forked from akshitagupta15june/Face-X
-
Notifications
You must be signed in to change notification settings - Fork 0
Commit
This commit does not belong to any branch on this repository, and may belong to a fork outside of the repository.
Merge pull request akshitagupta15june#848 from NEERAJAP2001/random_fo…
…rest Added Face Recognition using Random forest
- Loading branch information
Showing
2 changed files
with
549 additions
and
0 deletions.
There are no files selected for viewing
533 changes: 533 additions & 0 deletions
533
.../Face Detection using Random forest/10.03-Using-Random-Forests-for-Face-Recognition.ipynb
Large diffs are not rendered by default.
Oops, something went wrong.
16 changes: 16 additions & 0 deletions
16
Face-Detection/Face Detection using Random forest/README.md
This file contains bidirectional Unicode text that may be interpreted or compiled differently than what appears below. To review, open the file in an editor that reveals hidden Unicode characters.
Learn more about bidirectional Unicode characters
Original file line number | Diff line number | Diff line change |
---|---|---|
@@ -0,0 +1,16 @@ | ||
## Random Forest : | ||
|
||
Random forests are an ensemble learning method for classification (and regression) that operate by constructing a multitude of decision trees at training time and outputting the class that is the mode of the classes output by individual trees. | ||
|
||
|
||
|
||
## Using Random Forests for Face Recognition | ||
A popular dataset that we haven't talked much about yet is the Olivetti face dataset. | ||
|
||
The Olivetti face dataset was collected in 1990 by AT&T Laboratories Cambridge. The dataset comprises facial images of 40 distinct subjects, taken at different times and under different lighting conditions. In addition, subjects varied their facial expression (open/closed eyes, smiling/not smiling) and their facial details (glasses/no glasses). | ||
|
||
Images were then quantized to 256 grayscale levels and stored as unsigned 8-bit integers. Because there are 40 distinct subjects, the dataset comes with 40 distinct target labels. Recognizing faces thus constitutes an example of a multiclass classification task. | ||
|
||
|
||
## Output : | ||
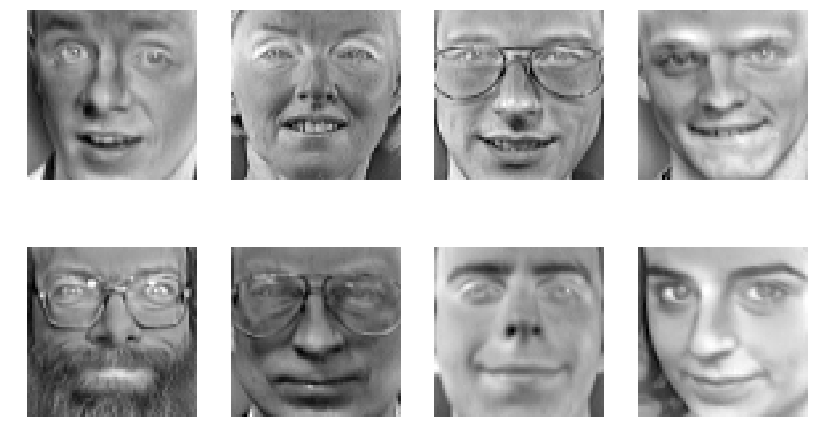 |