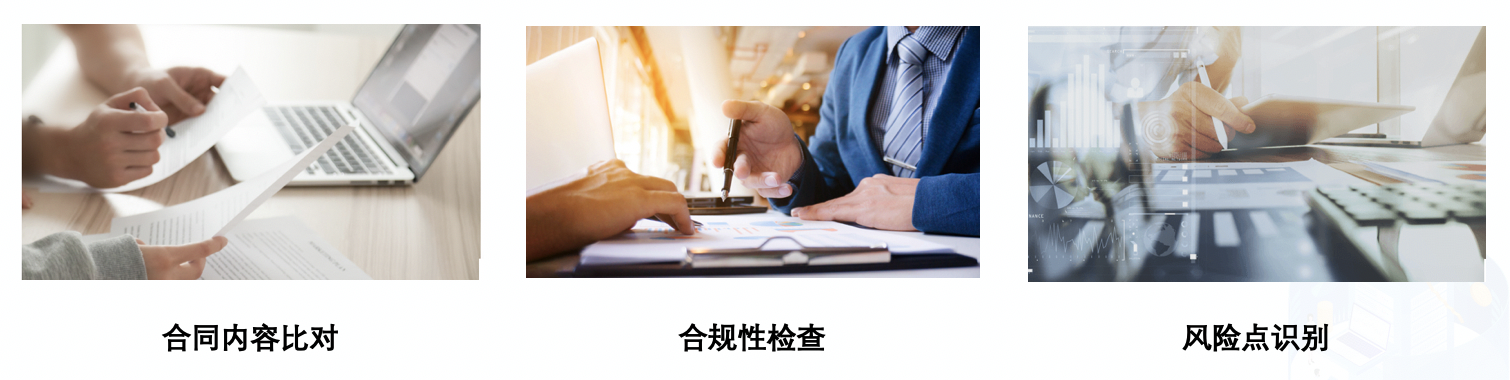 | ||
|
||
传统业务中大多使用人工进行纸质版合同审核,存在成本高,工作量大,效率低的问题,且一旦出错将造成巨额损失。 | ||
|
||
|
||
本项目针对以上场景,使用PaddleOCR+PaddleNLP快速提取文本内容,经过少量数据微调即可准确抽取关键信息,**高效完成合同内容对比、合规性检查、风险点识别等任务,提高效率,降低风险**。 | ||
|
||
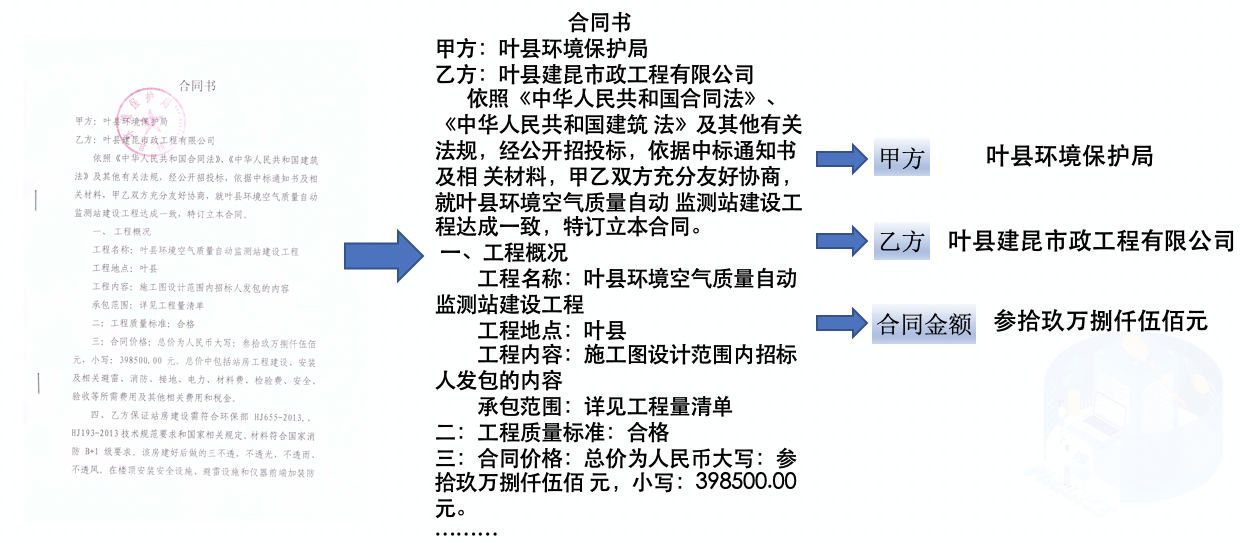 | ||
|
||
|
||
## 2. 解决方案 | ||
|
||
### 2.1 扫描合同文本内容提取 | ||
|
||
使用PaddleOCR开源的模型可以快速完成扫描文档的文本内容提取,在清晰文档上识别准确率可达到95%+。下面来快速体验一下: | ||
|
||
#### 2.1.1 环境准备 | ||
|
||
[PaddleOCR](https://github.com/PaddlePaddle/PaddleOCR)提供了适用于通用场景的高精轻量模型,提供数据预处理-模型推理-后处理全流程,支持pip安装: | ||
|
||
``` | ||
python -m pip install paddleocr | ||
``` | ||
|
||
#### 2.1.2 效果测试 | ||
|
||
使用一张合同图片作为测试样本,感受ppocrv3模型效果: | ||
|
||
<img src=https://ai-studio-static-online.cdn.bcebos.com/46258d0dc9dc40bab3ea0e70434e4a905646df8a647f4c49921e217de5142def width=300> | ||
|
||
使用中文检测+识别模型提取文本,实例化PaddleOCR类: | ||
|
||
``` | ||
from paddleocr import PaddleOCR, draw_ocr | ||
# paddleocr目前支持中英文、英文、法语、德语、韩语、日语等80个语种,可以通过修改lang参数进行切换 | ||
ocr = PaddleOCR(use_angle_cls=False, lang="ch") # need to run only once to download and load model into memory | ||
``` | ||
|
||
一行命令启动预测,预测结果包括`检测框`和`文本识别内容`: | ||
|
||
``` | ||
img_path = "./test_img/hetong2.jpg" | ||
result = ocr.ocr(img_path, cls=False) | ||
for line in result: | ||
print(line) | ||
# 可视化结果 | ||
from PIL import Image | ||
image = Image.open(img_path).convert('RGB') | ||
boxes = [line[0] for line in result] | ||
txts = [line[1][0] for line in result] | ||
scores = [line[1][1] for line in result] | ||
im_show = draw_ocr(image, boxes, txts, scores, font_path='./simfang.ttf') | ||
im_show = Image.fromarray(im_show) | ||
im_show.show() | ||
``` | ||
|
||
#### 2.1.3 图片预处理 | ||
|
||
通过上图可视化结果可以看到,印章部分造成的文本遮盖,影响了文本识别结果,因此可以考虑通道提取,去除图片中的红色印章: | ||
|
||
``` | ||
import cv2 | ||
import numpy as np | ||
import matplotlib.pyplot as plt | ||
#读入图像,三通道 | ||
image=cv2.imread("./test_img/hetong2.jpg",cv2.IMREAD_COLOR) #timg.jpeg | ||
#获得三个通道 | ||
Bch,Gch,Rch=cv2.split(image) | ||
#保存三通道图片 | ||
cv2.imwrite('blue_channel.jpg',Bch) | ||
cv2.imwrite('green_channel.jpg',Gch) | ||
cv2.imwrite('red_channel.jpg',Rch) | ||
``` | ||
#### 2.1.4 合同文本信息提取 | ||
|
||
经过2.1.3的预处理后,合同照片的红色通道被分离,获得了一张相对更干净的图片,此时可以再次使用ppocr模型提取文本内容: | ||
|
||
``` | ||
import numpy as np | ||
import cv2 | ||
img_path = './red_channel.jpg' | ||
result = ocr.ocr(img_path, cls=False) | ||
# 可视化结果 | ||
from PIL import Image | ||
image = Image.open(img_path).convert('RGB') | ||
boxes = [line[0] for line in result] | ||
txts = [line[1][0] for line in result] | ||
scores = [line[1][1] for line in result] | ||
im_show = draw_ocr(image, boxes, txts, scores, font_path='./simfang.ttf') | ||
im_show = Image.fromarray(im_show) | ||
vis = np.array(im_show) | ||
im_show.show() | ||
``` | ||
|
||
忽略检测框内容,提取完整的合同文本: | ||
|
||
``` | ||
txts = [line[1][0] for line in result] | ||
all_context = "\n".join(txts) | ||
print(all_context) | ||
``` | ||
|
||
通过以上环节就完成了扫描合同关键信息抽取的第一步:文本内容提取,接下来可以基于识别出的文本内容抽取关键信息 | ||
|
||
### 2.2 合同关键信息抽取 | ||
|
||
#### 2.2.1 环境准备 | ||
|
||
安装PaddleNLP | ||
|
||
|
||
``` | ||
pip install --upgrade pip | ||
pip install --upgrade paddlenlp | ||
``` | ||
|
||
#### 2.2.2 合同关键信息抽取 | ||
|
||
PaddleNLP 使用 Taskflow 统一管理多场景任务的预测功能,其中`information_extraction` 通过大量的有标签样本进行训练,在通用的场景中一般可以直接使用,只需更换关键字即可。例如在合同信息抽取中,我们重新定义抽取关键字: | ||
|
||
甲方、乙方、币种、金额、付款方式 | ||
|
||
|
||
将使用OCR提取好的文本作为输入,使用三行命令可以对上文中提取到的合同文本进行关键信息抽取: | ||
|
||
``` | ||
from paddlenlp import Taskflow | ||
schema = ["甲方","乙方","总价"] | ||
ie = Taskflow('information_extraction', schema=schema) | ||
ie.set_schema(schema) | ||
ie(all_context) | ||
``` | ||
|
||
可以看到UIE模型可以准确的提取出关键信息,用于后续的信息比对或审核。 | ||
|
||
## 3.效果优化 | ||
|
||
### 3.1 文本识别后处理调优 | ||
|
||
实际图片采集过程中,可能出现部分图片弯曲等问题,导致使用默认参数识别文本时存在漏检,影响关键信息获取。 | ||
|
||
例如下图: | ||
|
||
<img src="https://ai-studio-static-online.cdn.bcebos.com/fe350481be0241c58736d487d1bf06c2e65911bf01254a79944be629c4c10091" height="300" width="300"> | ||
|
||
|
||
直接进行预测: | ||
|
||
``` | ||
img_path = "./test_img/hetong3.jpg" | ||
# 预测结果 | ||
result = ocr.ocr(img_path, cls=False) | ||
# 可视化结果 | ||
from PIL import Image | ||
image = Image.open(img_path).convert('RGB') | ||
boxes = [line[0] for line in result] | ||
txts = [line[1][0] for line in result] | ||
scores = [line[1][1] for line in result] | ||
im_show = draw_ocr(image, boxes, txts, scores, font_path='./simfang.ttf') | ||
im_show = Image.fromarray(im_show) | ||
im_show.show() | ||
``` | ||
|
||
可视化结果可以看到,弯曲图片存在漏检,一般来说可以通过调整后处理参数解决,无需重新训练模型。漏检问题往往是因为检测模型获得的分割图太小,生成框的得分过低被过滤掉了,通常有两种方式调整参数: | ||
- 开启`use_dilatiion=True` 膨胀分割区域 | ||
- 调小`det_db_box_thresh`阈值 | ||
|
||
``` | ||
# 重新实例化 PaddleOCR | ||
ocr = PaddleOCR(use_angle_cls=False, lang="ch", det_db_box_thresh=0.3, use_dilation=True) | ||
# 预测并可视化 | ||
img_path = "./test_img/hetong3.jpg" | ||
# 预测结果 | ||
result = ocr.ocr(img_path, cls=False) | ||
# 可视化结果 | ||
image = Image.open(img_path).convert('RGB') | ||
boxes = [line[0] for line in result] | ||
txts = [line[1][0] for line in result] | ||
scores = [line[1][1] for line in result] | ||
im_show = draw_ocr(image, boxes, txts, scores, font_path='./simfang.ttf') | ||
im_show = Image.fromarray(im_show) | ||
im_show.show() | ||
``` | ||
|
||
可以看到漏检问题被很好的解决,提取完整的文本内容: | ||
|
||
``` | ||
txts = [line[1][0] for line in result] | ||
context = "\n".join(txts) | ||
print(context) | ||
``` | ||
|
||
### 3.2 关键信息提取调优 | ||
|
||
UIE通过大量有标签样本进行训练,得到了一个开箱即用的高精模型。 然而针对不同场景,可能会出现部分实体无法被抽取的情况。通常来说有以下几个方法进行效果调优: | ||
|
||
|
||
- 修改 schema | ||
- 添加正则方法 | ||
- 标注小样本微调模型 | ||
|
||
**修改schema** | ||
|
||
Prompt和原文描述越像,抽取效果越好,例如 | ||
``` | ||
三:合同价格:总价为人民币大写:参拾玖万捌仟伍佰 | ||
元,小写:398500.00元。总价中包括站房工程建设、安装 | ||
及相关避雷、消防、接地、电力、材料费、检验费、安全、 | ||
验收等所需费用及其他相关费用和税金。 | ||
``` | ||
schema = ["总金额"] 时无法准确抽取,与原文描述差异较大。 修改 schema = ["总价"] 再次尝试: | ||
|
||
``` | ||
from paddlenlp import Taskflow | ||
# schema = ["总金额"] | ||
schema = ["总价"] | ||
ie = Taskflow('information_extraction', schema=schema) | ||
ie.set_schema(schema) | ||
ie(all_context) | ||
``` | ||
|
||
|
||
**模型微调** | ||
|
||
UIE的建模方式主要是通过 `Prompt` 方式来建模, `Prompt` 在小样本上进行微调效果非常有效。详细的数据标注+模型微调步骤可以参考项目: | ||
|
||
[PaddleNLP信息抽取技术重磅升级!](https://aistudio.baidu.com/aistudio/projectdetail/3914778?channelType=0&channel=0) | ||
|
||
[工单信息抽取](https://aistudio.baidu.com/aistudio/projectdetail/3914778?contributionType=1) | ||
|
||
[快递单信息抽取](https://aistudio.baidu.com/aistudio/projectdetail/4038499?contributionType=1) | ||
|
||
|
||
## 总结 | ||
|
||
扫描合同的关键信息提取可以使用 PaddleOCR + PaddleNLP 组合实现,两个工具均有以下优势: | ||
|
||
* 使用简单:whl包一键安装,3行命令调用 | ||
* 效果领先:优秀的模型效果可覆盖几乎全部的应用场景 | ||
* 调优成本低:OCR模型可通过后处理参数的调整适配略有偏差的扫描文本, UIE模型可以通过极少的标注样本微调,成本很低。 | ||
|
||
## 作业 | ||
|
||
尝试自己解析出 `test_img/homework.png` 扫描合同中的 [甲方、乙方] 关键词: | ||
|
||
|
||
|
||
<img src=https://ai-studio-static-online.cdn.bcebos.com/50a49a3c9f8348bfa04e8c8b97d3cce0d0dd6b14040f43939268d120688ef7ca width=300 hight=400> | ||
|
||
|
||
|
||
更多场景下的垂类模型获取,请扫下图二维码填写问卷,加入PaddleOCR官方交流群获取模型下载链接、《动手学OCR》电子书等全套OCR学习资料🎁 | ||
|
||
<img src=https://ai-studio-static-online.cdn.bcebos.com/606538b59ea845cb99943b1dec6efe724e78f75c1e9c49228c7bf7da9f8837f5 width=300 hight=300> |